- Skip to main content
- Skip to primary sidebar
- Skip to footer
- QuestionPro

- Solutions Industries Gaming Automotive Sports and events Education Government Travel & Hospitality Financial Services Healthcare Cannabis Technology Use Case NPS+ Communities Audience Contactless surveys Mobile LivePolls Member Experience GDPR Positive People Science 360 Feedback Surveys
- Resources Blog eBooks Survey Templates Case Studies Training Help center

Home Market Research
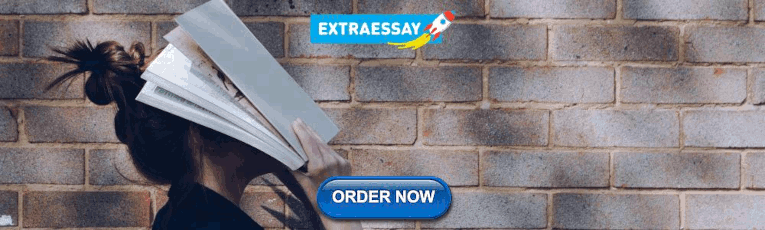
Empirical Research: Definition, Methods, Types and Examples
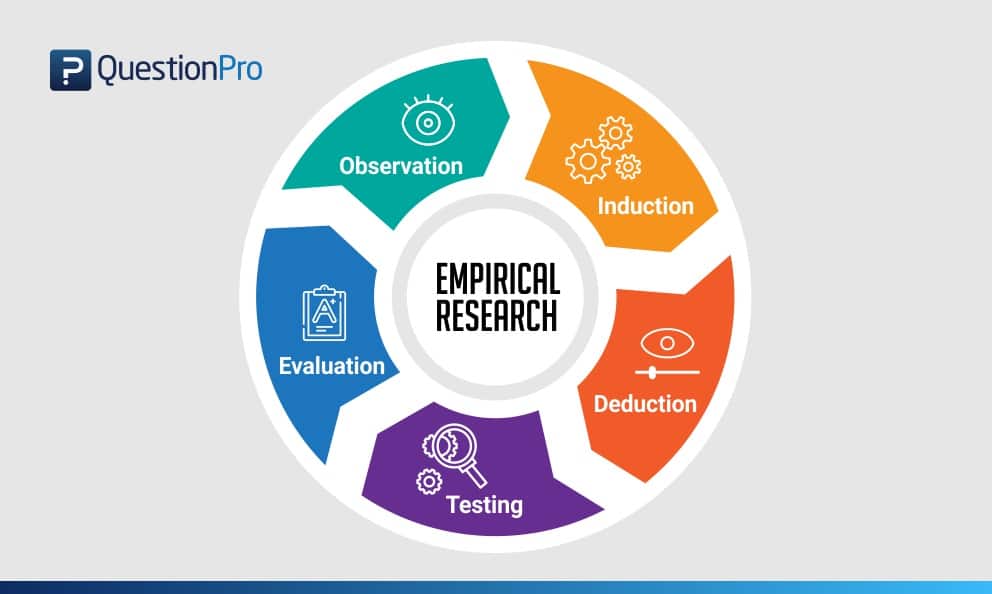
Content Index
Empirical research: Definition
Empirical research: origin, quantitative research methods, qualitative research methods, steps for conducting empirical research, empirical research methodology cycle, advantages of empirical research, disadvantages of empirical research, why is there a need for empirical research.
Empirical research is defined as any research where conclusions of the study is strictly drawn from concretely empirical evidence, and therefore “verifiable” evidence.
This empirical evidence can be gathered using quantitative market research and qualitative market research methods.
For example: A research is being conducted to find out if listening to happy music in the workplace while working may promote creativity? An experiment is conducted by using a music website survey on a set of audience who are exposed to happy music and another set who are not listening to music at all, and the subjects are then observed. The results derived from such a research will give empirical evidence if it does promote creativity or not.
LEARN ABOUT: Behavioral Research
You must have heard the quote” I will not believe it unless I see it”. This came from the ancient empiricists, a fundamental understanding that powered the emergence of medieval science during the renaissance period and laid the foundation of modern science, as we know it today. The word itself has its roots in greek. It is derived from the greek word empeirikos which means “experienced”.
In today’s world, the word empirical refers to collection of data using evidence that is collected through observation or experience or by using calibrated scientific instruments. All of the above origins have one thing in common which is dependence of observation and experiments to collect data and test them to come up with conclusions.
LEARN ABOUT: Causal Research
Types and methodologies of empirical research
Empirical research can be conducted and analysed using qualitative or quantitative methods.
- Quantitative research : Quantitative research methods are used to gather information through numerical data. It is used to quantify opinions, behaviors or other defined variables . These are predetermined and are in a more structured format. Some of the commonly used methods are survey, longitudinal studies, polls, etc
- Qualitative research: Qualitative research methods are used to gather non numerical data. It is used to find meanings, opinions, or the underlying reasons from its subjects. These methods are unstructured or semi structured. The sample size for such a research is usually small and it is a conversational type of method to provide more insight or in-depth information about the problem Some of the most popular forms of methods are focus groups, experiments, interviews, etc.
Data collected from these will need to be analysed. Empirical evidence can also be analysed either quantitatively and qualitatively. Using this, the researcher can answer empirical questions which have to be clearly defined and answerable with the findings he has got. The type of research design used will vary depending on the field in which it is going to be used. Many of them might choose to do a collective research involving quantitative and qualitative method to better answer questions which cannot be studied in a laboratory setting.
LEARN ABOUT: Qualitative Research Questions and Questionnaires
Quantitative research methods aid in analyzing the empirical evidence gathered. By using these a researcher can find out if his hypothesis is supported or not.
- Survey research: Survey research generally involves a large audience to collect a large amount of data. This is a quantitative method having a predetermined set of closed questions which are pretty easy to answer. Because of the simplicity of such a method, high responses are achieved. It is one of the most commonly used methods for all kinds of research in today’s world.
Previously, surveys were taken face to face only with maybe a recorder. However, with advancement in technology and for ease, new mediums such as emails , or social media have emerged.
For example: Depletion of energy resources is a growing concern and hence there is a need for awareness about renewable energy. According to recent studies, fossil fuels still account for around 80% of energy consumption in the United States. Even though there is a rise in the use of green energy every year, there are certain parameters because of which the general population is still not opting for green energy. In order to understand why, a survey can be conducted to gather opinions of the general population about green energy and the factors that influence their choice of switching to renewable energy. Such a survey can help institutions or governing bodies to promote appropriate awareness and incentive schemes to push the use of greener energy.
Learn more: Renewable Energy Survey Template Descriptive Research vs Correlational Research
- Experimental research: In experimental research , an experiment is set up and a hypothesis is tested by creating a situation in which one of the variable is manipulated. This is also used to check cause and effect. It is tested to see what happens to the independent variable if the other one is removed or altered. The process for such a method is usually proposing a hypothesis, experimenting on it, analyzing the findings and reporting the findings to understand if it supports the theory or not.
For example: A particular product company is trying to find what is the reason for them to not be able to capture the market. So the organisation makes changes in each one of the processes like manufacturing, marketing, sales and operations. Through the experiment they understand that sales training directly impacts the market coverage for their product. If the person is trained well, then the product will have better coverage.
- Correlational research: Correlational research is used to find relation between two set of variables . Regression analysis is generally used to predict outcomes of such a method. It can be positive, negative or neutral correlation.
LEARN ABOUT: Level of Analysis
For example: Higher educated individuals will get higher paying jobs. This means higher education enables the individual to high paying job and less education will lead to lower paying jobs.
- Longitudinal study: Longitudinal study is used to understand the traits or behavior of a subject under observation after repeatedly testing the subject over a period of time. Data collected from such a method can be qualitative or quantitative in nature.
For example: A research to find out benefits of exercise. The target is asked to exercise everyday for a particular period of time and the results show higher endurance, stamina, and muscle growth. This supports the fact that exercise benefits an individual body.
- Cross sectional: Cross sectional study is an observational type of method, in which a set of audience is observed at a given point in time. In this type, the set of people are chosen in a fashion which depicts similarity in all the variables except the one which is being researched. This type does not enable the researcher to establish a cause and effect relationship as it is not observed for a continuous time period. It is majorly used by healthcare sector or the retail industry.
For example: A medical study to find the prevalence of under-nutrition disorders in kids of a given population. This will involve looking at a wide range of parameters like age, ethnicity, location, incomes and social backgrounds. If a significant number of kids coming from poor families show under-nutrition disorders, the researcher can further investigate into it. Usually a cross sectional study is followed by a longitudinal study to find out the exact reason.
- Causal-Comparative research : This method is based on comparison. It is mainly used to find out cause-effect relationship between two variables or even multiple variables.
For example: A researcher measured the productivity of employees in a company which gave breaks to the employees during work and compared that to the employees of the company which did not give breaks at all.
LEARN ABOUT: Action Research
Some research questions need to be analysed qualitatively, as quantitative methods are not applicable there. In many cases, in-depth information is needed or a researcher may need to observe a target audience behavior, hence the results needed are in a descriptive analysis form. Qualitative research results will be descriptive rather than predictive. It enables the researcher to build or support theories for future potential quantitative research. In such a situation qualitative research methods are used to derive a conclusion to support the theory or hypothesis being studied.
LEARN ABOUT: Qualitative Interview
- Case study: Case study method is used to find more information through carefully analyzing existing cases. It is very often used for business research or to gather empirical evidence for investigation purpose. It is a method to investigate a problem within its real life context through existing cases. The researcher has to carefully analyse making sure the parameter and variables in the existing case are the same as to the case that is being investigated. Using the findings from the case study, conclusions can be drawn regarding the topic that is being studied.
For example: A report mentioning the solution provided by a company to its client. The challenges they faced during initiation and deployment, the findings of the case and solutions they offered for the problems. Such case studies are used by most companies as it forms an empirical evidence for the company to promote in order to get more business.
- Observational method: Observational method is a process to observe and gather data from its target. Since it is a qualitative method it is time consuming and very personal. It can be said that observational research method is a part of ethnographic research which is also used to gather empirical evidence. This is usually a qualitative form of research, however in some cases it can be quantitative as well depending on what is being studied.
For example: setting up a research to observe a particular animal in the rain-forests of amazon. Such a research usually take a lot of time as observation has to be done for a set amount of time to study patterns or behavior of the subject. Another example used widely nowadays is to observe people shopping in a mall to figure out buying behavior of consumers.
- One-on-one interview: Such a method is purely qualitative and one of the most widely used. The reason being it enables a researcher get precise meaningful data if the right questions are asked. It is a conversational method where in-depth data can be gathered depending on where the conversation leads.
For example: A one-on-one interview with the finance minister to gather data on financial policies of the country and its implications on the public.
- Focus groups: Focus groups are used when a researcher wants to find answers to why, what and how questions. A small group is generally chosen for such a method and it is not necessary to interact with the group in person. A moderator is generally needed in case the group is being addressed in person. This is widely used by product companies to collect data about their brands and the product.
For example: A mobile phone manufacturer wanting to have a feedback on the dimensions of one of their models which is yet to be launched. Such studies help the company meet the demand of the customer and position their model appropriately in the market.
- Text analysis: Text analysis method is a little new compared to the other types. Such a method is used to analyse social life by going through images or words used by the individual. In today’s world, with social media playing a major part of everyone’s life, such a method enables the research to follow the pattern that relates to his study.
For example: A lot of companies ask for feedback from the customer in detail mentioning how satisfied are they with their customer support team. Such data enables the researcher to take appropriate decisions to make their support team better.
Sometimes a combination of the methods is also needed for some questions that cannot be answered using only one type of method especially when a researcher needs to gain a complete understanding of complex subject matter.
We recently published a blog that talks about examples of qualitative data in education ; why don’t you check it out for more ideas?
Since empirical research is based on observation and capturing experiences, it is important to plan the steps to conduct the experiment and how to analyse it. This will enable the researcher to resolve problems or obstacles which can occur during the experiment.
Step #1: Define the purpose of the research
This is the step where the researcher has to answer questions like what exactly do I want to find out? What is the problem statement? Are there any issues in terms of the availability of knowledge, data, time or resources. Will this research be more beneficial than what it will cost.
Before going ahead, a researcher has to clearly define his purpose for the research and set up a plan to carry out further tasks.
Step #2 : Supporting theories and relevant literature
The researcher needs to find out if there are theories which can be linked to his research problem . He has to figure out if any theory can help him support his findings. All kind of relevant literature will help the researcher to find if there are others who have researched this before, or what are the problems faced during this research. The researcher will also have to set up assumptions and also find out if there is any history regarding his research problem
Step #3: Creation of Hypothesis and measurement
Before beginning the actual research he needs to provide himself a working hypothesis or guess what will be the probable result. Researcher has to set up variables, decide the environment for the research and find out how can he relate between the variables.
Researcher will also need to define the units of measurements, tolerable degree for errors, and find out if the measurement chosen will be acceptable by others.
Step #4: Methodology, research design and data collection
In this step, the researcher has to define a strategy for conducting his research. He has to set up experiments to collect data which will enable him to propose the hypothesis. The researcher will decide whether he will need experimental or non experimental method for conducting the research. The type of research design will vary depending on the field in which the research is being conducted. Last but not the least, the researcher will have to find out parameters that will affect the validity of the research design. Data collection will need to be done by choosing appropriate samples depending on the research question. To carry out the research, he can use one of the many sampling techniques. Once data collection is complete, researcher will have empirical data which needs to be analysed.
LEARN ABOUT: Best Data Collection Tools
Step #5: Data Analysis and result
Data analysis can be done in two ways, qualitatively and quantitatively. Researcher will need to find out what qualitative method or quantitative method will be needed or will he need a combination of both. Depending on the unit of analysis of his data, he will know if his hypothesis is supported or rejected. Analyzing this data is the most important part to support his hypothesis.
Step #6: Conclusion
A report will need to be made with the findings of the research. The researcher can give the theories and literature that support his research. He can make suggestions or recommendations for further research on his topic.
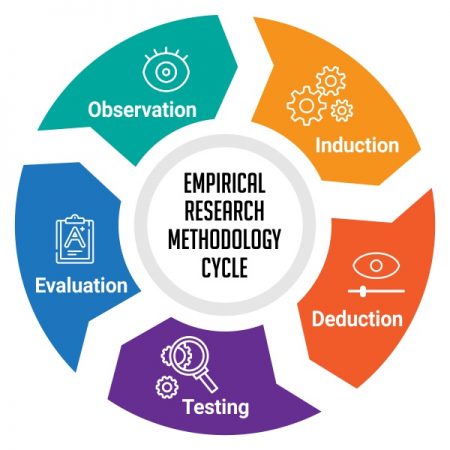
A.D. de Groot, a famous dutch psychologist and a chess expert conducted some of the most notable experiments using chess in the 1940’s. During his study, he came up with a cycle which is consistent and now widely used to conduct empirical research. It consists of 5 phases with each phase being as important as the next one. The empirical cycle captures the process of coming up with hypothesis about how certain subjects work or behave and then testing these hypothesis against empirical data in a systematic and rigorous approach. It can be said that it characterizes the deductive approach to science. Following is the empirical cycle.
- Observation: At this phase an idea is sparked for proposing a hypothesis. During this phase empirical data is gathered using observation. For example: a particular species of flower bloom in a different color only during a specific season.
- Induction: Inductive reasoning is then carried out to form a general conclusion from the data gathered through observation. For example: As stated above it is observed that the species of flower blooms in a different color during a specific season. A researcher may ask a question “does the temperature in the season cause the color change in the flower?” He can assume that is the case, however it is a mere conjecture and hence an experiment needs to be set up to support this hypothesis. So he tags a few set of flowers kept at a different temperature and observes if they still change the color?
- Deduction: This phase helps the researcher to deduce a conclusion out of his experiment. This has to be based on logic and rationality to come up with specific unbiased results.For example: In the experiment, if the tagged flowers in a different temperature environment do not change the color then it can be concluded that temperature plays a role in changing the color of the bloom.
- Testing: This phase involves the researcher to return to empirical methods to put his hypothesis to the test. The researcher now needs to make sense of his data and hence needs to use statistical analysis plans to determine the temperature and bloom color relationship. If the researcher finds out that most flowers bloom a different color when exposed to the certain temperature and the others do not when the temperature is different, he has found support to his hypothesis. Please note this not proof but just a support to his hypothesis.
- Evaluation: This phase is generally forgotten by most but is an important one to keep gaining knowledge. During this phase the researcher puts forth the data he has collected, the support argument and his conclusion. The researcher also states the limitations for the experiment and his hypothesis and suggests tips for others to pick it up and continue a more in-depth research for others in the future. LEARN MORE: Population vs Sample
LEARN MORE: Population vs Sample
There is a reason why empirical research is one of the most widely used method. There are a few advantages associated with it. Following are a few of them.
- It is used to authenticate traditional research through various experiments and observations.
- This research methodology makes the research being conducted more competent and authentic.
- It enables a researcher understand the dynamic changes that can happen and change his strategy accordingly.
- The level of control in such a research is high so the researcher can control multiple variables.
- It plays a vital role in increasing internal validity .
Even though empirical research makes the research more competent and authentic, it does have a few disadvantages. Following are a few of them.
- Such a research needs patience as it can be very time consuming. The researcher has to collect data from multiple sources and the parameters involved are quite a few, which will lead to a time consuming research.
- Most of the time, a researcher will need to conduct research at different locations or in different environments, this can lead to an expensive affair.
- There are a few rules in which experiments can be performed and hence permissions are needed. Many a times, it is very difficult to get certain permissions to carry out different methods of this research.
- Collection of data can be a problem sometimes, as it has to be collected from a variety of sources through different methods.
LEARN ABOUT: Social Communication Questionnaire
Empirical research is important in today’s world because most people believe in something only that they can see, hear or experience. It is used to validate multiple hypothesis and increase human knowledge and continue doing it to keep advancing in various fields.
For example: Pharmaceutical companies use empirical research to try out a specific drug on controlled groups or random groups to study the effect and cause. This way, they prove certain theories they had proposed for the specific drug. Such research is very important as sometimes it can lead to finding a cure for a disease that has existed for many years. It is useful in science and many other fields like history, social sciences, business, etc.
LEARN ABOUT: 12 Best Tools for Researchers
With the advancement in today’s world, empirical research has become critical and a norm in many fields to support their hypothesis and gain more knowledge. The methods mentioned above are very useful for carrying out such research. However, a number of new methods will keep coming up as the nature of new investigative questions keeps getting unique or changing.
Create a single source of real data with a built-for-insights platform. Store past data, add nuggets of insights, and import research data from various sources into a CRM for insights. Build on ever-growing research with a real-time dashboard in a unified research management platform to turn insights into knowledge.
LEARN MORE FREE TRIAL
MORE LIKE THIS
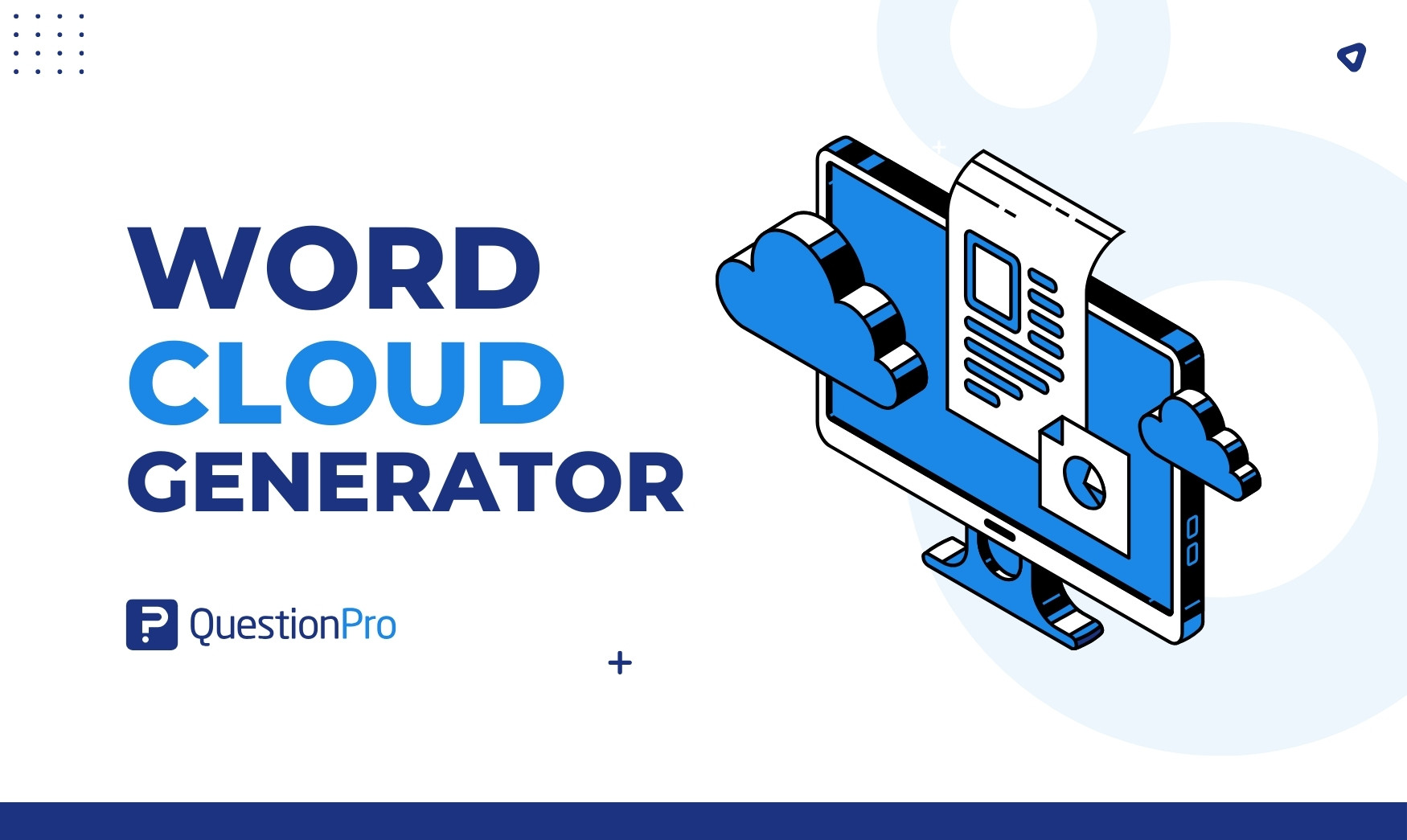
9 Best Word Cloud Generator Uses, Pros & Cons
Mar 15, 2024
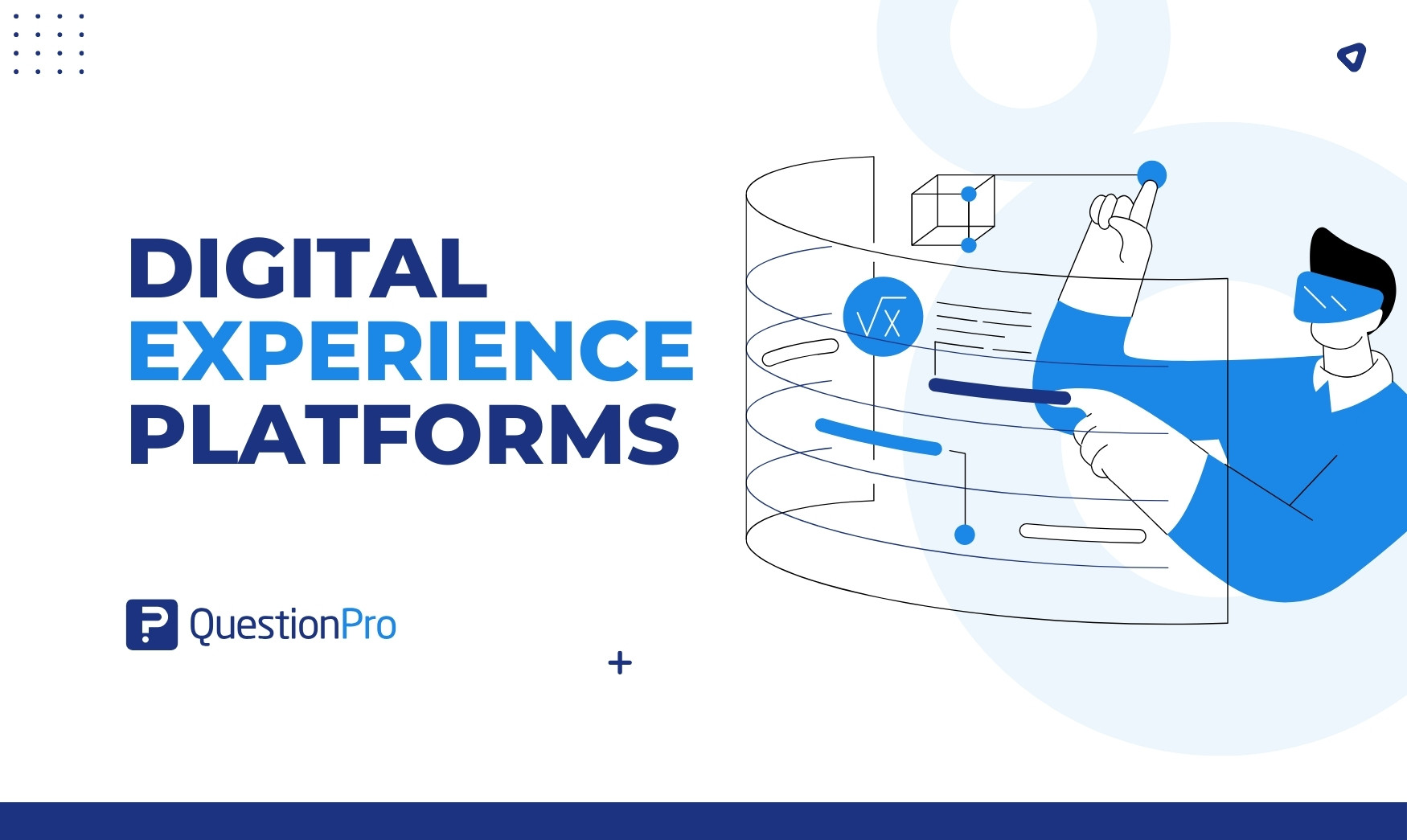
Top 8 Best Digital Experience Platforms in 2024
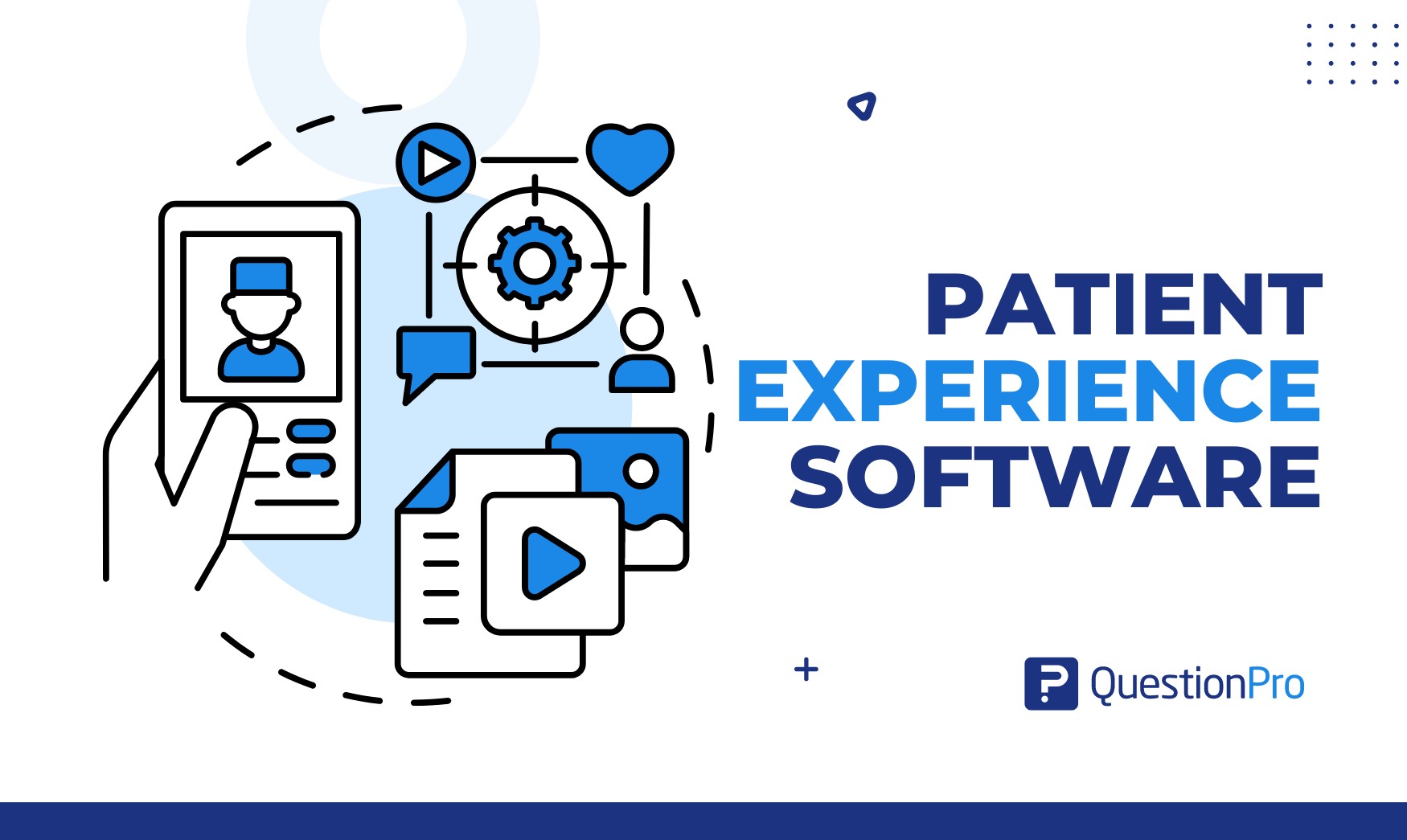
Top 10 Patient Experience Software to Shape Modern Healthcare
Mar 14, 2024
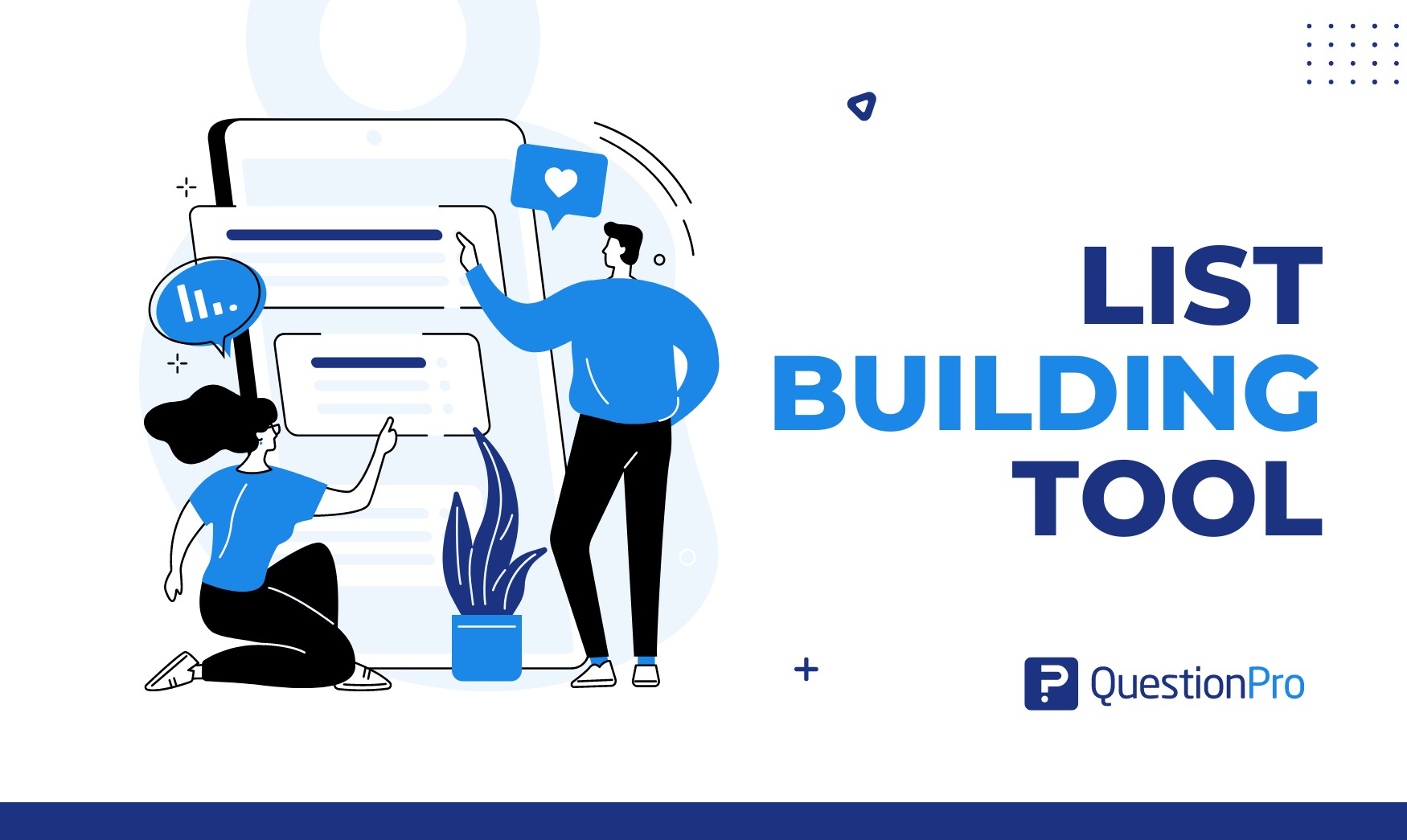
Email List Building Tool: Choose The Best From These 9 Tools
Other categories.
- Academic Research
- Artificial Intelligence
- Assessments
- Brand Awareness
- Case Studies
- Communities
- Consumer Insights
- Customer effort score
- Customer Engagement
- Customer Experience
- Customer Loyalty
- Customer Research
- Customer Satisfaction
- Employee Benefits
- Employee Engagement
- Employee Retention
- Friday Five
- General Data Protection Regulation
- Insights Hub
- Life@QuestionPro
- Market Research
- Mobile diaries
- Mobile Surveys
- New Features
- Online Communities
- Question Types
- Questionnaire
- QuestionPro Products
- Release Notes
- Research Tools and Apps
- Revenue at Risk
- Survey Templates
- Training Tips
- Uncategorized
- Video Learning Series
- What’s Coming Up
- Workforce Intelligence

- University of Memphis Libraries
- Research Guides
Empirical Research: Defining, Identifying, & Finding
Defining empirical research, what is empirical research, quantitative or qualitative.
- Introduction
- Database Tools
- Search Terms
- Image Descriptions
Calfee & Chambliss (2005) (UofM login required) describe empirical research as a "systematic approach for answering certain types of questions." Those questions are answered "[t]hrough the collection of evidence under carefully defined and replicable conditions" (p. 43).
The evidence collected during empirical research is often referred to as "data."
Characteristics of Empirical Research
Emerald Publishing's guide to conducting empirical research identifies a number of common elements to empirical research:
- A research question , which will determine research objectives.
- A particular and planned design for the research, which will depend on the question and which will find ways of answering it with appropriate use of resources.
- The gathering of primary data , which is then analysed.
- A particular methodology for collecting and analysing the data, such as an experiment or survey.
- The limitation of the data to a particular group, area or time scale, known as a sample [emphasis added]: for example, a specific number of employees of a particular company type, or all users of a library over a given time scale. The sample should be somehow representative of a wider population.
- The ability to recreate the study and test the results. This is known as reliability .
- The ability to generalize from the findings to a larger sample and to other situations.
If you see these elements in a research article, you can feel confident that you have found empirical research. Emerald's guide goes into more detail on each element.
Empirical research methodologies can be described as quantitative, qualitative, or a mix of both (usually called mixed-methods).
Ruane (2016) (UofM login required) gets at the basic differences in approach between quantitative and qualitative research:
- Quantitative research -- an approach to documenting reality that relies heavily on numbers both for the measurement of variables and for data analysis (p. 33).
- Qualitative research -- an approach to documenting reality that relies on words and images as the primary data source (p. 33).
Both quantitative and qualitative methods are empirical . If you can recognize that a research study is quantitative or qualitative study, then you have also recognized that it is empirical study.
Below are information on the characteristics of quantitative and qualitative research. This video from Scribbr also offers a good overall introduction to the two approaches to research methodology:
Characteristics of Quantitative Research
Researchers test hypotheses, or theories, based in assumptions about causality, i.e. we expect variable X to cause variable Y. Variables have to be controlled as much as possible to ensure validity. The results explain the relationship between the variables. Measures are based in pre-defined instruments.
Examples: experimental or quasi-experimental design, pretest & post-test, survey or questionnaire with closed-ended questions. Studies that identify factors that influence an outcomes, the utility of an intervention, or understanding predictors of outcomes.
Characteristics of Qualitative Research
Researchers explore “meaning individuals or groups ascribe to social or human problems (Creswell & Creswell, 2018, p3).” Questions and procedures emerge rather than being prescribed. Complexity, nuance, and individual meaning are valued. Research is both inductive and deductive. Data sources are multiple and varied, i.e. interviews, observations, documents, photographs, etc. The researcher is a key instrument and must be reflective of their background, culture, and experiences as influential of the research.
Examples: open question interviews and surveys, focus groups, case studies, grounded theory, ethnography, discourse analysis, narrative, phenomenology, participatory action research.
Calfee, R. C. & Chambliss, M. (2005). The design of empirical research. In J. Flood, D. Lapp, J. R. Squire, & J. Jensen (Eds.), Methods of research on teaching the English language arts: The methodology chapters from the handbook of research on teaching the English language arts (pp. 43-78). Routledge. http://ezproxy.memphis.edu/login?url=http://search.ebscohost.com/login.aspx?direct=true&db=nlebk&AN=125955&site=eds-live&scope=site .
Creswell, J. W., & Creswell, J. D. (2018). Research design: Qualitative, quantitative, and mixed methods approaches (5th ed.). Thousand Oaks: Sage.
How to... conduct empirical research . (n.d.). Emerald Publishing. https://www.emeraldgrouppublishing.com/how-to/research-methods/conduct-empirical-research .
Scribbr. (2019). Quantitative vs. qualitative: The differences explained [video]. YouTube. https://www.youtube.com/watch?v=a-XtVF7Bofg .
Ruane, J. M. (2016). Introducing social research methods : Essentials for getting the edge . Wiley-Blackwell. http://ezproxy.memphis.edu/login?url=http://search.ebscohost.com/login.aspx?direct=true&db=nlebk&AN=1107215&site=eds-live&scope=site .
- << Previous: Home
- Next: Identifying Empirical Research >>
- Last Updated: Jan 8, 2024 11:48 AM
- URL: https://libguides.memphis.edu/empirical-research
Canvas | University | Ask a Librarian
- Library Homepage
- Arrendale Library
Empirical Research: Quantitative & Qualitative
- Introduction: Empirical Research
What is Empirical Research?
Quantitative methods, qualitative methods.
- Quantitative vs. Qualitative
- Reference Works for Social Sciences Research
- Contact Us!
Call us at 706-776-0111
Chat with a Librarian
Send Us Email
Library Hours
Empirical research is based on observed and measured phenomena and derives knowledge from actual experience rather than from theory or belief.
Key characteristics of empirical research to look for:
- Specific research questions to be answered;
- Definitions of the population, behavior, or phenomena being studied;
- Description of the methodology or research design used to study this population or phenomena, including selection criteria, controls, and testing instruments (such as surveys);
- There are two basic research processes or methods in empirical research: quantitative methods and qualitative methods (see below for more about these methods).
How do you know if a study is empirical? Read the subheadings within the article, book, or report and look for a description of the research methodology .
Some scholarly journals use a specific article layout called the IMRaD format to communicate empirical research findings. Such articles typically have 4 components:
- Introduction : sometimes called literature review - what is currently known about the topic - usually includes a theoretical framework and/or discussion of previous studies
- Methodology : sometimes called research design - how to recreate the study - usually describes the population, research process, and analytical tools
- Results : sometimes called findings - what was learned through the study - usually appears as statistical data or as substantial quotations from research participants
- Discussion : sometimes called conclusion or implications - why the study is important - usually describes how the research results influence professional practices or future studies.
(based on the original from the Connelly LIbrary of LaSalle University)
Quantitative Research
A quantitative research project is characterized by having a population about which the researcher wants to draw conclusions, but it is not possible to collect data on the entire population.
- For an observational study, it is necessary to select a proper, statistical random sample and to use methods of statistical inference to draw conclusions about the population.
- For an experimental study, it is necessary to have a random assignment of subjects to experimental and control groups in order to use methods of statistical inference.
Statistical methods are used in all three stages of a quantitative research project.
For observational studies, the data are collected using statistical sampling theory. Then, the sample data are analyzed using descriptive statistical analysis. Finally, generalizations are made from the sample data to the entire population using statistical inference.
For experimental studies, the subjects are allocated to experimental and control group using randomizing methods. Then, the experimental data are analyzed using descriptive statistical analysis. Finally, just as for observational data, generalizations are made to a larger population.
Iversen, G. (2004). Quantitative research . In M. Lewis-Beck, A. Bryman, & T. Liao (Eds.), Encyclopedia of social science research methods . (pp. 897-898). Thousand Oaks, CA: SAGE Publications, Inc.
Qualitative Research
What makes a work deserving of the label qualitative research is the demonstrable effort to produce richly and relevantly detailed descriptions and particularized interpretations of people and the social, linguistic, material, and other practices and events that shape and are shaped by them.
Qualitative research typically includes, but is not limited to, discerning the perspectives of these people, or what is often referred to as the actor’s point of view. Although both philosophically and methodologically a highly diverse entity, qualitative research is marked by certain defining imperatives that include its case (as opposed to its variable) orientation, sensitivity to cultural and historical context, and reflexivity.
In its many guises, qualitative research is a form of empirical inquiry that typically entails some form of purposive sampling for information-rich cases; in-depth interviews and open-ended interviews, lengthy participant/field observations, and/or document or artifact study; and techniques for analysis and interpretation of data that move beyond the data generated and their surface appearances.
Sandelowski, M. (2004). Qualitative research . In M. Lewis-Beck, A. Bryman, & T. Liao (Eds.), Encyclopedia of social science research methods . (pp. 893-894). Thousand Oaks, CA: SAGE Publications, Inc.
- Next: Quantitative vs. Qualitative >>
- Last Updated: Mar 18, 2024 12:23 PM
- URL: https://library.piedmont.edu/empirical-research
- Ebooks & Online Video
- New Materials
- Renew Checkouts
- Faculty Resources
- Friends of the Library
- Library Services
- Request Books from Demorest
- Our Mission
- Library History
- Ask a Librarian!
- Making Citations
- Working Online

Arrendale Library Piedmont University 706-776-0111

- Ask a Librarian
Research: Overview & Approaches
- Getting Started with Undergraduate Research
- Planning & Getting Started
- Building Your Knowledge Base
- Locating Sources
- Reading Scholarly Articles
- Creating a Literature Review
- Productivity & Organizing Research
- Scholarly and Professional Relationships
Introduction to Empirical Research
Databases for finding empirical research, guided search, google scholar, examples of empirical research, sources and further reading.
- Interpretive Research
- Action-Based Research
- Creative & Experimental Approaches
Your Librarian

- Introductory Video This video covers what empirical research is, what kinds of questions and methods empirical researchers use, and some tips for finding empirical research articles in your discipline.

- Guided Search: Finding Empirical Research Articles This is a hands-on tutorial that will allow you to use your own search terms to find resources.

- Study on radiation transfer in human skin for cosmetics
- Long-Term Mobile Phone Use and the Risk of Vestibular Schwannoma: A Danish Nationwide Cohort Study
- Emissions Impacts and Benefits of Plug-In Hybrid Electric Vehicles and Vehicle-to-Grid Services
- Review of design considerations and technological challenges for successful development and deployment of plug-in hybrid electric vehicles
- Endocrine disrupters and human health: could oestrogenic chemicals in body care cosmetics adversely affect breast cancer incidence in women?
- << Previous: Scholarly and Professional Relationships
- Next: Interpretive Research >>
- Last Updated: Mar 18, 2024 3:36 PM
- URL: https://guides.lib.purdue.edu/research_approaches

- Brandeis Library
- Research Guides
- Find Empirical Studies
- Find Articles & Databases
- Reference Works
- Find Data & Statistics
What is an Empirical Study?
Where to look for empirical studies, find empirical studies: apa psycinfo & apa psycarticles, find empirical studies: eric.
- Search Strategies
An empirical study reports the findings from a study that uses data derived from an actual experiment or observation. Key components of an empirical study:
- Abstract - Provides a brief overview of the research.
- Introduction - The introduction contextualizes the research by providing a review of previous research on the topic. It also is the section where the hypothesis is stated.
- Method - The methods area describes how the research was conducted, including the design of the study, who the participants were and what they did, and any measurements that were taken during the study.
- Results - The results section describes the outcome of the study.
- Discussion - The discussion section addresses the researchers' interpretations of their study and any future implications from their findings.
- References - A list of works that were cited in the study.
Try searching in the following databases for empirical studies in education:
- APA PsycArticles This link opens in a new window Covers general psychology and specialized, basic, applied, clinical and theoretical research in psychology. Contains all journal articles, letters to the editor and errata from each of 49 journals by the APA and 9 from allied organizations. Coverage 1988 to the present.
- APA PsycInfo (EBSCO) This link opens in a new window Citations and summaries of journal articles, book chapters, books, dissertations and technical reports in psychology. Includes information about the psychological aspects of related disciplines such as medicine, psychiatry, nursing, sociology, education, pharmacology, physiology, linguistics, anthropology, business and law. Coverage 1887 to present, includes 1,700+ international sources in over 35 languages.
- Education Research Complete (EBSCO) This link opens in a new window Scholarly journal articles, dissertations, professional development resources, and other materials on topics related to the field of education. Coverage includes early childhood through adult education and all education specialties.
- ERIC (ProQuest) This link opens in a new window Database sponsored by the U.S. Department of Education that provides access to scholarly journals, curriculum and teaching guides, research reports, and other materials related to the field of education.
There are a few strategies you can use to limit your search results to empirical studies within education and related disciplines.
Two of our databases, APA PsycINFO and APA PsycARTICLES offer the option to limit search results to a specific methodology, including empirical studies. The methodology search facet is located on the advanced search page for APA PsycINFO and APA PsycARTICLES near the bottom of the page:

ERIC (ProQuest) doesn't have an easy way to search for empirical studies. You can try adding "empirical" into your search terms or you can try limiting your search results to research reports by completing the following steps:
- Use the "Advanced Search" page
- Type in your search terms
- Scroll down the page to "Document Type" and select "143: Reports- Research"
- Click "Search"

Another strategy for finding empirical studies is to add different combinations of the following search terms:
- methodology (or method)
- action research
- participant observation OR participants
- qualitative or quantitative
- << Previous: Find Data & Statistics
- Next: Search Strategies >>
- Last Updated: Feb 5, 2024 1:36 PM
- URL: https://guides.library.brandeis.edu/education
- What is Empirical Research Study? [Examples & Method]

The bulk of human decisions relies on evidence, that is, what can be measured or proven as valid. In choosing between plausible alternatives, individuals are more likely to tilt towards the option that is proven to work, and this is the same approach adopted in empirical research.
In empirical research, the researcher arrives at outcomes by testing his or her empirical evidence using qualitative or quantitative methods of observation, as determined by the nature of the research. An empirical research study is set apart from other research approaches by its methodology and features hence; it is important for every researcher to know what constitutes this investigation method.
What is Empirical Research?
Empirical research is a type of research methodology that makes use of verifiable evidence in order to arrive at research outcomes. In other words, this type of research relies solely on evidence obtained through observation or scientific data collection methods.
Empirical research can be carried out using qualitative or quantitative observation methods , depending on the data sample, that is, quantifiable data or non-numerical data . Unlike theoretical research that depends on preconceived notions about the research variables, empirical research carries a scientific investigation to measure the experimental probability of the research variables
Characteristics of Empirical Research
- Research Questions
An empirical research begins with a set of research questions that guide the investigation. In many cases, these research questions constitute the research hypothesis which is tested using qualitative and quantitative methods as dictated by the nature of the research.
In an empirical research study, the research questions are built around the core of the research, that is, the central issue which the research seeks to resolve. They also determine the course of the research by highlighting the specific objectives and aims of the systematic investigation.
- Definition of the Research Variables
The research variables are clearly defined in terms of their population, types, characteristics, and behaviors. In other words, the data sample is clearly delimited and placed within the context of the research.
- Description of the Research Methodology
An empirical research also clearly outlines the methods adopted in the systematic investigation. Here, the research process is described in detail including the selection criteria for the data sample, qualitative or quantitative research methods plus testing instruments.
An empirical research is usually divided into 4 parts which are the introduction, methodology, findings, and discussions. The introduction provides a background of the empirical study while the methodology describes the research design, processes, and tools for the systematic investigation.
The findings refer to the research outcomes and they can be outlined as statistical data or in the form of information obtained through the qualitative observation of research variables. The discussions highlight the significance of the study and its contributions to knowledge.
Uses of Empirical Research
Without any doubt, empirical research is one of the most useful methods of systematic investigation. It can be used for validating multiple research hypotheses in different fields including Law, Medicine, and Anthropology.
- Empirical Research in Law : In Law, empirical research is used to study institutions, rules, procedures, and personnel of the law, with a view to understanding how they operate and what effects they have. It makes use of direct methods rather than secondary sources, and this helps you to arrive at more valid conclusions.
- Empirical Research in Medicine : In medicine, empirical research is used to test and validate multiple hypotheses and increase human knowledge.
- Empirical Research in Anthropology : In anthropology, empirical research is used as an evidence-based systematic method of inquiry into patterns of human behaviors and cultures. This helps to validate and advance human knowledge.
Discover how Extrapolation Powers statistical research: Definition, examples, types, and applications explained.
The Empirical Research Cycle
The empirical research cycle is a 5-phase cycle that outlines the systematic processes for conducting and empirical research. It was developed by Dutch psychologist, A.D. de Groot in the 1940s and it aligns 5 important stages that can be viewed as deductive approaches to empirical research.
In the empirical research methodological cycle, all processes are interconnected and none of the processes is more important than the other. This cycle clearly outlines the different phases involved in generating the research hypotheses and testing these hypotheses systematically using the empirical data.
- Observation: This is the process of gathering empirical data for the research. At this stage, the researcher gathers relevant empirical data using qualitative or quantitative observation methods, and this goes ahead to inform the research hypotheses.
- Induction: At this stage, the researcher makes use of inductive reasoning in order to arrive at a general probable research conclusion based on his or her observation. The researcher generates a general assumption that attempts to explain the empirical data and s/he goes on to observe the empirical data in line with this assumption.
- Deduction: This is the deductive reasoning stage. This is where the researcher generates hypotheses by applying logic and rationality to his or her observation.
- Testing: Here, the researcher puts the hypotheses to test using qualitative or quantitative research methods. In the testing stage, the researcher combines relevant instruments of systematic investigation with empirical methods in order to arrive at objective results that support or negate the research hypotheses.
- Evaluation: The evaluation research is the final stage in an empirical research study. Here, the research outlines the empirical data, the research findings and the supporting arguments plus any challenges encountered during the research process.
This information is useful for further research.
Learn about qualitative data: uncover its types and examples here.
Examples of Empirical Research
- An empirical research study can be carried out to determine if listening to happy music improves the mood of individuals. The researcher may need to conduct an experiment that involves exposing individuals to happy music to see if this improves their moods.
The findings from such an experiment will provide empirical evidence that confirms or refutes the hypotheses.
- An empirical research study can also be carried out to determine the effects of a new drug on specific groups of people. The researcher may expose the research subjects to controlled quantities of the drug and observe research subjects to controlled quantities of the drug and observe the effects over a specific period of time to gather empirical data.
- Another example of empirical research is measuring the levels of noise pollution found in an urban area to determine the average levels of sound exposure experienced by its inhabitants. Here, the researcher may have to administer questionnaires or carry out a survey in order to gather relevant data based on the experiences of the research subjects.
- Empirical research can also be carried out to determine the relationship between seasonal migration and the body mass of flying birds. A researcher may need to observe the birds and carry out necessary observation and experimentation in order to arrive at objective outcomes that answer the research question.
Empirical Research Data Collection Methods
Empirical data can be gathered using qualitative and quantitative data collection methods. Quantitative data collection methods are used for numerical data gathering while qualitative data collection processes are used to gather empirical data that cannot be quantified, that is, non-numerical data.
The following are common methods of gathering data in empirical research
- Survey/ Questionnaire
A survey is a method of data gathering that is typically employed by researchers to gather large sets of data from a specific number of respondents with regards to a research subject. This method of data gathering is often used for quantitative data collection , although it can also be deployed during quantitative research.
A survey contains a set of questions that can range from close-ended to open-ended questions together with other question types that revolve around the research subject. A survey can be administered physically or with the use of online data-gathering platforms like Formplus.
Empirical data can also be collected by carrying out an experiment. An experiment is a controlled simulation in which one or more of the research variables is manipulated using a set of interconnected processes in order to confirm or refute the research hypotheses.
An experiment is a useful method of measuring causality; that is cause and effect between dependent and independent variables in a research environment. It is an integral data gathering method in an empirical research study because it involves testing calculated assumptions in order to arrive at the most valid data and research outcomes.
T he case study method is another common data gathering method in an empirical research study. It involves sifting through and analyzing relevant cases and real-life experiences about the research subject or research variables in order to discover in-depth information that can serve as empirical data.
- Observation
The observational method is a method of qualitative data gathering that requires the researcher to study the behaviors of research variables in their natural environments in order to gather relevant information that can serve as empirical data.
How to collect Empirical Research Data with Questionnaire
With Formplus, you can create a survey or questionnaire for collecting empirical data from your research subjects. Formplus also offers multiple form sharing options so that you can share your empirical research survey to research subjects via a variety of methods.
Here is a step-by-step guide of how to collect empirical data using Formplus:
Sign in to Formplus
In the Formplus builder, you can easily create your empirical research survey by dragging and dropping preferred fields into your form. To access the Formplus builder, you will need to create an account on Formplus.
Once you do this, sign in to your account and click on “Create Form ” to begin.
Unlock the secrets of Quantitative Data: Click here to explore the types and examples.
Edit Form Title
Click on the field provided to input your form title, for example, “Empirical Research Survey”.
Edit Form
- Click on the edit button to edit the form.
- Add Fields: Drag and drop preferred form fields into your form in the Formplus builder inputs column. There are several field input options for survey forms in the Formplus builder.
- Edit fields
- Click on “Save”
- Preview form.
Customize Form
Formplus allows you to add unique features to your empirical research survey form. You can personalize your survey using various customization options. Here, you can add background images, your organization’s logo, and use other styling options. You can also change the display theme of your form.
- Share your Form Link with Respondents
Formplus offers multiple form sharing options which enables you to easily share your empirical research survey form with respondents. You can use the direct social media sharing buttons to share your form link to your organization’s social media pages.
You can send out your survey form as email invitations to your research subjects too. If you wish, you can share your form’s QR code or embed it on your organization’s website for easy access.
Empirical vs Non-Empirical Research
Empirical and non-empirical research are common methods of systematic investigation employed by researchers. Unlike empirical research that tests hypotheses in order to arrive at valid research outcomes, non-empirical research theorizes the logical assumptions of research variables.
Definition: Empirical research is a research approach that makes use of evidence-based data while non-empirical research is a research approach that makes use of theoretical data.
Method: In empirical research, the researcher arrives at valid outcomes by mainly observing research variables, creating a hypothesis and experimenting on research variables to confirm or refute the hypothesis. In non-empirical research, the researcher relies on inductive and deductive reasoning to theorize logical assumptions about the research subjects.
The major difference between the research methodology of empirical and non-empirical research is while the assumptions are tested in empirical research, they are entirely theorized in non-empirical research.
Data Sample: Empirical research makes use of empirical data while non-empirical research does not make use of empirical data. Empirical data refers to information that is gathered through experience or observation.
Unlike empirical research, theoretical or non-empirical research does not rely on data gathered through evidence. Rather, it works with logical assumptions and beliefs about the research subject.
Data Collection Methods : Empirical research makes use of quantitative and qualitative data gathering methods which may include surveys, experiments, and methods of observation. This helps the researcher to gather empirical data, that is, data backed by evidence.
Non-empirical research, on the other hand, does not make use of qualitative or quantitative methods of data collection . Instead, the researcher gathers relevant data through critical studies, systematic review and meta-analysis.
Advantages of Empirical Research
- Empirical research is flexible. In this type of systematic investigation, the researcher can adjust the research methodology including the data sample size, data gathering methods plus the data analysis methods as necessitated by the research process.
- It helps the research to understand how the research outcomes can be influenced by different research environments.
- Empirical research study helps the researcher to develop relevant analytical and observation skills that can be useful in dynamic research contexts.
- This type of research approach allows the researcher to control multiple research variables in order to arrive at the most relevant research outcomes.
- Empirical research is widely considered as one of the most authentic and competent research designs.
- It improves the internal validity of traditional research using a variety of experiments and research observation methods.
Disadvantages of Empirical Research
- An empirical research study is time-consuming because the researcher needs to gather the empirical data from multiple resources which typically takes a lot of time.
- It is not a cost-effective research approach. Usually, this method of research incurs a lot of cost because of the monetary demands of the field research.
- It may be difficult to gather the needed empirical data sample because of the multiple data gathering methods employed in an empirical research study.
- It may be difficult to gain access to some communities and firms during the data gathering process and this can affect the validity of the research.
- The report from an empirical research study is intensive and can be very lengthy in nature.
Conclusion
Empirical research is an important method of systematic investigation because it gives the researcher the opportunity to test the validity of different assumptions, in the form of hypotheses, before arriving at any findings. Hence, it is a more research approach.
There are different quantitative and qualitative methods of data gathering employed during an empirical research study based on the purpose of the research which include surveys, experiments, and various observatory methods. Surveys are one of the most common methods or empirical data collection and they can be administered online or physically.
You can use Formplus to create and administer your online empirical research survey. Formplus allows you to create survey forms that you can share with target respondents in order to obtain valuable feedback about your research context, question or subject.
In the form builder, you can add different fields to your survey form and you can also modify these form fields to suit your research process. Sign up to Formplus to access the form builder and start creating powerful online empirical research survey forms.
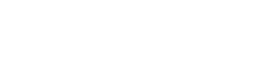
Connect to Formplus, Get Started Now - It's Free!
- advantage of empirical research
- disadvantages of empirical resarch
- empirical research characteristics
- empirical research cycle
- empirical research method
- example of empirical research
- uses of empirical research
- busayo.longe
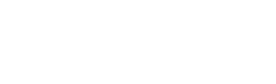
You may also like:
What is Pure or Basic Research? + [Examples & Method]
Simple guide on pure or basic research, its methods, characteristics, advantages, and examples in science, medicine, education and psychology

Extrapolation in Statistical Research: Definition, Examples, Types, Applications
In this article we’ll look at the different types and characteristics of extrapolation, plus how it contrasts to interpolation.
Research Questions: Definitions, Types + [Examples]
A comprehensive guide on the definition of research questions, types, importance, good and bad research question examples
Recall Bias: Definition, Types, Examples & Mitigation
This article will discuss the impact of recall bias in studies and the best ways to avoid them during research.
Formplus - For Seamless Data Collection
Collect data the right way with a versatile data collection tool. try formplus and transform your work productivity today..
- Connelly Library
Qualitative and Quantitative Research
What is "empirical research".
- empirical research
- Locating Articles in Cinahl and PsycInfo
- Locating Articles in PubMed
- Getting the Articles
Empirical research is based on observed and measured phenomena and derives knowledge from actual experience rather than from theory or belief.
How do you know if a study is empirical? Read the subheadings within the article, book, or report and look for a description of the research "methodology." Ask yourself: Could I recreate this study and test these results?
Key characteristics to look for:
- Specific research questions to be answered
- Definition of the population, behavior, or phenomena being studied
- Description of the process used to study this population or phenomena, including selection criteria, controls, and testing instruments (such as surveys)
Another hint: some scholarly journals use a specific layout, called the "IMRaD" format, to communicate empirical research findings. Such articles typically have 4 components:
- Introduction : sometimes called "literature review" -- what is currently known about the topic -- usually includes a theoretical framework and/or discussion of previous studies
- Methodology: sometimes called "research design" -- how to recreate the study -- usually describes the population, research process, and analytical tools
- Results : sometimes called "findings" -- what was learned through the study -- usually appears as statistical data or as substantial quotations from research participants
- Discussion : sometimes called "conclusion" or "implications" -- why the study is important -- usually describes how the research results influence professional practices or future studies
- << Previous: Home
- Next: Locating Articles in Cinahl and PsycInfo >>

© Copyright La Salle University. All rights reserved.

EDU 610: Educational Research: Empirical Research
- Library Catalog
- Tips for GALILEO
- Check for Full Text
- Citation Help
- Web Resources
- Learning Commons This link opens in a new window
- Empirical Research
- Postgraduate Research
- Microsoft Word
- Word - Basic Formatting
- Google Docs
- Google - Basic Formatting
- TU Writing Resources
- APA Sample Paper
- APA Citations
- Reference Examples
- Book with Editor(s)
- Chapters and Parts of Books
- Company Reports
- Journal Article
- Magazine Article
- Patents & Laws
- Unpublished Manuscripts/Informal Publications (i.e. course packets and dissertations)
- Installing Zotero
- Gathering Citations with Zotero
- Word Processing with Zotero
- Troubleshooting
229-227-6959
Make an appointment with a Librarian
Make an appointment with a Tutor
Follow us on:

Empirical Research Studies
- Defining Empirical Articles
- Searching for Empirical Articles
- Examples of Empirical Articles
What is an empirical article?
Empirical research articles are scholarly, peer-reviewed journal articles that are based on data collected during the authors' real-life experiments or observations. They are primary research documents that contain either qualitative or quantitative research methods:
- Qualitative research focuses on collecting in-depth information from small sample sizes in order to describe a trend. Data is typically collected through one-on-one interviews with participants.
- Quantitative research uses large, representative sample sizes to collect a variety of statistics that can then be generalized. Data is typically collected through a questionnaire, attitude scale, or achievement test.
How can I tell if an article is empirical?
Several factors can help you decide whether or not an article is empirical:
- Academic journals such as Review of Educational Research , Journal of Educational Psychology , and Child Development often publish empirical articles.
- Popular magazines such as Time or Newsweek don't publish empirical articles.
- Professional journals such as the Journal of Technology and Teacher Education or Educational Leadership will publish empirical articles, while professional magazines (e.g., TEACH , Education Today , and Education Matters ) won't publish empirical articles.
- Did the author(s):
- Administer a survey or questionnaire ?
- Conduct an interview ?
- Collect data ?
- Use an assessment to measure results?
- Empirical articles include many of the above characteristics.
- Introduction/Literature Review
- This section will include information on how the study was conducted: how it was designed, who the participants were and how they participated, and how the results were measured.
- This information allows other researchers to replicate the study with their own participants.
- Results/Findings
- Discussion/Conclusion/Implications
- Although authors might combine some sections, label them differently, or not use any headings at all, empirical articles will contain all of the above information.
- Because empirical articles contain so many details about their studies, they tend to be longer.
- They also contain charts, tables, and other graphics to help display the data that was collected.
College of Southern Maryland. CSM Library. (2018, Jan. 19). Empirical research article. Retrieved July 15, 2019, from https://libguides.csmd.edu/empirical_research
Pan, M. L. (2016). Preparing literature reviews: Qualitative and quantitative approaches (5th ed.). New York, NY: Routledge.
Penn State University Libraries. (2019, May 9). Empirical research in the social sciences and education. Retrieved July 15, 2019, from https://guides.libraries.psu.edu/emp
University of La Verne. Wilson Library. (2018, June 26). Identify empirical research articles. Retrieved July 15, 2019, from https://laverne.libguides.com/empirical-articles
GALILEO (and EBSCOhost databases)
Consider adding one of the following key terms to your search:
- Data Analysis
- Field Study
- Investigation
- Observation
- Questionnaire
- Statistical Analysis
For best results, use GALILEO's Advanced Search option. This will let you put your chosen key term in its own search box using the AND operator.
Another option is to limit your results by type:

- Look for the Refine Results column on the left-hand side of your results list.
- Underneath the Limit by Type section, click on Reports .
- Underneath the Limit To section, click on both the Full Text and Scholarly (Peer Reviewed) Journals options.
Look for similar options when searching subject-specific databases.
Education Database (ProQuest)
- Click on the Document type section on the left-hand side of your results list.
- In the drop-down menu that appears, click on the More option.
- Statistics/Data Report
Education Database (ProQuest) http://www.galileo.usg.edu/express?link=zued&inst=thco

ERIC (https://eric.ed.gov/)
- Look for the Descriptor section on the left-hand side of your results list.
- Questionnaires
- Qualitative Research
- Click on the More option to view more descriptors.

- Look for the Publication Type section on the left-hand side of your results list.
- Reports - Research
- Reports - Evaluative
- Tests/Questionnaires
- Numerical/Qualitative Data
- Click on the More option to view more publication types.

Lawrence, A. C., Al-Bataineh, A. T., & Hatch, D. (2018). Educator perspectives on the instructional effects of one-to-one computing implementation. Contemporary Educational Technology, 9 (2), 206-224. https://doi.org/10.30935/cet.414950

Semerci, A. (2018). Students' views on the use of tablet computers in education. World Journal on Educational Technology: Current Issues, 10 (2), 104-114. https://doi.org/10.18844/wjet.v10i2.3420

Turner, K. (2019). One-to-one learning and self-determination theory. International Journal of Instruction, 12 (2), 1-16. https://doi.org/10.29333/iji.2019.1221a

USU Libraries. (2019, April 22). What's empirical research? [Video file]. Retrieved from https://www.youtube.com/watch?v=fZ-LGZdqWLU
- << Previous: Learning Commons
- Next: Postgraduate Research >>
- Last Updated: Oct 23, 2023 1:26 PM
- URL: https://libguides.thomasu.edu/edu610

Identifying Empirical Research Articles
Identifying empirical articles.
- Searching for Empirical Research Articles
What is Empirical Research?
An empirical research article reports the results of a study that uses data derived from actual observation or experimentation. Empirical research articles are examples of primary research. To learn more about the differences between primary and secondary research, see our related guide:
- Primary and Secondary Sources
By the end of this guide, you will be able to:
- Identify common elements of an empirical article
- Use a variety of search strategies to search for empirical articles within the library collection
Look for the IMRaD layout in the article to help identify empirical research. Sometimes the sections will be labeled differently, but the content will be similar.
- I ntroduction: why the article was written, research question or questions, hypothesis, literature review
- M ethods: the overall research design and implementation, description of sample, instruments used, how the authors measured their experiment
- R esults: output of the author's measurements, usually includes statistics of the author's findings
- D iscussion: the author's interpretation and conclusions about the results, limitations of study, suggestions for further research
Parts of an Empirical Research Article
Parts of an empirical article.
The screenshots below identify the basic IMRaD structure of an empirical research article.
Introduction
The introduction contains a literature review and the study's research hypothesis.

The method section outlines the research design, participants, and measures used.

Results
The results section contains statistical data (charts, graphs, tables, etc.) and research participant quotes.

The discussion section includes impacts, limitations, future considerations, and research.

Learn the IMRaD Layout: How to Identify an Empirical Article
This short video overviews the IMRaD method for identifying empirical research.
- Next: Searching for Empirical Research Articles >>
- Last Updated: Nov 16, 2023 8:24 AM
CityU Home - CityU Catalog

The Library Is Open
The Wallace building is now open to the public. More information on services available.
- RIT Libraries
- Empirical Studies
This guide will help you get started with your psychology research. In addition to recommending useful databases, this guide provides information on finding and accessing tests and measures, and other helpful tips to guide you along the way.
- Journals & Databases
- Tests and Measures
- Get Better Results
- APA Citation Guide This link opens in a new window
What is an empirical study?
An empirical study is one that is based on "observation, investigation, or experiment rather than on abstract reasoning, theoretical analysis, or speculation." * Empirical studies should be divided into the following parts: abstract, introduction, method, results, discussion, and references. Typically these studies also include tables, figures, and charts to display collected data.
Here is an example of an empirical study:
Westervelt, H. J., Bruce, J. M., & Faust, M. A. (2016). Distinguishing Alzheimer’s disease and dementia with Lewy bodies using cognitive and olfactory measures . Neuropsychology , 30 (3), 304-311. doi:10.1037/neu0000230
Finding empirical studies
Finding empirical studies is simple using RIT Library's databases! Both PsycArticles and PyscINFO allow you to narrow your results to empirical studies before you even click "Search." The steps outlined below apply to both:
- switch from basic search to advanced search
- look below the search bars for your available filters/limiters
- go the the "Methodology" set of options
- click on "Empirical"
- Now begin your search
A Couple Quick Tips :
- such as, "multiple personality disorder"
- such as, addict* will tell the search software to look for addict, addicted, addictive, addictions, addicts, addicting...
- Use keywords and phrases, never use sentences or as a question
- Use more of your filters for choosing: a date range, FULL TEXT, scholarly/peer reviewed articles, geography (region/country), language, etc.
- Grab the citation in the format you need. You may have to correct it for complete accuracy, but at least you'll have a start
- Save the link or download and save the article. Trying to find something awesome after the fact can be brutal
*EVEN MORE TIPS ON THE "Get Better Results" page :-)
- APA PsycArticles This link opens in a new window
- APA PsycINFO This link opens in a new window
- << Previous: Journals & Databases
- Next: Tests and Measures >>
Edit this Guide
Log into Dashboard
Use of RIT resources is reserved for current RIT students, faculty and staff for academic and teaching purposes only. Please contact your librarian with any questions.

Help is Available

Email a Librarian
A librarian is available by e-mail at [email protected]
Meet with a Librarian
Call reference desk voicemail.
A librarian is available by phone at (585) 475-2563 or on Skype at llll
Or, call (585) 475-2563 to leave a voicemail with the reference desk during normal business hours .
Chat with a Librarian
Psychology infoguide url.
https://infoguides.rit.edu/psychology
Use the box below to email yourself a link to this guide
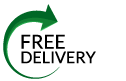
The Plagiarism Checker Online For Your Academic Work
Start Plagiarism Check
Editing & Proofreading for Your Research Paper
Get it proofread now
Online Printing & Binding with Free Express Delivery
Configure binding now
- Academic essay overview
- The writing process
- Structuring academic essays
- Types of academic essays
- Academic writing overview
- Sentence structure
- Academic writing process
- Improving your academic writing
- Titles and headings
- APA style overview
- APA citation & referencing
- APA structure & sections
- Citation & referencing
- Structure and sections
- APA examples overview
- Commonly used citations
- Other examples
- British English vs. American English
- Chicago style overview
- Chicago citation & referencing
- Chicago structure & sections
- Chicago style examples
- Citing sources overview
- Citation format
- Citation examples
- College essay overview
- Application
- How to write a college essay
- Types of college essays
- Commonly confused words
- Definitions
- Dissertation overview
- Dissertation structure & sections
- Dissertation writing process
- Graduate school overview
- Application & admission
- Study abroad
- Master degree
- Harvard referencing overview
- Language rules overview
- Grammatical rules & structures
- Parts of speech
- Punctuation
- Methodology overview
- Analyzing data
- Experiments
- Observations
- Inductive vs. Deductive
- Qualitative vs. Quantitative
- Types of validity
- Types of reliability
- Sampling methods
- Theories & Concepts
- Types of research studies
- Types of variables
- MLA style overview
- MLA examples
- MLA citation & referencing
- MLA structure & sections
- Plagiarism overview
- Plagiarism checker
- Types of plagiarism
- Printing production overview
- Research bias overview
- Types of research bias
- Example sections
- Types of research papers
- Research process overview
- Problem statement
- Research proposal
- Research topic
- Statistics overview
- Levels of measurment
- Frequency distribution
- Measures of central tendency
- Measures of variability
- Hypothesis testing
- Parameters & test statistics
- Types of distributions
- Correlation
- Effect size
- Hypothesis testing assumptions
- Types of ANOVAs
- Types of chi-square
- Statistical data
- Statistical models
- Spelling mistakes
- Tips overview
- Academic writing tips
- Dissertation tips
- Sources tips
- Working with sources overview
- Evaluating sources
- Finding sources
- Including sources
- Types of sources
Your Step to Success
Plagiarism Check within 10min
Printing & Binding with 3D Live Preview
Empirical Research – Characteristics & Advantages
How do you like this article cancel reply.
Save my name, email, and website in this browser for the next time I comment.
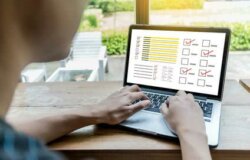
Many people ascribe to the quote, “I will not believe it unless I see it.” What most don’t know is that this quote came from empirical research enthusiasts in the renaissance period and is currently a cornerstone for modern science and research. The word empirical has a Greek origin from empeirikos, meaning experienced.

Inhaltsverzeichnis
- 1 Empirical Research – FAQs
- 2 Empirical Research: Definition
- 3 Characteristics of Empirical Research
- 4 Uses of Empirical Research
- 5 Empirical Research Methods
- 6 Empirical vs Non-Empirical Research
- 7 Advantages of Empirical Research
- 8 In a Nutshell
Empirical Research – FAQs
What is empirical research.
This is a type of research whose findings and conclusions are based on valid data or evidence. These pieces of evidence are collected using either or both qualitative research and quantitative research . In philosophy, empiricism is concluding direct observations and assessment instead of using logic alone.
What are examples of Empirical Research?
An excellent example of empirical research is the process of DNA testing and its findings.
What is Empirical and Non-Empirical Research?
While empirical research focuses on individual pieces of evidence and facts, the non-empirical study considers personal judgement and authoritative experience as necessary as the data collected.
What are the three types of research questions?
A research project can use either descriptive, relational, or causal questions to collect and analyse data.
What's the need for Empirical Research?
This form of research is quite useful in science, history, and business as it validates hypothesis, thus increasing human knowledge with tangible pieces of evidence.
Empirical Research: Definition
Empirical Resarch is a type of research where the conclusions and decisions are based on valid data or evidence. Empirical research can be analyzed quantitatively or qualitatively. Instead of using only logic, in philosophy empiricism is concluding direct observations and assessment.
Characteristics of Empirical Research
The distinctive feature of empirical research is its strictness in collecting and observing data and experiences to collect useful information that is used to create a provable analysis. For this reason, the research has six main characteristics, better known as the steps to complete empirical research.

Abstract/Purpose
The first step involves coming up with the foundational purpose of the research. The researcher answers questions like:
- What do I want to find out?
- How do I define the problem statement?
- Is the data readily available?
- Are there challenges that might limit the collection of data in terms of time, resources, language barrier, etc.?
- What is the ratio between the research itself and the finding in terms of profitability? In short, is it worth the effort?
This is the area where the researcher goes deep into why he/she wants to perform it and what he/she seeks to find or prove from the outcome.

Introduction
The second step involves having an initial report for the research. Here, any facts ever documented on the same are highlighted plus the statement of hypotheses for the current study. Also, the parties involved describe tolerable errors, measurements, and acceptable methods in this section.

This is where the actual research takes place, plus the recording of the process and data. Researchers decide whether they will use experimental or non-experimental methods for data collection in relation t the hypotheses. Depending on the field of research, they will then carve out a research design that can maximise their resources and methods of data collection. Data collection techniques are then used, which might include sampling and questionnaire methods.

Some researchers merge this part with step 3, while others handle them separately. This is where the findings from the data collection methods are put together. The data is then cleaned and analysed.

Data analysis methods include qualitative or quantitative methods. From either or both of these methods, researchers check their findings to support or reject their hypothesis. A discussion on the interpretation and implications of the findings plus the study as a whole is then documented for formal use.

Finally, credit is given to whomever the credit is due. Citations or a bibliography with materials used in the report are also included.
Uses of Empirical Research
Thanks to it insisting on having tangible pieces of evidence, empirical research comes in handy in various disciplines. The most prevalent uses are in medicine, anthropology and law. In medicine, the research helps to prove several hypotheses that increase human knowledge on the body’s behaviour and adaptability to new methods of handling diseases.
In the legal field, this type of research helps to study how rules and regulations impact the society or a certain institution understudy while in anthropology, it follows human patterns to understand cultures.
Empirical Research Methods
The two main methods used in empirical research are quantitative and qualitative. In quantitative research , numerical data is used to collect information or any other quantifiable process. The most common examples are polls, longitudinal studies and surveys.
On the other hand, qualitative research focuses more on meaning, underlying reasons and opinions from the data collected. The method takes on an unstructured approach or semi-structured. By the nature of its data, the method provides in-depth information and is often a smaller sample. Its examples include interviews, groups, and experiments.
Empirical vs Non-Empirical Research
While empirical research stops at verifiable data, non-empirical research includes authoritative opinions and logic. This is to add some meaning to the data for further elaboration. In short, data from the non-empirical study is theorised. While empirical research uses qualitative and quantitative methods, a researcher using the non-empirical method will use a lot of systematic reviews, meta-analysis, and critical studies to conclude the matter at hand.
GOOD TO KNOW: Read our article about Research Methodology !
Advantages of Empirical Research
First and foremost, this form of research has increased the credibility of findings, making it easy for someone to make an argument out of facts instead of theoretical assumptions. It keeps works authentic, formal, and verifiable, thus increasing the quality depth of information.
Secondly, empirical research is quite flexible. The researcher has the authority to adjust methodologies and also the sample size to suit any form of necessity. All in all, it is easy and the most reliable type of research.
In a Nutshell
Empirical research is, in short, an essential approach to building up information and making provable arguments to support specific implications. To wrap this up:
- Empirical research is a valid form of research that leaves no room for misinterpretation of information.
- Both qualitative and quantitative methods help to draw viable conclusions and are useful in this form of research.
- A researcher is free to explore the best approach to reach a viable conclusion within the empirical survey.
We use cookies on our website. Some of them are essential, while others help us to improve this website and your experience.
- External Media
Individual Privacy Preferences
Cookie Details Privacy Policy Imprint
Here you will find an overview of all cookies used. You can give your consent to whole categories or display further information and select certain cookies.
Accept all Save
Essential cookies enable basic functions and are necessary for the proper function of the website.
Show Cookie Information Hide Cookie Information
Statistics cookies collect information anonymously. This information helps us to understand how our visitors use our website.
Content from video platforms and social media platforms is blocked by default. If External Media cookies are accepted, access to those contents no longer requires manual consent.
Privacy Policy Imprint
Penn State University Libraries
Empirical research in the social sciences and education.
- What is Empirical Research and How to Read It
- Finding Empirical Research in Library Databases
- Designing Empirical Research
- Ethics, Cultural Responsiveness, and Anti-Racism in Research
- Citing, Writing, and Presenting Your Work
Contact the Librarian at your campus for more help!

Introduction
Empirical research is published in books and in scholarly, peer-reviewed journals. However, most library databases do not offer straightforward ways to locate empirical research. Below are tips for some of Penn State's most popular Education and Behavioral/Social Sciences databases. If you need further help, contact a Librarian at your location .
Finding Empirical Research in LionSearch
- LionSearch This link opens in a new window
LionSearch does not have a method for locating empirical research. Using "empirical" as a keyword will find some studies, but miss many others. Consider using one of the more specialized databases below.
Finding Empirical Research in PsycINFO (ProQuest version, for Psychology topics)
- PsycINFO (via ProQuest) This link opens in a new window more... less... PsycINFO provides access to international literature in psychology and related disciplines. Unrivaled in its depth of psychological coverage and respected worldwide for its high quality, the database is enriched with literature from an array of disciplines related to psychology such as psychiatry, education, business, medicine, nursing, pharmacology, law, linguistics, and social work. Nearly all records contain nonevaluative summaries, and all records from 1967 to the present are indexed using the Thesaurus of Psychological Index Terms.
To find empirical articles in PsycINFO (ProQuest version):
- Use the "Advanced Search"
- Type your keywords into the search boxes
- Scroll down the page to "Methodology," and choose "Empirical Study"
- Choose other limits, such as publication date, if needed
- Click on the "Search" button
Finding Empirical Research in ERIC (ProQuest version, for Education topics)
- ERIC (ProQuest) This link opens in a new window more... less... ERIC (Educational Resources Information Center) is the major database for education literature, sponsored by the U.S. Department. of Education. The same database content is available on many platforms.
- Scroll down the page to "Document Type," and choose "143 Reports: Research"
- Action Research
- Case Studies
- Content Analysis
- Data Analysis
- Ethnography
- Evaluation Methods
- Evaluation Research
- Experiments
- Focus Groups
- Field Studies
- Longitudinal Studies
- Mail Surveys
- Mixed Methods Research
- Naturalistic Observation
- Online Surveys
- Participant Observation
- Participatory Research
- Qualitative Research
- Questionnaires
- Statistical Analysis
- Statistical Studies
- Statistical Surveys
- Telephone Surveys
- Use Studies
Finding Empirical Research in Sociological Abstracts (ProQuest version)
- Sociological Abstracts This link opens in a new window more... less... CSA Sociological Abstracts abstracts and indexes the international literature in sociology and related disciplines in the social and behavioral sciences. The database provides abstracts of journal articles and citations to book reviews drawn from over 1,700 serials publications, and also provides abstracts of books, book chapters, dissertations, and conference papers. Records added after 1974 contain in-depth and nonevaluative abstracts of journal articles.
- Archival Research
- Discourse Analysis
- Grounded Theory
- Observation
- Oral History
- Quantitative Analysis
Finding Empirical Research in Criminal Justice Abstracts (EBSCO version)
- Criminal Justice Abstracts This link opens in a new window more... less... Provides abstracts of articles from the major journals in criminology and related disciplines, as well as books and reports from government and nongovernmental agencies. For each document, an informative summary of the findings, methodology, and conclusions is provided. Topics include crime trends, prevention projects, corrections, juvenile delinquency, police, courts, offenders, victims, and sentencing.
Criminal Justice Abstracts (EBSCO version) does not have a simple method to locate empirical research. Using "empirical" as a keyword will find some studies, but miss others. Consider using terminology recommended by the Criminal Justice Abstracts subject index. Some useful keywords are:
- Empirical Research
- Quantitative Research
Finding Empirical Research in Worldwide Political Science Abstracts (ProQuest version)
- Worldwide Political Science Abstracts This link opens in a new window more... less... Worldwide Political Science Abstracts is building on the merged backfiles of Political Science Abstracts, published by IFI / Plenum, 1975-2000, and ABC POL SCI, published by ABC-CLIO, 1984-2000. The database provides citations, abstracts, and indexing of the international serials literature in political science and its complementary fields, including international relations, law, and public administration / policy. The serials list of the new database is actively under construction, with a focus on expanding international coverage. As of February 2004 approximately 1,432 titles are being monitored for coverage; this list will continue to grow.
Worldwide Political Science Abstracts (ProQuest version) does not have a simple method to locate empirical research. Using "empirical" as a keyword will find some studies, but miss others. Consider using terminology recommended by the Worldwide Political Science Abstracts thesaurus. Some useful keywords are:
- Public Opinion Research
Finding Empirical Research in Linguistics and Language Behavior Abstracts (ProQuest version)
- Linguistics and Language Behavior Abstracts (LLBA) This link opens in a new window more... less... The definitive database on the nature and use of language, Linguistics and Language Behavior Abstracts covers three fundamental areas: research in linguistics (the nature and structure of human speech); research in language.
- Computer Modeling and Simulation
Finding Empirical Research in CINAHL (EBSCO version, for Nursing and Allied Health topics)
- CINAHL (Cumulative Index for Nursing and Allied Health) This link opens in a new window more... less... One of two major databases for nursing, providing references to over 1,800 nursing and allied health journal articles in addition to citations for book chapters, nursing dissertations, association publications, educational software, conference proceedings and selected full-text for state nursing journal articles, legal cases, patient education material, research instruments, standards of practice, critical paths, nurse practice acts, drugs, clinical innovations and government publications. References for alternative/complementary medicine, consumer health and health sciences librarianship are also included. Coverage: 1982 - Present. Updates: Monthly.
- Clinical Trials
- Ethnographic Research
- Experimental Studies
- Naturalistic Inquiry
- Nonexperimental Studies
- One-Shot Case Study
- Phenomenological Research
- Qualitative Studies
- Quantitative Studies
- Randomized Controlled Trials
- Time and Motion Studies
- Under "Limit your results," check off "Evidence-Based Practice"
- Choose other limits, such as published date, if needed
Finding Empirical Research in PubMed (NIH version, for health topics)
- PubMed (Medline) This link opens in a new window more... less... PubMed is a web interface that allows you to search MEDLINE, the National Library of Medicine's premier database of citations and abstracts for biomedical research articles. The core subject is medicine, but subject coverage also includes bioethics, biology, chemistry, dentistry, environmental health, genetics, gerontology, health care planning and administration, history of medicine, hospital administration, microbiology, nutrition, nursing (International Nursing Index), physiology, pre-clinical sciences, public health, sports medicine, veterinary medicine and zoology. MEDLINE covers over 4,800 journals published in the United States and 70 other countries. The database contains over 15 million citations dating back to 1950. Coverage is worldwide and updated weekly. Learn more about PubMed at: https://pubmed.ncbi.nlm.nih.gov/about/. or Try the Tutorial at: http://www.nlm.nih.gov/bsd/pubmed_tutorial/m1001.html
There are 2 ways to find empirical articles in PubMed (NIH version):
One technique is to limit your search results after you perform a search:
- Type in your keywords and click on the "Search" button
- To the left of your results, under "Article Types," check off the types of studies that interest you
Another alternative is to construct a more sophisticated search:
- From PubMed's main screen, click on "Advanced" link underneath the search box
- On the Advanced Search Builder screen type your keywords into the search boxes
- Change one of the empty boxes from "All Fields" to "Publication Type"
- To the right of Publication Type, click on "Show Index List" and choose a methodology that interests you. You can choose more than one by holding down the "Ctrl" or "⌘" on your keyboard as you click on each methodology
- << Previous: What is Empirical Research and How to Read It
- Next: Designing Empirical Research >>
- Last Updated: Feb 18, 2024 8:33 PM
- URL: https://guides.libraries.psu.edu/emp
- Survey Software The world’s leading omnichannel survey software
- Online Survey Tools Create sophisticated surveys with ease.
- Mobile Offline Conduct efficient field surveys.
- Text Analysis
- Close The Loop
- Automated Translations
- NPS Dashboard
- CATI Manage high volume phone surveys efficiently
- Cloud/On-premise Dialer TCPA compliant Cloud on-premise dialer
- IVR Survey Software Boost productivity with automated call workflows.
- Analytics Analyze survey data with visual dashboards
- Panel Manager Nurture a loyal community of respondents.
- Survey Portal Best-in-class user friendly survey portal.
- Voxco Audience Conduct targeted sample research in hours.
- Predictive Analytics
- Customer 360
- Customer Loyalty
- Fraud & Risk Management
- AI/ML Enablement Services
- Credit Underwriting

Find the best survey software for you! (Along with a checklist to compare platforms)
Get Buyer’s Guide
- 100+ question types
- Drag-and-drop interface
- Skip logic and branching
- Multi-lingual survey
- Text piping
- Question library
- CSS customization
- White-label surveys
- Customizable ‘Thank You’ page
- Customizable survey theme
- Reminder send-outs
- Survey rewards
- Social media
- SMS surveys
- Website surveys
- Correlation analysis
- Cross-tabulation analysis
- Trend analysis
- Real-time dashboard
- Customizable report
- Email address validation
- Recaptcha validation
- SSL security
Take a peek at our powerful survey features to design surveys that scale discoveries.
Download feature sheet.
- Hospitality
- Financial Services
- Academic Research
- Customer Experience
- Employee Experience
- Product Experience
- Market Research
- Social Research
- Data Analysis
- Banking & Financial Services
- Retail Solution
- Risk Management
- Customer Lifecycle Solutions
- Net Promoter Score
- Customer Behaviour Analytics
- Customer Segmentation
- Data Unification
Explore Voxco
Need to map Voxco’s features & offerings? We can help!
Watch a Demo
Download Brochures
Get a Quote
- NPS Calculator
- CES Calculator
- A/B Testing Calculator
- Margin of Error Calculator
- Sample Size Calculator
- CX Strategy & Management Hub
- Market Research Hub
- Patient Experience Hub
- Employee Experience Hub
- Market Research Guide
- Customer Experience Guide
- The Voxco Guide to Customer Experience
- NPS Knowledge Hub
- Survey Research Guides
- Survey Template Library
- Webinars and Events
- Feature Sheets
- Try a sample survey
- Professional services
- Blogs & White papers
- Case Studies
Find the best customer experience platform
Uncover customer pain points, analyze feedback and run successful CX programs with the best CX platform for your team.
Get the Guide Now

We’ve been avid users of the Voxco platform now for over 20 years. It gives us the flexibility to routinely enhance our survey toolkit and provides our clients with a more robust dataset and story to tell their clients.
VP Innovation & Strategic Partnerships, The Logit Group
- Client Stories
- Voxco Reviews
- Why Voxco Research?
- Why Voxco Intelligence?
- Careers at Voxco
- Vulnerabilities and Ethical Hacking
Explore Regional Offices
- Cloud/On-premise Dialer TCPA compliant Cloud & on-premise dialer
- Fraud & Risk Management
Get Buyer’s Guide
- Banking & Financial Services
Explore Voxco
Watch a Demo
Download Brochures
- CX Strategy & Management Hub
- Blogs & White papers
VP Innovation & Strategic Partnerships, The Logit Group
- Our clients
- Client stories
- Featuresheets
Dive into Empirical Research: An Insightful Overview
- April 1, 2021
Market Research Tool kit
Get started with Voxco’s Market Research Toolkit.
Market Research trends guide + Online Surveys guide + Agile MArket Research Guide + 5 Market Research Templates
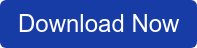
SHARE THE ARTICLE ON

What is Empirical research?
In empirical study, conclusions of the study are drawn from concrete empirical evidence. This evidence is also referred to as “verifiable” evidence. This evidence is gathered either through quantitative market research or qualitative market research methods.
An example of empirical analysis would be if a researcher was interested in finding out whether listening to happy music promotes prosocial behaviour. An experiment could be conducted where one group of the audience is exposed to happy music and the other is not exposed to music at all. The participants could be given an opportunity to either help a stranger with something or not. The results are then evaluated to find whether happy music increases prosaically behavior or not.

See Voxco survey software in action with a Free demo.
What is an Empirical Study?
The origin of empirical methods starts from the quote “I will not believe it unless I see it myself.” Empirical observation emerged during the renaissance with medieval science. The word empirical is derived from the Greek word ‘empeirikos’ meaning ‘experienced’.
The word empirical, in today’s day and age, refers to collecting empirical data through methods of observation, experience, or by specific scientific instruments. All of these methods are dependent on observation and experiments which are used to collect data and test the same for arriving at conclusions. Online survey tools are an extremely effective technique which can be used for empirical methods.
Types and methodologies of empirical research
Empirical study uses qualitative or quantitative methods to conduct research and analyze results.
- Quantitative research: Quantitative research is referred to as the process of collecting as well as analyzing numerical data. It is generally used to find patterns, averages, predictions, as well as cause-effect relationships between the variables being studied. It is also used to generalize the results of a particular study to the population in consideration.

- Qualitative research: Qualitative research can be defined as a method used for market research which aims at obtaining data through open-ended questions and conversations with the intended consumers. This method aims at establishing not only “what” people think but “how” they come to that opinion as well as “why” they think so.
Step by Step guide to Descriptive Research
Get ready to uncover the how, when, what, and where questions in a research problem
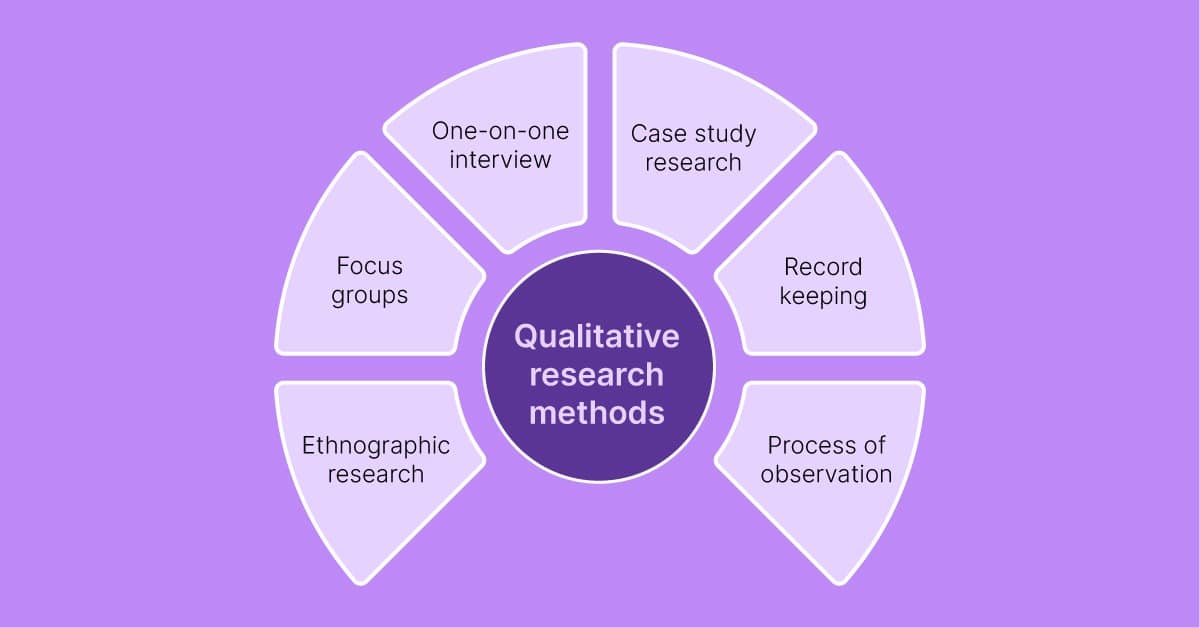
The empirical data that is collected from either of these methods has to be analyzed. Empirical evidence is analyzed using qualitative or quantitative methods. These methods are used to answer empirical questions that are clearly defined. The type of research design used by the researcher depends on the field and the nature of the problem. Some researchers use a combination of quantitative and qualitative methods to answer the questions set for the research.
Quantitative research methods
Quantitative research methods help in the analysis of the empirical evidence that has been gathered. By using these methods researchers can find support for their hypotheses.
- Survey research: Survey research is the most common and widely used tool for quantitative research. Surveys are used to gather data by asking relevant questions to the respondents who are thought to have the relevant information we are seeking to acquire. Generally, a formal list of questionnaires is prepared which is circulated to the respondents and they can self-report their thoughts. Researchers use a non-disguised approach so that the participants of the survey know exactly what they are answering. In general, respondents are asked questions regarding their demographic details, and the opinion that the researcher is interested in studying. Surveys can be conducted through online polls, paper-pencil questionnaires, web-intercept surveys, etc.
For example: In market research, customers are deemed as the most important part of the organisation. It is a known fact that satisfied customers will help your organisation grow directly by remaining loyal to your company and also by becoming an advocate for your brand. Researchers can use customer satisfaction survey templates to assess their brand’s value and how likely their customers are to recommend their brand to others.
- Experimental research : This is one of the most recommended and reliant research methods in natural as well as social sciences. As the name suggests, experimental research (also known as experimentation) is usually based on one of more theories as its driving principle or rationale. In this method, the theory which is under study has not yet proven, it is merely a speculation. Thus, an experiment is performed in order to either prove or disprove the theory. If the results of the experiment are in line with the prediction made by the theory, then the theory is supported. If not, then the theory is refuted.
For instance, if a researcher wants to study whether their dandruff protection product is successful in curing dandruff, and the only difference between the two groups under study is the product of interest (one group uses the product while group 2 uses a placebo), then dandruff could be considered as the dependent variable and the product curing it would be called an independent variable. Now, the independent variable, here, is “manipulated” in the sense that one group is exposed to it and one is not. All things being constant, if the product cures dandruff in group 1 as opposed to the group that is using a placebo, the experimental research findings are successful. This will help in establishing a cause and effect relationship, the product is “causing” the treatment (“effect”) of dandruff.
- Correlational research : A correlation refers to an association or a relationship between two entities. A correlational research studies how one entity impacts the other and what are the changes that are observed when either one of them changes. correlation coefficient ranges from -1 to +1. A correlation coefficient of +1 indicates a perfect positive correlation whereas a correlation coefficient of -1 indicates a perfect negative correlation between two variables. A correlation coefficient of 0 indicates that there is no relationship between the variables under study.
Some examples of correlational research questions:
- What is the relationship between gender and the purchase of a particular product under study?
- The relationship between stress and burnout in employees of an organisation.
- The relationship between choosing to work from home and the level of corona-phobia in employees.
- Longitudinal study : Longitudinal surveys, on the other hand, involve studying variables for a long period of time and observing the changes in them from time to time. Here, the data is collected from the respondents at the beginning of the study, and then the researcher collects data at different time intervals until the end of the study. Longitudinal surveys are more popularly used in medicinal science to understand and evaluate the effects of medicines, or vaccines, in the long-run on participants. Because longitudinal surveys take place for several years, researchers can establish the sequence of events that may affect the variable under study.
For example: If researchers want to understand how smoking affects the development of cancer in later stages of life, they would choose participants who are different from other observable variables but similar in one: smoking. In this case, researchers would observe the participants who started smoking from adolescence into later adulthood and examine the changes in their body that are caused due to smoking. They can see how smoking has influenced the immunity of participants, their reaction to stress, and other variables relevant to the researcher. Over time, researchers can also observe the effects of quitting smoking if some participants decide to quit smoking later in their life. This will help researchers understand the interaction between health and smoking in more detail.
- Cross sectional: In cross-sectional surveys, the study takes place at a single point in time. Hence, cross-sectional surveys do not entail the manipulation of the variables under study, and are limited in that way. Cross-sectional surveys allow researchers to study various characteristics, such as the demographic structure of the consumers, their interests, and attitudes, all at once. It aims to provide information about the population at the current moment in time. For example, cross-sectional surveys will tell us how the consumer is responding and feeling about the product at the present moment. It does not study the other variables that may affect the consumers’ reactions to the product in the future.
For example: Let us consider a researcher who is aiming to study developmental psychology. He/she may select groups of people who are of different ages but study them at one point in time. In this way, the difference between the groups will be attributed to their age differences instead of other variables that may happen over time.
Download Market Research Toolkit
Get market research trends guide, Online Surveys guide, Agile Market Research Guide & 5 Market research Template

Qualitative research methods
A qualitative approach is more appropriate when tackling some research questions. This is especially true if the researcher wishes to observe the behaviors of the target audience in-depth. The results here are in descriptive form. Qualitative research is not predictive in nature. It enables researchers to build and support their theories to advance future potential quantitative research. Qualitative research methods are used to come up with conclusions to support the theory or hypothesis under study.
- Case study: Case studies have evolved to become a valuable method for qualitative research. It is used for explaining a case of an organization or an entity. This is one of the simplest ways of conducting research because it involves an exhaustive understanding of the data collected and the interpretation of the same.
For example: For example; let’s assume that a researcher is interested in understanding how to effectively solve the problems of turnover in organizations. While exploring, he came across an organization that had high rates of turnover and was able to solve the problem by the end of the year. The researcher can study this case in detail and come up with methods that increased the chances of success for this organization.
- Observational method : When doing qualitative research, maintaining the existing records can be a valuable source of information in the future. This data can be used in new research and also provide insights for the same. Observation is one of the common aspects that is used in every method we described above. It can be systematic or naturalistic. Qualitative observation of respondents’ answers, or their behaviors in particular settings can yield enriching insights. Hence, observation in qualitative research is used to gather information about relevant characteristics that the researcher is interested in studying.
For instance, if a smartphone brand wants to see how customers react to its products in a showroom, observers may be hired to note the same. The observers can use the recorded observations to evaluate and draw inferences about the customers.
- One-on-one interview : Interviewing people of interest is one of the most common practices in qualitative research. Here, there is an in-depth personal interview carried out either face-to-face or through online mediums with one respondent at a time. This is a conversational method of gathering information and it invites the researcher with an opportunity to get a detailed response from the respondent.
For example: A one-on-one interview with an environmentalist will help to gather data on the current climate crisis in the world.
- Focus groups : Another most commonly used method in qualitative research apart from interviewing people is focus group. In this method, data is usually conducted once a researcher includes a limited number of consumers (usually ranging from 6 to 10) from the target market and forms a group.
For example: Let’s assume a researcher wants to explore what are qualities consumers value when buying a laptop. This could be the display quality, battery life, brand value, or even the color. The researcher can make a focus group of people who buy laptops regularly and understand the dynamics a consumer considers when buying electronic devices.
- Text analysis : In text analysis, researchers analyze the social life of the respondents in the study and aim to decode the actions and the words of the respondents. Hence, text analysis is distinct from other qualitative research methods as it focuses on the social life of the respondents. In the last decade or so, text analysis has become increasingly popular due to the analysis of what consumers share on social media platforms in the form of blogs, images, and other texts.
For example: Companies ask their customers to give detailed feedback on how satisfied they are with their customer support team. This data helps them make appropriate decisions to improve their team.
Sometimes researchers use a combination of methods to answer the questions. This is especially true when researchers tackle complex subject matters.
Exploratory Research Guide
Conducting exploratory research seems tricky but an effective guide can help.
Steps for conducting empirical research
Since empirical methods are based on observation and capturing experiences, it is important to plan the steps to conduct the experiment and how to analyze it. This will enable the researcher to resolve problems or obstacles which can occur during the experiment.
Step #1: Define the purpose of the research
The very first step is for the researcher to identify the area of research and the problem can be addressed by finding out ways to solve it. The researcher should come up with various questions regarding what is the problem, who will benefit from the research, how should they go about the process, etc. The researchers should explore the purpose of the research in detail.
Step #2 : Supporting theories and relevant literature
After exploring and finding out the purpose of the research, the researcher must aim to find if there are existing theories that have addressed this before. The researcher has to figure out whether any previous studies can help them support their research. During this stage of empirical study, the researcher should aim at finding all relevant literature that will help them understand the problem at hand. The researcher should also come up with his/her own set of assumptions or problem statements that they wish to explore.
Step #3: Creation of Hypothesis and measurement
If the researcher is aiming to solve a problem the problem has not been resolved efficiently in previous research, then the researcher creates his/her own problem statement. This problem statement, also called hypothesis, will be based on the questions that the researcher came up with while identifying the area of concern. The researcher can also form a hypothesis on the basis of prior research they found and studied during the literature review phase of the study.
Step #4: Methodology, research design and empirical data collection
Here the researcher has to define the strategies to be used for conducting the research. They can set up experiments in collecting data that can help them come up with probable hypotheses. On the basis of the hypotheses, researchers can decide whether they will require experimental or non-experimental methods for the conduction of the research. The research design will depend upon the field in which the research is to be conducted. The researchers will need to find parameters that can affect the validity of the research design. Researchers also need to choose appropriate methods of data collection, which in turn depends on the research question. There are many sampling methods that can be used by the researcher. Once, the data is collected, it has to be analysed.
Step #5: Data Analysis and result
Data can be analyzed either qualitatively and quantitatively. Researchers will need to decide which method they will employ depending upon the nature of the empirical data collected. Researchers can also use a combination of both for their study. On the basis of the analysis, the hypothesis will either be supported or rejected. Data analysis is the most important aspect of empirical observation.
Step #6: Conclusion
The researcher will have to collate the findings and make a report based on the empirical observations. The researcher can use previous theories and literature to support their hypothesis and lineage of findings. The researcher can also make recommendations for future research on similar issues.
Advantages of Empirical research
The advantages of empirical study are highlighted below:
- Used for authentication. Empirical study is used to authenticate previous findings of experiments and empirical observations. This research methodology makes the conducted study more authentic and accurate.
- Empirical approach is useful for understanding dynamic changes. Due to the detailed process of literature review, empirical analysis is used in helping researchers understand dynamic changes in the field. It also enables them to strategies accordingly.
- Provides a level of control . Empirical approach empowers researchers to demonstrate a level of control by allowing them to control multiple variables under study.
- Empirical methods Increase internal validity . The high level of control in the research process makes an empirical method demonstrate high internal validity.
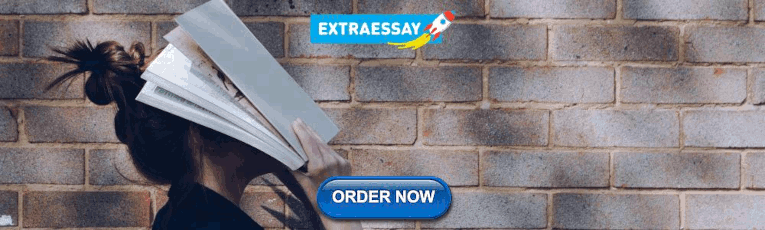
Disadvantages of Empirical research
Empirical approach is not without its limitations. Some of them include:
- Time consuming . Empirical studies are time consuming because it requires researchers to collect data through multiple sources. It also requires them to assess various parameters involved in the research.
- Empirical approach is Expensive. The researcher may have to conduct the research at different locations or environments which may be expensive.
- Difficult to acquire consent/permission. Sometimes empirical studies may be difficult to conduct due to the rules that are to be followed when conducting it.
- Data collection in the empirical approach can be a problem. Since empirical data has to be collected from different methods and sources, it can pose a problem to the researchers.
Transform your insight generation process
Create an actionable feedback collection process.
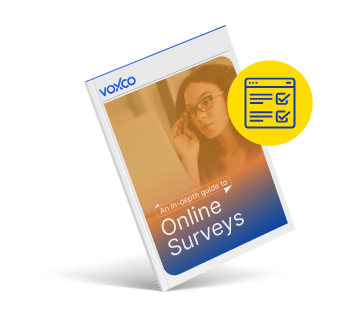
Why is there a need for empirical research?
Because most people today only believe in their experiences, empirical observation is increasingly becoming important. It is used to validate various hypotheses or refute them in the face of evidence. It also increases human knowledge and advances scientific progression.
For instance, empirical analysis is used by pharmaceutical companies to test specific drugs. This is done by administering the drug on an experimental group, while giving a placebo to the control group. This is done to prove theories about the proposed drug and check its efficacy. This is the most crucial way in which leading evidence for various drugs have been found for many years.
Empirical methods are used not just in medical science, but also in history, social science, market research, etc.
In today’s world it has become critical to conduct empirical analysis in order to support hypotheses and gather knowledge in several fields. The methods under empirical studies mentioned above help researchers to carry out research.
Explore Voxco Survey Software
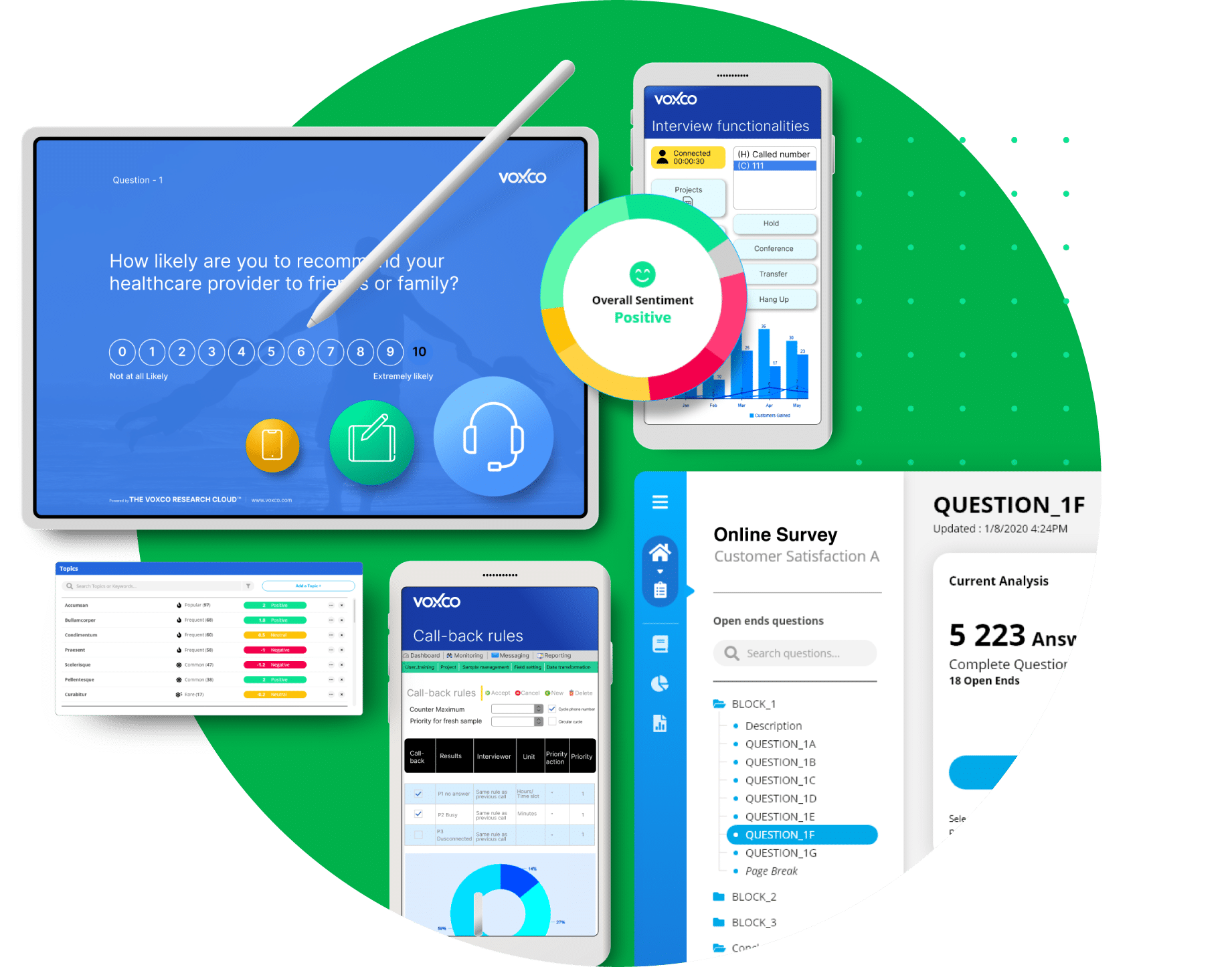
+ Omnichannel Survey Software
+ Online Survey Software
+ CATI Survey Software
+ IVR Survey Software
+ Market Research Tool
+ Customer Experience Tool
+ Product Experience Software
+ Enterprise Survey Software
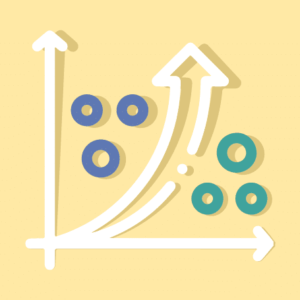
Linear regression vs logistic regression: difference and working
Distinguishing Linear Regression from Logistic Regression Get a 15-min Free consultation with our experts. Book a Demo SHARE THE ARTICLE ON Table of Contents Machine
Process Automation
Process Automation SHARE THE ARTICLE ON Share on facebook Share on twitter Share on linkedin Table of Contents Process automation speeds up or simplifies manual
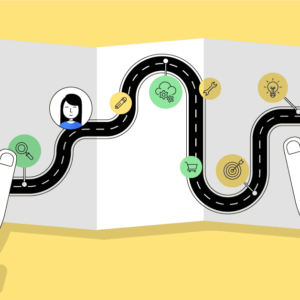
How to Create a Customer Journey Map
How to Elevate CX using Customer Journey Maps SHARE THE ARTICLE ON Table of Contents So, you don’t know what a customer journey map is
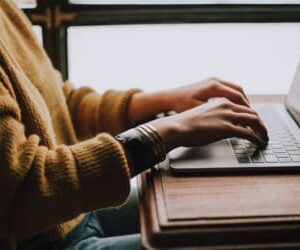
Which Survey Mode Is Best: Face-To-Face or Self-Completion Surveys?
Face-To-Face and Self-Completion Surveys: Pros, Cons & Insights Design engaging surveys & start collecting feedback. Book a quick call to get started. Book Now SHARE

How to Use Market Research Survey Software to Improve Customer Experience
How to use market research survey software to improve customer experience SHARE THE ARTICLE ON Table of Contents Kickstart your market research with a step-by-step
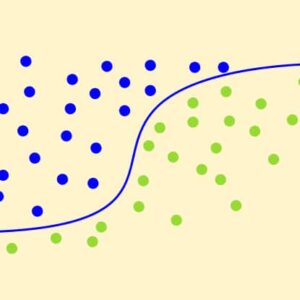
Multivariate regression: Definition, Example and steps
Multivariate regression: Definition, Example and steps SHARE THE ARTICLE ON Table of Contents What is multivariate regression? Most of the statistically analysed data does not
We use cookies in our website to give you the best browsing experience and to tailor advertising. By continuing to use our website, you give us consent to the use of cookies. Read More

An official website of the United States government
The .gov means it’s official. Federal government websites often end in .gov or .mil. Before sharing sensitive information, make sure you’re on a federal government site.
The site is secure. The https:// ensures that you are connecting to the official website and that any information you provide is encrypted and transmitted securely.
- Publications
- Account settings
Preview improvements coming to the PMC website in October 2024. Learn More or Try it out now .
- Advanced Search
- Journal List

The Neuroscience of Growth Mindset and Intrinsic Motivation
Our actions can be triggered by intentions, incentives or intrinsic values. Recent neuroscientific research has yielded some results about the growth mindset and intrinsic motivation. With the advances in neuroscience and motivational studies, there is a global need to utilize this information to inform educational practice and research. Yet, little is known about the neuroscientific interplay between growth mindset and intrinsic motivation. This paper attempts to draw on the theories of growth mindset and intrinsic motivation, together with contemporary ideas in neuroscience, outline the potential for neuroscientific research in education. It aims to shed light on the relationship between growth mindset and intrinsic motivation in terms of supporting a growth mindset to facilitate intrinsic motivation through neural responses. Recent empirical research from the educational neuroscience perspective that provides insights into the interplay between growth mindset and intrinsic motivation will also be discussed.
1. Introduction
With an emphasis on inquiry and scientific skills, students are encouraged to discover, produce and evaluate knowledge, using inquiry and scientific skills [ 1 ]. Such inquiry learning should be structured in a way that student learning is facilitated, while encouraging students to plan and conduct their own investigation. An autonomy-supportive environment facilitates autonomous learning, and fosters self-determined motivation in students [ 2 ]. Students learn to synthesize contradictory perspectives and rise to intellectual meta-levels of thinking, which is a crucial trait for the 21st-century operating environment [ 3 ]. As such, it is fundamental to nurture the young generation in becoming adaptive, self-regulated and self-determined.
In the 21st century, there has been a strong proliferation of research on growth mindset and intrinsic motivation in learning. The constructs of mindset and motivation have been important foci among educators seeking to positively impact student learning and outcomes. The underlying mechanism for students to have their own agency in finding out new knowledge is intrinsic motivation. However, much of this research has relied on quantitative approaches for assessing students’ self-reports on motivational regulations and learning outcomes [ 4 , 5 ]. Some of these quantitative findings are used to generalize across school settings. Although the multiple facets of student motivation and learning have been identified in quantitative analyses, they have not provided a detailed understanding of students’ motivational processes. Neuroscience methods may offer new insights regarding students’ motivation and learning processes.
Most neuroscience studies have focused on research related to cognitive functions, such as attention, memory and decision-making. In addition to these cognitive studies, there is also the implicit nature of mindsets that lead to the malleability of self-attributes (e.g., intelligence) [ 6 ]. Subtle feedback and messages related to growth mindset can have noticeable effects on students’ attitudes and motivation that may transfer to long-term outcomes. Likewise, human motivation is important, as it is one’s intrinsic desire to learn and obtain information. Growth mindset is the belief that intelligence can be nurtured through learning and effort, while intrinsic motivation is the volition to engage in a task for inherent satisfaction. Individuals with growth mindset believe that motivation can be nurtured, and that extrinsic motivation can be internalized (i.e., from extrinsic regulation to integrated regulation that is similar to intrinsically motivated behavior). In an integrative view, growth mindset and intrinsic motivation are important and interrelated, thus raising fundamental questions about the neural mechanisms of mindset-motivation interaction. The links among growth mindset, brain and motivation are important to academic performance. Therefore, it is important to draw on neuroscientific findings to show the way the brain is motivated, and how it learns by changing mindset (i.e., from a fixed to a growth mindset). Such intervention studies are still not common, and there is potential in these research areas.
This paper reviews the theoretical frameworks of growth mindset and intrinsic motivation, and how they are linked to neuroscientific evidence. It also reviews a number of recent neuroscience studies related to growth mindset and intrinsic motivation. It is important to survey the progress of neuroscience research on growth mindset and intrinsic motivation, as understanding the neural substrates will provide insights into human motivation and drive. Neuroscience research has the potential to support and refine models of motivation and cognitive skill. It may play a pivotal role in developing classroom interventions and understanding non-cognitive skills (e.g., mindset). Knowing the key brain regions that are associated with growth mindset and intrinsic motivation, researchers and practitioners could work together to investigate the granular processes of motivation in relation to growth mindset.
Most empirical research on growth mindset and intrinsic motivation has focused on behavioral methods and self-reports of experiences. There is little information about the internal processes of motivation at a higher level of resolution. It is, therefore, relevant and timely to examine the existing literature and empirical research that is associated with intrinsic motivation. Neuroscientific evidence has the potential to uncover new insights and refine the conceptual ideas of intrinsic motivation by articulating the granular processes of motivation that behavioral methods alone cannot afford. This paper offers recommendations for potential neuroscience research in studying growth mindset and intrinsic motivation.
2. Growth Mindset
Growth mindset is defined as a belief that construes intelligence as malleable and improvable [ 6 ]. Students with growth mindset are likely to learn by a mastery approach, embrace challenges and put in effort to learn. For instance, growth-minded individuals perceive task setbacks as a necessary part of the learning process and they “bounce back” by increasing their motivational effort [ 7 , 8 ]. One recent study on elementary students showed that leveraging an online educational game (the BrainPOP website) with in-game rewards can promote a growth mindset by directly incentivizing effort and encouraging persistence in low performing students [ 7 ]. Learners with growth mindset tend to embrace lifelong learning and the joy of incremental personal growth. In addition, they do not see their intelligence or personality as fixed traits. They will mobilize their learning resources without being defeated by the threat of failure. This paper aims to provide some insights into the cultivation of resilience and mastery in university students, preparing them to overcome challenges in the real working world.
Empirical studies have revealed that growth mindset has positive effects on student motivation and academic performance [ 9 , 10 ]. Recent research has also shown that mindset is related to student outcomes and behaviors including academic achievement, engagement, and willingness to attempt new challenges [ 11 , 12 ]. Numerous studies have shown the effects of growth mindset interventions on students’ achievement at all ages. According to Dweck [ 9 ], teaching growth mindset to junior high school students resulted in increased motivation and better academic achievement. Her findings revealed that students in the growth mindset intervention group outperformed those in the control group (who received excellent training in study skills), indicating improved learning and desire to work hard. The growth mindset intervention teaches students that intelligence is not a fixed quality [ 13 ]. Intelligence can be nurtured through challenging tasks, as intelligence grows with hard work on challenging problems. A growth mindset intervention was especially impactful with student outcomes in particular subjects such as science and mathematics [ 14 ].
An individual with a growth mindset works hard and improves without an incentive reward in mind as the outcome. The conceptualization of growth mindset is similar to that of intrinsic motivation. A learner with a growth mindset tends to self-regulate their own learning and has the propensity to cope with academic tasks. Hence, encouraging a growth mindset can improve the academic performance of college students [ 14 , 15 ] and middle school math students [ 9 ].
Most of the abovementioned empirical studies reported the utility of questionnaires or self-report measures. There is still limited neuroscientific research on the neural mechanism of growth mindset. It is, therefore, important to examine data from other means such as neuroscientific information about how the brain changes with experience of learning and how it is associated to growth mindset. The subsequent sections will discuss the neuroscientific evidence of growth mindset.
3. Intrinsic Motivation
Intrinsic motivation is inherent, as it drives the direction of an individual’s behavior and self-determination [ 16 ]. Self-determination is important in the development of beings to become more effective and refined in their reflection of ongoing experiences [ 17 ]. When students experience the inherent satisfaction of the activity itself, they will show intrinsically motivated behavior. If students are doing the activity in order to attain some reward, such as grades or social recognition, they are extrinsically motivated [ 18 ]. Students’ motivated behaviors pertaining to choice, effort and persistence in academic tasks correspond directly with their level of intrinsic motivation [ 19 , 20 ].
Numerous studies have examined the effects of intrinsic motivation, including the adaptive consequences for individuals such as exposing them to novel situations and developing their diverse competencies to cope with unforeseen circumstances [ 21 ]. In addition, intrinsic motivation is the propensity for individuals to learn about new subjects and to differentiate their interests, thereby fostering a sense of purpose and meaning [ 22 ]. Recent empirical findings have shown that intrinsic motivation is a key factor in academic achievement [ 23 ] and pursuit of interest [ 24 ], thus fostering learning and growth.
Dopamine is the predominant neurotransmitter in the brain that aids in controlling the brain’s reward and pleasure centers, as well as motivated and emotional behaviors [ 25 ]. Dopamine neurons that are excited by unexpected reward events project to the striatum, cortex, limbic system and hypothalamus, thus affecting physiological functions and motivated behaviors. Dopamine is considered a key substrate of intrinsic motivation, thus promoting attentiveness and behavioral engagement [ 25 ]. For instance, participants were likely to voluntarily engage with the task during a free-choice time period [ 26 ] or a self-determined choice condition [ 27 ]. These consistent findings indicate that an enhanced activity within the dopaminergic value system whereby perceived autonomy support promotes intrinsic motivation. As such, learning is a neural process that requires the reinforcement of synaptic functioning and is strongly mediated by dopamine and attentional gain in the frontal cortex [ 28 ]. Positive and negative affect will also strengthen or weaken the learner’s intrinsic motivation in a particular subject, thus influencing the attitude towards that subject.
Over the past few decades, behavioral evidence has established the importance of intrinsic motivation and how it impacts one’s learning. However, our understanding of the underlying mechanism of intrinsic motivation is still in its infancy, and it is unclear how one’s intrinsic motivation progresses or changes over time. More evidence is needed to establish the mechanism of intrinsic motivation at a granular level. The recommendation is to include neuroscientific evidence to track and understand which aspects of one’s learning progress determine intrinsic motivation, complementing the existing behavioral evidence. An approach of the neuroscience method is to foster intrinsically motivated behaviors based on task complexity in various contexts, thereby addressing intrinsic motivation through different forms of exploration. The following sections will discuss in detail the neuroscience methods and neuroscientific evidence of intrinsic motivation.
4. Neuroscience Methods
The main neuroscience methods that have been applied in motivation studies are electroencephalography (EEG) and functional magnetic resonance imaging (fMRI). Such neuroscientific research is still considered novel, as most motivational studies have focused on behavioral methods. Both neuroscientific techniques are non-invasive procedures for measuring brain activity. The key difference is fMRI has a higher spatial resolution than EEG, whereas EEG has a better temporal resolution than fMRI.
Neuroscience methods (e.g., fMRI) could provide insights into neural substrates of growth mindset and intrinsic motivation. We could measure the learner’s brain activity and neural responses to a specific task in relation to internal processes of motivation. For instance, intrinsic motivation could be assessed by an experimental task or free-choice behavior measures.
The use of neuroscientific techniques enables us to focus on the learning process rather than the learning outcomes [ 29 ]. The neuroimaging findings offer an understanding of the brain, indicating the specific areas of brain activation which could in turn correlate with the behavioral results. As such, neuroimaging findings might support the self-reported data and explore brain regions with neural activation in relation to changes in performance during an online activity.
5. Neural Correlates of Growth Mindset and Intrinsic Motivation
There is a small body of existing growth mindset studies using neuroscience methods. The study by Moser et al. [ 30 ] suggested that individuals with a growth mindset are receptive to corrective feedback, exhibiting a higher Pe (error positivity) waveform response, which is correlated with a heightened awareness of and attention to mistakes. Enhanced Pe amplitude was associated with enhanced attention to corrective feedback following errors and subsequent error correction. Individuals with growth mindset are likely to have heightened awareness of and attention to errors. In addition, growth-minded individuals may neutralize the affective response to negative feedback, which could be indicated by neural activation. Anterior cingulate cortex (ACC) is the region of frontal midline cortex that is related to learning and control [ 31 ]. A recent study [ 32 ] found that growth mindset was related to both ventral and dorsal striatal connectivity with dorsal ACC. Dorsal ACC and dorsolateral prefrontal cortex (DLPFC) are critical to error-monitoring and behavioral adaptation. Growth mindset was strongly associated with dorsal and ventral striatal connectivity, as well as DLPFC. Learners with growth mindset are efficient in error-monitoring and receptive to corrective feedback. Hence, growth mindset has the potential to encourage intrinsically motivated behaviors in schools and promote lifelong learning.
Neuroscientific evidence has shown that ACC is associated to cognitive control and motivation [ 31 ]. Neural correlates revealed that dopamine is critical for motivation and cognitive control, with motivation-cognition interactions between midbrain regions and lateral frontal cortex [ 33 ]. Cognitive control is influenced by reward motivation. Participants were assigned to three levels of cognitive controls (low, mid and high). Different beneficial effects of reward (high versus low) were exhibited. Participants with high versus low reward anticipation showed increased activity in the medial and lateral frontal cortex. Brain activity was also stronger at the low level of cognitive control than mid and high levels. These findings demonstrated that motivation plays an important role in the cognitive control. In addition, high-level control tasks may demand an enhancing effect of motivation.
A recent EEG study [ 34 ] showed that school children with growth mindset endorsement performed with higher accuracy after mistakes (i.e., post-error accuracy). The event-related potential (ERP), which is a measure of brain response due to the result of error and correct trials, revealed that Pe amplitude difference was largest at site Pz (i.e., midline parietal). Together with the behavioral data, correlational analyses showed that having a higher growth mindset was associated with a larger Pe difference. Students with attentional resources are able to remember their mistakes and able to make sense of their mistakes, thus correcting themselves during the learning process. Students do not like to take risks that show their weaknesses, such as making mistakes [ 35 ]. However, with growth mindset endorsement, students are not afraid to make mistakes, as they have the ability to learn with post-error accuracy. Hence, growth-minded students will be resilient and self-regulated when faced with obstacles or challenges during their learning process.
Little is known about the interplay between neural responses and intrinsic motivation. Intrinsically motivated action can be characterized by an individual’s engagement in behavior for one’s own sake, with free-choice time on a task [ 36 ]. An empirical study measured intrinsic motivation by examining a network of brain regions as the participants spent free-choice time on a word problem task [ 37 ]. Using fMRI, a network of brain regions revealed diminished task-related activity, predicting subsequent increased intrinsic motivation. The neuroimaging data suggest that decreased activation of neural cognitive control is associated with increased intrinsic motivation, thus extending one’s task engagement. Another recent study by Lee and Reeve [ 38 ] examined the neural substrates of intrinsic motivation during task performance. Their findings showed activated anterior insular cortex (AIC; a limbic-related cortex region) when students performed intrinsically motivated tasks. These neural findings are consistent with the concept of intrinsic motivation in terms of pursuit and interest satisfaction as intrinsic rewards. Based on these findings, it was concluded that AIC activity and its functional interactions are linked to an intrinsic-motivation neural system [ 38 ].
Two recent motivation studies used free-choice measures, such as a stop-watch (SW) game, as an experimental task to assess participants’ intrinsic motivation [ 39 , 40 ]. A traditional SW game includes a stopwatch that starts automatically, and the player tries to stop the watch at a specific time. Experimental stimuli were presented on the computer screen and participants were required to use the keypad to complete the SW tasks. It is interesting to note the relationship between the optimal challenge condition and intrinsic motivation using EEG [ 39 ]. Students performed better when they felt optimally challenged, and had enhanced intrinsic motivation in the game experiment. Stimulus-preceding negativity (SPN) is considered to be an electrophysiological indicator of motivation level. The EEG findings showed a larger SPN during the feedback anticipation period of the near miss condition than in the complete defeat condition, suggesting that participants were more intrinsically motivated to win in close games [ 39 ]. For the second study, fMRI was used to explore the degree of enjoyment for the preference levels of SW game [ 40 ]. It was found that participants had enhanced intrinsic motivation when they played the SW game with the action-outcome contingency condition. The fMRI findings revealed significant activation in the regions of the mid brain and ventral striatum in the action-outcome contingency condition, indicating that the intrinsic value of an action and achieving success. These two studies suggest that neuroscience methods are used to assess individuals’ intrinsic motivation using a free-choice experiment, such as a SW game. However, using game elements and design may have implications for authentic learning programs. Using the game approach, students may have enhanced intrinsic motivation for doing the activities in a gaming format or platform. Adopting the game approach and translating such motivation-enhancing elements into classrooms may seem challenging and time-consuming. Such experimental tasks are usually carried out in a closed environment, such as in a controlled laboratory setting within the fMRI facility.
Intrinsic motivation is associated with sensitivity of feedback processing in the striatum [ 41 ]. The striatum plays a key role in reinforcing learning as it receives input from midbrain dopamine neurons and produces adaptive behaviors. Striatum activity is associated with reward processing, indicating that an intrinsically motivated task could foster the individual’s intrinsic motivation. For instance, feedback-related responses in the striatum can potentially promote or undermine intrinsic motivation of a desired behavior. Positive feedback was viewed as a rewarding outcome, and highly motivated subjects could attune to the feedback despite of fatigue through the study [ 41 ]. Performance-feedback may have affective salient response to striatum and produce a motivated behavior. A study by Lee [ 42 ] showed that intrinsic motivation was related to the AIC that is known to be associated with the sense of agency, while extrinsic motivation was associated with posterior parietal regions (e.g., posterior cingulate cortex, angular gyrus). The type of task also plays a very important role in activating the AIC. Lee [ 42 ] also found that interesting tasks activated the AIC and ventral striatum (i.e., brain region for reward processing), but not uninteresting tasks. AIC relates to the satisfaction of intrinsic need, whereas ventral striatum relates to the feeling of reward. His findings suggest that AIC and ventral striatum activations are associated with intrinsic motivation.
Intrinsic motivation is difficult to measure in an objective manner. In order to track one’s intrinsic motivation, it requires one to perform an experimental task over time. For instance, one’s brain activity can be tracked during the process of performing an intrinsically motivated or optimally challenged task. Together with behavioral measures, contemporary methods such as fMRI can be used to track the changes in intrinsic motivation during a free-choice activity.
6. The Neuroscientific Interplay between Growth Mindset and Intrinsic Motivation
Based on the abovementioned empirical findings, there is a distinctive neuroscientific interplay between growth mindset and intrinsic motivation. EEG findings could not directly show the brain regions that are related to mindset and motivation. Compared to the EEG, which is based on brain waveforms, fMRI is a better method for showing insights into the brain regions that are associated with growth mindset and intrinsic motivation. It is interesting to note that growth mindset is mainly associated with the dorsal regions of the brain, whereas intrinsic motivation is associated with the mid-brain regions. The common brain areas that are related to both growth mindset and intrinsic motivation are ACC and ventral striatum. Knowing the behavioral correlates for these two brain regions, potential research could investigate the neural correlates of growth mindset and intrinsic motivation. This brings us a step closer to understand the neural mechanism between growth mindset and intrinsic motivation. Below is a table that highlights the neuroscientific evidence of growth mindset and intrinsic motivation in relation to cognition. The behavioral correlate for the brain region is included in parentheses (see Table 1 ).
Neuroscientific evidence of growth mindset and intrinsic motivation.
Growth mindset relates to brain processes, and brain processes relate to motivated behaviors. Likewise, motivated behaviors can affect cognition as motivation shapes what and how people think [ 43 ]. As such, individuals’ goals and needs may be exemplified when they steer their thinking towards desired outcomes. Research has shown that growth mindset has an impact on children’s behavior, particularly in terms of effort, motivation and resilience [ 12 , 44 ]. By understanding the underlying mechanism of intrinsic motivation, teachers are able to guide students in applying the relevant self-regulatory strategies at school. When individuals have intrinsic motivation for performing a task at work or school, their work or educational performance will improve [ 45 , 46 ]. With the inculcation of growth mindset, individuals will perceive the intrinsic value of a given task and self-regulate their behaviors to perform the task. Through internalization, individuals will generate intrinsically motivated behaviors at work or school.
As our brain is plastic, it is able to undergo reorganization and development. Brain plasticity or neuroplasticity refers to the ability of our brain to change throughout our life. It is thereby important to understand how our brain changes if we undergo growth mindset intervention and whether there are changes in our intrinsic motivation as well. This phenomenon is yet to be explored in educational research. It is thus an avenue worth pursuing for educators who hope to make the best of their students with regard to learning and personal growth. Such educational neuroscience research may impact teaching and learning, thus providing a better understanding of the neuroscientific interplay between growth mindset and intrinsic motivation. Future educational neuroscience research may include classroom interventions such as a growth mindset induction and how it affects the neuroscience of intrinsic motivation.
7. Future Directions
The principal intent of this paper is to highlight a potential educational neuroscience research in areas of growth mindset and intrinsic motivation. Although there are some empirical studies on mindset and motivation, the neuroscience of intrinsic motivation is still unclear and at its infancy. There are also limited neuroscientific studies on students’ motivation and learning. As educational neuroscience research looks promising in the near future, we should be aware of the potential integration between neuroscience methods and behavioral measures. For successful intervention studies, there are some considerations that need to be warranted.
First, educators should design a task that has intrinsic value for students to be engaged in doing. For instance, an interesting task will instill curiosity into students, when compared to an uninteresting one. Inculcating the value of doing the task or task value will definitely stimulate the students’ interest. Second, teachers should provide the autonomy or choice for students. Autonomy or the agency of learning is the key substrate to intrinsic motivation [ 17 ]. Research has shown that autonomy is the strongest predictor of intrinsic motivation [ 47 ]. Autonomy is considered the self-endorsement of actions, whereby individuals feel less coerced and they generate autonomous behavior at work or school. In the same vein, choice is the opportunity for individuals to decide and exert control over the situation. A recent study found that the provision of choice, however trivial or inconsequential, might also increase an individual’s intrinsic motivation [ 48 ]. The researchers used behavioral and electrophysiological (i.e., electroencephalogram) evidence to explain the importance of need satisfaction for autonomy to enhance one’s intrinsic motivation toward the task.
Third and finally, performance-related feedback could influence intrinsic motivation [ 41 ]. Participants are likely to perceive their performance on the task differently based on the type of performance-related feedback. For instance, positive feedback may enhance one’s intrinsic motivation, while negative feedback may undermine one’s motivation. In addition, the frequency of performance-related feedback may affect one’s neural processing (i.e., posterior cingulate cortex) in supporting task performance. There were enhanced activity of posterior cingulate cortex and performance gains after the performance-feedback manipulation. This shows that posterior cingulate cortex might facilitate the learning of a task [ 41 ]. The current level of a learner’s intrinsic motivation may also influence the way he or she processes the performance-related feedback. It is still not fully clear how the nature of performance-feedback could affect an individual’s feedback processing. Perhaps neuroscience methods could provide some insights into this area of research.
Based on the neuroscientific evidence, there is an undermining effect of monetary reward on intrinsic motivation; that is, one’s intrinsic motivation is undermined when extrinsic reward is no longer promised [ 26 ]. Neuroscience findings suggest that there are connections between the striatum and the prefrontal cortex in determining the outcome; decreased activation of the striatum and midbrain when the subjects do not get the task value, as well as decreased activation of the lateral prefrontal cortex (LPFC) when they are not motivated to show cognitive engagement with the task. Since growth mindset is a belief system that favors hard work and performance monitoring [ 32 ], a learner’s subjective belief in determining the outcome may modulate activity of the striatum, in response to cognitive feedback that nurtures growth mindset. Hence, neuroscientific evidence may provide insights into the learning and motivational processes that could be helpful for teachers and practitioners in improving their learning and teaching practices, thus supporting student learning and motivation.
8. Concluding Remarks
This paper reviewed the recent empirical neuroscientific studies on growth mindset and intrinsic motivation. Research in these areas is still in its infancy. This paper attempted to provide an overview of the underlying mechanism between growth mindset and intrinsic motivation. Educating students about growth mindset and how they can improve their learning experience is a step toward increased intrinsic motivation in our society. From a personal perspective, intrinsic motivation is the key substrate to learning and development. The promotion of a growth mindset can nurture individuals to learn as they understand that intelligence is malleable. It is important that, as teachers, we show our students the value and importance of learning at schools. With a growth mindset, students will learn with a positive attitude, and they will identify the importance of the contents. Teachers should also embrace a growth mindset such that they will understand the importance of providing autonomy over student learning to enhance self-regulation. As such, students will be more motivated to learn subjects at school, rather than relying on the presumption that students will be interested in learning. This preliminary review paper offers a useful road map for identifying the areas that need to be addressed in neuroscientific research related to growth mindset and intrinsic motivation. However, this paper did not discuss the potential roles of socio-demographic variables and personality traits on growth mindset and intrinsic motivation. Future research will benefit from the continued development of neuroscientific evidence to connect the substantial behavioral evidence of these variables and traits associated with growth mindset and intrinsic motivation.
Acknowledgments
Thanks to all reviewers who contributed to improving the manuscript.
Conflicts of Interest
The author declares no conflict of interest.
Thank you for visiting nature.com. You are using a browser version with limited support for CSS. To obtain the best experience, we recommend you use a more up to date browser (or turn off compatibility mode in Internet Explorer). In the meantime, to ensure continued support, we are displaying the site without styles and JavaScript.
- View all journals
- My Account Login
- Explore content
- About the journal
- Publish with us
- Sign up for alerts
- Open access
- Published: 15 March 2024
A study on the influencing factors of corporate digital transformation: empirical evidence from Chinese listed companies
- Xu Zhao 1 , 2 ,
- Qi-an Chen 2 ,
- Haitao Zhang 3 ,
- Pengyu Chen 1 &
- Shen Chen 1
Scientific Reports volume 14 , Article number: 6243 ( 2024 ) Cite this article
138 Accesses
Metrics details
- Human behaviour
- Socioeconomic scenarios
- Sustainability
In an era where digital technology is reshaping business landscapes, understanding the factors that drive corporate digital transformation is essential. In this paper we explore these influencing factors, focusing on Chinese A-share listed companies from 2007 to 2021. Our approach involved a comprehensive analysis of multiple variables through regression techniques to determine their impact on digital transformation. The findings reveal the drive for reform in the digital transformation endeavours of enterprises. Notably, companies with higher gearing, overhead, and accounts receivable ratios exhibit a stronger inclination towards digital transformation. Conversely, enterprises in monopolistic industries and those at the inception stage of their life cycle show less propensity for such transformation. The findings of this research not only shed light on the strategic decisions behind digital transformation in response to financial and competitive challenges but also provide actionable insights for policymakers and business strategists. This study underscores the importance of contextualizing digital transformation efforts within the unique framework of industry characteristics and company development phases.
Similar content being viewed by others
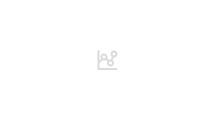
Study on the impact of digital transformation on the innovation potential based on evidence from Chinese listed companies
Xu Zhao, Qi-an Chen, … Haitao Zhang
Digital transformation, entrepreneurship, and disruptive innovation: evidence of corporate digitalization in China from 2010 to 2021
Yuan Wu & Ziwei Li
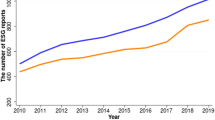
Does corporate digital transformation restrain ESG decoupling? Evidence from China
Xiangyu Chen, Peng Wan, … Yu Yang
Introduction
At the dawn of the twenty-first century, a digital revolution, fuelled by rapid advancements in Internet technology, has swept across the globe, reshaping the socio-economic fabric and heralding a new era of industrial and technological transformation. This digital wave has not only sparked dynamic economic activities but also profoundly influenced the innovative locus of enterprises worldwide. Within this context, China’s digital economy has witnessed a revolution. According to the relevant data in the "2022 China Digital Economy White Paper", the scale of China's digital economy reached 45.5 trillion yuan by 2021, with a nominal growth of 16.2% year-on-year, higher than the nominal GDP growth rate of 3.4 percentage points in the same period, and accounting for 39.8% of the GDP, all of which manifest that the position of the digital economy in the national economy has become more stable and its supporting role more obvious. As a result, digital transformation has become a national development strategy, and the digital economy is developing at an unprecedented speed, with diverse and far-reaching impacts, which will bring profound changes to the production, life, and business behaviour of the current society. The Chinese government is proactively leading and encouraging the digitalization of small and medium-sized enterprises (SMEs), with supportive policies prompting businesses to embark on this transformation journey. For instance, Zhejiang Province, designated as a national pioneer in advancing the digital economy, has enthusiastically heeded the central government's directive. Large enterprises in the region are prioritizing innovation and growth, aiding smaller companies in their transition towards digitalization. As a result, the province has witnessed a continuous increase in its GDP, injecting dynamism into its economic expansion. Moreover, the Zhejiang provincial government offers assistance to enterprises seeking to transform, providing services such as talent development and vocational guidance 1 . It will become an important driving force that can reshape the core competitiveness of enterprises, improve the quality and efficiency of their operations, transform dynamic energy sources, and play a crucial role in leading China's economy to new economic growth points 2 .
However, the journey towards digital transformation is not without its challenges. The onset of the COVID-19 pandemic in 2020 laid bare the vulnerabilities of traditional business models, placing unprecedented pressure on companies striving for survival. In such a critical situation, the development of digital technologies and the digital economy has largely helped companies to reduce the risks associated with market uncertainty. Data from the National Development and Reform Commission 3 illustrates this impact, showing substantial improvements in business and administrative efficiency through digital adoption. Yet, the digital transformation landscape is diverse and uneven across sectors. The results of the "Accenture 2021 China Digital Transformation Index" 4 , a survey of 398 companies, show that traditional retailers have consistent expectations for increased digital investment; technology-intensive industries such as electronics, high-tech, automotive, and construction have a high starting point when it comes to creating digital opportunities and are leading the way in digital transformation. In contrast, only 4% of companies in the chemical and building materials industries plan to increase their investment budgets for digital transformation in the next 1–2 years. The survey also reveals that even within the same industry different companies have different intentions for digital investment. For example, in the logistics industry, emerging courier companies are more willing to make digital investments because they have seen the benefits of digitalization in their past operations. The more traditional manpower-dependent transportation companies will be relatively less willing to invest in the face of digital transformation, and companies need to protect their capital by compressing investment costs. Such disparities necessitate an examination of the factors influencing digital transformation, taking into account the unique developmental trajectories and sector-specific challenges faced by enterprises.
Recognizing these complexities, in this paper we delve into an empirical exploration of the factors driving digital transformation in enterprises. Utilizing the Chinese capital market as our canvas, we examine A-share listed companies through corporate characteristics, governance, international openness, life cycle, and industry competition. China's A-shares include a wide range of industries, and during the time period selected for this study, a total of 84 industries of Chinese A-share companies were included in the statistics, 70 of which are included in this study (about 83.3% of total industries), as shown in the table below (Table 1 ).
In addition to this, China's A-share companies consist of three main sectors: private, state-owned, and foreign companies, all of which are covered in this study, with the relevant ratios as shown in the table below (Table 2 ).
The aim of this research is to offer actionable insights for companies navigating the digital era, guiding them in refining their digital strategies to effectively harness the opportunities and tackle the challenges presented by the digital economy. Furthermore, through our findings, we aim to contribute valuable policy recommendations, supporting the sustainable and healthy growth of China's real economy in this digital epoch.
Literature review
The meaning of enterprise digital transformation.
At the core of digital transformation is the use of digital technologies to improve the existing organizational model of business management, fill the "data gaps" between different departments of the company, and enable the redesign of production and operation structures and management models, thus improving the efficiency of resource allocation and innovating management models 5 . For traditional companies, digital transformation means integrating digital technologies into different aspects of production and operations. This is evident in the case of China Huashi Enterprises Co., Ltd., which incorporated the "Shanjian Cloud" digital platform into its construction processes, fundamentally altering its approach to project management and operational efficiency.
The amplifying effect of digital technologies on economic development is leveraged to adapt the company's vision, strategy, organizational structure, capabilities, culture, and processes to the rapidly changing digital environment, as demonstrated by Xuzhou Construction Machinery Group Co. Ltd. (XCMG). Through its digital transformation, initiated with an SAP ERP project in 2009, XCMG has evolved into a digital platform serving external enterprises 6 , showcasing a complete change into a digital enterprise 7 .
Digital transformation is a systematic process to improve the spatio-temporal distribution of technology, capital, human resources, and materials through efficient data flows. This process also mitigates the impact of environmental uncertainty on the enterprise, as seen in the broader trend of Chinese companies embracing digital transformation by deploying cloud computing (CC), artificial intelligence (AI), and big data (BD) 8 .
The digital transformation process is characterized by "uninterrupted" and "break-and-build" innovation, which requires companies to move away from traditional business management models. This shift places higher demands on changes in organizational structure and input movement forms 9 . It follows that the TOE theoretical framework equally applies to the study of which factors influence the adoption and the advancement of digital transformation in firms. On this basis, in this paper we combine existing research results and the actual situation in China. We focus on variables under two levels, specifically organizational and environmental, to further explore how they affect the degree of digital transformation of firms. More specifically, at the corporate level, the main focus is on internal factors, i.e., firm characteristics and degree of internationalization; at the environmental level, the emphasis is on the role of industry competition from the external perspective.
Drivers of digital transformation
The drivers of digital transformation can be understood as the reasons why companies undergo digital transformation, and the study of these drivers can help companies better carry out digital transformation by enabling them to understand the difficulties they face when undergoing such transformation. Hence, the study of digital transformation drivers is a very important topic, and scholars have studied both internal and external drivers.
In their analysis of the internal drivers of digital transformation of enterprises, Bhattacharya et al. 10 argues that enterprises in different life cycles have different levels of willingness to engage in digital transformation, and therefore see the life of the enterprise as one of the internal drivers of enterprises' digital transformation. Zhang 11 believes that the main reason companies want to undergo digital transformation is because they want to better respond to market demand and are committed to reduce their production costs and increase productivity. Globally renowned enterprises, such as Microsoft and Alibaba, have made use of digital technology to carry out digital and intelligent transformation in all aspects of enterprise management, production, operation, and marketing, thereby facilitating the rapid development of enterprises. Wang and Chen 12 found that in the face of the expanding scale of enterprises, a lot of time and energy are wasted in the process of information transfer between departments, and digital transformation can effectively solve the problem of poor information transfer, so the initial purpose of digital transformation for many enterprises is to solve the problem of information transfer between internal organizations. For example, Schneider Electric opened up the digital technology and data silos scattered all over the enterprise, integrated the dispersed digital technology with its own operation technology, enabled the digital technology to empower the operation technology, and gradually realised digital connectivity and data interconnection in the aspects of enterprise production and operation, management, and business 13 .
In their analysis of the external drivers of enterprise digital transformation, Zhao 14 points out that along with the development of shared technologies, which has played a driving role in the innovation of enterprise digital transformation, the deployment of new generation shared technologies is the key to the digital transformation of enterprises. Wang 15 considered the impact of specific environmental factors on enterprise digital transformation and found that in the face of the COVID-19 pandemic, under the influence of changing market demand, enterprises that carry out digital transformation can better face the complex market situation, so during the pandemic many enterprises accelerated the pace of digital transformation. In 2020, Yintai Department Store became a department store "in the cloud", allowing consumers to "shop in the cloud" through the Meow Street App and Taobao Live Streaming Room, and making itself the world's first 24-h "new retail" department store, the first "new retail" department store in the world to stay open 24 h a day. Thanks to its early digital transformation layout, even in 2020, when the pandemic hit hard, the sales performance of Yintai Department Store showed a counter-trend growth 16 . This shows that changes in the market environment can be the main driving force for digital transformation.
In summary, we believe that the drivers of enterprise digital transformation can be divided into two aspects, which are the internal drivers and the external drivers of enterprises; external drivers include the needs of market competition, the push-back mechanism of clients and the leading role of technological development, while internal drivers encompass the life cycle of the enterprise, the financial situation, and the organizational situation of the enterprise.
Theoretical mechanisms
The toe framework and its application.
The TOE (Technology, Organization, Environment) framework, introduced by Tornatzky and Fleischer in 1990, serves as a fundamental model that offers a comprehensive perspective for examining how firms adopt innovative technologies. It categorizes the influencing factors into three critical dimensions: technology, organization, and environment. Technological factors refer to existing technologies and technologies that have not yet been introduced into the market by the enterprise 17 ; organisational factors refer to some organisational characteristics related to resource utilisation and adoption, including organisational size and scope, characteristics of the management structure, and other relevant resources available within the organisation 18 ; and environmental factors refer to the macro-environment in which the enterprise is situated, including the governmental environment, the macro-environment, the industry environment, and the intensity of competition 19 . This framework has been widely applied in research to explore the complex dynamics of technological innovation and adoption across various industries and geographical regions.
For instance, Oliveira and Martins 20 utilized the TOE framework to delve into ERP adoption in European firms, revealing the critical roles of technological benefits, organizational readiness, and the competitive environment in facilitating adoption. Another Lin 21 application in Taiwan's industries examined cloud computing adoption, pointing out key drivers such as technological compatibility, organizational agility, and the regulatory environment. These studies highlight the TOE framework's capability to provide valuable methodological insights and identify relevant factors that drive or hinder technology adoption in different contexts.
The choice to employ the TOE framework for investigating digital transformation in Chinese-listed companies is supported by its proven adaptability and depth of analysis in these examples. Despite critiques regarding its potential oversimplification of the adoption process and the challenge of capturing the whole socio-technical interplay, the successful application of the TOE framework in the studies showcases how careful operationalization of its dimensions and integration with other theoretical perspectives can yield insightful conclusions. Therefore, this study aims to adapt and extend the TOE framework to the context of digital transformation among Chinese listed companies.
Corporate governance theory
Corporate governance theory, originating in the United Kingdom and rapidly developed in the United States, has branched into various research directions, with "shareholder primacy theory" and "stakeholder theory" being the most recognized. The shareholder primacy theory views the maximization of shareholders' interests as the primary purpose of a business. This theory posits that shareholders, as the company's owners, promote a stable "capital-employed labor" governance model, emphasizing the importance of focusing on shareholder value as a guiding principle for corporate governance 22 . In this model, corporate governance mechanisms are designed to ensure that managers act in the best interests of shareholders, thereby aligning management actions with shareholder goals.
In contrast, stakeholder theory, diverging from shareholder primacy, thinks that the company is a responsible entity whose operational goals should encompass not only shareholder interests but also social responsibility and stability 23 . This perspective broadens the scope of corporate governance by advocating for considering a more comprehensive range of interests beyond those of shareholders alone. According to Chen and Zheng 24 , stakeholder theory asserts that stakeholders' involvement impacts company development, enabling them to share in the firm's control and influence governance decisions. This implies a governance framework that integrates diverse interests, promoting balanced decision-making that accounts for the needs of all stakeholders.
An organization's equity structure and ownership can measure the corporate governance dimension. With the emergence of the information economy, principal-agent theory has gained prominence, advocating for the separation of ownership and management in companies. This theory, as described by Shi, Connelly, and Hoskisson 25 , clarifies the relationship between principals (shareholders) and agents (management). It highlights the governance challenges associated with this separation, including potential conflicts of interest between owners and managers. The principals elect a board of directors who, in turn, appoint management to make operational decisions, establishing a governance mechanism to oversee management actions and ensure they align with shareholder interests.
Li and Li 26 suggest that the management expense ratio serves as a metric for principal-agent costs, capturing the extent of agency costs, such as inappropriate managerial consumption and on-the-job expenses. This ratio is pivotal in assessing the effectiveness and efficiency of corporate governance, particularly in principal-agent dynamics. It provides an empirical measure to evaluate how well the governance structure mitigates agency problems, ensuring that management acts in the shareholders' best interests while balancing other stakeholders' needs.
The role of corporate governance theory in this context is to provide a conceptual framework for understanding and addressing the complexities of managing and governing corporations. By delineating the rights, roles, and responsibilities of different actors within the corporate structure, these theories help craft governance mechanisms that aim to reduce conflicts, enhance accountability, and promote sustainable business practices. In the digital transformation era, these governance theories are particularly relevant as they offer insights into how companies can navigate innovation and change challenges, ensuring that governance structures support strategic objectives while maintaining a commitment to ethical standards and stakeholder engagement.
Factors influencing the digital transformation and research hypothesis
Among the existing studies, there is a rich discussion around the role of enterprise digital transformation outcomes, whereas there is still some room for exploring the areas of their driving mechanisms and influencing factors. Currently, domestic scholars have conducted in-depth studies from the perspectives of transformation strategy 27 , audit 28 , and social responsibility 29 based on the digital transformation practices of Chinese enterprises, while the exploration of the influencing factors of enterprise digital transformation is relatively underdeveloped and rarely approached from the economic and technological perspectives, which is not conducive to the complete revelation of the logic of corporate decision-making in the digital economy era, and cannot clarify the behavioural mechanism of important corporate strategies. Although the central government of China has issued a series of construction standards and improved the top-level design for the digital development of the real economy, the digital transformation of enterprises is still affected by various factors such as enterprise characteristics, corporate governance, internationalization (degree of openness), life cycle, and industry competition due to their different actual operating conditions.
In order to better analyse the influencing factors of enterprise digital transformation, in this paper we start from the organizational level under the TOE framework, and conduct research from three perspectives: enterprise characteristics, corporate governance, and life cycle. More specifically: enterprise characteristics are further refined into six specific variables: enterprise size, capital structure, accounts receivable ratio, management expense ratio, profitability, and revenue share of overseas business; based on corporate governance theory, two variables, equity structure and ownership, are selected; in terms of life cycle, enterprise life cycle and age are taken as two variables; at the environmental level of TOE, the focus is on the impact of industry competition, so the selection of the Herfindahl–Hirschman Index (HHI) index as a variable.
Enterprise size
From the perspective of enterprises, promoting digital transformation requires a large amount of capital for technology investment, which also generates a huge scale of sunk costs. Digital transformation requires the support of the latest digital technologies such as Big Data (BD), Cloud Computing (CC), Artificial Intelligence (AI), and Blockchain, which will inevitably generate a large amount of investment in research and development, and only sufficient funds can guarantee the stable operation of enterprises in their digital transformation. Compared with small and medium-sized enterprises (SMEs), large enterprises have larger capital and richer resources, and their own information technology expertise is relatively high, so they have more advantages in digital transformation. In addition, because digital transformation involves more digital technology supply, and has certain requirements for enterprise-related infrastructure, enterprises need to have higher risk tolerance to be able to quickly complete digital transformation. SMEs already have the natural disadvantages of small scale and weak risk resistance, and with their generally single business model and limited financing channels, they will have difficulty coping with the huge cost pressure brought about by digital transformation in the opposite direction. At the same time, digital transformation requires large investments in market development and raw material procurement, and specialized networks, equipment, and information systems as the infrastructure for transformation. However, since most SMEs lack awareness of digital transformation, the internal digital infrastructure of these enterprises is often not sound enough, which means that SMEs need to spend a significant amount of money for the procurement of related equipment and facilities to improve digital transformation, which will certainly increase the cost of digital transformation of SMEs, and even seriously affect their security and possibly even survival. The larger enterprises, on the other hand, can recognize the necessity of digital transformation due to their understanding of the basic principles of digital transformation in the process of daily development, and their own capabilities and resources are sufficient to support digital transformation.
Bai et al. 30 empirically concludes that at the organisational level, firm size, board independence and availability of finance have a significantly positive impact on digital transformation of firms, suggesting that expanding the size of the firm is conducive to facilitating digital transformation of firms. Based on this, we propose:
H1: The size of an enterprise is proportional to its degree of digital transformation.
Capital structure
The financing problem has been an important obstacle to business development and reducing the cost of capital has been considered as a business development goal; according to Wen et al. 31 , the increase in business risk leads to additional financing needs and high leverage, which generates more interest costs. Moreover, external investors, especially bank investors, are aware of such problems, and in the face of high risk, will inevitably demand higher returns that match the potential risk, which will inevitably increase the financing costs of firms. It follows that highly leveraged firms will have higher financing costs than existing non-highly leveraged firms. Digital transformation can improve information processing capabilities and market expectations, further optimizing and improving firms' investment and financing behaviour, laying the foundation for lower financing costs.
First, digital transformation reduces information asymmetry, reinforces positive market expectations, and reduces financing costs. The strengths of digitally transformed organizations in the areas of information acquisition, storage, transmission, and identification significantly improve their ability to process large amounts of information internally and externally. On the one hand, companies can use their established information data experience to improve their operations and gain insight into market needs through data processing. On the other hand, Che, Duan, and Wang 32 argue that companies that process and export information effectively can "market" this information to gain more support from external investors. This two-way access to information significantly reduces information asymmetry and has a more positive effect on highly leveraged firms, helping to increase the availability of capital and reduce liquidity constraints. Since investors have a better understanding of the company, they can demand a lower "risk premium" from the company, thus reducing the cost of financing.
Second, digital transformation has a positive effect on the creditworthiness of highly indebted companies and can improve their investment and financing behaviour to a certain extent and reduce financial bottlenecks. With digital transformation, companies are able to allocate resources more efficiently and are able to improve access to capital, which can significantly reduce the financial risk of highly indebted companies and reduce their need to wean themselves off financial risk. With the impact of digital transformation, the potential for business growth can be further stimulated, managers can allocate capital more wisely, and companies can improve the efficiency of their operations in pursuit of higher returns. Recognizing this higher potential, external investors lower their expectations of risk, which in turn lowers their financing costs.
In the process of digital transformation, through the close integration of all aspects of business management and digital technology, can effectively reduce costs, improve efficiency and increase profits, therefore, the pursuit of production efficiency, economic profits and market position of the enterprise, more likely to digital transformation 33 ; Zhang 11 research found that to meet the market demand for small and medium-sized enterprises is the primary driver of the digital transformation, and substantially cost reduction and productivity improvement are the other two important driving factors. Based on this, we propose:
H2: There is a significant positive effect of corporate indebtedness on corporate digital transformation, and the higher the indebtedness, the more willing the company is to undergo digital transformation.
Percentage of accounts receivable
As an important component of a company's working capital, accounts receivable have a direct impact on a company's ability to realize funds and the speed of cash flow 34 . Accounts receivable are an indispensable component of an entity's liquidity, and can help companies gain more customers in the face of fierce market competition. However, the effective management of accounts receivable is also a difficult task for companies, which can increase the workload of accounting processing; companies also need to face the pressure when collecting accounts receivable. Once accounts receivable are deferred and not effectively collected, this will seriously affect the liquidity of the enterprise and increase the operating expenses and cost of capital; secondly, the existence of accounts receivable inevitably exaggerates part of the company's operating performance, and even if an allowance for doubtful accounts is established, the risk of potential loss still exists; finally, accounts receivable do not have cash flow corresponding to operating profit, and the related costs must be provided by capital, which increases the risk of impaired liquidity and leads to an increase in corporate financial risk.
With digital transformation, companies are able to manage their receivables in a networked and digital way, which can significantly improve the quality of their receivables management. This is mainly due to the fact that under the influence of digitalization, enterprises are able to assess customer quality more accurately, reducing the risk of bad debts and improving the efficiency of their capital investments. The perfection of accounts receivable analysis systems cannot be achieved without comprehensive data support. Digital transformation of enterprises can improve the efficiency of data processing and therefore can promote the increase of accounts receivable turnover. Compared with traditional offline channels, online platforms have more stable revenue and shorter repayment cycles, and therefore higher accounts receivable turnover rates. This not only reduces the risk of bad debts, but also improves capital turnover and operational efficiency. Gregory 35 found that with the emergence of the concept of digital transformation, a series of industrial analyses claimed that digital transformation improves firm performance; and Hu 36 confirmed that digital transformation of firms has a positive contribution to firm performance. Based on this, we propose:
H3: The accounts receivable ratio is directly proportional to the degree of digital transformation, and the higher the ratio, the more willing the company is to undergo digital transformation.
Management overhead ratio
Digital transformation can promote information sharing among enterprises, which in turn can help them improve management efficiency. According to Liu, Bai, and Dong 37 , new-generation digital technologies such as BD, blockchain, and CC are breaking down the "data silos" of enterprises, enabling the sharing of enterprise information, accelerating the "disintermediation" of enterprise internal governance, integrating and rationalizing management methods, and achieving the effect of cutting management costs and improving management efficiency. Digital transformation enables enterprises to create an intelligent, rational, and efficient information management model for operation and management, thereby improving work efficiency. At the same time, digital transformation allows the enhancement of enterprise information technology management, which helps break the business barriers between departments, reduce information asymmetry between departments in the enterprise, eliminate barriers to information transmission, improve timeliness, enhance the efficiency of enterprise operations, and reduce administrative costs. Digital transformation helps to increase the awareness of information management among business managers, which in turn improves management efficiency, and can support the development and application of digital technologies by increasing the resources and energy invested in the business 27 . Firms can achieve better production management, reduce their market transaction costs such as those for search, information, transportation, delivery, and management, promote better organization and resource allocation, improve supply chain management capabilities, and enhance value creation through data aggregation, data analysis, and data-driven decision-making 38 , 39 . In an experiment with listed companies, Li et al. 39 found that the digitization of companies themselves could effectively improve the efficiency of internal management. Digital transformation enables management to manage human resources more effectively, while the use of systematic and intelligent contracts can avoid cost problems, contract disputes, and fraud issues, thus reducing risks and management costs and improving management efficiency. Based on this, we propose:
H4: The overhead ratio is directly proportional to the degree of digital transformation, and the higher the expense ratio, the more willing the digital transformation is.
Profitability (ROA)
The pursuit of increased profitability is the main reason for companies to make the change and transformation. The transformation of a company involves a great cost, and if the benefits gained after the transformation are not project to match the costs paid, then the incentive to transform the company will be greatly affected. Digital transformation of an enterprise means new costs, increased training costs and an uncertain future for the enterprise. Significant investments in building teams of experts, acquiring new hardware and software, developing and designing business products, establishing digital channels, and redesigning intelligent processes are all elements of a successful digital transformation. However, it is clear that these changes are not entirely due to digital technologies and that increased investments do not necessarily lead to increased production. From the initial investment phase (mainly investments in hardware, software, and training services) to the mid-term internalization phase (training and adaptation of the hardware, software, and services, integration into existing enterprise management systems, etc.) and subsequent value creation, digital transformation requires a very complex and time-consuming process for a company to be able to finally achieve the goal of increasing its profitability. Digital transformation will inevitably change the original organizational structure of enterprises, change their management process and operation model, and affect the whole enterprise management system. As a consequence, companies with relatively strong profitability will be open to digital transformation, but they also tend to maintain the current management model, will not readily try risky operations, and will have a more conservative attitude when facing digital transformation. Based on this, we propose:
H5: Profitability has no significant effect on the willingness of companies to undergo digital transformation.
Revenue share of overseas business
With the implementation of "going global", China's Foreign Direct Investment (FDI) has grown rapidly and has become one of the world's largest investors. Following the "One Belt, One Road" strategy, more and more Chinese companies are venturing into foreign markets and doing business abroad 40 . Less attention has been paid in the existing literature to the issue of how the share of overseas business affects digital transformation, and different scholars hold different views on this issue. Stallkamp, Hunt, and Schotter 41 argue that companies with a high share of overseas operations are more willing and motivated to undertake digital transformation, and companies with a high degree of internationalization have a broader perspective and are more likely to be exposed to international cutting-edge ideas. In addition, the digital economy can promote technological and industrial innovation and upgrading, which can lead to efficient use of resources and thus optimize the efficiency of resource use, which also encourages companies with a high degree of internationalization to undergo digital transformation.
Another view is that companies with a high degree of internationalization are not more willing to undergo digital transformation. Wu and Tian 42 suggest that companies with a high proportion of overseas operations have more barriers to digital transformation, which affects their willingness to engage in digital transformation and the extent to which they do so. It is more difficult to obtain real-time data and other information between business units based in and outside of China. At the same time, countries have imposed various controls on the flow of cross-border data to ensure information security, resulting in the restricted flow of such data. In recent years, the Personal Information Protection Law, the Data Security Law, and other laws and regulations on data management have been promulgated one after another, and the management of international information transmission has become stricter. Furthermore, due to the geographical dispersion and differences in business environments, the offshore operations of multinational enterprises are often highly heterogeneous, making it difficult to achieve integrated domestic and foreign operations 41 . Consequently, the degree of internationalization may affect the unified coordination of domestic and overseas resources in the process of digital transformation of enterprises, and thus the degree of digital transformation of enterprises. Zhan and Ouyang 43 argue that in addition to objective-level constraints, the weak motivation of some enterprises with a high proportion of overseas business to carry out reform is due to the fact that such enterprises are generally dependent on upstream enterprises and have a stable revenue stream. This makes them lack the willingness and motivation for digital transformation. However, it is easy to find that the above views are based on theoretical level analysis and lack empirical support. Based on this, in this paper we aim to explore the relationship between digital transformation and internationalization of enterprises from both theoretical and empirical levels, and propose the following hypotheses:
H6: A high percentage of overseas revenue has an inverse effect on the digital transformation of enterprises, and enterprises with a high percentage of overseas revenue have a lower willingness to engage in digital transformation.
For ease of reading, the following table summarises the six assumptions about firm characteristics at the organisational level (i.e., 'O' in the TOE theoretical framework) (Table 3 ).
Shareholding structure
The impact of equity structure factors on the digital transformation of firms has been extensively explored in the existing literature. On the one hand, some scholars argue that the higher the concentration of equity in a company, the easier it may be to make production and management decisions 44 ; on the other hand, there is also literature that shows that excessive concentration of equity may lead to "one voice" that can affect the optimal transformation of a company 45 , 46 .
Mao et al. 45 find empirically that shareholders are more inclined to participate in the decision-making process of digital transformation than in other business decisions because of the more far-reaching impact of digital transformation strategy on the company. This leads to more arbitrariness and difficulty to change corporate decisions, which affects the decision of digital transformation. Therefore, Vial 46 states that the willingness of companies to digitally transform is more pronounced in companies with less concentrated shareholdings than in companies with relatively concentrated shareholdings.
Yin et al. 44 assert that companies with a concentrated shareholding have a greater sense of "big ownership" when making business decisions, which encourages them to actively exercise their supervisory power and thus facilitates the efficient implementation of decisions. Gul, Kim, and Qiu 47 contend that high equity concentration can bring about a certain degree of monitoring and control, which is more pivotal in markets with poor external corporate governance mechanisms. This is because in the absence of external management constraints, shareholders are forced to actively participate in supervisory management, which can only be effective if ownership is concentrated 48 . Similarly, controlling shareholders who own a large percentage of shares have a strong incentive to actively constrain and influence the actual power of management. This helps mitigate agency problems and thus facilitates decision-making 49 . In summary, we propose:
H7: Corporate shareholding structure plays an inverse effect on digital transformation; the more concentrated the shareholding structure, the lower the willingness of firms to transform digitally.
The ownership type of a firm represents the organizational system, background, and resource environment of the firm, and firms with different ownership types have different corporate goals, which in turn influence their investment decision preferences 49 . Chinese enterprises can be divided into state-owned (SOEs) and non-state-owned (mostly private and a few foreign-owned) based on the nature of ownership (property ownership). In studies of ownership and digital transformation of firms, scholars have mostly discussed state-owned enterprises in terms of their operations and the personal characteristics of their executives 50 , 51 .
SOEs have relatively fixed business models and business philosophies, which are not easily influenced by the market and can take their place in the market with their unique advantages 51 . This leads to a lack of incentive for SOEs to innovate in the process of digital transformation. In contrast, non-SOEs, which are in a fierce and uncertain market environment, need to conform to the digital technology trends in the new era in order to create competitive advantages in future development. At the same time, digital transformation fits the innovation needs of non-SOEs to create advantages, thus driving them to invest more in innovation activities for digital transformation 52 .
In terms of corporate executives, Porfírio et al. 53 noted that SOEs are subject to stricter government regulation than non-SOEs, and executive appointments and decisions are subject to government interference. The selection and promotion of SOE executives is based on managerial competence and political considerations 54 , which hinders the full development of their managerial capabilities and makes them more willing to stay in their comfort zone rather than risk innovative transformation. In addition, executive compensation in SOEs is subject to the government's Executive Compensation Regulations, which reduces the incentive for executives to initiate digital transformation 52 . Based on this, we propose:
H8: SOEs have a lower willingness to undertake digital transformation compared to private enterprises.
The 2 hypotheses proposed under the corporate governance theory at the organisational level (i.e., "O" in the TOE theoretical framework) are presented in the following table (Table 4 ).
Enterprise life cycle
According to Gort and Kleppe's 55 enterprise life cycle theory, an enterprise is like a living organism, and its growth until death is called a life cycle. In different life cycle stages, enterprises' own business characteristics, resource reserves, and financing channels differ greatly, and also encounter different degrees of agency and information asymmetry problems. As a result, there are also some differences in the willingness of enterprises to engage in digital transformation in each cycle. Digital transformation is a major strategic decision, which makes it important for companies to avoid blind digital-related investments in the process of digital transformation. Several scholars have studied the impact of digital transformation on firm performance across different life cycles and found that its role differs across the life cycle 10 , 56 . The same differences may exist across time in terms of the impact of the life cycle a firm is in on its willingness to digitally transform, which needs to be verified empirically.
Chanias, Myers, and Hess 57 argue that the willingness to transform digitally increases as the business development cycle progresses. Miller and Friesen 58 suggest that start-ups are young and do not have sufficient resources and experience to support a large-scale transformation. For companies in the growth stage, development focuses on gradual stabilization and rapid sales growth and resource accumulation, trying to achieve advantage accumulation to help the company gain greater scale. Therefore, companies in the growth stage have the motivation to carry out digital transformation to achieve cumulative development. Companies in the maturity period have a stable and mature business model and organizational structure, relatively rich and stable profits, and a strong willingness to innovate on their own 48 . The mature structure and strong willingness and ability to innovate enable mature enterprises to have higher willingness to face digital transformation. In addition, mature enterprises tend to accumulate certain digital resources and capital in their development, so mature enterprises have a certain foundation for digital transformation 59 .
Finally, Bhattacharya, Chang, and Li 60 argue that digital transformation can bring new dynamism to declining firms and new ways to improve firm performance. Therefore, companies in recession also have some willingness to transform digitally. However, some scholars point out that in the majority of enterprises in recession, even if they are willing to carry out digital transformation, few of them can really implement it, due to objective capacity constraints and lack of funds 61 . In summary, in this paper we propose the following hypothesis:
H9: Firms in the growth and maturity stages of the enterprise life cycle have a stronger willingness to engage in digital transformation.
Business age
Yin 44 empirically verified the effect of firm's year of establishment (Age) on willingness to engage in digital transformation. The results demonstrate that the more experienced the firm is in operation, the more motivated it is to undergo digital transformation. Wu et al. 62 pointed out that longer-established companies with more operational experience are more likely to have sufficient capabilities and resources to effectively support the digital transformation reform process, make full use of information advantages, and achieve scale effects; at the same time, the large amount of resources within the company can promote the synergistic development of the company through digital technology, thus improving its innovation capability. Therefore, companies with more operational experience have a higher willingness to engage in digital transformation.
Companies with operational experience undergo digital transformation with the aim of using digital technologies to enhance and innovate existing products 63 , and explore and develop new, potentially disruptive business models to remain competitive and generate new revenues 11 , 64 . In contrast, companies that are still young and lack operational experience have a relatively low willingness to undergo digital transformation because they differ from experienced companies in managing their operational focus. Meanwhile, Strange, Chen, and Fleury 61 point out that the management capacity and cost required to conduct digital transformation are huge challenges for most start-ups. Therefore, start-ups have low motivation to undertake digital transformation due to lack of experience, capacity, and cost constraints. In summary, we propose the following hypothesis:
H10: Enterprise age has a positive effect on digital transformation willingness; the earlier an enterprise is established, the more experienced it is in operation, and the more willing it is to undergo digital transformation.
The 2 assumptions made by considering the life cycle of the firm at the organisational level (i.e., "O" in the TOE theoretical framework) are summarised in the table below (Table 5 ).
Herfindahl–Hirschman Index (HHI)
The Herfindahl–Hirschman Index (HHI) measures market concentration and competition among firms within an industry 65 . It is calculated by summing the squares of the market share percentages held by all firms within the market, offering insights into the competitive dynamics and the concentration level in an industry 65 . A higher HHI indicates a higher level of market concentration, suggesting fewer competitors and potentially less competition. In contrast, a lower HHI reflects a more fragmented market with more competitors and higher levels of competition.
HHI is a tool that measures the level of competition among firms. The degree of industry competition reflects the intensity of competition among firms with limited resources, and Nasiri 66 states that within industries with low levels of industry competition, firms are less exposed to competitors' interference with their resources and access to financing, making them less motivated to create competitive advantage through digital transformation reforms. Conversely, the higher the level of competition within an industry, the more motivated firms are to make digital transformation reforms, expecting to take advantage of the digital dividend and promote competition in the industry. Some scholars have pointed out that the competitive environment and market changes are the key factors and main drivers for digital transformation from the internal and external perspectives of companies 8 , 67 . The evolution of consumer demands and industry technologies can drive companies to use digital platforms and technologies to find creative solutions. Changes in the external environment can have an impact on organizational behaviour and structure, which in turn can have an effect on the propensity to adopt technology in the digital transformation of companies. Firms with low HHI face a more difficult competitive environment and have a higher willingness to undergo digital transformation. At the same time, some scholars argue that firms with low HHI are more willing to digitally transform because they recognize that digital technologies bring disruptions to the markets in which they operate 40 . They facilitate the recombination of existing products and services to generate new forms of digital offerings 68 , lower barriers to entry, and hinder the sustainability of the competitive advantage of existing players 69 . For example, online platforms enable the redefinition of existing markets by facilitating the exchange of digital goods and services 70 .
In contrast, Nasiri 66 notes that firms with high HHI operate in a business with high barriers to entry and a stable flow of benefits. Such companies have little or no need to quickly differentiate themselves in a homogenous competitive market. Digital technology can help enterprises quickly grasp market development trends through calculation and analysis, find insightful problems, and make timely optimization and rectification in the rapidly changing market environment. Therefore, the willingness of enterprises with high HHI to carry out digital transformation is low. Therefore, we propose the following hypothesis:
H11: The higher the HHI, the lower the willingness of enterprises to engage in digital transformation.
In other words, the hypotheses that emerge from the environmental dimension of the TOE theoretical framework (i.e., the "E" in TOE) are the following (Table 6 ).
Data, variables, and model setting
Data sources.
In this study we utilized data from A-share listed enterprises spanning from 2007 to 2021 as the research subjects, based on a combination of data availability and reliability. The variables for the degree of digital transformation of listed enterprises and the Herfindahl–Hirschman Index (HHI) of industry concentration were acquired from the China Stock Market & Accounting Research Database (CSMAR), while all other financial data were obtained from the Wind Financial Terminal (WIND). To ensure the validity of the results, several measures were taken to screen and purify the data, including: (1) exclusion of the financial industry sample to account for differences in accounting standards and industry-specific characteristics; (2) elimination of companies with abnormal listing statuses, such as ST and PT, to prevent abnormalities in their operations from affecting the regression results; and (3) exclusion of observed samples with significant missing data and removal of the first and last 1% of extreme outliers to eliminate their interference. These steps were taken to ensure the reliability of the research results.
In this study we use data collected over a long period of time, from 2007 to 2021, as the research sample, consisting of a diverse range of enterprises, including those in various sectors and industries. This broad sample allows for a comprehensive examination of the digital transformation of enterprises, including those that have undergone the transformation and those that have not yet done so at the time of observation. This approach reduces the potential for selective bias and increases the reliability of the regression results by avoiding the restriction of the research scope to only those enterprises that have undergone digital transformation.
Selection of variables and model setting
The aim of the present study is to investigate the relationship between digital transformation and financial performance, with the degree of digital transformation serving as the explanatory variable. This concept has been extensively explored in both the business community and academia. As suggested by Qi and Xiao 71 , the digital transformation of enterprises is primarily underpinned by the integration of AI, blockchain, CC, and BD technologies into the everyday operations of the enterprise. This digital transformation not only enhances the underlying technologies but also empowers the enterprise's production, management, and sales through digital technology applications. To measure the degree of digital transformation, in this study we draw on previous research 72 and use the annual reports of the sample enterprises. The reports are analysed for the frequency of occurrence of terms such as "AI technology", "blockchain technology", "CC technology", "BD technology", and "digital technology application". To avoid heteroscedasticity, the combined number of occurrences of these five terms is logarithmised, and 1 is added. In a robustness test, the "digital technology application" term is excluded and the study focuses on the basic digital technology level, including AI, blockchain, CC, and BD technologies. The frequency of occurrence of these four terms is increased by 1, de-logarithmised, and used as replacement variables in the robustness test. According to Wu et al. 73 , based on the annual reports of A-share listed enterprises, we use the text recognition function of Python crawler to measure the level of digital transformation of enterprises by using the method of keyword "searching-matching-totaling" in this paper.
To determine the impacting factors on the level of digital transformation in enterprises, in this paper we select several variables at both the enterprise and industry levels as explanatory variables. Subsequently, we carry out regression analysis to investigate the correlation between these variables and the degree of digital transformation:
In this paper, we gauge the size of the enterprise by taking the natural logarithm of its total assets. We believe that larger companies are more inclined and pressured to embrace digital transformation due to the significant financial commitment it entails. Moreover, the ability to undergo digital transformation varies among enterprises of different sizes.
To evaluate the capital structure of a firm, we look at its gearing ratio. Capital availability plays a critical role in determining the level of digital transformation a company can undertake, and the gearing ratio offers insights into a company's capacity to secure external funding and manage its debt effectively.
We measure receivable structure by examining current accounts receivable as a percentage of total assets. Companies with a higher proportion of accounts receivable may be more motivated to engage in digital transformation, which can optimize accounts receivable management, enhance business distribution, and improve capital turnover efficiency.
The profitability of a company is evaluated using the return on total assets. Highly profitable companies typically have a strong financial position and are better positioned to take on the risks associated with digital transformation. However, they may also be more cautious and resistant to altering their business strategies, potentially leading to reluctance in embracing digital transformation.
Management costs are analyzed by looking at a company's overhead ratio as a percentage of operating income. Companies with higher management costs are incentivized to reduce day-to-day expenses through digital transformation, which can improve overall management efficiency.
The ownership concentration within a company is determined by the percentage of shares held by its largest shareholder. While a concentrated shareholding structure may aid decision-making in digital transformation, concerns have been raised regarding the authoritarian nature of management in such cases. Therefore, the influence of shareholding structure on digital transformation effectiveness requires further investigation. Moreover, a dummy variable is employed to distinguish between state-owned and private enterprises and evaluate their readiness for digital transformation.
The level of international openness of a business, indicated by the ratio of overseas revenue to total revenue, serves as a proxy for assessing its degree of global engagement. Generally, a higher proportion of overseas revenue correlates with a greater level of internationalization within the enterprise.
This study combines Dickinson's 74 cash flow-based classification with Gort and Kleppe's 55 five-stage life cycle model, comprising introduction, growth, maturity, obsolescence, and decline stages. The research concentrates on variations in digital transformation readiness across companies at different life cycle phases. Companies in the introductory and growth stages may prioritize steady expansion and adhere to a conservative business approach. A dummy variable is introduced to represent the company's life cycle; a value of 1 denotes companies in growth and introduction phases, while a value of 0 signifies those in maturity, obsolescence, and decline stages, allowing for an examination of digital transformation readiness across various life cycle stages.
While business life cycle theory evaluates a company's operational, investing, and financing activities based on cash flows, it offers limited insights into the company's managerial expertise. Conversely, a company's longevity and business experience exhibit a stronger correlation with its willingness to embrace digital transformation. This study employs the establishment years of a company as a proxy for business experience in empirical analysis.
Industry competition is measured using the Herfindahl index as a proxy variable. Companies are classified based on the 2012 SEC Industry Classification Code, and the Herfindahl index is computed utilizing individual companies' operating revenue shares to gauge market concentration. A higher coefficient signifies a more monopolistic industry, while a lower coefficient indicates higher competition. Unlike competitive markets, companies in monopolistic industries may lack a strong incentive to pursue digital transformation, as they can generate substantial profits without emphasizing operational enhancements (Table 7 ).
In summary, combined with the relevant theory in this paper, the empirical model is set up as follows:
where i represents the observed sample firms, t represents the year of observation, and j represents the industry to which the firm belongs. u_i represents individual fixed effects, v_t represents time-fixed effects, and ∈ is the residual of the model fit. STATA, EXCEL, and SPSS software were used for data operations and analysis.
Empirical results and analysis
Descriptive statistics.
Before performing the baseline regression, we conducted descriptive statistics on the variables used in the study to comprehend the overall distribution of the sample. The mean and standard deviation of the core explanatory variable ln_digi2, which measures the extent of digital transformation, were 1.1911 and 1.3617, respectively. The minimum and maximum values of this variable were 0 and 6.3008, indicating a wide range of digital transformation among the sample enterprises. Some enterprises had not undergone digital transformation, while others varied in their extent of transformation (Table 8 ).
The other explanatory variables exhibit similar characteristics and highlight the fact that, despite all the companies being listed on the A-share market, there are significant variations in their financial, operational, and managerial conditions. This further validates the representativeness of the research sample. Therefore, a comprehensive description of all the variables is not required in this study.
Multicollinearity test
To prevent the presence of multicollinearity in the regression results, the variance inflation factor (VIF) test was applied to eliminate multicollinearity among the explanatory variables (Table 9 ).
The results of the variance inflation factor test revealed that the mean value of each explanatory variable and the model was below the threshold of 10, which is considered the indicator for the presence of multicollinearity. Therefore, it can be concluded that there is no multicollinearity among the variables selected for this study, and the baseline regression analysis can proceed.
Baseline regression
In this section, factors that were previously theorized to impact a firm's willingness to undergo digital transformation will be tested empirically. To ensure the validity of the results, a double fixed effects model that accounts for individual and time-level omitted variables will be employed in the empirical regressions. The outcomes of the baseline regressions are presented below (Table 10 ).
To validate the robustness of the preliminary test model and eliminate the potential impact of variables at different levels, we sequentially add different sets of explanatory variables to the regressions. The first column presents the results obtained by including only the basic firm level variables, while the second column displays the results of adding corporate governance variables. The third column represents the results of incorporating corporate openness variables, the fourth column displays the results of incorporating firm life cycle-related variables, and the last column shows the results of incorporating industry competition variables. An analysis of the signs and significance levels of the regression coefficients in each column indicates that the signs and significance levels of the coefficients remain unchanged and statistically significant after the progressive addition of various influencing factors, thus providing initial validation of the reliability of the model's findings.
The results of the regression analysis reveal that the coefficient for firm size is positive and significant at the 1% level, providing evidence for a positive correlation between firm size and the extent of a company's digital transformation, thereby supporting Hypothesis H1. This aligns with economic theory, which postulates that larger firms are more likely to have a higher degree of readiness and to undergo a greater extent of digital transformation. The larger a company's size, the more pressing the need to improve operational and management efficiency through digital transformation. This supports the credibility of Hypothesis H1.
The estimated coefficient of the gearing variable, which represents the capital structure, shows a significant positive relationship (at the 1% significance level) with the degree of digital transformation of A-share listed companies in the sample. This supports the hypothesis that higher gearing drives firms towards digital transformation, as companies with higher leverage seek to improve capital management efficiency and reduce the cost of capital through digital transformation. The same applies to the estimated coefficient of the accounts receivable percentage, which also has a significant positive relationship (at the 1% significance level) with the degree of digital transformation. This suggests that a higher percentage of accounts receivable prompts firms to deepen their digital transformation to improve their capital turnover and reduce their accounts receivable percentage. These results align with the logic of hypotheses H2 and H3, providing empirical evidence to support them.
The results of the regression analysis indicate that the coefficient for the overhead ratio (i.e. Management costs in Table 7 ) is positively significant at the 10% level, implying that enterprises with higher overhead costs are more motivated to undergo digital transformation with the aim of reducing expenses. These findings provide evidence to support Hypothesis H4, which posits that companies with higher overhead costs are more likely to increase their digital transformation efforts. Additionally, the regression results suggest that the effect of return on equity on digital transformation is negative, but not statistically significant, implying that return on equity is not a significant factor influencing digital transformation. This result supports the robustness of Hypothesis H5, which holds that return on equity is not a major determinant of digital transformation.
The results of the empirical study show that the equity concentration variable (i.e. Shareholding structure in Table 7 ), as a measure of corporate governance, has a significant negative correlation with the level of digital transformation, as indicated by its estimated coefficient, which is negative and significant at the 1% level. This supports hypothesis H7, which posits a negative relationship between equity concentration and the level of digital transformation. Furthermore, the heterogeneity test that we used to analyse the attributes of firms, such as duality, the share of overseas business, and the firm's life cycle, suggests that the level of digital transformation is influenced by the nature, characteristics, and stage of the firm, thus providing evidence in support of hypotheses H6, H8, H9, and H10.
In conclusion, the empirical results demonstrate that firms with higher debt ratios, higher accounts receivable ratios, and higher overhead costs are more likely to increase their digital transformation efforts in order to improve their operational efficiency and reduce costs.
Robustness tests
In order to further verify the reliability of the empirical findings of this paper, robustness tests will be conducted using different methods.
Removal of exogenous interference
The COVID-19 pandemic in 2020 had a significant impact on the A-share listed companies, leading to a dual-faceted effect. On one hand, companies in a state of crisis may face difficulties in acquiring the financial and material resources required for digital transformation due to stagnant production and operations and disrupted cash flows. On the other hand, the pandemic lockdown prompted many companies to adopt digital transformation as a means of self-help, with some companies finding opportunities in the crisis to resume production to the greatest extent possible. As a result, in this study we exclude the observations from 2020 and 2021 to examine if the impact of each factor on the extent of digital transformation is in line with the regression results from the baseline model, which is not affected by exogenous shocks (Table 11 ).
The robustness tests, performed with the exclusion of the potential impact of the COVID-19 epidemic on the digital transformation of enterprises, demonstrate the consistency of the results. Despite a slight reduction in the significance level of the firm attribute variable, the sign of the estimated coefficients of the other variables remained unchanged and statistically significant, indicating that the impact of the factors on the degree of digital transformation remains robust even in the absence of exogenous interference.
Substitution of explanatory variables
This subsection focuses on the exclusion of the term "digital technology applications" at the application level, and the retention of only the four basic digital technologies: "artificial intelligence technology", "blockchain technology", "cloud computing technology", and "big data technology". The frequency of occurrence of these four technologies is increased by 1, and the natural logarithm is used as a robustness test for the replacement variable. The results are as follows (Table 12 ).
After adjusting the variables, the results of the regression are largely consistent with the benchmark regression, with the exception of a lower significance level of the overhead percentage, firm attribute, and life cycle variables (the direction of their estimated coefficients remain unchanged). This further illustrates the robustness of the empirical findings.
Heterogeneity analysis
We further examine the impact of firm heterogeneity on the degree of digital transformation. The heterogeneity is evaluated in terms of enterprise attributes (state-owned enterprises vs. private enterprises), enterprise duality (whether the director and general manager are held by the same person), enterprise industry characteristics (whether it is a manufacturing industry), and enterprise life cycle (whether it is a recession). We also compare the differences and similarities in the effect of various factors on digital transformation for different types of firms (Table 13 ).
Dimensions of firm attribute heterogeneity
In this research we have found significant differences in the influence of corporate capital structure and overhead ratios on the extent of digital transformation among firms of different attributes. The study has shown that there is a positive correlation between the degree of gearing and the degree of digital transformation in private firms. However, this relationship does not exist in state-owned enterprises as they can receive financial support from financial institutions and major shareholders in times of financial strain. In contrast, private firms often face challenges in obtaining financing and have high overhead costs, making digital transformation more important for them so as to reduce costs and minimize debt costs.
The impact of profitability on the extent of digital transformation is significant in state-owned enterprises but not in private companies. This can be attributed to the different perspectives of digital transformation held by the two types of firms. While private companies view digital transformation as a means to improve their performance, state-owned enterprises may see it as a way to enhance their already established success. The managerial mindset of state-owned enterprises, which is often influenced by prior experience in government work, inclines towards a more cautious and risk-averse approach, contributing to this difference in perception.
The analysis of both the entire sample and the private sample showed that there is a positive correlation between a firm's age and the degree of its digital transformation. This result is consistent with the difference in profitability between the two samples, reflecting the overall cautious approach of state-owned enterprises compared to private firms in terms of the factors impacting digital transformation.
Dimensions of corporate duality
In our examination of the effect of corporate duality on the level of digital transformation, we found a notable distinction in the impact of the firm's age. For firms where the Chairman and the General Manager are the same individual, there is a positive correlation between the firm's age and the level of digital transformation, meaning that as the firm grows older, the level of digital transformation increases. Conversely, for firms where the Chairman and General Manager are different individuals, there is no statistically significant association between the firm's age and the degree of digital transformation. This discrepancy may be due to information asymmetry between the principal and agent in the latter type of firms, leading to a lack of transfer of operational experience from one General Manager to the next and causing a generation gap in management as the company ages.
Dimensions of industry heterogeneity
For the manufacturing sector, there is no statistically significant correlation between a firm's gearing and the degree of digital transformation. Conversely, in non-manufacturing industries, a higher gearing ratio was found to correspond with a higher degree of digital transformation. This disparity can be attributed to the fact that manufacturing companies typically have high gearing ratios, while non-manufacturing companies are more asset-light and may find it more challenging to secure financing, leading to a greater need to reduce their debt burden through digital transformation.
The results of the analysis showed that the relationship between the degree of digital transformation and the percentage of overseas business revenue differs between manufacturing and non-manufacturing companies. In the case of non-manufacturing firms, there is a negative correlation between the percentage of overseas business revenue and the degree of digital transformation, whereas for manufacturing firms, there is no significant association between the two variables. Additionally, the results indicated that for manufacturing firms, the degree of digital transformation is highest during the maturity stage of the life cycle, whereas for non-manufacturing firms, there is no significant difference in the degree of digital transformation among companies in different life cycle stages.
Dimensions of life cycle heterogeneity
We found that companies experiencing recession tend to adopt digital transformation more intensively to decrease their gearing. This relationship between gearing and digital transformation was not found for companies at other stages of their life cycle. This can be attributed to the difficulty faced by companies in recession in obtaining external financing, leading them to optimize their operations and create new revenue streams through digital transformation to reduce their debt. The same phenomenon was observed with respect to overhead ratios, with non-recessionary companies being more tolerant of high overhead costs due to the characteristics of their operations prior to the recession.
For firms that are not in recession, no significant correlation was found between the degree of digital transformation and equity structure, company age, and industry competition, which are factors that usually have a major impact. This can be attributed to the need for companies to have a clear understanding of the factors affecting their digital transformation during the initial and growth stages. Management philosophies may vary, leading to diverse decision-making processes and a lack of uniformity in comparison to companies in the declining stage.
Research findings
This study has uncovered several key insights into the influencing factors of corporate digital transformation among Chinese listed companies. Firstly, the gearing ratio analysis illustrates that digital transformation facilitates optimised asset allocation, reducing gearing ratios and enhancing asset utilisation efficiency. JD.com is a prime example, where digital technologies streamlined inventory and supply chain management, reducing the gearing ratio and improving asset turnover 75 . The inclination towards digital transformation in companies with higher gearing ratios can be attributed to the pressure to enhance financial stability and reduce dependency on borrowed capital. Companies aim to attract better investment opportunities and mitigate financial risks by optimising assets and reducing liabilities.
Secondly, adopting automation and intelligent technology significantly decreases management expenses regarding the management overhead ratio. This results in improved efficiency and reduced workforce costs, boosting overall competitiveness. Xiaomi Inc.'s integration of AI into its operations exemplifies how digital transformation can lead to operational efficiencies and cost reductions 76 . Companies with high overhead ratios pursue digital transformation to streamline operations and reduce costs, driven by the need to enhance profitability and competitiveness in a fast-evolving market.
Finally, from the perspective of accounts receivable ratio, digital transformation can help enterprises optimise accounts receivable management, accelerate the speed of capital turnover, reduce the risk of bad debts, and improve the liquidity and solvency of enterprises. Alibaba Group's use of blockchain technology for accounts receivable processes exemplifies the potential of digital solutions to enhance financial operations 77 . The motivation here stems from the desire to accelerate capital turnover and improve financial health, particularly for companies facing high accounts receivable ratios that can hinder cash flow and increase financial vulnerability. Therefore, companies with high gearing, overhead, and accounts receivable ratios are more likely to drive digital transformation to minimize costs and enhance performance.
However, companies in monopolistic industries and those in the inception stage of their life cycle are less likely to undertake significant digital transformation. This cautiousness is attributed to the potential risks of disrupting established processes and the significant investments required for major digital overhauls. Instead, they may adopt a more cautious approach and make incremental changes to avoid disrupting the existing business environment.
This research reinforces the observations by Ferreira, Fernandes and Ferreira 68 and Chanias, Myers and Hess 57 , who highlighted the role of digital transformation in optimizing asset allocation and reducing management expenses in European and North American firms, respectively. However, we extend these insights by demonstrating the pronounced effect of digital initiatives in enhancing financial stability and reducing the gearing ratio. Digital transformation can help enterprises optimise asset allocation, reduce gearing ratio and improve asset utilisation efficiency, thus creating more value for enterprises. Also, in terms of management expense ratio, digital transformation can reduce the management expense ratio of the enterprise through automation and intelligent technology, improve management efficiency, reduce manpower costs and improve the overall competitiveness of the enterprise.
Furthermore, this study enriches the discourse initiated by Strange, Chen and Fleury 61 on the benefits of digital transformation in the manufacturing industries by exploring its impact on the Chinese economy. It uncovers that digital practices significantly expedite capital turnover and diminish debt risks, improving liquidity and solvency in an acutely relevant manner for Chinese firms. From the perspective of accounts receivable ratio, digital transformation can help enterprises optimise accounts receivable management, accelerate the speed of capital turnover, reduce the risk of bad debts, and improve the liquidity and solvency of enterprises.
Rooted in existing research, the main contributions of this paper are altogether as follows: first, to identify the factors that influence the intensity of digital transformation of firms, including both internal firm factors and external industry factors. Although previous studies have examined the economic impact of digital transformation, the research in this paper is able to explain in more detail the reasons for the differences in the degree of digital transformation among different firms; second, this paper incorporates enterprise micro characteristics and industry factors into the analysis, examining the heterogeneity of enterprise attributes, enterprise duality, industry characteristics and enterprise life cycle, so as to propose targeted policy recommendations; third. The complexity of the enterprise digital transformation process makes it difficult to measure the degree of transformation, and relevant statistics are relatively limited. This research further complements and enriches the index portrayal of digital transformation by measuring it through regression models with the help of annual reports of A-share listed companies.
Recommendations
Policymakers: Offer public and platform assistance in science and technology policies to facilitate the extensive utilization of digital advancements like big data, artificial intelligence, and cloud computing in all facets of business activities such as research and development, design, production, operation, and sales management. Bai et al. 30 suggest that government should enhance funding for capital, workforce, and technological innovation initiatives to cater to the public and quasi-public service requirements of enterprises, particularly small and medium-sized businesses (SMEs), in order to facilitate their digital transition, including the establishment of industry-wide digital technology platforms.
Business leaders: To navigate the complexities of digital transformation successfully, Chinese listed companies must adopt a strategic approach that aligns with their unique operational and market contexts. As Osmundsen, Iden and Bygstad 78 emphasize, the path to a digital ecosystem necessitates a tailored strategy that considers the specific needs and challenges of each company, ensuring that digital transformation initiatives are not just adopted for their own sake but are integrated in ways that genuinely enhance organizational performance and competitive advantage. Hence, enterprises should selectively carry out digital transformation according to their own situation and development needs. Before embarking on digital transformation, the management should make adequate preliminary preparations such as conducting an internal review of the enterprise, getting a full grasp of the enterprise's development, and setting a clear corporate development strategy and long-term goals, to understand the situation of the enterprise itself; subsequently they should engage in such exercises as market research to gain an in-depth understanding of market demand, as well as consumer preferences, and so on, to understand the external environment of the enterprise 79 .
Other stakeholders: Stakeholders including relevant practitioners, employees should cultivate digital thinking and receive digital training. Cultivating practitioners' digital thinking is beneficial to strengthening their understanding of digital transformation, which helps them make the right decisions and is conducive to the survival and development of enterprises. Digital thinking includes building a digital vision, grasping the direction of transformation, maintaining strategic strength and other dimensions; enterprises should use digital thinking to solve practical problems in the process of enterprise development 80 . Meanwhile, navigating and leveraging government policies and incentives for digital transformation is crucial for maximizing the benefits of these initiatives. Verhoef et al. 70 provide a roadmap for Chinese enterprises to effectively engage with government-led digital ecosystems and take advantage of policy support, underscoring the strategic importance of public-private partnerships in facilitating digital innovation. By actively participating in these ecosystems, companies can access a broader network of resources, technologies, and market opportunities essential for successful digital transformation.
Conclusion and outlook
This article focuses on the influencing factors of the degree of digital transformation of enterprises, and selects the important influencing factors at the levels of enterprise characteristics, corporate governance, enterprise internationalization, enterprise life cycle, and industry competitiveness, and explores the influence of these factors on the degree of digital transformation of enterprises. The empirical results also reflect the motivation of enterprises' digital transformation, which seems to be more of a "sending charcoal in the snow" motivation than an "adding flowers on the cake" motivation in this paper's sample observation range.
The paper concludes with countermeasures for enterprise digital transformation from the enterprise and government levels. Enterprises need to carry out digital transformation according to their own situation and needs, and make full use of top-level design and market research. Enterprise practitioners should have a deep understanding of the connotation of digital culture, and strengthen the construction of the digital talent team. At the government level, investment in digital infrastructure should be increased to ensure its viability and receive political and financial support.
One limitation of this research is its insufficient coverage of the technological aspect within the TOE framework. Given the significant role that technology plays in today's business landscape, overlooking this perspective could hinder the comprehension of how the TOE framework is implemented within an organization. The focus of this study is primarily on the influence of organizations and environments on technology adoption and usage, rather than delving into the technology itself. This lack of emphasis on technology may result in readers not fully grasping the practical applications and impacts of technology on the TOE framework. Neglecting to properly address the technological aspect may lead to an incomplete understanding of how corporate decisions regarding technology adoption and utilization are made, potentially resulting in biased or erroneous conclusions in real-world scenarios.
To gain a more comprehensive understanding of how the TOE framework operates in practice, future research endeavors should integrate the technical viewpoint to offer a more well-rounded perspective on technology adoption. We intend to manually gather pertinent data in order to provide a thorough and detailed examination of the subject matter.
Data availability
The data that support the findings of this study are available from the corresponding author upon reasonable request.
Li,K. Y. Wang, Y. J., Yang, W. Y. & Wang, S. F. Influencing factors of digital transformation of SMEs: A sample survey and empirical analysis based on 314 enterprises. https://doi.org/10.19699/j.cnki.issn2096-0298.2024.03.165 (2024).
Li, W. L., Pan, W. D. & Yuan, K. B. Enterprise digital transformation and the development of China’s real economy. J. Quant. Tech. Econ. 39 (9), 5–25. https://doi.org/10.13653/j.cnki.jqte.2022.09.001 (2022).
Article Google Scholar
National Development and Reform Commission. The State Council on the issuance of the "14th Five-Year Plan" for the development of the digital economy notice. www.gov.cn (2021).
Accenture. 2021 Accenture China Enterprise Digital Transformation Index Report. Available at: accenture.com (2021).
Yi, L. X., Wu, F. & Xu, S. Y. Research on the performance driving effect of enterprise digital transformation. Securities Market Herald 8 , 15–25, 69 (2021).
XCMG: Digital business transformation of a manufacturing giant. (n.d.). Retrieved 23 November 2023, from https://www.imd.org/research-knowledge/china/case-studies/xcmg-digital-business-transformation-of-a-manufacturing-giant/
Kumar, A. & Krishnamoorthy, B. Business Analytics Adoption in Firms: A Qualitative Study Elaborating TOE Framework in India. Int. J. Glob. Bus. Compet. 15 (2), 1–14. https://doi.org/10.1007/s42943-020-00013-5 (2020).
Article CAS Google Scholar
Cheng, Q. J., Wang, Y. M. & Wang, M. F. Research on peer effect of enterprise digital transformation and influencing factors. Chin. J. Manag. 18 (5), 653–663 (2021).
MathSciNet Google Scholar
Wang, Y. G. & Wang, L. L. The typology of digital transformation strategies and the choice of transformation modes of traditional firm. Manag. Rev. 33 (11), 84–93. https://doi.org/10.14120/j.cnki.cn11-5057/f.20211123.001 (2021).
Article ADS Google Scholar
Bhattacharya, D., Chang, C.-W. & Li, W. -H. Stages of firm life cycle, transition, and dividend policy. Finance Res. Lett. 33 , 101226. https://doi.org/10.1016/j.frl.2019.06.024 (2020).
Zhang, X. H. Obstacles, driving factors and path dependence of digital transformation of small and medium-sized enterprises—An investigation based on the survey of 377 small and medium-sized enterprises in the tertiary industry. China Business Market 34 (12), 72–82. https://doi.org/10.14089/j.cnki.cn11-3664/f.2020.12.008 (2020).
Wang, C. Y. & Chen, H. M. Research on the problems of digital transformation of enterprises in the context of digital economy. Mod. Manag. 41 (02), 29–31. https://doi.org/10.19634/j.cnki.11-1403/c.2021.02.008 (2021).
Wang, Y. G., Wang, L. N. & Li, X. Research on the iterative transformation from digital search to digital ecosystem: A case study of digital transformation based on Schneider. J. Manag. World. 39 (08), 91–114. https://doi.org/10.19744/j.cnki.11-1235/f.2023.0094 (2023).
Zhao, J. B. The Integration of the New Generation of Information Technology with the Real Economy:Based on the Paradigm of Smart Manufacturing. Science of Science and Management of S. & T. 41 (03), 3-16 (2020).
Google Scholar
Wang, Y. J., Tang, X. B. & Chen, X. H. Development opportunities and strategies for the digital economy industry in China under the influence of new coronavirus pneumonia. Sci. Res. Manag. 41 (6), 157–171. https://doi.org/10.19571/j.cnki.1000-2995.2020.06.017 (2020).
Wu, Y. H. Research on the digital transformation path of retail enterprises in the post-pandemic era. Spec. Zone Econ. 04 , 93–96 (2023).
Qiu, Z. Q. Technology and organization: Themes and theoretical trends in sociology and other disciplines. Sociol. Stud . 32 (04), 167–192, 245–246. https://doi.org/10.19934/j.cnki.shxyj.2017.04.008 (2017).
Walker, R. M. Internal and external antecedents of process in novation. Public Manag. Rev. 16 (1), 21–44 (2014).
Oliveira, T. & Martins, M. F. Literature review of information technology adoption models at firm level. Electron. J. Inform. Syst. Evaluat. 1 (2), 312–323 (2011).
Oliveira, T. & Martins, M. F. Literature review of information technology adoption models at firm level. Electron. J. Inform. Syst. Evaluat. 14 (1), 110–121 (2011).
Lin, H.-F. An empirical investigation of mobile banking adoption: The effect of innovation attributes and knowledge-based trust. Int. J. Inform. Manag. 31 (3), 252–260. https://doi.org/10.1016/j.ijinfomgt.2010.07.006 (2011).
Smith, N. C. & Rönnegard, D. Shareholder primacy, corporate social responsibility, and the role of business schools. J. Business Ethics. 134 (3), 463–478. https://doi.org/10.1007/s10551-014-2427-x (2016).
Stoelhorst, J. W. & Vishwanathan, P. Beyond primacy: A stakeholder theory of corporate governance. Acad. Manag. Rev. 49 (1), 107–134. https://doi.org/10.5465/amr.2020.0268 (2024).
Chen, S. H. & Zheng, W. Q. Latest advances of corporate governance theory: A new analysis framework. Manag. World. https://doi.org/10.19744/j.cnki.11-1235/f.2010.02.015 (2010).
Shi, W., Connelly, B. L. & Hoskisson, R. E. External corporate governance and financial fraud: Cognitive evaluation theory insights on agency theory prescriptions. Strategic Manag. J. 38 (6), 1268–1286. https://doi.org/10.1002/smj.2560 (2017).
Li, D. & Li, E. X. N. Corporate governance and costs of equity: Theory and evidence. Manag. Sci. 64 (1), 83–101. https://doi.org/10.1287/mnsc.2016.2570 (2018).
Article ADS MathSciNet Google Scholar
Wang, W. H. & Zhou, L. Y. How can digital transformation in logistics industry improve financial performance? Based on the dual path of financing cost and management efficiency. Commun. Accounting Finance. https://doi.org/10.16144/j.cnki.issn1002-8072.2022.20.031 (2022).
Zhang, Y. S., Li, X. B. & Xing, M. Q. Enterprise digital transformation and audit pricing. Auditing Res. 03 , 62–71 (2021).
Xiao, H. J., Yang, Z. & Liu, M. Y. The promoting effect of corporate digitalization on social responsibility: Examination of internal and external dual paths. Econ. Manag. 43 (11), 52–69. https://doi.org/10.19616/j.cnki.bmj.2021.11.004 (2021).
Bai, X. J., Wang, X. Y. & Song, P. Factors influencing digital transformation of Chinese enterprises: Empirical analysis in the framework of TOE. Stud. Sci. Sci. https://doi.org/10.16192/j.cnki.1003-2053.20240019.003 (2024).
Wen, C. H., Li, S. L., Guo, L. H. & Yu, J. J. Excessively financing, squeezing-out effect and capital degenerating to virtue: Evidence of China’s Real Listed Firms from 2007 to 2015. Business Manag. J. 40 (07), 39–55. https://doi.org/10.19616/j.cnki.bmj.2018.07.003 (2018).
Che, D. X., Duan, M. Y. & Wang, F. The impact and mechanism of enterprise digital transformation on financing cost. Financial Regulat. Res. 12 , 56–74. https://doi.org/10.13490/j.cnki.frr.2021.12.006 (2021).
Bjorkdahl, J. Strategies for digitalization in manufacturing firms [J]. California Manag. Rev. 62 (4), 17–36 (2020).
Shi, T. Taking port enterprises as an example to discuss the construction of enterprise accounts receivable chain, digital control and analysis system. Finance Account. Transp. 10 , 7–10 (2021).
Gregory, V. Understanding digital transformation: A review and a research agenda [J]. J. Strategic Inform. Syst. 28 (2), 118–214 (2019).
Hu, Q. The mechanism and performance of enterprises’ digital transformation [J]. Zhejiang Academic J. 2 , 146–154 (2020).
Liu, D. H., Bai, F. P. & Dong, K. Y. Study on the mechanisms of digital transformation’s impact on corporate performance. Commun. Finance Account. https://doi.org/10.16144/j.cnki.issn1002-8072.2022.16.010 (2022).
Huang, Q. H., Yu, Y. Z. & Zhang, S. L. Internet development and productivity growth in manufacturing industry: Internal mechanism and China experiences. China Ind. Econ. https://doi.org/10.19581/j.cnki.ciejournal.2019.08.001 (2019).
Li, H. J., Tian, Y. X. & Li, W. J. Mobile internet thinking and traditional; business reengineering. China Ind. Econ. https://doi.org/10.19581/j.cnki.ciejournal.2014.10.011 (2014).
Matarazzo, M. et al. Digital transformation and customer value creation in Made in Italy SMEs: A dynamic capabilities perspective. J. Business Res. 123 , 642–656. https://doi.org/10.1016/j.jbusres.2020.10.033 (2021).
Stallkamp, M., Hunt, R. A. & Schotter, A. P. J. Scaling, fast and slow: The internationalization of digital ventures. J. Business Res. 146 , 95–106. https://doi.org/10.1016/j.jbusres.2022.03.070 (2022).
Wu, W. Q. & Tian, Y. J. Can corporate digital transformation reduce cost stickiness? From the perspective of cost adjustment capability. Account. Res. 04 , 89–112 (2022).
Zhan, X. J. & Ouyang, Y. F. The new trend of global investment and new strategy of china's utilization of foreign capital under the digital economy. 34 (03), 78–86. https://doi.org/10.19744/j.cnki.11-1235/f.2018.03.007 (2018).
Yin, X. N., Zhan, X. M. & Tang, S. Q. The impact mechanism of manufacturing enterprises' digital transformation on financial performance. China Business Market . 36 (07), 96–106. https://doi.org/10.14089/j.cnki.cn11-3664/f.2022.07.009 (2022).
Mao, J., Li, J., & Zhang, B. W. ‘CEOs’ Multi-career background and enterprise digital transformation’. Modern Finance Econ.-J. Tianjin Univ. Finance Econ . 42 (09), 37–58. https://doi.org/10.19559/j.cnki.12-1387.2022.09.002 (2022).
Vial, G. Understanding digital transformation: A review and a research agenda. in Managing Digital Transformation. Routledge. 228 (2021).
Gul, F. A., Kim, J.-B. & Qiu, A. A. Ownership concentration, foreign shareholding, audit quality, and stock price synchronicity: Evidence from China. J. Financial Econ. 95 (3), 425–442. https://doi.org/10.1016/j.jfineco.2009.11.005 (2010).
Tang, S., Su, X. S. & Zhao, D. N. Fintech and enterprise digital transformation—From the perspective of enterprise life cycle. Finance Econ. 02 , 17–32 (2022).
Choi, S. B., Lee, S. H. & Williams, C. Ownership and firm innovation in a transition economy: Evidence from China. Res. Policy 40 (3), 441–452. https://doi.org/10.1016/j.respol.2011.01.004 (2021).
Mao, J., Li, J. & Zhang, B. W. ‘CEOs’ multi-career background and enterprise digital transformation. Modern Finance Econ.-J. Tianjin Univ. Finance Econ . 42 (09), 37–58. https://doi.org/10.19559/j.cnki.12-1387.2022.09.002 (2022).
Zhang, Z. et al. Comparisons of multiple modes of digital transformation of state-owned enterprises: A case analysis based on 50 state-owned enterprise. Science & Technology Progress and Policy, pp. 1–11. http://kns.cnki.net/kcms/detail/42.1224.G3.20220916.1655.005.html (2022).
Li, S. X. & Wang, Y. H. Enterprise digital transformation and enterprise innovation—Empirical evidence from electronic manufacturing industry. J. Ind. Technol. Econ. 41 (08), 19–26 (2022).
Porfírio, J. A. et al. Leadership characteristics and digital transformation. J. Business Res. 124 , 610–619. https://doi.org/10.1016/j.jbusres.2020.10.058 (2021).
Zhang, J. B. The integration of the new generation of information technology with the real economy: Based on the paradigm of smart manufacturing. Sci. Sci. Manag. Sci. Technol. 41 (3), 3–16 (2020).
MathSciNet CAS Google Scholar
Gort, M. & Klepper, S. Time paths in the diffusion of product innovations. Econ. J. 92 (367), 630–653 https://doi.org/10.2307/2232554 (1982).
Lei, G. Y., Mai, R. D. & Zuo, J. J. Digital transformation and capital market efficiency: Based on the perspective of stock price synchronization. Secur. Mark. Her. 55 (08), 48–59 (2022).
Chanias, S., Myers, M. D. & Hess, T. Digital transformation strategy making in pre-digital organizations: The case of a financial services provider. J. Strategic Inform. Syst. 28 (1), 17–33. https://doi.org/10.1016/j.jsis.2018.11.003 (2019).
Miller, D. & Friesen, P. H. A longitudinal study of the corporate life cycle. Manag. Sci. 30 (10), 1161–1183. https://doi.org/10.1287/mnsc.30.10.1161 (1984).
Li, H. & Yu, C. Y. Digitalization and M&A performance of distribution firms—Based on a firm lifecycle perspective. J. Commercial Econ. 05 , 130–133 (2022).
Bhattacharya, D., Chang, C.-W. & Li, W.-H. Stages of firm life cycle, transition, and dividend policy. Finance Res. Lett. 33 , 101226. https://doi.org/10.1016/j.frl.2019.06.024 (2020).
Strange, R., Chen, L. & Fleury, M. T. L. Digital transformation and international strategies. J. Int. Manag. https://doi.org/10.1016/j.intman.2022.100968 (2022).
Wu, F. et al. Enterprise digital transformation and capital market performance: Empirical evidence from stock liquidity. J. Manag. World. 37 (07), 130–144. https://doi.org/10.19744/j.cnki.11-1235/f.2021.0097 (2021).
Vogelsang, K. et al. Success factors for fostering a digital transformation in manufacturing companies. J. Enterprise Transformation. 8 (1–2), 121–142. https://doi.org/10.1080/19488289.2019.1578839 (2018).
Shang, H. T. & Wu, T. Enterprise digital transformation, corporate social responsibility and firm value. J. Technol. Econ. 41 (07), 159–168 (2022).
Rhoades, S. A. The Herfindahl-Hirschman Index. Federal Reserve Bulletin 79 , 188 (1993).
Nasiri, M. et al. Managing the digital supply chain: The role of smart technologies. Technovation 96–97 , 102–121. https://doi.org/10.1016/j.technovation.2020.102121 (2020).
Yang, Z., Chen, J. & Shang, H. C. Which experiences drive digitalization: executives’ academic experience and enterprise digital transformation. On Econ. Problems. https://doi.org/10.16011/j.cnki.jjwt.2022.10.001 (2022).
Ferreira, J. J. M., Fernandes, C. I. & Ferreira, F. A. F. To be or not to be digital, that is the question: Firm innovation and performance. J. Business Res. 101 , 583–590. https://doi.org/10.1016/j.jbusres.2018.11.013 (2019).
Li, M. Y. & Zhang, N. L. Enterprise digital transformation and going global: promoting or inhibiting? The evidence from Chinese A-share listed companies. World Econ. Stud. https://doi.org/10.13516/j.cnki.wes.2022.10.008 (2022).
Verhoef, P. C. et al. Digital transformation: A multidisciplinary reflection and research agenda. J. Business Res. 122 , 889–901. https://doi.org/10.1016/j.jbusres.2019.09.022 (2021).
Qi, Y. D. & Xiao, X. Transformation of enterprise management in the era of digital economy. J. Manag. World . 36 (06), 135–152+250. https://doi.org/10.19744/j.cnki.11-1235/f.2020.0091 (2020).
Luo, J. H. & Wu, Y. L. Level of digital operation and real earnings management. J. Manag. Sci. 34 (04), 3–18 (2021).
Wu, F., Hu, H. Z., Lin, H. Y. & Ren, X. Y. Enterprise digital transformation and capital market performance: Empirical evidence from stock liquidity. https://doi.org/10.19744/j.cnki.11-1235/f.2021.0097 (2021).
Dickinson, V. Cash flow patterns as a proxy for firmlife cycle[J ]. Working paper Fisher School of Accounting University of Florida (2007).
Yang, V. Pioneering Digital Transformation: JD.com’s Robust Presence at CIFTIS 2023, JD Corporate Blog . https://jdcorporateblog.com/pioneering-digital-transformation-jd-coms-robust-presence-at-ciftis-2023/ (2023).
Stanford Graduate School of Business. Xiaomi’s Globalization Strategy and Challenges. https://www.gsb.stanford.edu/faculty-research/case-studies/xiaomis-globalization-strategy-challenges (2019).
INSEAD Alibaba in Blockchain: Integrating Blockchain-based Remittances into Cloud Services. https://publishing.insead.edu/case/alibaba-blockchain (2018).
Osmundsen, K., Iden, J. & Bygstad, B. Digital transformation: Drivers, success factors, and implications. Digital Transformation, p. 16. (2018).
Jin, G. F. & Ma, M. Y. Digital transformation of small and medium-sized enterprises in post-epidemic era. Inf. Res. 07 , 59–65. https://doi.org/10.3969/j.issn.1005-8095.2022.07.009 (2022).
Li, X. D. & Chen, S. R. Main obstacles, driving factors and countermeasures of digital transformation: Insights from Zaozhuang Chemical Industry. J. New Industrialization. 13 (11), 80–88 (2023).
Download references
Acknowledgements
The authors would like to thank all of the people who participated in the studies.
This work was supported by the National Social Science Foundation of China (19AGL013); Project supported by Office of the Foundation of Liaoning Province Education Administration (JG22DB240), and the Fundamental Research Funds for the Central Universities (2020CDJSK02TD03).
Author information
Authors and affiliations.
Surrey International Institute, Dongbei University of Finance and Economics, Dalian, 116025, Liaoning, China
Xu Zhao, Pengyu Chen & Shen Chen
School of Economics and Business Administration, Chongqing University (Campus B), Chongqing, 400030, China
Xu Zhao & Qi-an Chen
Cesar Ritz Colleges Switzerland, English Gruss Strasse 43, 3902, Brig, Switzerland
Haitao Zhang
You can also search for this author in PubMed Google Scholar
Contributions
Xu Zhao, Pengyu Chen and Shen Chen: contributed to conceptualization, methodology, analysis, and writing; Haitao Zhang: contributed to validation and resources; Xu Zhao, Qi-an Chen and Haitao Zhang: contributed to experiment design, and data collection; Qi-an Chen and Xu Zhao: contributed to investigation, supervision, and review editing. All authors have read and agreed to the published version of the manuscript.
Corresponding author
Correspondence to Qi-an Chen .
Ethics declarations
Competing interests.
The authors declare no competing interests.
Additional information
Publisher's note.
Springer Nature remains neutral with regard to jurisdictional claims in published maps and institutional affiliations.
Rights and permissions
Open Access This article is licensed under a Creative Commons Attribution 4.0 International License, which permits use, sharing, adaptation, distribution and reproduction in any medium or format, as long as you give appropriate credit to the original author(s) and the source, provide a link to the Creative Commons licence, and indicate if changes were made. The images or other third party material in this article are included in the article's Creative Commons licence, unless indicated otherwise in a credit line to the material. If material is not included in the article's Creative Commons licence and your intended use is not permitted by statutory regulation or exceeds the permitted use, you will need to obtain permission directly from the copyright holder. To view a copy of this licence, visit http://creativecommons.org/licenses/by/4.0/ .
Reprints and permissions
About this article
Cite this article.
Zhao, X., Chen, Qa., Zhang, H. et al. A study on the influencing factors of corporate digital transformation: empirical evidence from Chinese listed companies. Sci Rep 14 , 6243 (2024). https://doi.org/10.1038/s41598-024-56729-4
Download citation
Received : 30 July 2023
Accepted : 11 March 2024
Published : 15 March 2024
DOI : https://doi.org/10.1038/s41598-024-56729-4
Share this article
Anyone you share the following link with will be able to read this content:
Sorry, a shareable link is not currently available for this article.
Provided by the Springer Nature SharedIt content-sharing initiative
- Digital transformation
- Influencing factors
- Chinese listed companies
By submitting a comment you agree to abide by our Terms and Community Guidelines . If you find something abusive or that does not comply with our terms or guidelines please flag it as inappropriate.
Quick links
- Explore articles by subject
- Guide to authors
- Editorial policies
Sign up for the Nature Briefing newsletter — what matters in science, free to your inbox daily.

Log in using your username and password
- Search More Search for this keyword Advanced search
- Latest content
- Current issue
- Browse by collection
- BMJ Journals More You are viewing from: Google Indexer
You are here
- Online First
- The role of COVID-19 vaccines in preventing post-COVID-19 thromboembolic and cardiovascular complications
- Article Text
- Article info
- Citation Tools
- Rapid Responses
- Article metrics

- Núria Mercadé-Besora 1 , 2 , 3 ,
- Xintong Li 1 ,
- Raivo Kolde 4 ,
- Nhung TH Trinh 5 ,
- Maria T Sanchez-Santos 1 ,
- Wai Yi Man 1 ,
- Elena Roel 3 ,
- Carlen Reyes 3 ,
- http://orcid.org/0000-0003-0388-3403 Antonella Delmestri 1 ,
- Hedvig M E Nordeng 6 , 7 ,
- http://orcid.org/0000-0002-4036-3856 Anneli Uusküla 8 ,
- http://orcid.org/0000-0002-8274-0357 Talita Duarte-Salles 3 , 9 ,
- Clara Prats 2 ,
- http://orcid.org/0000-0002-3950-6346 Daniel Prieto-Alhambra 1 , 9 ,
- http://orcid.org/0000-0002-0000-0110 Annika M Jödicke 1 ,
- Martí Català 1
- 1 Pharmaco- and Device Epidemiology Group, Health Data Sciences, Botnar Research Centre, NDORMS , University of Oxford , Oxford , UK
- 2 Department of Physics , Universitat Politècnica de Catalunya , Barcelona , Spain
- 3 Fundació Institut Universitari per a la recerca a l'Atenció Primària de Salut Jordi Gol i Gurina (IDIAPJGol) , IDIAP Jordi Gol , Barcelona , Catalunya , Spain
- 4 Institute of Computer Science , University of Tartu , Tartu , Estonia
- 5 Pharmacoepidemiology and Drug Safety Research Group, Department of Pharmacy, Faculty of Mathematics and Natural Sciences , University of Oslo , Oslo , Norway
- 6 School of Pharmacy , University of Oslo , Oslo , Norway
- 7 Division of Mental Health , Norwegian Institute of Public Health , Oslo , Norway
- 8 Department of Family Medicine and Public Health , University of Tartu , Tartu , Estonia
- 9 Department of Medical Informatics, Erasmus University Medical Center , Erasmus University Rotterdam , Rotterdam , Zuid-Holland , Netherlands
- Correspondence to Prof Daniel Prieto-Alhambra, Pharmaco- and Device Epidemiology Group, Health Data Sciences, Botnar Research Centre, NDORMS, University of Oxford, Oxford, UK; daniel.prietoalhambra{at}ndorms.ox.ac.uk
Objective To study the association between COVID-19 vaccination and the risk of post-COVID-19 cardiac and thromboembolic complications.
Methods We conducted a staggered cohort study based on national vaccination campaigns using electronic health records from the UK, Spain and Estonia. Vaccine rollout was grouped into four stages with predefined enrolment periods. Each stage included all individuals eligible for vaccination, with no previous SARS-CoV-2 infection or COVID-19 vaccine at the start date. Vaccination status was used as a time-varying exposure. Outcomes included heart failure (HF), venous thromboembolism (VTE) and arterial thrombosis/thromboembolism (ATE) recorded in four time windows after SARS-CoV-2 infection: 0–30, 31–90, 91–180 and 181–365 days. Propensity score overlap weighting and empirical calibration were used to minimise observed and unobserved confounding, respectively.
Fine-Gray models estimated subdistribution hazard ratios (sHR). Random effect meta-analyses were conducted across staggered cohorts and databases.
Results The study included 10.17 million vaccinated and 10.39 million unvaccinated people. Vaccination was associated with reduced risks of acute (30-day) and post-acute COVID-19 VTE, ATE and HF: for example, meta-analytic sHR of 0.22 (95% CI 0.17 to 0.29), 0.53 (0.44 to 0.63) and 0.45 (0.38 to 0.53), respectively, for 0–30 days after SARS-CoV-2 infection, while in the 91–180 days sHR were 0.53 (0.40 to 0.70), 0.72 (0.58 to 0.88) and 0.61 (0.51 to 0.73), respectively.
Conclusions COVID-19 vaccination reduced the risk of post-COVID-19 cardiac and thromboembolic outcomes. These effects were more pronounced for acute COVID-19 outcomes, consistent with known reductions in disease severity following breakthrough versus unvaccinated SARS-CoV-2 infection.
- Epidemiology
- PUBLIC HEALTH
- Electronic Health Records
Data availability statement
Data may be obtained from a third party and are not publicly available. CPRD: CPRD data were obtained under the CPRD multi-study license held by the University of Oxford after Research Data Governance (RDG) approval. Direct data sharing is not allowed. SIDIAP: In accordance with current European and national law, the data used in this study is only available for the researchers participating in this study. Thus, we are not allowed to distribute or make publicly available the data to other parties. However, researchers from public institutions can request data from SIDIAP if they comply with certain requirements. Further information is available online ( https://www.sidiap.org/index.php/menu-solicitudesen/application-proccedure ) or by contacting SIDIAP ([email protected]). CORIVA: CORIVA data were obtained under the approval of Research Ethics Committee of the University of Tartu and the patient level data sharing is not allowed. All analyses in this study were conducted in a federated manner, where analytical code and aggregated (anonymised) results were shared, but no patient-level data was transferred across the collaborating institutions.
This is an open access article distributed in accordance with the Creative Commons Attribution 4.0 Unported (CC BY 4.0) license, which permits others to copy, redistribute, remix, transform and build upon this work for any purpose, provided the original work is properly cited, a link to the licence is given, and indication of whether changes were made. See: https://creativecommons.org/licenses/by/4.0/ .
https://doi.org/10.1136/heartjnl-2023-323483
Statistics from Altmetric.com
Request permissions.
If you wish to reuse any or all of this article please use the link below which will take you to the Copyright Clearance Center’s RightsLink service. You will be able to get a quick price and instant permission to reuse the content in many different ways.
WHAT IS ALREADY KNOWN ON THIS TOPIC
COVID-19 vaccines proved to be highly effective in reducing the severity of acute SARS-CoV-2 infection.
While COVID-19 vaccines were associated with increased risk for cardiac and thromboembolic events, such as myocarditis and thrombosis, the risk of complications was substantially higher due to SARS-CoV-2 infection.
WHAT THIS STUDY ADDS
COVID-19 vaccination reduced the risk of heart failure, venous thromboembolism and arterial thrombosis/thromboembolism in the acute (30 days) and post-acute (31 to 365 days) phase following SARS-CoV-2 infection. This effect was stronger in the acute phase.
The overall additive effect of vaccination on the risk of post-vaccine and/or post-COVID thromboembolic and cardiac events needs further research.
HOW THIS STUDY MIGHT AFFECT RESEARCH, PRACTICE OR POLICY
COVID-19 vaccines proved to be highly effective in reducing the risk of post-COVID cardiovascular and thromboembolic complications.
Introduction
COVID-19 vaccines were approved under emergency authorisation in December 2020 and showed high effectiveness against SARS-CoV-2 infection, COVID-19-related hospitalisation and death. 1 2 However, concerns were raised after spontaneous reports of unusual thromboembolic events following adenovirus-based COVID-19 vaccines, an association that was further assessed in observational studies. 3 4 More recently, mRNA-based vaccines were found to be associated with a risk of rare myocarditis events. 5 6
On the other hand, SARS-CoV-2 infection can trigger cardiac and thromboembolic complications. 7 8 Previous studies showed that, while slowly decreasing over time, the risk for serious complications remain high for up to a year after infection. 9 10 Although acute and post-acute cardiac and thromboembolic complications following COVID-19 are rare, they present a substantial burden to the affected patients, and the absolute number of cases globally could become substantial.
Recent studies suggest that COVID-19 vaccination could protect against cardiac and thromboembolic complications attributable to COVID-19. 11 12 However, most studies did not include long-term complications and were conducted among specific populations.
Evidence is still scarce as to whether the combined effects of COVID-19 vaccines protecting against SARS-CoV-2 infection and reducing post-COVID-19 cardiac and thromboembolic outcomes, outweigh any risks of these complications potentially associated with vaccination.
We therefore used large, representative data sources from three European countries to assess the overall effect of COVID-19 vaccines on the risk of acute and post-acute COVID-19 complications including venous thromboembolism (VTE), arterial thrombosis/thromboembolism (ATE) and other cardiac events. Additionally, we studied the comparative effects of ChAdOx1 versus BNT162b2 on the risk of these same outcomes.
Data sources
We used four routinely collected population-based healthcare datasets from three European countries: the UK, Spain and Estonia.
For the UK, we used data from two primary care databases—namely, Clinical Practice Research Datalink, CPRD Aurum 13 and CPRD Gold. 14 CPRD Aurum currently covers 13 million people from predominantly English practices, while CPRD Gold comprises 3.1 million active participants mostly from GP practices in Wales and Scotland. Spanish data were provided by the Information System for the Development of Research in Primary Care (SIDIAP), 15 which encompasses primary care records from 6 million active patients (around 75% of the population in the region of Catalonia) linked to hospital admissions data (Conjunt Mínim Bàsic de Dades d’Alta Hospitalària). Finally, the CORIVA dataset based on national health claims data from Estonia was used. It contains all COVID-19 cases from the first year of the pandemic and ~440 000 randomly selected controls. CORIVA was linked to the death registry and all COVID-19 testing from the national health information system.
Databases included sociodemographic information, diagnoses, measurements, prescriptions and secondary care referrals and were linked to vaccine registries, including records of all administered vaccines from all healthcare settings. Data availability for CPRD Gold ended in December 2021, CPRD Aurum in January 2022, SIDIAP in June 2022 and CORIVA in December 2022.
All databases were mapped to the Observational Medical Outcomes Partnership Common Data Model (OMOP CDM) 16 to facilitate federated analytics.
Multinational network staggered cohort study: study design and participants
The study design has been published in detail elsewhere. 17 Briefly, we used a staggered cohort design considering vaccination as a time-varying exposure. Four staggered cohorts were designed with each cohort representing a country-specific vaccination rollout phase (eg, dates when people became eligible for vaccination, and eligibility criteria).
The source population comprised all adults registered in the respective database for at least 180 days at the start of the study (4 January 2021 for CPRD Gold and Aurum, 20 February 2021 for SIDIAP and 28 January 2021 for CORIVA). Subsequently, each staggered cohort corresponded to an enrolment period: all people eligible for vaccination during this time were included in the cohort and people with a history of SARS-CoV-2 infection or COVID-19 vaccination before the start of the enrolment period were excluded. Across countries, cohort 1 comprised older age groups, whereas cohort 2 comprised individuals at risk for severe COVID-19. Cohort 3 included people aged ≥40 and cohort 4 enrolled people aged ≥18.
In each cohort, people receiving a first vaccine dose during the enrolment period were allocated to the vaccinated group, with their index date being the date of vaccination. Individuals who did not receive a vaccine dose comprised the unvaccinated group and their index date was assigned within the enrolment period, based on the distribution of index dates in the vaccinated group. People with COVID-19 before the index date were excluded.
Follow-up started from the index date until the earliest of end of available data, death, change in exposure status (first vaccine dose for those unvaccinated) or outcome of interest.
COVID-19 vaccination
All vaccines approved within the study period from January 2021 to July 2021—namely, ChAdOx1 (Oxford/AstraZeneca), BNT162b2 (BioNTech/Pfizer]) Ad26.COV2.S (Janssen) and mRNA-1273 (Moderna), were included for this study.
Post-COVID-19 outcomes of interest
Outcomes of interest were defined as SARS-CoV-2 infection followed by a predefined thromboembolic or cardiac event of interest within a year after infection, and with no record of the same clinical event in the 6 months before COVID-19. Outcome date was set as the corresponding SARS-CoV-2 infection date.
COVID-19 was identified from either a positive SARS-CoV-2 test (polymerase chain reaction (PCR) or antigen), or a clinical COVID-19 diagnosis, with no record of COVID-19 in the previous 6 weeks. This wash-out period was imposed to exclude re-recordings of the same COVID-19 episode.
Post-COVID-19 outcome events were selected based on previous studies. 11–13 Events comprised ischaemic stroke (IS), haemorrhagic stroke (HS), transient ischaemic attack (TIA), ventricular arrhythmia/cardiac arrest (VACA), myocarditis/pericarditis (MP), myocardial infarction (MI), heart failure (HF), pulmonary embolism (PE) and deep vein thrombosis (DVT). We used two composite outcomes: (1) VTE, as an aggregate of PE and DVT and (2) ATE, as a composite of IS, TIA and MI. To avoid re-recording of the same complication we imposed a wash-out period of 90 days between records. Phenotypes for these complications were based on previously published studies. 3 4 8 18
All outcomes were ascertained in four different time periods following SARS-CoV-2 infection: the first period described the acute infection phase—that is, 0–30 days after COVID-19, whereas the later periods - which are 31–90 days, 91–180 days and 181–365 days, illustrate the post-acute phase ( figure 1 ).
- Download figure
- Open in new tab
- Download powerpoint
Study outcome design. Study outcomes of interest are defined as a COVID-19 infection followed by one of the complications in the figure, within a year after infection. Outcomes were ascertained in four different time windows after SARS-CoV-2 infection: 0–30 days (namely the acute phase), 31–90 days, 91–180 days and 181–365 days (these last three comprise the post-acute phase).
Negative control outcomes
Negative control outcomes (NCOs) were used to detect residual confounding. NCOs are outcomes which are not believed to be causally associated with the exposure, but share the same bias structure with the exposure and outcome of interest. Therefore, no significant association between exposure and NCO is to be expected. Our study used 43 different NCOs from previous work assessing vaccine effectiveness. 19
Statistical analysis
Federated network analyses.
A template for an analytical script was developed and subsequently tailored to include the country-specific aspects (eg, dates, priority groups) for the vaccination rollout. Analyses were conducted locally for each database. Only aggregated data were shared and person counts <5 were clouded.
Propensity score weighting
Large-scale propensity scores (PS) were calculated to estimate the likelihood of a person receiving the vaccine based on their demographic and health-related characteristics (eg, conditions, medications) prior to the index date. PS were then used to minimise observed confounding by creating a weighted population (overlap weighting 20 ), in which individuals contributed with a different weight based on their PS and vaccination status.
Prespecified key variables included in the PS comprised age, sex, location, index date, prior observation time in the database, number of previous outpatient visits and previous SARS-CoV-2 PCR/antigen tests. Regional vaccination, testing and COVID-19 incidence rates were also forced into the PS equation for the UK databases 21 and SIDIAP. 22 In addition, least absolute shrinkage and selection operator (LASSO) regression, a technique for variable selection, was used to identify additional variables from all recorded conditions and prescriptions within 0–30 days, 31–180 days and 181-any time (conditions only) before the index date that had a prevalence of >0.5% in the study population.
PS were then separately estimated for each staggered cohort and analysis. We considered covariate balance to be achieved if absolute standardised mean differences (ASMDs) were ≤0.1 after weighting. Baseline characteristics such as demographics and comorbidities were reported.
Effect estimation
To account for the competing risk of death associated with COVID-19, Fine-and-Grey models 23 were used to calculate subdistribution hazard ratios (sHRs). Subsequently, sHRs and confidence intervals were empirically calibrated from NCO estimates 24 to account for unmeasured confounding. To calibrate the estimates, the empirical null distribution was derived from NCO estimates and was used to compute calibrated confidence intervals. For each outcome, sHRs from the four staggered cohorts were pooled using random-effect meta-analysis, both separately for each database and across all four databases.
Sensitivity analysis
Sensitivity analyses comprised 1) censoring follow-up for vaccinated people at the time when they received their second vaccine dose and 2) considering only the first post-COVID-19 outcome within the year after infection ( online supplemental figure S1 ). In addition, comparative effectiveness analyses were conducted for BNT162b2 versus ChAdOx1.
Supplemental material
Data and code availability.
All analytic code for the study is available in GitHub ( https://github.com/oxford-pharmacoepi/vaccineEffectOnPostCovidCardiacThromboembolicEvents ), including code lists for vaccines, COVID-19 tests and diagnoses, cardiac and thromboembolic events, NCO and health conditions to prioritise patients for vaccination in each country. We used R version 4.2.3 and statistical packages survival (3.5–3), Empirical Calibration (3.1.1), glmnet (4.1-7), and Hmisc (5.0–1).
Patient and public involvement
Owing to the nature of the study and the limitations regarding data privacy, the study design, analysis, interpretation of data and revision of the manuscript did not involve any patients or members of the public.
All aggregated results are available in a web application ( https://dpa-pde-oxford.shinyapps.io/PostCovidComplications/ ).
We included over 10.17 million vaccinated individuals (1 618 395 from CPRD Gold; 5 729 800 from CPRD Aurum; 2 744 821 from SIDIAP and 77 603 from CORIVA) and 10.39 million unvaccinated individuals (1 640 371; 5 860 564; 2 588 518 and 302 267, respectively). Online supplemental figures S2-5 illustrate study inclusion for each database.
Adequate covariate balance was achieved after PS weighting in most studies: CORIVA (all cohorts) and SIDIAP (cohorts 1 and 4) did not contribute to ChAdOx1 subanalyses owing to sample size and covariate imbalance. ASMD results are accessible in the web application.
NCO analyses suggested residual bias after PS weighting, with a majority of NCOs associated positively with vaccination. Therefore, calibrated estimates are reported in this manuscript. Uncalibrated effect estimates and NCO analyses are available in the web interface.
Population characteristics
Table 1 presents baseline characteristics for the weighted populations in CPRD Aurum, for illustrative purposes. Online supplemental tables S1-25 summarise baseline characteristics for weighted and unweighted populations for each database and comparison. Across databases and cohorts, populations followed similar patterns: cohort 1 represented an older subpopulation (around 80 years old) with a high proportion of women (57%). Median age was lowest in cohort 4 ranging between 30 and 40 years.
- View inline
Characteristics of weighted populations in CPRD Aurum database, stratified by staggered cohort and exposure status. Exposure is any COVID-19 vaccine
COVID-19 vaccination and post-COVID-19 complications
Table 2 shows the incidence of post-COVID-19 VTE, ATE and HF, the three most common post-COVID-19 conditions among the studied outcomes. Outcome counts are presented separately for 0–30, 31–90, 91–180 and 181–365 days after SARS-CoV-2 infection. Online supplemental tables S26-36 include all studied complications, also for the sensitivity and subanalyses. Similar pattern for incidences were observed across all databases: higher outcome rates in the older populations (cohort 1) and decreasing frequency with increasing time after infection in all cohorts.
Number of records (and risk per 10 000 individuals) for acute and post-acute COVID-19 cardiac and thromboembolic complications, across cohorts and databases for any COVID-19 vaccination
Forest plots for the effect of COVID-19 vaccines on post-COVID-19 cardiac and thromboembolic complications; meta-analysis across cohorts and databases. Dashed line represents a level of heterogeneity I 2 >0.4. ATE, arterial thrombosis/thromboembolism; CD+HS, cardiac diseases and haemorrhagic stroke; VTE, venous thromboembolism.
Results from calibrated estimates pooled in meta-analysis across cohorts and databases are shown in figure 2 .
Reduced risk associated with vaccination is observed for acute and post-acute VTE, DVT, and PE: acute meta-analytic sHR are 0.22 (95% CI, 0.17–0.29); 0.36 (0.28–0.45); and 0.19 (0.15–0.25), respectively. For VTE in the post-acute phase, sHR estimates are 0.43 (0.34–0.53), 0.53 (0.40–0.70) and 0.50 (0.36–0.70) for 31–90, 91–180, and 181–365 days post COVID-19, respectively. Reduced risk of VTE outcomes was observed in vaccinated across databases and cohorts, see online supplemental figures S14–22 .
Similarly, the risk of ATE, IS and MI in the acute phase after infection was reduced for the vaccinated group, sHR of 0.53 (0.44–0.63), 0.55 (0.43–0.70) and 0.49 (0.38–0.62), respectively. Reduced risk associated with vaccination persisted for post-acute ATE, with sHR of 0.74 (0.60–0.92), 0.72 (0.58–0.88) and 0.62 (0.48–0.80) for 31–90, 91–180 and 181–365 days post-COVID-19, respectively. Risk of post-acute MI remained lower for vaccinated in the 31–90 and 91–180 days after COVID-19, with sHR of 0.64 (0.46–0.87) and 0.64 (0.45–0.90), respectively. Vaccination effect on post-COVID-19 TIA was seen only in the 181–365 days, with sHR of 0.51 (0.31–0.82). Online supplemental figures S23-31 show database-specific and cohort-specific estimates for ATE-related complications.
Risk of post-COVID-19 cardiac complications was reduced in vaccinated individuals. Meta-analytic estimates in the acute phase showed sHR of 0.45 (0.38–0.53) for HF, 0.41 (0.26–0.66) for MP and 0.41 (0.27–0.63) for VACA. Reduced risk persisted for post-acute COVID-19 HF: sHR 0.61 (0.51–0.73) for 31–90 days, 0.61 (0.51–0.73) for 91–180 days and 0.52 (0.43–0.63) for 181–365 days. For post-acute MP, risk was only lowered in the first post-acute window (31–90 days), with sHR of 0.43 (0.21–0.85). Vaccination showed no association with post-COVID-19 HS. Database-specific and cohort-specific results for these cardiac diseases are shown in online supplemental figures S32-40 .
Stratified analyses by vaccine showed similar associations, except for ChAdOx1 which was not associated with reduced VTE and ATE risk in the last post-acute window. Sensitivity analyses were consistent with main results ( online supplemental figures S6-13 ).
Figure 3 shows the results of comparative effects of BNT162b2 versus ChAdOx1, based on UK data. Meta-analytic estimates favoured BNT162b2 (sHR of 0.66 (0.46–0.93)) for VTE in the 0–30 days after infection, but no differences were seen for post-acute VTE or for any of the other outcomes. Results from sensitivity analyses, database-specific and cohort-specific estimates were in line with the main findings ( online supplemental figures S41-51 ).
Forest plots for comparative vaccine effect (BNT162b2 vs ChAdOx1); meta-analysis across cohorts and databases. ATE, arterial thrombosis/thromboembolism; CD+HS, cardiac diseases and haemorrhagic stroke; VTE, venous thromboembolism.
Key findings
Our analyses showed a substantial reduction of risk (45–81%) for thromboembolic and cardiac events in the acute phase of COVID-19 associated with vaccination. This finding was consistent across four databases and three different European countries. Risks for post-acute COVID-19 VTE, ATE and HF were reduced to a lesser extent (24–58%), whereas a reduced risk for post-COVID-19 MP and VACA in vaccinated people was seen only in the acute phase.
Results in context
The relationship between SARS-CoV-2 infection, COVID-19 vaccines and thromboembolic and/or cardiac complications is tangled. Some large studies report an increased risk of VTE and ATE following both ChAdOx1 and BNT162b2 vaccination, 7 whereas other studies have not identified such a risk. 25 Elevated risk of VTE has also been reported among patients with COVID-19 and its occurrence can lead to poor prognosis and mortality. 26 27 Similarly, several observational studies have found an association between COVID-19 mRNA vaccination and a short-term increased risk of myocarditis, particularly among younger male individuals. 5 6 For instance, a self-controlled case series study conducted in England revealed about 30% increased risk of hospital admission due to myocarditis within 28 days following both ChAdOx1 and BNT162b2 vaccines. However, this same study also found a ninefold higher risk for myocarditis following a positive SARS-CoV-2 test, clearly offsetting the observed post-vaccine risk.
COVID-19 vaccines have demonstrated high efficacy and effectiveness in preventing infection and reducing the severity of acute-phase infection. However, with the emergence of newer variants of the virus, such as omicron, and the waning protective effect of the vaccine over time, there is a growing interest in understanding whether the vaccine can also reduce the risk of complications after breakthrough infections. Recent studies suggested that COVID-19 vaccination could potentially protect against acute post-COVID-19 cardiac and thromboembolic events. 11 12 A large prospective cohort study 11 reports risk of VTE after SARS-CoV-2 infection to be substantially reduced in fully vaccinated ambulatory patients. Likewise, Al-Aly et al 12 suggest a reduced risk for post-acute COVID-19 conditions in breakthrough infection versus SARS-CoV-2 infection without prior vaccination. However, the populations were limited to SARS-CoV-2 infected individuals and estimates did not include the effect of the vaccine to prevent COVID-19 in the first place. Other studies on post-acute COVID-19 conditions and symptoms have been conducted, 28 29 but there has been limited reporting on the condition-specific risks associated with COVID-19, even though the prognosis for different complications can vary significantly.
In line with previous studies, our findings suggest a potential benefit of vaccination in reducing the risk of post-COVID-19 thromboembolic and cardiac complications. We included broader populations, estimated the risk in both acute and post-acute infection phases and replicated these using four large independent observational databases. By pooling results across different settings, we provided the most up-to-date and robust evidence on this topic.
Strengths and limitations
The study has several strengths. Our multinational study covering different healthcare systems and settings showed consistent results across all databases, which highlights the robustness and replicability of our findings. All databases had complete recordings of vaccination status (date and vaccine) and are representative of the respective general population. Algorithms to identify study outcomes were used in previous published network studies, including regulatory-funded research. 3 4 8 18 Other strengths are the staggered cohort design which minimises confounding by indication and immortal time bias. PS overlap weighting and NCO empirical calibration have been shown to adequately minimise bias in vaccine effectiveness studies. 19 Furthermore, our estimates include the vaccine effectiveness against COVID-19, which is crucial in the pathway to experience post-COVID-19 complications.
Our study has some limitations. The use of real-world data comes with inherent limitations including data quality concerns and risk of confounding. To deal with these limitations, we employed state-of-the-art methods, including large-scale propensity score weighting and calibration of effect estimates using NCO. 19 24 A recent study 30 has demonstrated that methodologically sound observational studies based on routinely collected data can produce results similar to those of clinical trials. We acknowledge that results from NCO were positively associated with vaccination, and estimates might still be influenced by residual bias despite using calibration. Another limitation is potential under-reporting of post-COVID-19 complications: some asymptomatic and mild COVID-19 infections might have not been recorded. Additionally, post-COVID-19 outcomes of interest might be under-recorded in primary care databases (CPRD Aurum and Gold) without hospital linkage, which represent a large proportion of the data in the study. However, results in SIDIAP and CORIVA, which include secondary care data, were similar. Also, our study included a small number of young men and male teenagers, who were the main population concerned with increased risks of myocarditis/pericarditis following vaccination.
Conclusions
Vaccination against SARS-CoV-2 substantially reduced the risk of acute post-COVID-19 thromboembolic and cardiac complications, probably through a reduction in the risk of SARS-CoV-2 infection and the severity of COVID-19 disease due to vaccine-induced immunity. Reduced risk in vaccinated people lasted for up to 1 year for post-COVID-19 VTE, ATE and HF, but not clearly for other complications. Findings from this study highlight yet another benefit of COVID-19 vaccination. However, further research is needed on the possible waning of the risk reduction over time and on the impact of booster vaccination.
Ethics statements
Patient consent for publication.
Not applicable.
Ethics approval
The study was approved by the CPRD’s Research Data Governance Process, Protocol No 21_000557 and the Clinical Research Ethics committee of Fundació Institut Universitari per a la recerca a l’Atenció Primària de Salut Jordi Gol i Gurina (IDIAPJGol) (approval number 4R22/133) and the Research Ethics Committee of the University of Tartu (approval No. 330/T-10).
Acknowledgments
This study is based in part on data from the Clinical Practice Research Datalink (CPRD) obtained under licence from the UK Medicines and Healthcare products Regulatory Agency. We thank the patients who provided these data, and the NHS who collected the data as part of their care and support. All interpretations, conclusions and views expressed in this publication are those of the authors alone and not necessarily those of CPRD. We would also like to thank the healthcare professionals in the Catalan healthcare system involved in the management of COVID-19 during these challenging times, from primary care to intensive care units; the Institut de Català de la Salut and the Program d’Analítica de Dades per a la Recerca i la Innovació en Salut for providing access to the different data sources accessible through The System for the Development of Research in Primary Care (SIDIAP).
- Pritchard E ,
- Matthews PC ,
- Stoesser N , et al
- Lauring AS ,
- Tenforde MW ,
- Chappell JD , et al
- Pistillo A , et al
- Duarte-Salles T , et al
- Hansen JV ,
- Fosbøl E , et al
- Chen A , et al
- Hippisley-Cox J ,
- Mei XW , et al
- Duarte-Salles T ,
- Fernandez-Bertolin S , et al
- Ip S , et al
- Bowe B , et al
- Prats-Uribe A ,
- Feng Q , et al
- Campbell J , et al
- Herrett E ,
- Gallagher AM ,
- Bhaskaran K , et al
- Raventós B ,
- Fernández-Bertolín S ,
- Aragón M , et al
- Makadia R ,
- Matcho A , et al
- Mercadé-Besora N ,
- Kolde R , et al
- Ostropolets A ,
- Makadia R , et al
- Rathod-Mistry T , et al
- Thomas LE ,
- ↵ Coronavirus (COVID-19) in the UK . 2022 . Available : https://coronavirus.data.gov.uk/
- Generalitat de Catalunya
- Schuemie MJ ,
- Hripcsak G ,
- Ryan PB , et al
- Houghton DE ,
- Wysokinski W ,
- Casanegra AI , et al
- Katsoularis I ,
- Fonseca-Rodríguez O ,
- Farrington P , et al
- Jehangir Q ,
- Li P , et al
- Byambasuren O ,
- Stehlik P ,
- Clark J , et al
- Brannock MD ,
- Preiss AJ , et al
- Schneeweiss S , RCT-DUPLICATE Initiative , et al
Supplementary materials
Supplementary data.
This web only file has been produced by the BMJ Publishing Group from an electronic file supplied by the author(s) and has not been edited for content.
- Data supplement 1
AMJ and MC are joint senior authors.
Contributors DPA and AMJ led the conceptualisation of the study with contributions from MC and NM-B. AMJ, TD-S, ER, AU and NTHT adapted the study design with respect to the local vaccine rollouts. AD and WYM mapped and curated CPRD data. MC and NM-B developed code with methodological contributions advice from MTS-S and CP. DPA, MC, NTHT, TD-S, HMEN, XL, CR and AMJ clinically interpreted the results. NM-B, XL, AMJ and DPA wrote the first draft of the manuscript, and all authors read, revised and approved the final version. DPA and AMJ obtained the funding for this research. DPA is responsible for the overall content as guarantor: he accepts full responsibility for the work and the conduct of the study, had access to the data, and controlled the decision to publish.
Funding The research was supported by the National Institute for Health and Care Research (NIHR) Oxford Biomedical Research Centre (BRC). DPA is funded through a NIHR Senior Research Fellowship (Grant number SRF-2018–11-ST2-004). Funding to perform the study in the SIDIAP database was provided by the Real World Epidemiology (RWEpi) research group at IDIAPJGol. Costs of databases mapping to OMOP CDM were covered by the European Health Data and Evidence Network (EHDEN).
Patient and public involvement Patients and/or the public were not involved in the design, or conduct, or reporting or dissemination plans of this research.
Provenance and peer review Not commissioned; externally peer reviewed.
Supplemental material This content has been supplied by the author(s). It has not been vetted by BMJ Publishing Group Limited (BMJ) and may not have been peer-reviewed. Any opinions or recommendations discussed are solely those of the author(s) and are not endorsed by BMJ. BMJ disclaims all liability and responsibility arising from any reliance placed on the content. Where the content includes any translated material, BMJ does not warrant the accuracy and reliability of the translations (including but not limited to local regulations, clinical guidelines, terminology, drug names and drug dosages), and is not responsible for any error and/or omissions arising from translation and adaptation or otherwise.
Read the full text or download the PDF:
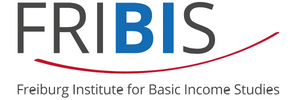
- WEF-FABI Online Seminar Series: Ubuntu, Social Contracts, UBI, WEF-Nexus, Social Protection, and Empirical Research
Mar 18, 2024 | News , Topical
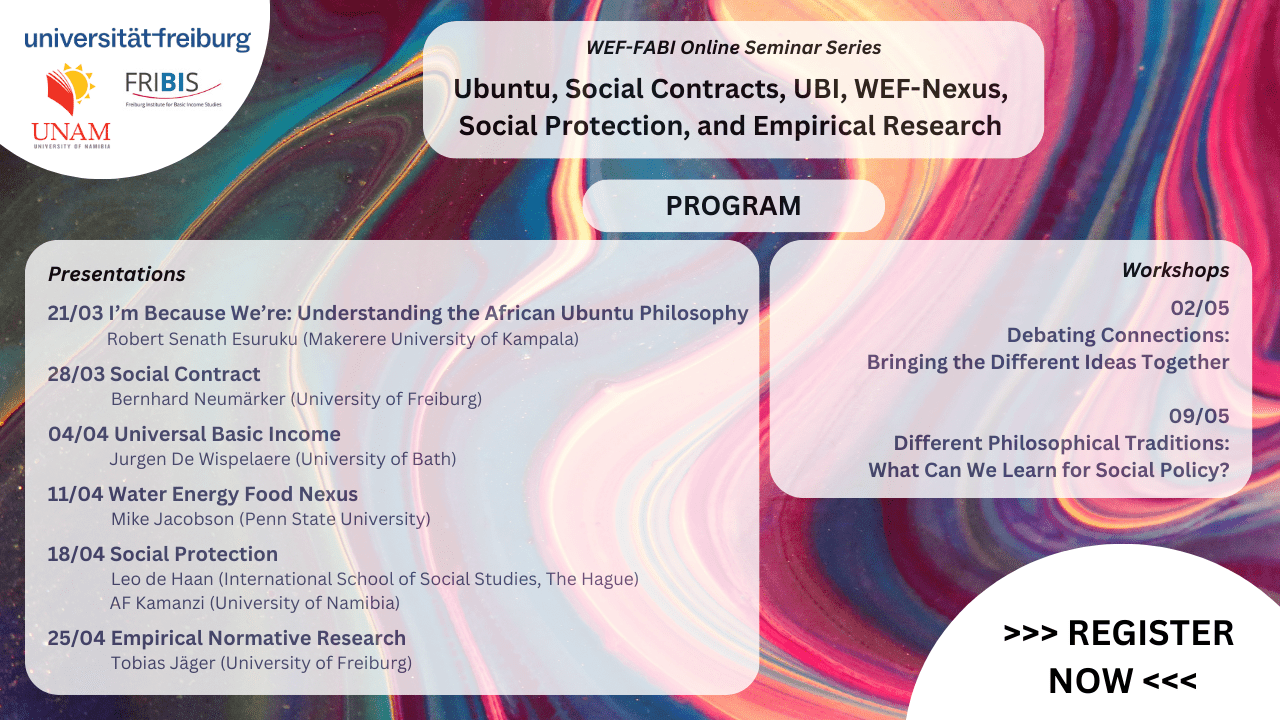
Together with our partners at UNAM the WEF-FABI Team will host an online seminar series on “Ubuntu, Social Contracts, UBI, Water-Energy-Food Nexus, Social Protection, and Empirical Research,” starting on 21/03/2024. With assorted guests, the series will create a path of interconnections and differences between these topics and ask how their elements fit together. We want to examine how Ubuntu can be used in social experiments. Finally, the workshop findings will be incorporated into joint work (e.g. joint papers or field studies).
“Ubuntu” is a bond of unity amongst the people of Africa (Asike 2016, p. 7). In this sense, ubuntu is a philosophy that justifies norms and deliberate rules. With that, it touches common ground with the tradition of the social contract theory. In our series, we will follow various questions connecting these two traditions of thought: What are the main positions in these philosophical traditions? What are the similarities/differences between ubuntu and social contract theory? How can the shared features of the two of them be connected? We will then confront philosophical insights with the idea of a universal basic income and further link the various strings to possible impacts on social policy interactions. We take a look at the trade-offs between different policies and sharpen our awareness of the water-energy-food nexus and social protection. Finally, we will see how we can use empirical methods to normative justify policies and contribute to solving trade-offs.
If you are interested in a workshop, see the dates below and join (register) through the registration form on the workshop eventpage. The presentations are free to join using the zoom link provided in the event.
All events will be held from 2 – 4 pm (CAT)
Presentations
21/03/2024 I’m Because We’re: Understanding the African Ubuntu Philosophy Robert Senath Esuruku (University of Makerere, Kampala)
28/03/2024 Social Contract Bernhard Neumärker (University of Freiburg)
04/04/2024 Universal Basic Income Jurgen De Wispelaere (University of Bath)
11/04/2024 Water-Energy-Food Nexus Mike Jacobson (Penn State University)
18/04/2024 Social Protection Leo de Haan (International School of Social Studies, The Hague) AF Kamanzi (University of Namibia)
25/04/2024 Empirical Normative Research Tobias Jäger (University of Freiburg)
02/05/2024 Debating Connections: Bringing the Different Ideas Together
09/05/2024 Different Philosophical Traditions: What Can We Learn for Social Policy?
Recent Posts
- Publication of two policy papers on the topic of empirical methods in basic income research
- Talk by Otto Lehto (NYU), 7th of March 2024: “Universal Basic Income (UBI) as a tool of adaptation and discovery”
- Thomas Straubhaar’s lecture now on YouTube: „Die 3-E des Bedingungslosen Grundeinkommens aus ökonomischer Sicht: einfach, effektiv und effizient”
- Competing perspectives: Bernhard Neumärker and Giacomo Corneo on the basic income on utopia.de
Our Newsletter
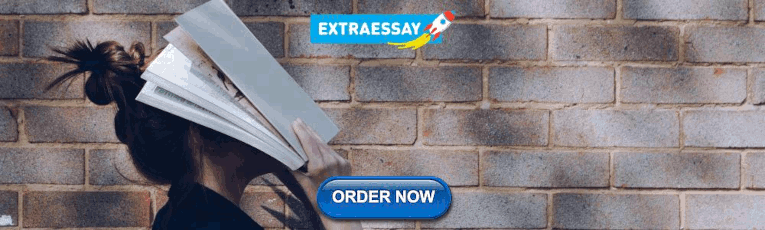
IMAGES
COMMENTS
A scientist gathering data for her research. Empirical research is research using empirical evidence.It is also a way of gaining knowledge by means of direct and indirect observation or experience. Empiricism values some research more than other kinds. Empirical evidence (the record of one's direct observations or experiences) can be analyzed quantitatively or qualitatively.
Empirical research is defined as any research where conclusions of the study is strictly drawn from concretely empirical evidence, and therefore "verifiable" evidence. This empirical evidence can be gathered using quantitative market research and qualitative market research methods. For example: A research is being conducted to find out if ...
Qualitative research -- an approach to documenting reality that relies on words and images as the primary data source (p. 33). Both quantitative and qualitative methods are empirical. If you can recognize that a research study is quantitative or qualitative study, then you have also recognized that it is empirical study.
Empirical research is defined as any study whose conclusions are exclusively derived from concrete, verifiable evidence. The term empirical basically means that it is guided by scientific experimentation and/or evidence. Likewise, a study is empirical when it uses real-world evidence in investigating its assertions.
empirical evidence, information gathered directly or indirectly through observation or experimentation that may be used to confirm or disconfirm a scientific theory or to help justify, or establish as reasonable, a person's belief in a given proposition. A belief may be said to be justified if there is sufficient evidence to make holding the ...
Empirical research is based on observed and measured phenomena and derives knowledge from actual experience rather than from theory or belief. How do you know if a study is empirical? Read the subheadings within the article, book, or report and look for a description of the research "methodology."
Description of the methodology or research design used to study this population or phenomena, including selection criteria, controls, and testing instruments (such as surveys); There are two basic research processes or methods in empirical research: quantitative methods and qualitative methods (see below for more about these methods).
Study on radiation transfer in human skin for cosmetics. Long-Term Mobile Phone Use and the Risk of Vestibular Schwannoma: A Danish Nationwide Cohort Study ... Strategies for Empirical Research in Writing is a particularly accessible approach to both qualitative and quantitative empirical research methods, helping novices appreciate the value ...
An empirical study reports the findings from a study that uses data derived from an actual experiment or observation. Key components of an empirical study: Abstract - Provides a brief overview of the research.; Introduction - The introduction contextualizes the research by providing a review of previous research on the topic.It also is the section where the hypothesis is stated.
The account is limited to individual, substantive, empirical, and quantitative research studies. Applied and basic/general research differ with respect to general aim. As defined here, whereas the aim in applied research is to generate knowledge to be used directly in specified professional/practical work or contexts, basic/general research is ...
Empirical research is a type of research methodology that makes use of verifiable evidence in order to arrive at research outcomes. In other words, this type of research relies solely on evidence obtained through observation or scientific data collection methods. Empirical research can be carried out using qualitative or quantitative ...
Empirical research is based on observed and measured phenomena and derives knowledge from actual experience rather than from theory or belief. How do you know if a study is empirical? Read the subheadings within the article, book, or report and look for a description of the research "methodology."
Share this content. Empirical research is research that is based on observation and measurement of phenomena, as directly experienced by the researcher. The data thus gathered may be compared against a theory or hypothesis, but the results are still based on real life experience. The data gathered is all primary data, although secondary data ...
Because empirical articles contain so many details about their studies, they tend to be longer. They also contain charts, tables, and other graphics to help display the data that was collected. Sources. College of Southern Maryland. CSM Library. (2018, Jan. 19). Empirical research article.
a "research paper" does not fit our definition of empirical research because doing so typically involves summarizing the analyses of other authors, not forming a new ... not continue your studies in research methods, by the end of this course you will have learned the basics of research, which means you will have more knowledge ...
An empirical research article reports the results of a study that uses data derived from actual observation or experimentation. Empirical research articles are examples of primary research. ... The introduction contains a literature review and the study's research hypothesis. Method. The method section outlines the research design, participants ...
An empirical study is one that is based on "observation, investigation, or experiment rather than on abstract reasoning, theoretical analysis, or speculation."*. Empirical studies should be divided into the following parts: abstract, introduction, method, results, discussion, and references. Typically these studies also include tables, figures ...
This is to add some meaning to the data for further elaboration. In short, data from the non-empirical study is theorised. While empirical research uses qualitative and quantitative methods, a researcher using the non-empirical method will use a lot of systematic reviews, meta-analysis, and critical studies to conclude the matter at hand.
The assessment of empirical studies provides real world effectiveness estimations that do not rely strongly on assumptions as do simulations in modeling. In this review, we summarize the current evidence from empirical studies on the comparative effectiveness of NPIs that have been implemented worldwide to control the current COVID-19 pandemic ...
Empirical research is published in books and in scholarly, peer-reviewed journals. However, most library databases do not offer straightforward ways to locate empirical research. Below are tips for some of Penn State's most popular Education and Behavioral/Social Sciences databases. If you need further help, contact a Librarian at your location.
In empirical study, conclusions of the study are drawn from concrete empirical evidence. This evidence is also referred to as "verifiable" evidence. This evidence is gathered either through quantitative market research or qualitative market research methods. An example of empirical analysis would be if a researcher was interested in finding ...
The Journal of Empirical Research on Human Research Ethics (JERHRE) is the only journal in the field of human research ethics dedicated exclusively to empirical research.Empirical knowledge translates ethical principles into procedures appropriate to specific cultures, contexts, and research topics. The journal's distinguished editorial and advisory board brings a range of expertise and ...
Empirical studies have revealed that growth mindset has positive effects on student motivation and academic performance [9,10]. Recent research has also shown that mindset is related to student outcomes and behaviors including academic achievement, engagement, and willingness to attempt new challenges [11,12]. Numerous studies have shown the ...
This research study enriches the Generative AI technology acceptance theory and extends the existing literature by introducing new adoption variables and stages specific to entrepreneurship. ... This research's empirical findings have important practical ramifications for entrepreneurs who want to use Generative AI technology, particularly ...
The results of the empirical study show that the equity concentration variable (i.e. Shareholding structure in Table 7), as a measure of corporate governance, has a significant negative ...
She is also a lecturer in the School of Law at Shihezi University. The interdisciplinary research experience makes her enthusiastically involved in research fields related to environmental law, especially green criminology, environmental justice, environmental regulations and management.
Objective To study the association between COVID-19 vaccination and the risk of post-COVID-19 cardiac and thromboembolic complications. Methods We conducted a staggered cohort study based on national vaccination campaigns using electronic health records from the UK, Spain and Estonia. Vaccine rollout was grouped into four stages with predefined enrolment periods. Each stage included all ...
Conclusion: Our empirical evaluation supports a recent theoretical functional connectivity framework. Future functional imaging studies need to comprehensively define the study-specific theoretical property of interest, the methodological property to assess the theoretical property, and the confounding property that may bias the conclusions.
Together with our partners at UNAM the WEF-FABI Team will host an online seminar series on "Ubuntu, Social Contracts, UBI, Water-Energy-Food Nexus, Social Protection, and Empirical Research," starting on 21/03/2024. With assorted guests, the series will create a path of interconnections and differences between these topics and ask how their elements fit together.