Have a language expert improve your writing
Run a free plagiarism check in 10 minutes, generate accurate citations for free.
- Knowledge Base
Methodology
- What is Secondary Research? | Definition, Types, & Examples
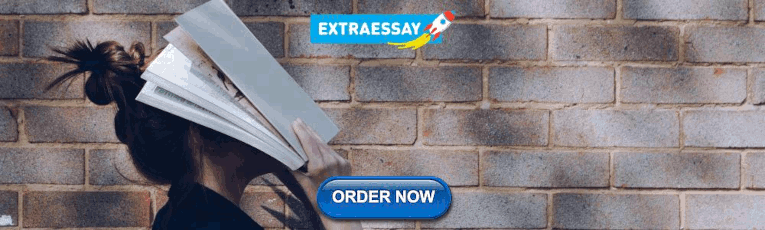
What is Secondary Research? | Definition, Types, & Examples
Published on January 20, 2023 by Tegan George . Revised on January 12, 2024.
Secondary research is a research method that uses data that was collected by someone else. In other words, whenever you conduct research using data that already exists, you are conducting secondary research. On the other hand, any type of research that you undertake yourself is called primary research .
Secondary research can be qualitative or quantitative in nature. It often uses data gathered from published peer-reviewed papers, meta-analyses, or government or private sector databases and datasets.
Table of contents
When to use secondary research, types of secondary research, examples of secondary research, advantages and disadvantages of secondary research, other interesting articles, frequently asked questions.
Secondary research is a very common research method, used in lieu of collecting your own primary data. It is often used in research designs or as a way to start your research process if you plan to conduct primary research later on.
Since it is often inexpensive or free to access, secondary research is a low-stakes way to determine if further primary research is needed, as gaps in secondary research are a strong indication that primary research is necessary. For this reason, while secondary research can theoretically be exploratory or explanatory in nature, it is usually explanatory: aiming to explain the causes and consequences of a well-defined problem.
Prevent plagiarism. Run a free check.
Secondary research can take many forms, but the most common types are:
Statistical analysis
Literature reviews, case studies, content analysis.
There is ample data available online from a variety of sources, often in the form of datasets. These datasets are often open-source or downloadable at a low cost, and are ideal for conducting statistical analyses such as hypothesis testing or regression analysis .
Credible sources for existing data include:
- The government
- Government agencies
- Non-governmental organizations
- Educational institutions
- Businesses or consultancies
- Libraries or archives
- Newspapers, academic journals, or magazines
A literature review is a survey of preexisting scholarly sources on your topic. It provides an overview of current knowledge, allowing you to identify relevant themes, debates, and gaps in the research you analyze. You can later apply these to your own work, or use them as a jumping-off point to conduct primary research of your own.
Structured much like a regular academic paper (with a clear introduction, body, and conclusion), a literature review is a great way to evaluate the current state of research and demonstrate your knowledge of the scholarly debates around your topic.
A case study is a detailed study of a specific subject. It is usually qualitative in nature and can focus on a person, group, place, event, organization, or phenomenon. A case study is a great way to utilize existing research to gain concrete, contextual, and in-depth knowledge about your real-world subject.
You can choose to focus on just one complex case, exploring a single subject in great detail, or examine multiple cases if you’d prefer to compare different aspects of your topic. Preexisting interviews , observational studies , or other sources of primary data make for great case studies.
Content analysis is a research method that studies patterns in recorded communication by utilizing existing texts. It can be either quantitative or qualitative in nature, depending on whether you choose to analyze countable or measurable patterns, or more interpretive ones. Content analysis is popular in communication studies, but it is also widely used in historical analysis, anthropology, and psychology to make more semantic qualitative inferences.
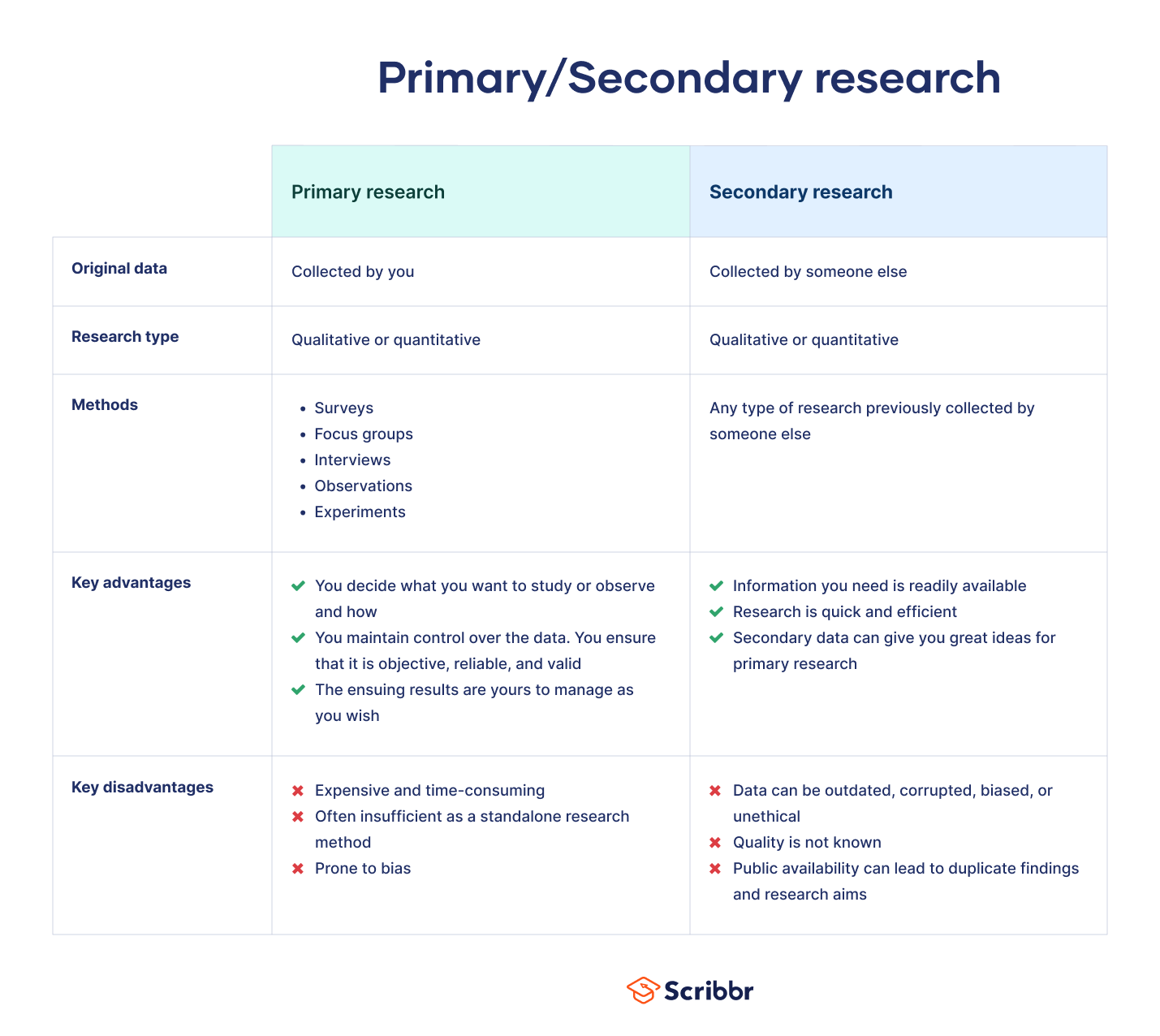
Secondary research is a broad research approach that can be pursued any way you’d like. Here are a few examples of different ways you can use secondary research to explore your research topic .
Secondary research is a very common research approach, but has distinct advantages and disadvantages.
Advantages of secondary research
Advantages include:
- Secondary data is very easy to source and readily available .
- It is also often free or accessible through your educational institution’s library or network, making it much cheaper to conduct than primary research .
- As you are relying on research that already exists, conducting secondary research is much less time consuming than primary research. Since your timeline is so much shorter, your research can be ready to publish sooner.
- Using data from others allows you to show reproducibility and replicability , bolstering prior research and situating your own work within your field.
Disadvantages of secondary research
Disadvantages include:
- Ease of access does not signify credibility . It’s important to be aware that secondary research is not always reliable , and can often be out of date. It’s critical to analyze any data you’re thinking of using prior to getting started, using a method like the CRAAP test .
- Secondary research often relies on primary research already conducted. If this original research is biased in any way, those research biases could creep into the secondary results.
Many researchers using the same secondary research to form similar conclusions can also take away from the uniqueness and reliability of your research. Many datasets become “kitchen-sink” models, where too many variables are added in an attempt to draw increasingly niche conclusions from overused data . Data cleansing may be necessary to test the quality of the research.
Receive feedback on language, structure, and formatting
Professional editors proofread and edit your paper by focusing on:
- Academic style
- Vague sentences
- Style consistency
See an example
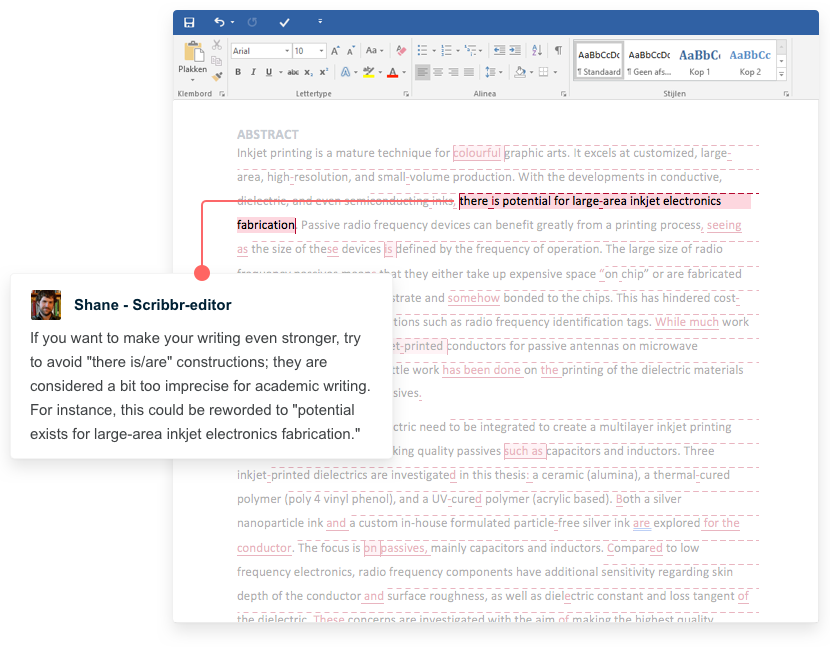
If you want to know more about statistics , methodology , or research bias , make sure to check out some of our other articles with explanations and examples.
- Normal distribution
- Degrees of freedom
- Null hypothesis
- Discourse analysis
- Control groups
- Mixed methods research
- Non-probability sampling
- Quantitative research
- Inclusion and exclusion criteria
Research bias
- Rosenthal effect
- Implicit bias
- Cognitive bias
- Selection bias
- Negativity bias
- Status quo bias
A systematic review is secondary research because it uses existing research. You don’t collect new data yourself.
The research methods you use depend on the type of data you need to answer your research question .
- If you want to measure something or test a hypothesis , use quantitative methods . If you want to explore ideas, thoughts and meanings, use qualitative methods .
- If you want to analyze a large amount of readily-available data, use secondary data. If you want data specific to your purposes with control over how it is generated, collect primary data.
- If you want to establish cause-and-effect relationships between variables , use experimental methods. If you want to understand the characteristics of a research subject, use descriptive methods.
Quantitative research deals with numbers and statistics, while qualitative research deals with words and meanings.
Quantitative methods allow you to systematically measure variables and test hypotheses . Qualitative methods allow you to explore concepts and experiences in more detail.
Sources in this article
We strongly encourage students to use sources in their work. You can cite our article (APA Style) or take a deep dive into the articles below.
George, T. (2024, January 12). What is Secondary Research? | Definition, Types, & Examples. Scribbr. Retrieved August 26, 2024, from https://www.scribbr.com/methodology/secondary-research/
Largan, C., & Morris, T. M. (2019). Qualitative Secondary Research: A Step-By-Step Guide (1st ed.). SAGE Publications Ltd.
Peloquin, D., DiMaio, M., Bierer, B., & Barnes, M. (2020). Disruptive and avoidable: GDPR challenges to secondary research uses of data. European Journal of Human Genetics , 28 (6), 697–705. https://doi.org/10.1038/s41431-020-0596-x
Is this article helpful?
Tegan George
Other students also liked, primary research | definition, types, & examples, how to write a literature review | guide, examples, & templates, what is a case study | definition, examples & methods, "i thought ai proofreading was useless but..".
I've been using Scribbr for years now and I know it's a service that won't disappoint. It does a good job spotting mistakes”
A Guide To Secondary Data Analysis
What is secondary data analysis? How do you carry it out? Find out in this post.
Historically, the only way data analysts could obtain data was to collect it themselves. This type of data is often referred to as primary data and is still a vital resource for data analysts.
However, technological advances over the last few decades mean that much past data is now readily available online for data analysts and researchers to access and utilize. This type of data—known as secondary data—is driving a revolution in data analytics and data science.
Primary and secondary data share many characteristics. However, there are some fundamental differences in how you prepare and analyze secondary data. This post explores the unique aspects of secondary data analysis. We’ll briefly review what secondary data is before outlining how to source, collect and validate them. We’ll cover:
- What is secondary data analysis?
- How to carry out secondary data analysis (5 steps)
- Summary and further reading
Ready for a crash course in secondary data analysis? Let’s go!
1. What is secondary data analysis?
Secondary data analysis uses data collected by somebody else. This contrasts with primary data analysis, which involves a researcher collecting predefined data to answer a specific question. Secondary data analysis has numerous benefits, not least that it is a time and cost-effective way of obtaining data without doing the research yourself.
It’s worth noting here that secondary data may be primary data for the original researcher. It only becomes secondary data when it’s repurposed for a new task. As a result, a dataset can simultaneously be a primary data source for one researcher and a secondary data source for another. So don’t panic if you get confused! We explain exactly what secondary data is in this guide .
In reality, the statistical techniques used to carry out secondary data analysis are no different from those used to analyze other kinds of data. The main differences lie in collection and preparation. Once the data have been reviewed and prepared, the analytics process continues more or less as it usually does. For a recap on what the data analysis process involves, read this post .
In the following sections, we’ll focus specifically on the preparation of secondary data for analysis. Where appropriate, we’ll refer to primary data analysis for comparison.
2. How to carry out secondary data analysis
Step 1: define a research topic.
The first step in any data analytics project is defining your goal. This is true regardless of the data you’re working with, or the type of analysis you want to carry out. In data analytics lingo, this typically involves defining:
- A statement of purpose
- Research design
Defining a statement of purpose and a research approach are both fundamental building blocks for any project. However, for secondary data analysis, the process of defining these differs slightly. Let’s find out how.
Step 2: Establish your statement of purpose
Before beginning any data analytics project, you should always have a clearly defined intent. This is called a ‘statement of purpose.’ A healthcare analyst’s statement of purpose, for example, might be: ‘Reduce admissions for mental health issues relating to Covid-19′. The more specific the statement of purpose, the easier it is to determine which data to collect, analyze, and draw insights from.
A statement of purpose is helpful for both primary and secondary data analysis. It’s especially relevant for secondary data analysis, though. This is because there are vast amounts of secondary data available. Having a clear direction will keep you focused on the task at hand, saving you from becoming overwhelmed. Being selective with your data sources is key.
Step 3: Design your research process
After defining your statement of purpose, the next step is to design the research process. For primary data, this involves determining the types of data you want to collect (e.g. quantitative, qualitative, or both ) and a methodology for gathering them.
For secondary data analysis, however, your research process will more likely be a step-by-step guide outlining the types of data you require and a list of potential sources for gathering them. It may also include (realistic) expectations of the output of the final analysis. This should be based on a preliminary review of the data sources and their quality.
Once you have both your statement of purpose and research design, you’re in a far better position to narrow down potential sources of secondary data. You can then start with the next step of the process: data collection.
Step 4: Locate and collect your secondary data
Collecting primary data involves devising and executing a complex strategy that can be very time-consuming to manage. The data you collect, though, will be highly relevant to your research problem.
Secondary data collection, meanwhile, avoids the complexity of defining a research methodology. However, it comes with additional challenges. One of these is identifying where to find the data. This is no small task because there are a great many repositories of secondary data available. Your job, then, is to narrow down potential sources. As already mentioned, it’s necessary to be selective, or else you risk becoming overloaded.
Some popular sources of secondary data include:
- Government statistics , e.g. demographic data, censuses, or surveys, collected by government agencies/departments (like the US Bureau of Labor Statistics).
- Technical reports summarizing completed or ongoing research from educational or public institutions (colleges or government).
- Scientific journals that outline research methodologies and data analysis by experts in fields like the sciences, medicine, etc.
- Literature reviews of research articles, books, and reports, for a given area of study (once again, carried out by experts in the field).
- Trade/industry publications , e.g. articles and data shared in trade publications, covering topics relating to specific industry sectors, such as tech or manufacturing.
- Online resources: Repositories, databases, and other reference libraries with public or paid access to secondary data sources.
Once you’ve identified appropriate sources, you can go about collecting the necessary data. This may involve contacting other researchers, paying a fee to an organization in exchange for a dataset, or simply downloading a dataset for free online .
Step 5: Evaluate your secondary data
Secondary data is usually well-structured, so you might assume that once you have your hands on a dataset, you’re ready to dive in with a detailed analysis. Unfortunately, that’s not the case!
First, you must carry out a careful review of the data. Why? To ensure that they’re appropriate for your needs. This involves two main tasks:
Evaluating the secondary dataset’s relevance
- Assessing its broader credibility
Both these tasks require critical thinking skills. However, they aren’t heavily technical. This means anybody can learn to carry them out.
Let’s now take a look at each in a bit more detail.
The main point of evaluating a secondary dataset is to see if it is suitable for your needs. This involves asking some probing questions about the data, including:
What was the data’s original purpose?
Understanding why the data were originally collected will tell you a lot about their suitability for your current project. For instance, was the project carried out by a government agency or a private company for marketing purposes? The answer may provide useful information about the population sample, the data demographics, and even the wording of specific survey questions. All this can help you determine if the data are right for you, or if they are biased in any way.
When and where were the data collected?
Over time, populations and demographics change. Identifying when the data were first collected can provide invaluable insights. For instance, a dataset that initially seems suited to your needs may be out of date.
On the flip side, you might want past data so you can draw a comparison with a present dataset. In this case, you’ll need to ensure the data were collected during the appropriate time frame. It’s worth mentioning that secondary data are the sole source of past data. You cannot collect historical data using primary data collection techniques.
Similarly, you should ask where the data were collected. Do they represent the geographical region you require? Does geography even have an impact on the problem you are trying to solve?
What data were collected and how?
A final report for past data analytics is great for summarizing key characteristics or findings. However, if you’re planning to use those data for a new project, you’ll need the original documentation. At the very least, this should include access to the raw data and an outline of the methodology used to gather them. This can be helpful for many reasons. For instance, you may find raw data that wasn’t relevant to the original analysis, but which might benefit your current task.
What questions were participants asked?
We’ve already touched on this, but the wording of survey questions—especially for qualitative datasets—is significant. Questions may deliberately be phrased to preclude certain answers. A question’s context may also impact the findings in a way that’s not immediately obvious. Understanding these issues will shape how you perceive the data.
What is the form/shape/structure of the data?
Finally, to practical issues. Is the structure of the data suitable for your needs? Is it compatible with other sources or with your preferred analytics approach? This is purely a structural issue. For instance, if a dataset of people’s ages is saved as numerical rather than continuous variables, this could potentially impact your analysis. In general, reviewing a dataset’s structure helps better understand how they are categorized, allowing you to account for any discrepancies. You may also need to tidy the data to ensure they are consistent with any other sources you’re using.
This is just a sample of the types of questions you need to consider when reviewing a secondary data source. The answers will have a clear impact on whether the dataset—no matter how well presented or structured it seems—is suitable for your needs.
Assessing secondary data’s credibility
After identifying a potentially suitable dataset, you must double-check the credibility of the data. Namely, are the data accurate and unbiased? To figure this out, here are some key questions you might want to include:
What are the credentials of those who carried out the original research?
Do you have access to the details of the original researchers? What are their credentials? Where did they study? Are they an expert in the field or a newcomer? Data collection by an undergraduate student, for example, may not be as rigorous as that of a seasoned professor.
And did the original researcher work for a reputable organization? What other affiliations do they have? For instance, if a researcher who works for a tobacco company gathers data on the effects of vaping, this represents an obvious conflict of interest! Questions like this help determine how thorough or qualified the researchers are and if they have any potential biases.
Do you have access to the full methodology?
Does the dataset include a clear methodology, explaining in detail how the data were collected? This should be more than a simple overview; it must be a clear breakdown of the process, including justifications for the approach taken. This allows you to determine if the methodology was sound. If you find flaws (or no methodology at all) it throws the quality of the data into question.
How consistent are the data with other sources?
Do the secondary data match with any similar findings? If not, that doesn’t necessarily mean the data are wrong, but it does warrant closer inspection. Perhaps the collection methodology differed between sources, or maybe the data were analyzed using different statistical techniques. Or perhaps unaccounted-for outliers are skewing the analysis. Identifying all these potential problems is essential. A flawed or biased dataset can still be useful but only if you know where its shortcomings lie.
Have the data been published in any credible research journals?
Finally, have the data been used in well-known studies or published in any journals? If so, how reputable are the journals? In general, you can judge a dataset’s quality based on where it has been published. If in doubt, check out the publication in question on the Directory of Open Access Journals . The directory has a rigorous vetting process, only permitting journals of the highest quality. Meanwhile, if you found the data via a blurry image on social media without cited sources, then you can justifiably question its quality!
Again, these are just a few of the questions you might ask when determining the quality of a secondary dataset. Consider them as scaffolding for cultivating a critical thinking mindset; a necessary trait for any data analyst!
Presuming your secondary data holds up to scrutiny, you should be ready to carry out your detailed statistical analysis. As we explained at the beginning of this post, the analytical techniques used for secondary data analysis are no different than those for any other kind of data. Rather than go into detail here, check out the different types of data analysis in this post.
3. Secondary data analysis: Key takeaways
In this post, we’ve looked at the nuances of secondary data analysis, including how to source, collect and review secondary data. As discussed, much of the process is the same as it is for primary data analysis. The main difference lies in how secondary data are prepared.
Carrying out a meaningful secondary data analysis involves spending time and effort exploring, collecting, and reviewing the original data. This will help you determine whether the data are suitable for your needs and if they are of good quality.
Why not get to know more about what data analytics involves with this free, five-day introductory data analytics short course ? And, for more data insights, check out these posts:
- Discrete vs continuous data variables: What’s the difference?
- What are the four levels of measurement? Nominal, ordinal, interval, and ratio data explained
- What are the best tools for data mining?
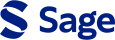
- Request new password
- Create a new account
The Essential Guide to Doing Your Research Project
Student resources, steps in secondary data analysis, stepping your way through effective secondary data analysis.
Determine your research question – As indicated above, knowing exactly what you are looking for
Locating data – Knowing what is out there and whether you can gain access to it. A quick Internet search, possibly with the help of a librarian, will reveal a wealth of options.
Evaluating relevance of the data – Considering things like the data’s original purpose, when it was collected, population, sampling strategy/sample, data collection protocols, operationalization of concepts, questions asked, and form/shape of the data.
Assessing credibility of the data – Establishing the credentials of the original researchers, searching for full explication of methods including any problems encountered, determining how consistent the data is with data from other sources, and discovering whether the data has been used in any credible published research.
Analysis – This will generally involve a range of statistical processes as discussed in Chapter 13.
How to Analyse Secondary Data for a Dissertation
Secondary data refers to data that has already been collected by another researcher. For researchers (and students!) with limited time and resources, secondary data, whether qualitative or quantitative can be a highly viable source of data. In addition, with the advances in technology and access to peer reviewed journals and studies provided by the internet, it is increasingly popular as a form of data collection. The question that frequently arises amongst students however, is: how is secondary data best analysed?
The process of data analysis in secondary research
Secondary analysis (i.e., the use of existing data) is a systematic methodological approach that has some clear steps that need to be followed for the process to be effective. In simple terms there are three steps:
- Step One: Development of Research Questions
- Step Two: Identification of dataset
- Step Three: Evaluation of the dataset.
Let’s look at each of these in more detail:
Step One: Development of research questions
Using secondary data means you need to apply theoretical knowledge and conceptual skills to be able to use the dataset to answer research questions. Clearly therefore, the first step is thus to clearly define and develop your research questions so that you know the areas of interest that you need to explore for location of the most appropriate secondary data.
Step Two: Identification of Dataset
This stage should start with identification, through investigation, of what is currently known in the subject area and where there are gaps, and thus what data is available to address these gaps. Sources can be academic from prior studies that have used quantitative or qualitative data, and which can then be gathered together and collated to produce a new secondary dataset. In addition, other more informal or “grey” literature can also be incorporated, including consumer report, commercial studies or similar. One of the values of using secondary research is that original survey works often do not use all the data collected which means this unused information can be applied to different settings or perspectives.
Key point: Effective use of secondary data means identifying how the data can be used to deliver meaningful and relevant answers to the research questions. In other words that the data used is a good fit for the study and research questions.
Step Three: Evaluation of the dataset for effectiveness/fit
A good tip is to use a reflective approach for data evaluation. In other words, for each piece of secondary data to be utilised, it is sensible to identify the purpose of the work, the credentials of the authors (i.e., credibility, what data is provided in the original work and how long ago it was collected). In addition, the methods used and the level of consistency that exists compared to other works. This is important because understanding the primary method of data collection will impact on the overall evaluation and analysis when it is used as secondary source. In essence, if there is no understanding of the coding used in qualitative data analysis to identify key themes then there will be a mismatch with interpretations when the data is used for secondary purposes. Furthermore, having multiple sources which draw similar conclusions ensures a higher level of validity than relying on only one or two secondary sources.
A useful framework provides a flow chart of decision making, as shown in the figure below.
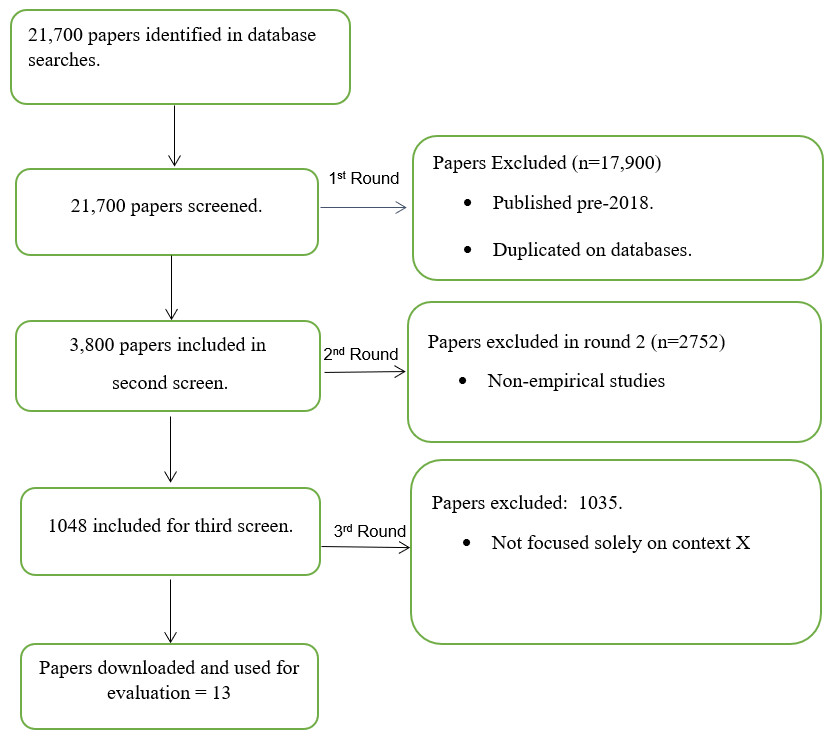
Following this process ensures that only those that are most appropriate for your research questions are included in the final dataset, but also demonstrates to your readers that you have been thorough in identifying the right works to use.
Writing up the Analysis
Once you have your dataset, writing up the analysis will depend on the process used. If the data is qualitative in nature, then you should follow the following process.
Pre-Planning
- Read and re-read all sources, identifying initial observations, correlations, and relationships between themes and how they apply to your research questions.
- Once initial themes are identified, it is sensible to explore further and identify sub-themes which lead on from the core themes and correlations in the dataset, which encourages identification of new insights and contributes to the originality of your own work.
Structure of the Analysis Presentation
Introduction.
The introduction should commence with an overview of all your sources. It is good practice to present these in a table, listed chronologically so that your work has an orderly and consistent flow. The introduction should also incorporate a brief (2-3 sentences) overview of the key outcomes and results identified.
The body text for secondary data, irrespective of whether quantitative or qualitative data is used, should be broken up into sub-sections for each argument or theme presented. In the case of qualitative data, depending on whether content, narrative or discourse analysis is used, this means presenting the key papers in the area, their conclusions and how these answer, or not, your research questions. Each source should be clearly cited and referenced at the end of the work. In the case of qualitative data, any figures or tables should be reproduced with the correct citations to their original source. In both cases, it is good practice to give a main heading of a key theme, with sub-headings for each of the sub themes identified in the analysis.
Do not use direct quotes from secondary data unless they are:
- properly referenced, and
- are key to underlining a point or conclusion that you have drawn from the data.
All results sections, regardless of whether primary or secondary data has been used should refer back to the research questions and prior works. This is because, regardless of whether the results back up or contradict previous research, including previous works shows a wider level of reading and understanding of the topic being researched and gives a greater depth to your own work.
Summary of results
The summary of the results section of a secondary data dissertation should deliver a summing up of key findings, and if appropriate a conceptual framework that clearly illustrates the findings of the work. This shows that you have understood your secondary data, how it has answered your research questions, and furthermore that your interpretation has led to some firm outcomes.
secondary data analysis
- August 2018

- Coventry University. UK
Discover the world's research
- 25+ million members
- 160+ million publication pages
- 2.3+ billion citations

- Shahrazad Hadad

- Charles C. Ragin
- Herbert F. Weisberg
- Bruce D. Bowen

- K S Cameron
- G W Driskill
- A L Brenton
- C F -Nachmias
- Recruit researchers
- Join for free
- Login Email Tip: Most researchers use their institutional email address as their ResearchGate login Password Forgot password? Keep me logged in Log in or Continue with Google Welcome back! Please log in. Email · Hint Tip: Most researchers use their institutional email address as their ResearchGate login Password Forgot password? Keep me logged in Log in or Continue with Google No account? Sign up

How to... Use secondary data & archival material
Find out what secondary data is – as opposed to primary data – and how to go about collecting and using it.
On this page
What is secondary data & archival material, using published data sets, using archival data, secondary data as part of the research design, gaining access to, and using, archives, primary & secondary data.
All research will involve the collection of data. Much of this data will be collected directly through some form of interaction between the researcher and the people or organisation concerned, using such methods as interviews, focus groups, surveys and participant observation. Such methods involve the collection of primary data, and herein lies the opportunity for the researcher to develop and demonstrate the greatest skill.
However sometimes the researcher will use data which has already been collected for other purposes – in other words, he or she is going to an existing source rather than directly interacting with people. The data may have been:
- Deliberately collected and analysed, for example for some official survey such as the UK Labour Market Trends (now published as Economic & Labour Market Review (ELMR) ) or General Household Survey .
- Created in a more informal sense as a record of people's activities, for example, letters or other personal items, household bills, company records, etc. At some point, they may have been deliberately collected and organised into an archive.
Either way, such material is termed secondary data.
Rather confusingly, the latter form of secondary data is also referred to as primary source material.
"Primary resources are sources that are usually created at the time of an event. Primary resources are the direct evidence or first hand accounts of historical events without secondary analysis or interpretation." (York University Libraries Archival Research Tutorial)
This distinguishes them from secondary sources which describe, analyse and refer to the primary sources.
The above definitions and distinctions can be described diagrammatically as follows:
Types of secondary data
Secondary data is found in print or electronic form, if the latter, on CD-ROM, as an online computer database, or on the Internet. Furthermore, it can be in the form of statistics collected by governments, trade associations, organisations that exist to collect and sell statistical data, or just as plain documents in archives or company records.
A crucial distinction is whether or not the data has been interpreted, or whether it exists in raw form.
- Raw data, also referred to as documentary or archival data, will exist in the form in which it was originally intended, for example meeting minutes, staff records, reports on new markets, accounts of sales of goods/services etc.
- Interpreted data, which may also be referred to as survey data, will have been collected for a particular purpose, for example, to analyse spending patterns.
Because interpreted data will have been collected deliberately, the plan behind its collection and interpretation will also have been deliberate – that is, it will have been subjected to a particular research design.
By contrast, raw data will not have been processed, and will exist in its original form. (See " Using archival data " section in this guide.)
When and why to use secondary data
There are various reasons for using secondary data:
- A particularly good collection of data already exists.
- You are doing a historical study – that is, your study begins and ends at a particular point in time.
- You are covering an extended period, and analysing development over that period – a longitudinal study.
- The unit that you are studying may be difficult, or simply too large, to study directly.
- You are doing a case study of a particular organisation/industry/area, and it is important to look at the relevant documents.
You should pay particular attention to the place of secondary documents within your research design. How prominent a role you give to this method may depend on your subject: for example, if you are researching in the area of accounting, finance or business history, secondary documentary sources are likely to play an important part. Otherwise, use of secondary data is likely to play a complementary part in your research design. For example, if you are studying a particular organisation, you would probably want to supplement observation/interviews with a look at particular documents produced by that organisation.
In " Learning lessons? The registration of lobbyists at the Scottish parliament " ( Journal of Communication Management , Vol. 10 No. 1), the author uses archival research at the Scottish parliament as a supplementary research method (along with the media and focus groups), his main method being interviews and participant observation of meetings.
This point is further developed in the " Secondary data as part of the research design " section of this guide. Reasons for using the different types of secondary data are further developed in the individual sections.
NB If you are doing a research project/dissertation/thesis, check your organisation's view of secondary data. Some organisations may require you to use primary data as your principle research method.
Advantages and disadvantages of secondary data collection
The advantages of using secondary data are:
- The fact that much information exists in documented form – whether deliberately processed or not – means that such information cannot be ignored by the researcher, and generally saves time and effort collecting data which would otherwise have to be collected directly. In particular:
- Many existing data sets are enormous, and far greater than the researcher would be able to collect him or herself, with a far larger sample.
- The data may be particularly good quality, which can apply both to archival data (e.g. a complete collection of records on a particular topic) and to published data sets, particularly those which come from a government source, or from one of the leading commercial providers of data and statistics.
- You can access information which you may otherwise have had to secure in a more obtrusive manner.
- Existence of a large amount of data can facilitate different types of analysis, such as:
- longitudinal or international analysis of information which would have otherwise been difficult to collect due to scale.
- manipulation of data within the particular data set, including the comparison of particular subsets.
- Unforseen discoveries can be made – for example, the link between smoking and lung cancer was made by analysing medical records.
The disadvantages of secondary data collection are:
- There may be a cost to acquiring the data set.
- You will need to familiarise yourself with the data, and if you are dealing with a large and complex data set, it will be hard to manage.
- The data may not match the research question: there may be too much data, or there may be gaps, or the data may have been collected for a completely different purpose.
- The measures, for example between countries/states/historical periods, may not be directly comparable. (See the " Secondary data as part of the research design " section of this guide for a further development of this topic.)
- The researcher has no control over the quality of the data, which may not be seen as rigorous and reliable as data which are specifically collected by the researcher, who has adopted a specific research design for the question.
- Collecting primary data builds up more research skills than collecting secondary data.
- Company data particularly may be seen as commercially sensitive, and it may be difficult to gain access to company archives, which may be stored in different departments or on the company intranet, to which access may be difficult.
What are they?
As discussed in the previous section, these are sources of data which have already been collected and worked on by someone else, according to a particular research design. Other points to note are:
- Mostly they will have been collect by means of a survey, which may be:
- a census, which is an "official count", normally carried out by the government, with obligatory participation, for example the UK population censuses carried out every ten years
- a repeated survey, which involves collecting information at regular intervals, for example government surveys about household expenditure
- an ad hoc survey, done just once for a particular purpose, such as for example a market research survey.
- Interpreted data as referring to a particular social unit is termed a data set.
- A database is a structured data set, produced as a matrix with each social unit having a row, and each variable a column.
- Sometimes, different data sets are combined to produce multiple source secondary data: for example, the publication Business Statistics of the United States: Patterns of Economic Change contains data on virtually all aspects of the US economy from 1929 onwards. Such multiple source data sets may have been compiled on:
- a time series basis, that is they are based on repeated surveys (see above) or on comparable variables from different surveys to provide longitudinal data
- a geographical basis, providing information on different areas.
Key considerations
There are a number of points to consider when using data sets, some practical and others associated with the research design (yours and theirs).
Practical considerations relate to cost and use:
- Whilst much data is freely available, there may be a charge. For example, Business Statistics of the United States: Patterns of Economic Change is priced US$147. So, when deciding what data to use it's a good idea to check what's already in your library.
- Is the data available in computerised form, or will you have to enter it manually? If it is available in computerised form, is it in a form suitable to your research design (see below) or will you have to tabulate the data in a different form?
Research considerations include:
- Is the data set so important to your research that you cannot ignore it? For example, if you were doing a project which involved top corporations, you could not afford to ignore the publications which provided data and statistics, such as Europe's 15,000 Largest Companies 2006 .
- Does the data generally cover the research question?
- Is the coverage relevant, or does it leave out areas (e.g. only Asia as opposed to Australasia) or time periods (e.g. only starting in 1942 when you wanted data from 1928)?
- Are the variables relevant, for example if you are interested in household expenditure does it break down the households in ways relevant to your project?
- Are the measures used the same, for example, is growth in sales expressed as an amount or a percentage?
- In the case of data from different countries, has the data been collected in the same way? For example, workers affected by strikes may include those directly affected in one country, and those indirectly affected in another.
- Is the data reliable, and current? Note that data from government, and reputable commercial sources, is likely to be trustworthy but you should be wary of information on the Internet unless you know its source. Data from trustworthy sources is likely to have been collected by a team of experts, with good quality research design and instruments.
- The advantage of survey data in particular is that you have access to a far larger sample than you would otherwise have been able to collect yourself.
- There is an obvious advantage to using a large data source, however you need to allow for the time needed to extract what you want, and to re-tabulate the data in a form suitable for your research.
- How has the data been collected, for example it it longitudinal or geographical? This will affect the type of research question it can help with, for example, if you were comparing France and Germany, you would obviously want geographical data.
- How intrinsic to your research design will the use of secondary data be? Beware of relying on it entirely, but it may be a useful way of triangulating other research, for example if you have done a survey of shopping habits, you can assess how generalisable your findings are by looking at a census.
- While use of secondary data sets may not be seen as rigorous as collecting data yourself, the big advantage is that they are in a permanently available form and can be checked by others, which is an important point for validity.
And finally...
- Will the benefits you gain from using secondary data sets as a research methods outweigh the costs of acquiring the data, and the time spent sorting out what is relevant?
Producers of published secondary data include:
- Governments and intergovermental organisations, who produce a wide variety of data. For example, from the US Government come such titles as Budget of the United States Government , Business Statistics of the United States: Patterns of Economic Change , County and City Extra (source of data for every state), and Handbook of U.S. Labor Statistics .
- Trade associations and organisations representing particular interests, such as for example the American Marketing Association. These may have data and information relevant to their particular interest group.
- company information: for example AMADEUS provides pan European information on companies that includes balance sheets, profit and loss, ratios, descriptive etc., while FAME does a similar job for companies in the UK and Ireland.
- market research: for example, Mintel specialises in consumer, media and market research and published reports into particular market sectors, whilst Key Note "boasts one of the most comprehensive databases available to corporations in the UK", having published almost 1,000 reports spanning 30 industry sectors.
Where to find such information? The key is to have a very clear idea of what it is you are trying to find: what particular aspects of the research question are you attempting to answer?
You may well find sources listed in your literature review, or your tutor may point you in certain directions, but at some point you will need to consult the tertiary literature, which will point you in the direction of archives, indexes, catalogues and gateways. Your library will probably have Subject Guides covering your areas of interest. The following is a very basic list:
- UK Economic and Social Data Services (ESDS) . Contains links to: UK Data Archive (University of Essex); Institute for Social and Economic Research (University of Essex); Manchester Information and Associated Services (University of Manchester); and Cathie Marsh Centre for Census and Survey Research (University of Manchester). These contain access to a wide range of national and international data sets.
- http://epp.eurostat.ec.europa.eu . Statistics of the European Union.
- University of Michigan . Gateway to statistical resources on the Web.
- D&B Hoovers . Company information on US and international companies.
Archival, or documentary secondary data, are documentary records left by people as a by product of their eveyday activity. They may be formally deposited in an archive or they may just exist as company records.
Historians make considerable use of archival material as a key research technique, using a wide range of personal documents such as letters, diaries, household bills, which are often stored in some sort of formal "archive".
Business researchers talk about "archival research" because they use many of the same techniques for recording and analysing information. Companies, by their very nature, tend to create records, both officially in the form of annual reports, declarations of share value etc., and unofficially in the e-mails, letters, meeting minutes and agendas, sales data, employee records etc. which are the by-product of their daily activities.
If you are studying a business and management related subject, you may make use of archival material for a number of reasons:
- Your research takes a historical perspective, and you want to gain insight into management decisions outside the memories of those whom you interview.
- Archival research is an important tool in your particular discipline – for example, finance and accounting.
- You wish to undertake archival research as part of qualitative research in order to triangulate with interviews, focus groups etc., or perhaps as exploratory research prior to the main research.
- You may be undertaking a case study, or basing your research project on your own organisation; in either case, you should look at company documents as part of this research.
In " Financial reporting and local government reform – a (mis)match? " ( Qualitative Research in Accounting & Management , Vol. 2 No. 2), Robyn Pilcher uses archival research – "Data was obtained from annual reports provided electronically to the DLG and checked against hard copies of these reports and supporting notes" – and interviews as exploratory research to investigate use of flawed financial figures by political parties, before carrying out a detailed examination of a few councils.
" Coalport Bridge Tollhouse, 1793-1995 " ( Structural Survey , Vol. 14 No. 4) is a historical study of this building drawing on such documents as maps, plans, photos, account books, meeting minutes, legal opinions and census records.
As distinct from published data sets, you will have to record and process the data yourself, in order to create your own data set.
Sometimes this archival material will be stored in "official" archives, such as the UK Public Record Office. Mostly however, it will be company specific, stored in official company archives or perhaps in smaller collections in individual departments or business units. Records can exist in physical or electronic form – the latter commonly on the company intranet.
Whatever the company's archiving policy, there is no doubt that businesses provide a rich source of data. Here is a (non exhaustive) list of the forms that data can take:
- Organisational records – for example HR, accounts, pay roll data etc.
- Data referring to the sales of goods or services
- Project files
- Organisation charts
- Meeting minutes and agendas
- Sales literature: catalogues, copies of adverts, brochures etc.
- Annual reports
- Reports to shareholders
- Transcripts of speeches
- Non textual material: maps and plans, videos, tapes, photographs.
Management Information Systems can hold a considerable amount of data. For example, the following HR records may be held:
- data on recruitment, e.g. details of vacancies, dates, job details and criteria
- staff employment details, for example job analysis and evaluation, salary grades, terms and conditions of employment, job objectives, job competencies, performance appraisals
- data relevant to succession and career planning, e.g. the effects of not filling jobs
- management training and development, e.g. training records showing types of training.
Source: Peter Kingsbury (1997), IT Answers to HR Questions , CIPD.
The media (newspapers, magazines, advertisements, television and radio programmes, books, the Internet) can also throw valuable light on events, and media sources should not be ignored.
There are a number of points to consider when using archival material:
- You will need to gain access to the company, and this may prove difficult (see the " Gaining access to, and using, archives " section in this guide). On the other hand, if you are doing a report/project on your own organisation, access may be a lot easier, although even here you should gain agreement to access and use of material.
- Even if you are successful in gaining access to the company, it may be difficult and time-consuming to locate all the information you need, especially if the company does not have a clear archiving policy, and you may need to go through a vast range of documents.
- The data may be incomplete, and may not answer your research question – for example, there may be a gap in records, correspondence may be one-sided and not include responses.
- The data may be biased, in other words it will be written by people who have a particular view. For example, meeting minutes are the "official" version and often things go on in meetings which are not recorded; profitability in annual reports may be reported in such a way as to show a positive rather than a true picture.
- Informal and verbal interactions cannot be captured.
- Archival research is time-consuming, both in locating and in recording documents, so for that reason may not be feasible for smaller projects.
- You will also need to decide how to record data: historians are used to laboriously copying out documents considered too frail to photocopy, and business researchers may need to resort to this if (as is likely) company documents are considered confidential, although in such cases, note-taking may also be out. You will also need to find a suitable way of coding and referring to particular documents.
- Finally, you will need to construct your own data set, for which you will need to have a particular research method.
In " Participatory group observation – a tool to analyse strategic decision-making " ( Qualitative Market Research , Vol. 5 No. 1), Christine Vallaster and Oliver Koll highlight the benefit of multiple methods for studying complex issues, it being thus possible to supplement the weaknesses of one method with the strengths of another and study a phenomenon from a diversity of views, and achieve a high degree of validity. In the case in question, archival research was used to analyse documents (organisation charts, company reports, memos, meeting minutes), and whilst the limitations in terms of incompleteness, selectivity, and not being authored by interviewees were acknowledged, so was their supporting value to interviews, and the same textual analysis method was used for both methods.
We have already mentioned, as part of our discussion of the two main types of secondary data, some considerations in respect to how they are used as part of the research. In this section, we shall look more generally at how secondary data can fit in to the overall research design.
Theoretical framework
Researchers take different views of the facts they are researching. For some, facts exist as independent reality; others admit the possibility of interpretation by the actors concerned. The two views, and their implication for the documents and data concerned, can be summed up as follows:
- Positivists see facts as existing independently of interpretation, so documents are an objective reflection of reality.
- Interpretivists , and even more so realists, see reality as influenced by the social environment, open to manipulation by those who are part of it. A document must be seen in its social context, and an attempt to make sense of that context.
Some examples would be:
- minutes of a sales meeting the purpose of which was to monitor sales, with sales being affected by external influences
- brochure or flyer which was created for a particular item, and designed to appeal to current fashions
- training records of people doing National Vocational Qualifications (used in the UK to acknowledge the value of existing skills).
Reliability and validity
Reliability and validity is important to any research design, and an important consideration with secondary data is the extent to which it relates to the research question, in other words how reliably it can answer it. You need to consider the fit very carefully before deciding to proceed. Some questions which may help here are:
How reliable is the data?
In the case of published data, you will be able to make a judgement by looking at its provenance: does it come from the government, or from a reputable commercial source? The same applies to the Internet – what is the source? Look for publisher information and copyright statements. How up to date is the material?
You also need to make intrinsic judgements, however: what is the methodology behind the survey, and how robust is it? How large was the sample and what was the response rate?
There are fewer obvious external measures you can use to check unpublished, archival material: that from businesses can be notoriously inconsistent and inaccurate. Records can be incomplete with some documents missing; sometimes, whole archives can disappear when companies are taken over. In addition, some documents such as letters, reports, e-mails, meeting minutes etc. have a subjective element, reflecting the view of the author, or the perceived wishes of the recipient. For example, meeting minutes may not reflect a controversial discussion that took place but only the agreed action points; a report on sales may be intended to put a positive spin on a situation and disguise its real seriousness. It helps when assessing reliability to consider who the intended audience is.
If you are using media reports, be aware that these may only include what they consider to be the most pertinent points.
Measurement validity
One of the biggest problems with secondary data is to do with the measurements involved. These may just not be the same as the ones you want (e.g. sales given in revenue rather than quantity), they may deliberately be distorted (e.g. non recording of minor accidents, sick leave etc.), or they may be different for different countries. If the measures are inexact, you need to take a view as to how serious the problem is and how you can address it.
Does the data cover the time frame, geographical area, and variable in which you are interested? For example, if you are studying a particular period in a company, do you have meeting minutes to cover that period, or do they stop/start at a time within the boundaries of that period? Do you have the sales figures for all the countries your are interested in, and all the product types?
You can greatly increase the validity and reliability of your use of secondary data if you triangulate with another research method. For example if you are seeking insights into a period of change within a company, you can use documentary records to compare with interviews with key informants.
" Leading beyond tragedy: the balance of personal identity and adaptability " ( Leadership & Organization Development Journal , Vol. 26 No. 6) is a case study of the Norwegian company Wilhelmson's Lines loss of key employees in a plane crash, and uses archival research along with on-site interviews and participant observation as the tools of case study analysis.
" The human resource management practice of retail branding: an ethnography within Oxfam Trading Division " ( International Journal of Retail & Distribution Management , Vol. 33 No. 7) uses an ethnographic approach and includes scanning the company intranet along with participant observation and interviews.
Quantitative or qualitative?
Documentary data can be used as part of a qualitative or quantitative research design.
Much data, whether from company archives or from published data sets, is statistical, and can therefore be used as part of a quantitative design, for example how many sales were made of a particular item, what were reasons for absenteeism, company profitability etc.
One way of using secondary data in quantitative research is to compare it with data you have collected yourself, probably by a survey. For example, you can compare your own survey data with that from a census or other published survey, which will inevitably have a much larger sample, thereby helping you generalise, and/or triangulate, your findings.
Textual data can also be used qualitatively, for example marketing literature can be used to as backup information on marketing campaigns, and e-mails, letters, meeting minutes etc. can throw additional light on management decisions.
Content analysis is often quoted as a method of analysis: this involves analysing occurrence of key concepts and ideas and either draw statistical inferences or carry out a qualitative assessment, looking at the main themes that emerge.
Archives may be found in national collections, such as the UK's Public Record Office, or as smaller collections associated with national, local or federal government organisations, academic libraries, professional or trade associations, or charities; they may also be found in companies. The latter are generally closely controlled; the former are most likely to be publically available. This page gives a brief overview of how to gain access to archival collections, and what you can expect when you get there.
Preparation
An archival collection, even an open one, is not like a library where you can just turn up. You need to establish opening hours, and then make arrangements to visit.
It is best to write ahead explaining:
- Your project
- Precisely what it is you are looking for.
In order to be clear about point 2, you will need to know not only the precise scope of your research but also how this particular collection can help you. You will therefore need to spend time researching (perhaps more than one) collection, so make sure that this is allowed for in your research plan.
You also need to understand the key difference between libraries and archives:
- Archives are collections of unpublished material, housed in closed stacks, organised according to the principles of the original collector. You can only access the material in situ, and you will need to handle the collection with special care.
- Libraries contain published material, in open stacks, classified according to a particular system, and you may be able to take the material out on loan.
Locating sources
Bibliographic databases are good sources for finding archival collections: you can search by subject, keyword, personal or geographical name. Whilst not containing records of each item, catalogue records of archival collections are generally lengthier than for published materials and may include a summary of materials contained in the collection.
More detailed information about the collection, usually at the level of the box or folder, is found in Finding Aids .
You can find suitable databases through your library's Subject Guides.
Gaining access to commercial collections
As indicated above, commercial archival or document collections are more tightly controlled than public ones, access to which will depend upon a clearly stated request and proof of identity.
Commercial sources, by contrast, may require more negotiation, and more convincing, because of the perceived sensitivity of their material and the fact that they exist for their customers and shareholders, and not as an archival collection. Companies understandably count the opportunity cost of time spent "helping a researcher with their enquiries", not to mentioning opening up possibly sensitive documents to the prying eyes of an outsider.
This can cause problems to the researcher because if the research project is based on one or a few companies, if access is denied then the overall validity of the research will be prejudiced. Given the likelihood that other research methods, such as interview, survey etc. are also being used, it is best to approach access in the widest sense, and stress the benefits to the organisation, the credibility of the researcher, and assurance of confidentiality.

An official website of the United States government
The .gov means it’s official. Federal government websites often end in .gov or .mil. Before sharing sensitive information, make sure you’re on a federal government site.
The site is secure. The https:// ensures that you are connecting to the official website and that any information you provide is encrypted and transmitted securely.
- Publications
- Account settings
- My Bibliography
- Collections
- Citation manager
Save citation to file
Email citation, add to collections.
- Create a new collection
- Add to an existing collection
Add to My Bibliography
Your saved search, create a file for external citation management software, your rss feed.
- Search in PubMed
- Search in NLM Catalog
- Add to Search
Reducing bias in secondary data analysis via an Explore and Confirm Analysis Workflow (ECAW): a proposal and survey of observational researchers
Affiliations.
- 1 Meta-Research Innovation Center at Stanford (METRICS), Stanford University, Stanford, CA 94305-6104, USA.
- 2 School of Psychological Science, University of Bristol, Bristol, UK.
- 3 Doctoral School of Psychology, ELTE Eotvos Lorand University, Budapest, Hungary.
- 4 Institute of Psychology, ELTE Eotvos Lorand University, Budapest, Hungary.
- 5 Melbourne School of Psychological Sciences, University of Melbourne, Melbourne, Australia.
- 6 Department of Psychology, University of Amsterdam, Amsterdam, Noord-Holland, The Netherlands.
- 7 Meta-Research Innovation Center Berlin (METRIC-B), QUEST Center for Transforming Biomedical Research, Berlin Institute of Health, Charité - Universitätsmedizin Berlin, Berlin, Germany.
- 8 MRC Integrative Epidemiology Unit, University of Bristol, Bristol, UK.
- PMID: 37830032
- PMCID: PMC10565389
- DOI: 10.1098/rsos.230568
Background. Although preregistration can reduce researcher bias and increase transparency in primary research settings, it is less applicable to secondary data analysis. An alternative method that affords additional protection from researcher bias, which cannot be gained from conventional forms of preregistration alone, is an Explore and Confirm Analysis Workflow (ECAW). In this workflow, a data management organization initially provides access to only a subset of their dataset to researchers who request it. The researchers then prepare an analysis script based on the subset of data, upload the analysis script to a registry, and then receive access to the full dataset. ECAWs aim to achieve similar goals to preregistration, but make access to the full dataset contingent on compliance. The present survey aimed to garner information from the research community where ECAWs could be applied-employing the Avon Longitudinal Study of Parents and Children (ALSPAC) as a case example. Methods. We emailed a Web-based survey to researchers who had previously applied for access to ALSPAC's transgenerational observational dataset. Results. We received 103 responses, for a 9% response rate. The results suggest that-at least among our sample of respondents-ECAWs hold the potential to serve their intended purpose and appear relatively acceptable. For example, only 10% of respondents disagreed that ALSPAC should run a study on ECAWs (versus 55% who agreed). However, as many as 26% of respondents agreed that they would be less willing to use ALSPAC data if they were required to use an ECAW (versus 45% who disagreed). Conclusion. Our data and findings provide information for organizations and individuals interested in implementing ECAWs and related interventions. Preregistration . https://osf.io/g2fw5 Deviations from the preregistration are outlined in electronic supplementary material A.
Keywords: ALSPAC; Explore and Confirm Analysis Workflow (ECAW); blind data analysis; meta-research; open science; preregistration.
© 2023 The Authors.
PubMed Disclaimer
Conflict of interest statement
At the time of submission, consideration and publication, both Tom Hardwicke and John Ioannidis were members of the Editorial Board of Royal Society Open Science, but had no involvement in the assessment of the paper.
Responses to the survey questions…
Responses to the survey questions on trustworthiness and reproducibility of observational research with…
Responses to survey questions about…
Responses to survey questions about the research practices of participants.
Responses to survey questions about using ECAWs. These bar charts exclude responses of…
Similar articles
- Folic acid supplementation and malaria susceptibility and severity among people taking antifolate antimalarial drugs in endemic areas. Crider K, Williams J, Qi YP, Gutman J, Yeung L, Mai C, Finkelstain J, Mehta S, Pons-Duran C, Menéndez C, Moraleda C, Rogers L, Daniels K, Green P. Crider K, et al. Cochrane Database Syst Rev. 2022 Feb 1;2(2022):CD014217. doi: 10.1002/14651858.CD014217. Cochrane Database Syst Rev. 2022. PMID: 36321557 Free PMC article.
- A survey on how preregistration affects the research workflow: better science but more work. Sarafoglou A, Kovacs M, Bakos B, Wagenmakers EJ, Aczel B. Sarafoglou A, et al. R Soc Open Sci. 2022 Jul 6;9(7):211997. doi: 10.1098/rsos.211997. eCollection 2022 Jul. R Soc Open Sci. 2022. PMID: 35814910 Free PMC article.
- Registered report: Survey on attitudes and experiences regarding preregistration in psychological research. Spitzer L, Mueller S. Spitzer L, et al. PLoS One. 2023 Mar 16;18(3):e0281086. doi: 10.1371/journal.pone.0281086. eCollection 2023. PLoS One. 2023. PMID: 36928664 Free PMC article.
- Making ERP research more transparent: Guidelines for preregistration. Paul M, Govaart GH, Schettino A. Paul M, et al. Int J Psychophysiol. 2021 Jun;164:52-63. doi: 10.1016/j.ijpsycho.2021.02.016. Epub 2021 Mar 4. Int J Psychophysiol. 2021. PMID: 33676957 Review.
- Preregistration of Analyses of Preexisting Data. Mertens G, Krypotos AM. Mertens G, et al. Psychol Belg. 2019 Aug 22;59(1):338-352. doi: 10.5334/pb.493. Psychol Belg. 2019. PMID: 31497308 Free PMC article. Review.
- Camerer CF, et al. . 2016. Evaluating replicability of laboratory experiments in economics. Science 351, 1433-1436. (10.1126/science.aaf0918) - DOI - PubMed
- Errington TM, Mathur M, Soderberg CK, Denis A, Perfito N, Iorns E, Nosek BA. 2021. Investigating the replicability of preclinical cancer biology. eLife 10, e71601. (10.7554/eLife.71601) - DOI - PMC - PubMed
- Ioannidis JPA. 2005. Why most published research findings are false. PLoS Med. 2, e124. (10.1371/journal.pmed.0020124) - DOI - PMC - PubMed
- Ioannidis JPA. 2008. Why most discovered true associations are inflated. Epidemiology 19, 640-648. (10.1097/EDE.0b013e31818131e7) - DOI - PubMed
- Open Science Collaboration. 2015. Estimating the reproducibility of psychological science. Science 349, aac4716. (10.1126/science.aac4716) - DOI - PubMed
Associated data
- figshare/10.6084/m9.figshare.c.6858285
Grants and funding
- MC_UU_00032/7/MRC_/Medical Research Council/United Kingdom
LinkOut - more resources
Full text sources.
- Europe PubMed Central
- PubMed Central
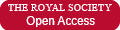
- Citation Manager
NCBI Literature Resources
MeSH PMC Bookshelf Disclaimer
The PubMed wordmark and PubMed logo are registered trademarks of the U.S. Department of Health and Human Services (HHS). Unauthorized use of these marks is strictly prohibited.

An official website of the United States government
The .gov means it’s official. Federal government websites often end in .gov or .mil. Before sharing sensitive information, make sure you’re on a federal government site.
The site is secure. The https:// ensures that you are connecting to the official website and that any information you provide is encrypted and transmitted securely.
- Publications
- Account settings
Preview improvements coming to the PMC website in October 2024. Learn More or Try it out now .
- Advanced Search
- Journal List
- Iran J Public Health
- v.42(12); 2013 Dec
Secondary Data Analysis: Ethical Issues and Challenges
Research does not always involve collection of data from the participants. There is huge amount of data that is being collected through the routine management information system and other surveys or research activities. The existing data can be analyzed to generate new hypothesis or answer critical research questions. This saves lots of time, money and other resources. Also data from large sample surveys may be of higher quality and representative of the population. It avoids repetition of research & wastage of resources by detailed exploration of existing research data and also ensures that sensitive topics or hard to reach populations are not over researched ( 1 ). However, there are certain ethical issues pertaining to secondary data analysis which should be taken care of before handling such data.
Secondary data analysis
Secondary analysis refers to the use of existing research data to find answer to a question that was different from the original work ( 2 ). Secondary data can be large scale surveys or data collected as part of personal research. Although there is general agreement about sharing the results of large scale surveys, but little agreement exists about the second. While the fundamental ethical issues related to secondary use of research data remain the same, they have become more pressing with the advent of new technologies. Data sharing, compiling and storage have become much faster and easier. At the same time, there are fresh concerns about data confidentiality and security.
Issues in Secondary data analysis
Concerns about secondary use of data mostly revolve around potential harm to individual subjects and issue of return for consent. Secondary data vary in terms of the amount of identifying information in it. If the data has no identifying information or is completely devoid of such information or is appropriately coded so that the researcher does not have access to the codes, then it does not require a full review by the ethical board. The board just needs to confirm that the data is actually anonymous. However, if the data contains identifying information on participants or information that could be linked to identify participants, a complete review of the proposal will then be made by the board. The researcher will then have to explain why is it unavoidable to have identifying information to answer the research question and must also indicate how participants’ privacy and the confidentiality of the data will be protected. If the above said concerns are satisfactorily addressed, the researcher can then request for a waiver of consent.
If the data is freely available on the Internet, books or other public forum, permission for further use and analysis is implied. However, the ownership of the original data must be acknowledged. If the research is part of another research project and the data is not freely available, except to the original research team, explicit, written permission for the use of the data must be obtained from the research team and included in the application for ethical clearance.
However, there are certain other issues pertaining to the data that is procured for secondary analysis. The data obtained should be adequate, relevant but not excessive. In secondary data analysis, the original data was not collected to answer the present research question. Thus the data should be evaluated for certain criteria such as the methodology of data collection, accuracy, period of data collection, purpose for which it was collected and the content of the data. It shall be kept for no longer than is necessary for that purpose. It must be kept safe from unauthorized access, accidental loss or destruction. Data in the form of hardcopies should be kept in safe locked cabinets whereas softcopies should be kept as encrypted files in computers. It is the responsibility of the researcher conducting the secondary analysis to ensure that further analysis of the data conducted is appropriate. In some cases there is provision for analysis of secondary data in the original consent form with the condition that the secondary study is approved by the ethics review committee. According to the British Sociological Association’s Statement of Ethical Practice (2004) the researchers must inform participants regarding the use of data and obtain consent for the future use of the material as well. However it also says that consent is not a once-and-for-all event, but is subject to renegotiation over time ( 3 ). It appears that there are no guidelines about the specific conditions that require further consent.
Issues in Secondary analysis of Qualitative data
In qualitative research, the culture of data archiving is absent ( 4 ). Also, there is a concern that data archiving exposes subject’s personal views. However, the best practice is to plan anonymisation at the time of initial transcription. Use of pseudonyms or replacements can protect subject’s identity. A log of all replacements, aggregations or removals should be made and stored separately from the anonymised data files. But because of the circumstances, under which qualitative data is produced, their reinterpretation at some later date can be challenging and raises further ethical concerns.
There is a need for formulating specific guidelines regarding re-use of data, data protection and anonymisation and issues of consent in secondary data analysis.
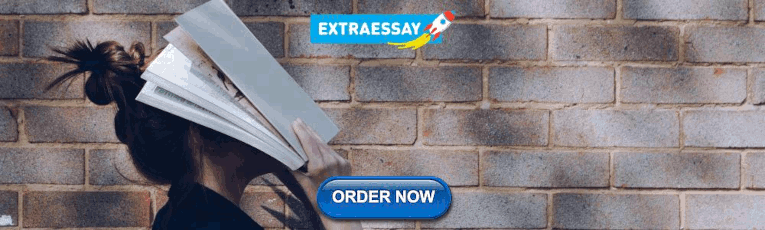
Acknowledgements
The authors declare that there is no conflict of interest.
- Fielding NG, Fielding JL (2003). Resistance and adaptation to criminal identity: Using secondary analysis to evaluate classic studies of crime and deviance . Sociology , 34 ( 4 ): 671–689. [ Google Scholar ]
- Szabo V, Strang VR (1997). Secondary analysis of qualitative data . Advances in Nursing Science , 20 ( 2 ): 66–74. [ PubMed ] [ Google Scholar ]
- Statement of Ethical Practice for the British Sociological Association (2004). The British Sociological Association, Durham . Available at: http://www.york.ac.uk/media/abouttheuniversity/governanceandmanagement/governance/ethicscommittee/hssec/documents/BSA%20statement%20of%20ethical%20practice.pdf (Last accessed 24November2013)
- Archiving Qualitative Data: Prospects and Challenges of Data Preservation and Sharing among Australian Qualitative Researchers. Institute for Social Science Research, The University of Queensland, 2009 . Available at: http://www.assda.edu.au/forms/AQuAQualitativeArchiving_DiscussionPaper_FinalNov09.pdf (Last accessed 05September2013)
- Open access
- Published: 17 August 2024
Epidemiology, ventilation management and outcomes of COVID–19 ARDS patients versus patients with ARDS due to pneumonia in the Pre–COVID era
- Fleur–Stefanie L. I. M. van der Ven 1 , 2 na1 ,
- Siebe G. Blok 1 na1 ,
- Luciano C. Azevedo 3 , 4 ,
- Giacomo Bellani 5 , 6 ,
- Michela Botta 1 ,
- Elisa Estenssoro 7 ,
- Eddy Fan 8 ,
- Juliana Carvalho Ferreira 9 , 10 , 11 ,
- John G. Laffey 12 , 13 ,
- Ignacio Martin–Loeches 14 , 15 ,
- Ana Motos 16 , 17 , 28 ,
- Tai Pham 18 , 19 ,
- Oscar Peñuelas 17 , 20 ,
- Antonio Pesenti 21 ,
- Luigi Pisani 1 , 22 , 24 ,
- Ary Serpa Neto 4 , 23 ,
- Marcus J. Schultz 1 , 24 , 25 , 26 , 27 ,
- Antoni Torres 16 , 17 , 28 , 29 ,
- Anissa M. Tsonas 1 ,
- Frederique Paulus 1 , 30 &
- David M. P. van Meenen 1 , 31
for the ERICC–, LUNG SAFE–, PRoVENT–COVID–, EPICCoV–, CIBERESUCICOVID–, SATI–COVID–19–investigators
Respiratory Research volume 25 , Article number: 312 ( 2024 ) Cite this article
652 Accesses
Metrics details
Ventilation management may differ between COVID–19 ARDS (COVID–ARDS) patients and patients with pre–COVID ARDS (CLASSIC–ARDS); it is uncertain whether associations of ventilation management with outcomes for CLASSIC–ARDS also exist in COVID–ARDS.
Individual patient data analysis of COVID–ARDS and CLASSIC–ARDS patients in six observational studies of ventilation, four in the COVID–19 pandemic and two pre–pandemic. Descriptive statistics were used to compare epidemiology and ventilation characteristics. The primary endpoint were key ventilation parameters; other outcomes included mortality and ventilator–free days and alive (VFD–60) at day 60.
This analysis included 6702 COVID–ARDS patients and 1415 CLASSIC–ARDS patients. COVID–ARDS patients received lower median V T (6.6 [6.0 to 7.4] vs 7.3 [6.4 to 8.5] ml/kg PBW; p < 0.001) and higher median PEEP (12.0 [10.0 to 14.0] vs 8.0 [6.0 to 10.0] cm H 2 O; p < 0.001), at lower median ΔP (13.0 [10.0 to 15.0] vs 16.0 [IQR 12.0 to 20.0] cm H 2 O; p < 0.001) and higher median Crs (33.5 [26.6 to 42.1] vs 28.1 [21.6 to 38.4] mL/cm H 2 O; p < 0.001). Following multivariable adjustment, higher ΔP had an independent association with higher 60–day mortality and less VFD–60 in both groups. Higher PEEP had an association with less VFD–60, but only in COVID–ARDS patients.
Conclusions
Our findings show important differences in key ventilation parameters and associations thereof with outcomes between COVID–ARDS and CLASSIC–ARDS.
Trial registration
Clinicaltrials.gov (identifier NCT05650957), December 14, 2022.
The high numbers of patients who needed invasive ventilation early in the unprecedented pandemic of coronavirus disease 2019 (COVID–19) has led to numerous studies of epidemiology, ventilation management and outcomes in patients with acute respiratory distress syndrome (ARDS) related to an infection with SARS–CoV–2. COVID–19 ARDS would differ from ARDS before the pandemic (CLASSIC–ARDS) in several aspects [ 1 , 2 ], and different phenotypes have even been suggested [ 3 , 4 ].
The number of studies that directly compared ventilation management of COVID–ARDS with CLASSIC–ARDS is limited [ 5 , 6 ]. It remains uncertain whether practice of invasive ventilation in COVID–ARDS patients really differed from that in CLASSIC–ARDS patients. It is also unknown whether associations of certain aspects of ventilation with outcomes found in CLASSIC–ARDS also exist in COVID–ARDS. This would have serious implications on how to set the ventilator in the two patient groups, as then certain recommendations in guidelines for ventilation in CLASSIC–ARDS may not apply in COVID–ARDS [ 7 ].
We performed an analysis of a conveniently–sized database that pooled the data of individual patients of six observational ventilation studies, four of which were conducted in the COVID–19 pandemic and two pre–pandemic, to compare epidemiology, ventilator management and associations of ventilation characteristics and outcome between COVID–ARDS and CLASSIC–ARDS patients. To have comparable patient groups, we only selected patients with ARDS from a respiratory infection from the two pre–pandemic studies. We hypothesized that key ventilator parameters would be different between the two groups, and used multivariable analyses to determine associations with outcomes.
Study design and participants
This is a meta–analysis using the individual patient data of patients in six preselected large observational studies focusing on a diverse representation of epidemiological features and ventilation management in both COVID–19 and pre–pandemic ARDS. The six studies were selected because they all contained detailed data on epidemiological features, ventilation data, and outcomes, originating from various regions worldwide, both in resource–limited and resource–rich settings.
The corresponding authors of the original studies accepted the invitation, after which the data dictionaries of the studies were compared to check whether the data could be harmonized. Then, the databases were transferred after local approval and agreement on the analysis plan of the current investigation.
The two pre–pandemic studies were the national ‘Epidemiology of Respiratory Insufficiency in Critical Care’ study (ERICC) conducted in 2011 in Brazil [ 8 ], and the international ‘Large Observational Study to UNderstand the Global Impact of Severe Acute Respiratory FailurE’ study (LUNG SAFE) conducted in 2014 in 50 countries worldwide [ 9 ]. All four studies were conducted during the COVID–19 pandemic, ranging from March 2020 to 2021 and included: the national ‘Practice of Ventilation in COVID–19 patients’ study (PRoVENT–COVID) from The Netherlands [ 10 ], the national ‘EPIdemiology of Critical COVID–19’ study (EPICCoV) from Brazil [ 11 , 12 ], the national ‘Centro de Investigación Biomédica en Red Enfermedades Respiratorias COVID–19 study’ (CIBERESUCICOVID) from Spain [ 13 ], and the national ‘Sociedad Argentina de Terapia Intensiva–COVID–19 study’ (SATI–COVID–19) from Argentina [ 14 ].
The study protocols of the original studies were approved by Institutional Review Boards if applicable, and need for individual patient informed consent was waived for all studies due to their observational designs. Details of all studies can be found in the original publications [ 8 , 9 , 10 , 12 , 13 , 14 ]. We invited the corresponding investigators of the original studies to provide us the case report forms and data dictionaries, and the data of all patients. The creation of the pooled database did not require additional ethical approval. The databases of the original studies were harmonised using the case report forms and data dictionaries, and finally merged. This current analysis is registered at clinicaltrials.gov (study identifier NCT05650957), and its statistical analysis plan was finalized before cleaning and closing of the database.
Patients in the merged database were eligible for participation in this current analysis if: (1) aged 18 years or higher; (2) having received invasive ventilation within the first 48 h of ICU admission, regardless of its duration; and (3) fulfilling the Berlin definition of ARDS. We excluded CLASSIC–ARDS patients when ARDS was reported not to be caused by a respiratory infection.
Data available for merging
The following baseline and demographic variables were available for merging into the new database—sex, age, body weight and height, comorbidities including hypertension and cardiac failure, chronic obstructive pulmonary disease (COPD), diabetes mellitus, kidney failure, liver failure, and cancer, date of hospital and intensive care unit (ICU) admission, and disease severity scores, including the Simplified Acute Physiology Score (SAPS) II at ICU admission and a daily Sequential Organ Failure Assessment (SOFA) scores.
Collected ventilation variables were––mode of ventilation, tidal volume (V T ), positive end–expiratory pressure (PEEP), fraction of inspired oxygen (FiO 2 ), respiratory rate (RR), peak pressure (Ppeak) in volume–controlled ventilation and plateau pressure (Pplat) in pressure–controlled ventilation, blood gas analyses results, and adjunctive therapies to improve oxygenation in case of refractory hypoxaemia. The first available measurement of the day was used. If multiple measurements were taken on the same day, we selected earliest one.
The dynamic driving pressure (ΔP) was calculated by subtracting PEEP from the maximum airway pressure [ 15 , 16 ]. Respiratory system compliance (Crs) was calculated by dividing V T by ΔP. MP was calculated using the power Eq. (17), wherein MP (J/min) = 0.098 * V T * RR * (Ppeak − 0.5 * ΔP) [ 17 ]; a modified power equation was used if no Ppeak was available 0.098 * V T * RR * (Pplat − 0.5 * ΔP) [ 16 ]. The ventilatory ratio was calculated as (minute ventilation * PaCO 2 )/(predicted bodyweight * 100 * 37.5) [ 18 ]. The number of ventilator–free days at day 60 (VFD–60) was calculated by subtracting the number of calendar days a patient received invasive ventilation up to the day of successful extubation from 60, similar to the method used for calculating VFD–28. Patients that died before or at day 60 received zero VFD–60 [ 19 , 20 ].
The following follow–up data were available for merging—last day of ventilation, tracheostomy use, last day in ICU and hospital, and life status at day 60.
The primary endpoint of this analysis was a combination of the following key ventilation characteristics as done before [ 10 ]—V T , PEEP, ΔP, and Crs. Secondary outcomes were other ventilator parameters, the use of prone positioning, muscle paralysis or extracorporeal membrane oxygenation, and 60–day mortality and the number of VFD–60.
Power analysis
We did not perform a formal power analysis; instead, the number of available patients served as the sample size.
Statistical analysis
Baseline demographics were compared using Fisher’s exact tests for categorical variables and Wilcoxon rank–sum tests for continuous variables. Continuous distributed variables are presented as medians and interquartile ranges, categorical variables are presented as frequencies and proportions.
The first day a patient received invasive ventilation and the first full calendar day were combined into ‘day 1’, the next day was designated as ‘day 2’. Information on missing values for each ventilation parameters and other variable can be found in the Supplementary Material (eTable 1). Only SOFA scores were available for all patients, therefore, we chose to only report these instead of other severity scores.
To compare ventilation characteristics between COVID–ARDS and CLASSIC–ARDS patients, a Wilcoxon rank–sum test was used. Cumulative distribution plots were constructed to visualize cumulative distribution frequencies of each ventilation variable or parameter, wherein vertical dotted lines represent broadly accepted safety cutoffs for each variable, and horizontal dotted lines show the respective proportion of patients reaching that cutoff.
As a post–hoc analysis to identify whether V T , PEEP and ΔP have independent associations with 60–day mortality and the number of VFD–60, a multivariable mixed–effects model with centre as random effect was performed. A linear mixed–effects model was used for the number of VFD–60 and a logistic mixed–effects model for 60–day mortality.
The following covariates, with a known or suspected association with these two outcomes were included in the model, based on clinical relevance: (1) PaO 2 /FiO 2 ; and (2) demographic variables, including sex, age, BMI, history of heart failure, COPD, diabetes mellitus, kidney failure, liver failure and cancer.
In this mixed model analysis, when a covariate exhibited more than 10% missing data, we utilized multiple imputation techniques implemented through the MICE package in R. The model was checked for collinearity using variance–inflation factors, wherein a variance–inflation factor < 5 was deemed acceptable. The variance–inflation factor was < 2 for all included variables in our model.
The estimate refers to the average effect of the ventilation parameter, i.e., V T , PEEP or ΔP on the outcome of interest, i.e., 60–day mortality and VFD–60 while controlling for the other variables in the model. A positive estimate indicates that an increase in the predictor variable tends to lead to a corresponding increase in the response variable, indicating a proportional relationship between them. Conversely, a negative estimate suggests that an increase in the predictor variable tends to result in a decrease in the response variable, indicating an inverse proportional relationship between them.
All analyses were conducted in R v.4.0.3 (R Foundation for Statistical Computing, Vienna, Austria). A p value < 0.05 was considered statistically significant.
We received the individual data of a total of 8374 COVID–ARDS patients and 3795 CLASSIC–ARDS patients (Fig. 1 ). After exclusion of patients that did not fulfil the Berlin definition of ARDS, patients that did not receive invasive ventilation on the first and second day in the study, and patients included in the two pre–pandemic studies who did not have a respiratory infection as the cause for ARDS, we had 6702 fully–analysable COVID–ARDS patients and 1415 fully–analysable CLASSIC–ARDS. COVID–ARDS patients were more often male, had higher median BMI, a history of diabetes more often, and a history of COPD or chronic kidney disease less often (Table 1 ). COVID–ARDS patients had lower median SOFA scores, and ARDS severity was more often classified as moderate or severe.
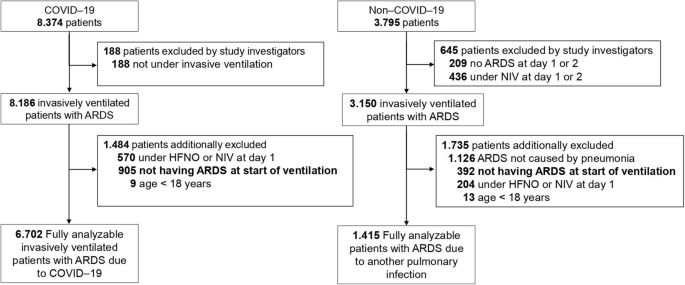
Flowchart of included studies. Abbreviations: ARDS = acute respiratory distress syndrome; COVID–19 = coronavirus disease 2019
COVID–ARDS patients were ventilated with volume–controlled ventilation more often than CLASSIC–ARDS patients (Table 2 ) and received ventilation with lower V T (6.6 [6.0 to 7.4] vs 7.3 [6.4 to 8.5] ml/kg PBW; p < 0.001), higher PEEP (12.0 [10.0 to 14.0] vs 8.0 [6.0 to 10.0] cm H 2 O; p < 0.001), at lower ΔP (13.0 [10.0 to 15.0] vs 16.0 [IQR 12.0 to 20.0] cm H 2 O; p < 0.001) and higher Crs (33.5 [26.6 to 42.1] vs 28.1 [21.6 to 38.4] mL/cm H 2 O; p < 0.001) (Fig. 2 ) COVID–ARDS patients received higher PEEP than CLASSIC–ARDS patients at any FiO 2 level (eFigure 2). Within each group, the ventilation characteristics were not different between day 1 and 2 (eTable 2 and eFigure 1 and 2).
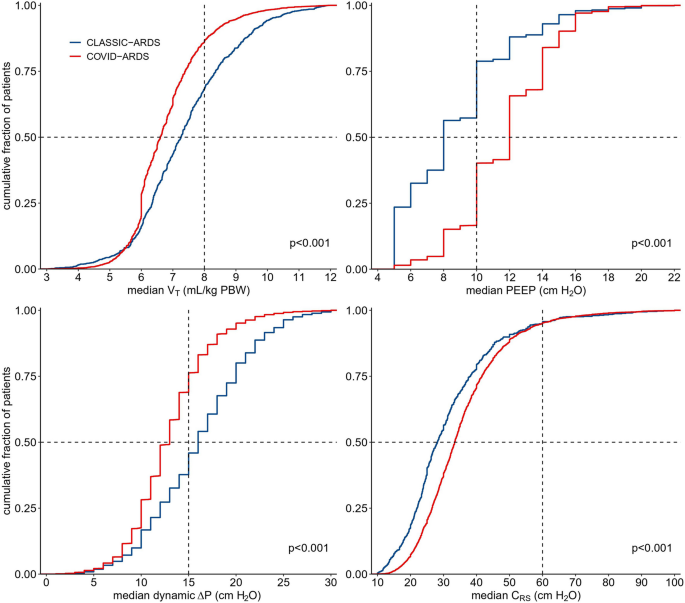
Key ventilation parameters. Cumulative frequency distribution of V T , PEEP, ΔP, and respiratory system compliance on the first calendar day for each variable. Vertical dotted lines represent broadly accepted safety cutoffs for each variable, and horizontal dotted lines show the respective proportion of patients reaching that cutoff. Abbreviations: V T = tidal volume; PBW = predicted bodyweight; PEEP = positive end–expiratory pressure; ΔP = driving pressure; C RS = respiratory system compliance
Prone positioning and neuromuscular blocking agents were more often used in COVID–ARDS patients than in CLASSIC–ARDS patients (Table 2 ). COVID–ARDS patients received a tracheostomy more often than CLASSIC–ARDS patients.
Mortality at day 60 was higher in COVID–ARDS patients compared to CLASSIC–ARDS patients (Table 2 and Fig. 3 ), and COVID–ARDS patients had significantly less VFD–60. Following multivariable adjustment, higher ΔP had an association with higher 60–day mortality and less VFD–60 in both groups. Higher PEEP also had an association with less VFD–60, but only in COVID–ARDS patients and not in CLASSIC–ARDS patients. In both groups, V T neither had an association with 60–day mortality nor with VFD–60 (eFigure 3 and eFigure 4).
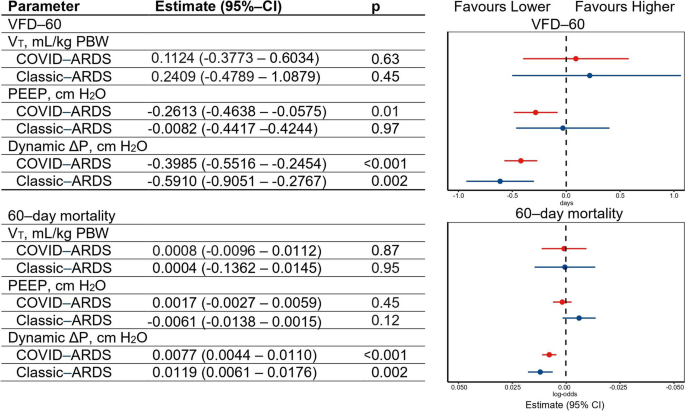
Mortality and ventilator–free days and Alive at day–60, and associations with ventilator parameters. The estimate is the average effect of the predictor variable on the response variable, while controlling for the other variables in the model. A positive estimate suggests a proportional effect, whereas a negative estimate suggests an inversely proportional effect. Abbreviations: ARDS = acute respiratory distress syndrome; VFD = ventilator–free days and alive; IQR = interquartile range; N = number; CI = confidence interval; V T = tidal volume; PBW = predicted bodyweight; PEEP = positive end–expiratory pressure; ΔP = driving pressure
We pooled the individual data of patients from six observational studies of ventilation and compared ventilation characteristics and associations with outcomes between COVID–ARDS with CLASSIC–ARDS. The main findings were: (1) compared to CLASSIC–ARDS patients, COVID–ARDS patients were ventilated with lower V T and higher PEEP, at lower ΔP and higher Crs, however with a higher MP; (2) 60–day mortality was not different between COVID–ARDS and CLASSIC–ARDS, but COVID–ARDS patients had less VFD–60; (3) higher ΔP had an association with higher 60–day mortality and less VFD–60 in COVID–ARDS and CLASSIC–ARDS; and (4) higher PEEP also had an association with less VFD–60, but only in COVID–ARDS.
Our findings add to the current understanding of differences and similarities between COVID–19 ARDS patients and pre–COVID ARDS patients. The international design of our study increases the generalizability of the findings across diverse healthcare systems, both in ARDS patients caused by COVID–19 and in patients with ARDS due to pneumonia from before the pandemic. The large sample size and high quality of the collected data allowed for sophisticated analyses of epidemiology, respiratory support strategies, and outcomes. Additionally, we found associations between key ventilator settings and patient outcomes.
Several studies have compared COVID–19 ARDS with pre–COVID ARDS. The epidemiological differences between COVID–19 ARDS and pre–COVID ARDS patients in our study align with previous findings [ 21 ]. As with other studies [ 22 , 23 ], we also found significant differences in ventilator variables like V T , PEEP, and ΔP, and in the use of adjunctive therapies. Our study contributes by demonstrating these differences specifically among ARDS patients and comparing COVID–19 ARDS to pre–COVID ARDS due to respiratory infections. Differences in outcomes found in our study are, at least in part, in line with prior research findings [ 21 , 23 ]. Our findings confirm that there are differences in mortality and the number of VFD–60 between COVID–19 ARDS and pre–COVID ARDS patients. However, these difference disappeared after propensity matching. This is important as it shows that, at least when comparing outcomes in ARDS patients from an infectious cause, outcomes are not different, opposite to what was thought at the start of the pandemic.
We observed more frequent use of lower V T in COVID–ARDS compared to CLASSIC–ARDS. Indeed, proportions of COVID–ARDS patients that received ventilation with a V T < 6 or between 6 and 8 ml/kg PBW was higher than in CLASSIC–ARDS patients. This finding can be explained in several ways––e.g., it could be that the use of lung–protective ventilation with a lower V T has improved in the last decade [ 15 ]. It is also conceivable that, at least early in the pandemic care for COVID–ARDS patients was provided by inexperienced ICU staff which could have been more adherent to existing guidelines for management of patients with ARDS [ 10 , 24 ]. It is also possible that use of low V T in COVID–ARDS is easier to control––these patients were often deeply sedated and paralyzed allowing a stricter adherence to lower V T . Of note, especially in those patients, ventilation with a lower V T might be more beneficial than in spontaneous breathing patients [ 25 ].
Higher PEEP was more often used in COVID–ARDS patients than in CLASSIC–ARDS patients, at any FiO 2 level. Indeed, proportions of COVID–ARDS patients that received ventilation with a PEEP between 8 and 12 cmH 2 O and even between 12 and 16 cmH 2 O was higher than in CLASSIC–ARDS patients. This finding can also be explained in several ways––e.g., a preference for use of higher PEEP in COVID–ARDS patients may have been triggered by the severity of ARDS, as COVID–ARDS was more often classified as moderate or severe, and more severe hypoxaemia naturally triggers the use of higher PEEP if PEEP/FiO 2 tables are used. It is also possible that higher PEEP was used in the assumption that lung lesions with COVID–ARDS are more recruitable than in CLASSIC–ARDS. This may at least explain the lower ΔP and higher Crs in COVID–ARDS patients.
In COVID–ARDS patients, mechanical power exceeded that of CLASSIC–ARDS, even though the driving pressure was lower. This observation marks the significance of considering factors beyond driving pressure, such as respiratory rate and PEEP, when evaluating the protective nature of invasive ventilation. These findings emphasize the complexity of respiratory management in COVID–ARDS and the need for a comprehensive approach to optimize lungprotective ventilation strategies.
COVID–ARDS patients received prone positioning more often than CLASSIC–ARDS patients. Before the pandemic, prone positioning remained underused, probably because it was more considered a rescue therapy for refractory hypoxaemia [ 26 ]. While we cannot rule out that use of prone positioning increased already before the pandemic, we favour the idea that the higher use of prone positioning in COVID–ARDS patients was triggered by the more severe hypoxaemia in COVID–ARDS patients.
Our analysis found several associations between ventilation parameters and outcome. The association of higher ΔP with higher 60–day mortality and less VFD–60 is in line with previous studies [ 27 , 28 , 29 ]. The association of higher PEEP with worse outcome confirms the findings of earlier studies [ 30 , 31 ]. Of note, this association was only found for COVID–ARDS. This may have been caused by the more frequent use of higher PEEP in COVID–ARDS than in CLASSIC–ARDS. One reason for the association between higher PEEP and worse outcome may be that sicker patients, with a higher chance of dying and prolonged ventilation, received higher PEEP than patients that were less sick. Nonetheless, a high PEEP is suggested to have detrimental effects [ 32 ], emphasizing the need to determine the optimal PEEP level based on lung recruitability rather than hypoxemia alone. Actually, one analysis of PRoVENT–COVID suggested worse outcomes if patients received ventilation according to a higher PEEP/lower FiO 2 table as compared to ventilation according to a lower PEEP/higher FiO 2 [ 30 ]. A post–hoc Bayesian analysis of a randomised clinical study, named the ‘Alveolar Recruitment for ARDS Trial’ (ART), wherein patients were randomized to receive ventilation with PEEP titrated to the best Crs and aggressive recruitment manoeuvres versus ventilation with a low PEEP strategy, suggested that higher PEEP with recruitment manoeuvres worsens the outcome of ARDS from pneumonia, while it may be beneficial in ARDS from another cause [ 33 ]. A posthoc analysis of a randomised clinical study named ‘Lung Imaging for Ventilator Setting in ARDS trial’ (LIFE), suggest that higher PEEP worsens outcomes in patients with ARDS with lesions that may not be recruitable with higher PEEP [ 34 ].
The findings of this pooled analysis extend the existing knowledge of the epidemiology, management of invasive ventilation and outcomes in COVID–ARDS. Our study shows that lung–protective ventilation was applied well in COVID–ARDS, and was comparable to best practice used in management for patients with CLASSIC–ARDS. Additionally, the effect of PEEP on major outcomes may have implications for care. At least it should trigger new studies that directly compare different PEEP strategies. Meanwhile, it could be more attractive to not use higher PEEP by default.
Our study has several strengths. We managed to receive and merge the datasets of four large observational studies of ventilation conducted in the COVID–19 pandemic with two well–performed pre–pandemic observational studies of ventilation––these six studies all focused on ventilation management and reported outcomes of invasively ventilated ARDS patients, allowing a robust analysis of ventilation management and the impact of certain ventilation parameters on outcome. While the COVID–19 studies were all national investigations, they are from different regions worldwide and were conducted in different types of hospitals, which increases the generalizability of our findings. The datasets from the original studies were rich and comprehensive, encompassing baseline and demographic data, granular ventilator settings and ventilation variables, and key clinical outcomes. All data could be harmonized and merged into one database.
We had an analysis plan in place before cleaning and closing of the new database, and this plan was strictly followed. The large numbers of patients allowed us to perform sophisticated statistical analyses of associations with outcomes.
This study has limitations. First, individual data was obtained from observational studies, which limits the ability to establish causality. Additionally, the willingness of data sharing could have led to selection bias towards the inclusion of ICUs with an interest in invasive ventilation and management of ARDS in the original studies. Second, studies in COVID–ARDS were conducted early in the COVID–19 pandemic, during which inexperienced staff and resource limitations could have influenced clinical decision making. Third, data was collected early in the pandemic when patient care took priority over data collection, resulting in more missing data than in previous studies. This affects the completeness and may impact the accuracy of our analysis. Fourth, we only reported on ventilation characteristics on day 1 and 2, because not all studies collected ventilation data beyond this timepoint. Therefore we were not able to compare ventilation management beyond day 2. Nevertheless, previous studies have shown ventilation characteristics don’t significantly change in the first four days after initiation of invasive ventilation [ 10 ]. Fifth, it is imperative to acknowledge the temporal distance between comparator cohorts. For the pre–COVID ARDS group we used patients of which data was collected between seven to nine years before the pandemic. We cannot exclude temporal differences, for instance due to studies that showed the importance of limiting liberal use of oxygen, and reducing the intensity of ventilation, e.g., by targeting a low driving pressure or a low mechanical power of ventilation, as well as the importance of early use of prone positioning. Sixth, is the lack of detailed subgroup analyses, particularly in patients with chronic respiratory comorbidities such as COPD. Although recent findings from a post–hoc analysis of the PRoVENT–COVID study by Tripipitsiriwat et al. [ 35 ] indicated that ventilation parameters did not show significant differences between COPD and non–COPD patients, it could be interesting to explore these subgroups. However, it was beyond the scope of our primary endpoint. Conducting such detailed subgroup investigations would require careful consideration to ensure the data from all included studies are appropriate for this type of analysis.
Finally, all COVID–19 ARDS patients, by definition, had a viral pneumonia, while patients in the classic ARDS group had respiratory infections of which the pathogen was not collected. This is an important limitation, as ARDS from a viral respiratory infection may differ from ARDS due to bacterial pneumonia. Consistent with other studies comparing COVID–19 ARDS to ARDS caused by other viruses, we found that the duration of ventilation was longer, and mortality was higher [ 21 , 36 , 37 ].
Epidemiology and key ventilation characteristics were different in patients with COVID–ARDS compared to CLASSIC–ARDS, also ΔP was lower in COVID––ARDS patients. ΔP had an independent association with outcome in both groups, whereas PEEP had an independent association with outcome only in COVID–ARDS patients.
Availability of data and materials
Data sharing: A de–identified dataset can be made available upon request to the corresponding authors one year after publication of this study, but only after permission of the principal investigators of all original studies. The request must include a statistical analysis plan.
Chiumello D, Busana M, Coppola S, Romitti F, Formenti P, Bonifazi M, Pozzi T, Palumbo MM, Cressoni M, Herrmann P, Meissner K, Quintel M, Camporota L, Marini JJ, Gattinoni L. Physiological and quantitative CT-scan characterization of COVID-19 and typical ARDS: a matched cohort study. Intensive Care Med. 2020;46:2187–96.
Article CAS PubMed PubMed Central Google Scholar
Grasselli G, Tonetti T, Protti A, Langer T, Girardis M, Bellani G, Laffey J, Carrafiello G, Carsana L, Rizzuto C, Zanella A, Scaravilli V, Pizzilli G, Grieco DL, Di Meglio L, de Pascale G, Lanza E, Monteduro F, Zompatori M, Filippini C, Locatelli F, Cecconi M, Fumagalli R, Nava S, Vincent JL, Antonelli M, Slutsky AS, Pesenti A, Ranieri VM. Pathophysiology of COVID-19-associated acute respiratory distress syndrome: a multicentre prospective observational study. Lancet Respir Med. 2020;8:1201–8.
Grasselli G, Zangrillo A, Zanella A, Antonelli M, Cabrini L, Castelli A, Cereda D, Coluccello A, Foti G, Fumagalli R, Iotti G, Latronico N, Lorini L, Merler S, Natalini G, Piatti A, Ranieri MV, Scandroglio AM, Storti E, Cecconi M, Pesenti A. Baseline Characteristics and Outcomes of 1591 Patients Infected With SARS-CoV-2 Admitted to ICUs of the Lombardy Region, Italy. JAMA. 2020;323:1574–81.
Gattinoni L, Chiumello D, Rossi S. COVID-19 pneumonia: ARDS or not? Crit Care. 2020;24:154.
Article PubMed PubMed Central Google Scholar
Le Pape M, Besnard C, Acatrinei C, Guinard J, Boutrot M, Genève C, Boulain T, Barbier F. Clinical impact of ventilator-associated pneumonia in patients with the acute respiratory distress syndrome: a retrospective cohort study. Ann Intensive Care. 2022;12:24.
Kutsogiannis DJ, Alharthy A, Balhamar A, Faqihi F, Papanikolaou J, Alqahtani SA, Memish ZA, Brindley PG, Brochard L, Karakitsos D. Mortality and Pulmonary Embolism in Acute Respiratory Distress Syndrome From COVID-19 vs. Non-COVID-19. Front Med (Lausanne). 2022;9:800241.
Article PubMed Google Scholar
Laffey JG, Bellani G, Pham T, Fan E, Madotto F, Bajwa EK, Brochard L, Clarkson K, Esteban A, Gattinoni L, van Haren F, Heunks LM, Kurahashi K, Laake JH, Larsson A, McAuley DF, McNamee L, Nin N, Qiu H, Ranieri M, Rubenfeld GD, Thompson BT, Wrigge H, Slutsky AS, Pesenti A. Potentially modifiable factors contributing to outcome from acute respiratory distress syndrome: the LUNG SAFE study. Intensive Care Med. 2016;42:1865–76.
Article CAS PubMed Google Scholar
Azevedo LC, Park M, Salluh JI, Rea-Neto A, Souza-Dantas VC, Varaschin P, Oliveira MC, Tierno PF, dal-Pizzol F, Silva UV, Knibel M, Nassar AP Jr, Alves RA, Ferreira JC, Teixeira C, Rezende V, Martinez A, Luciano PM, Schettino G, Soares M. Clinical outcomes of patients requiring ventilatory support in Brazilian intensive care units: a multicenter, prospective, cohort study. Crit Care. 2013;17:R63.
Bellani G, Laffey JG, Pham T, Fan E, Brochard L, Esteban A, Gattinoni L, van Haren F, Larsson A, McAuley DF, Ranieri M, Rubenfeld G, Thompson BT, Wrigge H, Slutsky AS, Pesenti A. Epidemiology, Patterns of Care, and Mortality for Patients With Acute Respiratory Distress Syndrome in Intensive Care Units in 50 Countries. JAMA. 2016;315:788–800.
Botta M, Tsonas AM, Pillay J, Boers LS, Algera AG, Bos LDJ, Dongelmans DA, Hollmann MW, Horn J, Vlaar APJ, Schultz MJ, Neto AS, Paulus F. Ventilation management and clinical outcomes in invasively ventilated patients with COVID-19 (PRoVENT-COVID): a national, multicentre, observational cohort study. Lancet Respir Med. 2021;9:139–48.
Ferreira JC, Ho YL, Besen B, Malbuisson LMS, Taniguchi LU, Mendes PV, Costa ELV, Park M, Daltro-Oliveira R, Roepke RML, Silva JM Jr, Carmona MJC, Carvalho CRR, Hirota A, Kanasiro AK, Crescenzi A, Fernandes AC, Miethke-Morais A, Bellintani AP, Canasiro AR, Carneiro BV, Zanbon BK, Batista B, Nicolao BR, Besen B, Biselli B, Macedo BR, Toledo CMG, Pompilio CE, Carvalho CRR, Mol CG, Stipanich C, Bueno CG, Garzillo C, Tanaka C, Forte DN, Joelsons D, Robira D, Costa ELV, Silva EMJ, Regalio FA, Segura GC, Marcelino GB, Louro GS, Ho YL, Ferreira IA, Gois JO, Silva JMJ, Reusing JOJ, Ribeiro JF, Ferreira JC, Galleti KV, Silva KR, Isensee LP, Oliveira LS, Taniguchi LU, Letaif LS, Lima LT, Park LY, Chaves LN, Nobrega LC, Haddad L, Hajjar L, Malbouisson LM, Pandolfi MCA, Park M, Carmona MJC, Andrade M, Santos MM, Bateloche MP, Suiama MA, Oliveira MF, Sousa ML, Louvaes M, Huemer N, Mendes P, Lins PRG, Santos PG, Moreira PFP, Guazzelli RM, Reis RB, Oliveira RD, Roepke RML, Pedro RAM, Kondo R, Rached SZ, Fonseca SRS, Borges TS, Ferreira T, Cobello VJ, Sales VVT, Ferreira WSC. Characteristics and outcomes of patients with COVID-19 admitted to the ICU in a university hospital in São Paulo. Brazil - study protocol Clinics (Sao Paulo). 2020;75:e2294.
Ferreira JC, Ho YL, Besen B, Malbouisson LMS, Taniguchi LU, Mendes PV, Costa ELV, Park M, Daltro-Oliveira R, Roepke RML, Silva-Jr JM, Carmona MJC, Carvalho CRR. Protective ventilation and outcomes of critically ill patients with COVID-19: a cohort study. Ann Intensive Care. 2021;11:92.
CAS PubMed PubMed Central Google Scholar
Torres A, Arguimbau M, Bermejo-Martín J, Campo R, Ceccato A, Fernandez-Barat L, Ferrer R, Jarillo N, Lorente-Balanza J, Menéndez R, Motos A, Muñoz J, Peñuelas Rodríguez Ó, Pérez R, Riera J, Rodríguez A, Sánchez M. Barbe F [CIBERESUCICOVID: A strategic project for a better understanding and clinical management of COVID-19 in critical patients]. Arch Bronconeumol. 2021;57:1–2.
Estenssoro E, Loudet CI, Ríos FG, KanooreEdul VS, Plotnikow G, Andrian M, Romero I, Piezny D, Bezzi M, Mandich V, Groer C, Torres S, Orlandi C, RubattoBirri PN, Valenti MF, Cunto E, Sáenz MG, Tiribelli N, Aphalo V, Reina R, Dubin A. Clinical characteristics and outcomes of invasively ventilated patients with COVID-19 in Argentina (SATICOVID): a prospective, multicentre cohort study. Lancet Respir Med. 2021;9:989–98.
Schuijt MTU, Hol L, Nijbroek SG, Ahuja S, van Meenen D, Mazzinari G, Hemmes S, Bluth T, Ball L, Gama-de Abreu M, Pelosi P, Schultz MJ, Serpa NA. Associations of dynamic driving pressure and mechanical power with postoperative pulmonary complications-posthoc analysis of two randomised clinical trials in open abdominal surgery. EClinicalMedicine. 2022;47:101397.
van Meenen DMP, Algera AG, Schuijt MTU, Simonis FD, van der Hoeven SM, Neto AS, Abreu MG, Pelosi P, Paulus F, Schultz MJ. Effect of mechanical power on mortality in invasively ventilated ICU patients without the acute respiratory distress syndrome: An analysis of three randomised clinical trials. Eur J Anaesthesiol. 2023;40:21–8.
Gattinoni L, Tonetti T, Cressoni M, Cadringher P, Herrmann P, Moerer O, Protti A, Gotti M, Chiurazzi C, Carlesso E, Chiumello D, Quintel M. Ventilator-related causes of lung injury: the mechanical power. Intensive Care Med. 2016;42:1567–75.
Sinha P, Fauvel NJ, Singh S, Soni N. Ventilatory ratio: a simple bedside measure of ventilation. Br J Anaesth. 2009;102:692–7.
Yehya N, Harhay MO, Curley MAQ, Schoenfeld DA, Reeder RW. Reappraisal of Ventilator-Free Days in Critical Care Research. Am J Respir Crit Care Med. 2019;200:828–36.
van Meenen DMP, van der Hoeven SM, Binnekade JM, de Borgie C, Merkus MP, Bosch FH, Endeman H, Haringman JJ, van der Meer NJM, Moeniralam HS, Slabbekoorn M, Muller MCA, Stilma W, van Silfhout B, Neto AS, Ter Haar HFM, Van Vliet J, Wijnhoven JW, Horn J, Juffermans NP, Pelosi P, Gama de Abreu M, Schultz MJ, Paulus F. Effect of On-Demand vs Routine Nebulization of Acetylcysteine With Salbutamol on Ventilator-Free Days in Intensive Care Unit Patients Receiving Invasive Ventilation: A Randomized Clinical Trial. Jama. 2018; 319:993–1001.
Richards-Belle A, Orzechowska I, Gould DW, Thomas K, Doidge JC, Mouncey PR, Christian MD, Shankar-Hari M, Harrison DA, Rowan KM. COVID-19 in critical care: epidemiology of the first epidemic wave across England, Wales and Northern Ireland. Intensive Care Med. 2020;46:2035–47.
Sjoding MW, Admon AJ, Saha AK, Kay SG, Brown CA, Co I, Claar D, McSparron JI, Dickson RP. Comparing Clinical Features and Outcomes in Mechanically Ventilated Patients with COVID-19 and Acute Respiratory Distress Syndrome. Ann Am Thorac Soc. 2021;18:1876–85.
Nolley EP, Sahetya SK, Hochberg CH, Hossen S, Hager DN, Brower RG, Stuart EA, Checkley W. Outcomes Among Mechanically Ventilated Patients With Severe Pneumonia and Acute Hypoxemic Respiratory Failure From SARS-CoV-2 and Other Etiologies. JAMA Netw Open. 2023;6:e2250401.
Brown-Brumfield D, DeLeon A. Adherence to a medication safety protocol: current practice for labeling medications and solutions on the sterile field. Aorn j. 2010;91:610–7.
Costa ELV, Slutsky AS, Brochard LJ, Brower R, Serpa-Neto A, Cavalcanti AB, Mercat A, Meade M, Morais CCA, Goligher E, Carvalho CRR, Amato MBP. Ventilatory Variables and Mechanical Power in Patients with Acute Respiratory Distress Syndrome. Am J Respir Crit Care Med. 2021;204:303–11.
Guérin C, Beuret P, Constantin JM, Bellani G, Garcia-Olivares P, Roca O, Meertens JH, Maia PA, Becher T, Peterson J, Larsson A, Gurjar M, Hajjej Z, Kovari F, Assiri AH, Mainas E, Hasan MS, Morocho-Tutillo DR, Baboi L, Chrétien JM, François G, Ayzac L, Chen L, Brochard L, Mercat A. A prospective international observational prevalence study on prone positioning of ARDS patients: the APRONET (ARDS Prone Position Network) study. Intensive Care Med. 2018;44:22–37.
Amato MB, Meade MO, Slutsky AS, Brochard L, Costa EL, Schoenfeld DA, Stewart TE, Briel M, Talmor D, Mercat A, Richard JC, Carvalho CR, Brower RG. Driving pressure and survival in the acute respiratory distress syndrome. N Engl J Med. 2015;372:747–55.
van Meenen DMP, SerpaNeto A, Paulus F, Merkies C, Schouten LR, Bos LD, Horn J, Juffermans NP, Cremer OL, van der Poll T, Schultz MJ. The predictive validity for mortality of the driving pressure and the mechanical power of ventilation. Intensive Care Med Exp. 2020;8:60.
Urner M, Jüni P, Hansen B, Wettstein MS, Ferguson ND, Fan E. Time-varying intensity of mechanical ventilation and mortality in patients with acute respiratory failure: a registry-based, prospective cohort study. Lancet Respir Med. 2020;8:905–13.
Valk CMA, Tsonas AM, Botta M, Bos LDJ, Pillay J, SerpaNeto A, Schultz MJ, Paulus F. Association of early positive end-expiratory pressure settings with ventilator-free days in patients with coronavirus disease 2019 acute respiratory distress syndrome: A secondary analysis of the Practice of VENTilation in COVID-19 study. Eur J Anaesthesiol. 2021;38:1274–83.
Cavalcanti AB, Suzumura É A, Laranjeira LN, Paisani DM, Damiani LP, Guimarães HP, Romano ER, Regenga MM, Taniguchi LNT, Teixeira C, Pinheiro de Oliveira R, Machado FR, Diaz-Quijano FA, Filho MSA, Maia IS, Caser EB, Filho WO, Borges MC, Martins PA, Matsui M, Ospina-Tascón GA, Giancursi TS, Giraldo-Ramirez ND, Vieira SRR, Assef M, Hasan MS, Szczeklik W, Rios F, Amato MBP, Berwanger O, Ribeiro de Carvalho CR. Effect of Lung Recruitment and Titrated Positive End-Expiratory Pressure (PEEP) vs Low PEEP on Mortality in Patients With Acute Respiratory Distress Syndrome: A Randomized Clinical Trial. Jama. 2017;318:1335–1345.
Tsolaki V, Zakynthinos GE, Makris D. The ARDSnet protocol may be detrimental in COVID-19. Crit Care. 2020;24:351.
Zampieri FG, Costa EL, Iwashyna TJ, Carvalho CRR, Damiani LP, Taniguchi LU, Amato MBP, Cavalcanti AB. Heterogeneous effects of alveolar recruitment in acute respiratory distress syndrome: a machine learning reanalysis of the Alveolar Recruitment for Acute Respiratory Distress Syndrome Trial. Br J Anaesth. 2019;123:88–95.
Constantin JM, Jabaudon M, Lefrant JY, Jaber S, Quenot JP, Langeron O, Ferrandière M, Grelon F, Seguin P, Ichai C, Veber B, Souweine B, Uberti T, Lasocki S, Legay F, Leone M, Eisenmann N, Dahyot-Fizelier C, Dupont H, Asehnoune K, Sossou A, Chanques G, Muller L, Bazin JE, Monsel A, Borao L, Garcier JM, Rouby JJ, Pereira B, Futier E. Personalised mechanical ventilation tailored to lung morphology versus low positive end-expiratory pressure for patients with acute respiratory distress syndrome in France (the LIVE study): a multicentre, single-blind, randomised controlled trial. Lancet Respir Med. 2019;7:870–80.
Tripipitsiriwat A, Suppapueng O, van Meenen DMP, Paulus F, Hollmann MW, Sivakorn C, Schultz MJ. Epidemiology, Ventilation Management and Outcomes of COPD Patients Receiving Invasive Ventilation for COVID-19-Insights from PRoVENT-COVID. J Clin Med. 2023;12.
Brinkman S, Termorshuizen F, Dongelmans DA, Bakhshi-Raiez F, Arbous MS, de Lange DW, de Keizer NF. Comparison of outcome and characteristics between 6343 COVID-19 patients and 2256 other community-acquired viral pneumonia patients admitted to Dutch ICUs. J Crit Care. 2022;68:76–82.
Virk S, Quazi MA, Nasrullah A, Shah A, Kudron E, Chourasia P, Javed A, Jain P, Gangu K, Cheema T, DiSilvio B, Sheikh AB. Comparing Clinical Outcomes of COVID-19 and Influenza-Induced Acute Respiratory Distress Syndrome: A Propensity-Matched Analysis. Viruses. 2023;15.
Download references
Acknowledgements
for the ERICC a –,LUNG SAFE b –, PRoVENT–COVID c –, EPICCoV d –, CIBERESUCICOVID e – and SATI–COVID–19 f –investigators
a ERICC, ‘Epidemiology of Respiratory Insufficiency in Critical Care’
b LUNG SAFE, ‘Large Observational Study to UNderstand the Global Impact of Severe Acute Respiratory FailurE’
c PRoVENT–COVID, ‘Practice of Ventilation in COVID–19 patients’
d EPICCoV, EPIdemiology of Critical COVID–19
e CIBERESUCICOVID, ‘Centro de Investigación Biomédica en Red Enfermedades Respiratorias COVID–19’
f SATI–COVID–19, ‘Sociedad Argentina de Terapia Intensiva–COVID–19’
No additional funding was received for this analysis.
Author information
Fleur–Stefanie L. I. M. van der Ven and Siebe G. Blok contributed equally to this work.
Authors and Affiliations
Department of Intensive Care, Amsterdam University Medical Centers, Location ‘AMC’, Meibergdreef 9, 1105, AZ, Amsterdam, The Netherlands
Fleur–Stefanie L. I. M. van der Ven, Siebe G. Blok, Michela Botta, Luigi Pisani, Marcus J. Schultz, Anissa M. Tsonas, Frederique Paulus & David M. P. van Meenen
Department of Intensive Care, Rode Kruis Ziekenhuis, Beverwijk, The Netherlands
Fleur–Stefanie L. I. M. van der Ven
Department of Emergency Medicine, Hospital das Clinicas HCFMUSP, Faculdade de Medicina, Universidade de São Paulo, São Paulo, Brazil
Luciano C. Azevedo
Department of Intensive Care, Hospital Israelita Albert Einstein, São Paulo, Brazil
Luciano C. Azevedo & Ary Serpa Neto
Centre for Medical Sciences (CISMed), University of Trento, Trento, Italy
Giacomo Bellani
Department of Anesthesia and Intensive Care, Santa Chiara Hospital, APSS Trento, Trento, Italy
Department of Intensive Care, Hospital Interzonal de Agudos General San Martin La Plata, Buenos Aires, Argentina
Elisa Estenssoro
Interdepartmental Division of Critical Care Medicine, University of Toronto, Toronto, ON, Canada
Department of Pulmonology, Instituto Do Coracao (InCor), Hospital das Clinicas HCFMUSP, Faculdade de Medicina, Universidade de Sao Paulo, São Paulo, Brazil
Juliana Carvalho Ferreira
Department of Intensive Care, AC Camargo Cancer Center, São Paulo, Brazil
Brazilian Research in Intensive Care Network (BRICNet), São Paulo, Brazil
Department of Anaesthesiology and Intensive Care, Galway University Hospital, Saolta Hospital Group, Galway, Ireland
John G. Laffey
School of Medicine, University of Galway, Galway, Ireland
Department of Intensive Care, Multidisciplinary Intensive Care Research Organization (MICRO), St James’ Hospital, Dublin, Ireland
Ignacio Martin–Loeches
Department of Intensive Care, Hospital Clínic de Barcelona, Barcelona, Spain
Departement of Pulmonology, Institut d’Investigacions Biomèdiques August Pi I Sunyer (IDIBAPS), Hospital Clínic de Barcelona, Barcelona, Spain
Ana Motos & Antoni Torres
Centro de Investigación Biomédica en Red en Enfermedades Respiratorias (CIBERES), Institute of Health Carlos III, Madrid, Spain
Ana Motos, Oscar Peñuelas & Antoni Torres
Equipe d’Epidémiologie Respiratoire Integrative, Université Paris–Saclay, Paris, France
Service de Médecine Intensive-Réanimation, DMU CORREVE, FHU SEPSIS, Groupe de Recherche Clinique CARMAS, Hôpital de Bicêtre, Paris, France
Department of Intensive Care, Hospital Universitario de Getafe, Getafe, Spain
Oscar Peñuelas
Fondazione IRCCS Ca’ Granda Ospedale Maggiore Policlinico, Milan, Italy
Antonio Pesenti
Department of Anesthesia and Intensive Care, Miulli Regional Hospital, Acquaviva Delle Fonti, Italy
Luigi Pisani
Australian and New Zealand Intensive Care Research Centre (ANZIC–RC), Monash University, Melbourne, Australia
Ary Serpa Neto
Mahidol–Oxford Tropical Medicine Research Unit (MORU), Mahidol University, Bangkok, Thailand
Luigi Pisani & Marcus J. Schultz
Nuffield Department of Medicine, University of Oxford, Oxford, UK
Marcus J. Schultz
Department of Anesthesia, General Intensive Care and Pain Management, Division of Cardiothoracic and Vascular Anesthesia & Critical Care Medicine, Medical University of Vienna, Vienna, Austria
Laboratory of Experimental Intensive Care & Anaesthesiology (L·E·I·C·A), Amsterdam UMC, Location AMC, Amsterdam, The Netherlands
University of Barcelona, Barcelona, Spain
Catalan Institution for Research and Advanced Studies (ICREA), Barcelona, Spain
Antoni Torres
Center of Expertise Urban Vitality, Faculty of Health, Amsterdam University of Applied Sciences, Amsterdam, The Netherlands
Frederique Paulus
Department of Anaesthesiology, Amsterdam UMC, Location AMC, Amsterdam, The Netherlands
David M. P. van Meenen
You can also search for this author in PubMed Google Scholar
Contributions
Author contribution: FV, SB, MS, FP and DM had full access to all the data and take responsibility for the integrity of the data and the accuracy of the data analysis. Concept and design: All authors Acquisition, analysis, or interpretation of data: FV, SB, MS, FP and DM Drafting of the manuscript: FV, SB, MS, FP and DM Critical revision of the manuscript for important intellectual content: All authors Statistical analysis and data verification: FV, SB, and DM Obtained funding: Not applicable; the original studies were performed with funding as stated in the original reports. Administrative, technical, or material support: LA, GB, MB, EE, JF, JL, TP, AT Supervision: MS, FP and DM.
Corresponding author
Correspondence to Fleur–Stefanie L. I. M. van der Ven .
Ethics declarations
Ethics approval and consent to participate.
The creation of the pooled database did not require additional ethical approval.
Consent for publication
Not applicable.
Competing interests
The authors declare no competing interests.
Additional information
Publisher’s note.
Springer Nature remains neutral with regard to jurisdictional claims in published maps and institutional affiliations.
Supplementary Information
Supplementary material 1, rights and permissions.
Open Access This article is licensed under a Creative Commons Attribution 4.0 International License, which permits use, sharing, adaptation, distribution and reproduction in any medium or format, as long as you give appropriate credit to the original author(s) and the source, provide a link to the Creative Commons licence, and indicate if changes were made. The images or other third party material in this article are included in the article's Creative Commons licence, unless indicated otherwise in a credit line to the material. If material is not included in the article's Creative Commons licence and your intended use is not permitted by statutory regulation or exceeds the permitted use, you will need to obtain permission directly from the copyright holder. To view a copy of this licence, visit http://creativecommons.org/licenses/by/4.0/ . The Creative Commons Public Domain Dedication waiver ( http://creativecommons.org/publicdomain/zero/1.0/ ) applies to the data made available in this article, unless otherwise stated in a credit line to the data.
Reprints and permissions
About this article
Cite this article.
van der Ven, F.L.I.M., Blok, S.G., Azevedo, L.C. et al. Epidemiology, ventilation management and outcomes of COVID–19 ARDS patients versus patients with ARDS due to pneumonia in the Pre–COVID era. Respir Res 25 , 312 (2024). https://doi.org/10.1186/s12931-024-02910-2
Download citation
Received : 24 December 2023
Accepted : 07 July 2024
Published : 17 August 2024
DOI : https://doi.org/10.1186/s12931-024-02910-2
Share this article
Anyone you share the following link with will be able to read this content:
Sorry, a shareable link is not currently available for this article.
Provided by the Springer Nature SharedIt content-sharing initiative
- Acute respiratory distress syndrome
- Critical care
- Mechanical ventilation
- Ventilation management
Respiratory Research
ISSN: 1465-993X
- General enquiries: [email protected]

Reassessing the management of uncomplicated urinary tract infection: A retrospective analysis using machine learning causal inference
- Find this author on Google Scholar
- Find this author on PubMed
- Search for this author on this site
- ORCID record for Ming-Chieh Shih
- ORCID record for Sanjat Kanjilal
- For correspondence: [email protected]
- Info/History
- Supplementary material
- Preview PDF
Background Uncomplicated urinary tract infection (UTI) is a common indication for outpatient antimicrobial therapy. National guidelines for the management of uncomplicated UTI were published by the Infectious Diseases Society of America in 2011, however it is not fully known the extent to which they align with current practices, patient diversity, and pathogen biology, all of which have evolved significantly in the time since their publication.
Objective We aimed to re-evaluate efficacy and adverse events for first-line antibiotics (nitrofurantoin, and trimethoprim-sulfamethoxazole), versus second-line antibiotics (fluoroquinolones) and versus alternative agents (oral β-lactams) for uncomplicated UTI in contemporary clinical practice by applying machine learning algorithms to a large claims database formatted into the Observational Medical Outcomes Partnership (OMOP) common data model.
Outcomes Our primary outcome was a composite endpoint for treatment failure, defined as outpatient or inpatient re-visit within 30 days for UTI, pyelonephritis or sepsis. Secondary outcomes were the risk of 4 common antibiotic-associated adverse events: gastrointestinal symptoms, rash, kidney injury and C. difficile infection.
Statistical methods We adjusted for covariate-dependent censoring and treatment indication using a broad set of domain-expert derived features. Sensitivity analyses were conducted using OMOP-learn , an automated feature engineering package for OMOP datasets.
Results Our study included 57,585 episodes of UTI from 49,037 patients. First-line antibiotics were prescribed in 35,018 (61%) episodes, second-line antibiotics were prescribed in 21,140 (37%) episodes and alternative antibiotics were prescribed in 1,427 (2%) episodes. After adjustment, patients receiving first-line therapies had an absolute risk difference of -2.1% [95% CI -2.9% to -1.6%] for having a revisit for UTI within 30 days of diagnosis relative to second-line antibiotics. First-line therapies had an absolute risk difference of -6.6% [95% CI -9.4% to -3.8%] for 30-day revisit compared to alternative β-lactam antibiotics. Differences in adverse events were clinically similar between first and second line agents, but lower for first-line agents relative to alternative antibiotics (−3.5% [95% CI -5.9% to -1.2%]). Results were similar for models built with OMOP-learn .
Conclusion Our study provides support for the continued use of first-line antibiotics for the management of uncomplicated UTI. Our results also provide proof-of-principle that automated feature extraction methods for OMOP formatted data can emulate manually curated models, thereby promoting reproducibility and generalizability.
Competing Interest Statement
SDA reports support from Centers for Disease Control and Prevention SHEPheRD 75D30121D12733-D5-E003 (grant no. 5U54CK000616-02), the Society for Healthcare Epidemiology of America, and the Duke Claude D. Pepper Older Americans Independence Center (National Institute on Aging grant no. P30AG028716), as well as consulting fees from Locus Biosciences, Sysmex America, GlaxoSmithKline, bioMerieux, and the Infectious Diseases Society of America. SDA became an employee of GSK/ViiV Healthcare one year after her contribution to this project.
Funding Statement
EH was supported by the National Science Foundation Graduate Research Fellowship Program (grant no. 2141064). SDA was supported by the National Institute of Diabetes and Digestive and Kidney Diseases (grant no. K12DK100024). DS and MCS were supported in part by grants from Independence Blue Cross and the Office of Naval Research (grant no. N00014-21-1-2807). SK was supported by AHRQ (grant no. K08 HS027841-01A1).
Author Declarations
I confirm all relevant ethical guidelines have been followed, and any necessary IRB and/or ethics committee approvals have been obtained.
The details of the IRB/oversight body that provided approval or exemption for the research described are given below:
This study was deemed exempt by the Institutional Review Board of the Massachusetts Institute of Technology (protocol E-3970)
I confirm that all necessary patient/participant consent has been obtained and the appropriate institutional forms have been archived, and that any patient/participant/sample identifiers included were not known to anyone (e.g., hospital staff, patients or participants themselves) outside the research group so cannot be used to identify individuals.
I understand that all clinical trials and any other prospective interventional studies must be registered with an ICMJE-approved registry, such as ClinicalTrials.gov. I confirm that any such study reported in the manuscript has been registered and the trial registration ID is provided (note: if posting a prospective study registered retrospectively, please provide a statement in the trial ID field explaining why the study was not registered in advance).
I have followed all appropriate research reporting guidelines, such as any relevant EQUATOR Network research reporting checklist(s) and other pertinent material, if applicable.
↵ * Co-last author
Data Availability
All data produced in the present study are available upon reasonable request to the authors
View the discussion thread.
Supplementary Material
Thank you for your interest in spreading the word about medRxiv.
NOTE: Your email address is requested solely to identify you as the sender of this article.

Citation Manager Formats
- EndNote (tagged)
- EndNote 8 (xml)
- RefWorks Tagged
- Ref Manager
- Tweet Widget
- Facebook Like
- Google Plus One
Subject Area
- Infectious Diseases (except HIV/AIDS)
- Addiction Medicine (343)
- Allergy and Immunology (666)
- Anesthesia (180)
- Cardiovascular Medicine (2630)
- Dentistry and Oral Medicine (314)
- Dermatology (222)
- Emergency Medicine (397)
- Endocrinology (including Diabetes Mellitus and Metabolic Disease) (932)
- Epidemiology (12181)
- Forensic Medicine (10)
- Gastroenterology (756)
- Genetic and Genomic Medicine (4070)
- Geriatric Medicine (387)
- Health Economics (678)
- Health Informatics (2627)
- Health Policy (998)
- Health Systems and Quality Improvement (981)
- Hematology (361)
- HIV/AIDS (845)
- Infectious Diseases (except HIV/AIDS) (13662)
- Intensive Care and Critical Care Medicine (792)
- Medical Education (399)
- Medical Ethics (109)
- Nephrology (431)
- Neurology (3840)
- Nursing (209)
- Nutrition (571)
- Obstetrics and Gynecology (735)
- Occupational and Environmental Health (691)
- Oncology (2011)
- Ophthalmology (582)
- Orthopedics (239)
- Otolaryngology (304)
- Pain Medicine (250)
- Palliative Medicine (74)
- Pathology (471)
- Pediatrics (1109)
- Pharmacology and Therapeutics (460)
- Primary Care Research (448)
- Psychiatry and Clinical Psychology (3405)
- Public and Global Health (6504)
- Radiology and Imaging (1390)
- Rehabilitation Medicine and Physical Therapy (808)
- Respiratory Medicine (869)
- Rheumatology (401)
- Sexual and Reproductive Health (407)
- Sports Medicine (341)
- Surgery (441)
- Toxicology (52)
- Transplantation (185)
- Urology (165)
Designing a Context-Driven Problem-Solving Method with Metacognitive Scaffolding Experience Intervention for Biology Instruction
- Open access
- Published: 27 August 2024
Cite this article
You have full access to this open access article
- Merga Dinssa Eticha ORCID: orcid.org/0009-0008-9263-3273 1 , 2 ,
- Adula Bekele Hunde 3 &
- Tsige Ketema 1
Learner-centered instructional practices, such as the metacognitive strategies scaffolding the problem-solving method for Biology instruction, have been shown to promote students’ autonomy and self-direction, significantly enhancing their understanding of scientific concepts. Thus, this study aimed to elucidate the importance and procedures of context analysis in the development of a context-driven problem-solving method with a metacognitive scaffolding instructional approach, which enhances students’ learning effectiveness in Biology. Therefore, the study was conducted in the Biology departments of secondary schools in Shambu Town, Oromia Region, Ethiopia. The study employed mixed-methods research to collect and analyze data, involving 12 teachers and 80 students. The data collection tools used were interviews, observations, and a questionnaire. The study revealed that conducting a context analysis that involves teachers, students, and learning contexts is essential in designing a context-driven problem-solving method with metacognitive scaffolding for Biology instruction, which provides authentic examples, instructional content, and engaging scenarios for teachers and students. As a result, the findings of this study provide a practical instructional strategy that can be applied to studies aimed at designing a context-driven problem-solving method with metacognitive scaffolding with the potential to influence instructional practices.
Explore related subjects
- Artificial Intelligence
- Digital Education and Educational Technology
Avoid common mistakes on your manuscript.
Introduction
Biology is a vital subject in the Natural Sciences and enables learners to understand the mechanisms of living organisms and their practical applications for humans (Agaba, 2013 ). Therefore, Biology instruction requires interactive, learner-centered instructional methods like the problem-solving method with metacognitive scaffolding (PSMMS), which foster students to develop critical thinking, problem-solving, metacognitive, and scientific process skills (Al Azmy & Alebous, 2020 ; Inel & Balim, 2010 ) and help them make informed decisions regarding health and the environment, thereby advancing scientific knowledge (Aurah et al., 2011 ).
Although the focus is on students acquiring scientific knowledge and higher-order thinking skills (Senyigit, 2021 ), research revealed gaps in implementing the PSMMS in Biology, mainly due to the teachers’ limited experience in learner-centered methods (Agena, 2010 ; Beyessa, 2014 ), poor enhancement practices (MoE, 2019 ), tendency to use conventional problem-solving approaches (Aurah et al., 2011 ), and limited understanding of the roles of metacognition in instructional processes (Cimer, 2012 ). On the other hand, there is limited study on the importance of metacognitive instruction in scaffolding the problem-solving method in Biology, although it has a significant impact on students’ performance in mathematics and logical reasoning (Guner & Erbay, 2021 ).
In addition, metacognitive instructional strategies in primary school sciences and the contributions of metacognitive instructional intervention in developing countries are other areas where limited research has been done (Sbhatu, 2006). These challenges offer a study ground for investigating the intervention of metacognitive instructional methods in secondary schools, focusing on the problem-solving method in Biology. This study, therefore, aims to answer the research question, “How can context analysis be used to design a context-driven PSMMS and suggest PSMMS instructional guidelines to enhance students’ effective Biology learning?”
Theoretical Background
The problem-solving method.
The problem-solving method is a learner-centered approach that focuses on identifying, investigating, and solving problems (Ahmady & Nakhostin-Ruhi, 2014 ). The problem-solving method in Biology promotes advanced and critical thinking skills, enhancing students’ attitudes, academic performance, and subject understanding (Albay, 2019 ; Khaparde, 2019 ). Research has shown that students who learn using the problem-solving method outperform those who are taught conventionally (Nnorom, 2019 ). Studies have discussed that the problem-solving method encourages experimentation or learning through trial-and-error and also facilitates a constructivist learning environment by encouraging brainstorming and inquiry (e.g., Ishaku, 2015).
Metacognition
Metacognition, introduced by John Flavell in 1976, refers to an individual’s awareness, critical thinking, reflective judgment, and control of cognitive processes and strategies (Tachie, 2019 ). It consists of two main components, namely metacognitive knowledge and metacognitive regulation (Lai, 2011 ). Metacognitive knowledge involves understanding one’s own thinking, influencing performance, and effective use of methods through declarative, procedural, and conditional knowledge (Schraw et al., 2006 ; Sperling et al., 2004 ), while metacognitive regulation is about controlling thought processes and monitoring cognition, which involves planning, implementing, monitoring, and evaluating strategies (Aaltonen & Ikavalko, 2002 ; Zumbrunn et al., 2011 ).
Metacognitive instructional strategies are used to enhance learners’ effectiveness and support their learning process during the stages of forethought, performance, and self-reflection (Okoro & Chukwudi, 2011 ; Zimmerman, 2008 ). Therefore, metacognitive scaffolding, as described by Zimmerman ( 2008 ), is important in classroom interventions because it promotes problem-solving processes and supports metacognitive activities. According to Sbhatu (2006), understanding metacognitive processes and methods is fundamental for complex problem-solving tasks. Metacognitive functions are categorized based on the phases of the problem-solving method, including problem recognition, presentation, planning, execution, and evaluation (Kapa, 2001 ).
PSMMS in the Face of Globalization and Twenty-First Century Advancements
In the twenty-first century, societies rely on scientific and technological advances, and promoting scientific literacy is crucial for their integration into interactive learning environments (Chu et al., 2017 ). Studies suggest that science, technology, engineering, and mathematics (STEM) education promotes critical thinking, creativity, and problem-solving skills (Widya et al., 2019 ). Therefore, teachers should adopt a learning science and learner-centered approach and focus on higher-order thinking skills and problem-based tasks (Darling-Hammond et al., 2020 ; Nariman, 2014).
The implementation of metacognitive strategies as a scaffold system for the problem-solving method, which simultaneously fosters the development of higher-order skills in their Biology learning, helps students advance in the age of globalization and the twenty-first century. According to Chu et al. ( 2017 ), twenty-first century skills are classified into four categories, such as ways of thinking, ways of working, tools for working, and ways of living in an advanced world. Therefore, studies suggest that teachers can help students develop twenty-first century skills and influence learning through metacognition, thereby promoting self-directed learning (Stehle & Peters-Burton, 2019 ; Tosun & Senocak, 2013 ).
The Problem-Solving Method and Metacognition in Biology Instruction in Ethiopia
The National Education and Training Policy emphasizes the importance of education, particularly in science and technology, in improving problem-solving skills, cultural development, and environmental conservation for holistic development (ETP, 1994 ). Similarly, the 2009 Ethiopian Education Curriculum Framework Document highlights higher-order skills as key competencies and promotes the application, analysis, synthesis, evaluation, and innovation of knowledge for the twenty-first century (MoE, 2009 ). Whereas, a third revision of the curriculum is needed to promote science and technology studies with an emphasis on advanced cognitive skills and a shift from teacher-centered to learner-centered instructional methods (MoE, 2020 ).
The 2009 curriculum framework also places a strong emphasis on Biology as a life science, promoting understanding of self and living things while encouraging critical thinking and problem-solving. Biology lessons that integrate the problem-solving method can enhance students’ academic performance and understanding of the subject (Agaba, 2013 ). However, the Ethiopian education system faces challenges due to limited instructional resources, poor instructional methods, and a lack of experience in practical (hands-on) activities (Eshete, 2001; ETP, 1994 ; MoE, 2005 ; Negash, 2006 ). On the other hand, teachers’ inability to demonstrate effective instructional practices may contribute to low academic performance (Ganyaupfu, 2013 ; Umar, 2011 ).
Challenges in Implementing the PSMMS in Biology Instruction
Metacognitive processes are crucial for guiding learners in problem-solving activities (Sbhatu, 2006), but assessing them can be challenging due to their covert nature (Georghiades, 2000 ). Just like other areas of study, implementing metacognitive scaffolding of the problem-solving method in Biology instruction faces challenges such as complex learning, outdated skills, self-study, overloaded curricula, and limited resources, as shown in Table 1 .
Context Analysis in the Design of the PSMMS for Biology Instruction
Biology lessons are designed for different contexts and consider factors such as the learning environment, prior knowledge, background information, and cultural orientation (Reich et al., 2006 ). For this study, the three domains of context analysis (learners, learning, and learning task contexts) of Smith and Ragan’s (2005) instructional design model (as cited in Getenet, 2020 ) are adapted to design a context-based PSMMS method to generate authentic examples, strong scenarios, and instructional content, as shown in Table 2 .
Research Design
The study analyzed the learning context, including the available instructional resources and facilities in selected schools in Shambu Town, considering teachers’ and students’ perspectives using a mixed-methods research design (Creswell, 2009 ; Creswell & Creswell, 2018 ).
Study Participants
The study was conducted in public secondary schools in Shambu Town. Two schools, namely Shambu Secondary and Preparatory School (ShSPS) and Shambu Secondary School (ShSS), were selected using purposive sampling. Additionally, two Natural Sciences grade 11 sections, one from each school, were selected for instructional intervention based on feedback from context analysis to design an instructional approach, specifically the PSMMS in this study. Thus, all 12 Biology teachers and 80 eleventh-grade students participated in this study (see Table 4 ).
Data Collection Instruments and Procedure
To analyze the contexts to design a context-driven PSMMS for Biology instruction, data were collected using interviews, observations, and a questionnaire. Interviews were conducted to get insights from teachers, while observations were used to assess classroom instructions and instructional resources. Likewise, a questionnaire was administered to students to collect quantitative data on their opinions about the use of PSMMS in Biology instruction. The questionnaire, which was adapted from existing literature (Kallio et al., 2017 ; Rahmawati et al., 2018 ), was initially produced in English and subsequently translated into local language (Afan Oromo) with the help of both software (English to Oromo translator software) and experts. The questionnaire was pilot-tested on a sample of 40 students (22 males and 18 females) to identify any deficiencies in the measuring instrument, and responses were rated on a five-point Likert scale ranging from strongly agree ( N = 5) to strongly disagree ( N = 1). The reliability score of the questionnaire was determined to be 0.895, which is at a good level of acceptability.
In this design-based research (DBR) to design an instructional approach for context-driven PSMMS, the data collection process follows a context analysis procedure. Subsequently, the quantitative data collection method is based on the qualitative approach. Accordingly, assessing the context and literature was the first step in the research process. The qualitative approach used interviews and observations for data collection and was also used to identify instructional deficiencies and formulate questions for quantitative data collection.
Data Analysis
This context-based study used both qualitative and quantitative methods to analyze the data collected. In this context-based study, data analysis was conducted on the complex networks of contextual components (Wang & Hannafin, 2005 ). According to Table 2 , the domains of context analysis and key themes that emerged and were applied in this study are listed in Table 3 .
Qualitative data included interviews and notes recorded on the observation checklist. These were analyzed through thematic categorization. Each record was first transcribed, imported into Excel for filtering, and then sent back to Microsoft Word for highlighting. The transcripts were read several times to get a feel for the whole thing. The observation checklist was assessed by watching video recordings and taking notes. However, SPSS software version 24.0 was used to analyze quantitative data using descriptive and inferential statistics, including frequency, percentage, mean, standard deviation, and one-sample t-test.
Results and Discussions
In the study, a total of 12 Biology teachers participated, with 11 males and one female. As displayed in Table 4 , 41.67% of the teacher participants were from ShSPS, while 58.33% were from ShSS. The majority of these teachers had master’s degrees and had over ten years of teaching experience. As for the students involved, 52.5% were from ShSS and 47.5% were from ShSPS. The sex ratio among the students was 51.25% males and 48.75% females (Table 4 ).
Teachers’ Context Analysis
Beliefs about the practices of using the psmms in biology instruction.
The study analyzed teachers’ beliefs about the importance of the PSMMS in Biology instruction. Accordingly, most teachers interviewed (10 out of 12) stated that PSMMS improves students’ learning by enhancing their thinking skills, subject understanding, self-directed learning techniques, and behavior change, suggesting that it has a significant impact on students’ learning. About this, the study participant gave the following illustrative response:
In my opinion, using PSMMS in Biology classes improves students’ higher-order thinking skills by allowing them to understand and articulate problems in their context, stimulate reflection, and promote practical application knowledge (Teacher 4, ShSPS).
Concerning supportive learning, most of the teachers (nine out of 12) believed that it could enhance students’ engagement despite challenges in understanding and learning. About this, research participants said the following:
The PSMMS provides an engaging approach to Biology learning that promotes students’ active engagement and strengthens their awareness and understanding of the objectives and concepts they are expected to understand (Teacher 1, ShSS). Despite the challenge, I believe that using metacognitive scaffolding in the problem-solving method will help students develop their critical thinking skills. In addition, both teachers and students enjoy participating in the teaching-learning process in a classroom environment that is conducive to learning (Teacher 4, ShSPS).
The majority of teachers (eight out of 12) interviewed about PSMMS in Biology instruction argued that it is not commonly used in classrooms and instead relies on established methods like group discussions, pre-learning questions, projects, and quizzes. Some sample responses from teachers are:
The problem-solving method augmented by metacognition is crucial to learning Biology, although students and teachers have limited experience. However, motivated students using this strategy can make the Biology learning experience attractive (Teacher 2, ShSPS). Most students find learning Biology through the PSMMS a tiresome activity and believe that it is too challenging to achieve their learning goals (Teacher 1, ShSPS). The inability to implement the PSMMS in Biology learning experiences is attributed to inadequate laboratory equipment, teaching aids, and school facilities (Teacher 7, ShSS). On some occasions, I provide students with classwork, plans for implementing teaching strategies, arrange group discussions, and assist them in practicing subject-related skills. I then provide background information, promote class engagement, guide responses to questions, assess students’ existing knowledge and goals, provide relevant comments, and guide their thinking (Teacher 4, ShSPS).
Based on the results of the data analysis, it was found that teachers’ perceptions of the importance of the PSMMS to students’ Biology learning contributed significantly to the analysis of the learning context. Accordingly, the contribution of the PSMMS was to enhance students’ Biology learning by improving their critical thinking and learning experiences. Consistent with these findings, teachers’ positive beliefs about classroom problem-solving processes influence their approach to effective Biology teaching (Ishaku, 2015), and integrating metacognitive classroom interventions improves student learning, as evidenced by changes in conceptual learning and problem-solving skills (Guterman, 2002 ; Howard et al., 2001 ).
Observation of Teachers’ Classroom Instruction
The classroom instructional situation was observed to examine the effectiveness of PSMMS for Biology instruction. Consequently, teachers’ use of the PSMMS in Biology lessons was observed. According to the observation checklist, a total of 12 lessons, each lasting 40 minutes, were audited. The first step was to examine teachers’ daily lesson plans. Objectives were found to center predominantly on cognitive domains, neglecting higher-order problem-solving and metacognitive skills. This was evident from the use of terms such as “understand,” “know,” “write,” “explain,” and “describe” in the lesson plan objectives, which hold little significance for teaching Biology using the PSMMS. This finding is consistent with previous research (Chandio et al., 2016 ; Hyder & Bhamani, 2016 ) showing that the objectives of classroom lesson plans often focus on the lower cognitive domain, indicating lower-level knowledge acquisition.
Observing how teachers deliver lessons in the classroom revealed that they often require students to participate in group discussions, which they believe is a learner-centered approach. However, student engagement was limited, and the details of the tasks that students were expected to discuss were not outlined. Additionally, in the lessons observed, teachers failed to engage students, connect theory with practical applications, or support activity-based learning. On the other hand, teachers still have limited opportunities to assess understanding through targeted questions and encourage the use of critical thinking skills. Only oral questions, tests, or quizzes are used as an assessment method. These results were contradictory to the findings of other researchers’ studies, such as Ahmady and Nakhostin-Ruhi ( 2014 ) and Ishaku (2015), where teachers’ classroom lesson delivery is based on students’ constructivist and learner-centered environment acquiring advanced and critical thinking skills from Biology lessons.
The observation raised further questions regarding multimodal lesson delivery, revealing the use of visual representations of figures and diagrams in addition to the usual lecture style (auditory), raising additional concerns about multimodal instructional delivery. Therefore, there was no way to verify whether students had acquired the required higher-order skills, such as problem-solving and metacognitive skills, during their Biology learning. This finding contradicts the findings of Syofyan and Siwi’s ( 2018 ) research, which claims that students’ learning approaches are influenced by their sensory experiences. Consequently, students employ all their senses to capture information when teachers employ visual, auditory, and kinesthetic learning styles.
Students’ Context Analysis
The section presents the results of students’ responses collected using survey questions. Using a questionnaire with a five-point Likert scale ranging from strongly agree to strongly disagree (5 = strongly agree, 4 = agree, 3 = neutral, 2 = disagree, and 1 = strongly disagree), the impact of using PSMMS in Biology learning practices on students’ problem-solving and metacognitive skills was examined. The questionnaire had a response rate of 80 out of 98 (81.63%), indicating satisfactory status and acceptable use of the instrument. Therefore, in students’ responses to the survey questions on Biology learning practices using the PSMMS, there is significant ( p < 0.05) variation across all dimensions of the items (M = 4.32, SD = 1.30), with mean scores above 4 indicating general students’ agreement with most items listed in Table 5 .
Regarding the problem-solving skills (Items 1–5) that students would acquire in their Biology learning practices using the PSMMS in Biology lessons, the strongest agreement was to investigate and identify the most effective problem-solving strategies (Item 4, M = 4.25, SD = 1.11), followed by creating the framework and design of the problem-solving activities (Item 2, M = 4.05, SD = 1.16), appropriately evaluating the results and providing alternative solutions to the problems (Item 5, M = 3.91, SD = 1.21), and identifying the problem in the problem sketch and interpreting the final result (Item 1, M = 3.90, SD = 1.28). On the other hand, students typically expressed less positive views about the PSMMS’s use of Biology instruction to enhance laboratory knowledge and problem-solving skills (Item 3, M = 3.25, SD = 1.57), despite significant differences in response patterns (Table 5 ).
Concerning students’ responses to the questionnaire items on metacognitive skills (Items 6–15) acquired in their Biology learning practices using the PSMMS, Table 5 shows that the most positive item states that the use of the PSMMS helps set clear learning objectives (Item 7, M = 4.36, SD = 1.09) and evaluates success by asking how well they did (Item 15, M = 4.29, SD = 1.10). Students tended to be less positive about learning Biology using the PSMMS, which is used to create examples and diagrams to make information more meaningful (Item 9, M = 3.83, SD = 1.21), despite the wide range of response patterns (Table 5 ). As a result, using PSMMS in Biology instruction helps students learn essential planning (Items 6–8), implementing (Items 9 and 10), monitoring (Items 11 and 12), and evaluating (Items 13–15) strategies for practice and to learn real-world applications of Biology (Table 5 ).
After data analysis of students’ responses to the survey questions, it was found that the PSMMS instructional approach is effective in helping students acquire problem-solving and metacognitive skills in their Biology learning practices. However, teachers’ responses, classroom observations, and resource availability indicated that the PSMMS approach was not effectively used to improve students’ problem-solving skills and strategies in Biology learning. The study highlights the disadvantages of shortages of laboratory facilities and large class sizes when implementing learner-centered practices in schools. These issues are supported by Kawishe’s (2016) study. Additionally, the PSMMS was not effectively applied in Biology instruction, resulting in students’ inability to develop metacognitive strategies and skills. Therefore, as studies have shown, students face challenges in acquiring metacognitive knowledge and regulation, which are crucial for the development of higher-order thinking skills in Biology learning (Aaltonen & Ikavalko, 2002 ; Lai, 2011 ).
Learning Context Analysis
This section presents the learning context analysis of PSMMS-based Biology instruction for two aspects, namely the availability of instructional resources in laboratories and pedagogical centers and the challenges in implementing the PSMMS in Biology instruction at Shambu Secondary and Preparatory School (ShSPS) and Shambu Secondary School (ShSS). Each is described below.
Availability of Instructional Resources in the Laboratories and Pedagogical Centers
In this section, a physical observation was conducted to assess the availability of instructional resources in Biology laboratories and pedagogical centers. The observation checklists were used to examine the impacts of their availability on Biology instruction using PSMMS.
Concerning the observations of the laboratory resources, it was noted that the two schools have independent Biology laboratories, but their functioning is hindered by poor organization, display tables, and a lack of water supply and waste disposal systems, as shown in Table 6 . Some basic laboratory equipment and chemicals, including dissecting kits, centrifuges, measuring cylinders, protein foods, sodium hydroxide solution, 1% copper (II) sulfate solution, gas syringes, and hydrogen peroxide, are missing. One school, ShSS, has only seven resources out of 20 identified for observation, making it difficult to conduct laboratory activities (Table 6 ).
Regarding the observations of instructional or teaching resources in the pedagogical centers, the results are shown in Table 7 . The results showed that there were no independent or autonomous pedagogical centers in the two schools; instead, they used the Biology department offices as a pedagogical center and kept some teaching and learning aids there. On the other hand, only DNA and RNA models were accessible in ShSPS, while models of DNA and RNA as well as illustrations depicting the organization of animal cell structures were available in ShSS (Table 7 ).
Challenges of Using the PSMMS in Biology Instruction
In this case, the results of interviews with teachers and survey results from students about the challenges they encountered when using the PSMMS in Biology instruction were used. The results of teachers’ and students’ responses are described below.
Teachers’ interview responses regarding the challenges they encountered in implementing the PSMMS in Biology instruction served as the basis for teachers’ perspectives . With the exception of two teachers who gave insignificant responses, the other teachers’ responses were categorized thematically. Therefore, Table 8 contains the response categories by themes, the number of respondents (N), and examples of responses. According to most teachers ( N = 10), there is a lack of the required up-to-date knowledge, skills, and experience, and for other teachers ( N = 7), there are shortages of equipment and chemicals (in Biology laboratories) as well as instructional aids (in pedagogical centers), which are challenges of using the PSMMS in Biology instruction. They also mentioned that challenging factors, such as the high student-teacher ratio and time constraints ( N = 4), students’ deficiency of knowledge and attitudes towards learning ( N = 3), and problems with school administrative functions ( N = 1), have an impact on how well students learn Biology while using the PSMMS instructional approach (Table 8 ).
Students’ perspectives , however, were based on their responses to survey questions concerning the challenges of using the PSMMS in Biology lessons, as shown in Table 9 below. The study found statistically significant ( p < 0.05) differences across the five-item dimensions, with an average mean of 3.62 and a standard deviation of 1.36. Consequently, mean scores above 3 indicated that students agreed with the challenges of implementing the PSMMS in Biology instruction (Table 9 ).
As shown in Table 9 , the majority of students identified two key challenges to successfully implementing the PSMMS in their learning. These are shortages of instructional resources (Item 2, M = 3.56, SD = 1.39) and student difficulty in connecting their prior knowledge with Biological concepts (Item 1, M = 3.44, SD = 1.42). On the other hand, students responded that their teachers had the knowledge and awareness to conduct instructional processes using the PSMMS (Item 4, M = 3.95, SD = 1.22) and had the skills and competence to conduct instructional processes using the PSMMS (Item 5, M = 3.98, SD = 1.35). Table 9 also shows that, despite significant differences in response patterns, students generally had a negative opinion about the dominance of some students in collaborative work (Item 3, M = 3.16, SD = 1.43).
According to the analyzed data, one of the challenging factors was that teachers often lack the required knowledge and skills to facilitate learning, scaffold it, and successfully implement PSMMS in Biology instruction. In contrast, Belland et al. ( 2013 ) suggested that instructional scaffolds increase students’ autonomy, competence, and intimacy, which improves their motivation and enables them to identify appropriate challenges. The other challenging factor that influenced the use of the PSMMS in Biology instruction was the shortage of instructional resources and facilities. Consistent with the studies of Daganaso et al. ( 2020 ) and Kawishe (2016), the use of the PSMMS for Biology instruction faces challenges due to inadequate instructional resources, time constraints, and large class sizes. However, as Eshete (2001) describes, students lack the importance of instructional resources, as instructional resources are necessary for students to learn Biology effectively as they are essential for a deeper understanding of science.
Generally, the important findings from the analyses of the teachers, learners, and learning contexts and their implications for design principles are summarized in Table 10 .
Conclusions
In this study, contexts (teachers, students, and learning) were analyzed with the aim of designing a context-driven problem-solving method with metacognitive scaffolding (PSMMS) for Biology instruction. Despite the potential benefits of the PSMMS, the findings of the current study indicate that the use of the PSMMS instructional approach faces challenges. These challenges include teachers’ lack of the required up-to-date knowledge and skills, students’ lack of awareness and positive attitude towards learning, an overloaded curriculum, scarcity of resources, large class sizes, and problems with school administrative functions. The study emphasizes the significance of context analysis in the design of an effective PSMMS instructional method for enhancing students’ learning in Biology. This analysis provides useful information for providing pertinent examples, practical content, and context-driven instruction.
The context-driven instructional design approach, using the PSMMS, addresses problems in teachers’ effectiveness, students’ effective learning, and the establishment of supportive teaching and learning environments. This approach considers the performance of both teachers and students, as well as the learning environment, including the availability of instructional resources. Consequently, this study concludes that understanding the needs of teachers in relation to the PSMMS can help both teachers and educational policymakers design a system that is well-suited to their specific requirements. Additionally, it can help students use their practical skills as well as establish connections between their prior knowledge and the Biology concepts they are learning. This process has the potential to generate innovative systems for applying the PSMMS instructional approach, with teachers serving as facilitators and students actively engaging and taking responsibility for their own learning progress.
The study investigated the importance of incorporating target groups into the design of the PSMMS for Biology instruction. The study’s empirical findings support the notion that the PSMMS should provide regular learning opportunities and foster the active engagement of teachers. The study also emphasizes the need to consider learning contexts while designing the PSMMS for Biology instruction that is deeply rooted in its particular context, as effective principles applied in one context could not yield the same results in another context. The study suggests that this strategy is particularly useful in developing countries like Ethiopia, where there is limited experience with metacognitive strategies to scaffold the problem-solving method in Biology instruction. As a result, the authors recommend expanding the target audience, considering the national context, and incorporating metacognitive knowledge and regulation strategies in designing context-driven PSMMS for secondary school Biology instruction.
Data Availability
The authors confirm that the results of this study are available in the article and its supplementary material, and raw data can be obtained from the corresponding author upon reasonable request.
Aaltonen, P., & Ikavalko, H. (2002). Implementing strategies successfully. Integrated Manufacturing Systems , 13 (6), 415–418.
Article Google Scholar
Agaba, K. C. (2013). Effect of Concept Mapping Instructional Strategy on Students Retention in Biology. African Education Indices , 5 (1), 1–8.
Google Scholar
Agena, D. (2010). Case Study: Ethiopia UNICEF .
Ahmady, G., & Nakhostin-Ruhi, N. (2014). The effect of problem-solving method on improving primary students’ mathematics achievement and creativity. Mathematics Education , 68 , 22708–22710.
Al Azmy, K. A., & Alebous, T. M. (2020). The degree of using metacognitive thinking strategies skills for problem-solving by a sample of biology female teachers at the secondary stage in the state of Kuwait. Educational Research and Reviews , 15 (12), 764–774. https://doi.org/10.5897/ERR2020.4094 .
Albay, E. M. (2019). Analyzing the effects of the problem-solving approach to the performance and attitude of first-year university students. Social Sciences & Humanities Open , 1 (1), 1–7. https://doi.org/10.1016/j.ssaho.2019.100006 .
Aurah, C. M., Koloi-Keaikitse, S., Isaacs, C., & Finch, H. (2011). The role of metacognition in everyday problem-solving among primary students in Kenya. Problems of Education in the 21st Century , 30 (2011), 9–21.
Belland, B. R., Kim, C., & Hannafin, M. J. (2013). A framework for designing scaffolds that improve motivation and cognition. Educational Psychologist , 48 (4), 243–270. https://doi.org/10.1080/00461520.2013.838920 .
Beyessa, F. (2014). Major factors that affect grade 10 students’ academic achievement in science education at Ilu Ababora general secondary of Oromia regional state, Ethiopia. International Letters of Social and Humanistic Sciences , 32 (21), 118–134. https://doi.org/10.18052/www.scipress.com/ILSHS.32.118 .
Chandio, M. T., Pandhiani, S. M., & Iqbal, R. (2016). Bloom’s Taxonomy: Improving Assessment and Teaching-Learning Process. Journal of Education and Educational Development , 3 (2), 203–221.
Chu, S. K. W., Reynolds, R. B., Tavares, N. J., Notari, M., & Lee, C. W. Y. (2017). 21st century skills development through inquiry-based learning from theory to practice . Springer International Publishing.
Cimer, A. (2012). What makes biology learning difficult and effective: Students’ views. Educational Research and Reviews , 7 (3), 61–71. https://doi.org/10.5897/ERR11.205 .
Creswell, J. W. (2009). Research design: Qualitative, quantitative, and mixed methods approaches (3rd ed.). SAGE Publications. Inc.
Creswell, J. W., & Creswell, J. D. (2018). Research design: Qualitative, quantitative, and mixed methods approaches (5th ed.). SAGE Publications, Inc.
Daganaso, R. O., Macasadogs, D. V. C., Tan, M. L. G., Pilande, C. J. A., Calipayan, N. J., & Santos, A. G. D. L (2020). Overcoming challenges in the use of teaching strategies: The case of grade eight biology teachers. Journal of International Academic Research for Multidisciplinary , 8 (1), 25–36.
Darling-Hammond, L., Flook, L., Cook-Harvey, C., Barron, B., & Osher, D. (2020). Implications for Educational Practice of the Science of Learning and Development. Applied Developmental Science , 24 (2), 97–140. https://doi.org/10.1080/10888691.2018.1537791 .
Dawal, B. S., & Mangut, M. (2021). Overloaded curriculum content: Factor responsible for students’ under achievement in basic science and technology in junior secondary schools in Plateau state, Nigeria. KIU Journal of Social Sciences , 7 (2), 123–128.
ETP. (1994). The Federal Democratic Republic of Ethiopia Education and Training Policy . St. George Printing.
Ganyaupfu, E. M. (2013). Teaching methods and students’ academic performance. International Journal of Humanities and Social Science Invention , 2 (9), 29–35.
Georghiades, P. (2000). Beyond conceptual change learning in science education: Focusing on transfer, durability, and Metacognition. Educational Research , 42 (2), 119–139.
Getenet, S. T. (2020). Designing a professional development program for mathematics teachers for effective use of technology in teaching. Education and Information Technologies , 25 (3), 1855–1873. https://doi.org/10.1007/s10639-019-10056-8 .
Guner, P., & Erbay, H. N. (2021). Metacognitive skills and problem-solving. International Journal of Research in Education and Science (IJRES) , 7 (3), 715–734. https://doi.org/10.46328/ijres.1594 .
Guterman, E. (2002). Toward Dynamic Assessment of Reading: Applying Metacognitive Awareness Guidance to Reading Assessment Tasks. Journal of Research in Reading , 25 (3), 283–298.
Howard, B. C., McGee, S., Shia, R., & Hong, N. S. (2001). The Influence of Metacognitive Self-Regulation and Ability Levels on Problem-Solving .
Hyder, I., & Bhamani, S. (2016). Bloom’s taxonomy (cognitive domain) in higher education settings: Reflection brief. Journal of Education and Educational Development , 3 (2), 288–300.
Inel, D., & Balim, A. G. (2010). The effects of using problem-based learning in science and technology teaching upon students’ academic achievement and levels of structuring concepts. Asia-Pacific Forum on Science Learning and Teaching , 11 (2), 1–23.
Kallio, H., Virta, K., Kallio, M., Virta, A., Hjardemaal, F. R., & Sandven, J. (2017). The utility of the metacognitive awareness inventory for teachers among in-service teachers. Journal of Education and Learning , 6 (4), 78–91. https://doi.org/10.5539/jel.v6n4p78 .
Kapa, E. (2001). A metacognitive support during the process of problem-solving in a computerized environment. Educational Studies in Mathematics , 47 (3), 317–336.
Khaparde, R. (2019). Experimental problem-solving: A plausible approach for conventional laboratory courses. Journal of Physics: Conference Series , 1286 (1), 1–7. https://doi.org/10.1088/1742-6596/1286/1/012031 .
Kim, N. J., Belland, B. R., & Axelrod, D. (2019). Scaffolding for optimal challenge in K–12 problem-based learning. Interdisciplinary Journal of Problem-Based Learning , 13 (1), 3–26. https://doi.org/10.7771/1541-5015.1712 .
Lai, E. R. (2011). Metacognition: A literature review. Pearson Research Report , 24 , 1–40. http://www.pearsonassessments.com/research .
MoE (2020). Ministry of Education Concept Note for Education Sector COVID 19-Preparedness and Response Plan .
MoE (2019). Federal Democratic Republic of Ethiopia Ministry of Education Curriculum for Doctor of Education in Biology .
MoE (2009). The Federal Democratic Republic of Ethiopia, Ministry of Education, Curriculum Framework for Ethiopian Education (KG – Grade 12) .
MoE (2005). The Federal Democratic Republic of Ethiopia: Education Sector Development Program III (ESDP-III) 2005/2006–2010/2011 (1998 EFY – 2002 EFY) Program Action Plan (PAP) .
Negash, T. (2006). Education in Ethiopia from Crisis to the Brink of Collapse . Nordiska Afrikainstitutet.
Nnorom, N. R. (2019). Effect of problem-based solving technique on secondary school students achievement in biology. International Journal of Scientific & Engineering Research , 10 (3), 1025–1029.
Okoro, C. O., & Chukwudi, E. K. (2011). Metacognitive strategies: A viable tool for self-directed learning. Journal of Educational and Social Research , 1 (4), 71–76.
Peterson, C. (2003). Bringing ADDIE to life: Instructional design at its best. Journal of Educational Multimedia and Hypermedia , 12 (3), 227–241.
Rahmawati, D., Sajidan, S., & Ashadi, A. (2018). Analysis of Problem-Solving Skill in Learning Biology at Senior High School of Surakarta. International Conference on Science Education (ICoSEd) , 1006 , 1–5. https://doi.org/10.1088/1742-6596/1006/1/012014 .
Reich, Y., Kolberg, E., & Levin, I. (2006). Designing contexts for learning design. International Journal of Engineering Education , 22 (3), 489–495.
Schraw, G., Crippen, K. J., & Hartley, K. (2006). Promoting self-regulation in science education: Metacognition as part of a broader perspective on learning. Research in Science Education , 36 , 111–139. https://doi.org/10.1007/s11165-005-3917-8 .
Senyigit, C. (2021). The effect of problem-based learning on pre-service primary school teachers’ conceptual understanding and misconceptions. International Online Journal of Primary Education (IOJPE) , 10 (1), 50–72.
Sperling, R. A., Howard, B. C., Staley, R., & DuBois, N. (2004). Metacognition and self-regulated learning constructs. Educational Research and Evaluation: An International Journal on Theory and Practice , 10 (2), 117–139. https://doi.org/10.1076/edre.10.2.117.27905 .
Stehle, S. M., & Peters-Burton, E. E. (2019). Developing student 21st century skills in selected exemplary inclusive STEM high schools. International Journal of STEM Education , 6 (1), 1–15. https://doi.org/10.1186/s40594-019-0192-1 .
Syofyan, R., & Siwi, M. K. (2018). The impact of visual, auditory, and kinesthetic learning styles on economics education teaching. Advances in Economics, Business, and Management Research , 57 , 642–649.
Tachie, S. A. (2019). Metacognitive skills and strategies application: How this helps learners in mathematics problem-solving. EURASIA Journal of Mathematics Science and Technology Education , 15 (5), 1–12. https://doi.org/10.29333/ejmste/105364 .
Tosun, C., & Senocak, E. (2013). The effects of problem-based learning on metacognitive awareness and attitudes toward chemistry of prospective teachers with different academic backgrounds. Australian Journal of Teacher Education , 38 (3), 61–73.
Umar, A. A. (2011). Effects of biology practical activities on students’ process skill acquisition in Minna, Nigeria state. Journal of Science Technology Mathematics and Education (JOSTMED) , 7 (2), 120–128.
Wang, F., & Hannafin, M. J. (2005). Design-based research and technology-enhanced learning environments. Educational Technology Research and Development , 53 (4), 5–23.
Widya, Rifandi, R., & Rahmi, Y. L. (2019). STEM education to fulfill the 21st century demand: A literature review. Journal of Physics: Conference Series , 1317 , 1–7. https://doi.org/10.1088/1742-6596/1317/1/012208 .
Zimmerman, B. J. (2008). Investigating self-regulation and motivation: Historical background, methodological developments, and future prospects. American Educational Research Journal , 45 (1), 166–183. https://doi.org/10.3102/0002831207312909 .
Zumbrunn, S., Tadlock, J., & Roberts, E. D. (2011). Encouraging self-regulated learning in the classroom: A review of the literature. Metropolitan Educational Research Consortium (MERC) , 1–28.
Download references
Acknowledgements
The authors would like to thank the teachers and students of Shambu Secondary Schools, Jimma University, and Shambu College of Teachers Education for their invaluable contributions in terms of information, resources, and financial support.
This editorial has not received financial support from any funding organizations.
Author information
Authors and affiliations.
College of Natural Sciences, Department of Biology, Jimma University, Jimma, Ethiopia
Merga Dinssa Eticha & Tsige Ketema
Department of Biology, Shambu College of Teachers Education, Shambu, Ethiopia
Merga Dinssa Eticha
Department of Curriculum and Instructional Sciences, Kotebe University of Education, Addis Ababa, Ethiopia
Adula Bekele Hunde
You can also search for this author in PubMed Google Scholar
Contributions
Merga Dinssa Eticha : Conceptualization, Methodology, Validation, Formal analysis, Investigation, Resources, Writing-original draft, Writing-review and editing.
Adula Bekele Hunde : Conceptualization, Methodology, Validation, Investigation, Supervision, Writing-review and editing.
Tsige Ketema : Conceptualization, Methodology, Validation, Investigation, Supervision, Writing-review and editing.
Corresponding author
Correspondence to Merga Dinssa Eticha .
Ethics declarations
Ethical approval.
All procedures performed in studies involving human participants followed the ethical standards of institutional and national research committees. Therefore, approval to conduct the research was accepted by the university’s institutional review board, and ethical guidelines were followed in conducting this study.
Competing Interests
The authors declare no conflicting and competing interests.
Informed Consent
All individual participants involved in the study provided informed consent.
Statement Regarding Research Involving Human Participants and/or Animals
This study entailed the involvement of human subjects and was conducted in accordance with ethical standards, which encompassed the principles of informed consent and approval from an ethics committee.
Consent to Participate
Consent was obtained from all individual participants involved in the study after ensuring that they were fully informed. To protect their privacy, participants’ names will not be linked to any publication or presentation that uses the data and research collected. Instead, the authors used codes to identify participants. Disclosure of identifiable information will only occur if required by law or with the written consent of the participant. Participants participated in the study voluntarily and had the option to withdraw at any time.
Consent to Publish
The authors hereby affirm that the participants in the human research have given their consent for the publication of the details in the journal and article.
Additional information
Publisher’s note.
Springer Nature remains neutral with regard to jurisdictional claims in published maps and institutional affiliations.
Rights and permissions
Open Access This article is licensed under a Creative Commons Attribution 4.0 International License, which permits use, sharing, adaptation, distribution and reproduction in any medium or format, as long as you give appropriate credit to the original author(s) and the source, provide a link to the Creative Commons licence, and indicate if changes were made. The images or other third party material in this article are included in the article’s Creative Commons licence, unless indicated otherwise in a credit line to the material. If material is not included in the article’s Creative Commons licence and your intended use is not permitted by statutory regulation or exceeds the permitted use, you will need to obtain permission directly from the copyright holder. To view a copy of this licence, visit http://creativecommons.org/licenses/by/4.0/ .
Reprints and permissions
About this article
Eticha, M.D., Hunde, A.B. & Ketema, T. Designing a Context-Driven Problem-Solving Method with Metacognitive Scaffolding Experience Intervention for Biology Instruction. J Sci Educ Technol (2024). https://doi.org/10.1007/s10956-024-10107-x
Download citation
Accepted : 27 February 2024
Published : 27 August 2024
DOI : https://doi.org/10.1007/s10956-024-10107-x
Share this article
Anyone you share the following link with will be able to read this content:
Sorry, a shareable link is not currently available for this article.
Provided by the Springer Nature SharedIt content-sharing initiative
- Biology learning
- Context analysis
- Metacognitive scaffolding
- Problem-solving method
- Find a journal
- Publish with us
- Track your research
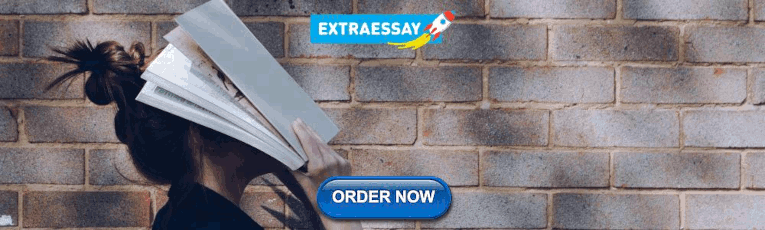
IMAGES
COMMENTS
Example of a Secondary Data Analysis. An example highlighting this method of reusing one's own data is Winters-Stone and colleagues' SDA of data from four previous primary studies they performed at one institution, published in the Journal of Clinical Oncology (JCO) in 2017. Their pooled sample was 512 breast cancer survivors (age 63 ± 6 years) who had been diagnosed and treated for ...
When to use secondary research. Secondary research is a very common research method, used in lieu of collecting your own primary data. It is often used in research designs or as a way to start your research process if you plan to conduct primary research later on.. Since it is often inexpensive or free to access, secondary research is a low-stakes way to determine if further primary research ...
Step 3: Design your research process. After defining your statement of purpose, the next step is to design the research process. For primary data, this involves determining the types of data you want to collect (e.g. quantitative, qualitative, or both) and a methodology for gathering them. For secondary data analysis, however, your research ...
Secondary data analysis is a valuable research approach that can be used to advance knowledge across many disciplines through the use of quantitative, qualitative, or mixed methods data to answer new research questions ( Polit & Beck, 2021 ). This research method dates to the 1960s and involves the utilization of existing or primary data ...
The analysis of existing data sets is routine in disciplines such as economics, political science, and sociology, but it is less well established in psychology (but see Brooks-Gunn & Chase-Lansdale, 1991; Brooks-Gunn, Berlin, Leventhal, & Fuligini, 2000).Moreover, biases against secondary data analysis in favor of primary research may be present in psychology (see McCall & Appelbaum, 1991).
Secondary analyses of large datasets provide a mechanism for researchers to address high impact questions that would otherwise be prohibitively expensive and time-consuming to study. This paper presents a guide to assist investigators interested in conducting secondary data analysis, including advice on the process of successful secondary data ...
Secondary data analysis can occur with any previously collected data, including data collected through quantitative or qualitative methods for original research, national survey data and data collected for non-research purposes (e.g., electronic health record or monitoring data).
Secondary analysis is the analysis of data that have originally been collected either for a different purpose or by a different researcher or organisation. Because of the cost and complexity of primary data collection, and because of the opportunities offered by "found" data not originally collected for research purposes (e.g ...
The earliest reference to the use of secondary data analysis in the nursing literature can be found as far back as the 1980's, when Polit & Hungler (1983 ), in the second edition of their classic nursing research methods textbook, discussed this emerging approach to analysis. At that time, this method was rarely used by nursing researchers.
Assessing credibility of the data - Establishing the credentials of the original researchers, searching for full explication of methods including any problems encountered, determining how consistent the data is with data from other sources, and discovering whether the data has been used in any credible published research. Analysis - This ...
Abstract. Secondary analysis is a research methodology in which preexisting data are used to investigate new questions or to verify the findings of previous work. It can be applied to both quantitative and qualitative data but is more established in relation to the former. Interest in the secondary analysis of qualitative data has grown since ...
Secondary analysis of qualitative data is a topic unto itself and is not discussed in this volume. The interested reader is referred to references such as James and Sorenson (2000) and Heaton (2004). The choice of primary or secondary data need not be an either/or ques-tion. Most researchers in epidemiology and public health will work with both ...
A secondary analysis occurs when a researcher uses data composed by another researcher or collector in order to conduct a study with a different purpose from the original study. Secondary data can be obtained from surveys, official records, official statistics, academic studies, and archival data repositories.
that secondary data analysis is a viable method to utilize in the process of inquiry when a systematic procedure is followed and presents an illustrative research application utilizing secondary data analysis in library and information science research. Keywords: secondary data analysis, school librarians, technology integration 1. Introduction
In addition to the challenges of secondary research as mentioned in subsection Secondary Data and Analysis, in current research realm of secondary analysis, there is a lack of rigor in the analysis and overall methodology (Ruggiano & Perry, 2019). This has the pitfall of possibly exaggerating the effects of researcher bias (Thorne, 1994, 1998 ...
While secondary data analysis of quantitative data has become commonplace and encouraged across disciplines, the practice of secondary data analysis with qualitative data has met more criticism and concerns regarding potential methodological and ethical problems.
Secondary data in mixed methods research is the process of identifying, evaluating, and incorporating one or more secondary qualitative or quantitative data sources into a mixed methods project. Incorporating secondary data expands on the original definition of mixed methods research, which involves collecting, analyzing, and integrating qualitative and quantitative approaches to study a ...
The secondary analysis followed the three steps proposed by Johnston (2014): (1) forming a research question, (2) identifying an appropriate data set, and (3) performing a comprehensive evaluation ...
Here are some common methods of secondary data analysis: Descriptive Analysis: This method involves describing the characteristics of a dataset, such as the mean, standard deviation, and range of the data. Descriptive analysis can be used to summarize data and provide an overview of trends. ... When exploring a new research area: Secondary data ...
The process of data analysis in secondary research. Secondary analysis (i.e., the use of existing data) is a systematic methodological approach that has some clear steps that need to be followed for the process to be effective. In simple terms there are three steps: Step One: Development of Research Questions. Step Two: Identification of dataset.
Secondary analysis is a research methodology by which researchers use pre-existing data in order to investigate new questions or for the verification of the findings of previous works (Heaton, 2019).
What is secondary data & archival material? Primary & secondary data. All research will involve the collection of data. Much of this data will be collected directly through some form of interaction between the researcher and the people or organisation concerned, using such methods as interviews, focus groups, surveys and participant observation.
Although preregistration can reduce researcher bias and increase transparency in primary research settings, it is less applicable to secondary data analysis. An alternative method that affords additional protection from researcher bias, which cannot be gained from conventional forms of preregistration alone, is an Explore and Confirm Analysis ...
By learning foundational data analysis methods, you can develop the ability to assess and analyze your data accurately, leading to informed insights within your industry. As you begin, start by learning key techniques such as regression, hypothesis, and cluster analysis. ... Learners are advised to conduct additional research to ensure that ...
4 METHODS 4.1 Design. This study is a secondary data analysis based on a cross-sectional study which was conducted from December 2015 to September 2016. The original study (Zhang & Duan, 2017) aimed to explore the relationship between asthma control and quality of life in children with asthma. During the data analysis, we found that family ...
Secondary data analysis. Secondary analysis refers to the use of existing research data to find answer to a question that was different from the original work ( 2 ). Secondary data can be large scale surveys or data collected as part of personal research. Although there is general agreement about sharing the results of large scale surveys, but ...
Methods. Individual patient data analysis of COVID-ARDS and CLASSIC-ARDS patients in six observational studies of ventilation, four in the COVID-19 pandemic and two pre-pandemic. ... Differences in outcomes found in our study are, at least in part, in line with prior research findings [21, ... A secondary analysis of the Practice of ...
Secondary outcomes were the risk of 4 common antibiotic-associated adverse events: gastrointestinal symptoms, rash, kidney injury and C. difficile infection. Statistical methods We adjusted for covariate-dependent censoring and treatment indication using a broad set of domain-expert derived features.
Analysis of data. To analyse the results, the variables were coded using the inductive method (Moreno Rosset & Ramírez Uclés, 2019). To this end, each of the articles was read in order to extract the variables related to the transition from high school to university for students who identify as autistic.
Therefore, the study was conducted in the Biology departments of secondary schools in Shambu Town, Oromia Region, Ethiopia. The study employed mixed-methods research to collect and analyze data, involving 12 teachers and 80 students. The data collection tools used were interviews, observations, and a questionnaire.