- Privacy Policy

Home » Tables in Research Paper – Types, Creating Guide and Examples
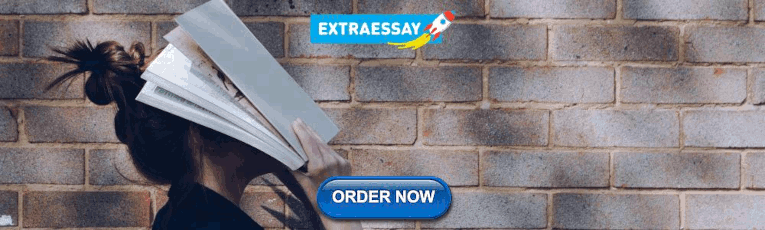
Tables in Research Paper – Types, Creating Guide and Examples
Table of Contents

Tables in Research Paper
Definition:
In Research Papers , Tables are a way of presenting data and information in a structured format. Tables can be used to summarize large amounts of data or to highlight important findings. They are often used in scientific or technical papers to display experimental results, statistical analyses, or other quantitative information.
Importance of Tables in Research Paper
Tables are an important component of a research paper as they provide a clear and concise presentation of data, statistics, and other information that support the research findings . Here are some reasons why tables are important in a research paper:
- Visual Representation : Tables provide a visual representation of data that is easy to understand and interpret. They help readers to quickly grasp the main points of the research findings and draw their own conclusions.
- Organize Data : Tables help to organize large amounts of data in a systematic and structured manner. This makes it easier for readers to identify patterns and trends in the data.
- Clarity and Accuracy : Tables allow researchers to present data in a clear and accurate manner. They can include precise numbers, percentages, and other information that may be difficult to convey in written form.
- Comparison: Tables allow for easy comparison between different data sets or groups. This makes it easier to identify similarities and differences, and to draw meaningful conclusions from the data.
- Efficiency: Tables allow for a more efficient use of space in the research paper. They can convey a large amount of information in a compact and concise format, which saves space and makes the research paper more readable.
Types of Tables in Research Paper
Most common Types of Tables in Research Paper are as follows:
- Descriptive tables : These tables provide a summary of the data collected in the study. They are usually used to present basic descriptive statistics such as means, medians, standard deviations, and frequencies.
- Comparative tables : These tables are used to compare the results of different groups or variables. They may be used to show the differences between two or more groups or to compare the results of different variables.
- Correlation tables: These tables are used to show the relationships between variables. They may show the correlation coefficients between variables, or they may show the results of regression analyses.
- Longitudinal tables : These tables are used to show changes in variables over time. They may show the results of repeated measures analyses or longitudinal regression analyses.
- Qualitative tables: These tables are used to summarize qualitative data such as interview transcripts or open-ended survey responses. They may present themes or categories that emerged from the data.
How to Create Tables in Research Paper
Here are the steps to create tables in a research paper:
- Plan your table: Determine the purpose of the table and the type of information you want to include. Consider the layout and format that will best convey your information.
- Choose a table format : Decide on the type of table you want to create. Common table formats include basic tables, summary tables, comparison tables, and correlation tables.
- Choose a software program : Use a spreadsheet program like Microsoft Excel or Google Sheets to create your table. These programs allow you to easily enter and manipulate data, format the table, and export it for use in your research paper.
- Input data: Enter your data into the spreadsheet program. Make sure to label each row and column clearly.
- Format the table : Apply formatting options such as font, font size, font color, cell borders, and shading to make your table more visually appealing and easier to read.
- Insert the table into your paper: Copy and paste the table into your research paper. Make sure to place the table in the appropriate location and refer to it in the text of your paper.
- Label the table: Give the table a descriptive title that clearly and accurately summarizes the contents of the table. Also, include a number and a caption that explains the table in more detail.
- Check for accuracy: Review the table for accuracy and make any necessary changes before submitting your research paper.
Examples of Tables in Research Paper
Examples of Tables in the Research Paper are as follows:
Table 1: Demographic Characteristics of Study Participants
Characteristic | N = 200 | % |
---|---|---|
Age (years) | ||
Mean (SD) | 35.2 (8.6) | |
Range | 21-57 | |
Gender | ||
Male | 92 | 46 |
Female | 108 | 54 |
Education | ||
Less than high school | 20 | 10 |
High school graduate | 60 | 30 |
Some college | 70 | 35 |
Bachelor’s degree or higher | 50 | 25 |
This table shows the demographic characteristics of 200 participants in a research study. The table includes information about age, gender, and education level. The mean age of the participants was 35.2 years with a standard deviation of 8.6 years, and the age range was between 21 and 57 years. The table also shows that 46% of the participants were male and 54% were female. In terms of education, 10% of the participants had less than a high school education, 30% were high school graduates, 35% had some college education, and 25% had a bachelor’s degree or higher.
Table 2: Summary of Key Findings
Variable | Group 1 | Group 2 | Group 3 |
---|---|---|---|
Mean score | 76.3 | 84.7 | 72.1 |
Standard deviation | 5.2 | 6.9 | 4.8 |
t-value | -2.67* | 1.89 | -1.24 |
p-value | < 0.01 | 0.06 | 0.22 |
This table summarizes the key findings of a study comparing three different groups on a particular variable. The table shows the mean score, standard deviation, t-value, and p-value for each group. The asterisk next to the t-value for Group 1 indicates that the difference between Group 1 and the other groups was statistically significant at p < 0.01, while the differences between Group 2 and Group 3 were not statistically significant.
Purpose of Tables in Research Paper
The primary purposes of including tables in a research paper are:
- To present data: Tables are an effective way to present large amounts of data in a clear and organized manner. Researchers can use tables to present numerical data, survey results, or other types of data that are difficult to represent in text.
- To summarize data: Tables can be used to summarize large amounts of data into a concise and easy-to-read format. Researchers can use tables to summarize the key findings of their research, such as descriptive statistics or the results of regression analyses.
- To compare data : Tables can be used to compare data across different variables or groups. Researchers can use tables to compare the characteristics of different study populations or to compare the results of different studies on the same topic.
- To enhance the readability of the paper: Tables can help to break up long sections of text and make the paper more visually appealing. By presenting data in a table, researchers can help readers to quickly identify the most important information and understand the key findings of the study.
Advantages of Tables in Research Paper
Some of the advantages of using tables in research papers include:
- Clarity : Tables can present data in a way that is easy to read and understand. They can help readers to quickly and easily identify patterns, trends, and relationships in the data.
- Efficiency: Tables can save space and reduce the need for lengthy explanations or descriptions of the data in the main body of the paper. This can make the paper more concise and easier to read.
- Organization: Tables can help to organize large amounts of data in a logical and meaningful way. This can help to reduce confusion and make it easier for readers to navigate the data.
- Comparison : Tables can be useful for comparing data across different groups, variables, or time periods. This can help to highlight similarities, differences, and changes over time.
- Visualization : Tables can also be used to visually represent data, making it easier for readers to see patterns and trends. This can be particularly useful when the data is complex or difficult to understand.
About the author
Muhammad Hassan
Researcher, Academic Writer, Web developer
You may also like

Research Approach – Types Methods and Examples

APA Research Paper Format – Example, Sample and...

Implications in Research – Types, Examples and...

Research Paper – Structure, Examples and Writing...

Research Methodology – Types, Examples and...

Appendices – Writing Guide, Types and Examples
- Research Process
- Manuscript Preparation
- Manuscript Review
- Publication Process
- Publication Recognition
- Language Editing Services
- Translation Services
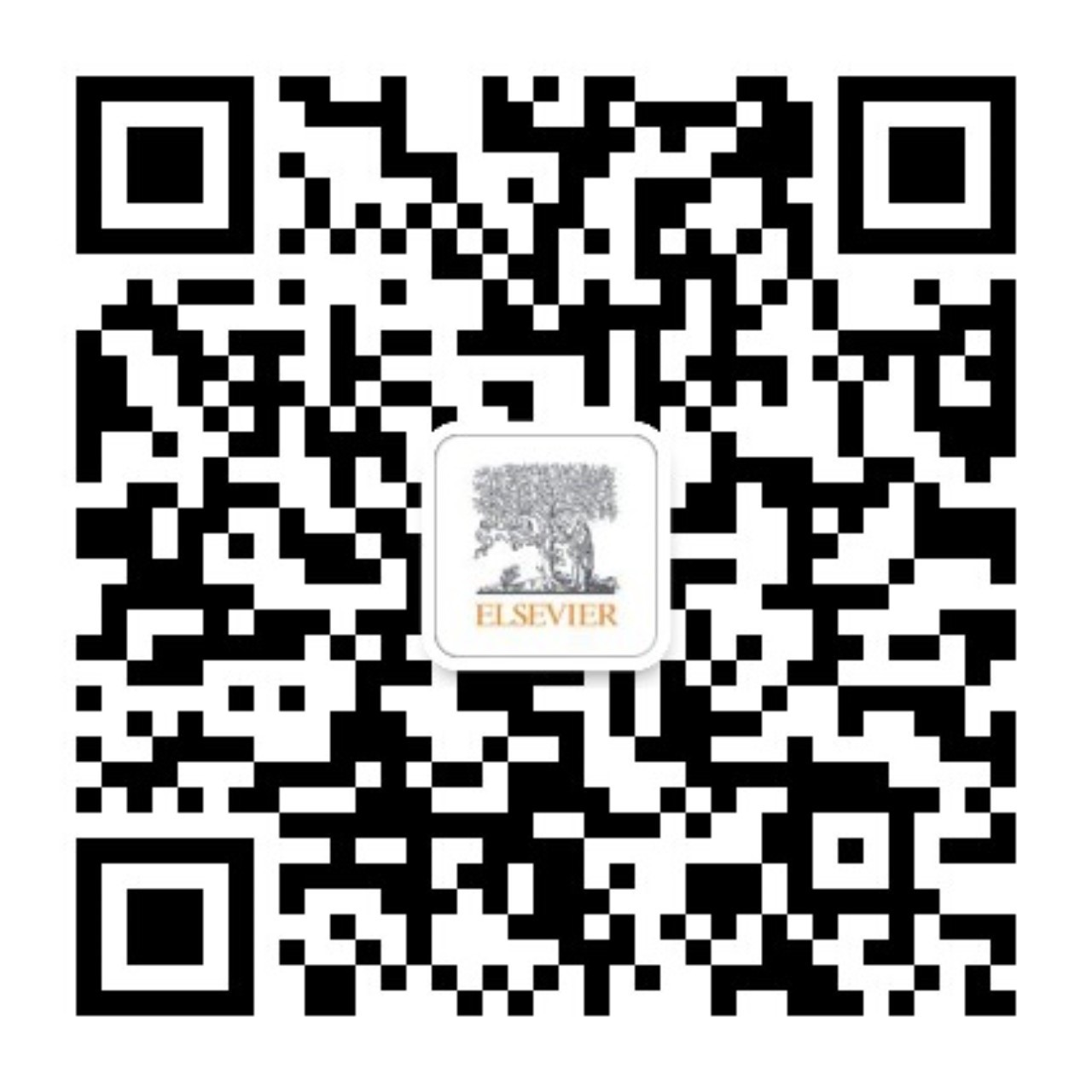
How to Use Tables and Figures effectively in Research Papers
- 3 minute read
- 51.6K views
Table of Contents
Data is the most important component of any research. It needs to be presented effectively in a paper to ensure that readers understand the key message in the paper. Figures and tables act as concise tools for clear presentation . Tables display information arranged in rows and columns in a grid-like format, while figures convey information visually, and take the form of a graph, diagram, chart, or image. Be it to compare the rise and fall of GDPs among countries over the years or to understand how COVID-19 has impacted incomes all over the world, tables and figures are imperative to convey vital findings accurately.
So, what are some of the best practices to follow when creating meaningful and attractive tables and figures? Here are some tips on how best to present tables and figures in a research paper.
Guidelines for including tables and figures meaningfully in a paper:
- Self-explanatory display items: Sometimes, readers, reviewers and journal editors directly go to the tables and figures before reading the entire text. So, the tables need to be well organized and self-explanatory.
- Avoidance of repetition: Tables and figures add clarity to the research. They complement the research text and draw attention to key points. They can be used to highlight the main points of the paper, but values should not be repeated as it defeats the very purpose of these elements.
- Consistency: There should be consistency in the values and figures in the tables and figures and the main text of the research paper.
- Informative titles: Titles should be concise and describe the purpose and content of the table. It should draw the reader’s attention towards the key findings of the research. Column heads, axis labels, figure labels, etc., should also be appropriately labelled.
- Adherence to journal guidelines: It is important to follow the instructions given in the target journal regarding the preparation and presentation of figures and tables, style of numbering, titles, image resolution, file formats, etc.
Now that we know how to go about including tables and figures in the manuscript, let’s take a look at what makes tables and figures stand out and create impact.
How to present data in a table?
For effective and concise presentation of data in a table, make sure to:
- Combine repetitive tables: If the tables have similar content, they should be organized into one.
- Divide the data: If there are large amounts of information, the data should be divided into categories for more clarity and better presentation. It is necessary to clearly demarcate the categories into well-structured columns and sub-columns.
- Keep only relevant data: The tables should not look cluttered. Ensure enough spacing.
Example of table presentation in a research paper
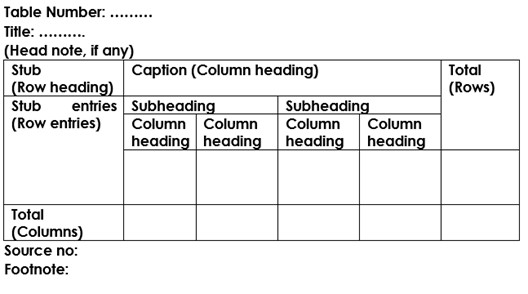
For comprehensible and engaging presentation of figures:
- Ensure clarity: All the parts of the figure should be clear. Ensure the use of a standard font, legible labels, and sharp images.
- Use appropriate legends: They make figures effective and draw attention towards the key message.
- Make it precise: There should be correct use of scale bars in images and maps, appropriate units wherever required, and adequate labels and legends.
It is important to get tables and figures correct and precise for your research paper to convey your findings accurately and clearly. If you are confused about how to suitably present your data through tables and figures, do not worry. Elsevier Author Services are well-equipped to guide you through every step to ensure that your manuscript is of top-notch quality.
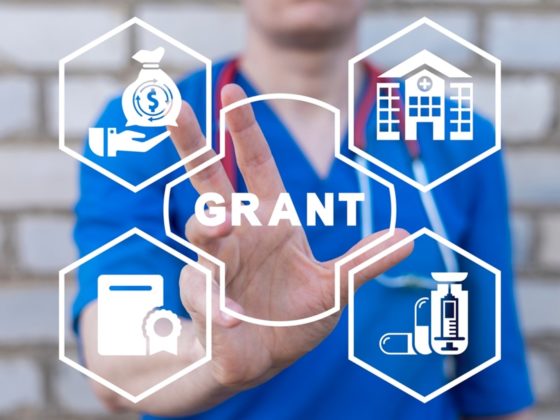
How to Write an Effective Problem Statement for Your Research Paper
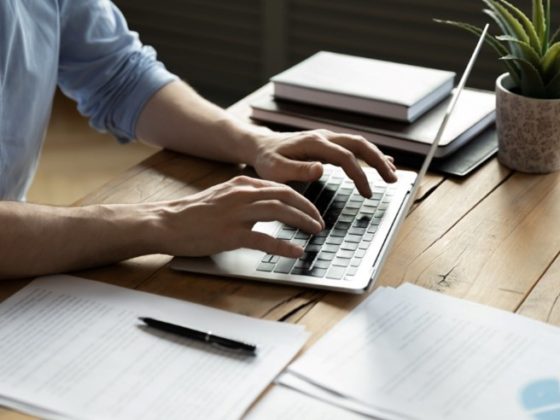
What is the Background of a Study and How Should it be Written?
You may also like.
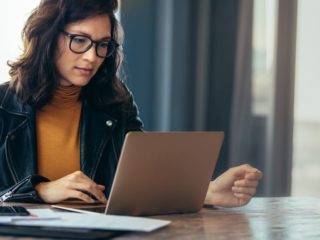
Submission 101: What format should be used for academic papers?
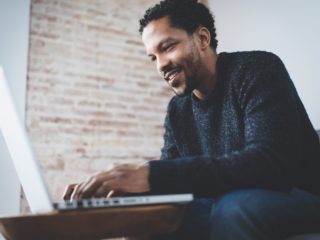
Page-Turner Articles are More Than Just Good Arguments: Be Mindful of Tone and Structure!
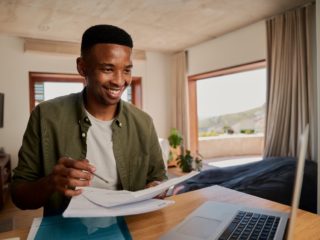
A Must-see for Researchers! How to Ensure Inclusivity in Your Scientific Writing
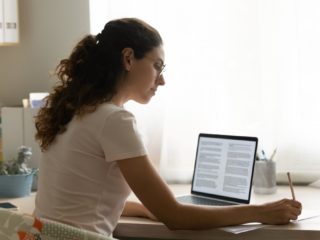
Make Hook, Line, and Sinker: The Art of Crafting Engaging Introductions
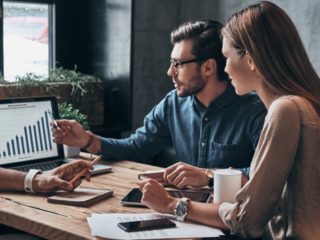
Can Describing Study Limitations Improve the Quality of Your Paper?
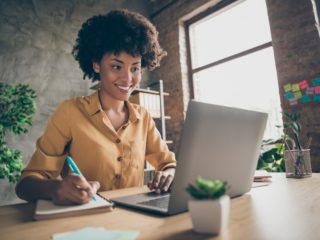
A Guide to Crafting Shorter, Impactful Sentences in Academic Writing
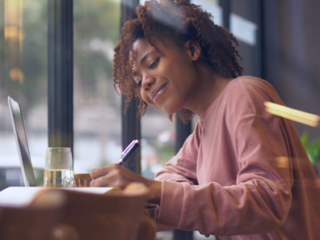
6 Steps to Write an Excellent Discussion in Your Manuscript
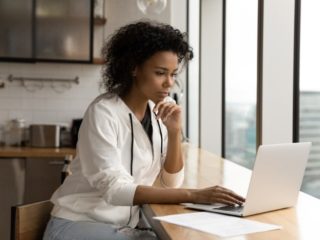
How to Write Clear and Crisp Civil Engineering Papers? Here are 5 Key Tips to Consider
Input your search keywords and press Enter.
Purdue Online Writing Lab Purdue OWL® College of Liberal Arts
Tables and Figures
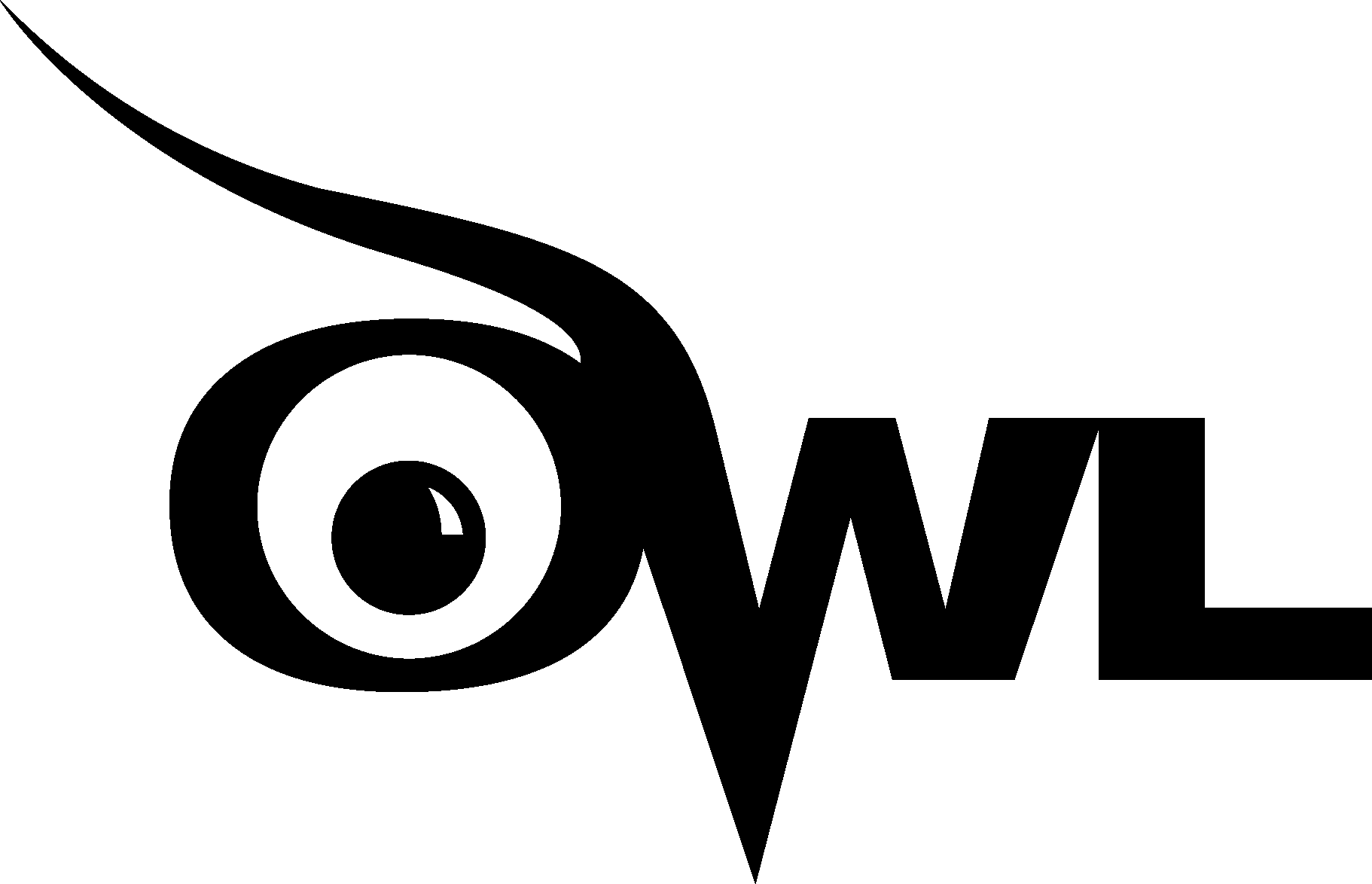
Welcome to the Purdue OWL
This page is brought to you by the OWL at Purdue University. When printing this page, you must include the entire legal notice.
Copyright ©1995-2018 by The Writing Lab & The OWL at Purdue and Purdue University. All rights reserved. This material may not be published, reproduced, broadcast, rewritten, or redistributed without permission. Use of this site constitutes acceptance of our terms and conditions of fair use.
Note: This page reflects the latest version of the APA Publication Manual (i.e., APA 7), which released in October 2019. The equivalent resources for the older APA 6 style can be found at this page as well as at this page (our old resources covered the material on this page on two separate pages).
The purpose of tables and figures in documents is to enhance your readers' understanding of the information in the document; usually, large amounts of information can be communicated more efficiently in tables or figures. Tables are any graphic that uses a row and column structure to organize information, whereas figures include any illustration or image other than a table.
General guidelines
Visual material such as tables and figures can be used quickly and efficiently to present a large amount of information to an audience, but visuals must be used to assist communication, not to use up space, or disguise marginally significant results behind a screen of complicated statistics. Ask yourself this question first: Is the table or figure necessary? For example, it is better to present simple descriptive statistics in the text, not in a table.
Relation of Tables or Figures and Text
Because tables and figures supplement the text, refer in the text to all tables and figures used and explain what the reader should look for when using the table or figure. Focus only on the important point the reader should draw from them, and leave the details for the reader to examine on their own.
Documentation
If you are using figures, tables and/or data from other sources, be sure to gather all the information you will need to properly document your sources.
Integrity and Independence
Each table and figure must be intelligible without reference to the text, so be sure to include an explanation of every abbreviation (except the standard statistical symbols and abbreviations).
Organization, Consistency, and Coherence
Number all tables sequentially as you refer to them in the text (Table 1, Table 2, etc.), likewise for figures (Figure 1, Figure 2, etc.). Abbreviations, terminology, and probability level values must be consistent across tables and figures in the same article. Likewise, formats, titles, and headings must be consistent. Do not repeat the same data in different tables.
Data in a table that would require only two or fewer columns and rows should be presented in the text. More complex data is better presented in tabular format. In order for quantitative data to be presented clearly and efficiently, it must be arranged logically, e.g. data to be compared must be presented next to one another (before/after, young/old, male/female, etc.), and statistical information (means, standard deviations, N values) must be presented in separate parts of the table. If possible, use canonical forms (such as ANOVA, regression, or correlation) to communicate your data effectively.

A generic example of a table with multiple notes formatted in APA 7 style.
Elements of Tables
Number all tables with Arabic numerals sequentially. Do not use suffix letters (e.g. Table 3a, 3b, 3c); instead, combine the related tables. If the manuscript includes an appendix with tables, identify them with capital letters and Arabic numerals (e.g. Table A1, Table B2).
Like the title of the paper itself, each table must have a clear and concise title. Titles should be written in italicized title case below the table number, with a blank line between the number and the title. When appropriate, you may use the title to explain an abbreviation parenthetically.
Comparison of Median Income of Adopted Children (AC) v. Foster Children (FC)
Keep headings clear and brief. The heading should not be much wider than the widest entry in the column. Use of standard abbreviations can aid in achieving that goal. There are several types of headings:
- Stub headings describe the lefthand column, or stub column , which usually lists major independent variables.
- Column headings describe entries below them, applying to just one column.
- Column spanners are headings that describe entries below them, applying to two or more columns which each have their own column heading. Column spanners are often stacked on top of column headings and together are called decked heads .
- Table Spanners cover the entire width of the table, allowing for more divisions or combining tables with identical column headings. They are the only type of heading that may be plural.
All columns must have headings, written in sentence case and using singular language (Item rather than Items) unless referring to a group (Men, Women). Each column’s items should be parallel (i.e., every item in a column labeled “%” should be a percentage and does not require the % symbol, since it’s already indicated in the heading). Subsections within the stub column can be shown by indenting headings rather than creating new columns:
Chemical Bonds
Ionic
Covalent
Metallic
The body is the main part of the table, which includes all the reported information organized in cells (intersections of rows and columns). Entries should be center aligned unless left aligning them would make them easier to read (longer entries, usually). Word entries in the body should use sentence case. Leave cells blank if the element is not applicable or if data were not obtained; use a dash in cells and a general note if it is necessary to explain why cells are blank. In reporting the data, consistency is key: Numerals should be expressed to a consistent number of decimal places that is determined by the precision of measurement. Never change the unit of measurement or the number of decimal places in the same column.
There are three types of notes for tables: general, specific, and probability notes. All of them must be placed below the table in that order.
General notes explain, qualify or provide information about the table as a whole. Put explanations of abbreviations, symbols, etc. here.
Example: Note . The racial categories used by the US Census (African-American, Asian American, Latinos/-as, Native-American, and Pacific Islander) have been collapsed into the category “non-White.” E = excludes respondents who self-identified as “White” and at least one other “non-White” race.
Specific notes explain, qualify or provide information about a particular column, row, or individual entry. To indicate specific notes, use superscript lowercase letters (e.g. a , b , c ), and order the superscripts from left to right, top to bottom. Each table’s first footnote must be the superscript a .
a n = 823. b One participant in this group was diagnosed with schizophrenia during the survey.
Probability notes provide the reader with the results of the tests for statistical significance. Asterisks indicate the values for which the null hypothesis is rejected, with the probability ( p value) specified in the probability note. Such notes are required only when relevant to the data in the table. Consistently use the same number of asterisks for a given alpha level throughout your paper.
* p < .05. ** p < .01. *** p < .001
If you need to distinguish between two-tailed and one-tailed tests in the same table, use asterisks for two-tailed p values and an alternate symbol (such as daggers) for one-tailed p values.
* p < .05, two-tailed. ** p < .01, two-tailed. † p <.05, one-tailed. †† p < .01, one-tailed.
Borders
Tables should only include borders and lines that are needed for clarity (i.e., between elements of a decked head, above column spanners, separating total rows, etc.). Do not use vertical borders, and do not use borders around each cell. Spacing and strict alignment is typically enough to clarify relationships between elements.

Example of a table in the text of an APA 7 paper. Note the lack of vertical borders.
Tables from Other Sources
If using tables from an external source, copy the structure of the original exactly, and cite the source in accordance with APA style .
Table Checklist
(Taken from the Publication Manual of the American Psychological Association , 7th ed., Section 7.20)
- Is the table necessary?
- Does it belong in the print and electronic versions of the article, or can it go in an online supplemental file?
- Are all comparable tables presented consistently?
- Are all tables numbered with Arabic numerals in the order they are mentioned in the text? Is the table number bold and left-aligned?
- Are all tables referred to in the text?
- Is the title brief but explanatory? Is it presented in italicized title case and left-aligned?
- Does every column have a column heading? Are column headings centered?
- Are all abbreviations; special use of italics, parentheses, and dashes; and special symbols explained?
- Are the notes organized according to the convention of general, specific, probability?
- Are table borders correctly used (top and bottom of table, beneath column headings, above table spanners)?
- Does the table use correct line spacing (double for the table number, title, and notes; single, one and a half, or double for the body)?
- Are entries in the left column left-aligned beneath the centered stub heading? Are all other column headings and cell entries centered?
- Are confidence intervals reported for all major point estimates?
- Are all probability level values correctly identified, and are asterisks attached to the appropriate table entries? Is a probability level assigned the same number of asterisks in all the tables in the same document?
- If the table or its data are from another source, is the source properly cited? Is permission necessary to reproduce the table?
Figures include all graphical displays of information that are not tables. Common types include graphs, charts, drawings, maps, plots, and photos. Just like tables, figures should supplement the text and should be both understandable on their own and referenced fully in the text. This section details elements of formatting writers must use when including a figure in an APA document, gives an example of a figure formatted in APA style, and includes a checklist for formatting figures.
Preparing Figures
In preparing figures, communication and readability must be the ultimate criteria. Avoid the temptation to use the special effects available in most advanced software packages. While three-dimensional effects, shading, and layered text may look interesting to the author, overuse, inconsistent use, and misuse may distort the data, and distract or even annoy readers. Design properly done is inconspicuous, almost invisible, because it supports communication. Design improperly, or amateurishly, done draws the reader’s attention from the data, and makes him or her question the author’s credibility. Line drawings are usually a good option for readability and simplicity; for photographs, high contrast between background and focal point is important, as well as cropping out extraneous detail to help the reader focus on the important aspects of the photo.
Parts of a Figure
All figures that are part of the main text require a number using Arabic numerals (Figure 1, Figure 2, etc.). Numbers are assigned based on the order in which figures appear in the text and are bolded and left aligned.
Under the number, write the title of the figure in italicized title case. The title should be brief, clear, and explanatory, and both the title and number should be double spaced.
The image of the figure is the body, and it is positioned underneath the number and title. The image should be legible in both size and resolution; fonts should be sans serif, consistently sized, and between 8-14 pt. Title case should be used for axis labels and other headings; descriptions within figures should be in sentence case. Shading and color should be limited for clarity; use patterns along with color and check contrast between colors with free online checkers to ensure all users (people with color vision deficiencies or readers printing in grayscale, for instance) can access the content. Gridlines and 3-D effects should be avoided unless they are necessary for clarity or essential content information.
Legends, or keys, explain symbols, styles, patterns, shading, or colors in the image. Words in the legend should be in title case; legends should go within or underneath the image rather than to the side. Not all figures will require a legend.
Notes clarify the content of the figure; like tables, notes can be general, specific, or probability. General notes explain units of measurement, symbols, and abbreviations, or provide citation information. Specific notes identify specific elements using superscripts; probability notes explain statistical significance of certain values.

A generic example of a figure formatted in APA 7 style.
Figure Checklist
(Taken from the Publication Manual of the American Psychological Association , 7 th ed., Section 7.35)
- Is the figure necessary?
- Does the figure belong in the print and electronic versions of the article, or is it supplemental?
- Is the figure simple, clean, and free of extraneous detail?
- Is the figure title descriptive of the content of the figure? Is it written in italic title case and left aligned?
- Are all elements of the figure clearly labeled?
- Are the magnitude, scale, and direction of grid elements clearly labeled?
- Are parallel figures or equally important figures prepared according to the same scale?
- Are the figures numbered consecutively with Arabic numerals? Is the figure number bold and left aligned?
- Has the figure been formatted properly? Is the font sans serif in the image portion of the figure and between sizes 8 and 14?
- Are all abbreviations and special symbols explained?
- If the figure has a legend, does it appear within or below the image? Are the legend’s words written in title case?
- Are the figure notes in general, specific, and probability order? Are they double-spaced, left aligned, and in the same font as the paper?
- Are all figures mentioned in the text?
- Has written permission for print and electronic reuse been obtained? Is proper credit given in the figure caption?
- Have all substantive modifications to photographic images been disclosed?
- Are the figures being submitted in a file format acceptable to the publisher?
- Have the files been produced at a sufficiently high resolution to allow for accurate reproduction?

Effective Use of Tables and Figures in Research Papers
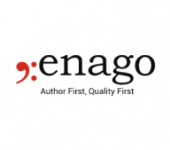
Research papers are often based on copious amounts of data that can be summarized and easily read through tables and graphs. When writing a research paper , it is important for data to be presented to the reader in a visually appealing way. The data in figures and tables, however, should not be a repetition of the data found in the text. There are many ways of presenting data in tables and figures, governed by a few simple rules. An APA research paper and MLA research paper both require tables and figures, but the rules around them are different. When writing a research paper, the importance of tables and figures cannot be underestimated. How do you know if you need a table or figure? The rule of thumb is that if you cannot present your data in one or two sentences, then you need a table .
Using Tables
Tables are easily created using programs such as Excel. Tables and figures in scientific papers are wonderful ways of presenting data. Effective data presentation in research papers requires understanding your reader and the elements that comprise a table. Tables have several elements, including the legend, column titles, and body. As with academic writing, it is also just as important to structure tables so that readers can easily understand them. Tables that are disorganized or otherwise confusing will make the reader lose interest in your work.
- Title: Tables should have a clear, descriptive title, which functions as the “topic sentence” of the table. The titles can be lengthy or short, depending on the discipline.
- Column Titles: The goal of these title headings is to simplify the table. The reader’s attention moves from the title to the column title sequentially. A good set of column titles will allow the reader to quickly grasp what the table is about.
- Table Body: This is the main area of the table where numerical or textual data is located. Construct your table so that elements read from up to down, and not across.
Related: Done organizing your research data effectively in tables? Check out this post on tips for citing tables in your manuscript now!
The placement of figures and tables should be at the center of the page. It should be properly referenced and ordered in the number that it appears in the text. In addition, tables should be set apart from the text. Text wrapping should not be used. Sometimes, tables and figures are presented after the references in selected journals.
Using Figures
Figures can take many forms, such as bar graphs, frequency histograms, scatterplots, drawings, maps, etc. When using figures in a research paper, always think of your reader. What is the easiest figure for your reader to understand? How can you present the data in the simplest and most effective way? For instance, a photograph may be the best choice if you want your reader to understand spatial relationships.
- Figure Captions: Figures should be numbered and have descriptive titles or captions. The captions should be succinct enough to understand at the first glance. Captions are placed under the figure and are left justified.
- Image: Choose an image that is simple and easily understandable. Consider the size, resolution, and the image’s overall visual attractiveness.
- Additional Information: Illustrations in manuscripts are numbered separately from tables. Include any information that the reader needs to understand your figure, such as legends.
Common Errors in Research Papers
Effective data presentation in research papers requires understanding the common errors that make data presentation ineffective. These common mistakes include using the wrong type of figure for the data. For instance, using a scatterplot instead of a bar graph for showing levels of hydration is a mistake. Another common mistake is that some authors tend to italicize the table number. Remember, only the table title should be italicized . Another common mistake is failing to attribute the table. If the table/figure is from another source, simply put “ Note. Adapted from…” underneath the table. This should help avoid any issues with plagiarism.
Using tables and figures in research papers is essential for the paper’s readability. The reader is given a chance to understand data through visual content. When writing a research paper, these elements should be considered as part of good research writing. APA research papers, MLA research papers, and other manuscripts require visual content if the data is too complex or voluminous. The importance of tables and graphs is underscored by the main purpose of writing, and that is to be understood.
Frequently Asked Questions
"Consider the following points when creating figures for research papers: Determine purpose: Clarify the message or information to be conveyed. Choose figure type: Select the appropriate type for data representation. Prepare and organize data: Collect and arrange accurate and relevant data. Select software: Use suitable software for figure creation and editing. Design figure: Focus on clarity, labeling, and visual elements. Create the figure: Plot data or generate the figure using the chosen software. Label and annotate: Clearly identify and explain all elements in the figure. Review and revise: Verify accuracy, coherence, and alignment with the paper. Format and export: Adjust format to meet publication guidelines and export as suitable file."
"To create tables for a research paper, follow these steps: 1) Determine the purpose and information to be conveyed. 2) Plan the layout, including rows, columns, and headings. 3) Use spreadsheet software like Excel to design and format the table. 4) Input accurate data into cells, aligning it logically. 5) Include column and row headers for context. 6) Format the table for readability using consistent styles. 7) Add a descriptive title and caption to summarize and provide context. 8) Number and reference the table in the paper. 9) Review and revise for accuracy and clarity before finalizing."
"Including figures in a research paper enhances clarity and visual appeal. Follow these steps: Determine the need for figures based on data trends or to explain complex processes. Choose the right type of figure, such as graphs, charts, or images, to convey your message effectively. Create or obtain the figure, properly citing the source if needed. Number and caption each figure, providing concise and informative descriptions. Place figures logically in the paper and reference them in the text. Format and label figures clearly for better understanding. Provide detailed figure captions to aid comprehension. Cite the source for non-original figures or images. Review and revise figures for accuracy and consistency."
"Research papers use various types of tables to present data: Descriptive tables: Summarize main data characteristics, often presenting demographic information. Frequency tables: Display distribution of categorical variables, showing counts or percentages in different categories. Cross-tabulation tables: Explore relationships between categorical variables by presenting joint frequencies or percentages. Summary statistics tables: Present key statistics (mean, standard deviation, etc.) for numerical variables. Comparative tables: Compare different groups or conditions, displaying key statistics side by side. Correlation or regression tables: Display results of statistical analyses, such as coefficients and p-values. Longitudinal or time-series tables: Show data collected over multiple time points with columns for periods and rows for variables/subjects. Data matrix tables: Present raw data or matrices, common in experimental psychology or biology. Label tables clearly, include titles, and use footnotes or captions for explanations."

Enago is a very useful site. It covers nearly all topics of research writing and publishing in a simple, clear, attractive way. Though I’m a journal editor having much knowledge and training in these issues, I always find something new in this site. Thank you
“Thank You, your contents really help me :)”
Rate this article Cancel Reply
Your email address will not be published.
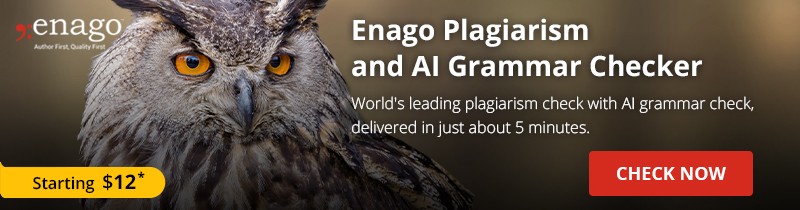
Enago Academy's Most Popular Articles
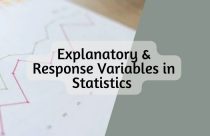
- Reporting Research
Explanatory & Response Variable in Statistics — A quick guide for early career researchers!
Often researchers have a difficult time choosing the parameters and variables (like explanatory and response…
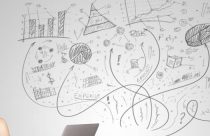
- Manuscript Preparation
- Publishing Research
How to Use Creative Data Visualization Techniques for Easy Comprehension of Qualitative Research
“A picture is worth a thousand words!”—an adage used so often stands true even whilst…
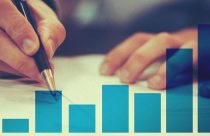
- Figures & Tables
Effective Use of Statistics in Research – Methods and Tools for Data Analysis
Remember that impending feeling you get when you are asked to analyze your data! Now…
- Old Webinars
- Webinar Mobile App
SCI中稿技巧: 提升研究数据的说服力
如何寻找原创研究课题 快速定位目标文献的有效搜索策略 如何根据期刊指南准备手稿的对应部分 论文手稿语言润色实用技巧分享,快速提高论文质量
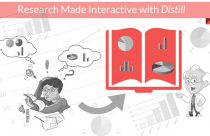
Distill: A Journal With Interactive Images for Machine Learning Research
Research is a wide and extensive field of study. This field has welcomed a plethora…
Explanatory & Response Variable in Statistics — A quick guide for early career…
How to Create and Use Gantt Charts

Sign-up to read more
Subscribe for free to get unrestricted access to all our resources on research writing and academic publishing including:
- 2000+ blog articles
- 50+ Webinars
- 10+ Expert podcasts
- 50+ Infographics
- 10+ Checklists
- Research Guides
We hate spam too. We promise to protect your privacy and never spam you.
- Industry News
- AI in Academia
- Promoting Research
- Career Corner
- Diversity and Inclusion
- Infographics
- Expert Video Library
- Other Resources
- Enago Learn
- Upcoming & On-Demand Webinars
- Peer-Review Week 2023
- Open Access Week 2023
- Conference Videos
- Enago Report
- Journal Finder
- Enago Plagiarism & AI Grammar Check
- Editing Services
- Publication Support Services
- Research Impact
- Translation Services
- Publication solutions
- AI-Based Solutions
- Thought Leadership
- Call for Articles
- Call for Speakers
- Author Training
- Edit Profile
I am looking for Editing/ Proofreading services for my manuscript Tentative date of next journal submission:
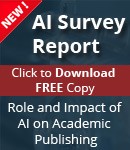
In your opinion, what is the most effective way to improve integrity in the peer review process?
- SpringerLink shop
Figures and tables
Figures and tables (display items) are often the quickest way to communicate large amounts of complex information that would be complicated to explain in text.
Many readers will only look at your display items without reading the main text of your manuscript. Therefore, ensure your display items can stand alone from the text and communicate clearly your most significant results.
Display items are also important for attracting readers to your work. Well designed and attractive display items will hold the interest of readers, compel them to take time to understand a figure and can even entice them to read your full manuscript.
Finally, high-quality display items give your work a professional appearance . Readers will assume that a professional-looking manuscript contains good quality science. Thus readers may be more likely to trust your results and your interpretation of those results.
When deciding which of your results to present as display items consider the following questions:
- Are there any data that readers might rather see as a display item rather than text?
- Do your figures supplement the text and not just repeat what you have already stated?
- Have you put data into a table that could easily be explained in the text such as simple statistics or p values?
Tables are a concise and effective way to present large amounts of data. You should design them carefully so that you clearly communicate your results to busy researchers.
The following is an example of a well-designed table:
- Clear and concise legend/caption
- Data divided into categories for clarity
- Sufficient spacing between columns and rows
- Units are provided
- Font type and size are legible
Educational resources and simple solutions for your research journey
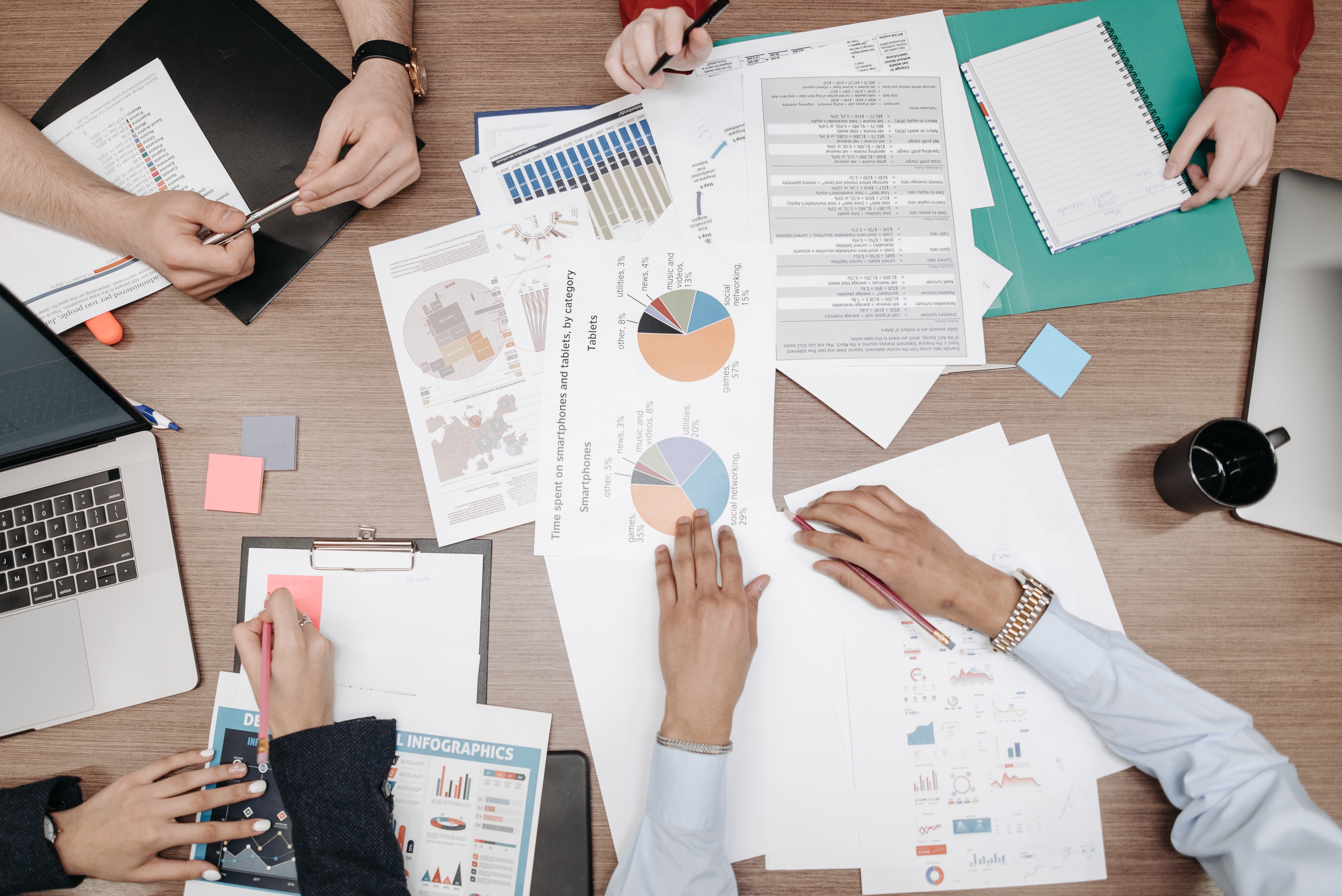
Tables and Figures in Research Papers: What Should you Use?
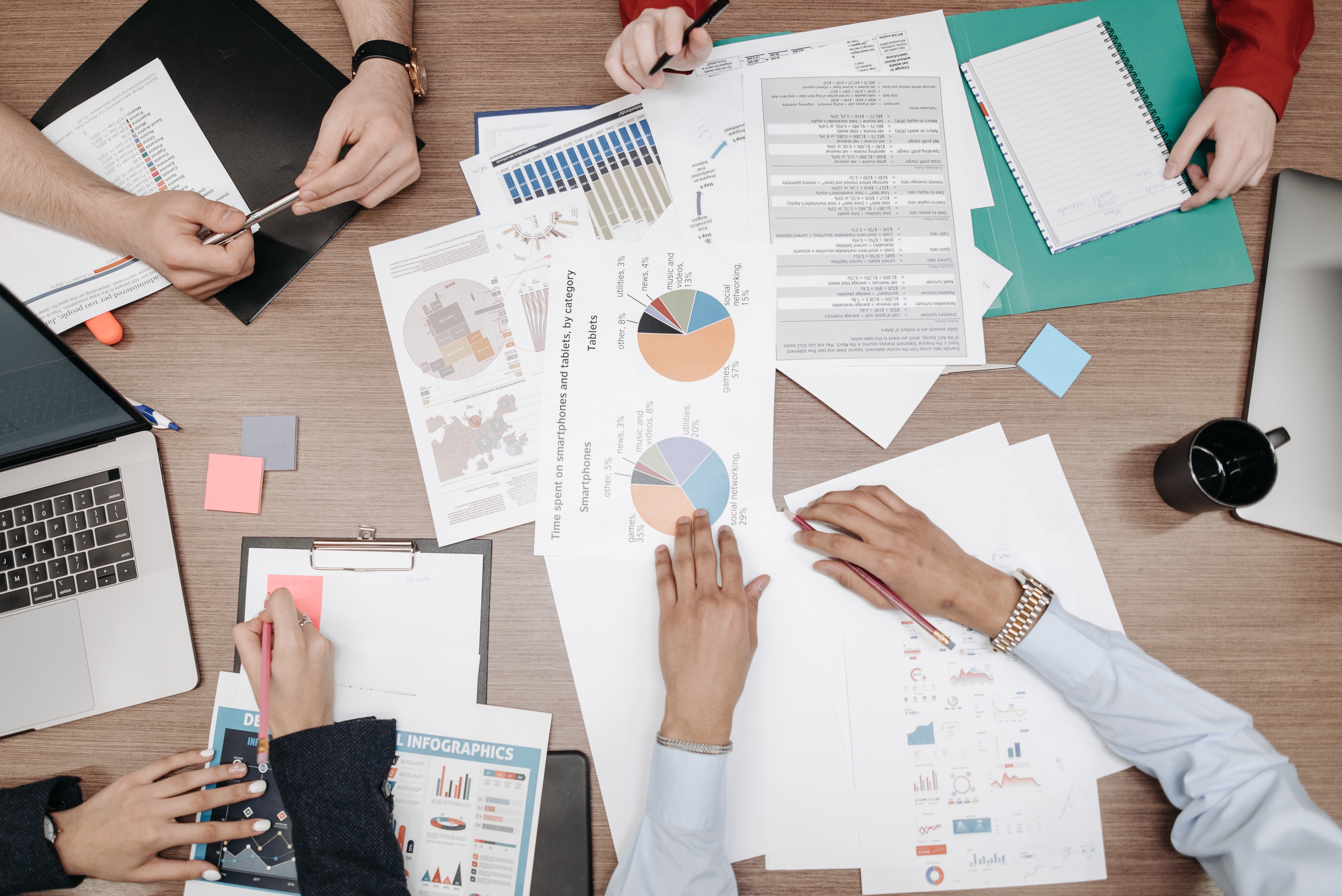
Researchers often use tables and figures in their research paper as visual representations to convey data in a simple way. Tables and figures in research papers not only enable readers to understand complex data at a glance but they also help create better engagement in one’s research. Instead of having to wade through dense paragraphs of text, readers of your research are able to quickly and easily identify patterns, gather important information, and understand interactions between data points with tables and figures.
While there are many kinds of visual tools that students and researchers can employ to explain the approach, methodology, research process and conclusion of their research, deciding whether to use a table, graph or a visual, and what kind – isn’t always easy. In this article, we will cover the basics of using tables and figures in research papers, so you know when and how to use them to accurately communicate research results.
Table of Contents
How to choose between tables and graphs
When choosing whether to use tables or figures in research papers, it is important to consider what type of information you want to convey with your visuals. This will help determine which format would be best suited for the data. If you are presenting numerical data, a table is often the best choice because tables are a great way to compare values or characteristics among related items and are particularly useful for presenting large sets of data in a systematic manner.
Figures or graphs, on the other hand, can be used to show trends, or relationships. Graphs and charts allow readers to quickly and easily identify patterns in data, and are particularly useful for presenting data over time or when comparing different variables.
So, if you have a lot of numerical data, then a tabular format would be more appropriate. If you have more textual data, then a graphical format would be more suitable.
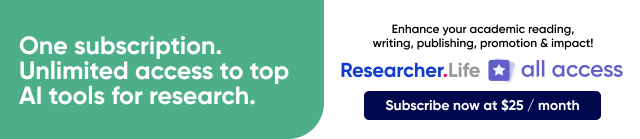
Choosing the appropriate table to present your data
Once you have decided on the type of data you will be presenting, you need to choose a format that can present your data in an easy to read and understand way. Tables can be overwhelming and difficult to read if they are too complex, and therefore, experts suggest keeping the format simple. It is also important to ensure that tables in research papers are accompanied by titles, labels or captions that are clear, concise, and engaging.
Identifying the right kind of graph to convey information
It is important to choose the right kind of graph or chart to communicate and highlight research findings. For example, if you are presenting data over time, a line graph is often the best choice, while a bar graph may be better for comparing different categories of data. Remember to ensure that the graphs, schematic diagrams, line drawings and data plots are clearly presented, and neatly composed. Using a prudent mix of colors and contrasts is a good way to highlight data without complicating it.
Using text is important too
It is important to keep in mind that using tables and figures in research papers may not always be required. It is okay to use text when you do not have extensive or complex data to share. Text can be used to provide context and explanation of the data being presented. Text can also help to provide a narrative to the data, making it easier for readers to understand its significance and implications. Using text is effective when one is not working towards presenting a large dataset or when one wants to present data marginal to the study.
Important points to keep in mind while using tables and figures in research papers
- Tables and figures in research papers must be clearly and simply presented to better clarity and ease of understanding. Figures that are complex or tables with too much information can dissuade readers from reading further and even dampen their interest.
- Remember to ensure that the data is not repeated in the main text of the research paper. Use only critical and necessary data and information in tables and figures that are pertinent to the research area or question being studied.
- Tables and figures in research papers must be integrated into the text in a way that is easy to understand and is visually appealing. It is important to ensure that the font size, style, and colors used are consistent with the overall design of the research paper. This will help to create a professional and cohesive look to the paper.
- It is crucial for researchers to adhere to uniformity in style and format while using tables and figures in research papers. Care must be taken to ensure that these align with the requirements and guidelines of the target journal or publication.
- When presenting tables and figures in research papers, it is important to ensure that they are clearly labelled and titled. This will help readers to understand what they are looking at and why it is important. It is also important to provide a brief explanation of the data or information being presented and provide context for the reader.
- If researchers use tables and figures that are not their own, it is essential that they correctly cite the sources to avoid being charged with plagiarism.
Keeping the above points in mind will help you in making sure that the tables and figures used in research papers effectively communicate critical aspects of your research with readers.
In conclusion, the choice between tables and figures in research papers is a nuanced decision that hinges on the nature of your data and the story you aim to tell. Tables are ideal for presenting precise numerical values and detailed comparisons, while figures excel at visually illustrating trends, patterns, and complex relationships. Consider your research goals, audience, and the most effective way to convey your findings. A strategic combination of tables and figures can enhance the clarity and impact of your research paper. By thoughtfully selecting these visual aids, you empower your readers to better comprehend and engage with your study’s insights.
Editage All Access is a subscription-based platform that unifies the best AI tools and services designed to speed up, simplify, and streamline every step of a researcher’s journey. The Editage All Access Pack is a one-of-a-kind subscription that unlocks full access to an AI writing assistant, literature recommender, journal finder, scientific illustration tool, and exclusive discounts on professional publication services from Editage.
Based on 22+ years of experience in academia, Editage All Access empowers researchers to put their best research forward and move closer to success. Explore our top AI Tools pack, AI Tools + Publication Services pack, or Build Your Own Plan. Find everything a researcher needs to succeed, all in one place – Get All Access now starting at just $14 a month !
Related Posts

Top 7 Tried and Tested Paraphrasing Techniques

Annex vs Appendix: What is the difference?
- Translators
- Graphic Designers
Please enter the email address you used for your account. Your sign in information will be sent to your email address after it has been verified.
Your Guide to Creating Effective Tables and Figures in Research Papers
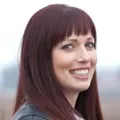
Research papers are full of data and other information that needs to be effectively illustrated and organized. Without a clear presentation of a study's data, the information will not reach the intended audience and could easily be misunderstood. Clarity of thought and purpose is essential for any kind of research. Using tables and figures to present findings and other data in a research paper can be effective ways to communicate that information to the chosen audience.
When manuscripts are screened, tables and figures can give reviewers and publication editors a quick overview of the findings and key information. After the research paper is published or accepted as a final dissertation, tables and figures will offer the same opportunity for other interested readers. While some readers may not read the entire paper, the tables and figures have the chance to still get the most important parts of your research across to those readers.
However, tables and figures are only valuable within a research paper if they are succinct and informative. Just about any audience—from scientists to the general public—should be able to identify key pieces of information in well-placed and well-organized tables. Figures can help to illustrate ideas and data visually. It is important to remember that tables and figures should not simply be repetitions of data presented in the text. They are not a vehicle for superfluous or repetitious information. Stay focused, stay organized, and you will be able to use tables and figures effectively in your research papers. The following key rules for using tables and figures in research papers will help you do just that.
Check style guides and journal requirements
The first step in deciding how you want to use tables and figures in your research paper is to review the requirements outlined by your chosen style guide or the submission requirements for the journal or publication you will be submitting to. For example, JMIR Publications states that for readability purposes, we encourage authors to include no more than 5 tables and no more than 8 figures per article. They continue to outline that tables should not go beyond the 1-inch margin of a portrait-orientation 8.5"x11" page using 12pt font or they may not be able to be included in your main manuscript because of our PDF sizing.
Consider the reviewers that will be examining your research paper for consistency, clarity, and applicability to a specific publication. If your chosen publication usually has shorter articles with supplemental information provided elsewhere, then you will want to keep the number of tables and figures to a minimum.
According to the Purdue Online Writing Lab (Purdue OWL), the American Psychological Association (APA) states that Data in a table that would require only two or fewer columns and rows should be presented in the text. More complex data is better presented in tabular format. You can avoid unnecessary tables by reviewing the data and deciding if it is simple enough to be included in the text. There is a balance, and the APA guideline above gives a good standard cutoff point for text versus table. Finally, when deciding if you should include a table or a figure, ask yourself is it necessary. Are you including it because you think you should or because you think it will look more professional, or are you including it because it is necessary to articulate the data? Only include tables or figures if they are necessary to articulate the data.
Table formatting
Creating tables is not as difficult as it once was. Most word processing programs have functions that allow you to simply select how many rows and columns you want, and then it builds the structure for you. Whether you create a table in LaTeX , Microsoft Word , Microsoft Excel , or Google Sheets , there are some key features that you will want to include. Tables generally include a legend, title, column titles, and the body of the table.
When deciding what the title of the table should be, think about how you would describe the table's contents in one sentence. There isn't a set length for table titles, and it varies depending on the discipline of the research, but it does need to be specific and clear what the table is presenting. Think of this as a concise topic sentence of the table.
Column titles should be designed in such a way that they simplify the contents of the table. Readers will generally skim the column titles first before getting into the data to prepare their minds for what they are about to see. While the text introducing the table will give a brief overview of what data is being presented, the column titles break that information down into easier-to-understand parts. The Purdue OWL gives a good example of what a table format could look like:
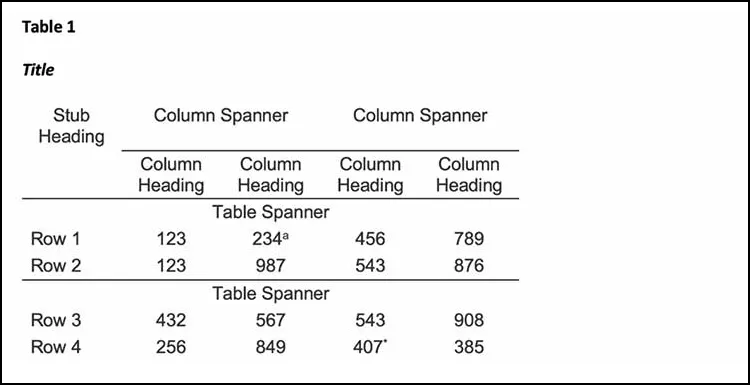
When deciding what your column titles should be, consider the width of the column itself when the data is entered. The heading should be as close to the length of the data as possible. This can be accomplished using standard abbreviations. When using symbols for the data, such as the percentage "%" symbol, place the symbol in the heading, and then you will not use the symbol in each entry, because it is already indicated in the column title.
For the body of the table, consistency is key. Use the same number of decimal places for numbers, keep the alignment the same throughout the table data, and maintain the same unit of measurement throughout each column. When information is changed within the same column, the reader can become confused, and your data may be considered inaccurate.
When creating tables, especially those derived from complex datasets or scanned documents, tools like JPG to Excel converter can be incredibly useful. These tools can automate the extraction of tabular data from images, ensuring accuracy and saving time. By converting image-based data into editable Excel sheets, researchers can easily format, analyze, and integrate the information into their papers. Utilizing such converters can enhance the efficiency of your data management process, making the preparation of tables for your research paper more seamless.
Figures in research papers
Figures can be of many different graphical types, including bar graphs, scatterplots, maps, photos, and more. Compared to tables, figures have a lot more variation and personalization. Depending on the discipline, figures take different forms. Sometimes a photograph is the best choice if you're illustrating spatial relationships or data hiding techniques in images. Sometimes a map is best to illustrate locations that have specific characteristics in an economic study. Carefully consider your reader's perspective and what detail you want them to see.
As with tables, your figures should be numbered sequentially and follow the same guidelines for titles and labels. Depending on your chosen style guide, keep the figure or figure placeholder as close to the text introducing it as possible. Similar to the figure title, any captions should be succinct and clear, and they should be placed directly under the figure.
Using the wrong kind of figure is a common mistake that can affect a reader's experience with your research paper. Carefully consider what type of figure will best describe your point. For example, if you are describing levels of decomposition of different kinds of paper at a certain point in time, then a scatter plot would not be the appropriate depiction of that data; a bar graph would allow you to accurately show decomposition levels of each kind of paper at time "t." The Writing Center of the University of North Carolina at Chapel Hill has a good example of a bar graph offering easy-to-understand information:
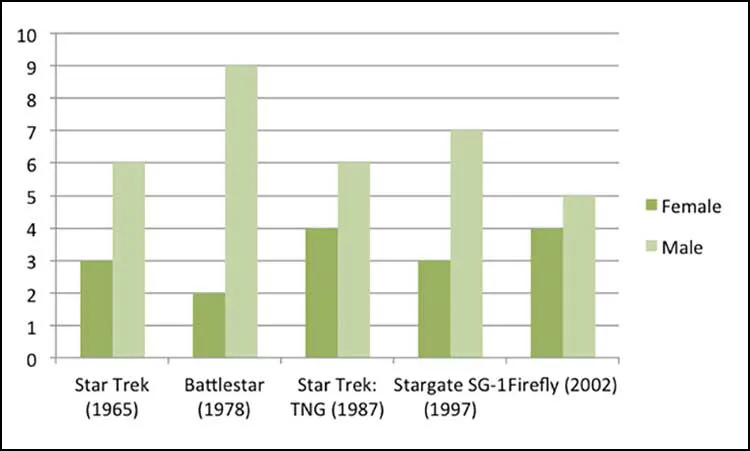
If you have taken a figure from another source, such as from a presentation available online, then you will need to make sure to always cite the source. If you've modified the figure in any way, then you will need to say that you adapted the figure from that source. Plagiarism can still happen with figures – and even tables – so be sure to include a citation if needed.
Using the tips above, you can take your research data and give your reader or reviewer a clear perspective on your findings. As The Writing Center recommends, Consider the best way to communicate information to your audience, especially if you plan to use data in the form of numbers, words, or images that will help you construct and support your argument. If you can summarize the data in a couple of sentences, then don't try and expand that information into an unnecessary table or figure. Trying to use a table or figure in such cases only lengthens the paper and can make the tables and figures meaningless instead of informative.
Carefully choose your table and figure style so that they will serve as quick and clear references for your reader to see patterns, relationships, and trends you have discovered in your research. For additional assistance with formatting and requirements, be sure to review your publication or style guide's instructions to ensure success in the review and submission process.
- Academic Writing Advice
- All Blog Posts
- Writing Advice
- Admissions Writing Advice
- Book Writing Advice
- Short Story Advice
- Employment Writing Advice
- Business Writing Advice
- Web Content Advice
- Article Writing Advice
- Magazine Writing Advice
- Grammar Advice
- Dialect Advice
- Editing Advice
- Freelance Advice
- Legal Writing Advice
- Poetry Advice
- Graphic Design Advice
- Logo Design Advice
- Translation Advice
- Blog Reviews
- Short Story Award Winners
- Scholarship Winners
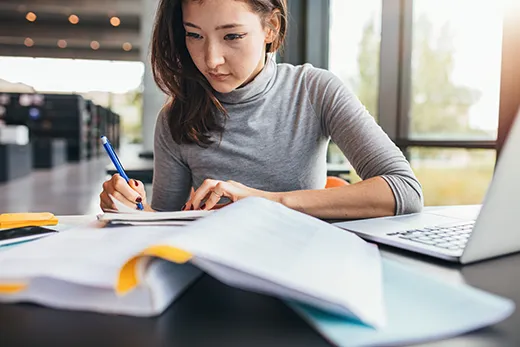
Need an academic editor before submitting your work?
Have a language expert improve your writing
Run a free plagiarism check in 10 minutes, generate accurate citations for free.
- Knowledge Base
- Dissertation
- Figure and Table Lists | Word Instructions, Template & Examples
Figure and Table Lists | Word Instructions, Template & Examples
Published on October 13, 2015 by Sarah Vinz . Revised on July 18, 2023 by Tegan George.
A list of figures and tables compiles all of the figures and tables that you used in your thesis or dissertation , along with their corresponding page numbers. These lists give your reader an overview of how you have used figures and tables in your document.
While these lists are often not required, you may want to include one as a way to stay organized if your dissertation topic leads you to use several figures and tables over the course of your paper. Your educational institution may require one, so be sure to check their guidelines. Ultimately, if you do choose to add one, it should go directly after your table of contents .
You can download our Microsoft Word template below to help you get started.
Download Word doc
- Table of contents
How to create a list of figures and tables in Word
Example of a list of tables and figures, additional lists to consider, other interesting articles, frequently asked questions about the list of tables and figures.
The first step to creating your list of figures and tables is to ensure that each of your figures and tables has a caption . This way, Microsoft Word will be able to find each one and compile them in your list automatically.
To do this, follow these steps:
- Navigate to the References tab, and click “Insert Caption,” which you can find in the Captions group.
- Give your caption a name. In the Label list, you can select the label that best describes your figure or table, or make your own by selecting “New Label.”
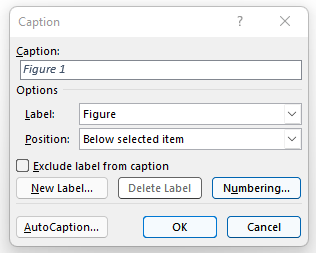
Next, you can insert the list of tables and figures directly by clicking “Insert Table of Figures,” which can be found to the right of the “Insert Caption” button. Be careful here—the list will only include items that you have marked using the “Insert Caption” tool!
You can choose the formatting and layout within this menu as well, as you can see below.
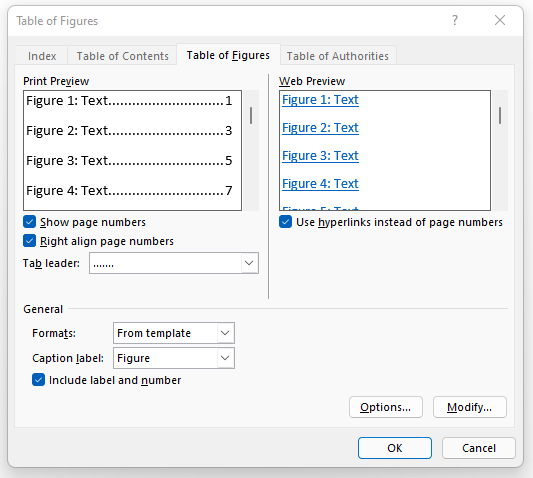
There are a few things to remember as you go:
- Figures and tables always need to be numbered, with clear titles.
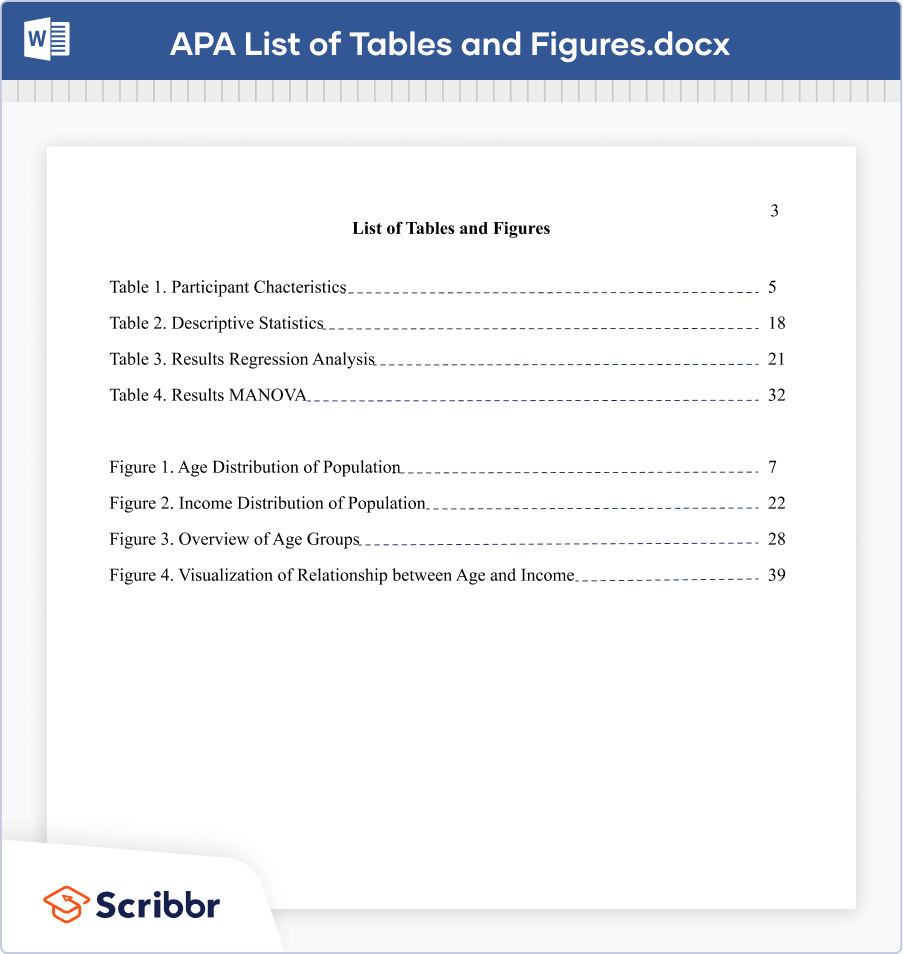
In addition to your list of tables and figures, there are a few other lists to consider for your thesis or dissertation. They can be placed in the following order:
- List of abbreviations
If you want to know more about AI for academic writing, AI tools, or research bias, make sure to check out some of our other articles with explanations and examples or go directly to our tools!
Research bias
- Anchoring bias
- Halo effect
- The Baader–Meinhof phenomenon
- The placebo effect
- Nonresponse bias
- Deep learning
- Generative AI
- Machine learning
- Reinforcement learning
- Supervised vs. unsupervised learning
(AI) Tools
- Grammar Checker
- Paraphrasing Tool
- Text Summarizer
- AI Detector
- Plagiarism Checker
- Citation Generator
Your list of tables and figures should go directly after your table of contents in your thesis or dissertation.
Lists of figures and tables are often not required, and aren’t particularly common. They specifically aren’t required for APA-Style, though you should be careful to follow their other guidelines for figures and tables .
If you have many figures and tables in your thesis or dissertation, include one may help you stay organized. Your educational institution may require them, so be sure to check their guidelines.
Copyright information can usually be found wherever the table or figure was published. For example, for a diagram in a journal article , look on the journal’s website or the database where you found the article. Images found on sites like Flickr are listed with clear copyright information.
If you find that permission is required to reproduce the material, be sure to contact the author or publisher and ask for it.
A list of figures and tables compiles all of the figures and tables that you used in your thesis or dissertation and displays them with the page number where they can be found.
APA doesn’t require you to include a list of tables or a list of figures . However, it is advisable to do so if your text is long enough to feature a table of contents and it includes a lot of tables and/or figures .
A list of tables and list of figures appear (in that order) after your table of contents, and are presented in a similar way.
Cite this Scribbr article
If you want to cite this source, you can copy and paste the citation or click the “Cite this Scribbr article” button to automatically add the citation to our free Citation Generator.
Vinz, S. (2023, July 18). Figure and Table Lists | Word Instructions, Template & Examples. Scribbr. Retrieved July 22, 2024, from https://www.scribbr.com/dissertation/figure-and-table-lists-in-your-dissertation/
Is this article helpful?
Sarah's academic background includes a Master of Arts in English, a Master of International Affairs degree, and a Bachelor of Arts in Political Science. She loves the challenge of finding the perfect formulation or wording and derives much satisfaction from helping students take their academic writing up a notch.
What is your plagiarism score?
Training videos | Faqs

Figures and Tables in Research Papers – Tips and Examples
In this blog, we will look at best practices for presenting tables and figures in your research paper. You must try to present your results in figures and tables wherever possible. This is a fantastic way to summarize large amounts of data in a visually pleasing way.
1. When to use Figures and Tables in a Research Paper?
One of the common questions asked by students is when should they present the data in figures and tables instead of putting it directly in the text. The best way to answer this question is if you cannot present your data in your text in one or two lines, then you should consider putting it in a figure or a table.
Tip: If you cannot present your data in your text in one or two lines then use a Table or Figure.
Another common question that comes up is how to choose between a figure and a table for presenting the data. The best way to answer this question is if you want to illustrate the trends and patterns in the data, then a figure is the best option. If you want to show the actual values or present a lot of numerical information in your paper. Then, a table might be the best way to go.

2. An Example of Table and Figure Usage
Here is an example. Look at the way the authors have used tables and figures to describe their results.
✔ The author explains the trend using a figure and points the reader to the table for full data In Figure 1.1, the values are plotted as a function of time. The two peaks in the plot correspond to the maximum and minimum temperature values. The specific values obtained for each experiment are given in Table 2.
In the first couple of lines, they are talking about a trend in their data that relates to the change in temperature over time. They are constantly referring to the figure to get their point across to the readers. And finally, in the last sentence, they are telling the readers that the actual numerical data is provided in the table, and they can refer to it if they want. This is a standard way to use figures and tables in your research paper.
3. Best Practices for Using Tables & Figures
When you are using figures and tables in your paper, you must reference them in your text. You cannot have a figure in your paper and not talk about it in your text.
Best practices All Figures/Tables should be referenced in the text Every Figure/Table should have a caption Caption should be concise and self-explanatory
Another important thing is that your figure should have a caption that clearly explains what is it about. Most people skim through the paper just going through figures and tables without reading any text in the paper. So, captions should be as short as possible but detailed enough for the readers to understand the figure or table without having to read the text.
4. Common Mistakes to Avoid
A common mistake made by both beginner writers and experienced writers is repeating the figure caption in the text when referring to the figure in the paper. Look at the following example, the author repeats exactly what it says in the figure caption in the text. The author is saying that the figure shows the change in temperature over time. This is already mentioned in the figure caption, there is nothing new here.
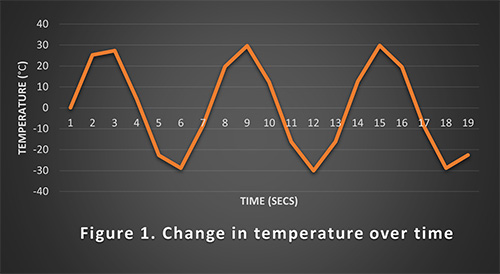
✖ Bad example – Repetition of figure title in the text when referring to it Figure 1 shows the change in temperature over time.
Now, look at the example below. While referring to the figure, the author provides some additional information to the readers by talking about an interesting trend in the figure instead of just repeating the figure caption.
✔ Good example – Interesting fact provided about the figure when referring to it The temperature fluctuation over time follows a sine wave pattern peaking at 30 degrees (Fig 1).
Tables and figures are important parts of your research work. They are your secret weapon for clearly presenting your data to your readers. We strongly recommend that you prepare the list of figures and tables that you want to include in your work before you start writing the text. Please follow the instructions provided in this blog while adding figures and tables to your research paper. If you have any questions, please drop a comment below, and we will answer as soon as possible. We also recommend you to refer to our other blogs on academic writing tools , academic writing resources , and academic phrase-bank , which are relevant to the topic discussed in this blog.
Similar Posts
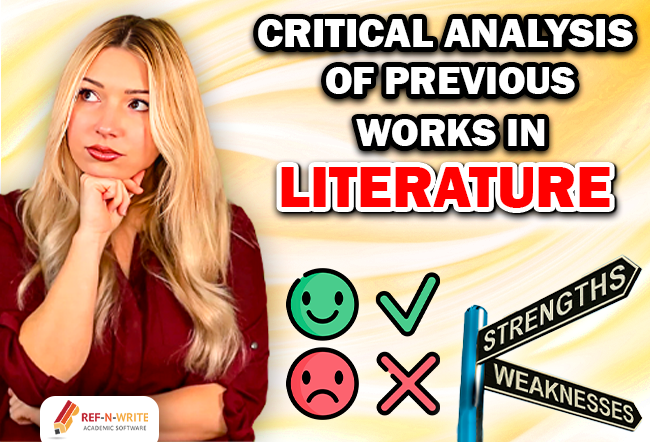
Critical Literature Review : How to Critique a Research Article?
In this blog, we will look at how to use constructive language when critiquing other’s work in your research paper.

How to Write Catchy Research Paper Titles with Examples
The title of your paper should be catchy yet informative. Let’s dive into some tips and tricks to ensure your title stands out in the academic crowd.

How to Create a Research Paper Outline?
In this blog we will see how to create a research paper outline and start writing your research paper.

Results Section Examples and Writing Tips
In this blog, we will go through many results section examples and understand how to write a great results section for your paper.
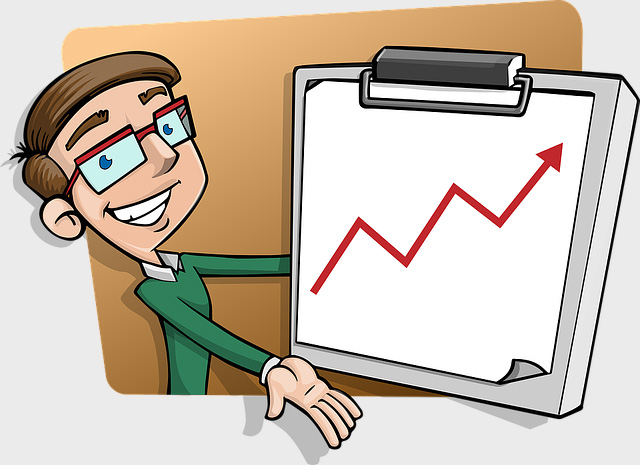
Discussion Section Examples and Writing Tips
In this blog, we will go through many discussion examples and understand how to write a great discussion for your research paper.
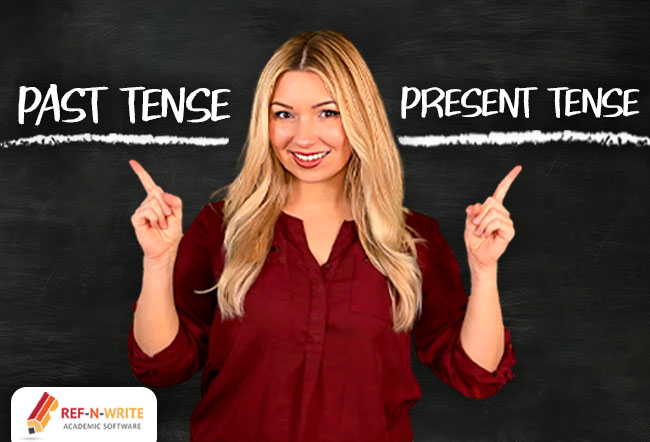
Mastering Verb Tenses in Literature Reviews
In this blog, we will see what tense you should use in the literature review section of your research paper.
Leave a Reply Cancel reply
Your email address will not be published. Required fields are marked *
Save my name, email, and website in this browser for the next time I comment.
- 1 Share Facebook
- 1 Share Twitter
- 0 Share LinkedIn
- 1 Share Email
Home → Academic Writing → Tips On Effective Use Of Tables And Figures In Research Papers
Tips On Effective Use Of Tables And Figures In Research Papers
- December 24, 2022

Several studies, journal guidelines, and discourses on scientific writing affirm the critical role that tables, figures, and graphs (or display items) play in enhancing the quality of manuscripts. Scientific tables and graphs can be utilized to represent sizeable numerical or statistical data in a time- and space-effective manner. Readers are often drawn towards tables and figures, because they perceive it as easy-reading, as compared to reading a verbose account of the same content. They rightly assume that these display items will provide them with a larger amount of information in a shorter time span.
At the manuscript screening stage, these display items offer reviewers and journal editors a quick overview of the study findings, and once the paper is published, they do the same for readers (some of whom look only at these display items and not at the rest of the manuscript). However, tables and figures only add value to the format of a research report, if they are brief yet sufficiently informative.
These visual elements help authors present detailed results and complex relationships, patterns, and trends clearly and concisely; reduce the length of the manuscript and enhance readers’ understanding of the study results. Therefore, these tools are integral to the format of a research paper because, if clear and well-organized, they speed up the comprehension and interpretation of the study’s findings.
But while well-presented tables and figures in research papers can efficiently capture and present information, poorly crafted tables and figures can confuse readers and impair the effectiveness of a paper. To help authors get the balance right, this article presents some essential guidelines to the effective use of tables and figures in research papers.
Planning your paper: When to use tables and figures in scientific papers
Producing effective tables and figures requires careful planning that begins at the manuscript writing stage itself. Here’s how to go about it:
- First, check out what your target journal has to say on the issue. Some journals limit the number of tables and figures and also have specific guidelines on the design aspects of these display items.
- Next, decide whether to use tables and figures or text to put across key information.(Refer to Table 1 below for help on making this decision.)
- After you’ve decided to use a display item, choose the display item that best fits your purpose based on what you wish readers to focus on and what you want to present (Refer to Table 1 below for more information).
- Finally, follow the best-practice guidelines outlined in section 3 and review the examples presented in section 4 of this paper to ensure that your tables and figures are well-designed.
Table 1: How to choose between tables, figures, and text to present data
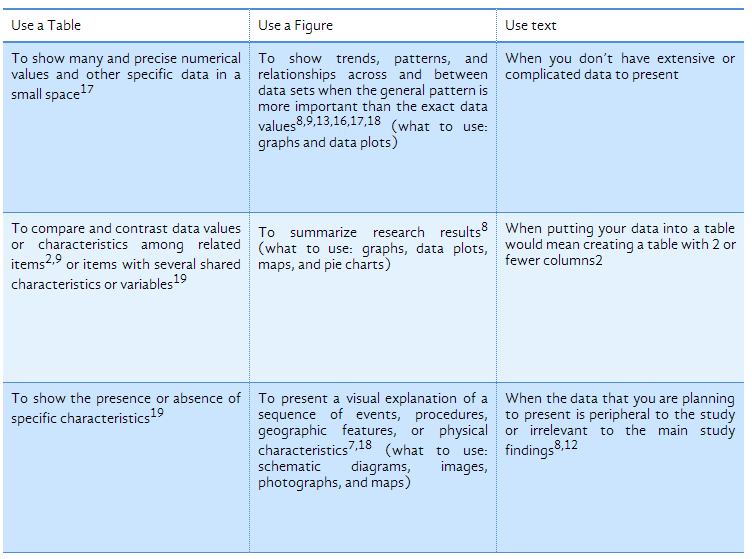
Best practices for presentation of tables and figures in scientific papers
General guidelines:
- Ensure that display items are self-explanatory : Some readers (and certainly reviewers and journal editors) turn their attention to the tables and figures before they read the entire text, so these display items should be self-contained.
- Refer, but don’t repeat : Use the text to draw the reader’s attention to the significance and key points of the table/figure, but don’t repeat details. So for example, you could highlight your main finding (e.g., “We found that the treatment was effective in only 24% of the cases, as shown in Figure 1”), but don’t repeat exact values (e.g., “As Table 2 shows, 32% of the subjects chose Option 1, 12% chose Option 2, 10% chose Option 3, and 46% chose Option 4”). This defeats the very purpose (efficiency and clarity) of having a table or figure.
- Be consistent : Ensure consistency between values or details in a table (e.g., abbreviations, group names, treatment names) and those in the text.
- Give clear, informative titles : Table and figure titles should not be vague but should concisely describe the purpose or contents of the table/figure and should ideally draw the reader’s attention to what you want him/her to notice (e.g., Advantages and disadvantages of using sleep therapy with patients suffering from schizophrenia). Also ensure that column heads, axis labels, figure labels, etc., are clearly and appropriately labelled.
- Adhere to journal guidelines : Check what your target journal has to say about issues like the number of tables and figures, the style of numbering, titles, image resolution, file formats, etc., and follow these instructions carefully.
Guidelines for tables:
- Combine repetitive tables : Tables and figures that present repetitive information will impair communication rather than enhance it. Examine the titles of all your tables and figures and check if they talk about the same or similar things. If they do, rethink the presentation and combine or delete the tables/graphs.
- Divide the data : When presenting large amounts of information, divide the data into clear and appropriate categories and present them in columns titled accurately and descriptively.
- Watch the extent of data in your tables : If the data you have to present is extensive and would make the tables too cluttered or long, consider making the tables a part of the Appendix or supplemental material.
- De-clutter your table : Ensure that there is sufficient spacing between columns and rows and that the layout does not make the table look too messy or crowded.
Guidelines for figures:
- Ensure image clarity : Make sure that all the parts of the figure are clear:18 Use standard font; check that labels are legible against the figure background; and ensure that images are sharp.
- Use legends to explain the key message : Figure legends are pivotal to the effectiveness of a figure. Use them to draw attention to the central message as well as to explain abbreviations and symbols.
- Label all important parts : Label the key sections and parts of schematic diagrams and photographs, and all axes, curves, and data sets in graphs and data plots.
- Give specifics : Include scale bars in images and maps; specify units wherever quantities are listed; include legends in maps and schematics; and specify latitudes and longitudes on maps. This section presents one example each of a well-prepared table and a well-designed figure.
Table 2: The table below is taken from a dietary study on chick-rearing macaroni penguins and is an example of an effective table for the following reasons:
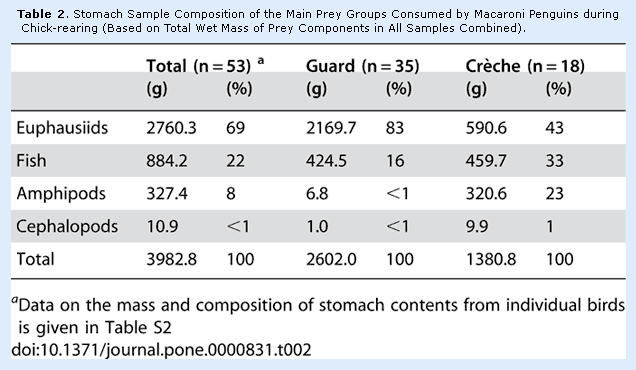
- The title clearly describes what the table is about.
- The column heads are descriptive and clearly indicate the nature of the data presented. The data is divided into categories for clarity.
- It is self-contained and can be understood quite well even without reference to the entire paper.
- Superscript letters and notes are used to offer additional, clarifying information.
- Sufficient spacing is present between columns and rows; the layout is clean, and the font is legible.
Examples of an effective figure (graph)
The figure below from a paper on the efficacy of oyster reefs as natural breakwaters, scores on several counts:
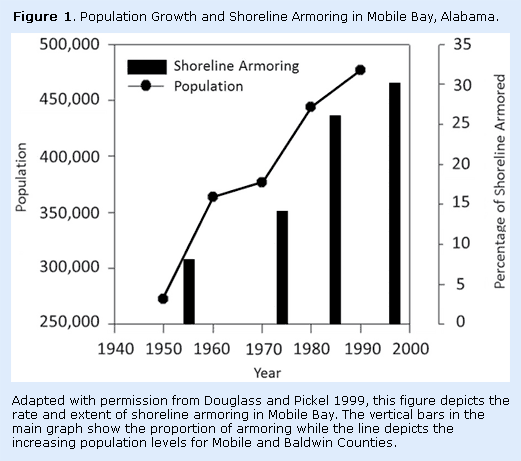
- The informative title that immediately tells the reader what to expect in the graph.
- The axes are labeled clearly.
- The key clearly identifies what each element in the graph stands for.
- A figure legend at the bottom draws the reader’s attention to the graph’s key points.
- A note at the bottom acknowledges the source.
- The graph is 2-dimensional, with no clutter.
Figures and tables, or display items, are powerful communication tools—they give your manuscript a professional feel, attract and sustain the interest of readers, and efficiently present large amounts of complex information. Moreover, as most journals editors and reviewers will glance at these display items before they begin a full reading of your paper, their importance cannot be overemphasized.
Keep striving, researchers! ✨
Table of Contents
Related articles.

How to Publish a Research Paper: A Step-by-Step Guide
You’re in academia. You’re going steady. Your research is going well and you begin to wonder: ‘How exactly do I get a

Behind the Scenes: What Does a Research Assistant Do?
Have you ever wondered what goes on behind the scenes in a research lab? Does it involve acting out the whims of
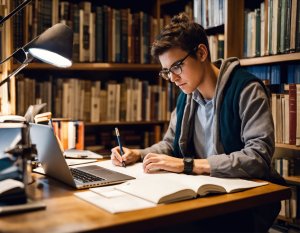
How to Write a Research Paper Introduction: Hook, Line, and Sinker
Want to know how to write a research paper introduction that dazzles? Struggling to hook your reader in with your opening sentences?

Blog Podcast
Privacy policy Terms of service
Subscribe to our newsletter!
Discover more from Audemic: Access any academic research via audio
Subscribe now to keep reading and get access to the full archive.
Type your email…
Continue reading
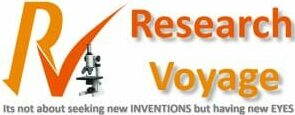
Research Voyage
Research Tips and Infromation
Best Practices for Designing and Formatting Tables in Research Papers
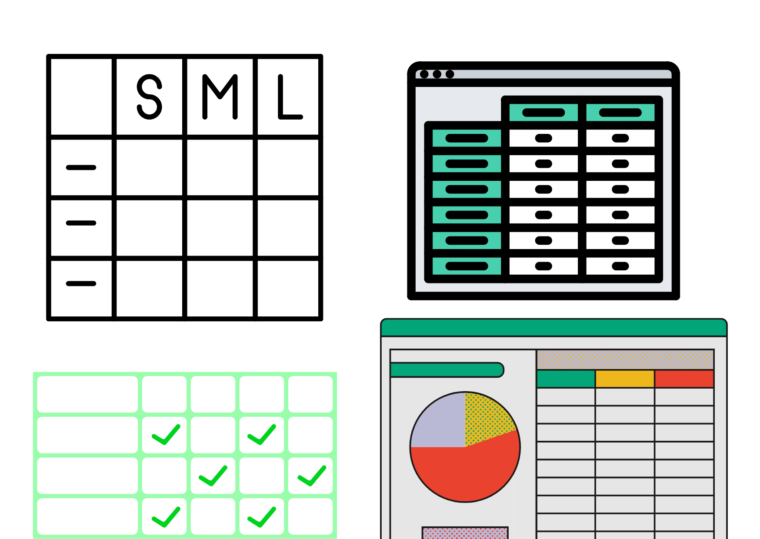
Introduction
Advantages of using tables in research papers, simple table, complex table, comparison table, statistical table, guidelines for effective use of tables in research papers, i. conditional formatting, ii. data bars, iii. highlighting cells:, how to fit my table by splitting it into multiple pages, can i give citations within the table , what is creative commons license, whether tables are also part of the plagiarism check, how many tables should be there in a research paper of 10 pages, can i put tables at the end of research paper, can i put table in single column in a two column research paper format.
Tables are a crucial aspect of research papers, providing a visual representation of data and results. They are used to effectively and concisely convey information to the reader. The purpose of using tables in research papers is to organize and present data in a manner that is easy to understand and interpret.
A table is a way of arranging data in rows and columns, allowing the reader to quickly identify patterns and trends within the data. It can be used to compare different results or to present large amounts of information in a clear and organized manner.
The importance of using tables in research papers cannot be overstated. Not only do they improve the overall clarity and organization of the paper, but they also make it easier for the reader to understand and interpret the results.
In this article, we will explore the advantages of using tables in research papers, the different types of tables commonly used, and how to effectively utilize tables in your research paper.
As a researcher or academic, you may have started out presenting data and information in your research papers in a simple format, such as just listing the data as plain text. However, as you progressed in your work, you soon realized the importance of presenting the information in a clear and organized manner.
You may have experienced the difficulties of presenting complex data in a simple format, and struggled with making the information easy for readers to understand. That’s when you discovered the power of tables.
Tables allow you to present complex data in a simple and easy-to-read format, helping your readers understand the information quickly and accurately. They also help to save space and make it easier to compare different data sets.
However, you soon learned that simply presenting the data in a table was not enough. You realized the importance of differentiating the rows in your tables to make the information stand out and easier to understand. You explored different ways to do this, such as using different background colors, shading, bold or italic text, different font sizes or styles, and different alignments.
Through your experience, you learned that tables play a crucial role in research papers, and that differentiating the rows in your tables can greatly improve the clarity and organization of your information. You continued to refine your table-making skills, ultimately resulting in the ability to present your data and information in the best possible way.
There are several benefits to using tables in research papers, including:
- Clarity and Organization of Data: Tables help to visually organize data and results, making it easier for the reader to understand and interpret the information. This can be particularly useful when presenting complex or detailed data sets.
- Easy Comparison of Results: Tables allow for quick and easy comparison of results, making it simpler for the reader to identify trends and patterns in the data. This is especially useful when presenting multiple results or comparing results from different experiments or studies.
- Ability to present large amounts of information: Tables are an effective way to present large amounts of data in a concise and organized manner. They help to break down complex information into manageable chunks, making it easier for the reader to comprehend.
- Improved Visual Appeal: Tables can improve the visual appeal of a research paper, breaking up long sections of text and making it easier for the reader to follow the information being presented. They can also help to clarify and emphasize key results or findings.
By utilizing tables in your research paper, you can effectively communicate your results and make it easier for the reader to understand the information you are presenting. The use of tables can also improve the overall clarity and organization of the paper, making it a valuable tool for any researcher.
Types of Tables Commonly Used in Research Papers
There are several types of tables commonly used in research papers, including:
A simple table presents data in a basic format, with columns and rows to organize the information. This type of table is useful for presenting simple data sets, such as small amounts of numerical or categorical data.
Fruit | Quantity |
---|---|
Apples | 10 |
Oranges | 5 |
Bananas | 7 |
A complex table is used to present more complex data sets, such as large amounts of numerical data or data with multiple categories. This type of table may also include subheadings, footnotes, or other additional information to help the reader understand the data being presented.
Country | Year | Population | GDP (in billions) | GDP per capita | Life Expectancy |
---|---|---|---|---|---|
USA | 2019 | 328,239,523 | $21,439.8 | $65,112 | 78.9 |
USA | 2020 | 331,449,281 | $20,807.8 | $62,776 | 77.3 |
USA | 2021 | 334,710,820 | $22,675.0 | $67,727 | 78.1 |
China | 2019 | 1,433,783,686 | $14,342.9 | $10,030 | 76.7 |
China | 2020 | 1,439,323,776 | $16,121.3 | $11,197 | 77.3 |
China | 2021 | 1,444,216,107 | $18,705.6 | $12,950 | 78.1 |
Japan | 2019 | 126,476,458 | $5,154.6 | $40,734 | 84.6 |
Japan | 2020 | 126,264,931 | $4,887.3 | $38,707 | 84.3 |
Japan | 2021 | 125,960,000 | $5,159.0 | $40,994 | 84.8 |
A comparison table is used to compare data or results from multiple sources, experiments, or studies. This type of table allows the reader to quickly identify similarities and differences between the data being presented.
Feature | Product A | Product B | Product C |
---|---|---|---|
Price | $50 | $60 | $70 |
Weight | 1 lb | 1.5 lb | 2 lb |
Battery Life | 5 hours | 7 hours | 8 hours |
Warranty | 1 year | 2 years | 3 years |
A statistical table presents numerical data and statistical results, such as means, standard deviations, and p-values. This type of table is useful for presenting results from statistical analyses and can be used to effectively communicate the significance of the results.
Year | Mean | Median | Standard Deviation | Sample Size |
---|---|---|---|---|
2019 | 65.2 | 68.0 | 8.7 | 100 |
2020 | 61.8 | 63.5 | 7.6 | 120 |
2021 | 67.5 | 69.0 | 9.3 | 90 |
When using tables in research papers, it is important to follow certain guidelines to effectively communicate the information being presented. Some of these guidelines include:
- Clearly label the table and provide a brief description: Label the table with a clear and descriptive title, and provide a brief description of the information being presented. This will help the reader understand the purpose of the table and what information they can expect to find.
- Choose the right type of table: Choose the right type of table for the data being presented, as outlined in the previous section. This will help to effectively communicate the results and make it easier for the reader to understand the information.
- Keep tables simple and concise: Keep tables simple and concise, using only the necessary information to effectively communicate the results. Avoid using overly complex or cluttered tables, as this can make it more difficult for the reader to understand the information being presented.
- Use appropriate formatting: Use appropriate formatting to effectively communicate the information being presented. For example, use bold or italic text to highlight important information, and align the columns and rows in a way that makes the information easy to read.
- Provide clear and concise captions: Provide clear and concise captions for each table, explaining the purpose and results of the data being presented. This will help the reader understand the information being presented and will also provide context for the results.
By following these guidelines, researchers can effectively utilize tables in their research papers to communicate their results in a clear and organized manner. The use of tables can improve the overall clarity and organization of the paper, making it easier for the reader to understand the information being presented.
Highlighting Key Information in Tables Through Row Differentiation
When presenting data in a table, it is important to make sure that the information is organized and easy to understand. One effective way to do this is by differentiating the rows in the table. Here are several ways to achieve this, including using different background colors, shading or borders, text formatting, alignment, row spacing, and highlighting cells with specific values. These methods can help to group similar data, highlight important data points, and make the table easier to read and understand. Whether you are presenting data in a research paper, a business report, or any other type of document, utilizing these techniques can enhance the clarity and impact of your data presentation.
- Background color: Different background colors can be used to distinguish between rows and highlight specific groups of data.
- Shading or borders: Using shading or borders can help to separate rows in a table and distinguish between different groups of data.
- Text formatting: Bold or italic text can be used to highlight specific rows and make them stand out from the rest of the data. Different font sizes or styles can also be used to differentiate between rows.
- Alignment: Different alignments, such as centre or right alignments, can be used to differentiate between rows and distinguish between different types of data.
- Row spacing: Increasing the row spacing can help to separate the rows and make the table easier to read.
- Differentiating rows based on values: This is a feature in spreadsheet programs like Microsoft Excel and Google Sheets that allows you to apply conditional formatting rules to cells based on their values.
These are just a few examples of ways to differentiate rows in a table. The best approach will depend on the type of data being presented and the purpose of the table. The goal should be to make it easy for the reader to understand the information being presented and distinguish between different rows. As points 1-5 are most familiar and well known, I will elaborate point 6 in the following section.
Differentiate Rows in a Table Based on the Values of the Table.
There are several ways to do this:
This is a feature in spreadsheet programs like Microsoft Excel and Google Sheets that allows you to apply conditional formatting rules to cells based on their values. For example, you could apply a certain color to cells with a certain value range or highlight cells with specific values.
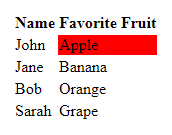
This is another feature in spreadsheet programs that allows you to add a bar to cells based on their values. This can help you visualize the relative magnitude of values within a table.
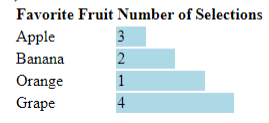
You can also manually highlight cells with specific values to draw attention to them. This can be done using the built-in highlighting tools in spreadsheet programs or by manually adding borders or shading to the cells.
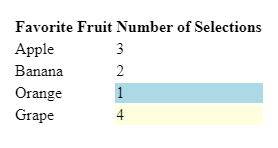
How to fit Big Table in a Research Paper?
Tables are a crucial component of research papers as they help to present data in a clear and organized manner. However, sometimes the amount of data you need to present can result in a table that is too big to fit on one page. In such cases, fitting the table into a research paper can become a challenge. But with a few adjustments and strategies, you can effectively fit a big table into your research paper and ensure that the information is presented in a clear and readable manner. In this article, we’ll discuss a few methods for fitting a large table into a research paper.
- Reduce the font size : Reducing the font size can help fit more data into the same amount of space, but it may make the table more difficult to read.
- Split the table into multiple smaller tables: Splitting the large table into smaller tables that focus on different aspects of the data can make it easier to read and understand.
- Use landscape orientation: Changing the orientation of the page to landscape can provide more space for the table.
- Use a smaller font for numerical values: If the data in the table consists mainly of numerical values, you can use a smaller font for the values and a larger font for the headings.
- Use abbreviations or symbols: Replacing lengthy text with abbreviations or symbols can reduce the size of the table while still conveying the necessary information.
- Use a table that scrolls horizontally: Some word processors and typesetting programs allow you to create tables that can be scrolled horizontally, allowing you to fit more data into the same amount of space.
- Omit non-essential information: If the table contains data that is not critical to your research, consider omitting it to reduce the size of the table.
The best option of all is to split a table and show it across multiple pages when the table contains more items row-wise. in a research paper. The exact method for doing so depends on the word processing software or typesetting system you are using.
For example, in Microsoft Word , you can split a table across multiple pages by selecting the row below which you want to split the table, and then going to “Layout” > “Breaks” > “Next Page” to insert a page break. The upper part of the table will be on one page and the lower part will start on the next page.
In LaTeX , you can split a table across multiple pages using the long table package. The long table package allows you to create tables that span multiple pages, with header and footer rows that repeat on each page.
Regardless of the method used, it is important to ensure that the split table is still readable and the data is easy to understand, even when split across multiple pages.
When splitting a table across multiple pages in a research paper, it is important to ensure that the headings are also repeated on each page to make the table readable and easy to understand.
In Microsoft Word, you can repeat the headings by selecting the first row of the table (which contains the headings) and then right-clicking and selecting “Table Properties.” In the “Row” tab, you can check the “Repeat as header row at the top of each page” option. This will cause the headings to be repeated at the top of each page on which the table is split.
In LaTeX, you can repeat the headings by using the long table package as described in my previous answer. The long table package provides options for defining the header and footer rows that are repeated on each page of the table.
Regardless of the method used, it is important to ensure that the headings are clearly visible and easily distinguishable from the rest of the table. This helps readers understand the data contained in the table and follow its structure, even when split across multiple pages.
When splitting a table across multiple pages in a research paper, it is important to ensure that the headings are also repeated on each page to make the table readable and easy to understand. In Microsoft Word, you can repeat the headings by selecting the first row of the table (which contains the headings) and then right-clicking and selecting “Table Properties.” In the “Row” tab, you can check the “Repeat as header row at the top of each page” option. This will cause the headings to be repeated at the top of each page on which the table is split.
In LaTeX, you can repeat the headings by using the longtable package. The longtable package provides options for defining the header and footer rows that are repeated on each page of the table. Regardless of the method used, it is important to ensure that the headings are clearly visible and easily distinguishable from the rest of the table. This helps readers understand the data contained in the table and follow its structure, even when split across multiple pages.
How to Refer Tables in a Research Paper?
In a research paper, tables are usually referred to in the text by their number, such as Table 1, Table 2, etc. To refer to a specific element within a table, such as a specific row or column, you can specify the table number followed by the row and column number, e.g. “Table 1, Row 2, Column 3”. When referring to a table, it is important to ensure that the reference is clear and accurate and that the table is properly cited if the information is taken from another source.
Yes, you can give references or citations within a table in a research paper. The exact method of citing within a table depends on the referencing style you are using, but common methods include adding a superscript number or symbol in the cell of the table and then listing the corresponding reference in a footnote or in a reference list at the end of the paper. It is important to be consistent and clear in your referencing within tables to avoid confusion and to give credit where it is due.
here is an example of referencing within a table:
Region | Year | Sales | Expenses | Reference |
---|---|---|---|---|
North | 2010 | 5000 | 4000 | [1] |
North | 2011 | 6000 | 4500 | [2] |
South | 2010 | 6000 | 4500 | [3] |
South | 2011 | 7000 | 5000 | [4] |
In this example, the reference column lists the number of sources where the information for each row was obtained. This information can then be referenced in the text of the research paper. For example, you could write “The sales and expenses for the North region in 2010 and 2011 are shown in Table 1 and are cited in references [1] and [2].”
Copyrights, Permissions and Plagiarism Check for Tables
Tables, like other types of data and images, can be subject to copyright protection. It depends on the specific circumstances surrounding the creation and use of the table. If the table is original and creative, it may be eligible for copyright protection as a literary work. On the other hand, if the table simply presents factual information in a straightforward manner, it may not be eligible for copyright protection. It’s important to consider the legal implications before using a table in a research paper or other publication. In general, it’s advisable to obtain permission from the copyright holder or to use tables that are in the public domain or licensed under a Creative Commons license.
To determine whether a table is under copyright protection, you can consider the following factors:
- Originality: If the table is original and creative, it may be eligible for copyright protection.
- Factual information: If the table simply presents factual information in a straightforward manner, it may not be eligible for copyright protection.
- Attribution: If the table was created by someone else, you should check for any attribution or copyright information. This information may be found in the table itself, in the source material from which the table was created, or in a separate copyright notice.
- Public domain: Tables that are in the public domain are not under copyright protection. You can use these tables without permission.
- Creative Commons license: Some tables may be licensed under a Creative Commons license, which allows you to use the table with certain conditions.
It’s important to check the specific circumstances surrounding the creation and use of the table to determine whether it’s under copyright protection. If in doubt, it’s advisable to obtain permission from the copyright holder or to use tables that are in the public domain or licensed under a Creative Commons license.
Creative Commons is a nonprofit organization that provides a set of standardized licenses for creators to use when making their work available to others. These licenses are designed to help creators maintain control over their work, while also making it possible for others to use, share, and build upon that work in ways that are legal and consistent with the creator’s intentions.
Some common creative commons licenses include Attribution (CC BY), Attribution-ShareAlike (CC BY-SA), and Attribution-NoDerivs (CC BY-ND) licenses. These licenses specify how others are allowed to use a creator’s work, such as by requiring attribution, allowing derivative works, or requiring that any derivative works be shared under the same license.
To obtain a Creative Commons license, one should approach the Creative Commons organization, which provides free, flexible copyright licenses that allow creators to share their work with the public while maintaining control over their rights.
The organization provides a license wizard that allows creators to choose the license that best suits their needs and provides guidance on how to properly use the license. The license can be applied to various types of creative works, including text, images, videos, and music.
Tables are also part of the plagiarism checks in research papers. All sources and information used in a research paper, including tables, should be properly cited to avoid plagiarism. Tables created from original data and analysis are also subject to plagiarism checks, as they are considered original content. It is important to ensure that all information in a research paper, including tables, is properly cited and does not violate copyright or plagiarism laws.
To avoid plagiarism in tables in a research paper, one should follow the following guidelines:
- Always properly cite any sources used to create the table, including any data, calculations, or other information that has been used.
- Use original language and explanations when writing captions and annotations for the table.
- Use a plagiarism detection tool, such as Turnitin, to check the content of the table.
- Make sure to use different sources to create the table, rather than relying on a single source.
- Use proper referencing styles and formatting, such as MLA, APA, or Chicago style, to avoid any accidental plagiarism.
- Avoid copying tables directly from other sources, even if you have cited the source.
- Create the table yourself, using data and calculations that you have obtained independently.
- If you must use a table from another source, make sure to make substantial changes to it so that it is no longer an exact copy.
- Always be mindful of copyright laws and make sure to obtain permission to use any copyrighted materials in your table.
- Regularly review and update your table to ensure that it is in compliance with plagiarism and copyright laws.
I have written an article on The Consequences of Plagiarism: What You Need to Know? . This article will help you to understand the importance of understanding consequences of plagiarism.
In conclusion, tables are an essential tool for presenting data and information in a clear and organized manner in research papers. They are used to present complex data in a simple and easy-to-read format, which helps readers to understand the information quickly and accurately. Tables also help to save space and make it easier to compare different data sets.
Differentiating the rows in a table is an important aspect of table design, as it helps to make the information stand out and makes it easier to understand. There are several ways to differentiate rows in a table, including using different background colors, shading, bold or italic text, different font sizes or styles, and different alignments. The most appropriate method will depend on the data being presented and the purpose of the table.
Frequently Asked Questions
The number of tables in a research paper can vary depending on the specific requirements of the paper and the nature of the research being presented. As a general guideline, there is no strict rule on how many tables should be included in a 10-page research paper, as it can vary greatly depending on the research topic, methodology, and the amount of data being presented. As a rough estimate, a 10-page research paper may include anywhere from 1 to 5 tables, but this can vary significantly based on the factors mentioned above.
Yes, it is common to include tables at the end of a research paper, after the references section. This is typically done to keep the main body of the research paper focused on the narrative and analysis, while providing supplementary information, such as tables or other supporting data, at the end.
Yes, it is possible to include a table in a single column format within a two-column research paper. In a two-column format, the text typically flows in two columns, side by side, across the page. However, if you need to include a table that requires a wider layout or if it is easier to read as a single column, you can insert a table that spans the entire width of the page in a single column format.
Upcoming Events
- Visit the Upcoming International Conferences at Exotic Travel Destinations with Travel Plan
- Visit for Research Internships Worldwide
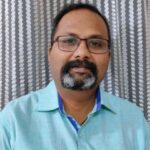
Recent Posts
- 05 Quick Review, High Impact, Best Research Journals for Submissions for July 2024
- Top Mistakes to Avoid When Writing a Research Paper
- Average Stipend for Research/Academic Internships
- These Institutes Offer Remote Research/Academic Internships
- How to Include Your Journal in the UGC-CARE List? A Guide for Publishers
- All Blog Posts
- Research Career
- Research Conference
- Research Internship
- Research Journal
- Research Tools
- Uncategorized
- Research Conferences
- Research Journals
- Research Grants
- Internships
- Research Internships
- Email Templates
- Conferences
- Blog Partners
- Privacy Policy
Copyright © 2024 Research Voyage
Design by ThemesDNA.com


An official website of the United States government
The .gov means it’s official. Federal government websites often end in .gov or .mil. Before sharing sensitive information, make sure you’re on a federal government site.
The site is secure. The https:// ensures that you are connecting to the official website and that any information you provide is encrypted and transmitted securely.
- Publications
- Account settings
Preview improvements coming to the PMC website in October 2024. Learn More or Try it out now .
- Advanced Search
- Journal List
- J Postgrad Med
- v.69(3); Jul-Sep 2023
- PMC10394528
Utilizing tables, figures, charts and graphs to enhance the readability of a research paper
Department of Pediatrics, College of Medicine and Health Sciences, National University of Science and Technology, Sohar, Sultanate of Oman
1 Department of Pediatrics, Seth G.S. Medical College and KEM Hospital, Mumbai, Maharashtra, India
Introduction
Every author aims to reach the maximum target audience through his/her research publication/s. Our previous editorials have touched upon the process of writing a quality research paper and its successful publication in an appropriate journal.[ 1 , 2 ] Journal-specific ”Instructions for Authors” generally have defined limits to the text and non-textual content for the benefit of space and presentation. Though the aim of a paper is to get its research point across through methodology, results, and discussion, readers often read the summary of data and analysis (only). Thus, the tables, figures, charts, and graphs are time and space-effective tools that not only help to understand the research presented in a simple manner but also engage and sustain the reader's interest.
Why use tables/figures/charts or graphs?
Reading text matter can often get monotonous – for the readers as well as the editors and reviewers. Using Tables/Figures/Charts or Graphs effectively provides a break from textual content monotony as well as provides an opportunity to process and connect information between text and images, promoting deeper learning. It is suggested that one non-textual element should be used for every 1000 words in a manuscript, which generally amounts to two for every three print pages.[ 3 ] The use of tables/figures/charts/graphs not only reduces the word count but also complements the text effectively. Although the text focuses on explaining findings, outlining trends, and providing contextual information, non-textual content allows readers to understand characteristics, distribution, and relationships between data, and visualize statistics/abstract concepts in a powerful manner. High-quality tables and figures also increase the likelihood of a manuscript being accepted for publication.[ 4 ] Note that the figures/artwork needs to be uploaded as separate files for most of the journals.
The CONSORT statement ( www.equator-ntework.org ) provides guidelines on how to report outcome-specific information in a published clinical trial report; however there are no definite recommendations on how to present non-textual elements, and this varies from one journal to another. Authors tend to prepare them based on their own understanding, often without much thought, and repeat the information presented in the main text. Moreover, while some journals have dedicated editors and resources to redraw or edit figures/tables, others simply publish whatever the authors submit. Thus, to improve the readability of the paper, it is primarily the author's responsibility to submit clear and useful tables, figures, charts, and graphs.
The heart of any research lies in its data, and most readers only get a glimpse of the data via the results. The closest one can get to raw statistics is through data presented in tables, figures, graphs, and supplementary material. Tables, figures, and graphs also help to classify and interpret data, highlight key findings, and present maximum data in a concise space. The author should make a deliberate decision on the presentation of his data early in the writing process. Using a sentence as text is more efficient while presenting up to half a dozen numbers in data or if the information can be summarized in three or lesser sentences.[ 5 ] Figures give an overall picture of concept (but without exact numerical data), while tables present exact values (but are less engaging and less interesting).[ 5 ] The final choice of the presentation depends on the type of data, statistical analysis, and relevant message to be delivered.[ 6 ]
General methodology of design and submission
The general structure followed by most non-textual elements is caption/legend/title, content, and footnotes. All data should be verified thoroughly for errors (especially outliers or unexpected spikes) and data sources should be cited in the footnotes/references. The presentation should be simple and clear enough for the reader to understand without any assumptions.[ 7 ] Each exhibit should be labeled clearly with a title and numbers (usually Arabic numerals) that are separate, unique, and consecutive based on their appearance in the text. The title should be self-explanatory and explain the information presented (what, where, and when) briefly. Footnotes should refer to restrictions, assumptions, abbreviations, explanatory notes, and unusual annotations. The formatting should be consistent throughout (across all tables/graphs) for easy comparison.[ 7 ] Design the figures, tables, and graphs to fit in one page on a scale that will be readable in print.[ 8 ] Always use the insert -> (arrow) page break function to ensure that each new Table/Figure/Graph is seen in the document on a new page. Data from the figures and tables should not be repeated in the text. Although tables/figures are often submitted separately or at the end of manuscript based on journal instructions, they should be referred to in the text at appropriate points by location statements i.e. Figures Figures1 1 and and2 2 or Tables Tables1 1 and and2 2 .[ 7 ] One should be careful during editing and proofreading, as contents and columns may get misplaced.[ 9 ] Ensure to follow the journal instructions regarding numbers and formats and glance through published examples in targeted journal. For additional data/tables/figures/graphs that do not fit into the journal's instructions or are still necessary to be displayed outside the word/Table limit, online appendages (or supplementary files) can be created. Do ask for feedback from experienced colleague/s (but not co-author) for the exhibit before final submissions.

A representative table already published in the JPGM earlier (reproduced from Shah S, Deshmukh CT, Tullu MS. The predictors of outcome and progression of pediatric sepsis and septic shock: A prospective observational study from western India. J Postgrad Med 2020;66:67-72 )

Representative figure/s already published in the JPGM earlier (reproduced from Mondkar SA, Tullu MS, Sathe P, Agrawal M. Lane-Hamilton syndrome – Is it really a needle in a haystack? J Postgrad Med 2022;68:162-7 )
Do’s and Don’ts while creating effective Tables.[ 8 , 9 , 12 , 14 , 15 ]
Do’s | Don’ts |
---|---|
• Reorient table (portrait to landscape) for better presentation of data if necessary. | • Don’t make crowded tables- avoid non-essential data/rows/columns (use as minimum necessary) |
• Use footnotes for single data point/similar values in a column, or few statistically significant values. | • Don’t make tables too large or complicated to follow. |
• Provide definitions of each abbreviation in the table legend or footnote so the reader does not have to refer to the text. | • Don’t repeat information from text, use tables to summarize/emphasize important or unexpected/remarkable findings. |
• Use consistent elements (uniform font/frame/box etc.) for all tables. | • If there is less or simple data, include it in the text instead. |
• Use tables to enhance/supplement text, they should be self-explanatory. | • Don’t repeat data in more than one table/figure. |
• Use title to reflect contents of table effectively. | • Do not include too much text, abbreviations, columns (the ideal table has three to five columns) or footnotes. |
• Order rows in meaningful order from top to bottom and place comparisons from left to right. |
Types of graphical representations and their characteristics.[ 5 , 6 , 7 , 8 , 20 , 21 ]
Line graphs | Depict trends or relationships between two or more variables over time. |
---|---|
Bar graphs | Compare values between discrete groups or categories. Can be oriented as vertical/horizontal with height/length representing magnitude. Data should be ordered/sorted, to identify trends- In horizontal graphs, larger values should be at the top while in vertical bars -from left to right. Both X and Y-axes begin from zero with endpoints representing largest and smallest categories- this aids visualization of gradual differences. Stacked vertical bar graph compares study groups as well as analyzes parts of each. |
Pie chart and Doughnut charts | Compare categories- parts of a whole or a larger category made up of smaller sub-categories. Shows relative frequencies/percentages, without precise values. Doughnut charts are complex pie charts with additional data series in layers. |
Histogram | Show frequency distribution of continuous data - bins are adjacent to each other but do not overlap. The column height denotes the number of samples corresponding to each bin, divided by fixed interval. |
Scatter plots | Presents a quick and clear picture of full distribution of raw data for two continuous variables as well as their relationship. Data are presented as points (for each individual/object) between X and Y axes and the pattern across multiple points demonstrates association between two variables (independent/with cause-effect relationship). Regression line can be used to determine if association between two variables can be explained. Can be used to evaluate residuals in regression analysis or visually check fit of a statistically estimated model. To highlight clusters instead of individual data points, bubble charts are used. The size of bubbles denotes to size of cluster. |
Line plots for averages | Used to represent data measured at set-time intervals or progression of a continuous variable like distance. Can be used for analyzing patterns across multiple data sets on a single line graph. X-axis denotes continuous variable; Y-axis represents scale and measurement values. Errors such as Standard Deviation must be displayed with representative values. Line plots are used primarily for averages, which are representative values of the measured data under specific conditions in the relevant group. |
Box and whisker chart | Represents variations in samples of a population. Used for non-parametric data. Expresses median and quartiles of data using box shape. Whisker extending as a line from each box represents the range of data. Individual points represent outliers, spacing between ends indicates dispersion in data. Displays data dispersion and kurtosis. Relative location of median in box demonstrates skewness. |
Three-dimensional effect | Add depth and perspective to graph but may make reading and interpreting chart difficult. |
Others | Receiver operating characteristic (ROC) curves, survival curves, regression curves by linear regression analysis, and dose-response curves. |
Copyright issues
Material from government publications/public domain may be used without seeking permission; however, permission is required for all fully borrowed, adapted, or modified tables/figures/graphs not in the public domain, usually from the publishers, with appropriate credit notes in footnotes (as stated for the Journal of Postgraduate Medicine – JPGM).[ 9 , 10 ] All data sources should be identified for tables/figures created using information from other studies.[ 9 ] Authors should seek permissions from publishers early in their writing, as their research cannot be published until all written permissions have been submitted and approved.[ 9 ] It is good practice to maintain a copy of such permissions with the corresponding author in case a dispute arises later on.
Use of tables
Tables are meant to give a systematic overview of the results and provide a richer understanding/comprehension of study participant characteristics and principal research findings.[ 11 ] Since tables deal with larger groups of data, they are suitable when all data requires equal attention and readers can selectively scan the data of interest.[ 6 ] Tables can present precise numerical values and information with different units' side-by-side but may not analyze data trends.[ 6 ] However, due to the sheer amount of data, interpretation may take longer.[ 6 ]
Generally, the first table summarizes key characteristics of the study population allowing readers to assess the generalizability of the findings. Subsequent tables present details of associations/comparisons between variables, often crude findings followed by models adjusted for confounding factors.[ 11 ] Other tables include tables of lists, tables of inclusion/exclusion criteria for review, and summary of characteristics/results of study (systematic reviews) and characteristics of participants in narrative format (qualitative studies).[ 11 ]
A good table draws attention to the data and not the table itself; the reader should be able to express an opinion about results just by looking at it.[ 12 ] It should neither be too long nor wide; designing more rows than columns makes it easier to scan in portrait orientation.[ 9 , 11 ] JPGM guidelines permit a maximum of 10 columns and 25 rows in a table.[ 10 ] They are generally created from word documents as insert table and constructed before writing the content in text.[ 9 ] Most tables consist of five basic components: title, rows, columns, data fields, and footnotes. The title/legend should be concise but sufficiently informative.[ 13 ] The first column generally lists the independent variables in rows while subsequent columns present the dependent data. Column and row headings should include group sizes and measurement units (preferably an international system of units). Stubs (row headings) on the left side of a table describe row contents and should also list numerical definitions for the data i.e. the median ± SD (normal distribution), median with IQR (non-normally distributed data), or percentages (dichotomous data)[ 9 , 14 ] Use fewest decimal points necessary for accurate reporting of data.[ 14 ] Columns should present statistical analysis and significance ( P values) to highlight key findings.[ 14 ] Use well-labeled columns with the same format throughout (mean or percentiles).[ 3 ]
Each cell (data field) should contain only one numerical value and never be blank [use dash (-), ellipsis (…) or designate as “no data”]. Abbreviations should be limited; use abbreviations like “NA” very cautiously as it may be misinterpreted as not applicable/not available/not analyzed.[ 13 ] Combine tables when single variable is cross-tabulated or divide tables with too much of data.[ 7 ]
Footnotes should be brief, define abbreviations, statistical results ( P values and level of significance) and explain restrictions/discrepancies in the data. Footnotes should be ordered starting with the title of the table and working downwards from left to right. Symbols applying to the entire table should be in the title and those applying to the entire row/column should be after the row/column heading.[ 13 ] Most journals prefer superscripted letters instead of numbers. Symbols recommended by JPGM for footnotes (in order) are: *, †, ‡, §, ||, ¶, **, ††, ‡‡.[ 10 ]
Alignment and formatting: All text should be aligned to the left and numbers to the right.[ 7 ] Data fields with decimal points, hyphens/slashes, plus/minus symbols, or parentheses are aligned to these elements. For stubs continuing onto a second line, the corresponding data field should be aligned to the top line of the stub.[ 13 ] Tables can be made more meaningful, by converting data to ratios/percentages and sorting data as per the significance of variables, generally from left to right and top to bottom.[ 7 ] Data included in tables should conform with those in the main text, and percentages in rows and columns should sum up accurately.
Most journals have specific instructions for gridlines – only the top and bottom horizontal lines are used, with no vertical lines as columns are inherently aligned.[ 7 ] If tables are used from other publications, copyright permission should be obtained to reproduce them, and they should be appropriately referenced in the legend. There may be limitations as to the number of tables allowed depending on the Journal instructions and the type of article. Some Do's and Don'ts while creating tables are summarized in Table 1 .[ 8 , 9 , 12 , 14 , 15 ] Also, a representative table already published in the JPGM earlier has been reproduced herewith for better understanding [ Figure 1 ].
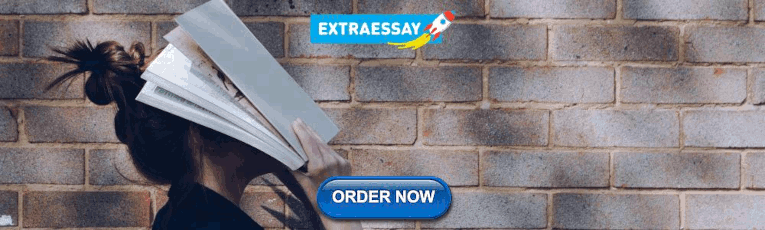
Use of figures
Figures are powerful communication tools that display patterns that are not visualized in the text or the tables. They can achieve a high educational impact by sustaining readers' interest and helping them understand trends, patterns, relationships among concepts and sequence of events.[ 3 ] Like tables, figures should be complete and self-explanatory. They should be designed thoughtfully, be relevant and be of good quality.[ 5 ] There may be limitations as to the number of figures allowed depending on the Journal instructions and type of the article. Figures can be statistical (graphs- as explained later) and non-statistical (clinical images, photographs, diagrams, illustrations and textual figures).[ 16 ] Non-statistical figures present visual information without data.[ 16 ] Clinical images and photographs [ultrasonograms, X-rays, computed tomography (CT) scans, magnetic resonance (MR) scans, images of patients, intraoperative photographs, tissue samples or microscopy findings] provide convincing and substantial information through illustrative examples from specific individuals and engage audiences, especially clinical professionals.[ 5 ] Illustrations help to explain structures, mechanisms, and relationships. Diagrams like “flowcharts”, “algorithms”, “pedigree charts”, and “maps” display complex relationships while “textual figures” describe steps of a procedure or summarize guidelines.
Structure: Figure legends (maximum of 40 words excluding credits) should be double-spaced and identified by consecutive Arabic numerals with the corresponding citation in the text. They reflect the data within, and consist of a brief title, experimental/statistical details, definitions of symbols/line or bar patterns and abbreviations/annotations.[ 15 ] Labels, numbers, and symbols should be clear, consistent, of uniform size and large enough to be legible after fitting figures to publication size.[ 15 ] Symbols, arrows, numbers, or letters used to identify parts of illustrations should be clearly identified, properly sized, placed, and explained in the legend. In case of photomicrographs, contrast the symbols/letters or arrows with background, and describe the internal scale (magnification) and method of staining.[ 10 ] If the figure has several parts (”collage”), they should be presented in order from left to right and top to bottom; this should be similarly followed for their description in the legend with labeling done as a, b, c, d, etc.[ 14 ]
Photos should have a minimum resolution of 300 dpi before digital manipulation, the acceptable formats for pictures/photos and figures in various journals being pdf, doc, ppt, jpg, gif, and tiff. Publication of color images may be chargeable which should be checked beforehand.[ 9 ] Often the print version of journal may present black and white images, with color images used in the online version.
Line diagrams: Black and white art with no shading often illustrates content better than a photograph, especially in the case of body anatomy or surgical techniques.[ 9 ] Their line weight should be consistent and not less than 0.25 pt. If scanned, they should be submitted as a tiff/jpeg image of at least 600 dpi and a width of 15 cm/6 inches.[ 14 ] Creating line diagrams may involve expensive professional help with issues of exclusive rights. Simple drawings can be scanned in a conventional office scanner at a minimum resolution of 600 dpi.[ 9 ] Drawings in shades of grey require a resolution of 1200 dpi or more, usually unavailable in regular office scanners.[ 9 ]
X-rays , which are photographic images, often lack good contrast, a problem magnified if the image must be enlarged. The quality of radiographs can be improved using Adobe Photoshop.[ 17 ] Figure captions in radiology should be utilized correctly and mention the modality, imaging plane and relevant technical information for images e.g. projection name on an x-ray, plane of a cross-sectional image, window setting of a CT section, and sequence name of an MR image.[ 17 ]
One may need to crop images to focus on the point of interest and maintain patient anonymity. Editing is usually done in tiff file format in software designed for image editing. Adjustments in brightness/contrast/color balance may help if raw image is not clear; however, it should not alter the meaning.[ 5 ] Colors should be easy to see (avoid yellow) and backgrounds should preferably be white. The tint should be no lower than 15%.[ 14 ] However, all digital modifications or enhancements of photographic images should be documented, step by step and the original raw files of unedited photographs/images should be available as supplementary files .[ 5 ]
Minimum resolution and design: Figures should be of high quality and resolution such that when final images are zoomed to 1600%, they should not blur or pixelate.[ 5 ] In case of reprints, care should be taken about picture quality.[ 3 ] JPGM requires a minimum resolution of 300 dpi or 1800 × 1600 pixels in TIFF format for digital images. Uploaded images should be within 4 MB in size and JPEG format. The JPGM also reserves the right to crop, rotate, reduce, or enlarge the photographs to an acceptable size.[ 10 ] One can use tools while creating figures and exporting data in another software; a few examples of open-source are Matplotlib (python plotting library), R, Inkscape, TikZ, PGF, GIMP, ImageMagick, D3 (Data-Driven-Documents), Cytoscape and Circos.[ 18 ]
Anonymity and Copyright: In the case of images, all unessential patient information or identifiers should be removed (masking or blurring only the eyes is no longer considered sufficient).[ 19 ] It is the author's responsibility to obtain written permission from the patient to use the photograph for educational purposes (whether the subject is identifiable or not) and archive it properly.[ 10 ] For images or descriptions that identify the patient, a statement about obtaining informed patient consent should be specified in the manuscript.[ 10 ] For figures published elsewhere, the original source should be acknowledged (via credit line in the figure legend) and the author should submit permission from copyright holder (usually the publisher) to reproduce the material before his/her manuscript is accepted.[ 3 , 19 ] Representative figure/s already published in the JPGM earlier have been reproduced herewith as an example [ Figure 2 ].
Use of graphs
Graphs allow the reader to visualize and compare data by highlighting patterns and relationships such as changes over time, frequency distribution, correlation, and relative share.[ 7 ] One should be precise with data values and presentation in graphs to avoid misinterpretation. Graphs can be created from data using the same software used for statistical analysis or by special programs. Depending on the results, data can be depicted in several different formats, such as line graphs, bar charts, data plots, maps, and pie charts.
What to use and when: The graphical format (bar graph, line graph, scatter lot, dot plot) can be decided based on the type of relationship to be shown. For example, line graphs demonstrate trends, bar graphs show magnitudes and pie charts show proportions.[ 9 , 16 ] The preferred graph also depends on the representative value of data – absolute value/fraction/average/median.[ 20 ] Graphs should accurately present findings, scale should start at zero and the axes should not be altered to make data meaningful.[ 15 ] Pie charts and 3D graphs are generally not recommended.[ 5 ] Table 2 summarizes different graphical formats with their brief description and uses.[ 5 , 6 , 7 , 8 , 20 , 21 ]
How to draw/construct: Most statistical programs create graphs with statistical computations. Special programs such as Prism and Sigmaplot can also be used.[ 14 ] Different formats can be visualized in the statistical program, and the one that best depicts the data can be chosen.[ 3 ] Actual numbers from which graphs are drawn should be provided.[ 10 ] Components of graphs include axes, labels, scales, tick/reference marks, symbols, and legends.[ 21 ] Independent variables are plotted on the horizontal axis while dependent variables on vertical axis.[ 4 ] Axis labels should be short, clear and indicate measurement variable/result, units, and number of group subjects (if any).[ 7 ] The axis scale should be proportional to data range so that visual data is not exaggerated/missed and minimum space is wasted.[ 20 ] Length of axes should be visually balanced (ratio of X to Y axis should be 1.0 to 1.3).[ 21 ] Provide explanations if the axis starts from non-zero values, is non-linear (logarithmic/exponential/rate) or scales before and after a break are different.[ 7 , 20 ] Symbols/lines/curves inside the two axes should be the most prominent features, wording in axes labels next prominent and axes and tick mark (outside of axes) least prominent.[ 21 ] Numbers and marks should be large enough to be legible even when compressed for print.[ 5 ] Symbols should be uniform and effectively used to designate important strata in figures. All graphs should be consistent in style and formatting. Footnotes should indicate P values (with appropriate statistical test) and discrepancies in data/items.[ 8 ]
A clear and concise legend (inside/outside) should describe the variables in the graph. It should also include values of lines, symbols and diagrams, abbreviations, acronyms as well as statistical tests, their levels of significance, sampling size, stains used for analysis, and magnification rate.[ 4 , 20 ] Annotations can highlight specific values/statistically significant differences in graphs.[ 20 ]
All unnecessary background lines (such as gridlines) are distracting and should be removed. The background should be the palest possible (preferably white) for the highest contrast and readability. Remove all default pre-styling formats and avoid 3D effects.[ 7 ] Data presentation can be intensified by eliminating clutter and refined in a vector graph editing program (without altering the position of marks representing data).[ 5 ] It is essential to minimize meaningless noise and maximize meaningful signals.[ 5 ]
Algorithms (combination of graph and table) are an excellent aid to demonstrate a decision tree. However, they can be difficult to construct due to decisions based on more than one variable. This presents clinical and technical difficulties in presenting all possible variations in a diagnosis or therapeutic decision.[ 9 ]
A representative graph and chart already published in the JPGM earlier has been reproduced herewith as an example [Figures [Figures3 3 and and4 4 ].

A representative graph already published in the JPGM earlier (reproduced from Bhatia S, Tullu MS, Kannan S, Gogtay NJ, Thatte UM, Lahiri KR. An unusual recurrence of antitubercular drug induced hepatotoxicity in a child. J Postgrad Med 2011;57:147-152 )

A representative chart already published in the JPGM earlier (reproduced from Agarwal S, Divecha C, Tullu MS, Deshmukh CT. A rare case of nephrotic syndrome: ‘Nailed’ the diagnosis. J Postgrad Med 2014;60:179-82 )
Use of supplementary materials
Supplementary materials refer to additional content (tables/graphs/appendices/videos/audios) that are not published in the main article. Scientific publications often come with strict word limits. Additional text or data which lengthens the print version can be accessed via digital supplementary files. Besides overcoming word restrictions, supplementary material provides additional information that is not essential in the main manuscript, but enhances understanding of research. They are available to interested readers to explore or replicate (methods/formulae/statistical models/algorithms/intervention pathways) the study for secondary research or teaching.[ 22 ] Thus, they serve as an online companion, complementing the main text. The most common supplementary files are tables and figures. Some instances of their use in various sections are as follows.[ 23 ]
In introduction: Table of summary of literature from various studies, detailed description of research topic, illustrations of concepts discussed, and glossaries of terms used.
In methodology: Participant details (sources, inclusion/exclusion lists, demography), instrumentation of constructs and variables, data collection techniques (survey questionnaires, participant forms), and data analysis techniques (coding sheets for content analysis, checklists) mathematical formulae used in calculations, data collection schedule.
In results and discussion: Additional results (often tables and figures), detailed analysis of limitations of the study or possible alternative explanations, programming code.
Other material includes references for supplementary files, translations, errata, audio, and video files.[ 23 ]
Examples of video/audio files include echocardiography recordings and ultrasound images. Specific information on the preparation of audio and video clips is available in the author guidelines. Video formats usually used are MPEG-4, QuickTime, or Windows media video. Audio supplements include WAV or MP3 format. Video size should be reduced to <10 MB and clips limited to 15–25 s. The resolution should be optimized by using video frame dimensions of 480 × 360 pixels and 640 × 480 pixels.[ 14 ]
However, supplemental material is available only in the online version- limiting immediate access to many readers.[ 5 ] Moreover, only readers with a strong interest in the research topic will access the online supplementary material.[ 5 ] The information in these files is often very extensive and not integrated with the main text appropriately, thus finding and extracting specific points from a supplement can be tedious.[ 24 ]
The utility of supplementary material varies as per the audience – additional tables and figures are more useful to readers, information about study protocol/data collection to peer reviewers, and completed checklists to journal editors. Due to the lack of guidance from journals (to both authors and reviewers) regarding its necessity or accuracy and due to the extensive nature of the files, supplementary material is rarely read/reviewed (though all the supplementary files are to be uploaded for peer-review with the main article files at the time of submission).[ 24 ] This increases the likelihood of missing errors in methods/analysis (submitted as supplementary files), thus placing the scientific accuracy and validity of the published research at risk.[ 24 ] Moreover, the availability of raw data to third parties via supplementary files raises concerns about security and data permanence.[ 22 ] The supplementary files often describe methods crucial to published research but are not included in references, thus many researchers remain uncited/unrecognized. Citations within supplementary material are also not appropriately tracked by citation indices. This can be overcome by direct hyperlinking sections of supplementary materials with the main manuscript.[ 24 ] Thus, supplementary data can be an asset if used thoughtfully; however, its indiscriminate use can hinder its actual purpose and be detrimental to the peer review process.
Concluding remarks
Tables, figures, graphs, and supplementary materials are vital tools which, when skillfully used, make complex data simple and clear to understand, within journal word restrictions. They engage and sustain interest and provide a brief visual summary narrative of study hypothesis- saving time and energy for readers, reviewers, and editors. They should be self-explanatory, complement the text and provide value to the paper. Producing clear, informative non-textual elements increases the chances of papers being published and read. Thus, the author should plan these elements early during the process of data collection/analysis and not as an afterthought. The author should have a good understanding of the types of data presentations and choose the simplest format that delivers his message best. They should be adapted to the journal's instructions to display and summarize essential content, without focusing too much on making it attractive or showcase one's technical expertise. Titles should be clear and simple, data should be consistent with results, and footnotes should be used effectively. Copyrights permissions, whenever necessary, should be obtained in advance and preserved appropriately.
- Admissions Essays
- Books and Manuscripts
- Business Proofreading and Editing
- Dissertations
- Editing Tools
- Personal Statements
- Professional Writing
- Proofreading and Editing
- Thesis Proposals
- Uncategorized
- Working From Home
- Writing Fiction
- Writing Guides
When to Use Tables and Figures
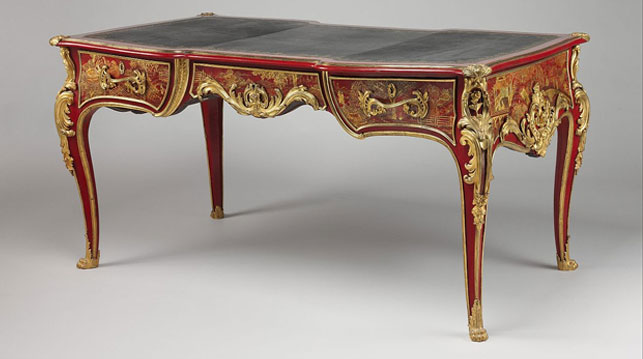
Get 400 words proofread and edited for free
In research papers, tables and figures can be helpful and even necessary to support your argument or to present a clearer, fuller picture of your topic. Creating effective tables and figures is not a simple task, however, and using a table or figure made by another author has intellectual property considerations. In today’s post, I’ll provide some information about tables and figures and some tips for including them correctly and thoughtfully .
Tables present numerical or non-numerical data in rows (across) and columns (down). They are useful to present lots of information, precise values, and data sets. Microsoft Excel is a common tool to create tables, but many specific data programs are out there for use depending on your field of study.
Get a free sample proofread and edit for your English document. Two professional proofreaders will proofread and edit your English document.
A table should have a concise title and should effectively use gridlines and consistent text formatting (e.g., bold for headings, italics for designations). Rows and columns are labeled with heads (or headings), and multiple rows or columns can be grouped and labeled together with spanner heads. Whole sections can be described with a table spanner. Units for numerical data (e.g., %, km) should be included in the relevant head, and numerical data should be rounded or not consistently.
Tables should be referred to and discussed in the body of the paper, not just plopped in. The idea is that table augments the reader’s understanding of the text by allowing them to view textual data in different ways. This may allow easy comparison of outcomes, a way to emphasize similarities and differences, an easy reference, or whatever benefits the text.
Table 1. Example showing table terminology
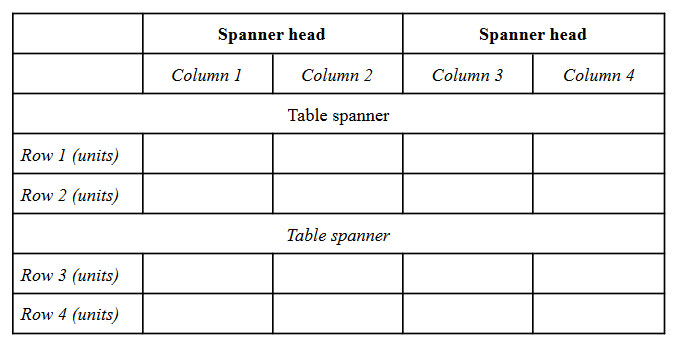
Figures are neither body text nor tabular information and may include graphs, photographs, charts, diagrams, and more. Graphs are useful to demonstrate trends or relationships in sets of data. Diagrams show processes and parts of a whole. Images/photographs allow readers to visualize in detail something specific.
Figures should have a concise, descriptive title (or caption, depending on your style guide) and, like tables, should be referred to and discussed in the body of your paper. See Figure 1 below for a common orientation of figure elements.
Figure 1. Sample figure showing common types of figures
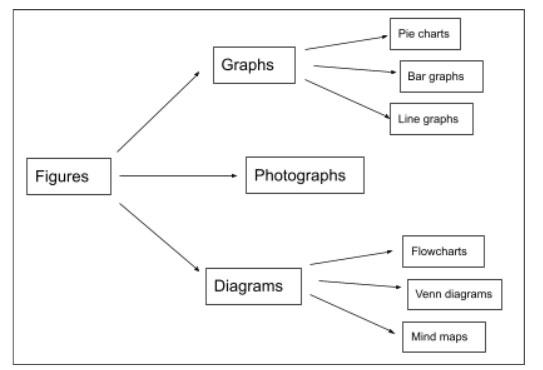
Note : Notes or citations for figures are placed here. Same goes for tables, if a note is needed. Information placed in notes is generally double-spaced like the body of your paper.
Stick Figure 1. Sample stick figure showing common types of stick figures
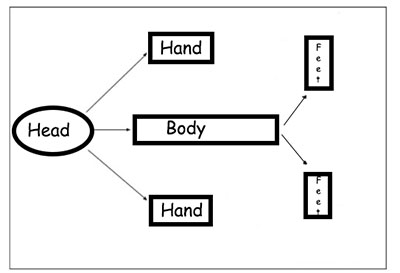
Tips and Considerations
Tables and figures can add to or detract from your paper. Follow the tips below to ensure your paper is best enhanced by additions of tables and figures.
- Style guides vary in how tables and figures should be formatted (e.g., use of a title vs. a caption for a figure, naming and numbering conventions for figures and tables). The Purdue Owl has a nice summary of information on presenting tables and figures in APA and MLA . Sheridan College has a good tip sheet on tables and figures in Chicago style. For complete information, see the full style guide of your choice.
- If you are writing for a publication, make sure to consult your press’s or publication’s author guidelines. Sometimes authors are required to follow a particular formatting or labeling system or to provide figures in a file separate from the body of the paper.
- Make sure to cite other authors’ tables and figures if you copy them into your paper or rely on them heavily to create your own tables and figures. Use a parenthetical citation below the table or figure, formatted per your style guide, or a note such as, “Note: Table adapted from ____.”
- Make sure the table or figure serves a real purpose in your paper. Tables and figures that don’t actually add to readers’ understanding of your topic don’t make your paper look smarter; they are just clutter.
- When using a graph, make sure to select the appropriate type of graph for your data. For example, a pie chart is what you want to show proportions of a whole, and a line plot is best to show change over time.
- If a table has only a couple of rows and columns, it is better to just present that information in the body of your paper in a sentence or two unless there is something specific and relevant you’re trying to show.
- Organization is key in tables, especially those with a lot of information. Careful consideration of table layout and effective use of column and row heads and spanners make information easier to digest. A well-written title and explanatory note below (as needed) also go far to make tables and figures useful. Keep in mind, readers often scan papers and look at tables and figures before reading the paper, so making them easily accessible is key.
- If including an image or graphic of some sort, make sure the resolution is such that when you insert it into your document, it is crisp and easy to see. Blurry images make your paper look sloppy.
- If you want to include both a graph and the related table, it is best to present only one, usually the graph, in the body of your paper and the other in an appendix.
Hopefully, this post is enough to get you started with tables and figures, but as abovementioned, make sure to see your style guide for more nuanced rules you may need to apply.
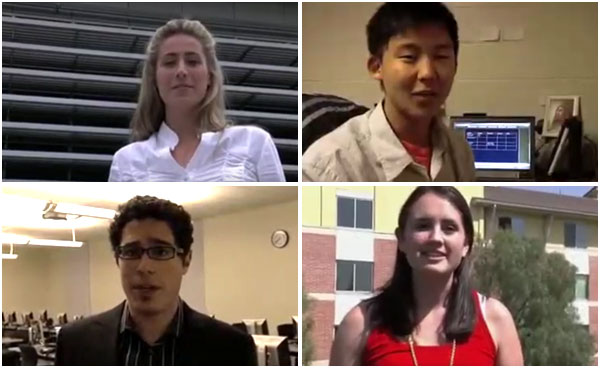
Get a free sample proofread and edit for your document. Two professional proofreaders will proofread and edit your document.
Get a Free Sample
We will get your free sample back in three to six hours!
We proofread documents 24/7 Support 888-833-8385
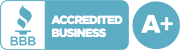
Customer Service
Get in touch.
ProofreadingPal LLC 105 Iowa Ave., Ste. 214 Iowa City, IA 52240
Call Us 888-833-8385
Live Customer Support Hours Sun.-Thurs. 8 a.m. to midnight CT and Fri.–Sat. 8 a.m. to 6 p.m. CT
Submit Documents 24/7

© 2010 - 2020 ProofreadingPal LLC - All Rights Reserved.
The Vocative Comma Is Important, People! · September 25, 2022
8 Tips to Make Your Writing Sound More Formal · August 29, 2022
Worlde Tips and Tricks · March 10, 2022
Worlde Tips and Tricks · February 25, 2022
Top 4 Misspelled Words · November 5, 2021
How to Capitalize Medicine · October 1, 2021
How to Capitalize Medicine · August 18, 2021
4 Fixes for Comment Boxes in MS Word · January 17, 2021
How to Avoid Wordiness · July 15, 2020
Write an Effective Blog Post · June 9, 2020
Proofreading Services Rates · April 19, 2020
How to Make Your Writing More Inclusive · March 5, 2020
How to Make Your Writing More Inclusive · February 27, 2020
Guide to Olde English · December 27, 2019
Guide to Olde English · December 26, 2019
Common Apostrophe Errors · December 19, 2019
Guide to Olde English · December 18, 2019
Capitalization in APA, Chicago, MLA, and AP · August 27, 2019
Avoiding Common Capitalization Errors · July 31, 2019
- Article Information
Data Sharing Statement
- As Ozempic’s Popularity Soars, Here’s What to Know About Semaglutide and Weight Loss JAMA Medical News & Perspectives May 16, 2023 This Medical News article discusses chronic weight management with semaglutide, sold under the brand names Ozempic and Wegovy. Melissa Suran, PhD, MSJ
- Patents and Regulatory Exclusivities on GLP-1 Receptor Agonists JAMA Special Communication August 15, 2023 This Special Communication used data from the US Food and Drug Administration to analyze how manufacturers of brand-name glucagon-like peptide 1 (GLP-1) receptor agonists have used patent and regulatory systems to extend periods of market exclusivity. Rasha Alhiary, PharmD; Aaron S. Kesselheim, MD, JD, MPH; Sarah Gabriele, LLM, MBE; Reed F. Beall, PhD; S. Sean Tu, JD, PhD; William B. Feldman, MD, DPhil, MPH
- What to Know About Wegovy’s Rare but Serious Adverse Effects JAMA Medical News & Perspectives December 12, 2023 This Medical News article discusses Wegovy, Ozempic, and other GLP-1 receptor agonists used for weight management and type 2 diabetes. Kate Ruder, MSJ
- GLP-1 Receptor Agonists and Gastrointestinal Adverse Events—Reply JAMA Comment & Response March 12, 2024 Ramin Rezaeianzadeh, BSc; Mohit Sodhi, MSc; Mahyar Etminan, PharmD, MSc
- GLP-1 Receptor Agonists and Gastrointestinal Adverse Events JAMA Comment & Response March 12, 2024 Karine Suissa, PhD; Sara J. Cromer, MD; Elisabetta Patorno, MD, DrPH
- GLP-1 Receptor Agonist Use and Risk of Postoperative Complications JAMA Research Letter May 21, 2024 This cohort study evaluates the risk of postoperative respiratory complications among patients with diabetes undergoing surgery who had vs those who had not a prescription fill for glucagon-like peptide 1 receptor agonists. Anjali A. Dixit, MD, MPH; Brian T. Bateman, MD, MS; Mary T. Hawn, MD, MPH; Michelle C. Odden, PhD; Eric C. Sun, MD, PhD
- Glucagon-Like Peptide-1 Receptor Agonist Use and Risk of Gallbladder and Biliary Diseases JAMA Internal Medicine Original Investigation May 1, 2022 This systematic review and meta-analysis of 76 randomized clinical trials examines the effects of glucagon-like peptide-1 receptor agonist use on the risk of gallbladder and biliary diseases. Liyun He, MM; Jialu Wang, MM; Fan Ping, MD; Na Yang, MM; Jingyue Huang, MM; Yuxiu Li, MD; Lingling Xu, MD; Wei Li, MD; Huabing Zhang, MD
- Cholecystitis Associated With the Use of Glucagon-Like Peptide-1 Receptor Agonists JAMA Internal Medicine Research Letter October 1, 2022 This case series identifies cases reported in the US Food and Drug Administration Adverse Event Reporting System of acute cholecystitis associated with use of glucagon-like peptide-1 receptor agonists that did not have gallbladder disease warnings in their labeling. Daniel Woronow, MD; Christine Chamberlain, PharmD; Ali Niak, MD; Mark Avigan, MDCM; Monika Houstoun, PharmD, MPH; Cindy Kortepeter, PharmD
See More About
Select your interests.
Customize your JAMA Network experience by selecting one or more topics from the list below.
- Academic Medicine
- Acid Base, Electrolytes, Fluids
- Allergy and Clinical Immunology
- American Indian or Alaska Natives
- Anesthesiology
- Anticoagulation
- Art and Images in Psychiatry
- Artificial Intelligence
- Assisted Reproduction
- Bleeding and Transfusion
- Caring for the Critically Ill Patient
- Challenges in Clinical Electrocardiography
- Climate and Health
- Climate Change
- Clinical Challenge
- Clinical Decision Support
- Clinical Implications of Basic Neuroscience
- Clinical Pharmacy and Pharmacology
- Complementary and Alternative Medicine
- Consensus Statements
- Coronavirus (COVID-19)
- Critical Care Medicine
- Cultural Competency
- Dental Medicine
- Dermatology
- Diabetes and Endocrinology
- Diagnostic Test Interpretation
- Drug Development
- Electronic Health Records
- Emergency Medicine
- End of Life, Hospice, Palliative Care
- Environmental Health
- Equity, Diversity, and Inclusion
- Facial Plastic Surgery
- Gastroenterology and Hepatology
- Genetics and Genomics
- Genomics and Precision Health
- Global Health
- Guide to Statistics and Methods
- Hair Disorders
- Health Care Delivery Models
- Health Care Economics, Insurance, Payment
- Health Care Quality
- Health Care Reform
- Health Care Safety
- Health Care Workforce
- Health Disparities
- Health Inequities
- Health Policy
- Health Systems Science
- History of Medicine
- Hypertension
- Images in Neurology
- Implementation Science
- Infectious Diseases
- Innovations in Health Care Delivery
- JAMA Infographic
- Law and Medicine
- Leading Change
- Less is More
- LGBTQIA Medicine
- Lifestyle Behaviors
- Medical Coding
- Medical Devices and Equipment
- Medical Education
- Medical Education and Training
- Medical Journals and Publishing
- Mobile Health and Telemedicine
- Narrative Medicine
- Neuroscience and Psychiatry
- Notable Notes
- Nutrition, Obesity, Exercise
- Obstetrics and Gynecology
- Occupational Health
- Ophthalmology
- Orthopedics
- Otolaryngology
- Pain Medicine
- Palliative Care
- Pathology and Laboratory Medicine
- Patient Care
- Patient Information
- Performance Improvement
- Performance Measures
- Perioperative Care and Consultation
- Pharmacoeconomics
- Pharmacoepidemiology
- Pharmacogenetics
- Pharmacy and Clinical Pharmacology
- Physical Medicine and Rehabilitation
- Physical Therapy
- Physician Leadership
- Population Health
- Primary Care
- Professional Well-being
- Professionalism
- Psychiatry and Behavioral Health
- Public Health
- Pulmonary Medicine
- Regulatory Agencies
- Reproductive Health
- Research, Methods, Statistics
- Resuscitation
- Rheumatology
- Risk Management
- Scientific Discovery and the Future of Medicine
- Shared Decision Making and Communication
- Sleep Medicine
- Sports Medicine
- Stem Cell Transplantation
- Substance Use and Addiction Medicine
- Surgical Innovation
- Surgical Pearls
- Teachable Moment
- Technology and Finance
- The Art of JAMA
- The Arts and Medicine
- The Rational Clinical Examination
- Tobacco and e-Cigarettes
- Translational Medicine
- Trauma and Injury
- Treatment Adherence
- Ultrasonography
- Users' Guide to the Medical Literature
- Vaccination
- Venous Thromboembolism
- Veterans Health
- Women's Health
- Workflow and Process
- Wound Care, Infection, Healing
Others Also Liked
- Download PDF
- X Facebook More LinkedIn
Sodhi M , Rezaeianzadeh R , Kezouh A , Etminan M. Risk of Gastrointestinal Adverse Events Associated With Glucagon-Like Peptide-1 Receptor Agonists for Weight Loss. JAMA. 2023;330(18):1795–1797. doi:10.1001/jama.2023.19574
Manage citations:
© 2024
- Permissions
Risk of Gastrointestinal Adverse Events Associated With Glucagon-Like Peptide-1 Receptor Agonists for Weight Loss
- 1 Faculty of Medicine, University of British Columbia, Vancouver, British Columbia, Canada
- 2 StatExpert Ltd, Laval, Quebec, Canada
- 3 Department of Ophthalmology and Visual Sciences and Medicine, University of British Columbia, Vancouver, Canada
- Medical News & Perspectives As Ozempic’s Popularity Soars, Here’s What to Know About Semaglutide and Weight Loss Melissa Suran, PhD, MSJ JAMA
- Special Communication Patents and Regulatory Exclusivities on GLP-1 Receptor Agonists Rasha Alhiary, PharmD; Aaron S. Kesselheim, MD, JD, MPH; Sarah Gabriele, LLM, MBE; Reed F. Beall, PhD; S. Sean Tu, JD, PhD; William B. Feldman, MD, DPhil, MPH JAMA
- Medical News & Perspectives What to Know About Wegovy’s Rare but Serious Adverse Effects Kate Ruder, MSJ JAMA
- Comment & Response GLP-1 Receptor Agonists and Gastrointestinal Adverse Events—Reply Ramin Rezaeianzadeh, BSc; Mohit Sodhi, MSc; Mahyar Etminan, PharmD, MSc JAMA
- Comment & Response GLP-1 Receptor Agonists and Gastrointestinal Adverse Events Karine Suissa, PhD; Sara J. Cromer, MD; Elisabetta Patorno, MD, DrPH JAMA
- Research Letter GLP-1 Receptor Agonist Use and Risk of Postoperative Complications Anjali A. Dixit, MD, MPH; Brian T. Bateman, MD, MS; Mary T. Hawn, MD, MPH; Michelle C. Odden, PhD; Eric C. Sun, MD, PhD JAMA
- Original Investigation Glucagon-Like Peptide-1 Receptor Agonist Use and Risk of Gallbladder and Biliary Diseases Liyun He, MM; Jialu Wang, MM; Fan Ping, MD; Na Yang, MM; Jingyue Huang, MM; Yuxiu Li, MD; Lingling Xu, MD; Wei Li, MD; Huabing Zhang, MD JAMA Internal Medicine
- Research Letter Cholecystitis Associated With the Use of Glucagon-Like Peptide-1 Receptor Agonists Daniel Woronow, MD; Christine Chamberlain, PharmD; Ali Niak, MD; Mark Avigan, MDCM; Monika Houstoun, PharmD, MPH; Cindy Kortepeter, PharmD JAMA Internal Medicine
Glucagon-like peptide 1 (GLP-1) agonists are medications approved for treatment of diabetes that recently have also been used off label for weight loss. 1 Studies have found increased risks of gastrointestinal adverse events (biliary disease, 2 pancreatitis, 3 bowel obstruction, 4 and gastroparesis 5 ) in patients with diabetes. 2 - 5 Because such patients have higher baseline risk for gastrointestinal adverse events, risk in patients taking these drugs for other indications may differ. Randomized trials examining efficacy of GLP-1 agonists for weight loss were not designed to capture these events 2 due to small sample sizes and short follow-up. We examined gastrointestinal adverse events associated with GLP-1 agonists used for weight loss in a clinical setting.
We used a random sample of 16 million patients (2006-2020) from the PharMetrics Plus for Academics database (IQVIA), a large health claims database that captures 93% of all outpatient prescriptions and physician diagnoses in the US through the International Classification of Diseases, Ninth Revision (ICD-9) or ICD-10. In our cohort study, we included new users of semaglutide or liraglutide, 2 main GLP-1 agonists, and the active comparator bupropion-naltrexone, a weight loss agent unrelated to GLP-1 agonists. Because semaglutide was marketed for weight loss after the study period (2021), we ensured all GLP-1 agonist and bupropion-naltrexone users had an obesity code in the 90 days prior or up to 30 days after cohort entry, excluding those with a diabetes or antidiabetic drug code.
Patients were observed from first prescription of a study drug to first mutually exclusive incidence (defined as first ICD-9 or ICD-10 code) of biliary disease (including cholecystitis, cholelithiasis, and choledocholithiasis), pancreatitis (including gallstone pancreatitis), bowel obstruction, or gastroparesis (defined as use of a code or a promotility agent). They were followed up to the end of the study period (June 2020) or censored during a switch. Hazard ratios (HRs) from a Cox model were adjusted for age, sex, alcohol use, smoking, hyperlipidemia, abdominal surgery in the previous 30 days, and geographic location, which were identified as common cause variables or risk factors. 6 Two sensitivity analyses were undertaken, one excluding hyperlipidemia (because more semaglutide users had hyperlipidemia) and another including patients without diabetes regardless of having an obesity code. Due to absence of data on body mass index (BMI), the E-value was used to examine how strong unmeasured confounding would need to be to negate observed results, with E-value HRs of at least 2 indicating BMI is unlikely to change study results. Statistical significance was defined as 2-sided 95% CI that did not cross 1. Analyses were performed using SAS version 9.4. Ethics approval was obtained by the University of British Columbia’s clinical research ethics board with a waiver of informed consent.
Our cohort included 4144 liraglutide, 613 semaglutide, and 654 bupropion-naltrexone users. Incidence rates for the 4 outcomes were elevated among GLP-1 agonists compared with bupropion-naltrexone users ( Table 1 ). For example, incidence of biliary disease (per 1000 person-years) was 11.7 for semaglutide, 18.6 for liraglutide, and 12.6 for bupropion-naltrexone and 4.6, 7.9, and 1.0, respectively, for pancreatitis.
Use of GLP-1 agonists compared with bupropion-naltrexone was associated with increased risk of pancreatitis (adjusted HR, 9.09 [95% CI, 1.25-66.00]), bowel obstruction (HR, 4.22 [95% CI, 1.02-17.40]), and gastroparesis (HR, 3.67 [95% CI, 1.15-11.90) but not biliary disease (HR, 1.50 [95% CI, 0.89-2.53]). Exclusion of hyperlipidemia from the analysis did not change the results ( Table 2 ). Inclusion of GLP-1 agonists regardless of history of obesity reduced HRs and narrowed CIs but did not change the significance of the results ( Table 2 ). E-value HRs did not suggest potential confounding by BMI.
This study found that use of GLP-1 agonists for weight loss compared with use of bupropion-naltrexone was associated with increased risk of pancreatitis, gastroparesis, and bowel obstruction but not biliary disease.
Given the wide use of these drugs, these adverse events, although rare, must be considered by patients who are contemplating using the drugs for weight loss because the risk-benefit calculus for this group might differ from that of those who use them for diabetes. Limitations include that although all GLP-1 agonist users had a record for obesity without diabetes, whether GLP-1 agonists were all used for weight loss is uncertain.
Accepted for Publication: September 11, 2023.
Published Online: October 5, 2023. doi:10.1001/jama.2023.19574
Correction: This article was corrected on December 21, 2023, to update the full name of the database used.
Corresponding Author: Mahyar Etminan, PharmD, MSc, Faculty of Medicine, Departments of Ophthalmology and Visual Sciences and Medicine, The Eye Care Center, University of British Columbia, 2550 Willow St, Room 323, Vancouver, BC V5Z 3N9, Canada ( [email protected] ).
Author Contributions: Dr Etminan had full access to all of the data in the study and takes responsibility for the integrity of the data and the accuracy of the data analysis.
Concept and design: Sodhi, Rezaeianzadeh, Etminan.
Acquisition, analysis, or interpretation of data: All authors.
Drafting of the manuscript: Sodhi, Rezaeianzadeh, Etminan.
Critical review of the manuscript for important intellectual content: All authors.
Statistical analysis: Kezouh.
Obtained funding: Etminan.
Administrative, technical, or material support: Sodhi.
Supervision: Etminan.
Conflict of Interest Disclosures: None reported.
Funding/Support: This study was funded by internal research funds from the Department of Ophthalmology and Visual Sciences, University of British Columbia.
Role of the Funder/Sponsor: The funder had no role in the design and conduct of the study; collection, management, analysis, and interpretation of the data; preparation, review, or approval of the manuscript; and decision to submit the manuscript for publication.
Data Sharing Statement: See Supplement .
- Register for email alerts with links to free full-text articles
- Access PDFs of free articles
- Manage your interests
- Save searches and receive search alerts
Unfortunately we don't fully support your browser. If you have the option to, please upgrade to a newer version or use Mozilla Firefox , Microsoft Edge , Google Chrome , or Safari 14 or newer. If you are unable to, and need support, please send us your feedback .
We'd appreciate your feedback. Tell us what you think! opens in new tab/window
Sharing research data
As a researcher, you are increasingly encouraged, or even mandated, to make your research data available, accessible, discoverable and usable.
Sharing research data is something we are passionate about too, so we’ve created this short video and written guide to help you get started.
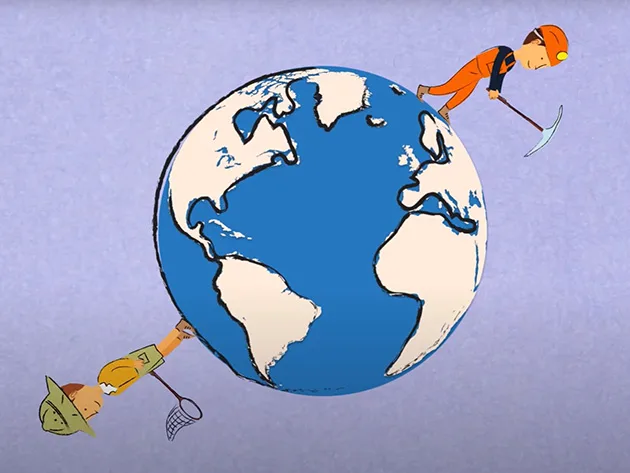
Research Data
What is research data.
While the definition often differs per field, generally, research data refers to the results of observations or experiments that validate your research findings. These span a range of useful materials associated with your research project, including:
Raw or processed data files
Research data does not include text in manuscript or final published article form, or data or other materials submitted and published as part of a journal article.
Why should I share my research data?
There are so many good reasons. We’ve listed just a few:
How you benefit
You get credit for the work you've done
Leads to more citations! 1
Can boost your number of publications
Increases your exposure and may lead to new collaborations
What it means for the research community
It's easy to reuse and reinterpret your data
Duplication of experiments can be avoided
New insights can be gained, sparking new lines of inquiry
Empowers replication
And society at large…
Greater transparency boosts public faith in research
Can play a role in guiding government policy
Improves access to research for those outside health and academia
Benefits the public purse as funding of repeat work is reduced
How do I share my research data?
The good news is it’s easy.
Yet to submit your research article? There are a number of options available. These may vary depending on the journal you have chosen, so be sure to read the Research Data section in its Guide for Authors before you begin.
Already published your research article? No problem – it’s never too late to share the research data associated with it.
Two of the most popular data sharing routes are:
Publishing a research elements article
These brief, peer-reviewed articles complement full research papers and are an easy way to receive proper credit and recognition for the work you have done. Research elements are research outputs that have come about as a result of following the research cycle – this includes things like data, methods and protocols, software, hardware and more.
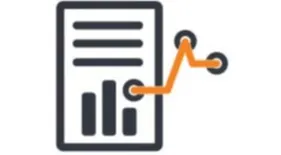
You can publish research elements articles in several different Elsevier journals, including our suite of dedicated Research Elements journals . They are easy to submit, are subject to a peer review process, receive a DOI and are fully citable. They also make your work more sharable, discoverable, comprehensible, reusable and reproducible.
The accompanying raw data can still be placed in a repository of your choice (see below).
Uploading your data to a repository like Mendeley Data
Mendeley Data is a certified, free-to-use repository that hosts open data from all disciplines, whatever its format (e.g. raw and processed data, tables, codes and software). With many Elsevier journals, it’s possible to upload and store your data to Mendeley Data during the manuscript submission process. You can also upload your data directly to the repository. In each case, your data will receive a DOI, making it independently citable and it can be linked to any associated article on ScienceDirect, making it easy for readers to find and reuse.
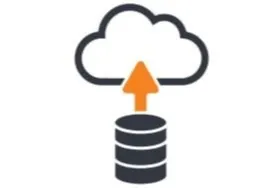
View an article featuring Mendeley data opens in new tab/window (just select the Research Data link in the left-hand bar or scroll down the page).
What if I can’t submit my research data?
Data statements offer transparency.
We understand that there are times when the data is simply not available to post or there are good reasons why it shouldn’t be shared. A number of Elsevier journals encourage authors to submit a data statement alongside their manuscript. This statement allows you to clearly explain the data you’ve used in the article and the reasons why it might not be available. The statement will appear with the article on ScienceDirect.
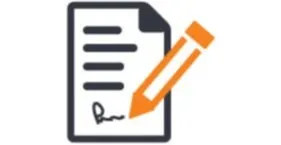
View a sample data statement opens in new tab/window (just select the Research Data link in the left-hand bar or scroll down the page).
Showcasing your research data on ScienceDirect
We have 3 top tips to help you maximize the impact of your data in your article on ScienceDirect.
Link with data repositories
You can create bidirectional links between any data repositories you’ve used to store your data and your online article. If you’ve published a data article, you can link to that too.
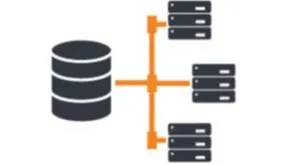
Enrich with interactive data visualizations
The days of being confined to static visuals are over. Our in-article interactive viewers let readers delve into the data with helpful functions such as zoom, configurable display options and full screen mode.
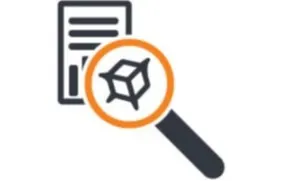
Cite your research data
Get credit for your work by citing your research data in your article and adding a data reference to the reference list. This ensures you are recognized for the data you shared and/or used in your research. Read the References section in your chosen journal’s Guide for Authors for more information.
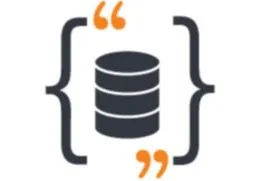
Ready to get started?
If you have yet to publish your research paper, the first step is to find the right journal for your submission and read the Guide for Authors .
Find a journal by matching paper title and abstract of your manuscript in Elsevier's JournalFinder opens in new tab/window
Find journal by title opens in new tab/window
Already published? Just view the options for sharing your research data above.
1 Several studies have now shown that making data available for an article increases article citations.
Thank you for visiting nature.com. You are using a browser version with limited support for CSS. To obtain the best experience, we recommend you use a more up to date browser (or turn off compatibility mode in Internet Explorer). In the meantime, to ensure continued support, we are displaying the site without styles and JavaScript.
- View all journals
- Explore content
- About the journal
- Publish with us
- Sign up for alerts
- Open access
- Published: 12 July 2024
The nature of the last universal common ancestor and its impact on the early Earth system
- Edmund R. R. Moody ORCID: orcid.org/0000-0002-8785-5006 1 ,
- Sandra Álvarez-Carretero ORCID: orcid.org/0000-0002-9508-6286 1 ,
- Tara A. Mahendrarajah ORCID: orcid.org/0000-0001-7032-6581 2 ,
- James W. Clark 3 ,
- Holly C. Betts 1 ,
- Nina Dombrowski ORCID: orcid.org/0000-0003-1917-2577 2 ,
- Lénárd L. Szánthó ORCID: orcid.org/0000-0003-3363-2488 4 , 5 , 6 ,
- Richard A. Boyle 7 ,
- Stuart Daines 7 ,
- Xi Chen ORCID: orcid.org/0000-0001-7098-607X 8 ,
- Nick Lane ORCID: orcid.org/0000-0002-5433-3973 9 ,
- Ziheng Yang ORCID: orcid.org/0000-0003-3351-7981 9 ,
- Graham A. Shields ORCID: orcid.org/0000-0002-7828-3966 8 ,
- Gergely J. Szöllősi 5 , 6 , 10 ,
- Anja Spang ORCID: orcid.org/0000-0002-6518-8556 2 , 11 ,
- Davide Pisani ORCID: orcid.org/0000-0003-0949-6682 1 , 12 ,
- Tom A. Williams ORCID: orcid.org/0000-0003-1072-0223 12 ,
- Timothy M. Lenton ORCID: orcid.org/0000-0002-6725-7498 7 &
- Philip C. J. Donoghue ORCID: orcid.org/0000-0003-3116-7463 1
Nature Ecology & Evolution ( 2024 ) Cite this article
58k Accesses
925 Altmetric
Metrics details
- Microbial genetics
- Molecular evolution
- Phylogenetics
The nature of the last universal common ancestor (LUCA), its age and its impact on the Earth system have been the subject of vigorous debate across diverse disciplines, often based on disparate data and methods. Age estimates for LUCA are usually based on the fossil record, varying with every reinterpretation. The nature of LUCA’s metabolism has proven equally contentious, with some attributing all core metabolisms to LUCA, whereas others reconstruct a simpler life form dependent on geochemistry. Here we infer that LUCA lived ~4.2 Ga (4.09–4.33 Ga) through divergence time analysis of pre-LUCA gene duplicates, calibrated using microbial fossils and isotope records under a new cross-bracing implementation. Phylogenetic reconciliation suggests that LUCA had a genome of at least 2.5 Mb (2.49–2.99 Mb), encoding around 2,600 proteins, comparable to modern prokaryotes. Our results suggest LUCA was a prokaryote-grade anaerobic acetogen that possessed an early immune system. Although LUCA is sometimes perceived as living in isolation, we infer LUCA to have been part of an established ecological system. The metabolism of LUCA would have provided a niche for other microbial community members and hydrogen recycling by atmospheric photochemistry could have supported a modestly productive early ecosystem.
Similar content being viewed by others
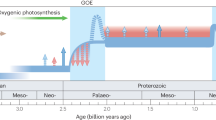
Co‐evolution of early Earth environments and microbial life
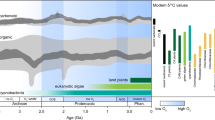
The curious consistency of carbon biosignatures over billions of years of Earth-life coevolution
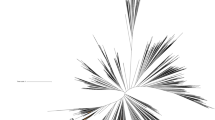
Phanerozoic radiation of ammonia oxidizing bacteria
The common ancestry of all extant cellular life is evidenced by the universal genetic code, machinery for protein synthesis, shared chirality of the almost-universal set of 20 amino acids and use of ATP as a common energy currency 1 . The last universal common ancestor (LUCA) is the node on the tree of life from which the fundamental prokaryotic domains (Archaea and Bacteria) diverge. As such, our understanding of LUCA impacts our understanding of the early evolution of life on Earth. Was LUCA a simple or complex organism? What kind of environment did it inhabit and when? Previous estimates of LUCA are in conflict either due to conceptual disagreement about what LUCA is 2 or as a result of different methodological approaches and data 3 , 4 , 5 , 6 , 7 , 8 , 9 . Published analyses differ in their inferences of LUCA’s genome, from conservative estimates of 80 orthologous proteins 10 up to 1,529 different potential gene families 4 . Interpretations range from little beyond an information-processing and metabolic core 6 through to a prokaryote-grade organism with much of the gene repertoire of modern Archaea and Bacteria 8 , recently reviewed in ref. 7 . Here we use molecular clock methodology, horizontal gene-transfer-aware phylogenetic reconciliation and existing biogeochemical models to address questions about LUCA’s age, gene content, metabolism and impact on the early Earth system.
Estimating the age of LUCA
Life’s evolutionary timescale is typically calibrated to the oldest fossil occurrences. However, the veracity of fossil discoveries from the early Archaean period has been contested 11 , 12 . Relaxed Bayesian node-calibrated molecular clock approaches provide a means of integrating the sparse fossil and geochemical record of early life with the information provided by molecular data; however, constraining LUCA’s age is challenging due to limited prokaryote fossil calibrations and the uncertainty in their placement on the phylogeny. Molecular clock estimates of LUCA 13 , 14 , 15 have relied on conserved universal single-copy marker genes within phylogenies for which LUCA represented the root. Dating the root of a tree is difficult because errors propagate from the tips to the root of the dated phylogeny and information is not available to estimate the rate of evolution for the branch incident on the root node. Therefore, we analysed genes that duplicated before LUCA with two (or more) copies in LUCA’s genome 16 . The root in these gene trees represents this duplication preceding LUCA, whereas LUCA is represented by two descendant nodes. Use of these universal paralogues also has the advantage that the same calibrations can be applied at least twice. After duplication, the same species divergences are represented on both sides of the gene tree 17 , 18 and thus can be assumed to have the same age. This considerably reduces the uncertainty when genetic distance (branch length) is resolved into absolute time and rate. When a shared node is assigned a fossil calibration, such cross-bracing also serves to double the number of calibrations on the phylogeny, improving divergence time estimates. We calibrated our molecular clock analyses using 13 calibrations (see ‘Fossil calibrations’ in Supplementary Information ). The calibration on the root of the tree of life is of particular importance. Some previous studies have placed a younger maximum constraint on the age of LUCA based on the assumption that life could not have survived Late Heavy Bombardment (LHB) (~3.7–3.9 billion years ago (Ga)) 19 . However, the LHB hypothesis is extrapolated and scaled from the Moon’s impact record, the interpretation of which has been questioned in terms of the intensity, duration and even the veracity of an LHB episode 20 , 21 , 22 , 23 . Thus, the LHB hypothesis should not be considered a credible maximum constraint on the age of LUCA. We used soft-uniform bounds, with the maximum-age bound based on the time of the Moon-forming impact (4,510 million years ago (Ma) ± 10 Myr), which would have effectively sterilized Earth’s precursors, Tellus and Theia 13 . Our minimum bound on the age of LUCA is based on low δ 98 Mo isotope values indicative of Mn oxidation compatible with oxygenic photosynthesis and, therefore, total-group Oxyphotobacteria in the Mozaan Group, Pongola Supergroup, South Africa 24 , 25 , dated minimally to 2,954 Ma ± 9 Myr (ref. 26 ).
Our estimates for the age of LUCA are inferred with a concatenated and a partitioned dataset, both consisting of five pre-LUCA paralogues: catalytic and non-catalytic subunits from ATP synthases, elongation factor Tu and G, signal recognition protein and signal recognition particle receptor, tyrosyl-tRNA and tryptophanyl-tRNA synthetases, and leucyl- and valyl-tRNA synthetases 27 . Marginal densities (commonly referred to as effective priors) fall within calibration densities (that is, user-specified priors) when topologically adjacent calibrations do not overlap temporally, but may differ when they overlap, to ensure the relative age relationships between ancestor-descendant nodes. We consider the marginal densities a reasonable interpretation of the calibration evidence given the phylogeny; we are not attempting to test the hypothesis that the fossil record is an accurate temporal archive of evolutionary history because it is not 28 . The duplicated LUCA node age estimates we obtained under the autocorrelated rates (geometric Brownian motion (GBM)) 29 , 30 and independent-rates log-normal (ILN) 31 , 32 relaxed-clock models with our partitioned dataset (GBM, 4.18–4.33 Ga; ILN, 4.09–4.32 Ga; Fig. 1 ) fall within our composite age estimate for LUCA ranging from 3.94 Ga to 4.52 Ga, comparable to previous studies 13 , 18 , 33 . Dating analyses based on single genes, or concatenations that excluded each gene in turn, returned compatible timescales (Extended Data Figs. 1 and 2 and ‘Additional methods’ in Methods ).
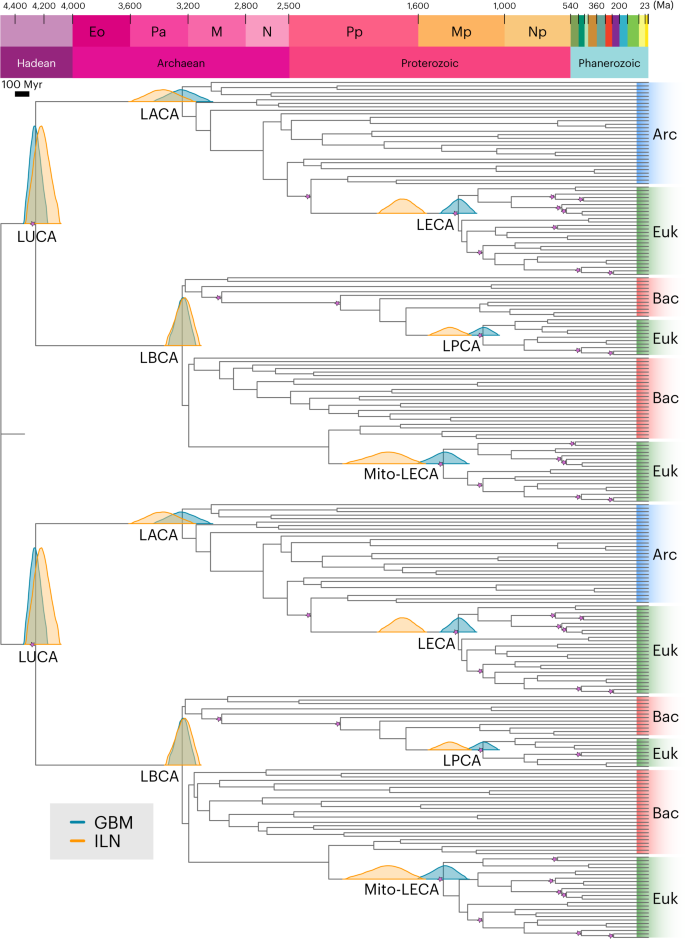
Our results suggest that LUCA lived around 4.2 Ga, with a 95% confidence interval spanning 4.09–4.33 Ga under the ILN relaxed-clock model (orange) and 4.18–4.33 Ga under the GBM relaxed-clock model (teal). Under a cross-bracing approach, nodes corresponding to the same species divergences (that is, mirrored nodes) have the same posterior time densities. This figure shows the corresponding posterior time densities of the mirrored nodes for the last universal, archaeal, bacterial and eukaryotic common ancestors (LUCA, LACA, LBCA and LECA, respectively); the last common ancestor of the mitochondrial lineage (Mito-LECA); and the last plastid-bearing common ancestor (LPCA). Purple stars indicate nodes calibrated with fossils. Arc, Archaea; Bac, Bacteria; Euk, Eukarya.
LUCA’s physiology
To estimate the physiology of LUCA, we first inferred an updated microbial phylogeny from 57 phylogenetic marker genes (see ‘Universal marker genes’ in Methods ) on 700 genomes, comprising 350 Archaea and 350 Bacteria 15 . This tree was in good agreement with recent phylogenies of the archaeal and bacterial domains of life 34 , 35 . For example, the TACK 36 and Asgard clades of Archaea 37 , 38 , 39 and Gracilicutes within Bacteria 40 , 41 were recovered as monophyletic. However, the analysis was equivocal as to the phylogenetic placement of the Patescibacteria (CPR) 42 and DPANN 43 , which are two small-genome lineages that have been difficult to place in trees. Approximately unbiased 44 tests could not distinguish the placement of these clades, neither at the root of their respective domains nor in derived positions, with CPR sister to Chloroflexota (as reported recently in refs. 35 , 41 , 45 ) and DPANN sister to Euryarchaeota. To account for this phylogenetic uncertainty, we performed LUCA reconstructions on two trees: our maximum likelihood (ML) tree (topology 1; Extended Data Fig. 3 ) and a tree in which CPR were placed as the sister of Chloroflexota, with DPANN sister to all other Archaea (topology 2; Extended Data Fig. 4 ). In both cases, the gene families mapped to LUCA were very similar (correlation of LUCA presence probabilities (PP), r = 0.6720275, P < 2.2 × 10 − 16 ). We discuss the results on the tree with topology 2 and discuss the residual differences in Supplementary Information , ‘Topology 1’ (Supplementary Data 1 ).
We used the probabilistic gene- and species-tree reconciliation algorithm ALE 46 to infer the evolution of gene family trees for each sampled entry in the KEGG Orthology (KO) database 47 on our species tree. ALE infers the history of gene duplications, transfers and losses based on a comparison between a distribution of bootstrapped gene trees and the reference species tree, allowing us to estimate the probability that the gene family was present at a node in the tree 35 , 48 , 49 . This reconciliation approach has several advantages for drawing inferences about LUCA. Most gene families have experienced gene transfer since the time of LUCA 50 , 51 and so explicitly modelling transfers enables us to include many more gene families in the analysis than has been possible using previous approaches. As the analysis is probabilistic, we can also account for uncertainty in gene family origins and evolutionary history by averaging over different scenarios using the reconciliation model. Using this approach, we estimated the probability that each KEGG gene family (KO) was present in LUCA and then used the resulting probabilities to construct a hypothetical model of LUCA’s gene content, metabolic potential (Fig. 2 ) and environmental context (Fig. 3 ). Using the KEGG annotation is beneficial because it allows us to connect our inferences to curated functional annotations; however, it has the drawback that some widespread gene families that were likely present in LUCA are divided into multiple KO families that individually appear to be restricted to particular taxonomic groups and inferred to have arisen later. To account for this limitation, we also performed an analysis of COG (Clusters of Orthologous Genes) 52 gene families, which correspond to more coarse-grained functional annotations (Supplementary Data 2 ).
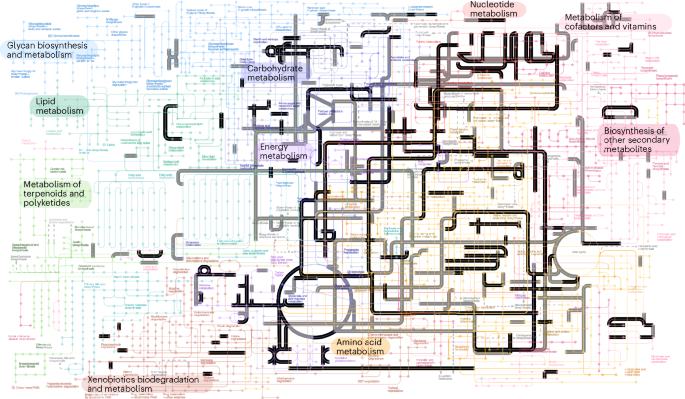
In black: enzymes and metabolic pathways inferred to be present in LUCA with at least PP = 0.75, with sampling in both prokaryotic domains. In grey: those inferred in our least-stringent threshold of PP = 0.50. The analysis supports the presence of a complete WLP and an almost complete TCA cycle across multiple confidence thresholds. Metabolic maps derived from KEGG 47 database through iPath 109 . GPI, glycosylphosphatidylinositol; DDT, 1,1,1-trichloro-2,2-bis(p-chlorophenyl)ethane.
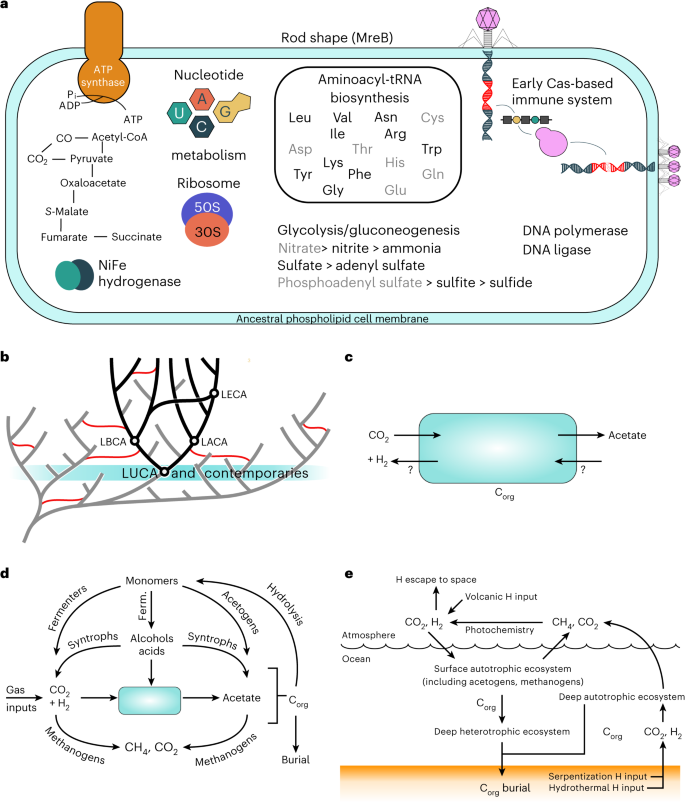
a , A representation of LUCA based on our ancestral gene content reconstruction. Gene names in black have been inferred to be present in LUCA under the most-stringent threshold (PP = 0.75, sampled in both domains); those in grey are present at the least-stringent threshold (PP = 0.50, without a requirement for presence in both domains). b , LUCA in the context of the tree of life. Branches on the tree of life that have left sampled descendants today are coloured black, those that have left no sampled descendants are in grey. As the common ancestor of extant cellular life, LUCA is the oldest node that can be reconstructed using phylogenetic methods. It would have shared the early Earth with other lineages (highlighted in teal) that have left no descendants among sampled cellular life today. However, these lineages may have left a trace in modern organisms by transferring genes into the sampled tree of life (red lines) before their extinction. c , LUCA’s chemoautotrophic metabolism probably relied on gas exchange with the immediate environment to achieve organic carbon (C org ) fixation via acetogenesis and it may also have run the metabolism in reverse. d , LUCA within the context of an early ecosystem. The CO 2 and H 2 that fuelled LUCA’s plausibly acetogenic metabolism could have come from both geochemical and biotic inputs. The organic matter and acetate that LUCA produced could have created a niche for other metabolisms, including ones that recycled CO 2 and H 2 (as in modern sediments). e , LUCA in an Earth system context. Acetogenic LUCA could have been a key part of both surface and deep (chemo)autotrophic ecosystems, powered by H 2 . If methanogens were also present, hydrogen would be released as CH 4 to the atmosphere, converted to H 2 by photochemistry and thus recycled back to the surface ecosystem, boosting its productivity. Ferm., fermentation.
Genome size and cellular features
By using modern prokaryotic genomes as training data, we used a predictive model to estimate the genome size and the number of protein families encoded by LUCA based on the relationship between the number of KEGG gene families and the total number of proteins encoded by modern prokaryote genomes (Extended Data Figs. 5 and 6 ). On the basis of the PPs for KEGG KO gene families, we identified a conservative subset of 399 KOs that were likely to be present in LUCA, with PPs ≥0.75, and found in both Archaea and Bacteria (Supplementary Data 1 ); these families form the basis of our metabolic reconstruction. However, by integrating over the inferred PPs of all KO gene families, including those with low probabilities, we also estimate LUCA’s genome size. Our predictive model estimates a genome size of 2.75 Mb (2.49–2.99 Mb) encoding 2,657 (2,451–2,855) proteins ( Methods ). Although we can estimate the number of genes in LUCA’s genome, it is more difficult to identify the specific gene families that might have already been present in LUCA based on the genomes of modern Archaea and Bacteria. It is likely that the modern version of the pathways would be considered incomplete based on LUCA’s gene content through subsequent evolutionary changes. We should therefore expect reconstructions of metabolic pathways to be incomplete due to this phylogenetic noise and other limitations of the analysis pipeline. For example, when looking at genes and pathways that can uncontroversially be mapped to LUCA, such as the ribosome and aminoacyl-tRNA synthetases for implementing the genetic code, we find that we map many (but not all) of the key components to LUCA (see ‘Notes’ in Supplementary Information ). We interpret this to mean that our reconstruction is probably incomplete but our interpretation of LUCA’s metabolism relies on our inference of pathways, not individual genes.
The inferred gene content of LUCA suggests it was an anaerobe as we do not find support for the presence of terminal oxidases (Supplementary Data 1 ). Instead we identified almost all genes encoding proteins of the archaeal (and most of the bacterial) versions of the Wood–Ljungdahl pathway (WLP) (PP > 0.7), indicating that LUCA had the potential for acetogenic growth and/or carbon fixation 53 , 54 , 55 (Supplementary Data 3 ). LUCA encoded some NiFe hydrogenase subunits ( K06281 , PP = 0.90; K14126 , PP = 0.92), which may have enabled growth on hydrogen (see ‘Notes’ in Supplementary Information ). Complexes involved in methanogenesis such as methyl-coenzyme M reductase and tetrahydromethanopterin S-methyltransferase were inferred to be absent, suggesting that LUCA was unlikely to function as a modern methanogen. We found strong support for some components of the TCA cycle (including subunits of oxoglutarate/2-oxoacid ferredoxin oxidoreductase ( K00175 and K00176 ), succinate dehydrogenase ( K00239 ) and homocitrate synthase ( K02594 )), although some steps are missing. LUCA was probably capable of gluconeogenesis/glycolysis in that we find support for most subunits of enzymes involved in these pathways (Supplementary Data 1 and 3 ). Considering the presence of the WLP, this may indicate that LUCA had the ability to grow organoheterotrophically and potentially also autotrophically. Gluconeogenesis would have been important in linking carbon fixation to nucleotide biosynthesis via the pentose phosphate pathway, most enzymes of which seem to be present in LUCA (see ‘Notes’ in Supplementary Information ). We found no evidence that LUCA was photosynthetic, with low PPs for almost all components of oxygenic and anoxygenic photosystems (Supplementary Data 3 ).
We find strong support for the presence of ATP synthase, specifically, the A ( K02117 , PP = 0.98) and B ( K02118 , PP = 0.94) subunit components of the hydrophilic V/A1 subunit, and the I (subunit a, K02123 , PP = 0.99) and K (subunit c, K02124 , PP = 0.82) subunits of the transmembrane V/A0 subunit. In addition, if we relax the sampling threshold, we also infer the presence of the F1-type β-subunit ( K02112 , PP = 0.94). This is consistent with many previous studies that have mapped ATP synthase subunits to LUCA 6 , 17 , 18 , 56 , 57 .
We obtain moderate support for the presence of pathways for assimilatory nitrate (ferredoxin-nitrate reductase, K00367 , PP = 0.69; ferredoxin-nitrite reductase, K00367 , PP = 0.53) and sulfate reduction (sulfate adenylyltransferase, K00957 , PP = 0.80, and K00958 , PP = 0.73; sulfite reductase, K00392 , PP = 0.82; phosphoadenosine phosphosulfate reductase, K00390 , PP = 0.56), probably to fuel amino acid biosynthesis, for which we inferred the presence of 37 partially complete pathways.
We found support for the presence of 19 class 1 CRISPR–Cas effector protein families in the genome of LUCA, including types I and III (cas3, K07012 , PP = 0.80, and K07475 , PP = 0.74; cas10, K07016 , PP = 0.96, and K19076 , PP = 0.67; and cas7, K07061 , PP = 0.90, K09002 , PP = 0.84, K19075 , PP = 0.97, K19115 , PP = 0.98, and K19140 , PP = 0.80). The absence of Cas1 and Cas2 may suggest LUCA encoded an early Cas system with the means to deliver an RNA-based immune response by cutting (Cas6/Cas3) and binding (CSM/Cas10) RNA, but lacking the full immune-system-site CRISPR. This supports the idea that the effector stage of CRISPR–Cas immunity evolved from RNA sensing for signal transduction, based on the similarities in RNA binding modules of the proteins 58 . This is consistent with the idea that cellular life was already involved in an arms race with viruses at the time of LUCA 59 , 60 . Our results indicate that an early Cas system was an ancestral immune system of extant cellular life.
Altogether, our metabolic reconstructions suggest that LUCA was a relatively complex organism, similar to extant Archaea and Bacteria 6 , 7 . On the basis of ancient duplications of the Sec and ATP synthase genes before LUCA, along with high PPs for key components of those systems, membrane-bound ATP synthase subunits, genes involved in peptidoglycan synthesis ( mraY , K01000 ; murC , K01924 ) and the cytoskeletal actin-like protein, MreB ( K03569 ) (Supplementary Data 3 ), it is highly likely that LUCA possessed the core cellular apparatus of modern prokaryotic life. This might include the basic constituents of a phospholipid membrane, although our analysis did not conclusively establish its composition. In particular, we recovered the following enzymes involved in the synthesis of ether and ester lipids, (alkyldihydroxyacetonephosphate synthase, glycerol 3-phosphate and glycerol 1-phosphate) and components of the mevalonate pathway (mevalonate 5-phosphate dehydratase (PP = 0.84), hydroxymethylglutaryl-CoA reductase (PP = 0.52), mevalonate kinase (PP = 0.51) and hydroxymethylglutaryl-CoA synthase (PP = 0.51)).
Compared with previous estimates of LUCA’s gene content, we find 81 overlapping COG gene families with the consensus dataset of ref. 7 and 69 overlapping KOs with the dataset of ref. 6 . Key points of agreement between previous studies include the presence of signal recognition particle protein, ffh (COG0541, K03106 ) 7 used in the targeting and delivery of proteins for the plasma membrane, a high number of aminoacyl-tRNA synthetases for amino acid synthesis and glycolysis/gluconeogenesis enzymes.
Ref. 6 inferred LUCA to be a thermophilic anaerobic autotroph using the WLP for carbon fixation based on the presence of a single enzyme (CODH), and similarly suggested that LUCA was capable of nitrogen fixation using a nitrogenase. Our reconstruction agrees with ref. 6 that LUCA was an anaerobic autotroph using the WLP for carbon fixation, but we infer the presence of a much more complete WLP than that previously obtained. We did not find strong evidence for nitrogenase or nitrogen fixation, and the reconstruction was not definitive with respect to the optimal growth environment of LUCA.
We used a probabilistic approach to reconstruct LUCA—that is, we estimated the probability with which each gene family was present in LUCA based on a model of how gene families evolve along an overarching species tree. This approach differs from analyses of phylogenetic presence–absence profiles 3 , 4 , 9 or those that used filtering criteria (such as broadly distributed or highly vertically evolving families) to define a high-confidence subset of modern genes that might have been present in LUCA. Our reconstruction maps many more genes to LUCA—albeit each with lower probability—than previous analyses 8 and yields an estimate of LUCA’s genome size that is within the range of modern prokaryotes. The result is an incomplete picture of a cellular organism that was prokaryote grade rather than progenotic 2 and that, similarly to prokaryotes today, probably existed as part of an ecosystem. As the common ancestor of sampled, extant prokaryotic life, LUCA is the oldest node on the species tree that we can reconstruct via phylogenomics but, as Fig. 3 illustrates, it was already the product of a highly innovative period in evolutionary history during which most of the core components of cells were established. By definition, we cannot reconstruct LUCA’s contemporaries using phylogenomics but we can propose hypotheses about their physiologies based on the reconstructed LUCA whose features immediately suggest the potential for interactions with other prokaryotic metabolisms.
LUCA’s environment, ecosystem and Earth system context
The inference that LUCA used the WLP helps constrain the environment and ecology in which it could have lived. Modern acetogens can grow autotrophically on H 2 (and CO 2 ) or heterotrophically on a wide range of alternative electron donors including alcohols, sugars and carboxylic acids 55 . This metabolic flexibility is key to their modern ecological success. Acetogenesis, whether autotrophic or heterotrophic, has a low energy yield and growth efficiency (although use of the reductive acetyl-CoA pathway for both energy production and biosynthesis reduces the energy cost of biosynthesis). This would be consistent with an energy-limited early biosphere 61 .
If LUCA functioned as an organoheterotrophic acetogen, it was necessarily part of an ecosystem containing autotrophs providing a source of organic compounds (because the abiotic source flux of organic molecules was minimal on the early Earth). Alternatively, if LUCA functioned as a chemoautotrophic acetogen it could (in principle) have lived independently off an abiotic source of H 2 (and CO 2 ). However, it is implausible that LUCA would have existed in isolation as the by-products of its chemoautotrophic metabolism would have created a niche for a consortium of other metabolisms (as in modern sediments) (Fig. 3d ). This would include the potential for LUCA itself to grow as an organoheterotroph.
A chemoautotrophic acetogenic LUCA could have occupied two major potential habitats (Fig. 3e ): the first is the deep ocean where hydrothermal vents and serpentinization of sea-floor provided a source of H 2 (ref. 62 ). Consistent with this, we find support for the presence of reverse gyrase (PP = 0.97), a hallmark enzyme of hyperthermophilic prokaryotes 6 , 63 , 64 , 65 , which would not be expected if early life existed at the ocean surface (although the evolution of reverse gyrase is complex 63 ; see ‘Reverse gyrase’ in Supplementary Information ). The second habitat is the ocean surface where the atmosphere would have provided a source of H 2 derived from volcanoes and metamorphism. Indeed, we detected the presence of spore photoproduct lyase (COG1533, K03716 , PP = 0.88) that in extant organisms repairs methylene-bridged thymine dimers occurring in spore DNA as a result of damage induced through ultraviolet (UV) radiation 66 , 67 . However, this gene family also occurs in modern taxa that neither form endospores nor dwell in environments where they are likely to accrue UV damage to their DNA and so is not an exclusive hallmark of environments exposed to UV. Previous studies often favoured a deep-ocean environment for LUCA as early life would have been better protected there from an episode of LHB. However, if the LHB was less intense than initially proposed 20 , 22 , or just a sampling artefact 21 , these arguments weaken. Another possibility may be that LUCA inhabited a shallow hydrothermal vent or a hot spring.
Hydrogen fluxes in these ecosystems could have been several times higher on the early Earth (with its greater internal heat source) than today. Volcanism today produces ~1 × 10 12 mol H 2 yr −1 and serpentinization produces ~0.4 × 10 12 mol H 2 yr − 1 . With the present H 2 flux and the known scaling of the H 2 escape rate to space, an abiotic atmospheric concentration of H 2 of ~150 ppmv is predicted 68 . Chemoautotrophic acetogens would have locally drawn down the concentration of H 2 (in either surface or deep niche) but their low growth efficiency would ensure H 2 (and CO 2 ) remained available. This and the organic matter and acetate produced would have created niches for other metabolisms, including methanogenesis (Fig. 3d ).
On the basis of thermodynamic considerations, CH 4 and CO 2 are expected to be the eventual metabolic end products of the resulting ecosystem, with a small fraction of the initial hydrogen consumption buried as organic matter. The resulting flux of CH 4 to the atmosphere would fuel photochemical H 2 regeneration and associated productivity in the surface ocean (Fig. 3e ). Existing models suggest the resulting global H 2 recycling system is highly effective, such that the supply flux of H 2 to the surface could have exceeded the volcanic input of H 2 to the atmosphere by at least an order of magnitude, in turn implying that the productivity of such a biosphere was boosted by a comparable factor 69 . Photochemical recycling to CO would also have supported a surface niche for organisms consuming CO (ref. 69 ).
In deep-ocean habitats, there could be some localized recycling of electrons (Fig. 3d ) but a quantitative loss of highly insoluble H 2 and CH 4 to the atmosphere and minimal return after photochemical conversion of CH 4 to H 2 means global recycling to depth would be minimal (Fig. 3e ). Hence the surface environment for LUCA could have become dominant (albeit recycling of the resulting organic matter could be spread through ocean depth; ‘Deep heterotrophic ecosystem’ in Fig. 3e ). The global net primary productivity of an early chemoautotrophic biosphere including acetogenic LUCA and methanogens could have been of order ~1 × 10 12 to 7 × 10 12 mol C yr − 1 (~3 orders of magnitude less than today) 69 .
The nutrient supply (for example, N) required to support such a biosphere would need to balance that lost in the burial flux of organic matter. Earth surface redox balance dictates that hydrogen loss to space and burial of electrons/hydrogen must together balance input of electrons/hydrogen. Considering contemporary H 2 inputs, and the above estimate of net primary productivity, this suggests a maximum burial flux in the order of ~10 12 mol C yr − 1 , which, with contemporary stoichiometry (C:N ratio of ~7) could demand >10 11 mol N yr − 1 . Lightning would have provided a source of nitrite and nitrate 70 , consistent with LUCA’s inferred pathways of nitrite and (possibly) nitrate reduction. However, it would only have been of the order 3 × 10 9 mol N yr − 1 (ref. 71 ). Instead, in a global hydrogen-recycling system, HCN from photochemistry higher in the atmosphere, deposited and hydrolysed to ammonia in water, would have increased available nitrogen supply by orders of magnitude toward ~3 × 10 12 mol N yr − 1 (refs. 71 , 72 ). This HCN pathway is consistent with the anomalously light nitrogen isotopic composition of the earliest plausible biogenic matter of 3.8–3.7 Ga (ref. 73 ), although that considerably postdates our inferred age of LUCA. These considerations suggest that the proposed LUCA biosphere (Fig. 3e ) would have been energy or hydrogen limited not nitrogen limited.
Conclusions
By treating gene presence probabilistically, our reconstruction maps many more genes (2,657) to LUCA than previous analyses and results in an estimate of LUCA’s genome size (2.75 Mb) that is within the range of modern prokaryotes. The result is a picture of a cellular organism that was prokaryote grade rather than progenotic 2 and that probably existed as a component of an ecosystem, using the WLP for acetogenic growth and carbon fixation. We cannot use phylogenetics to reconstruct other members of this early ecosystem but we can infer their physiologies based on the metabolic inputs and outputs of LUCA. How evolution proceeded from the origin of life to early communities at the time of LUCA remains an open question, but the inferred age of LUCA (~4.2 Ga) compared with the origin of the Earth and Moon suggests that the process required a surprisingly short interval of geologic time.
Universal marker genes
A list of 298 markers were identified by creating a non-redundant list of markers used in previous studies on archaeal and bacterial phylogenies 10 , 35 , 38 , 74 , 75 , 76 , 77 , 78 , 79 . These markers were mapped to the corresponding COG, arCOG and TIGRFAM profile to identify which profile is best suited to extract proteins from taxa of interest. To evaluate whether the markers cover all archaeal and bacterial diversity, proteins from a set of 574 archaeal and 3,020 bacterial genomes were searched against the COG, arCOG and TIGRFAM databases using hmmsearch (v.3.1b2; settings, hmmsearch–tblout output–domtblout–notextw) 52 , 80 , 81 , 82 . Only hits with an e-value less than or equal to 1 × 10 −5 were investigated further and for each protein the best hit was determined based on the e-value (expect value) and bit-score. Results from all database searches were merged based on the protein identifiers and the table was subsetted to only include hits against the 298 markers of interest. On the basis of this table we calculated whether the markers occurred in Archaea, Bacteria or both Archaea and Bacteria. Markers were only included if they were present in at least 50% of taxa and contained less than 10% of duplications, leaving a set of 265 markers. Sequences for each marker were aligned using MAFFT L-INS-i v.7.407 (ref. 83 ) for markers with less than 1,000 sequences or MAFFT 84 for those with more than 1,000 sequences (setting, –reorder) 84 and sequences were trimmed using BMGE 85 , set for amino acids, a BLOcks SUbstitution Matrix 30 similarity matrix, with a entropy score of 0.5 (v.1.12; settings, -t AA -m BLOSUM30 -h 0.5). Single gene trees were generated with IQ-TREE 2 (ref. 86 ), using the LG substitution matrix, with ten-profile mixture models, four CPUs, with 1,000 ultrafast bootstraps optimized by nearest neighbour interchange written to a file retaining branch lengths (v.2.1.2; settings, -m LG + C10 + F + R -nt 4 -wbtl -bb 1,000 -bnni). These single gene trees were investigated for archaeal and bacterial monophyly and the presence of paralogues. Markers that failed these tests were not included in further analyses, leaving a set of 59 markers (3 arCOGs, 46 COGs and 10 TIGRFAMs) suited for phylogenies containing both Archaea and Bacteria (Supplementary Data 4 ).
Marker gene sequence selection
To limit selecting distant paralogues and false positives, we used a bidirectional or reciprocal approach to identify the sequences corresponding to the 59 single-copy markers. In the first inspection (query 1), the 350 archaeal and 350 bacterial reference genomes were queried against all arCOG HMM (hidden Markov model) profiles (All_Arcogs_2018.hmm), all COG HMM profiles (NCBI_COGs_Oct2020.hmm) and all TIGRFAM HMM profiles (TIGRFAMs_15.0_HMM.LIB) using a custom script built on hmmsearch: hmmsearchTable <genomes.faa> <database.hmm> -E 1 × 10 −5 >HMMscan_Output_e5 (HMMER v.3.3.2) 87 . HMM profiles corresponding to the 59 single-copy marker genes (Supplementary Data 4 ) were extracted from each query and the best-hit sequences were identified based on the e-value and bit-score. We used the same custom hmmsearchTable script and conditions (see above) in the second inspection (query 2) to query the best-hit sequences identified above against the full COG HMM database (NCBI_COGs_Oct2020.hmm). Results were parsed and the COG family assigned in query 2 was compared with the COG family assigned to sequences based on the marker gene identity (Supplementary Data 4 ). Sequence hits were validated using the matching COG identifier, resulting in 353 mismatches (that is, COG family in query 1 does not match COG family in query 2) that were removed from the working set of marker gene sequences. These sequences were aligned using MAFFT L-INS-i 83 and then trimmed using BMGE 85 with a BLOSUM30 matrix. Individual gene trees were inferred under ML using IQ-TREE 2 (ref. 86 ) with model fitting, including both the default homologous substitution models and the following complex heterogeneous substitution models (LG substitution matrices with 10–60-profile mixture models, with empirical base frequencies and a discrete gamma model with four categories accounting for rate heterogeneity across sites): LG + C60 + F + G, LG + C50 + F + G, LG + C40 + F + G, LG + C30 + F + G, LG + C20 + F + G and LG + C10 + F + G, with 10,000 ultrafast bootstraps and 10 independent runs to avoid local optima. These 59 gene trees were manually inspected and curated over multiple rounds. Any horizontal gene transfer events, paralogous genes or sequences that violated domain monophyly were removed and two genes (arCOG01561, tuf ; COG0442, ProS ) were dropped at this stage due to the high number of transfer events, resulting in 57 single-copy orthologues for further tree inference.
Species-tree inference
These 57 orthologous sequences were concatenated and ML trees were inferred after three independent runs with IQ-TREE 2 (ref. 86 ) using the same model fitting and bootstrap settings as described above. The tree with the highest log-likelihood of the three runs was chosen as the ML species tree (topology 1). To test the effect of removing the CPR bacteria, we removed all CPR bacteria from the alignment before inferring a species tree (same parameters as above). We also performed approximately unbiased 44 tree topology tests (with IQ-TREE 2 (ref. 86 ), using LG + C20 + F + G) when testing the significance of constraining the species-tree topology (ML tree; Supplementary Fig. 1 ) to have a DPANN clade as sister to all other Archaea (same parameters as above but with a minimally constrained topology with monophyletic Archaea and DPANN sister to other Archaea present in a polytomy (Supplementary Fig. 2 )) and testing a constraint of CPR to be sister to Chloroflexi (Supplementary Fig. 3 ), and a combination of both the DPANN and CPR constraints (topology 2); these were tested against the ML topology, both using the normal 20 amino acid alignments and also with Susko–Roger recoding 88 .
Gene families
For the 700 representative species 15 , gene family clustering was performed using EGGNOGMAPPER v.2 (ref. 89 ), with the following parameters: using the DIAMOND 90 search, a query cover of 50% and an e-value threshold of 0.0000001. Gene families were collated using their KEGG 47 identifier, resulting in 9,365 gene families. These gene families were then aligned using MAFFT 84 v.7.5 with default settings and trimmed using BMGE 85 (with the same settings as above). Five independent sets of ML trees were then inferred using IQ-TREE 2 (ref. 86 ), using LG + F + G, with 1,000 ultrafast bootstrap replicates. We also performed a COG-based clustering analysis in which COGs were assigned based on the modal COG identifier annotated for each KEGG gene family based on the results from EGGNOGMAPPER v.2 (ref. 89 ). These gene families were aligned, trimmed and one set of gene trees (with 1,000 ultrafast bootstrap replicates) was inferred using the same parameters as described above for the KEGG gene families.
Reconciliations
The five sets of bootstrap distributions were converted into ALE files, using ALEobserve, and reconciled against topology 1 and topology 2 using ALEml_undated 91 with the fraction missing for each genome included (where available). Gene family root origination rates were optimized for each COG functional category as previously described 35 and families were categorized into four different groups based on the probability of being present in the LUCA node in the tree. The most-stringent category was that with sampling above 1% in both domains and a PP ≥ 0.75, another category was with PP ≥ 0.75 with no sampling requirement, another with PP ≥ 0.5 with the sampling requirement; the least stringent was PP ≥ 0.5 with no sampling requirement. We used the median probability at the root from across the five runs to avoid potential biases from failed runs in the mean and to account for variation across bootstrap distributions (see Supplementary Fig. 4 for distributions of the inferred ratio of duplications, transfers and losses for all gene families across all tips in the species tree; see Supplementary Data 5 for the inferred duplications, transfers and losses ratios for LUCA, the last bacterial common ancestor and the last archaeal common ancestor).
Metabolic pathway analysis
Metabolic pathways for gene families mapped to the LUCA node were inferred using the KEGG 47 website GUI and metabolic completeness for individual modules was estimated with Anvi’o 92 (anvi-estimate-metabolism), with pathwise completeness.
Additional testing
We tested for the effects of model complexity on reconciliation by using posterior mean site frequency LG + C20 + F + G across three independent runs in comparison with 3 LG + F + G independent runs. We also performed a 10% subsampling of the species trees and gene family alignments across two independent runs for two different subsamples, one with and one without the presence of Asgard archaea. We also tested the likelihood of the gene families under a bacterial root (between Terrabacteria and Gracilicutes) using reconciliations of the gene families under a species-tree topology rooted as such.
Fossil calibrations
On the basis of well-established geological events and the fossil record, we modelled 13 uniform densities to constrain the maximum and minimum ages of various nodes in our phylogeny. We constrained the bounds of the uniform densities to be either hard (no tail probability is allowed after the age constraint) or soft (a 2.5% tail probability is allowed after the age constraint) depending on the interpretation of the fossil record ( Supplementary Information ). Nodes that refer to the same duplication event are identified by MCMCtree as cross-braced (that is, one is chosen as the ‘driver’ node, the rest are ‘mirrored’ nodes). In other words, the sampling during the Markov chain Monte Carlo (MCMC) for cross-braced nodes is not independent: the same posterior time density is inferred for matching mirror–driver nodes (see ‘Additional methods’ for details on our cross-bracing approach).
Timetree inference analyses
Timetree inference with the program MCMCtree (PAML v.4.10.7 (ref. 93 )) proceeded under both the GBM and ILN relaxed-clock models. We specified a vague rate prior with the shape parameter equal to 2 and the scale parameter equal to 2.5: Γ(2, 2.5). This gamma distribution is meant to account for the uncertainty on our estimate for the mean evolutionary rate, ~0.81 substitutions per site per time unit, which we calculated by dividing the tree height of our best-scoring ML tree ( Supplementary Information ) into the estimated mean root age of our phylogeny (that is, 4.520 Ga, time unit = 10 9 years; see ‘Fossil calibrations’ in Supplementary Information for justifications on used calibrations). Given that we are estimating very deep divergences, the molecular clock may be seriously violated. Therefore, we applied a very diffuse gamma prior on the rate variation parameter ( σ 2 ), Γ(1, 10), so that it is centred around σ 2 = 0.1. To incorporate our uncertainty regarding the tree shape, we specified a uniform kernel density for the birth–death sampling process by setting the birth and death processes to 1, λ (per-lineage birth rate) = μ (per-lineage death rate) = 1, and the sampling frequency to ρ (sampling fraction) = 0.1. Our main analysis consisted of inferring the timetree for the partitioned dataset under both the GBM and the ILN relaxed-clock models in which nodes that correspond to the same divergences are cross-braced (that is, hereby referred to as cross-bracing A). In addition, we ran 10 additional inference analyses to benchmark the effect that partitioning, cross-bracing and relaxed-clock models can have on species divergence time estimation: (1) GBM + concatenated alignment + cross-bracing A, (2) GBM + concatenated alignment + cross-bracing B (only nodes that correspond to the same divergences for which there are fossil constraints are cross-braced), (3) GBM + concatenated alignment + without cross-bracing, (4) GBM + partitioned alignment + cross-bracing B, (5) GBM + partitioned alignment + without cross-bracing, (6) ILN + concatenated alignment + cross-bracing A, (7) ILN + concatenated alignment + cross-bracing B, (8) ILN + concatenated alignment + without cross-bracing, (9) ILN + partitioned alignment + cross-bracing B, and (10) ILN + partitioned alignment + without cross-bracing. Lastly, we used (1) individual gene alignments, (2) a leave-one-out strategy (rate prior changed for alignments without ATP and Leu , Γ(2, 2.2), and without Tyr , Γ(2, 2.3), but was Γ(2, 2.5) for the rest; see ‘Additional methods’), and (3) a more complex substitution model 94 to assess their impact on timetree inference. Refer to ‘Additional methods’ for details on how we parsed the dataset we used for timetree inference analyses, ran PAML programs CODEML and MCMCtree to approximate the likelihood calculation 95 , and carried out the MCMC diagnostics for the results obtained under each of the previously mentioned scenarios.
We simulated 100 samples of ‘KEGG genomes’ based on the probabilities of each of the (7,467) gene families being present in LUCA using the random.rand function in numpy 96 . The mean number of KEGG gene families was 1,298.25, the 95% HPD (highest posterior density) minimum was 1,255 and the maximum was 1,340. To infer the relationship between the number of KEGG KO gene families encoded by a genome, the number of proteins and the genome size, we used LOESS (locally estimated scatter-plot smoothing) regression to estimate the relationship between the number of KOs and (1) the number of protein-coding genes and (2) the genome size for the 700 prokaryotic genomes used in the LUCA reconstruction. To ensure that our inference of genome size is robust to uncertainty in the number of paralogues that can be expected to have been present in LUCA, we used the presence of probability for each of these KEGG KO gene families rather than the estimated copy number. We used the predict function to estimate the protein-coding genes and genome size of LUCA using these models and the simulated gene contents encoded with 95% confidence intervals.
Additional methods
Cross-bracing approach implemented in mcmctree.
The PAML program MCMCtree was implemented to allow for the analysis of duplicated genes or proteins so that some nodes in the tree corresponding to the same speciation events in different paralogues share the same age. We used the tree topology depicted in Supplementary Fig. 5 to explain how users can label driver or mirror nodes (more on these terms below) so that the program identifies them as sharing the same speciation events. The tree topology shown in Supplementary Fig. 5 can be written in Newick format as:
(((A1,A2),A3),((B1,B2),B3));
In this example, A and B are paralogues and the corresponding tips labelled as A1–A3 and B1–B3 represent different species. Node r represents a duplication event, whereas other nodes are speciation events. If we want to constrain the same speciation events to have the same age (that is, Supplementary Fig. 5 , see labels a and b (that is, A1–A2 ancestor and B1–B2 ancestor, respectively) and labels v and b (that is, A1–A2–A3 ancestor and B1–B2–B3 ancestor, respectively), we use node labels in the format #1, #2, and so on to identify such nodes:
(((A1, A2) #1, A3) #2, ((B1, B2) [#1 B{0.2, 0.4}], B3) #2) 'B(0.9,1.1)';
Node a and node b are assigned the same label (#1) and so they share the same age ( t ): t a = t b . Similarly, node u and node v have the same age: t u = t v . The former nodes are further constrained by a soft-bound calibration based on the fossil record or geological evidence: 0.2 < t a = t b < 0.4. The latter, however, does not have fossil constraints and thus the only restriction imposed is that both t u and t v are equal. Finally, there is another soft-bound calibration on the root age: 0.9 < t r < 1.1.
Among the nodes on the tree with the same label (for example, those nodes labelled with #1 and those with #2 in our example), one is chosen as the driver node, whereas the others are mirror nodes. If calibration information is provided on one of the shared nodes (for example, nodes a and b in Supplementary Fig. 5 ), the same information therefore applies to all shared nodes. If calibration information is provided on multiple shared nodes, that information has to be the same (for example, you could not constrain node a with a different calibration used to constrain node b in Supplementary Fig. 5 ). The time prior (or the prior on all node ages on the tree) is constructed by using a density at the root of the tree, which is specified by the user (for example, 'B(0.9,1.1)' in our example, which has a minimum of 0.9 and a maximum of 1.1). The ages of all non-calibrated nodes are given by the uniform density. This time prior is similar to that used by ref. 29 . The parameters in the birth–death sampling process ( λ , μ , ρ ; specified using the option BDparas in the control file that executes MCMCtree) are ignored. It is noteworthy that more than two nodes can have the same label but one node cannot have two or more labels. In addition, the prior on rates does not distinguish between speciation and duplication events. The implemented cross-bracing approach can only be enabled if option duplication = 1 is included in the control file. By default, this option is set to 0 and users are not required to include it in the control file (that is, the default option is duplication = 0 ).
Timetree inference
Data parsing.
Eight paralogues were initially selected based on previous work showing a likely duplication event before LUCA: the amino- and carboxy-terminal regions from carbamoyl phosphate synthetase, aspartate and ornithine transcarbamoylases, histidine biosynthesis genes A and F , catalytic and non-catalytic subunits from ATP synthase ( ATP ), elongation factor Tu and G ( EF ), signal recognition protein and signal recognition particle receptor ( SRP ), tyrosyl-tRNA and tryptophanyl-tRNA synthetases ( Tyr ), and leucyl- and valyl-tRNA synthetases ( Leu ) 27 . Gene families were identified using BLASTp 97 . Sequences were downloaded from NCBI 98 , aligned with MUSCLE 99 and trimmed with TrimAl 100 (-strict). Individual gene trees were inferred under the LG + C20 + F + G substitution model implemented in IQ-TREE 2 (ref. 86 ). These trees were manually inspected and curated to remove non-homologous sequences, horizontal gene transfers, exceptionally short or long sequences and extremely long branches. Recent paralogues or taxa of inconsistent and/or uncertain placement inferred with RogueNaRok 101 were also removed. Independent verification of an archaeal or bacterial deep split was achieved using minimal ancestor deviation 102 . This filtering process resulted in the five pairs of paralogous gene families 27 ( ATP , EF , SRP , Tyr and Leu ) that we used to estimate the origination time of LUCA. The alignment used for timetree inference consisted of 246 species, with the majority of taxa having at least two copies (for some eukaryotes, they may be represented by plastid, mitochondrial and nuclear sequences).
To assess the impact that partitioning can have on divergence time estimates, we ran our inference analyses with both a concatenated and a partitioned alignment (that is, gene partitioning scheme). We used PAML v.4.10.7 (programs CODEML and MCMCtree) for all divergence time estimation analyses. Given that a fixed tree topology is required for timetree inference with MCMCtree, we inferred the best-scoring ML tree with IQ-TREE 2 under the LG + C20 + F + G4 (ref. 103 ) model following our previous phylogenetic analyses. We then modified the resulting inferred tree topology following consensus views of species-level relationships 34 , 35 , 104 , which we calibrated with the available fossil calibrations (see below). In addition, we ran three sensitivity tests: timetree inference (1) with each gene alignment separately, (2) under a leave-one-out strategy in which each gene alignment was iteratively removed from the concatenated dataset (for example, remove gene ATP but keep genes EF , Leu , SRP and Tyr concatenated in a unique alignment block; apply the same procedure for each gene family), and (3) using the vector of branch lengths, the gradient vector and the Hessian matrix estimated under a complex substitution model (bsinBV method described in ref. 94 ) with the concatenated dataset used for our core analyses. Four of the gene alignments generated for the leave-one-out strategy had gap-only sequences, these were removed when re-inferring the branch lengths under the LG + C20 + F + G4 model (that is, without ATP , 241 species; without EF , 236 species; without Leu , 243 species; without Tyr , 244 species). We used these trees to set the rate prior used for timetree inference for those alignments not including ATP , EF , Leu or Tyr , respectively. The β value (scale parameter) for the rate prior used when analysing alignments without ATP , Leu and Tyr changed minimally but we updated the corresponding rate priors accordingly (see above). When not including SRP , the alignment did not have any sequences removed (that is, 246 species). All alignments were analysed with the same rate prior, Γ(2, 2.5), except for the three previously mentioned alignments.
Approximating the likelihood calculation during timetree inference using PAML programs
Before timetree inference, we ran the CODEML program to infer the branch lengths of the fixed tree topology, the gradient (first derivative of the likelihood function) and the Hessian matrix (second derivative of the likelihood function); the vectors and matrix are required to approximate the likelihood function in the dating program MCMCtree 95 , an approach that substantially reduces computational time 105 . Given that CODEML does not implement the CAT (Bayesian mixture model for across-site heterogeneity) model, we ran our analyses under the closest available substitution model: LG + F + G4 (model = 3). We calculated the aforementioned vectors and matrix for each of the five gene alignments (that is, required for the partitioned alignment), for the concatenated alignment and for the concatenated alignments used for the leave-one-out strategy; the resulting values are written out in an output file called rst2. We appended the rst2 files generated for each of the five individual alignments in the same order the alignment blocks appear in the partitioned alignment file (for example, the first alignment block corresponds to the ATP gene alignment, and thus the first rst2 block will be the one generated when analysing the ATP gene alignment with CODEML). We named this file in_5parts.BV. There is only one rst2 output file for the concatenated alignments, which we renamed in.BV (main concatenated alignment and concatenated alignments under leave-one-out strategy). When analysing each gene alignment separately, we renamed the rst2 files generated for each gene alignment as in.BV.
MCMC diagnostics
All the chains that we ran with MCMCtree for each type of analysis underwent a protocol of MCMC diagnostics consisting of the following steps: (1) flagging and removal of problematic chains; (2) generating convergence plots before and after chain filtering; (3) using the samples collected by those chains that passed the filters (that is, assumed to have converged to the same target distribution) to summarize the results; (4) assessing chain efficiency and convergence by calculating statistics such as R-hat, tail-ESS and bulk-ESS (in-house wrapper function calling Rstan functions, Rstan v.2.21.7; https://mc-stan.org/rstan/ ); and (5) generating the timetrees for each type of analysis with confidence intervals and high-posterior densities to show the uncertainty surrounding the estimated divergence times. Tail-ESS is a diagnostic tool that we used to assess the sampling efficiency in the tails of the posterior distributions of all estimated divergence times, which corresponds to the minimum of the effective sample sizes for quantiles 2.5% and 97.5%. To assess the sampling efficiency in the bulk of the posterior distributions of all estimated divergence, we used bulk-ESS, which uses rank-normalized draws. Note that if tail-ESS and bulk-ESS values are larger than 100, the chains are assumed to have been efficient and reliable parameter estimates (that is, divergence times in our case). R-hat is a convergence diagnostic measure that we used to compare between- and within-chain divergence time estimates to assess chain mixing. If R-hat values are larger than 1.05, between- and within-chain estimates do not agree and thus mixing has been poor. Lastly, we assessed the impact that truncation may have on the estimated divergence times by running MCMCtree when sampling from the prior (that is, the same settings specified above but without using sequence data, which set the prior distribution to be the target distribution during the MCMC). To summarize the samples collected during this analysis, we carried out the same MCMC diagnostics procedure previously mentioned. Supplementary Fig. 6 shows our calibration densities (commonly referred to as user-specified priors, see justifications for used calibrations above) versus the marginal densities (also known as effective priors) that MCMCtree infers when building the joint prior (that is, a prior built without sequence data that considers age constraints specified by the user, the birth–death with sampling process to infer the time densities for the uncalibrated nodes, the rate priors, and so on). We provide all our results for these quality-control checks in our GitHub repository ( https://github.com/sabifo4/LUCA-divtimes ) and in Extended Data Fig. 1 , Supplementary Figs. 7 – 10 and Supplementary Data 6 . Data, figures and tables used and/or generated following a step-by-step tutorial are detailed in the GitHub repository for each inference analysis.
Additional sensitivity analyses
We compared the divergence times we estimated with the concatenated dataset under the calibration strategy cross-bracing A with those inferred (1) for each gene, (2) for gene alignments analysed under a leave-one-out strategy, and (3) for the main concatenated dataset but when using the vector of branch lengths, the gradient vector and the Hessian matrix estimated under a more complex substitution model 94 . The results are summarized in Extended Data Fig. 2 and Supplementary Data 7 and 8 . The same pattern regarding the calibration densities and marginal densities when the tree topology was pruned (that is, see above for details on the leave-one-out strategy) was observed, and thus no additional figures have been generated. As for our main analyses, the results for these additional sensitivity analyses can be found on our GitHub repository ( https://github.com/sabifo4/LUCA-divtimes ).
Reporting summary
Further information on research design is available in the Nature Portfolio Reporting Summary linked to this article.
Data availability
All data required to interpret, verify and extend the research in this article can be found at our figshare repository at https://doi.org/10.6084/m9.figshare.24428659 (ref. 106 ) for the reconciliation and phylogenomic analyses and GitHub at https://github.com/sabifo4/LUCA-divtimes (ref. 107 ) for the molecular clock analyses. Additional data are available at the University of Bristol data repository, data.bris, at https://doi.org/10.5523/bris.405xnm7ei36d2cj65nrirg3ip (ref. 108 ).
Code availability
All code relating to the dating analysis can be found on GitHub at https://github.com/sabifo4/LUCA-divtimes (ref. 107 ), and other custom scripts can be found in our figshare repository at https://doi.org/10.6084/m9.figshare.24428659 (ref. 106 ).
Theobald, D. L. A formal test of the theory of universal common ancestry. Nature 465 , 219–222 (2010).
Article CAS PubMed Google Scholar
Woese, C. R. & Fox, G. E. The concept of cellular evolution. J. Mol. Evol. 10 , 1–6 (1977).
Mirkin, B. G., Fenner, T. I., Galperin, M. Y. & Koonin, E. V. Algorithms for computing parsimonious evolutionary scenarios for genome evolution, the last universal common ancestor and dominance of horizontal gene transfer in the evolution of prokaryotes. BMC Evol. Biol. 3 , 2 (2003).
Article PubMed PubMed Central Google Scholar
Ouzounis, C. A., Kunin, V., Darzentas, N. & Goldovsky, L. A minimal estimate for the gene content of the last universal common ancestor—exobiology from a terrestrial perspective. Res. Microbiol. 157 , 57–68 (2006).
Gogarten, J. P. & Deamer, D. Is LUCA a thermophilic progenote? Nat. Microbiol 1 , 16229 (2016).
Weiss, M. C. et al. The physiology and habitat of the last universal common ancestor. Nat. Microbiol 1 , 16116 (2016).
Crapitto, A. J., Campbell, A., Harris, A. J. & Goldman, A. D. A consensus view of the proteome of the last universal common ancestor. Ecol. Evol. 12 , e8930 (2022).
Kyrpides, N., Overbeek, R. & Ouzounis, C. Universal protein families and the functional content of the last universal common ancestor. J. Mol. Evol. 49 , 413–423 (1999).
Koonin, E. V. Comparative genomics, minimal gene-sets and the last universal common ancestor. Nat. Rev. Microbiol. 1 , 127–136 (2003).
Harris, J. K., Kelley, S. T., Spiegelman, G. B. & Pace, N. R. The genetic core of the universal ancestor. Genome Res. 13 , 407–412 (2003).
Article CAS PubMed PubMed Central Google Scholar
Javaux, E. J. Challenges in evidencing the earliest traces of life. Nature 572 , 451–460 (2019).
Lepot, K. Signatures of early microbial life from the Archean (4 to 2.5 Ga) eon. Earth Sci. Rev. 209 , 103296 (2020).
Article CAS Google Scholar
Betts, H. C. et al. Integrated genomic and fossil evidence illuminates life’s early evolution and eukaryote origin. Nat. Ecol. Evol. 2 , 1556–1562 (2018).
Zhu, Q. et al. Phylogenomics of 10,575 genomes reveals evolutionary proximity between domains Bacteria and Archaea. Nat. Commun. 10 , 5477 (2019).
Moody, E. R. R. et al. An estimate of the deepest branches of the tree of life from ancient vertically evolving genes. eLife 11 , e66695 (2022).
Schwartz, R. M. & Dayhoff, M. O. Origins of prokaryotes, eukaryotes, mitochondria, and chloroplasts. Science 199 , 395–403 (1978).
Shih, P. M. & Matzke, N. J. Primary endosymbiosis events date to the later Proterozoic 994 with cross-calibrated phylogenetic dating of duplicated ATPase proteins. Proc. Natl Acad. Sci. USA 110 , 996 (2013).
Article Google Scholar
Mahendrarajah, T. A. et al. ATP synthase evolution on a cross-braced dated tree of life. Nat. Commun. 14 , 7456 (2023).
Bottke, W. F. & Norman, M. D. The Late Heavy Bombardment. Annu. Rev. Earth Planet. Sci. 45 , 619–647 (2017).
Reimink, J. et al. Quantifying the effect of late bombardment on terrestrial zircons. Earth Planet. Sci. Lett. 604 , 118007 (2023).
Boehnke, P. & Harrison, T. M. Illusory Late Heavy Bombardments. Proc. Natl Acad. Sci. USA 113 , 10802–10806 (2016).
Ryder, G. Mass flux in the ancient Earth–Moon system and benign implications for the origin of life on Earth. J. Geophys. Res. 107 , 6-1–6-13 (2002).
Google Scholar
Hartmann, W. K. History of the terminal cataclysm paradigm: epistemology of a planetary bombardment that never (?) happened. Geosciences 9 , 285 (2019).
Planavsky, N. J. et al. Evidence for oxygenic photosynthesis half a billion years before the great oxidation event. Nat. Geosci. 7 , 283–286 (2014).
Ossa, F. O. et al. Limited oxygen production in the Mesoarchean ocean. Proc. Natl Acad. Sci. USA 116 , 6647–6652 (2019).
Mukasa, S. B., Wilson, A. H. & Young, K. R. Geochronological constraints on the magmatic and tectonic development of the Pongola Supergroup (Central Region), South Africa. Precambrian Res. 224 , 268–286 (2013).
Zhaxybayeva, O., Lapierre, P. & Gogarten, J. P. Ancient gene duplications and the root(s) of the tree of life. Protoplasma 227 , 53–64 (2005).
Article PubMed Google Scholar
Donoghue, P. C. J. & Yang, Z. The evolution of methods for establishing evolutionary timescales. Philos. Trans. R. Soc. Lond. B Biol. Sci. 371 , 3006–3010 (2016).
Thorne, J. L., Kishino, H. & Painter, I. S. Estimating the rate of evolution of the rate of molecular evolution. Mol. Biol. Evol. 15 , 1647–1657 (1998).
Yang, Z. & Rannala, B. Bayesian estimation of species divergence times under a molecular clock using multiple fossil calibrations with soft bounds. Mol. Biol. Evol. 23 , 212–226 (2006).
Rannala, B. & Yang, Z. Inferring speciation times under an episodic molecular clock. Syst. Biol. 56 , 453–466 (2007).
Lemey, P., Rambaut, A., Welch, J. J. & Suchard, M. A. Phylogeography takes a relaxed random walk in continuous space and time. Mol. Biol. Evol. 27 , 1877–1885 (2010).
Craig, J. M., Kumar, S. & Hedges, S. B. The origin of eukaryotes and rise in complexity were synchronous with the rise in oxygen. Front. Bioinform. 3 , 1233281 (2023).
Aouad, M. et al. A divide-and-conquer phylogenomic approach based on character supermatrices resolves early steps in the evolution of the Archaea. BMC Ecol. Evol. 22 , 1 (2022).
Coleman, G. A. et al. A rooted phylogeny resolves early bacterial evolution. Science 372 , eabe0511 (2021).
Guy, L. & Ettema, T. J. G. The archaeal ‘TACK’ superphylum and the origin of eukaryotes. Trends Microbiol. 19 , 580–587 (2011).
Spang, A. et al. Complex Archaea that bridge the gap between prokaryotes and eukaryotes. Nature 521 , 173–179 (2015).
Zaremba-Niedzwiedzka, K. et al. Asgard Archaea illuminate the origin of eukaryotic cellular complexity. Nature 541 , 353–358 (2017).
Eme, L. et al. Inference and reconstruction of the heimdallarchaeial ancestry of eukaryotes. Nature 618 , 992–999 (2023).
Raymann, K., Brochier-Armanet, C. & Gribaldo, S. The two-domain tree of life is linked to a new root for the Archaea. Proc. Natl Acad. Sci. USA 112 , 6670–6675 (2015).
Megrian, D., Taib, N., Jaffe, A. L., Banfield, J. F. & Gribaldo, S. Ancient origin and constrained evolution of the division and cell wall gene cluster in Bacteria. Nat. Microbiol. 7 , 2114–2127 (2022).
Brown, C. T. et al. Unusual biology across a group comprising more than 15% of domain Bacteria. Nature 523 , 208–211 (2015).
Rinke, C. et al. Insights into the phylogeny and coding potential of microbial dark matter. Nature 499 , 431–437 (2013).
Shimodaira, H. An approximately unbiased test of phylogenetic tree selection. Syst. Biol. 51 , 492–508 (2002).
Taib, N. et al. Genome-wide analysis of the Firmicutes illuminates the diderm/monoderm transition. Nat. Ecol. Evol. 4 , 1661–1672 (2020).
Szöllõsi, G. J., Rosikiewicz, W., Boussau, B., Tannier, E. & Daubin, V. Efficient exploration of the space of reconciled gene trees. Syst. Biol. 62 , 901–912 (2013).
Kanehisa, M. & Goto, S. KEGG: kyoto encyclopedia of genes and genomes. Nucleic Acids Res. 28 , 27–30 (2000).
Williams, T. A. et al. Integrative modeling of gene and genome evolution roots the archaeal tree of life. Proc. Natl Acad. Sci. USA 114 , E4602–E4611 (2017).
Dharamshi, J. E. et al. Gene gain facilitated endosymbiotic evolution of Chlamydiae. Nat. Microbiol. 8 , 40–54 (2023).
Doolittle, W. F. Phylogenetic classification and the universal tree. Science 284 , 2124–2128 (1999).
Dagan, T. & Martin, W. The tree of one percent. Genome Biol. 7 , 118 (2006).
Tatusov, R. L. et al. The COG database: an updated version includes eukaryotes. BMC Bioinf. 4 , 41 (2003).
Ragsdale, S. W. & Pierce, E. Acetogenesis and the Wood–Ljungdahl pathway of CO 2 fixation. Biochim. Biophys. Acta 1784 , 1873–1898 (2008).
Schuchmann, K. & Müller, V. Autotrophy at the thermodynamic limit of life: a model for energy conservation in acetogenic bacteria. Nat. Rev. Microbiol. 12 , 809–821 (2014).
Schuchmann, K. & Müller, V. Energetics and application of heterotrophy in acetogenic bacteria. Appl. Environ. Microbiol. 82 , 4056–4069 (2016).
Iwabe, N., Kuma, K., Hasegawa, M., Osawa, S. & Miyata, T. Evolutionary relationship of archaebacteria, eubacteria, and eukaryotes inferred from phylogenetic trees of duplicated genes. Proc. Natl Acad. Sci. USA 86 , 9355–9359 (1989).
Gogarten, J. P. et al. Evolution of the vacuolar H + -ATPase: implications for the origin of eukaryotes. Proc. Natl Acad. Sci. USA 86 , 6661–6665 (1989).
Koonin, E. V. & Makarova, K. S. Origins and evolution of CRISPR–Cas systems. Phil. Trans. R. Soc. Lond. B Biol. Sci. 374 , 20180087 (2019).
Krupovic, M., Dolja, V. V. & Koonin, E. V. The LUCA and its complex virome. Nat. Rev. Microbiol. 18 , 661–670 (2020).
Koonin, E. V., Dolja, V. V. & Krupovic, M. The logic of virus evolution. Cell Host Microbe 30 , 917–929 (2022).
Lever, M. A. Acetogenesis in the energy-starved deep biosphere—a paradox? Front. Microbiol. 2 , 284 (2011).
PubMed Google Scholar
Martin, W. & Russell, M. J. On the origin of biochemistry at an alkaline hydrothermal vent. Phil. Trans. R. Soc. Lond. B Biol. Sci. 362 , 1887–1925 (2007).
Catchpole, R. J. & Forterre, P. The evolution of reverse gyrase suggests a nonhyperthermophilic last universal common ancestor. Mol. Biol. Evol. 36 , 2737–2747 (2019).
Groussin, M., Boussau, B., Charles, S., Blanquart, S. & Gouy, M. The molecular signal for the adaptation to cold temperature during early life on Earth. Biol. Lett. 9 , 20130608 (2013).
Boussau, B., Blanquart, S., Necsulea, A., Lartillot, N. & Gouy, M. Parallel adaptations to high temperatures in the Archaean eon. Nature 456 , 942–945 (2008).
Chandor, A. et al. Dinucleotide spore photoproduct, a minimal substrate of the DNA repair spore photoproduct lyase enzyme from Bacillus subtilis. J. Biol. Chem. 281 , 26922–26931 (2006).
Chandra, T. et al. Spore photoproduct lyase catalyzes specific repair of the 5R but not the 5S spore photoproduct. J. Am. Chem. Soc. 131 , 2420–2421 (2009).
Kasting, J. F. The evolution of the prebiotic atmosphere. Orig. Life 14 , 75–82 (1984).
Kharecha, P. A. A Coupled Atmosphere–Ecosystem Model of the Early Archean Biosphere . PhD thesis, Pennsylvania State Univ. (2005).
Barth, P. et al. Isotopic constraints on lightning as a source of fixed nitrogen in Earth’s early biosphere. Nat. Geosci. 16 , 478–484 (2023).
Tian, F., Kasting, J. F. & Zahnle, K. Revisiting HCN formation in Earth’s early atmosphere. Earth Planet. Sci. Lett. 308 , 417–423 (2011).
Zahnle, K. J. Photochemistry of methane and the formation of hydrocyanic acid (HCN) in the Earth’s early atmosphere. J. Geophys. Res. 91 , 2819–2834 (1986).
Stüeken, E. E., Boocock, T., Szilas, K., Mikhail, S. & Gardiner, N. J. Reconstructing nitrogen sources to Earth’s earliest biosphere at 3.7 Ga. Front. Earth Sci. 9 , 675726 (2021).
Ciccarelli, F. D. et al. Toward automatic reconstruction of a highly resolved tree of life. Science 311 , 1283–1287 (2006).
Yutin, N., Makarova, K. S., Mekhedov, S. L., Wolf, Y. I. & Koonin, E. V. The deep archaeal roots of eukaryotes. Mol. Biol. Evol. 25 , 1619–1630 (2008).
Petitjean, C., Deschamps, P., López-García, P. & Moreira, D. Rooting the domain Archaea by phylogenomic analysis supports the foundation of the new kingdom Proteoarchaeota. Genome Biol. Evol. 7 , 191–204 (2014).
Williams, T. A., Cox, C. J., Foster, P. G., Szöllősi, G. J. & Embley, T. M. Phylogenomics provides robust support for a two-domains tree of life. Nat. Ecol. Evol. 4 , 138–147 (2020).
Rinke, C. et al. A standardized archaeal taxonomy for the Genome Taxonomy Database. Nat. Microbiol. 6 , 946–959 (2021).
Parks, D. H. et al. Selection of representative genomes for 24,706 bacterial and archaeal species clusters provide a complete genome-based taxonomy. Preprint at bioRxiv https://doi.org/10.1101/771964 (2019).
Finn, R. D., Clements, J. & Eddy, S. R. HMMER web server: interactive sequence similarity searching. Nucleic Acids Res. 39 , W29–W37 (2011).
Makarova, K. S., Wolf, Y. I. & Koonin, E. V. Archaeal Clusters of Orthologous Genes (arCOGs): an update and application for analysis of shared features between Thermococcales, Methanococcales, and Methanobacteriales. Life 5 , 818–840 (2015).
Haft, D. H., Selengut, J. D. & White, O. The TIGRFAMs database of protein families. Nucleic Acids Res. 31 , 371–373 (2003).
Katoh, K., Kuma, K.-I., Toh, H. & Miyata, T. MAFFT version 5: improvement in accuracy of multiple sequence alignment. Nucleic Acids Res. 33 , 511–518 (2005).
Katoh, K., Misawa, K., Kuma, K.-I. & Miyata, T. MAFFT: a novel method for rapid multiple sequence alignment based on fast Fourier transform. Nucleic Acids Res. 30 , 3059–3066 (2002).
Criscuolo, A. & Gribaldo, S. BMGE (Block Mapping and Gathering with Entropy): a new software for selection of phylogenetic informative regions from multiple sequence alignments. BMC Evol. Biol. 10 , 210 (2010).
Minh, B. Q. et al. IQ-TREE 2: new models and efficient methods for phylogenetic inference in the genomic era. Mol. Biol. Evol. 37 , 1530–1534 (2020).
Eddy, S. R. Accelerated profile HMM searches. PLoS Comput. Biol. 7 , e1002195 (2011).
Susko, E. & Roger, A. J. On reduced amino acid alphabets for phylogenetic inference. Mol. Biol. Evol. 24 , 2139–2150 (2007).
Cantalapiedra, C. P., Hernández-Plaza, A., Letunic, I., Bork, P. & Huerta-Cepas, J. eggNOG-mapper v2: functional annotation, orthology assignments, and domain prediction at the metagenomic scale. Mol. Biol. Evol. 38 , 5825–5829 (2021).
Buchfink, B., Reuter, K. & Drost, H.-G. Sensitive protein alignments at tree-of-life scale using DIAMOND. Nat. Methods 18 , 366–368 (2021).
Szöllősi, G. J., Davín, A. A., Tannier, E., Daubin, V. & Boussau, B. Genome-scale phylogenetic analysis finds extensive gene transfer among fungi. Phil. Trans. R. Soc. Lond. B Biol. Sci. 370 , 20140335 (2015).
Eren, A. M. et al. Community-led, integrated, reproducible multi-omics with anvi’o. Nat. Microbiol. 6 , 3–6 (2021).
Yang, Z. PAML 4: phylogenetic analysis by maximum likelihood. Mol. Biol. Evol. 24 , 1586–1591 (2007).
Wang, S. & Luo, H. Dating the bacterial tree of life based on ancient symbiosis. Preprint at bioRxiv https://doi.org/10.1101/2023.06.18.545440 (2023).
dos Reis, M. & Yang, Z. Approximate likelihood calculation on a phylogeny for Bayesian estimation of divergence times. Mol. Biol. Evol. 28 , 2161–2172 (2011).
Harris et al. Array programming with NumPy. Nature 585 , 357–362 (2020).
Altschul, S. F., Gish, W., Miller, W., Myers, E. W. & Lipman, D. J. Basic local alignment search tool. J. Mol. Biol. 215 , 403–410 (1990).
Sayers, E. W. et al. Database resources of the National Center for Biotechnology Information. Nucleic Acids Res. 39 , D38–D51 (2011).
Edgar, R. C. MUSCLE: multiple sequence alignment with high accuracy and high throughput. Nucleic Acids Res. 32 , 1792–1797 (2004).
Capella-Gutiérrez, S., Silla-Martínez, J. M. & Gabaldón, T. trimAl: a tool for automated alignment trimming in large-scale phylogenetic analyses. Bioinformatics 25 , 1972–1973 (2009).
Aberer, A. J., Krompaß, D. & Stamatakis, A. RogueNaRok: An efficient and exact algorithm for rogue taxon identification. Exelixis-RRDR-2011–10 (Heidelberg Institute for Theoretical Studies, 2011).
Tria, F. D. K., Landan, G. & Dagan, T. Phylogenetic rooting using minimal ancestor deviation. Nat. Ecol. Evol. 1 , 193 (2017).
Hoang, D. T., Chernomor, O., von Haeseler, A., Minh, B. Q. & Vinh, L. S. UFBoot2: improving the ultrafast bootstrap approximation. Mol. Biol. Evol. 35 , 518–522 (2018).
Burki, F., Roger, A. J., Brown, M. W. & Simpson, A. G. B. The new tree of eukaryotes. Trends Ecol. Evol. 35 , 43–55 (2020).
Battistuzzi, F. U., Billing-Ross, P., Paliwal, A. & Kumar, S. Fast and slow implementations of relaxed-clock methods show similar patterns of accuracy in estimating divergence times. Mol. Biol. Evol. 28 , 2439–2442 (2011).
Moody, E. R. R. The nature of the last universal common ancestor and its impact on the early Earth system. figshare https://doi.org/10.6084/m9.figshare.24428659 (2024).
Álvarez-Carretero, S. The nature of the last universal common ancestor and its impact on the early Earth system—timetree inference analyses. Zenodo https://doi.org/10.5281/zenodo.11260523 (2024).
Moody, E. R. R. et al. The nature of the Last Universal Common Ancestor and its impact on the early Earth system. Nat. Ecol. Evol. https://doi.org/10.5523/bris.405xnm7ei36d2cj65nrirg3ip (2024).
Darzi, Y., Letunic, I., Bork, P. & Yamada, T. iPath3.0: interactive pathways explorer v3. Nucleic Acids Res. 46 , W510–W513 (2018).
Download references
Acknowledgements
Our research is funded by the John Templeton Foundation (62220 to P.C.J.D., N.L., T.M.L., D.P., G.A.S., T.A.W. and Z.Y.; the opinions expressed in this publication are those of the authors and do not necessarily reflect the views of the John Templeton Foundation), Biotechnology and Biological Sciences Research Council (BB/T012773/1 to P.C.J.D. and Z.Y.; BB/T012951/1 to Z.Y.), by the European Research Council under the European Union’s Horizon 2020 research and innovation programme (947317 ASymbEL to A.S.; 714774, GENECLOCKS to G.J.S.), Leverhulme Trust (RF-2022-167 to P.C.J.D.), Gordon and Betty Moore Foundation (GBMF9741 to T.A.W., D.P., P.C.J.D., A.S. and G.J.S.; GBMF9346 to A.S.), Royal Society (University Research Fellowship (URF) to T.A.W.), the Simons Foundation (735929LPI to A.S.) and the University of Bristol (University Research Fellowship (URF) to D.P.).
Author information
Authors and affiliations.
Bristol Palaeobiology Group, School of Earth Sciences, University of Bristol, Bristol, UK
Edmund R. R. Moody, Sandra Álvarez-Carretero, Holly C. Betts, Davide Pisani & Philip C. J. Donoghue
Department of Marine Microbiology and Biogeochemistry, NIOZ, Royal Netherlands Institute for Sea Research, Den Burg, The Netherlands
Tara A. Mahendrarajah, Nina Dombrowski & Anja Spang
Milner Centre for Evolution, Department of Life Sciences, University of Bath, Bath, UK
James W. Clark
Department of Biological Physics, Eötvös University, Budapest, Hungary
Lénárd L. Szánthó
MTA-ELTE ‘Lendulet’ Evolutionary Genomics Research Group, Budapest, Hungary
Lénárd L. Szánthó & Gergely J. Szöllősi
Institute of Evolution, HUN-REN Center for Ecological Research, Budapest, Hungary
Global Systems Institute, University of Exeter, Exeter, UK
Richard A. Boyle, Stuart Daines & Timothy M. Lenton
Department of Earth Sciences, University College London, London, UK
Xi Chen & Graham A. Shields
Department of Genetics, Evolution and Environment, University College London, London, UK
Nick Lane & Ziheng Yang
Model-Based Evolutionary Genomics Unit, Okinawa Institute of Science and Technology Graduate University, Okinawa, Japan
Gergely J. Szöllősi
Department of Evolutionary & Population Biology, Institute for Biodiversity and Ecosystem Dynamics (IBED), University of Amsterdam, Amsterdam, The Netherlands
Bristol Palaeobiology Group, School of Biological Sciences, University of Bristol, Bristol, UK
Davide Pisani & Tom A. Williams
You can also search for this author in PubMed Google Scholar
Contributions
The project was conceived and designed by P.C.J.D., T.M.L., D.P., G.J.S., A.S. and T.A.W. Dating analyses were performed by H.C.B., J.W.C., S.Á.-C., P.J.C.D. and E.R.R.M. T.A.M., N.D. and E.R.R.M. performed single-copy orthologue analysis for species-tree inference. L.L.S., G.J.S., T.A.W. and E.R.R.M. performed reconciliation analysis. E.R.R.M. performed homologous gene family annotation, sequence, alignment, gene tree inference and sensitivity tests. E.R.R.M., A.S. and T.A.W. performed metabolic analysis and interpretation. T.M.L., S.D. and R.A.B. provided biogeochemical interpretation. E.R.R.M., T.M.L., A.S., T.A.W., D.P. and P.J.C.D. drafted the article to which all authors (including X.C., N.L., Z.Y. and G.A.S.) contributed.
Corresponding authors
Correspondence to Edmund R. R. Moody , Davide Pisani , Tom A. Williams , Timothy M. Lenton or Philip C. J. Donoghue .
Ethics declarations
Competing interests.
The authors declare no competing interests.
Peer review
Peer review information.
Nature Ecology & Evolution thanks Aaron Goldman and the other, anonymous, reviewer(s) for their contribution to the peer review of this work. Peer reviewer reports are available.
Additional information
Publisher’s note Springer Nature remains neutral with regard to jurisdictional claims in published maps and institutional affiliations.
Extended data
Extended data fig. 1 comparison of the mean divergence times and confidence intervals estimated for the two duplicates of luca under each timetree inference analysis..
Black dots refer to estimated mean divergence times for analyses without cross-bracing, stars are used to identify those under cross-bracing and triangles for estimated upper and lower confidence intervals. Straight lines are used to link mean divergence time estimates across the various inference analyses we carried out, while dashed lines are used to link the estimated confidence intervals. The node label for the driver node is “248”, while it is “368” for the mirror node, as shown in the title of each graph. Coloured stars and triangles are used to identify which LUCA time estimates were inferred under the same cross-braced analysis for the driver-mirror nodes (that is, equal time and CI estimates). Black dots and triangles are used to identify those inferred when cross-bracing was not enabled (that is, different time and CI estimates). -Abbreviations. “GBM”: Geometric Brownian motion relaxed-clock model; “ILN”: Independent-rate log-normal relaxed-clock model; “conc, cb” dots/triangles: results under cross-bracing A when the concatenated dataset was analysed under GBM (red) and ILN (blue); “conc, fosscb”: results under cross-bracing B when the concatenated dataset was analysed under GBM (orange) and ILN (cyan); “part, cb” dots/triangles: results under cross-bracing A when the partitioned dataset was analysed under GBM (pink) and ILN (purple); “part, fosscb”: results under cross-bracing B when the concatenated dataset was analysed under GBM (light green) and ILN (grey); black dots and triangles: results when cross-bracing was not enabled for both concatenated and partitioned datasets.
Extended Data Fig. 2 Comparison of the posterior time estimates and confidence intervals for the two duplicates of LUCA inferred under the main calibration strategy cross-bracing A with the concatenated dataset and with the datasets for the three additional sensitivity analyses.
Dots refer to estimated mean divergence times and triangles to estimated 2.5% and 97.5% quantiles. Straight lines are used to link the mean divergence times estimated in the same analysis under the two different relaxed-clock models (GBM and ILN). Labels in the x axis are informative about the clock model under which the analysis ran and the type of analysis we carried (see abbreviations below). Coloured dots are used to identify which time estimates were inferred when using the same dataset and strategy under GBM and ILN, while triangles refer to the corresponding upper and lower quantiles for the 95% confidence interval. -Abbreviations. “GBM”: Geometric Brownian motion relaxed-clock model; “ILN”: Independent-rate log-normal relaxed-clock model; “main-conc”: results obtained with the concatenated dataset analysed in our main analyses under cross-bracing A; “ATP/EF/Leu/SRP/Tyr”: results obtained when using each gene alignment separately; “noATP/noEF/noLeu/noSRP/noTyr”: results obtained when using concatenated alignments without the gene alignment mentioned in the label as per the “leave-one-out” strategy; “main-bsinbv”: results obtained with the concatenated dataset analysed in our main analyses when using branch lengths, Hessian, and gradient calculated under a more complex substitution model to infer divergence times.
Extended Data Fig. 3 Maximum Likelihood species tree.
The Maximum Likelihood tree inferred across three independent runs, under the best fitting model (according to BIC: LG + F + G + C60) from a concatenation of 57 orthologous proteins, support values are from 10,000 ultrafast bootstraps. Referred to as topology I in the main text. Tips coloured according to taxonomy: Euryarchaeota (teal), DPANN (purple), Asgardarchaeota (cyan), TACK (blue), Gracilicutes (orange), Terrabacteria (red), DST (brown), CPR (green).
Extended Data Fig. 4 Maximum Likelihood tree for focal reconciliation analysis.
Maximum Likelihood tree (topology II in the main text), where DPANN is constrained to be sister to all other Archaea, and CPR is sister to Chloroflexi. Tips coloured according to taxonomy: Euryarchaeota (teal), DPANN (purple), Asgardarchaeota (cyan), TACK (blue), Gracilicutes (orange), Terrabacteria (red), DST (brown), CPR (green). AU topology test, P = 0.517, this is a one-sided statistical test.
Extended Data Fig. 5 The relationship between the number of KO gene families encoded on a genome and its size.
LOESS regression of the number of KOs per sampled genome against the genome size in megabases. We used the inferred relationship for modern prokaryotes to estimate LUCA’s genome size based on reconstructed KO gene family content, as described in the main text. Shaded area represents the 95% confidence interval.
Extended Data Fig. 6 The relationship between the number of KO gene families encoded on a genome and the total number of protein-coding genes.
LOESS regression of the number of KOs per sampled genome against the number of proteins encoded for per sampled genome. We used the inferred relationship for modern prokaryotes to estimate the total number of protein-coding genes encoded by LUCA based on reconstructed KO gene family content, as described in the main text. Shaded area represents the 95% confidence interval.
Supplementary information
Supplementary information.
Supplementary Notes and Figs. 1–10.
Reporting Summary
Peer review file, supplementary data 1.
This table contains the results of the reconciliations for each gene family. KEGG_ko is the KEGG orthology ID; arc_domain_prop is the proportion of the sampled Archaea; bac_domain_prop is the proportion of the sampled bacteria; gene refers to gene name, description and enzyme code; map refers to the different KEGG maps of which this KEGG gene family is a component; pathway is a text description of the metabolic pathways of which these genes are a component; alignment_length refers to the length of the alignment in amino acids; highest_COG_cat refers to the number of sequences placed in the most frequent COG category; difference_1st_and_2nd is the difference between the most frequent COG category and the second most frequent COG category; categories is the number of different COG categories assigned to this KEGG gene family; COG_freq is the proportion of the sequences placed in the most frequent COG category; COG_cat is the most frequent COG functional category; Archaea is the number of archaeal sequences sampled in the gene family; Bacteria is the number of bacterial sequences sampled in the gene family; alternative_COGs is the number of alternative COG gene families assigned across this KEGG orthologous gene family; COG_perc is the proportion of the most frequent COG ID assigned to this KEGG gene family; COG is the COG ID of the most frequenty COG assigned to this gene family; COG_NAME is the description of the most frequent COG ID assigned to this gene family; COG_TAG is the symbol associated with the most frequent COG gene familiy; sequences is the total number of sequences assigned to this gene family; Arc_prop is the proportion of Archaea that make up this gene family; Bac_prop is the proportion of Bacteria that make up this gene family; constrained_median is the median probability (PP) that this gene was present in LUCA from our reconciliation under the focal constrained tree search across the 5 independent bootstrap distribution reconciliations; ML_median is the median PP of the gene family being present in LUCA with gene tree bootstrap distributions against the ML species-tree topology across the 15 independent bootstrap distribution reconciliations; MEAN_OF_MEDIANS is the mean value across the constrained and ML PP results; RANGE_OF_MEDIANS is the range of the PPs for the constrained and ML topology PPs for LUCA; Probable_and_sampling_threshold_met is our most stringent category of gene families inferred in LUCA with 0.75 + PP and a sampling requirement of 1% met in both Archaea and Bacteria; Possible_and_sampling_threshold_met is a threshold of 0.50 + PP and sampling both domains; probable is simply 0.75 + PP; and possible is 0.50 + PP.
Supplementary Data 2
PP for COGs. This table contains the results for the reconciliations of COG-based gene family clustering against the constrained focal species-tree topology. Columns are named similarly to Supplementary Data 1 but each row is a different COG family. The column Modal_KEGG_ko refers to the most frequent KEGG gene family in which a given COG is found; sequences_in_modal_KEGG refers to the number of sequences in the most frequent KEGG gene family.
Supplementary Data 3
Module completeness. Estimated pathway completeness for KEGG metabolic modules (with a completeness greater than zero in at least one confidence threshold) using Anvi’o’s stepwise pathway completeness 48 . Module_name is the name of the module; module_category is the broader category into which the module falls; module_subcategory is a more specific category; possible_anvio includes the gene families with a median PP ≥ 0.50; probable_anvio related to gene families PP ≥ 0.75; and _ws refers to the sampling requirement being met (presence in at least 1% of the sampled Archaea and Bacteria).
Supplementary Data 4
Marker gene metadata for all markers checked during marker gene curation, including the initial 59 single-copy marker genes used in species-tree inference (see Methods ). Data include marker gene set provenance, marker gene name, marker gene description, presence in different marker gene sets 49 , 50 , 51 , 52 , 53 , 54 , 55 , 56 , 57 , 58 , and presence in Archaea and Bacteria. When available, marker genes are matched with their arCOG, TIGR, and COG ID and their respective occurrence across different taxonomic sets is quantified.
Supplementary Data 5
The ratio of duplications, transfers and losses in relation to the total number of copies for the deep ancestral nodes: the LUCA, archaeal (LACA) and bacterial (LBCA) common ancestors, and the average (mean) and 95th percentile.
Supplementary Data 6
Spreadsheet containing a list of the estimated divergence times for all timetree inferences carried out and the corresponding results of the MCMC diagnostics. Tabs Divtimes_GBM-allnodes and Divtimes_ILN-allnodes represent a list of the estimated divergence times (Ma) for all nodes under the 12 inference analyses we ran under GBM and ILN, respectively. Tabs Divtimes_GBM-highlighted and Divtimes_ILN-highlighted represent a list of the estimated divergence times (Ma) for selected nodes ordered according to their mirrored nodes under the 12 inference analyses we ran under GBM and ILN, respectively. Each of the tabs MCMCdiagn_prior, MCMCdiagn_postGBM and MCMCdiagn_postILN contains the statistical results of the MCMC diagnostics we ran for each inference analysis. Note that, despite the analyses carried out when sampling from the prior could have only been done three times (that is, data are not used, and thus only once under each calibration strategy was enough), we repeated them with each dataset regardless. In other words, results for (1) ‘concatenated + cross-bracing A’ and ‘partitioned + cross-bracing A’; (2) ‘concatenated + without cross-bracing’ and ‘partitioned + without cross-bracing’; and (3) ‘concatenated + cross-bracing B’ and ‘partitioned + cross-bracing B’ would be equivalent, respectively. For tabs 1–4, part represents partitioned dataset; conc, concatenated dataset; cb, cross-bracing A; notcb, without cross-bracing; fosscb, cross-bracing B; mean_t, mean posterior time estimate; 2.5%q, 2.5% quantile of the posterior time density for a given node; and 97.5%q, 97.5% quantile of the posterior time density for a given node. For tabs 5–7, med. num. samples collected per chain represents median of the total amount of samples collected per chain; min. num. samples collected per chain, minimum number of samples collected per chain; max. num. samples collected per chain, minimum number of samples collected per chain; num. samples used to calculate stats, number of samples collected by all chains that passed the filters that were used to calculate the tail-ESS, bulk-ESS and R-hat values. For tail-ESS, we report the median, minimum, and maximum tail-ESS values; all larger than 100 as required for assuming reliable parameter estimates. For bulk-ESS, we report the median, minimum and maximum bulk-ESS values; all larger than 100 as required for assuming reliable parameter estimates. For R-hat, minimum and maximum values reported, all smaller than 1.05 as required to assume good mixing.
Supplementary Data 7
Spreadsheet containing a list of the posterior time estimates for LUCA obtained under the main calibration strategy cross-bracing A with the concatenated dataset and with the datasets for the three additional sensitivity analyses. The first column ‘label’ contains the node number for both the driver and mirror nodes for LUCA (the latter includes the term -dup in the label). Columns mean_t, 2.5%q, and 97.5%q refer to the estimated mean divergence times, and the 2.5%/97.5% quantiles of the posterior time density for the corresponding node. Main-conc, refers to results obtained with the concatenated dataset analysed in our main analyses under cross-bracing A; ATP/EF/Leu/SRP/Tyr, results obtained when using each gene alignment separately; noATP/noEF/noLeu/noSRP/noTyr, results obtained when using concatenated alignments without the gene alignment mentioned in the label as per the leave-one-out strategy; main-bsinbv, results obtained with the concatenated dataset analysed in our main analyses when using branch lengths, Hessian and gradient calculated under a more complex substitution model to infer divergence times.
Supplementary Data 8
Spreadsheet containing a list of the estimated divergence times for all timetree inferences carried out for the sensitivity analyses and the corresponding results for the MCMC diagnostics. Tabs Divtimes_GBM-allnodes and Divtimes_ILN-allnodes represent a list of the estimated divergence times (Ma) for all nodes under the 11 inference analyses we ran under GBM and ILN when testing the impact on divergence times estimation when (1) analysing each gene alignment individually, (2) following a leave-one-out strategy, and (3) using the branch lengths, Hessian and gradient estimated under a more complex model for timetree inference (bsinBV approach). Tabs Divtimes_GBM-highlighted and Divtimes_ILN-highlighted represent a list of the estimated divergence times (Ma) for selected nodes ordered according to their mirrored nodes we ran under GBM and ILN for the sensitivity analyses (we also included the results with the main concatenated dataset for reference). Each of tabs MCMCdiagn_prior, MCMCdiagn_postGBM and MCMCdiagn_postILN contains the statistical results of the MCMC diagnostics we ran for the sensitivity analyses. Note that, despite the analyses carried out when sampling from the prior could have only been done once for each different tree topology (that is, data are not used, only topological changes may affect the resulting marginal densities), we ran them with each dataset regardless as part of our pipeline. For tabs 1–4, main-conc represents results obtained with the concatenated dataset analysed in our main analyses under cross-bracing A; ATP/EF/Leu/SRP/Tyr, results obtained when using each gene alignment separately; noATP/noEF/noLeu/noSRP/noTyr, results obtained when using concatenated alignments without the gene alignment mentioned in the label as per the leave-one-out strategy; main-bsinbv, results obtained with the concatenated dataset analysed in our main analyses when using branch lengths, Hessian and gradient calculated under a more complex substitution model to infer divergence times; mean_t, mean posterior time estimate; 2.5%q, 2.5% quantile of the posterior time density for a given node; and 97.5%q, 97.5% quantile of the posterior time density for a given node. For tabs 5–7, med. num. samples collected per chain represents the median of the total amount of samples collected per chain; min. num. samples collected per chain, minimum number of samples collected per chain; max. num. samples collected per chain, minimum number of samples collected per chain; num. samples used to calculate stats, number of samples collected by all chains that passed the filters that were used to calculate the tail-ESS, bulk-ESS and R-hat values. For tail-ESS, we report the median, minimum and maximum tail-ESS values; all larger than 100 as required for assuming reliable parameter estimates. For bulk-ESS, we report the median, minimum and maximum bulk-ESS values; all larger than 100 as required for assuming reliable parameter estimates. For R-hat, minimum and maximum values are reported, all smaller than 1.05 as required to assume good mixing.
Rights and permissions
Open Access This article is licensed under a Creative Commons Attribution 4.0 International License, which permits use, sharing, adaptation, distribution and reproduction in any medium or format, as long as you give appropriate credit to the original author(s) and the source, provide a link to the Creative Commons licence, and indicate if changes were made. The images or other third party material in this article are included in the article’s Creative Commons licence, unless indicated otherwise in a credit line to the material. If material is not included in the article’s Creative Commons licence and your intended use is not permitted by statutory regulation or exceeds the permitted use, you will need to obtain permission directly from the copyright holder. To view a copy of this licence, visit http://creativecommons.org/licenses/by/4.0/ .
Reprints and permissions
About this article
Cite this article.
Moody, E.R.R., Álvarez-Carretero, S., Mahendrarajah, T.A. et al. The nature of the last universal common ancestor and its impact on the early Earth system. Nat Ecol Evol (2024). https://doi.org/10.1038/s41559-024-02461-1
Download citation
Received : 19 January 2024
Accepted : 04 June 2024
Published : 12 July 2024
DOI : https://doi.org/10.1038/s41559-024-02461-1
Share this article
Anyone you share the following link with will be able to read this content:
Sorry, a shareable link is not currently available for this article.
Provided by the Springer Nature SharedIt content-sharing initiative
Quick links
- Explore articles by subject
- Guide to authors
- Editorial policies
Sign up for the Nature Briefing: Microbiology newsletter — what matters in microbiology research, free to your inbox weekly.

- Corpus ID: 271245120
An Evaluation of Continual Learning for Advanced Node Semiconductor Defect Inspection
- Amit Prasad , Bappaditya Dey , +1 author Sandip Halder
- Published 17 July 2024
- Engineering, Computer Science, Materials Science
Figures and Tables from this paper
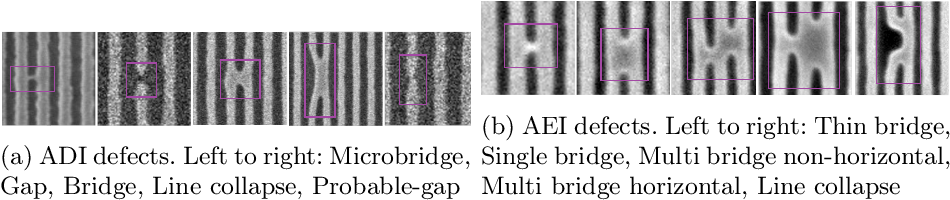
7 References
A comparative study of deep-learning object detectors for semiconductor defect detection, deep learning-based defect classification and detection in sem images, class-incremental learning: survey and performance evaluation on image classification, yolov8 for defect inspection of hexagonal directed self-assembly patterns: a data-centric approach, incremental object detection via meta-learning, faster r-cnn: towards real-time object detection with region proposal networks, related papers.
Showing 1 through 3 of 0 Related Papers
- Open access
- Published: 15 July 2024
Enhancing psychological well-being in college students: the mediating role of perceived social support and resilience in coping styles
- Shihong Dong 1 ,
- Huaiju Ge 1 ,
- Wenyu Su 1 ,
- Weimin Guan 1 ,
- Xinquan Li 1 ,
- Yan Liu 2 ,
- Qing Yu 1 ,
- Yuantao Qi 2 ,
- Huiqing Zhang 3 &
- Guifeng Ma 1
BMC Psychology volume 12 , Article number: 393 ( 2024 ) Cite this article
332 Accesses
Metrics details
The prevalence of depression among college students is higher than that of the general population. Although a growing body of research suggests that depression in college students and their potential risk factors, few studies have focused on the correlation between depression and risk factors. This study aims to explore the mediating role of perceived social support and resilience in the relationship between trait coping styles and depression among college students.
A total of 1262 college students completed questionnaires including the Trait Coping Styles Questionnaire (TCSQ), the Patient Health Questionnaire-9 (PHQ-9), the Perceived Social Support Scale (PSSS), and the Resilience Scale-14 (RS-14). Common method bias tests and spearman were conducted, then regressions and bootstrap tests were used to examine the mediating effects.
In college students, there was a negative correlation between perceived control PC and depression, with a significant direct predictive effect on depression ( β = -0.067, P < 0.01); in contrast, negative control NC showed the opposite relationship ( β = 0.057, P < 0.01). PC significantly positively predicted perceived social support ( β = 0.575, P < 0.01) and psychological resilience ( β = 1.363, P < 0.01); conversely, NC exerted a significant negative impact. Perceived social support could positively predict psychological resilience ( β = 0.303, P < 0.01), and both factors had a significant negative predictive effect on depression. Additionally, Perceived social support and resilience played a significant mediating role in the relationship between trait coping styles and depression among college students, with three mediating paths: PC/NC → perceived social support → depression among college students (-0.049/0.033), PC/NC→ resilience → depression among college students (-0.122/-0.021), and PC/NC → perceived social support → resilience → depression among college students (-0.016/0.026).
The results indicate that trait coping styles among college students not only directly predict lower depression but also indirectly influence them through perceived social support and resilience. This suggests that guiding students to confront and solve problems can alleviate their depression.
Peer Review reports
Introduction
Depression is a complex mental disorder, characterized by cognitive, affective and psychosocial symptoms [ 1 , 2 ]. It is projected that by 2030, depression will rank first globally in terms of years lived with disability [ 3 , 4 ]. Depression is also one of the most common mental health issues among contemporary college students [ 5 , 6 ]. Studies have shown that the detection rate of depression among Chinese college students ranges from 23–34% [ 7 , 8 ]. Compared to non-student populations, college students have a higher prevalence of depression, and this rate seems to be increasing [ 9 ]. This vulnerable group of college students is in a unique developmental stage, facing pressures not only from life but also from the demands of academic coursework and complex interpersonal relationships, making the factors influencing depression among college students, particularly complex [ 9 , 10 ].
Exploring the mechanisms by which influencing factors affect the occurrence of depression in college students is of significant importance for early prevention [ 11 ]. Research has demonstrated that trait coping style is one of the risk factors for depression among college students. Trait coping refers to the strategies individuals employ in challenging situations, categorized into positive coping and negative coping [ 12 , 13 ]. Positive coping focuses on taking effective action and changing stressful situations, typically associated with problem-solving behaviors and regulation of positive emotions, which can help reduce the incidence of depression [ 14 ]. Conversely, negative coping is a passive approach centered around negative evaluations and emotional expression, often involving avoiding problems and social isolation, which is more likely to lead to the development of depression [ 14 ]. Research indicates that positive coping strategies are inversely correlated with depression, serving as protective factors against depression. Conversely, negative coping strategies are positively associated with depression, acting as risk factors for its onset [ 15 ].
Perceived social support refers to an individual’s subjective emotional state of feeling supported and understood by family, friends, and other sources [ 16 , 17 ]. Prior studies have shown that perceived social support can directly impact an individual’s level of depression and also have indirect effects [ 18 ]. The data indicate that social support can significantly influence coping mechanisms, with groups having higher levels of social support tended to respond more actively and positively to stress from various sources [ 19 ]. Social support is considered an important mediating factor in determining the relationship between psychological stress and health, representing an emotional experience where individuals feel supported, respected, and understood [ 16 ]. The relationship between individuals’ coping strategies and depression may be influenced by the mediating role of perceived social support [ 20 , 21 ]. In addition to this, resilience plays a role in all three.Resilience refers to the ability to adapt to stress and adversity, enhancing an individual’s psychological well-being [ 22 ]. Both coping styles and perceived social support significantly predict resilience positively [ 23 ]. For individuals with strong resilience, possessing a high level of adaptive capacity can mitigate the negative effects of stress on individuals, thereby enhancing their mental health.
In recent years, there has been a growing body of research on the prevalence of depression among college students. However, the rates of depression vary in different environments, and there is limited research on the mechanisms through which trait coping styles, perceived social support, and resilience impact depression. Therefore, this study aims to investigate the mechanisms through which positive coping styles(PC), negative coping styles(NC), perceived social support, and resilience influence depression among college students. Additionally, it seeks to analyze the mediating roles of perceived social support and resilience in this context. The goal is to provide insights into the reasons behind depression among college students under different coping strategies, aiding in timely psychological adjustment to promote the comprehensive development of the mental and physical well-being of college students.
The following assumptions were made:
Hypothesis 1
PC has a significant negative predictive effect on depression among college students. NC has a significant positive predictive effect on depression among college students.
Hypothesis 2
Perceived social support serves as a mediator between PC/NC and depression among college students.
Hypothesis 3
Resilience mediates the relationship between PC/NC and depression among college students.
Hypothesis 4
Perceived social support and psychological resilience mediate the relationship between PC/NC and depression among college students in a serial manner.
Data and methods
This is a cross-sectional study that was conducted from January through February 2024. Using the Questionnaire Star network platform, we presented the questionnaire online, which was openly accessible to college students at a university in Shandong. The average time to complete the survey was 15 min. Participation was voluntary and students were informed about the purpose of the study. Confidentiality was assured and questionnaires were submitted anonymously. A total of 1267 enrolled college students participated in the questionnaire survey. After excluding invalid questionnaires, 1262 valid questionnaires were included, resulting in an effective rate of 99.57%.
Trait coping style questionnaire
The Trait Coping Style Questionnaire (TCSQ) [ 24 ], developed by Qianjin Jiang, was utilized to assess the trait coping styles of college students. This questionnaire reflects the participants’ approaches to coping with situations, comprising a total of 20 items. It consists of two dimensions: negative coping style and positive coping style, each with 10 items. Using a 5-point Likert scale ranging from “definitely not” to “definitely yes,” scores were assigned from 1.00 to 5.00. The Cronbach’s α coefficient for negative coping style was 0.906 and for positive coping style was 0.786 in this study.
Depression scale
The Patient Health Questionnaire-9 (PHQ-9) [ 25 ] was used to assess depressive symptoms in the past two weeks. This scale consists of 9 items rated on a 4-point Likert scale ranging from “not at all” to “nearly every day,” with scores from 0 to 3. The total score ranges from 0 to 27, with higher scores indicating more severe depressive symptoms. The Cronbach’s α coefficient for this scale in the current study was 0.884.
Perceived Social Support Scale
The Perception Social Support Scale (PSSS) was compiled by James A.Blumenthal in 1987 and later translated and modified by Qianjin Jiang to form the Chinese version of the Zimetm Perception Social Support Scale (PSSS) [ 26 , 27 ]. PSSS comprises 12 self-assessment items rated on a 7-point Likert scale. The scale includes three dimensions: family support (items 3, 4, 8, 11), friend support (items 6, 7, 9, 12), and other support (items 1, 2, 5, 10), with a total score ranging from 12 to 84. Scores of 12–36 indicate low support, 37–60 indicate moderate support, and 61–84 indicate high support. The Cronbach’s α for this scale in the current survey was 0.968.
Resilience scale
The Resilience Scale (RS-14) [ 28 ] Chinese version consists of 14 items, each rated on a 7-point Likert scale from “not at all” to “completely,” with scores ranging from 1 to 7. The total score ranges from 14 to 98, with higher scores indicating better resilience. The Cronbach’s α for this scale in the current study was 0.925.
Statistical analysis
Data were organized and analyzed using SPSS 26.0 software. Confirmatory factor analysis was first conducted on the questionnaires. Descriptive analysis was then performed on the scores of each scale. Spearman was used to examine the relationships between trait coping styles, perceived social support, resilience, and depression. Mediation analysis was carried out using the SPSS PROCESS macro 3.4.1 software model 6 developed by Hayes, specifically designed for testing complex models. Model 6 was applied for two mediating variables, followed by the bias-corrected percentile Bootstrap method with 5000 resamples to estimate the 95% confidence interval of the mediation effect. A significant mediation effect was indicated if the 95% confidence interval (CI) did not include zero. A significance level of P < 0.05 was considered statistically significant.
Examination of common method bias
Systematic errors in indicator data results caused by the same data collection method or measurement environment can typically be assessed through the Harman single-factor test on 55 items in the dataset to examine common method bias. The results indicated that there were 7 factors with eigenvalues greater than 1, and the variance explained by the first factor was 34.84%, which was below the critical threshold of 40%. Therefore, this study may not have a significant common method bias.
Descriptive statistics and correlation analysis
The mean scores, standard deviations, and correlations of each variable are presented in Table 1 . PC ( r = -0.326, P < 0.01), resilience ( r =-0.445, P < 0.01), and perceived social support ( r =-0.405, P < 0.01) were negatively correlated with depression. PC ( r = 0.336, P < 0.01) and resilience ( r = 0.469, P < 0.01) were significantly positively correlated with perceived social support. PC was significantly positively correlated with resilience( r = 0.635, P < 0.01). NC was significantly positively correlated with depression( r = 0.322, P < 0.01) and PC( r = 0.146, P < 0.01). NC was significantly negatively correlated with perceived social support ( r =-0.325, P < 0.01).
Analysis of chain mediation effects
The chain mediation model was validated using SPSS PROCESS Model 6. Trait coping styles were considered as the independent variable, while depression among college students was treated as the dependent variable. Perceived social support and resilience were included as the mediating variables, culminating in the path model depicted in Figs. 1 and 2 .
The results of the regression analysis, as shown in Table 2 , indicated that PC could significantly predict perceived social support in a positive direction ( β = 0.575, P < 0.01). Both PC ( β = 1.363, P < 0.01) and perceived social support ( β = 0.303, P < 0.01) had significant positive predictive effects on psychological resilience. When simultaneously predicting depression using PC, perceived social support, and psychological resilience, all three exhibited significant negative predictive effects ( β = -0.067, β = -0.085, β = -0.090, P < 0.01). NC could significantly predict perceived social support in a negative direction ( β = -0.457, P < 0.01). When NC ( β = 0.191, P < 0.01) and perceived social support ( β = 0.508, P < 0.01) jointly predict psychological resilience, they both had significant positive predictive effects. When simultaneously predicting depression using NC, perceived social support, and psychological resilience, NC ( β = 0.057, P < 0.01) showed a significant positive predictive effect, while perceived social support ( β = -0.072, P < 0.01) and psychological resilience ( β = -0.112, P < 0.01) demonstrated significant negative predictive effects.
Further employing the Bootstrap sampling method, with 5000 repetitions, the significance of the mediating effects and chain mediation effects between trait coping styles and depression among college students was examined. The results indicated that the direct effects of PC/NC on depression were significant, with direct impact values of -0.067/0.057 (26.38%/60.00%). Perceived social support and psychological resilience mediated the relationship between PC/NC and depression, with this mediation encompassing three pathways: the separate mediating effect of perceived social support, with effect values of -0.049 and 0.033 respectively; the separate mediating effect of resilience, with effect values of -0.122 and − 0.021 respectively; and the serial mediating effect from perceived social support to resilience, with effect values of -0.016, -0.021, and 0.026. The 95% confidence intervals for all pathways did not include 0, indicating significant indirect effects. Therefore, the total indirect effects were − 0.187 (73.62%) and 0.038 (40.00%), showing that PC had a weaker direct effect on depression compared to NC, but a stronger indirect effect. This was illustrated in Table 3 .
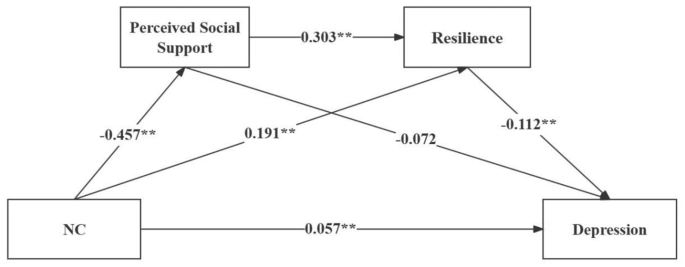
Chain mediation model of perceived social support and resilience between PC and depression. ** p < 0.01
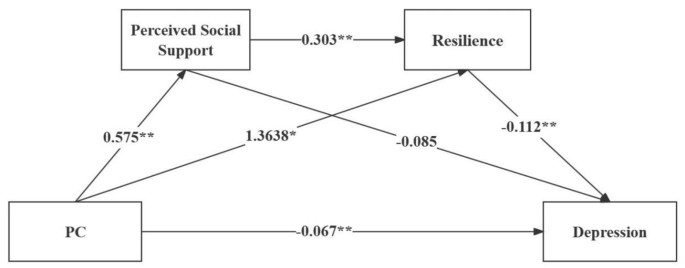
Chain mediation model of perceived social support and resilience between NC and depression. ** p < 0.01
Previous research on the associations and specific pathways among depressive symptoms, trait coping styles, perceived social support, and resilience in college students has been limited. Therefore, this study utilized a chain mediation model to examine how trait coping styles, perceived social support, and resilience influence depressive symptoms in college students. The results indicate that perceived social support and resilience not only act as separate mediators between PC/NC and depression but also exhibit a chain mediation effect.
Mechanisms of the impact of PC/NC on depression in college students
This study found that trait coping styles can significantly and negatively predict depressive symptoms in college students directly, consistent with previous research [ 29 ]. In recent years, amidst the backdrop of the pandemic, numerous studies have emerged domestically and internationally focusing on college students’ mental health from the perspective of crisis event coping [ 30 ]. These studies have predominantly concentrated on trait coping styles as a mediating variable in predicting the occurrence of depressive symptoms, with fewer studies examining the direct impact of trait coping styles on depressive symptoms. College students, being in a unique developmental stage, face challenges from various aspects and bear the pressures of academic coursework, interpersonal relationships, and future employment. Research indicates that trait coping styles are a key factor influencing mental health [ 31 ]. Implementing healthy coping techniques and interventions can help individuals overcome negative emotions caused by stress, which is an adaptive coping mechanism that assists college students in facing stress and enhancing problem-solving abilities, thus preventing or reducing the occurrence of depression. Conversely, adopting passive or avoidant coping strategies, leading to inadequate resolution of stress events, can increase psychological stress [ 14 ], thereby exerting a negative impact on the mental health of college students [ 32 ]. Therefore, trait coping styles play a negative predictive role in depressive symptoms among college students. PC was a positive predictor of depression and NC was a negative predictor of depression. This is consistent with previous studies [ 24 , 29 ].
Separate mediating effects of perceived social support and resilience
After introducing perceived social support and resilience as two mediating variables, the predictive effect of PC/NC on depressive symptoms in college students remained significant. The results show that PC can positively predict perceived social support, and NC is the opposite, consistent with previous research [ 33 ]. Trait coping styles are an important predictive factor in altering college students’ perceptions of social support and the occurrence of depression. Individuals who adopt negative coping styles tend to perceive relatively less external support. Some argue that social support plays a reverse predictive role in trait coping styles; the more social support college students receive and feel, the more likely they are to actively adopt positive coping strategies to alleviate stress, potentially due to variations in study subjects and time [ 34 ]. In this pathway, perceived social support can significantly and negatively predict depressive symptoms, aligning with previous research findings [ 35 ]. Perceived social support is considered a crucial mediating factor influencing mental health, referring to an individual’s ability to perceive support and understanding from family, friends, and others. College students with lower levels of perceived social support often feel neglected and undervalued, leading to negative evaluations and self-doubt, making them more susceptible to depression. PC/NC and perceived social support can interact and influence the occurrence of depressive symptoms in college students [ 16 ].
Research indicates that PC can significantly and positively predict resilience, with an indirect effect value of 48.03%.In this pathway, the mediating effect of resilience is more pronounced, consistent with previous studies [ 36 ]. There is a close connection between resilience and coping styles; college students who adopt positive coping strategies often exhibit stronger psychological resilience, being more willing to confront issues and seek help from others to solve problems. When facing pressures such as academic challenges, they approach them with a positive mindset, overcoming adversity [ 37 ]. It is believed that adopting positive coping strategies to address problems can enhance college students’ levels of psychological resilience [ 10 , 38 ]. Resilience can significantly and negatively predict depressive symptoms. depressive symptoms, College students with higher levels of resilience tend to define the severity of events less severely when stress events occur, resulting in lower psychological burdens and reduced likelihood of experiencing depressive symptoms [ 10 ]. Additionally, when facing setbacks or stress, individuals who adopt positive coping strategies actively utilize internal and external protective factors to combat current difficulties and pressures, and employ effective emotional control to mitigate the impact, thereby enhancing their levels of psychological resilience and reducing the occurrence of depression.
Chain mediation effect of perceived social support and psychological resilience
This study elucidates that PC/NC perceived social support, and psychological resilience are independent factors influencing depressive symptoms in college students, with perceived social support and psychological resilience playing a mediating role between coping styles and depressive symptoms. The share of total indirect effect values is 73.62% and 40.00%, respectively, with the third chain path accounting for 6.30% and 27.37% of the total effect ratio, respectively. This confirms the existence of this chain mediation effect, although the chain mediation effect is not as pronounced as the individual mediation effects. Positive coping styles not only directly negatively predict depressive symptoms in college students but also exert an indirect influence on depressive symptoms through perceived social support and psychological resilience. Likewise, negative coping styles not only directly positively predict depressive symptoms in college students but also have an indirect impact on depressive symptoms through perceived social support and psychological resilience, thus demonstrating the value and significance of these two mediating variables in reducing the occurrence of depressive symptoms in college students.
Initially, adopting positive coping styles and being able to perceive social support are crucial factors influencing psychological resilience in college students. There exists a relatively stable systemic relationship between students’ social support and psychological resilience, confirming that social support can enhance individuals’ levels of psychological resilience [ 16 ]. Furthermore, coping styles can affect the occurrence of depressive symptoms from both internal and external perspectives. This is because the social support perceived by college students includes not only tangible social support resources but also their subjective perception of social support, with these two factors constituting external and internal protective factors of psychological resilience [ 39 ]. Positive coping and effective adaptation can enhance college students’ perception of social support, enabling them to mobilize personal, familial, and societal protective factors better when facing various life challenges, thereby mitigating or eliminating difficulties and suppressing the onset of depressive symptoms, whereas negative coping styles yield the opposite effect. The chain mediation proposed in this study integrates the research on perceived social support, psychological resilience, and depressive symptoms in college students, facilitating a more comprehensive understanding of the internal mechanisms through which coping styles influence depressive symptoms in college students. This holds significance in advocating for a proactive attitude in college students to confront and resolve difficulties and in increasing attention to the mental health of college students.
Limitations, strengths and future research
The findings of this study hold theoretical value and practical implications, offering a reference basis for improving the mental health of college students. However, there are certain limitations to consider. Firstly, the survey in this study was conducted through self-reporting, which may introduce certain biases. Future research could explore data collection through various methods. Secondly, this study employed a cross-sectional design to investigate the impact of trait coping styles, on depression among college students and its potential mechanisms. However, this research approach does not allow for causal inferences between variables, and further validation of the study’s conclusions could be achieved through longitudinal or experimental research.
In summary, this study aims to improve the mental health of college students by examining how their coping styles, along with their perceived social support and psychological resilience, affect depressive symptoms. The research analyzes the connections between these factors and suggests that positive coping styles may help prevent depression. However, the study has its limitations and future research should use long-term experiments to better understand these relationships. Since depression in college students can be influenced by many factors, future studies should also consider additional variables and use a mix of experimental and longitudinal approaches to more clearly understand how to reduce depression in this group.
Data availability
The datasets used and analysed during the current study are available from the corresponding author upon reasonable request.
Abbreviations
Patient Health Questionnaire-9
Trait Coping Style Questionnaire
Positive coping styles
Negative coping styles
Resilience Scale
Platania GA, Savia Guerrera C, Sarti P, et al. Predictors of functional outcome in patients with major depression and bipolar disorder: a dynamic network approach to identify distinct patterns of interacting symptoms[J]. PLoS ONE. 2023;18(2):e0276822.
Article PubMed PubMed Central Google Scholar
Guerrera CS, Platania GA, Boccaccio FM, et al. The dynamic interaction between symptoms and pharmacological treatment in patients with major depressive disorder: the role of network intervention analysis[J]. BMC Psychiatry. 2023;23(1):885.
Liu Y, Chen J, Chen K, et al. The associations between academic stress and depression among college students: a moderated chain mediation model of negative affect, sleep quality, and social support[J]. Acta Psychol. 2023;239:104014.
Article Google Scholar
Gao L, Xie Y, Jia C, et al. Prevalence of depression among Chinese university students: a systematic review and meta-analysis[J]. Sci Rep. 2020;10(1):15897.
Naja WJ, Kansoun AH, Haddad RS. Prevalence of Depression in Medical students at the Lebanese University and exploring its correlation with Facebook Relevance: a Questionnaire Study[J]. JMIR Res Protocols. 2016;5(2):e96.
Brenneisen Mayer F, Souza Santos I, Silveira PSP, et al. Factors associated to depression and anxiety in medical students: a multicenter study[J]. BMC Med Educ. 2016;16(1):282.
Liu Y, Zhang N, Bao G, et al. Predictors of depressive symptoms in college students: a systematic review and meta-analysis of cohort studies[J]. J Affect Disord. 2019;244:196–208.
Article PubMed Google Scholar
Moutinho ILD, Maddalena N, D C P, Roland RK, et al. Depression, stress and anxiety in medical students: a cross-sectional comparison between students from different semesters[J]. Volume 63. Revista da Associação Médica Brasileira; 2017. pp. 21–8. 1.
Acharya L, Jin L, Collins W. College life is stressful today – emerging stressors and depressive symptoms in college students[J]. J Am Coll Health. 2018;66(7):655–64.
Ibrahim AK, Kelly SJ, Adams CE, et al. A systematic review of studies of depression prevalence in university students[J]. J Psychiatr Res. 2013;47(3):391–400.
Zvauya R, Oyebode F, Day EJ, et al. A comparison of stress levels, coping styles and psychological morbidity between graduate-entry and traditional undergraduate medical students during the first 2 years at a UK medical school[J]. BMC Res Notes. 2017;10(1):93.
Undheim AM, Sund AM. Associations of stressful life events with coping strategies of 12–15-year-old Norwegian adolescents[J]. Eur Child Adolesc Psychiatry. 2017;26(8):993–1003.
Lau Y, Wang Y, Kwong DHK, et al. Are different coping styles Mitigating Perceived stress Associated with depressive symptoms among pregnant women? Are different coping styles Mitigating Perceived stress Associated with depressive symptoms among pregnant women?[J]. Perspect Psychiatr Care. 2016;52(2):102–12.
Ding Y, Yang Y, Yang X, et al. The Mediating Role of coping style in the relationship between Psychological Capital and Burnout among Chinese Nurses[J]. PLoS ONE. 2015;10(4):e0122128.
Gandzha IM. [Immune disorders in internal diseases and the ways for their correction][J]. Vrach Delo, 1978(7): 14–9.
Howard S, Creaven AM, Hughes BM, et al. Perceived social support predicts lower cardiovascular reactivity to stress in older adults[J]. Biol Psychol. 2017;125:70–5.
Barrera M. Distinctions between social support concepts, measures, and models[J]. Am J Community Psychol. 1986;14(4):413–45.
Xin M, Yang C, Zhang L, et al. The impact of perceived life stress and online social support on university students’ mental health during the post-COVID era in Northwestern China: gender-specific analysis[J]. BMC Public Health. 2024;24(1):467.
Sun J, Harris K, Vazire S. Is well-being associated with the quantity and quality of social interactions?[J]. J Personal Soc Psychol. 2020;119(6):1478–96.
Wang J, Chen Y, Chen H, et al. The mediating role of coping strategies between depression and social support and the moderating effect of the parent–child relationship in college students returning to school: during the period of the regular prevention and control of COVID-19[J]. Front Psychol. 2023;14:991033.
Ball S, Bax A. Self-care in Medical Education: effectiveness of health-habits interventions for First-Year Medical Students[J]. Acad Med. 2002;77(9):911–7.
Howe A, Smajdor A, Stöckl A. Towards an understanding of resilience and its relevance to medical training[J]. Med Educ. 2012;46(4):349–56.
Louise Duncan D. What the COVID-19 pandemic tells us about the need to develop resilience in the nursing workforce[J]. Nurs Manag. 2020;27(3):22–7.
Google Scholar
Luo Y, Wang H. Correlation research on psychological health impact on nursing students against stress, coping way and social support[J]. Nurse Educ Today. 2009;29(1):5–8.
Luo Mming, Hao M, Li Xhuan, et al. Prevalence of depressive tendencies among college students and the influence of attributional styles on depressive tendencies in the post-pandemic era[J]. Front Public Health. 2024;12:1326582.
Zhang Y, Jia Y, MuLaTiHaJi M, et al. A cross-sectional mental-health survey of Chinese postgraduate students majoring in stomatology post COVID-19 restrictions[J]. Front Public Health. 2024;12:1376540.
Blumenthal JA, Burg MM, Barefoot J, et al. Social support, type A behavior, and coronary artery disease.:[J]. Psychosom Med. 1987;49(4):331–40.
Quintiliani L, Sisto A, Vicinanza F, et al. Resilience and psychological impact on Italian university students during COVID-19 pandemic. Distance learning and health[J]. Volume 27. Psychology, Health & Medicine; 2022. pp. 69–80. 1.
Shao R, He P, Ling B, et al. Prevalence of depression and anxiety and correlations between depression, anxiety, family functioning, social support and coping styles among Chinese medical students[J]. BMC Psychol. 2020;8(1):38.
Riedel B, Horen SR, Reynolds A, et al. Mental Health disorders in Nurses during the COVID-19 pandemic: implications and coping Strategies[J]. Front Public Health. 2021;9:707358.
Faisal-Cury A, Savoia MG, Menezes PR. Coping style and depressive symptomatology during pregnancy in a private setting Sample[J]. Span J Psychol. 2012;15(1):295–305.
Platania GA, Varrasi S, Guerrera CS, et al. Impact of stress during COVID-19 pandemic in Italy: a study on dispositional and behavioral dimensions for supporting evidence-based targeted Strategies[J]. Int J Environ Res Public Health. 2024;21(3):330.
Xu Y, Zheng Q, Jiang X, et al. Effects of coping on nurses’ mental health during the COVID-19 pandemic: mediating role of social support and psychological resilience[J]. Nurs Open. 2023;10(7):4619–29.
Kassam S. Understanding experiences of Social Support as Coping resources among immigrant and Refugee women with Postpartum Depression: an Integrative Literature Review[J]. Issues Ment Health Nurs. 2019;40(12):999–1011.
Xu J, Wei Y. Social Support as a moderator of the relationship between anxiety and depression: an empirical study with adult survivors of Wenchuan Earthquake[J]. PLoS ONE. 2013;8(10):e79045.
Luthar SS, Cicchetti D, Becker B. The Construct of Resilience: a critical evaluation and guidelines for future Work[J]. Child Dev. 2000;71(3):543–62.
Lee SH, Cho SJ. Cognitive Behavioral Therapy and Mindfulness-Based Cognitive Therapy for Depressive Disorders[M]//, Kim YK. Major Depressive Disorder: Vol. 1305. Singapore: Springer Singapore, 2021: 295–310.
Thompson G, McBride RB, Hosford CC, et al. Resilience among medical students: the role of coping style and social Support[J]. Teach Learn Med. 2016;28(2):174–82.
Murphy J, McGrane B, White RL, et al. Self-Esteem, meaningful experiences and the Rocky Road—contexts of physical activity that impact Mental Health in Adolescents[J]. Int J Environ Res Public Health. 2022;19(23):15846.
Download references
Acknowledgements
We would like to provide our extreme thanks and appreciation to all students who participated in our study.
This work was financially supported by the National Food Safety Risk Center Joint Research Program [grant number (LH2022GG06)] and the Weifang Medical College Teaching Reform Program (2023YBC008).
Author information
Authors and affiliations.
School of Public Health, Shandong Second Medical University, No. 7166, Baotong West Street, Weicheng District, Weifang City, 261053, China
Shihong Dong, Huaiju Ge, Wenyu Su, Weimin Guan, Xinquan Li, Qing Yu & Guifeng Ma
Shandong Cancer Research Institute (Shandong Tumor Hospital), No.440, Jiyan Road, Huaiyin District, Jinan, 250117, China
Yan Liu & Yuantao Qi
The First Affiliated Hospital of Shandong Second Medical University (Weifang People’s Hospital), No.151 Guangwen Street, Weicheng District, Weifang City, 261041, China
Huiqing Zhang
You can also search for this author in PubMed Google Scholar
Contributions
SD and GM conceived and designed the study. HG, WS, WG, and YL undertook the data collection and analysis. SD, QY, YQ, XLand HZ drafted the manuscript. SD and GM reviewed the manuscript. The authors read and approved the final manuscript.
Corresponding authors
Correspondence to Huiqing Zhang or Guifeng Ma .
Ethics declarations
Ethics approval and consent to participate.
In accordance with the Declaration of Helsinki, the study was approved by the Ethical Committee of Shandong Second Medical University and written informed consent was required from all participants. Participation was voluntary and students were informed about the purpose of the study. Confidentiality was assured and questionnaires were submitted anonymously.
Consent for publication
Not Applicable.
Competing interests
The authors declare no competing interests.
Additional information
Publisher’s note.
Springer Nature remains neutral with regard to jurisdictional claims in published maps and institutional affiliations.
Rights and permissions
Open Access This article is licensed under a Creative Commons Attribution 4.0 International License, which permits use, sharing, adaptation, distribution and reproduction in any medium or format, as long as you give appropriate credit to the original author(s) and the source, provide a link to the Creative Commons licence, and indicate if changes were made. The images or other third party material in this article are included in the article’s Creative Commons licence, unless indicated otherwise in a credit line to the material. If material is not included in the article’s Creative Commons licence and your intended use is not permitted by statutory regulation or exceeds the permitted use, you will need to obtain permission directly from the copyright holder. To view a copy of this licence, visit http://creativecommons.org/licenses/by/4.0/ . The Creative Commons Public Domain Dedication waiver ( http://creativecommons.org/publicdomain/zero/1.0/ ) applies to the data made available in this article, unless otherwise stated in a credit line to the data.
Reprints and permissions
About this article
Cite this article.
Dong, S., Ge, H., Su, W. et al. Enhancing psychological well-being in college students: the mediating role of perceived social support and resilience in coping styles. BMC Psychol 12 , 393 (2024). https://doi.org/10.1186/s40359-024-01902-7
Download citation
Received : 04 March 2024
Accepted : 12 July 2024
Published : 15 July 2024
DOI : https://doi.org/10.1186/s40359-024-01902-7
Share this article
Anyone you share the following link with will be able to read this content:
Sorry, a shareable link is not currently available for this article.
Provided by the Springer Nature SharedIt content-sharing initiative
- College students
- Perceived social support
BMC Psychology
ISSN: 2050-7283
- General enquiries: [email protected]
- Helldivers 2
- Dragon’s Dogma 2
- Wuthering Waves
- Genshin Impact
- Counter Strike 2
- Honkai Star Rail
- Lego Fortnite
- Stardew Valley
- NYT Strands
- NYT Connections
- Apple Watch
- Crunchyroll
- Prime Video
- Jujutsu Kaisen
- Demon Slayer
- Chainsaw Man
- Solo Leveling
- Beebom Gadgets
10 Best AI Tools for Research: Consensus, Scite, Elicit & More
1. Consensus
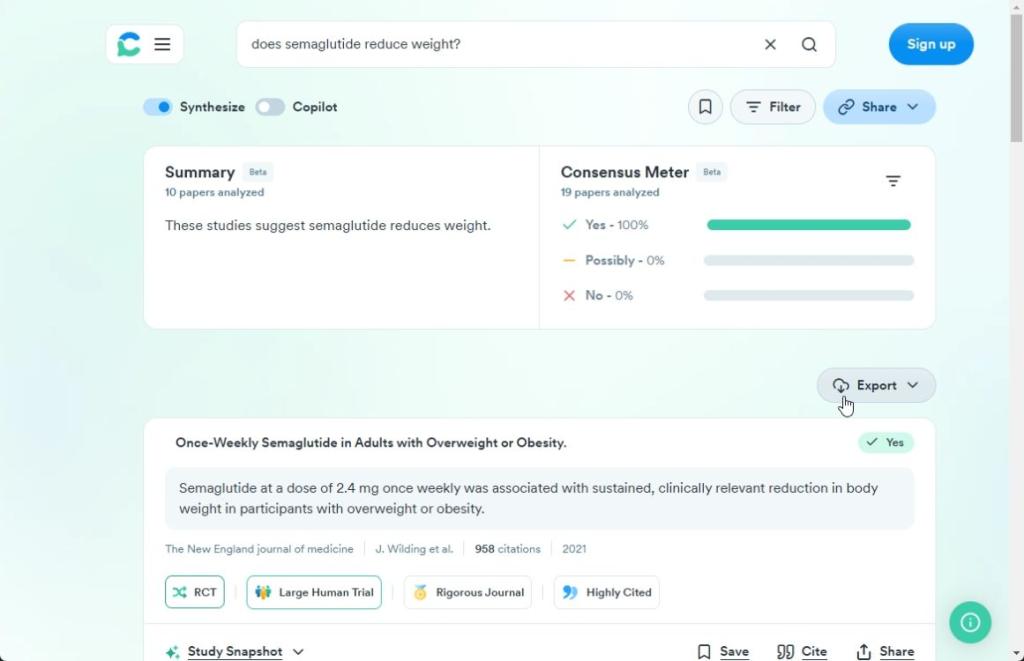
Scite is another powerful AI-powered tool that is ideal for students and researchers. It evaluates scientific studies to answer your questions, backed by real research and citations. Scite is widely used by universities, publishers, and corporations across the world. The feature called Smart Citations adds context to each citation and checks references to provide reliability of studies.
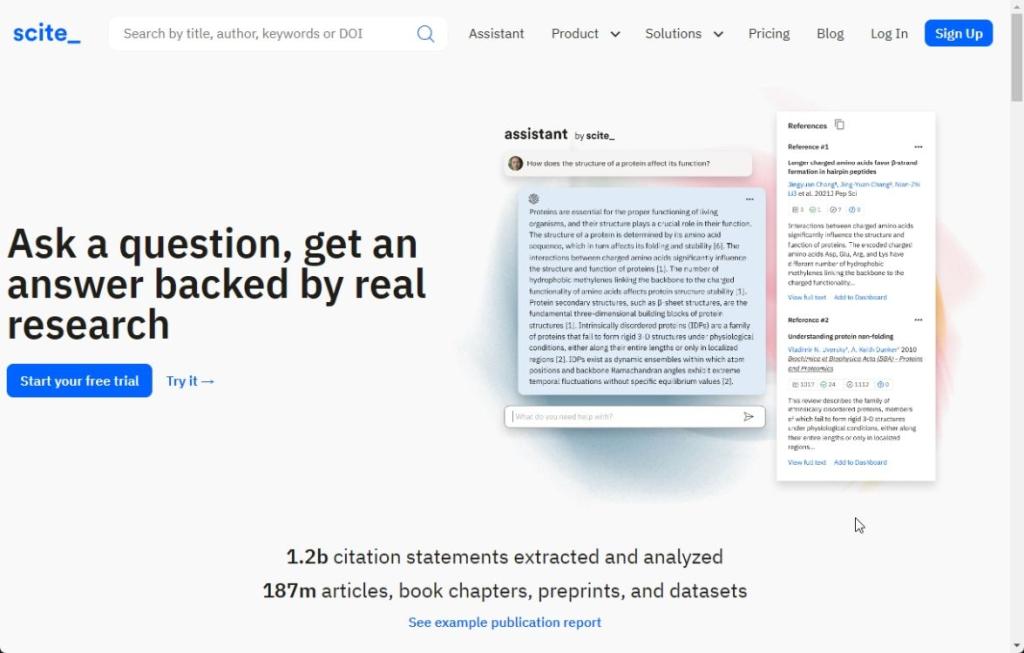
3. SciSpace
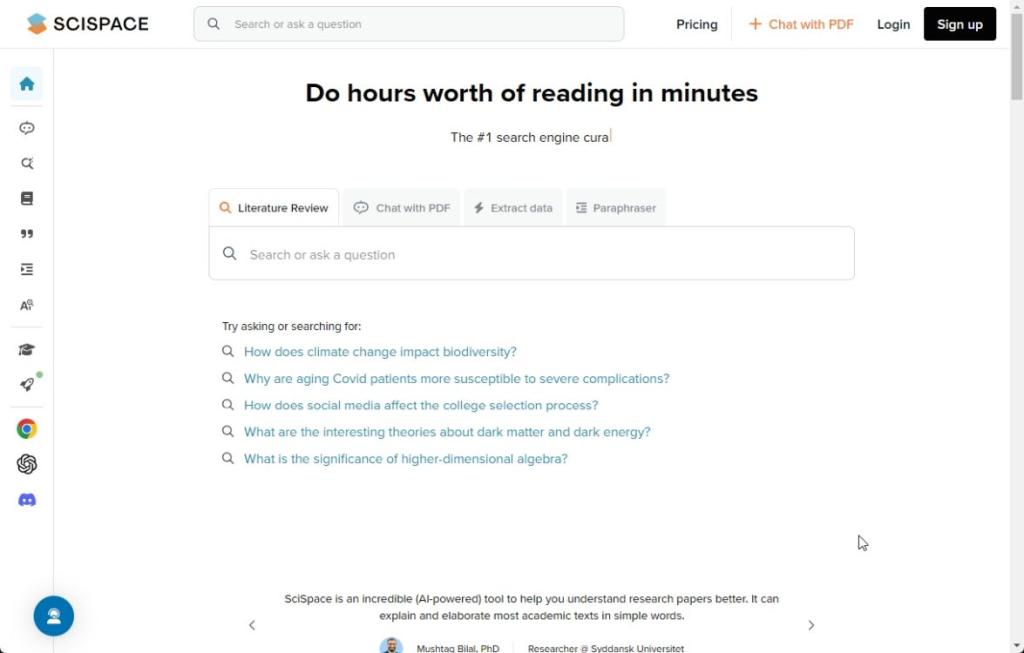
4. Semantic Scholar
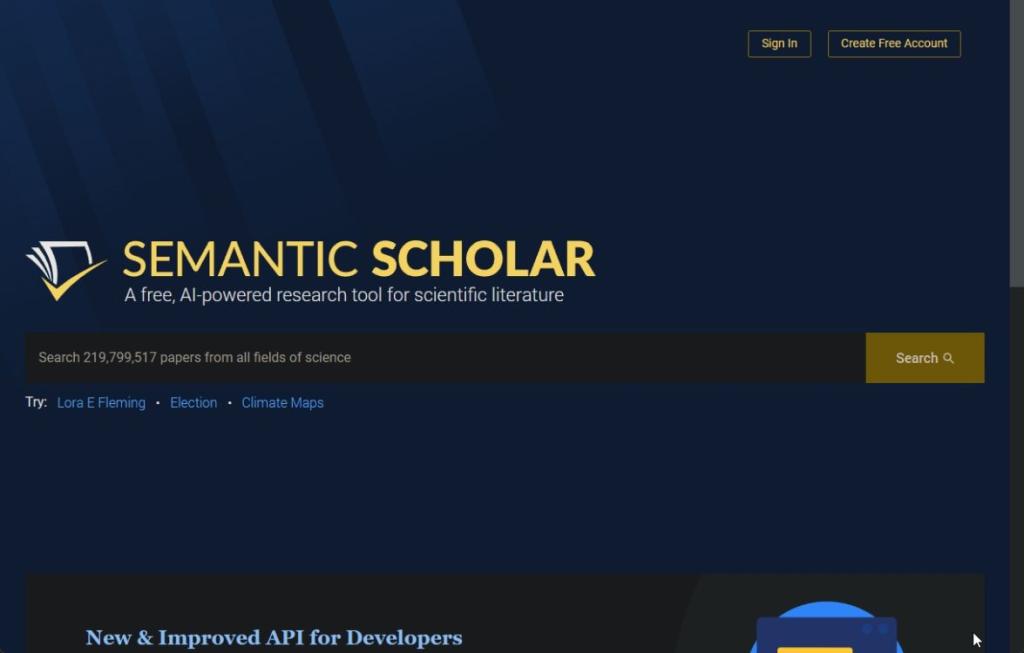
Furthermore, you can check out all the references and move to the DOI link to find the paper. The standout feature of Semantic Scholar is that it can perform advanced citation analysis across its large library of databases to provide relevant papers. If you want to discover scientific literature, Semantic Scholar is a great free AI tool.
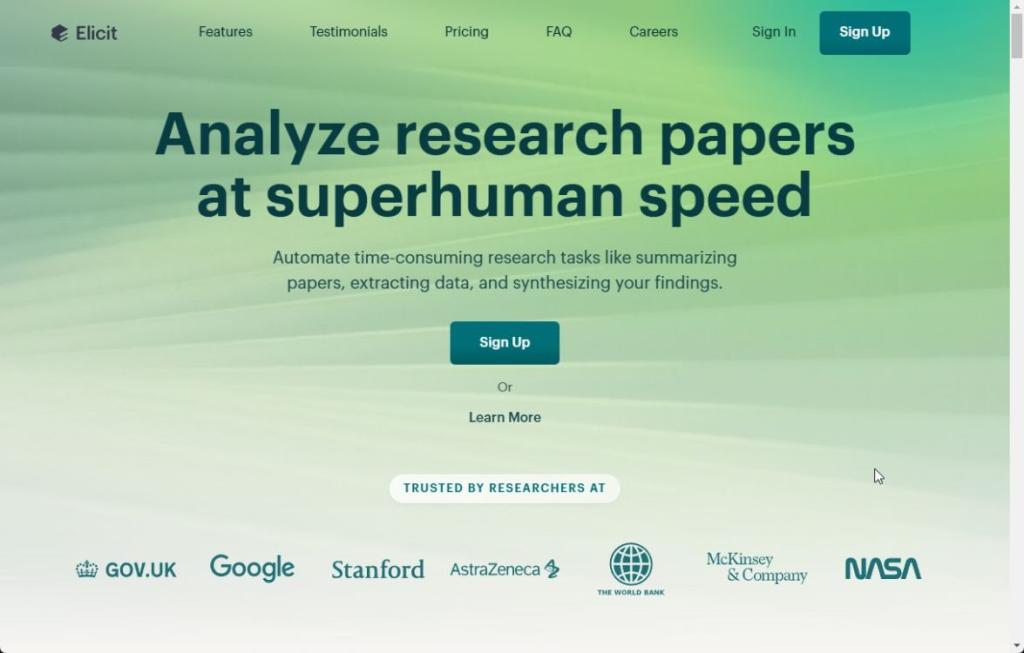
6. ScholarGPT
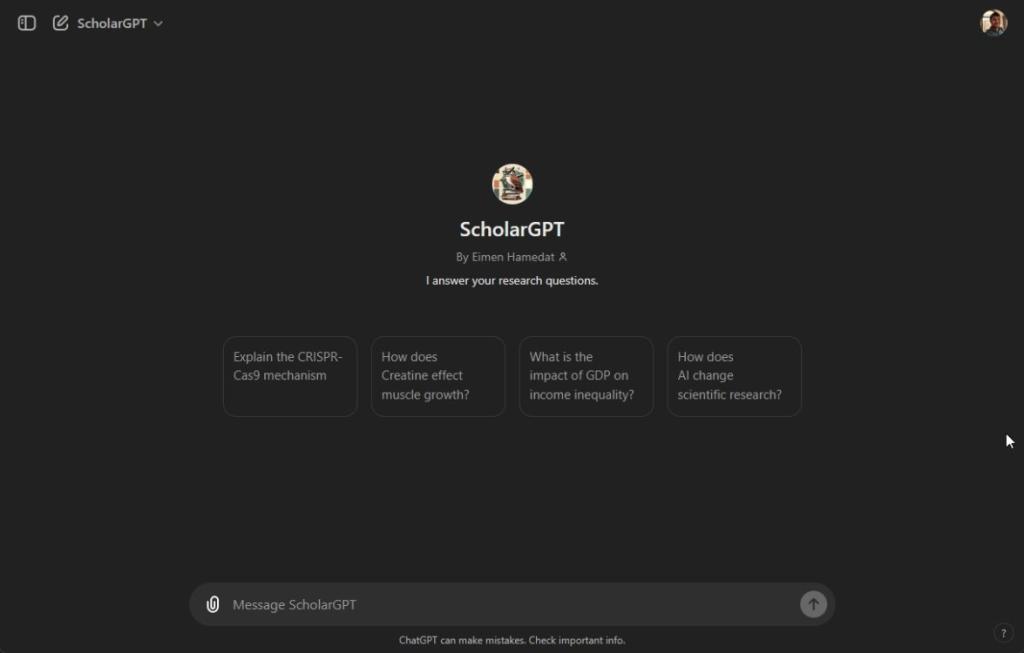
And now that OpenAI has opened access to GPTs for all, free ChatGPT users can also chat with ScholarGPT or any other GPT without subscribing to the paid plan. So to find answers to your research questions, you must check out ScholarGPT.
7. Coral AI
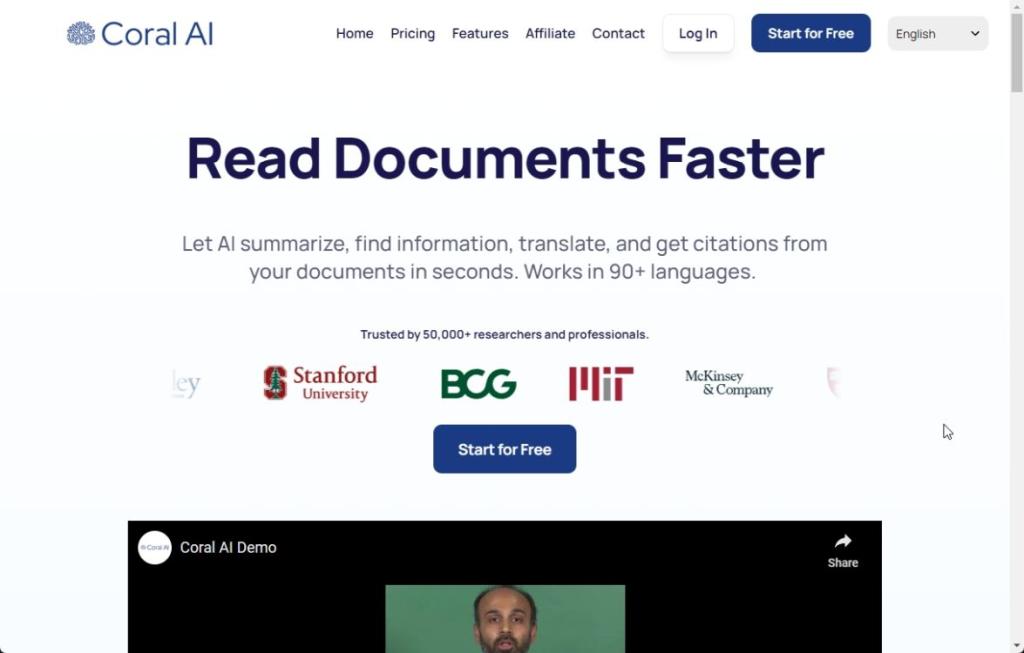
8. ResearchRabbit
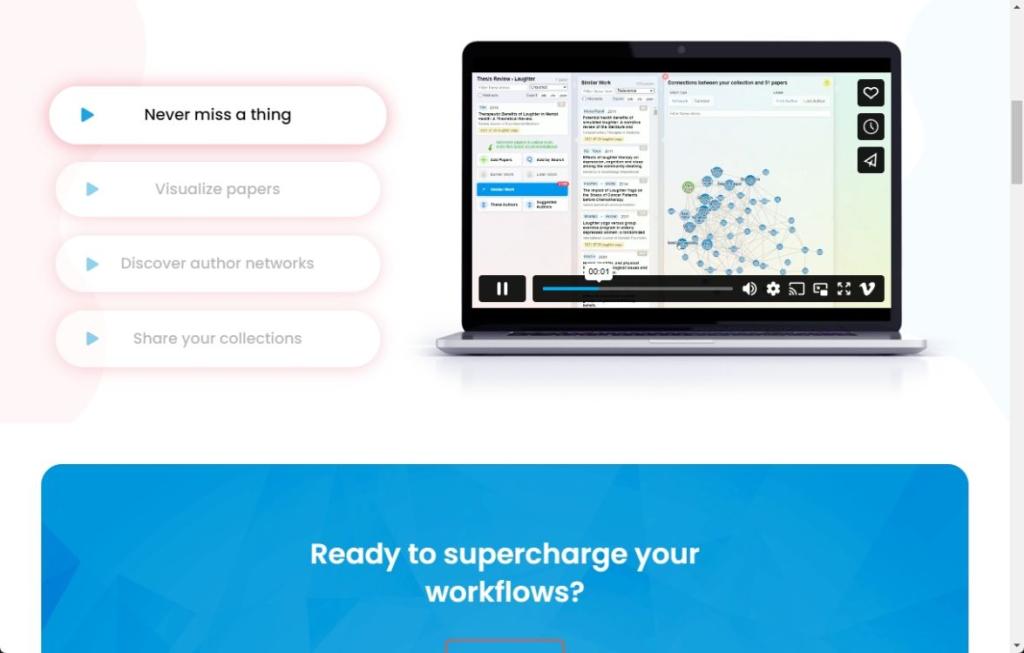
9. Connected Papers
Connected Papers is not an AI tool per se, but it’s incredibly useful for researchers. It helps students discover new and relevant academic papers that might be under the radar. This is done through a visual graph of papers, showing a starting point and connections based on similarity.
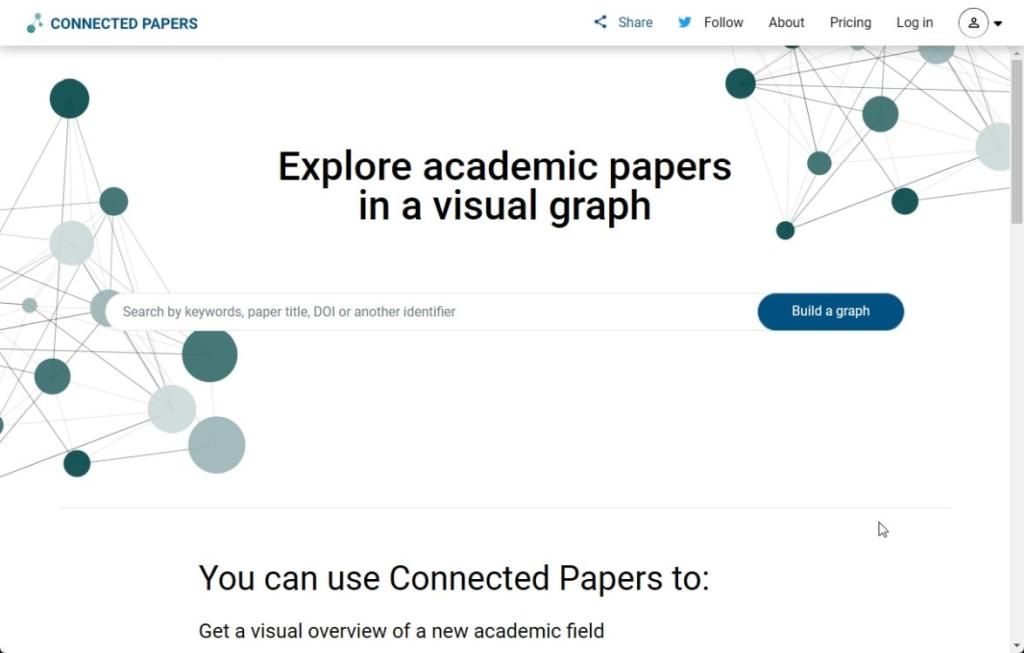
10. ChatGPT and Perplexity
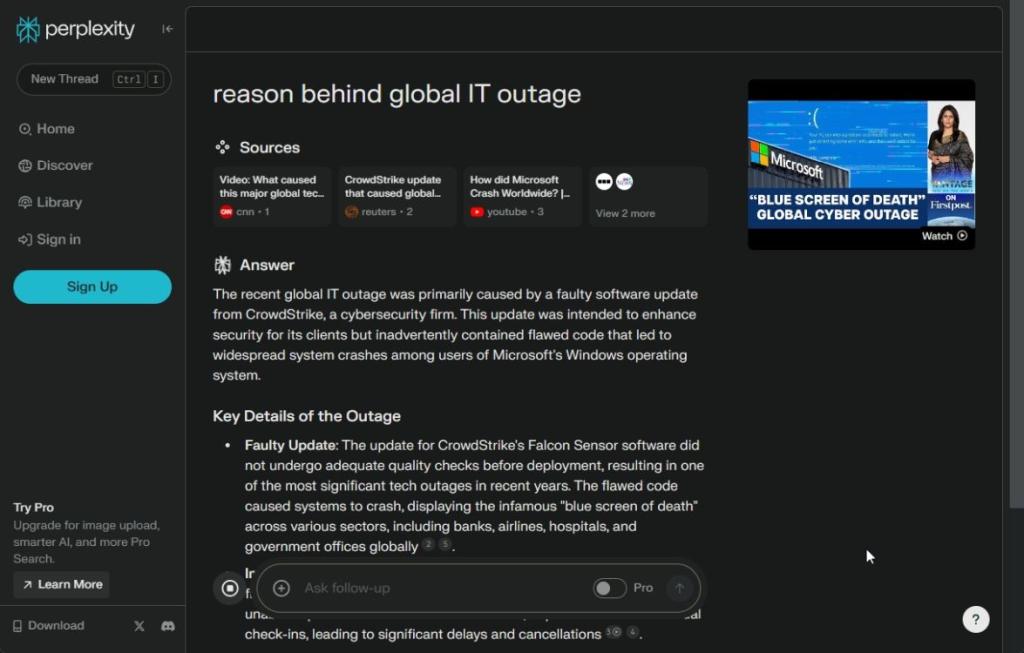
Passionate about Windows, ChromeOS, Android, security and privacy issues. Have a penchant to solve everyday computing problems.
Add new comment
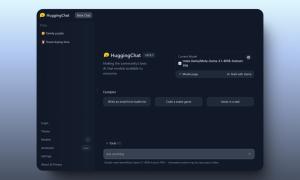
The state of AI in early 2024: Gen AI adoption spikes and starts to generate value
If 2023 was the year the world discovered generative AI (gen AI) , 2024 is the year organizations truly began using—and deriving business value from—this new technology. In the latest McKinsey Global Survey on AI, 65 percent of respondents report that their organizations are regularly using gen AI, nearly double the percentage from our previous survey just ten months ago. Respondents’ expectations for gen AI’s impact remain as high as they were last year , with three-quarters predicting that gen AI will lead to significant or disruptive change in their industries in the years ahead.
About the authors
This article is a collaborative effort by Alex Singla , Alexander Sukharevsky , Lareina Yee , and Michael Chui , with Bryce Hall , representing views from QuantumBlack, AI by McKinsey, and McKinsey Digital.
Organizations are already seeing material benefits from gen AI use, reporting both cost decreases and revenue jumps in the business units deploying the technology. The survey also provides insights into the kinds of risks presented by gen AI—most notably, inaccuracy—as well as the emerging practices of top performers to mitigate those challenges and capture value.
AI adoption surges
Interest in generative AI has also brightened the spotlight on a broader set of AI capabilities. For the past six years, AI adoption by respondents’ organizations has hovered at about 50 percent. This year, the survey finds that adoption has jumped to 72 percent (Exhibit 1). And the interest is truly global in scope. Our 2023 survey found that AI adoption did not reach 66 percent in any region; however, this year more than two-thirds of respondents in nearly every region say their organizations are using AI. 1 Organizations based in Central and South America are the exception, with 58 percent of respondents working for organizations based in Central and South America reporting AI adoption. Looking by industry, the biggest increase in adoption can be found in professional services. 2 Includes respondents working for organizations focused on human resources, legal services, management consulting, market research, R&D, tax preparation, and training.
Also, responses suggest that companies are now using AI in more parts of the business. Half of respondents say their organizations have adopted AI in two or more business functions, up from less than a third of respondents in 2023 (Exhibit 2).

Future frontiers: Navigating the next wave of tech innovations
Join Lareina Yee and Roger Roberts on Tuesday, July 30, at 12:30 p.m. EDT/6:30 p.m. CET as they discuss the future of these technological trends, the factors that will fuel their growth, and strategies for investing in them through 2024 and beyond.
Gen AI adoption is most common in the functions where it can create the most value
Most respondents now report that their organizations—and they as individuals—are using gen AI. Sixty-five percent of respondents say their organizations are regularly using gen AI in at least one business function, up from one-third last year. The average organization using gen AI is doing so in two functions, most often in marketing and sales and in product and service development—two functions in which previous research determined that gen AI adoption could generate the most value 3 “ The economic potential of generative AI: The next productivity frontier ,” McKinsey, June 14, 2023. —as well as in IT (Exhibit 3). The biggest increase from 2023 is found in marketing and sales, where reported adoption has more than doubled. Yet across functions, only two use cases, both within marketing and sales, are reported by 15 percent or more of respondents.
Gen AI also is weaving its way into respondents’ personal lives. Compared with 2023, respondents are much more likely to be using gen AI at work and even more likely to be using gen AI both at work and in their personal lives (Exhibit 4). The survey finds upticks in gen AI use across all regions, with the largest increases in Asia–Pacific and Greater China. Respondents at the highest seniority levels, meanwhile, show larger jumps in the use of gen Al tools for work and outside of work compared with their midlevel-management peers. Looking at specific industries, respondents working in energy and materials and in professional services report the largest increase in gen AI use.
Investments in gen AI and analytical AI are beginning to create value
The latest survey also shows how different industries are budgeting for gen AI. Responses suggest that, in many industries, organizations are about equally as likely to be investing more than 5 percent of their digital budgets in gen AI as they are in nongenerative, analytical-AI solutions (Exhibit 5). Yet in most industries, larger shares of respondents report that their organizations spend more than 20 percent on analytical AI than on gen AI. Looking ahead, most respondents—67 percent—expect their organizations to invest more in AI over the next three years.
Where are those investments paying off? For the first time, our latest survey explored the value created by gen AI use by business function. The function in which the largest share of respondents report seeing cost decreases is human resources. Respondents most commonly report meaningful revenue increases (of more than 5 percent) in supply chain and inventory management (Exhibit 6). For analytical AI, respondents most often report seeing cost benefits in service operations—in line with what we found last year —as well as meaningful revenue increases from AI use in marketing and sales.
Inaccuracy: The most recognized and experienced risk of gen AI use
As businesses begin to see the benefits of gen AI, they’re also recognizing the diverse risks associated with the technology. These can range from data management risks such as data privacy, bias, or intellectual property (IP) infringement to model management risks, which tend to focus on inaccurate output or lack of explainability. A third big risk category is security and incorrect use.
Respondents to the latest survey are more likely than they were last year to say their organizations consider inaccuracy and IP infringement to be relevant to their use of gen AI, and about half continue to view cybersecurity as a risk (Exhibit 7).
Conversely, respondents are less likely than they were last year to say their organizations consider workforce and labor displacement to be relevant risks and are not increasing efforts to mitigate them.
In fact, inaccuracy— which can affect use cases across the gen AI value chain , ranging from customer journeys and summarization to coding and creative content—is the only risk that respondents are significantly more likely than last year to say their organizations are actively working to mitigate.
Some organizations have already experienced negative consequences from the use of gen AI, with 44 percent of respondents saying their organizations have experienced at least one consequence (Exhibit 8). Respondents most often report inaccuracy as a risk that has affected their organizations, followed by cybersecurity and explainability.
Our previous research has found that there are several elements of governance that can help in scaling gen AI use responsibly, yet few respondents report having these risk-related practices in place. 4 “ Implementing generative AI with speed and safety ,” McKinsey Quarterly , March 13, 2024. For example, just 18 percent say their organizations have an enterprise-wide council or board with the authority to make decisions involving responsible AI governance, and only one-third say gen AI risk awareness and risk mitigation controls are required skill sets for technical talent.
Bringing gen AI capabilities to bear
The latest survey also sought to understand how, and how quickly, organizations are deploying these new gen AI tools. We have found three archetypes for implementing gen AI solutions : takers use off-the-shelf, publicly available solutions; shapers customize those tools with proprietary data and systems; and makers develop their own foundation models from scratch. 5 “ Technology’s generational moment with generative AI: A CIO and CTO guide ,” McKinsey, July 11, 2023. Across most industries, the survey results suggest that organizations are finding off-the-shelf offerings applicable to their business needs—though many are pursuing opportunities to customize models or even develop their own (Exhibit 9). About half of reported gen AI uses within respondents’ business functions are utilizing off-the-shelf, publicly available models or tools, with little or no customization. Respondents in energy and materials, technology, and media and telecommunications are more likely to report significant customization or tuning of publicly available models or developing their own proprietary models to address specific business needs.
Respondents most often report that their organizations required one to four months from the start of a project to put gen AI into production, though the time it takes varies by business function (Exhibit 10). It also depends upon the approach for acquiring those capabilities. Not surprisingly, reported uses of highly customized or proprietary models are 1.5 times more likely than off-the-shelf, publicly available models to take five months or more to implement.
Gen AI high performers are excelling despite facing challenges
Gen AI is a new technology, and organizations are still early in the journey of pursuing its opportunities and scaling it across functions. So it’s little surprise that only a small subset of respondents (46 out of 876) report that a meaningful share of their organizations’ EBIT can be attributed to their deployment of gen AI. Still, these gen AI leaders are worth examining closely. These, after all, are the early movers, who already attribute more than 10 percent of their organizations’ EBIT to their use of gen AI. Forty-two percent of these high performers say more than 20 percent of their EBIT is attributable to their use of nongenerative, analytical AI, and they span industries and regions—though most are at organizations with less than $1 billion in annual revenue. The AI-related practices at these organizations can offer guidance to those looking to create value from gen AI adoption at their own organizations.
To start, gen AI high performers are using gen AI in more business functions—an average of three functions, while others average two. They, like other organizations, are most likely to use gen AI in marketing and sales and product or service development, but they’re much more likely than others to use gen AI solutions in risk, legal, and compliance; in strategy and corporate finance; and in supply chain and inventory management. They’re more than three times as likely as others to be using gen AI in activities ranging from processing of accounting documents and risk assessment to R&D testing and pricing and promotions. While, overall, about half of reported gen AI applications within business functions are utilizing publicly available models or tools, gen AI high performers are less likely to use those off-the-shelf options than to either implement significantly customized versions of those tools or to develop their own proprietary foundation models.
What else are these high performers doing differently? For one thing, they are paying more attention to gen-AI-related risks. Perhaps because they are further along on their journeys, they are more likely than others to say their organizations have experienced every negative consequence from gen AI we asked about, from cybersecurity and personal privacy to explainability and IP infringement. Given that, they are more likely than others to report that their organizations consider those risks, as well as regulatory compliance, environmental impacts, and political stability, to be relevant to their gen AI use, and they say they take steps to mitigate more risks than others do.
Gen AI high performers are also much more likely to say their organizations follow a set of risk-related best practices (Exhibit 11). For example, they are nearly twice as likely as others to involve the legal function and embed risk reviews early on in the development of gen AI solutions—that is, to “ shift left .” They’re also much more likely than others to employ a wide range of other best practices, from strategy-related practices to those related to scaling.
In addition to experiencing the risks of gen AI adoption, high performers have encountered other challenges that can serve as warnings to others (Exhibit 12). Seventy percent say they have experienced difficulties with data, including defining processes for data governance, developing the ability to quickly integrate data into AI models, and an insufficient amount of training data, highlighting the essential role that data play in capturing value. High performers are also more likely than others to report experiencing challenges with their operating models, such as implementing agile ways of working and effective sprint performance management.
About the research
The online survey was in the field from February 22 to March 5, 2024, and garnered responses from 1,363 participants representing the full range of regions, industries, company sizes, functional specialties, and tenures. Of those respondents, 981 said their organizations had adopted AI in at least one business function, and 878 said their organizations were regularly using gen AI in at least one function. To adjust for differences in response rates, the data are weighted by the contribution of each respondent’s nation to global GDP.
Alex Singla and Alexander Sukharevsky are global coleaders of QuantumBlack, AI by McKinsey, and senior partners in McKinsey’s Chicago and London offices, respectively; Lareina Yee is a senior partner in the Bay Area office, where Michael Chui , a McKinsey Global Institute partner, is a partner; and Bryce Hall is an associate partner in the Washington, DC, office.
They wish to thank Kaitlin Noe, Larry Kanter, Mallika Jhamb, and Shinjini Srivastava for their contributions to this work.
This article was edited by Heather Hanselman, a senior editor in McKinsey’s Atlanta office.
Explore a career with us
Related articles.

Moving past gen AI’s honeymoon phase: Seven hard truths for CIOs to get from pilot to scale
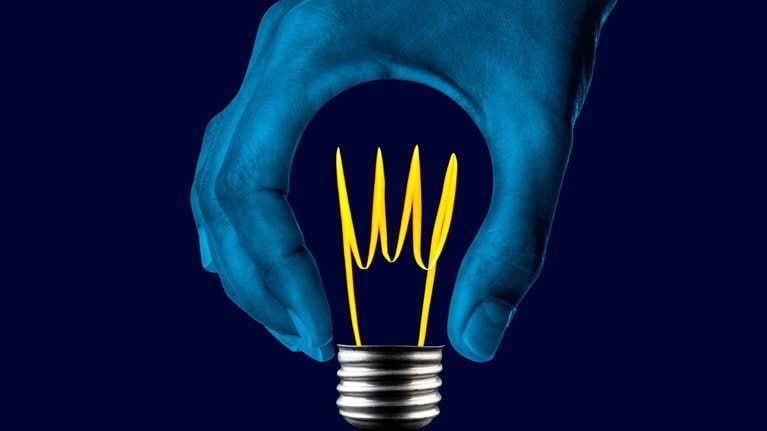
A generative AI reset: Rewiring to turn potential into value in 2024

Implementing generative AI with speed and safety
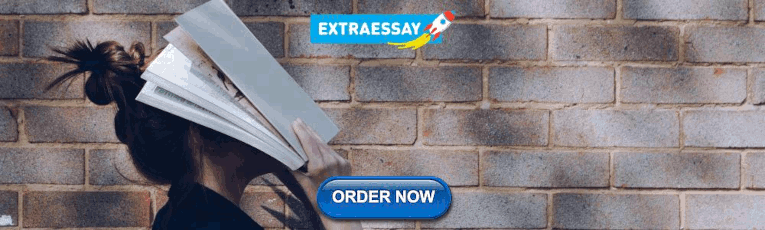
IMAGES
VIDEO
COMMENTS
Step 2. Create your table. All word processing programs include an option to create a table. For example, in Word's top menu bar you can either click on the "Table" tab or select Insert -> Table -> New. To keep your tables consistent, it's important that you use the same formatting throughout your dissertation.
But while well-presented tables and figures in research papers can efficiently capture and present information, poorly crafted tables and figures can confuse readers and impair the effectiveness of a paper. 16 To help authors get the balance right, this article presents some essential guidelines to the effective use of tables and figures in ...
How to Create Tables in Research Paper. Here are the steps to create tables in a research paper: Plan your table: Determine the purpose of the table and the type of information you want to include. Consider the layout and format that will best convey your information. Choose a table format: Decide on the type of table you want to create.
So, the tables need to be well organized and self-explanatory. Avoidance of repetition: Tables and figures add clarity to the research. They complement the research text and draw attention to key points. They can be used to highlight the main points of the paper, but values should not be repeated as it defeats the very purpose of these elements.
It will be appropriate to indicate other demographic numerical details in tables or figures. As an example elucidating the abovementioned topics a research paper written by the authors of this review article, and published in the Turkish Journal of Urology in the year 2007 (Türk Üroloji Dergisi 2007;33:18-23) is presented below:
Do not use suffix letters (e.g. Table 3a, 3b, 3c); instead, combine the related tables. If the manuscript includes an appendix with tables, identify them with capital letters and Arabic numerals (e.g. Table A1, Table B2). Titles. Like the title of the paper itself, each table must have a clear and concise title.
How to make tables for research paper? "To create tables for a research paper, follow these steps: 1) Determine the purpose and information to be conveyed. 2) Plan the layout, including rows, columns, and headings. 3) Use spreadsheet software like Excel to design and format the table. 4) Input accurate data into cells, aligning it logically.
Figures and tables. Figures and tables (display items) are often the quickest way to communicate large amounts of complex information that would be complicated to explain in text. Many readers will only look at your display items without reading the main text of your manuscript. Therefore, ensure your display items can stand alone from the text ...
Background Tables are often overlooked by many readers of papers who tend to focus on the text. Good tables tell much of the story of a paper and give a richer insight into the details of the study participants and the main research findings. Being confident in reading tables and constructing clear tables are important skills for researchers to master. Method Common forms of tables were ...
Researchers often use tables and figures in their research paper as visual representations to convey data in a simple way. Tables and figures in research papers not only enable readers to understand complex data at a glance but they also help create better engagement in one's research. Instead of having to wade through dense paragraphs of ...
Research papers are full of data and other information that needs to be effectively illustrated and organized. Without a clear presentation of a study's data, the information will not reach the intended audience and could easily be misunderstood. Clarity of thought and purpose is essential for any kind of research. Using tables and figures to present findings and other data in a research paper ...
Tables are used to organize data that is too detailed or complicated to be described adequately in the text, allowing the reader to quickly see the results. They can be used to highlight trends or patterns in the data and to make a manuscript more readable by removing numeric data from the text. Tables can also be used to synthesize existing ...
To do this, follow these steps: Navigate to the References tab, and click "Insert Caption," which you can find in the Captions group. Give your caption a name. In the Label list, you can select the label that best describes your figure or table, or make your own by selecting "New Label.". Next, you can insert the list of tables and ...
The rules for the use of tables and graphs in abstracts (Table 1) are different from the rules for their insertion in a full report published in a journal, where space is less limited. In contrast to abstracts, in a full manuscript in a journal, multiple illustrations should be used and can be expanded. Tables, graphs, and figures can be used ...
This is a standard way to use figures and tables in your research paper. 3. Best Practices for Using Tables & Figures. When you are using figures and tables in your paper, you must reference them in your text. You cannot have a figure in your paper and not talk about it in your text. Best practices
To help authors get the balance right, this article presents some essential guidelines to the effective use of tables and figures in research papers. Planning your paper: When to use tables and figures in scientific papers . Producing effective tables and figures requires careful planning that begins at the manuscript writing stage itself.
The following pointers will help you prepare clear, effective tables: 1. Ensure that the title of your table is concise and informative. A lengthy title that does not clearly indicate what your table is about will distract your reader. The titles of a table's columns should also be clear such that they can be viewed without referring to the text.
1. Learn your target journal's requirements for preparing and presenting visual elements, e.g., how many tables and figures you can include or if there are specific design-related guidelines. 2. Check whether your data can be presented as text. Use tables and figures if your data is large or complex, or if you need to show trends or patterns ...
The purpose of using tables in research papers is to organize and present data in a manner that is easy to understand and interpret. A table is a way of arranging data in rows and columns, allowing the reader to quickly identify patterns and trends within the data. It can be used to compare different results or to present large amounts of ...
Introduction. Every author aims to reach the maximum target audience through his/her research publication/s. Our previous editorials have touched upon the process of writing a quality research paper and its successful publication in an appropriate journal.[1,2] Journal-specific "Instructions for Authors" generally have defined limits to the text and non-textual content for the benefit of ...
May 31, 2022. Dissertations. In research papers, tables and figures can be helpful and even necessary to support your argument or to present a clearer, fuller picture of your topic. Creating effective tables and figures is not a simple task, however, and using a table or figure made by another author has intellectual property considerations.
We used a random sample of 16 million patients (2006-2020) from the PharMetrics Plus for Academics database (IQVIA), a large health claims database that captures 93% of all outpatient prescriptions and physician diagnoses in the US through the International Classification of Diseases, Ninth Revision (ICD-9) or ICD-10. In our cohort study, we included new users of semaglutide or liraglutide, 2 ...
These brief, peer-reviewed articles complement full research papers and are an easy way to receive proper credit and recognition for the work you have done. Research elements are research outputs that have come about as a result of following the research cycle - this includes things like data, methods and protocols, software, hardware and more.
Integration of phylogenetics, comparative genomics and palaeobiological approaches suggests that the last universal common ancestor lived about 4.2 billion years ago and was a complex prokaryote ...
This work introduces a task-agnostic, meta-learning approach, which enables the incremental addition of new defect classes and scales to create a more robust and generalized model for semiconductor defect inspection. Deep learning-based semiconductor defect inspection has gained traction in recent years, offering a powerful and versatile approach that provides high accuracy, adaptability, and ...
To investigate the effect of different length-diameter ratios of cylindrical tanks on the roll stability of tank semi-trailers, this paper uses an equivalent mechanical pendulum model to simulate liquid sloshing in the tank.
The prevalence of depression among college students is higher than that of the general population. Although a growing body of research suggests that depression in college students and their potential risk factors, few studies have focused on the correlation between depression and risk factors. This study aims to explore the mediating role of perceived social support and resilience in the ...
In academic research, the process of literature review takes up a lot of time. Elicit is one such AI tool for research that automates summarizing papers, extracting data, and evaluating new findings seamlessly. You can search, ask research questions, and get relevant papers from its massive library of 125 million research papers.
If 2023 was the year the world discovered generative AI (gen AI), 2024 is the year organizations truly began using—and deriving business value from—this new technology. ... About the research. The online survey was in the field from February 22 to March 5, 2024, and garnered responses from 1,363 participants representing the full range of ...
The flow field of the low reactivity fuel at the inlet manifold is analysed using the Computational Fluid Dynamics principle, which is used to determine the best flow rate for improving combustion quality. ... Journal of Mechanical Engineering Research & Developments (JMERD) 2019; 42(1): 104-108. ... Figures and tables Figures & Media Tables ...