Thank you for visiting nature.com. You are using a browser version with limited support for CSS. To obtain the best experience, we recommend you use a more up to date browser (or turn off compatibility mode in Internet Explorer). In the meantime, to ensure continued support, we are displaying the site without styles and JavaScript.
- View all journals
- Explore content
- About the journal
- Publish with us
- Sign up for alerts
- Open access
- Published: 03 February 2022
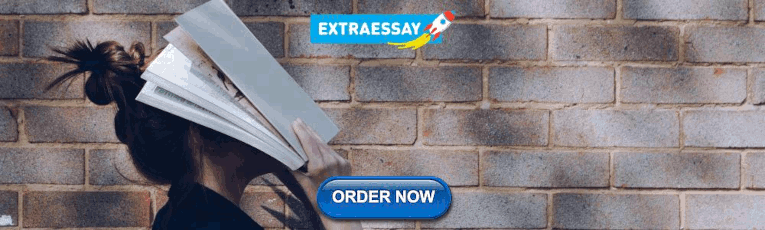
Cortical neural dynamics unveil the rhythm of natural visual behavior in marmosets
- Takaaki Kaneko ORCID: orcid.org/0000-0002-3364-9020 1 , 2 na1 ,
- Misako Komatsu ORCID: orcid.org/0000-0003-4464-4484 3 na1 ,
- Tetsuo Yamamori 3 ,
- Noritaka Ichinohe 4 &
- Hideyuki Okano ORCID: orcid.org/0000-0001-7482-5935 1 , 5
Communications Biology volume 5 , Article number: 108 ( 2022 ) Cite this article
4221 Accesses
5 Citations
23 Altmetric
Metrics details
- Oculomotor system
- Sensorimotor processing
- Sensory processing
- Visual system
Numerous studies have shown that the visual system consists of functionally distinct ventral and dorsal streams; however, its exact spatial-temporal dynamics during natural visual behavior remain to be investigated. Here, we report cerebral neural dynamics during active visual exploration recorded by an electrocorticographic array covering the entire lateral surface of the marmoset cortex. We found that the dorsal stream was activated before the primary visual cortex with saccades and followed by the alteration of suppression and activation signals along the ventral stream. Similarly, the signal that propagated from the dorsal to ventral visual areas was accompanied by a travelling wave of low frequency oscillations. Such signal dynamics occurred at an average of 220 ms after saccades, which corresponded to the timing when whole-brain activation returned to background levels. We also demonstrated that saccades could occur at any point of signal flow, indicating the parallel computation of motor commands. Overall, this study reveals the neural dynamics of active vision, which are efficiently linked to the natural rhythms of visual exploration.
Similar content being viewed by others
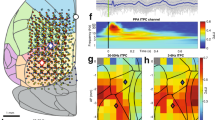
Visual evoked feedforward–feedback traveling waves organize neural activity across the cortical hierarchy in mice
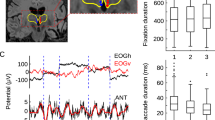
Neural activity in the human anterior thalamus during natural vision
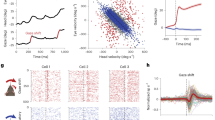
A dynamic sequence of visual processing initiated by gaze shifts
Introduction.
The primate visual system is one of the most investigated cortical circuitries. More than 30 cortical areas have visual functions and are organized hierarchically into complex feedforward and feedback connections 1 , 2 . The dual-stream hypothesis models how visual information is processed in this circuitry, in which the visual input received by the retina is transmitted to the primary visual cortex (V1) and then flows to the functionally distinct dorsal and ventral visual streams 3 , 4 , 5 ; the former is dedicated to the analysis of scenes and object semantics 6 , while the latter is important for the analysis of the spatial properties of visual information 7 .
However, several recent studies have shed light on a complementary or alternative schema for visual information flow proposed in this theory. First, functional 8 , 9 , 10 and anatomical studies 11 , 12 , 13 , 14 have shown that there are many alternative routes that bypass V1, which is often assumed to be the entry point for visual information into the cerebral cortex, such as the extrastriate cortex and temporoparietal regions. Second, recent advances in our understanding of occipital and temporoparietal white matter revealed that communication across the dorsal and ventral visual areas is far richer than previously thought 15 , 16 . Third, natural visual behavior in primates is an intrinsically recurrent process consisting of recursive sampling of the same visual scene with different eye positions, and saccades are the result of ongoing visual processing. This forms a circular process of visual computation, a cognitive decision for the next saccade target, and motor execution 17 , 18 . While the neural mechanisms underlying each step of this behavioral sequence have been studied in detail, the neural dynamics of the entire cycle of active vision have been described rarely. A precise description of the timing at which cortical areas are activated and which regions exchange signals under active vision is essential for the construction of a computation model for active vision.
To capture the neural dynamics in the cerebral cortex during natural visual behavior, it is crucial to examine the exact spatiotemporal dynamics and trajectories of neural signal around saccades and the interactions of multiple areas during active visual exploration. To address these issues, we recorded the cortical neural dynamics of natural visual behavior in marmoset monkeys by using an electrocorticographic (ECoG) array covering almost the entire lateral hemisphere, over 63 cortical areas with 96 electrodes at an inter-electrode distance of 2.5 mm 19 , while marmosets freely viewed naturalistic movie stimuli (Fig. 1a, b ). Marmosets are an ideal primate model for this type of study as their brain shares most of the organizational features of the visual system found in other primates 15 , 20 , 21 , 22 , 23 , and their smooth brain surface without complex sulci allows cortical-wide recording using ECoG electrodes. In this study, we found that the dorsal stream was activated prior to the ventral stream under natural visual behavior. The information traversed the cortical sheet from the dorsal to ventral areas. Furthermore, we identified the recursive nature of active vision in which neural architecture and visual behavior are coordinated for the efficient exploration of visual scenes.
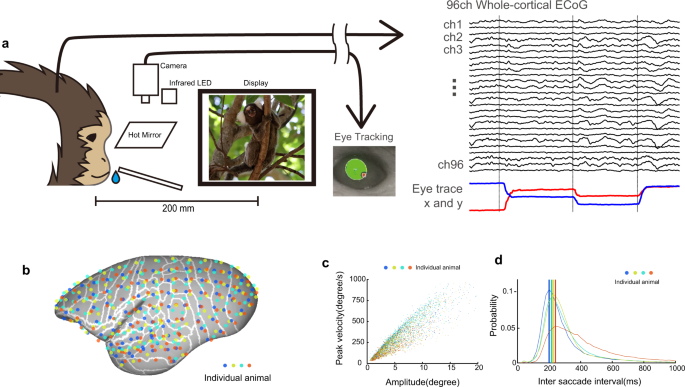
a A schematic illustration of the experimental design. The marmoset viewed naturalistic movies while its eye movements and ECoG signals were recorded. A liquid reward was delivered at a random interval irrespective of behavior to keep the subject awake for a longer duration. b The electrode positions of the four subject animals. The ECoG array with 96 channels covered almost the entire lateral hemisphere of the marmoset brain. The electrode positions were determined by in vivo CT scans and the cortical areas inferred from registration of subject MR images to the marmoset atlas. c The main sequence of saccades during the free-viewing paradigm. d Inter-saccade interval. The marmoset made rapid eye movements every 220 ms during natural visual behavior. Color represents individual marmosets as in ( b ).
Signal flow under natural visual behavior
During natural visual behavior, the marmosets performed rapid eye movements (saccades) to sample a visual scene with different eye positions every 220 ms (Fig. 1c, d ). These saccades refresh the visual information reaching the eyes and are thus a major driver of visual neural activity during natural visual behavior.
We first computed spectrograms from the ECoG signals (Fig. 2a ) around saccade onset and then extracted the high-gamma band (Fig. 2b ), which is highly correlated with local neural activity 24 , 25 , 26 . We found the smooth transition of post-saccadic activity along the ventral stream (Fig. 2c and Supplementary Fig. 1a ), which was evident by the gradual shift of high-gamma activity after saccade from V1 (the most posterior part of the marmoset brain) to the anterior part of the temporal cortex (Fig. 2d ). Conversely, the signal flow corresponding to the dorsal stream, i.e., the gradual shift of neural activity from early visual areas to temporoparietal regions, was less prominent (Fig. 2c ). Instead, several cortical regions located along the dorsal stream showed a faster response than V1 (Fig. 2d ). The fastest response was observed in the dorsal part of the middle temporal area (MT) and the dorsal part of the superior temporal sulcus (STS) (Fig. 2c, d ), which we collectively termed the dorsal STS (dSTS). The second region that showed a faster response than V1 covered a large area, including the posterior parietal cortex (PPC) and dorsal occipital cortex (Fig. 2c, d ).
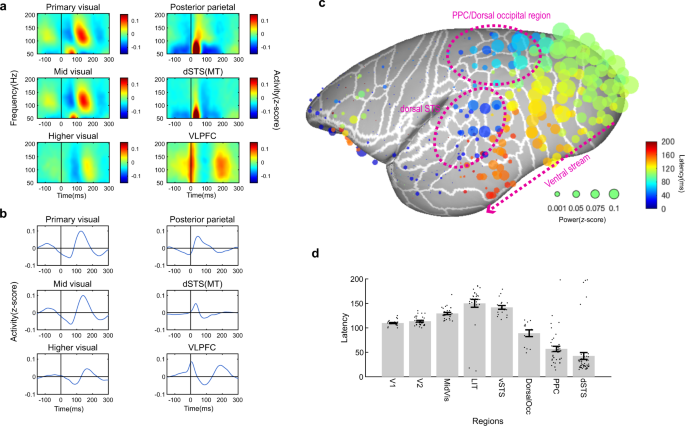
Post-saccadic neural activity of whole electrodes. a ECoG perisaccadic spectrogram of representative electrodes. The ECoG signals were aligned by saccade onset. Robust activity can be seen in visual areas after saccades. b High-gamma (100–160 Hz) ECoG signals in representative channels. c The magnitude and latency of the high-gamma signal peak from saccade onset. The data from four animals and 96 × 4 channels distributed over the entire lateral cortex (63 cortical areas) are plotted. The electrodes on the right hemisphere are computationally mapped onto the left hemisphere for visualization purposes. The smooth transition of visual information can be seen in the ventral stream. The areas in the dorsal stream (such as the dSTS including the MT, PPC, and dorsal occipital areas) were activated prior to the ventral stream. Statistical assessment of signal modulation by randomization is shown in Supplementary Fig. 1 . d Mean latency of post-saccadic neural activity in each cortical region. Error bars showed standard error of the mean. Dots show individual electrodes. MidVis mid-visual areas (e.g., V3, TEO), LIT lateral inferior temporal areas (e.g., TE1, TE2), vSTS ventral superior temporal sulcus (e.g., PG/IPa, vFST), dSTS dorsal superior temporal sulcus (e.g., MST, MT, dFST), DorsalOcc. dorsal occipital areas (e.g., V6, V3a), PPC posterior parietal cortex (e.g., LIP, AIP, Opt). Supplementary Table 1 shows detailed area names included in each region of interest.
Interestingly, high-gamma activity was suppressed prior to post-saccade excitation along the areas of the ventral stream (Fig. 3a, b and Supplementary Fig. 1b ). This was less prominent in the regions of the dorsal stream, except for the dorsal occipital cortex (Fig. 3a, b ). Surprisingly, the timing of suppression differed drastically across the areas (Fig. 3c ), i.e., it changed systematically according to the excitation timing of each area, so that the time difference between suppression and excitation remained constant, and the gradual shift of peak suppression could be seen along the ventral stream, as observed for the excitation signal.
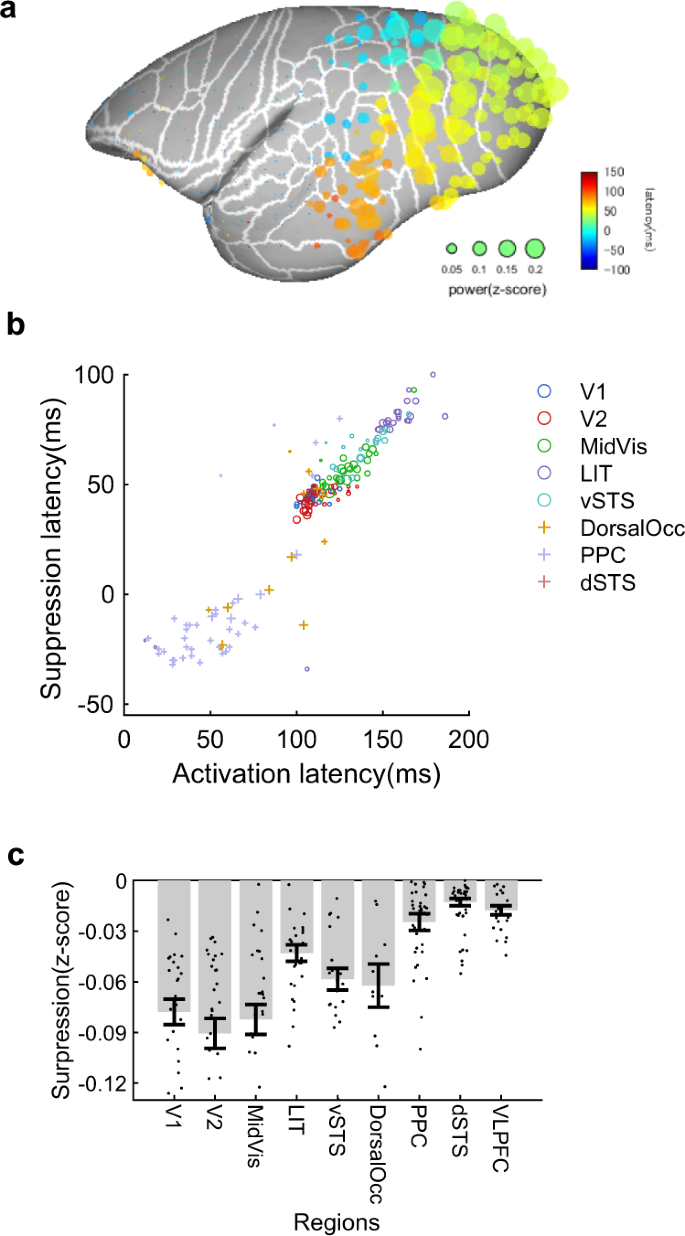
a Presaccadic suppression pattern across the whole lateral hemisphere from four animals. The size and color of the filled circles represent the magnitude and latency of the local minimum before saccade-evoked activation, respectively. The suppression magnitude of negative log P -values based on a randomization test is shown in Supplementary Fig. 1 . b Correlation of activation and suppression timing across electrodes. Circles represent electrodes on the ventral stream, and the crosses are for the dorsal regions. A linear correlation can be seen between the latency of activation and suppression along the ventral stream. c Average suppression magnitude for each region. Error bars are the standard error of the mean. Dots show individual electrodes. The largest degree of suppression was observed across the entire ventral stream, and it was less prominent in the dorsal regions, except for the dorsal occipital cortices.
These results suggest that in natural vision, in which saccades are critical for refreshing visual information on the retina, the dorsal stream plays a major role prior to V1, and then the ventral stream is activated for further visual analysis.
Distinct patterns of motor- and visual-related signals in the frontal and the dorsal regions
Perisaccade neural activity can be derived from various processes such as the reafferent visual signal from the eyes or motor-related signals, e.g., motor preparation 27 or efference copy/corollary discharge 28 , 29 . Here, we aimed to characterize further the potential differences across the dorsal areas that were activated prior to V1.
First, we tested contralateral dominance before saccade onset (i.e., −30–0 ms) as cortical saccadic motor control is known to be contralaterally dominated. We found that the ventrolateral prefrontal cortex (VLPFC) showed the strongest contralateral dominance among the entire cortex, and this was followed by the PPC and dorsal occipital regions (Fig. 4 ). This result indicated that the activation of these regions before or just after saccades is largely biased by saccade generation such as target selection and motor command. On the contrary, such contralateral dominance before saccades was virtually absent in the dSTS (Fig. 4 ). Moreover, the dSTS showed strong ipsilateral dominance from saccade onset, which contrasted with the VLPFC, PPC, and dorsal occipital regions. The VLPFC was activated before saccades and reached an activity peak at saccade onset (Fig. 2b ), presumably because the signal is dominated by neural activity for the generation of eye movements. The second peak in this region was after ~200 ms, which is consistent with the typical visual response in primate prefrontal neurons 30 . Such a clear separation of the 1st and 2nd peaks was not evident in the dSTS and PPC (Fig. 2b ). The activity peak of those regions occurred after eye movement was initiated (Fig. 2b ). The dSTS and PPC are known to be involved in the control of eye movement 9 , 31 , 32 , but the critical time window in which the dSTS and PPC function in natural visual behavior is quite distinct from that of the VLPFC.
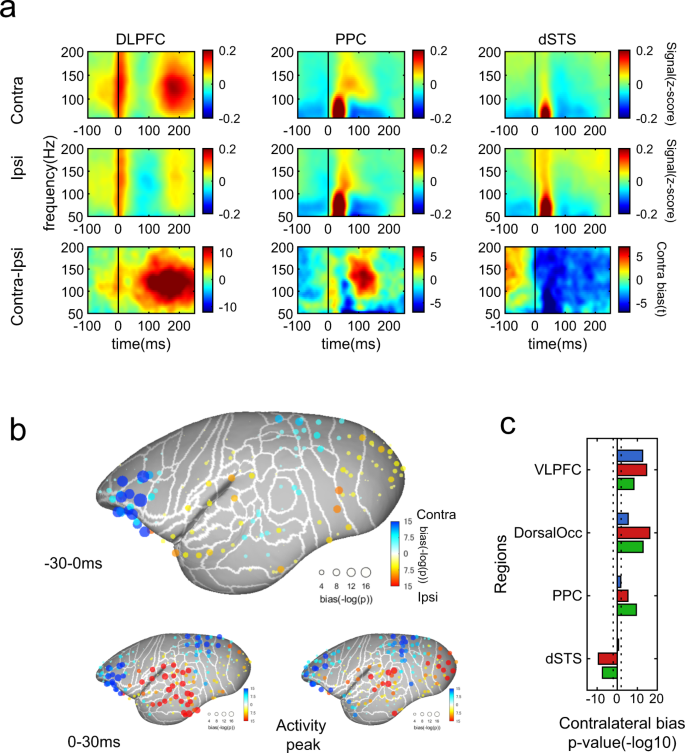
a Perisaccade spectrogram for an example electrode on the ventrolateral frontal cortex for contralateral and ipsilateral saccades. b The degree of contralateral dominance around saccade onset is shown by the color and size of the symbol on the 3D brain model. Blue color shows activity was larger for contralateral saccades. The largest degree of contralateral dominance appeared on the dorsolateral PFC (DLPFC) region. c Average contralateral dominance in each region of interest. Dotted lines represent statistical significance level with false discovery rate correction (alpha = 0.05).
Second, we attempted to disentangle the characteristics of the dSTS and PPC regions. Here, we examined whether the rapid response just after saccades in these regions is derived from saccade onset or fixation onset. These two events are temporally close, but their impact on the retina is distinct, e.g., the former blurs the image, while the latter stabilizes it. In natural visual behavior, a variety of saccade amplitudes occur so that saccade duration ranges from 20 to 60 ms. This enabled us to test whether post-saccade activity was aligned by saccade onset or fixation onset (saccade offset). Interestingly, activation of the dSTS, including the MT, was strongly aligned with saccade onset, but not with saccade offset (Fig. 5a, b and Supplementary Fig. 2 ). Furthermore, suppression of the dSTS, which occurred after activation, was well explained by the timing of fixation onset, but not by saccade onset. This indicates that the dSTS was active when the eyes were moving and was suppressed when they stopped moving. The activity of the PPC contrasted with this pattern (Fig. 5a, b and Supplementary Fig. 2 ) and aligned well with fixation onset, while its suppression, which occurred prior to activation, was tightly aligned with saccade onset rather than fixation onset. This indicates that the PPC was suppressed while eyes were moving and was activated once they became stationary.
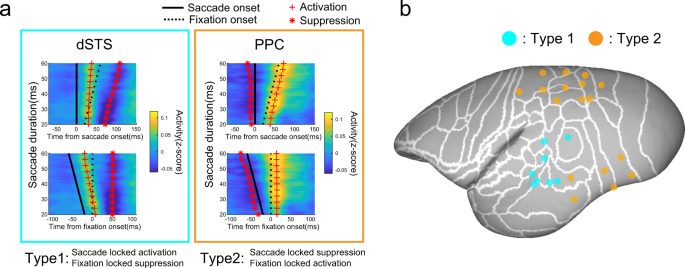
a Examples of perisaccadic high-gamma signals aligned either to saccade or fixation onset. High-gamma signal modulation with different saccade durations was computed to test whether the signal was better explained by saccade (top row) or fixation onset (bottom row). The solid and dotted lines show saccade and fixation onset, respectively. The red crosses are the timing of the activation peak for each saccade duration, and the asterisks are for suppression. For the dSTS, activation timing was well explained by saccade onset, whereas suppression was according to fixation onset (type 1), and vice versa for the PPC (type 2). b Electrode positions either classified as type 1 (cyan) or type 2 (orange). Many type 2 electrodes were in the PPC region, while type 1 electrodes clustered around the dSTS.
In summary, these results showed that the characteristics of cortical activation prior to ventral stream activation differed across regions among the frontal and dorsal areas. Activation of the VLPFC was dominated by contralateral saccades, indicating signal-related saccade generation. The dSTS was activated while the eyes were moving and suppressed once they were fixated, and the majority of PPC activity was suppressed while the eyes were moving and then activated at fixation onset.
High-gamma signal trajectory accompanied by a traveling wave of theta oscillations
Next, we sought to describe the spatiotemporal pattern of signal flow across the cerebral cortex. As high-gamma activity was transient and its timing differed across regions, it seems that the high activation spot should be limited in space and time. Figure 6a shows the high-gamma power of each time window around saccades. Indeed, the high-gamma signal was compact in space, and the activated area shifted gradually over time (Fig. 6a, b ). The center of gravity of the active region traversed from the dorsal region, from anterior to posterior, and was eventually transmitted along the ventral stream (Fig. 6c and Supplementary Fig. 3 ), which contrasts with passive visual presentation, in which the signal generated in the occipital cortex was transmitted to the dorsal and ventral streams (Fig. 6c and Supplementary Fig. 4 ). The signal trajectory from the dorsal areas, which are usually placed in the dorsal stream, to the earlier visual areas (V1 and V2) is plausible as a substantial number of anatomical connections exist in this direction (Supplementary Fig. 5 ) 33 . Granger causality analysis also supported the presence of signal influence from the dorsal visual areas to the posterior part of the occipital visual areas (Supplementary Fig. 6 ).
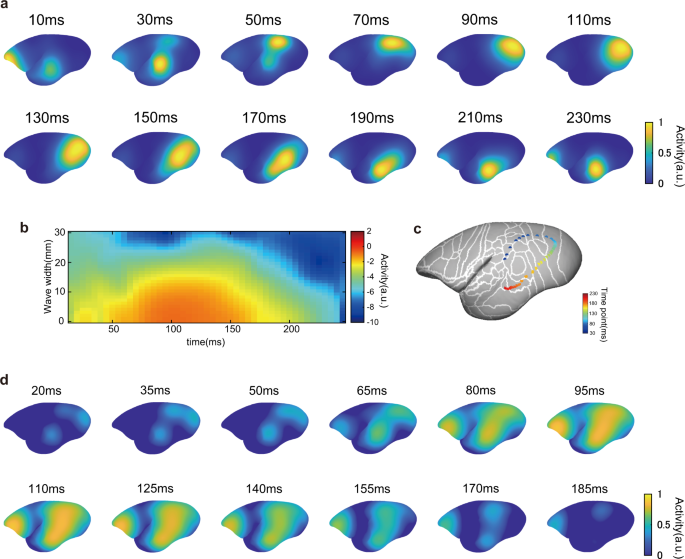
a Magnitude of the high-gamma signal in each time bin around saccades. Electrodes with a high-intensity signal were spatially clustered and moved across time. b High-gamma signal distribution across the cortical sheet as a function of distance from the center of high-gamma activity on the cortical surface in each time point. The high-gamma signal was spatially localized. c Center of gravity of the high-gamma signal. Colors represent the time from the saccade. The high-gamma signal peak moved from the dorsal region to the ventral stream. d Signal dynamics evoked by passive visual stimulus onset. In contrast to active vision, the initial response emerged at the most posterior part of the occipital regions and dSTS and this expanded toward the temporal and parietal cortices, which correspond to the ventral and dorsal stream, respectively.
Growing evidence suggests that synchronized neural activity is accompanied by low-frequency oscillations. The phase of these oscillations forms a spatial gradient on the cortical surface and travels as a wave along the cortex carrying information across distant regions, namely the traveling wave 34 , 35 , 36 . A recent study showed that the phase of the spontaneous traveling wave alters the visibility of subtle visual stimuli in marmosets 37 . Here, we tested whether the transition of the high-gamma signal peak observed in this study might be accompanied by such a traveling wave of lower frequency oscillations. We computed phase-amplitude coupling of the perisaccade period across the low-frequency phase and high-gamma signal amplitude. We found that the high-gamma signal was coupled with low-frequency oscillations of 6–12 Hz (Fig. 7a–c ). Next, we analyzed the directionality of phases in this frequency range. We found that the traveling wave propagated from the posterior terminal of the occipital cortex to the anterior pole of the temporal cortex along the ventral stream (Fig. 7d–f ). Interestingly, in the frontal lobe and dorsal regions, the direction of the wave was reversed; namely, it propagated from the frontal pole to the posterior parietal regions. In addition, the wave around the dorsal occipital regions changed its direction of propagation to the ventral regions. This pattern of wave propagation was consistent with the trajectory of the high-gamma signal peak, suggesting high-gamma activity carried a traveling wave at a lower frequency range across the cortical surface from the dSTS, PPC, and dorsal occipital regions, and then to the ventral stream.
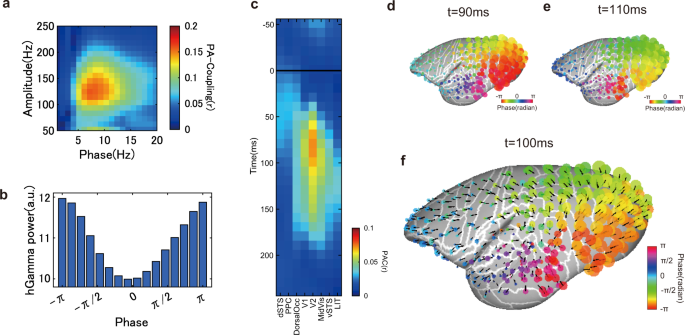
a Phase-amplitude coupling (PAC) of the perisaccadic period from an example electrode. High-gamma signal amplitude correlated best with the phase of theta and alpha oscillations (6–12 Hz). b High-gamma signal relative to the phase of theta oscillations (8 Hz) in the same electrodes as in ( a ). The High-gamma signal is highest at ±π rad and lowest at 0 rad. c Perisaccadic theta phase–high-gamma amplitude coupling across areas. Each column represents the average PAC from multiple electrodes belonging to the same cortical region. The solid black line is saccade onset. d , e Phase of theta oscillations at 90 and 110 ms from saccade onset. The phase of theta oscillations showed a gradient across adjacent electrodes. The phase of two adjusted time points shows the direction in which the phase moved along the cortical surface. f Directionality of traveling wave propagation. The black thick lines represent the direction of the traveling wave (the direction in which the same phase appeared at the next time point). The pattern of wave propagation was comparable to that of a high-gamma signal trajectory.
The rhythm of brain dynamics linked to saccade behavior
Such high-gamma activity propagation occurred 5 times per second, which corresponds to the rhythm of saccades in natural visual behavior. How is the cycle of the signal dynamics determined? When the neural activity of representative electrodes was aligned by saccade (saccade i ), each area showed three distinct local peaks that consisted of the activity of saccade i − 1, saccade i , and saccade i + 1. This resulted in an interesting situation in which at least some cortical areas were always active between two adjacent saccades, and the signal was relayed from one area to another without any moment at which the activity of the entire brain returned to background levels (Fig. 8a and Supplementary Fig. 7 ). It seems that the saccades are timed to occur before the activity of the whole brain returns to pre-activation levels and occurs at an interval that is sufficiently long to ensure that the signal reaches a variety of cortical areas.
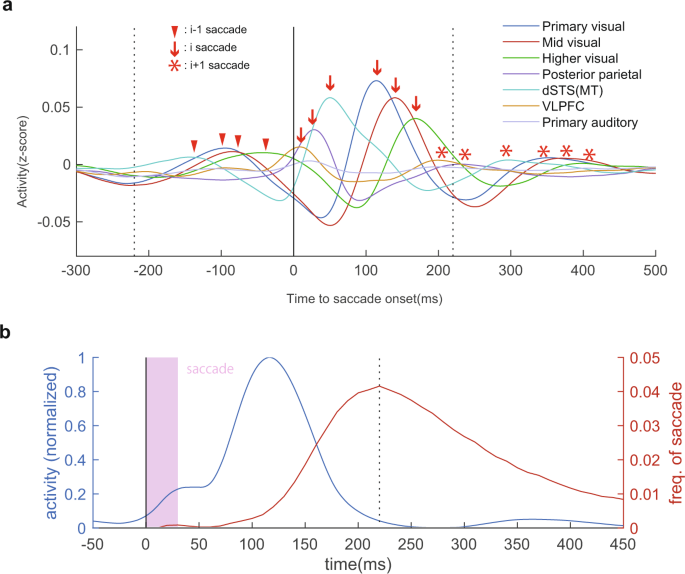
a Relay of information across cortical areas around a saccade. Red arrows, representing the local peak activity of each electrode, show three peaks that were derived from the i − 1, i , and i + 1 saccade, respectively. Local maxima of high-gamma activity were successively relayed across cortical areas during natural vision, and the cortex did not return to baseline levels of activity as the next saccade occurred every 220 ms. b Inter-saccade interval and temporal dynamics of whole-brain activity. Whole-brain activity almost returned to background levels at 200–250 ms after the saccade, and this timing corresponds to when the next saccade emerged most frequently.
To test this hypothesis directly, we computed the total activity of the whole brain and compared it with the inter-saccade interval. We found that a saccade occurred most frequently at ~220 ms after the previous saccade, and this timing coincided with the total activity of the whole cerebral cortex returning to background levels (Fig. 8b and Supplementary Fig. 8 ). In this manner, cortical computation is maintained at a certain level of activity without being completely inactive, and thus the neural network and behavior are optimized for the efficient computation of a visual scene.
Parallel saccade generation at various visual processing stages
Our observation of the correspondence between the timing of saccade generation and cortical activity returning to background levels raises two possible explanations for how saccades are generated during natural visual behavior. First, a saccade occurs when the analysis of a visual scene with a new eye position has been completed along the entirety of the visual stream. Alternatively, any part of the sensory cortical hierarchy could influence saccade generation in parallel, for example, via direct projections to the midbrain, the superior colliculus 38 . In this view, the correspondence between saccade timing and the timing of cortical silencing is simply a balance point between parallel saccade generation from different cortical computational stages. That is, a saccade may sometimes be generated by an earlier part of the cortical hierarchy or at the final stage. However, on average, the rhythm of active vision is designed to be neither too long so that the entire cortical computational process returns to background levels nor too short so that many cortical regions are unable to contribute to determine the next saccade.
To test these hypotheses, we examined how activity patterns changed according to fixation duration before the next saccade, which is the time that can be used for visual computation of the current eye position. Figure 6a shows the saccade-evoked cortical-wide signal dynamics followed by different fixation durations. The signal peak after the termination of fixation (the 2nd local peak from the saccade) was systematically delayed according to fixation duration, which confirmed our analysis worked well as the 2nd peak is derived from the subsequent saccade that terminates fixation, and this timing was delayed according to fixation duration. Conversely, we found that cortical signal dynamics were very similar after a saccade until fixation termination, regardless of fixation duration (Fig. 9a and Supplementary Fig. 9 ). In turn, when the fixation period was short, the activity level of early cortical areas remained high, even just before saccade onset, and the higher visual areas did not reach their activity peak (Fig. 9b and Supplementary Fig. 10 ). Furthermore, the activity pattern of this fixation duration was very similar to the activity pattern for longer fixation durations (Fig. 9c, d ). This suggests that the speed and pattern of a signal traversing the cortical sheet did not change according to the subsequent fixation duration. Conversely, the pattern of cortical activity just before the saccade was drastically different according to fixation duration before the saccade (Fig. 9b , magenta boxes; Fig. 9d ). When fixation duration was short, the anterior part of the inferior temporal regions did not receive full visual information, and only the early and mid-visual areas reached a high activation level before the next saccade target was selected. Conversely, when fixation duration was long, the anterior part of the temporal cortex tended to increase its activity toward saccade generation (Fig. 9b ). These results support the view that the visual cortices are able to contribute to the generation of saccades at any stage of cortical visual computation in parallel.
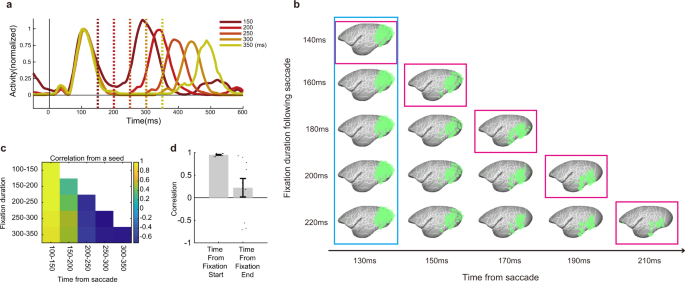
a Time course of whole-brain activity at different fixation durations. Regardless of fixation duration, which is the time available for determining the next saccade, brain dynamics were consistent (0–200 ms after saccade onset), i.e., the timing when whole-brain activity returned to background levels remained at ~200 ms. b Activity patterns for saccades with different fixation durations. Even when fixation duration was very short, the activity wave did not propagate rapidly across the cortex; instead, the activity dynamics were very similar within the same time range from saccade, regardless of the following fixation duration (blue box). On the contrary, the activity patterns when fixation terminated were drastically different across fixation duration (magenta box). c Example of the correlation matrix of the cortical activity fingerprint. The seed was the top-left image of ( b ). Again, activity was similar at the same time range after the saccade, rather than the time before fixation termination. d Mean correlation for activity in the same time range just after the saccade (blue box in b) and in the same time range just before fixation termination (magenta box). Dots show individual pairwise correlation The brain state of fixation termination that is drastically different according to time can be used to determine the next saccade target ( t (18) = 3.6, P < 0.001), suggesting parallel computation of saccade target.
In Fig. 9a , there appears to be a relationship between fixation duration and the power of subsequent peaks. We are not sure for the precise reason why the power of subsequent peaks differed across fixation duration. The signal pattern after fixation initiation (0–200 ms; 0–130 ms for the 150 ms fixation condition) was quite similar regardless of how long the subsequent fixation was maintained, however, the activity profiles just before the next saccade differed drastically according to the prior fixation duration. This difference might explain the difference in the power of the subsequent peak.
It should be noted that the absence of cortical activity before saccade onset shown in Fig. 7b cannot be attributed solely to saccade suppression 39 . If this was the case, then the timing when cortical activity returned to background levels should systematically shift according to the timing of the next saccade; however, this was not supported by our results (Fig. 9a and Supplementary Fig. 9 ).
In this study, we identified several distinctive features of brain dynamics during active visual behavior captured by an ECoG array covering almost the entire lateral surface of the marmoset cortex. During the course of active visual behavior, we found that the dorsal stream acts even earlier than V1, and activation of the ventral stream follows. The high-gamma signal traverses the cortical sheet from the dorsal to ventral areas accompanied by a traveling wave of theta oscillations. This signal was generated five times per second, just before the activity of the entire brain returned to background levels. We further demonstrated that saccades could be generated at any step of visual computation, indicating the parallel computation of motor commands. In this manner, neural network architecture and visual behavior are coordinated for the efficient exploration of the environment.
Primate eyes sample a visual scene recursively with different eye positions, and the timing and target of the next saccade are determined by the results of ongoing visual processing. Our study showed, in the free-viewing condition, the VLPFC plays a prominent role in triggering saccades, and visual information, while eyes are moving, may first arise in the dSTS. Area MT (in the dSTS) has a neural pathway that bypasses the primary visual area to receive visual input from the eyes 11 , 14 , and can thus receive visual information even faster than V1. Rosa and Tweedale 40 claimed that the MT can be thought of as “an additional primary visual area” as it has strong direct input from the lateral geniculate nucleus 41 . In this sense, V1 is not a unique cortical area that receives the earliest visual input from subcortical structures. This information may merge with the signal in the PPC and dorsal occipital cortices, presumably involving computation for the spatial conversion of visual information across different eye positions 29 . Then, with a slight delay to this process, information flows along the ventral stream for further analysis of object semantics. At approximately the time when the anterior part of the temporal cortex receives visual information, which coincides with the second peak of frontal cortical activity, the next saccade will likely occur before the whole-brain computation process becomes silent. This represents a cyclic flow of information that fits the recursive and dynamic nature of visual behavior.
Our findings raise several questions that remain to be investigated. First, what is the neural basis for the distinctive timing of saccade suppression along the ventral stream? The detailed mechanism for saccadic suppression is still controversial, but one of the most plausible hypotheses assumes that the visual areas might receive extra-retinal information that indicates the timing and profile of saccades from the areas controlling saccadic eye movements such as the superior colliculus 42 . However, solely with these models, the differential timing of saccadic suppression along the ventral stream might not be explained. Second, what computational process underlies the distinctive activation pattern of perisaccadic periods across the dSTS, PPC, and VLPFC? At mesoscopic levels of ECoG recording, we found that the dSTS was active while the eyes were moving and suppressed at fixation onset, and vice versa for the PPC. We speculate that the signal in the dSTS is dominated by the reafferent signal from the eyes, as ipsilateral dominant activity was seen just after saccade onset in these electrodes (Fig. 4 ). Eye position was not controlled in our experiment, so gaze position on saccade onset was random across the stimulus movies; however, ipsilateral saccades, on average, tended to bring the stimulus inside the receptive field of neurons under the dSTS electrode. This view is consistent with a previous study of single-unit recordings of macaques showing that MT/medial superior temporal neurons retain stimulus-evoked activation during saccades, and the receptive field moves along with the trajectory of saccades on the stimulus display coordinates 43 . The activation and suppression patterns of the PPC are apparently counterintuitive. The lateral intraparietal region in the primate PPC is known as the parietal eye field that controls saccadic eye movement. This apparent discrepancy might be explained by that fact that the motor receptive field differs across neurons covering the entire lateral hemifield as a population, and they are suppressed if saccades are away from the receptive field. Thus, as ECoG signals represent a summation of multiple neurons, the high-gamma signal was suppressed before saccades and activated at fixation onset. The signal increased rapidly with fixation onset, which was stronger for contralateral saccades and this bias was extended further during fixation. This signal might include a mixture of the eye-position signal, efference copy, and visual information; however, it is difficult to come to a conclusion on this issue with the findings of the present study. A combination of saccade experiments under complete darkness or the precise control of eye position is necessary to disentangle further the precise content of the signal and its interactions across those areas in natural vision.
In any case, it is worth noting the signal dynamics of natural active visual behavior. The visual system is one of the most investigated cortical circuitries, and an enormous number of studies have characterized the functional properties of each region. Many different behavioral tasks have given different functional labels to single cortical regions, which makes it difficult to extract the principal computation of each region. Therefore, a precise description of the timing of activation of cortical areas and which regions exchange signals under natural behavior might provide unique insights for the construction of a visual computation model.
Not only primate visual behavior but also many sensory systems, such as mice whisking and sniffing samples, occur rhythmically 44 , while the rationale for such oscillatory processes is elusive 45 , 46 . The present study provides a simple view of how this timing is regulated. As the visual system is organized hierarchically, it takes a certain amount of time until the final visual center has completed the analysis of new visual information, and then the visual system can decide which direction the eyes should be moved in. This network property may simply determine the interval of visual exploration, and thus the rhythm of visual exploration is intrinsic to the hierarchical neural architecture.
Note that we do not assume that saccade generation is a completely serial process, rather we believe it is a parallel computational process. Indeed, many saccades are generated far earlier than when the higher visual areas receive the information for a new eye position. In this case, the saccade target is determined without the full contribution of the higher visual areas. In fact, a saccade following short fixation is influenced more by visual salience than a saccade after long fixation, probably due to faster computation within the superior colliculus than for semantic analysis in the ventral stream 47 . Guillery and Sherman 38 claimed that any of the steps of cortical sensory computation can send a certain type of instructor signal to the motor apparatus; on the basis of neuroanatomical evidence, they further conceptualized such circuitry in which motor instructions are sent from multiple cortical areas in parallel at different levels of the cortical hierarchy. Our data, showing a distinctive activity profile at fixation termination, support this view. Along with this theory, it might be fruitful to disentangle the information dynamics according to saccade parameters such as prior fixation duration or the visual features of the target that triggered the saccade. Schall et al. 48 proposed a beautiful schema showing an anatomical connectivity gradient in the anterior bank of the arcuate sulcus that coexists with a functional gradient in saccade amplitude at the frontal eye field. The visual system is designed to work under natural behavior, so there must be a reasonable link across different types of saccade (amplitude, interval, and target visual features), its underlying neural architecture, and the visual environment. Our current analysis focused on neural dynamics of the trial averaged signal. The analysis with a single-trial level may reveal such a link across behavior, neural architecture, and the environment in future work.
Our results demonstrate only the most prominent route of signal flow by ECoG recording, which represents the summation of the activity of the heterogeneous neural population on the cortical surface under each electrode. To differentiate information dynamics with a finer resolution by ECoG, further technical challenges need to be addressed. For example, the magnocellular and parvocellular layers in the lateral geniculate nucleus represent functionally distinct information, and these convey information to succeeding areas while keeping a certain degree of segregation across channels 49 , 50 , 51 . In this study, we did not track these two streams independently. Similarly, signal transmission from early visual areas to the dorsal stream (as expected from the dual-stream model) could not be identified in the active exploration condition (while it was supported by Granger causality analysis), and was only observed in the passive-viewing condition. The activity of all heterogenous neurons in the dorsal regions was mixed in a single channel in our analysis, and this may have prevented us from observing signal transmission from V1 to the dorsal stream because of the dominance of the faster visuomotor response just after saccades (Fig. 4a ). Similarly, our study primarily focused on the high-gamma signal as this frequency range is known to be highly correlated with local neural activity; however, signals in different frequency ranges are likely to convey different types of information that are complementary to the high-gamma signal 52 . Future studies require technical advances such as a combination of ECoG and circuit-specific genetic manipulation 53 , 54 , 55 to capture the information stream with a finer resolution and to provide further details for the model of visual information dynamics in active vision.
Ethics information
All procedures in the animal experiments were approved by the Wako Animal Experiment Committee (Animal Care and Use Committee), RIKEN, and all experiments followed the institutional ethical guidelines for the care and use of experimental animals.
ECoG implantation
The ECoG array, consisting of 96 electrodes, was chronically implanted in a half hemisphere of four marmoset monkeys ( Callithrix jacchus ) (two each for the left and right hemispheres) following the protocol developed by Komatsu et al. 19 . The contact area of each gold-plated copper electrode was 0.8 mm in diameter (Cir-Tech Co., Ltd.). We performed a craniotomy of ~2 × 2 cm and gradually inserted the ECoG sheet between the skull and dura. The reference electrode was placed on the dorsal part of the somatosensory cortex on the contralateral hemisphere, and the ground electrode was attached to the skull of the contralateral hemisphere. About 6–8 plastic screws (1.4 × 2.5 mm) were implanted in the skull, and a connector of the electrodes was fixed with the skull and the screws using acrylic cement. A plastic headpost was also attached to the skull beside the connector. The marmoset was immobilized by ketamine(15 mg/kg) and maintained under anesthesia using isoflurane (1–3%) during all surgical procedures. The condition of the animal was monitored by body temperature and arterial blood oxygen saturation. Each surgery was conducted under aseptic conditions.
Electrode localization
We localized the electrodes using computed tomography (CT) and magnetic resonance (MR) imaging. We acquired T2-weighted MR images in advance of ECoG implantation. The marmoset was anesthetized and maintained by 1–3% isoflurane during imaging while their body temperature, heart rate, respiration, and SP0 2 were monitored. T2-weighted MR images were taken using a RARE sequence with the following parameters: TE = 11 ms, TR = 4,000 ms, FA = 90°, RARE factor = 4, matrix size = 178 × 178, slice number = 48, resolution = 0.27 × 0.27 × 0.54 μm. CT images were taken at 1 week after ECoG implantation when the animal had recovered fully. The animal was anesthetized by ketamine (30 mg/kg i.m.) while its respiration was monitored. CT images were taken at an isotropic resolution of 60 μm. To infer electrode positions on the brain surface, the CT images were aligned manually to the T2-weighed images using AFNI software 56 ( http://afni.nimh.nih.gov ). The location of the electrodes was segmented manually on the CT images using 3D Slicer 57 ( http://www.slicer.org ). To help annotate the regions where the electrodes were attached to the brain surface, the cortical annotation of the marmoset MR imaging atlas 58 was transformed onto the subject space by using a free-form deformation named Symmetric Normalization, implemented in the ANTs toolbox 59 . We determined the putative cortical areas of the electrodes based on visual inspection of the MR images compared to the standard atlas 60 and digital annotation based on spatial deformation on the subject brain.
Behavioral paradigm
Four marmoset monkeys observed 18 variations of 10-min movies that contained a variety of naturalistic scenes (such as social interactions of monkeys). To maintain the arousal level of the marmoset, a small amount of liquid reward was delivered while movie viewing. In the passive-viewing task, static images, which were from the same movies of the free-viewing task, were presented sequentially to the marmoset with an average stimulus interval of 1200 ms. Reward timing was distributed randomly with a mean interval of 1500 ms and was independent of gaze behavior. The marmoset viewed 2–4 movies per day. The viewing distance was ~20 cm and the stimulus had a visual angle of 40 × 22.5°. Stimulus and reward timing was controlled by a custom-written MATLAB program using Psychtoolbox 61 , 62 ( http://psychtoolbox.org ) and synchronized with the ECoG signal by an analog trigger signal via the National Instrument DAQ system. Eye movement was obtained by pupil-corneal reflection methods using an infrared camera (GS3-U3-32S4; Point Gray) at 500 Hz with iRecHS software 63 . We performed gaze calibration using a protocol based on the one described by Mitchell et al. 64 . In short, small images of interest to the marmoset were presented on the display for 7 s, and this was repeated with a different stimulus set for a total of five times. Four parameters adjusting gain and shift in the x and y directions were estimated to maximize their gaze to be on one of the images as they spent more time viewing those stimuli than blank space on the display.
ECoG recording
The ECoG signal was recorded at 1 kHz with a bandpass filter ranging from 0.3 to 250 Hz using a data acquisition system (Ripple; Grapevine). The signal was digitalized and multiplexed at the head stage using the common reference, which was placed on the foot region of the somatosensory cortex on the contralateral hemisphere. We analyzed 79 movie viewings with approximately 137,000 saccades, 70 with 135,000, 70 with 128,000, and 63 with 81,000 for each animal, respectively, for the free-viewing task. For the passive-viewing task (Supplementary Fig. S 1 ), we analyzed approximately 40 sessions per animal including 7680 stimulus presentations. We performed this task in two of the four animals with implanted electrodes.
Saccade analysis
Saccades were extracted by an acceleration filter and logistic fitting to eye-movement data following the protocol proposed by Mitchell et al. 64 . Raw eye traces were smoothed by a median filter (100 ms window size) and a second-order Butterworth noncausal filter (−3dB at 50 Hz)to reduce high-frequency noise. Then, the candidates for saccade onset and offset were extracted by the velocity(over 10 degrees/s) and acceleration (over 1000 degrees 2 /seconds) profile of the smoothed eye trace. For each candidate saccade, we fitted the logistic function to the eye trace of the perisaccadic period and compared that with a spline model having the same number of parameters. The logistic model consisted of three parameters fitted to the mean, linear and quadratic trends over 150 ms time series of eye-position data, centered by a pair of candidate saccade onset and offset. The other two parameters fit the width and the amplitude of the logistic function. The spline model was fourth-order spline with an additional parameter for the mean. We consider a saccade only when logistic fitting explained the variance of the perisaccadic eye trace 50% better than that of the spline model.
ECoG spectrogram analysis
To create spectrograms from the ECoG data, we applied a bandpass filter to the raw ECoG signals and then obtained the envelope by applying the Hilbert transformation as the signal intensity of each frequency band. Bandpass width was 4 Hz and center frequency was moved from 4 to 200 Hz. The resulting time courses of the envelope were z -scored in each frequency range and in each channel, and then this was highpass-filtered to remove signal drift (cutoff = 0.1 Hz). High-gamma signal intensity was obtained as an average across 100–160 Hz, which is known to be highly correlated with local neural activity 24 , 25 , 26 . The magnitude and latency of perisaccadic activation peak were obtained as the local maximum from 0 to 250 ms from saccade onset. This was obtained from the averaged signal time course aligned by saccade onset. Saccadic suppression was a local minimum before saccade-evoked activation for each channel.
Contralateral dominance and activity with different fixation durations
To estimate the activation pattern of ipsi/contralateral saccades, we performed the same analysis as above, except that only a subset of saccade onset was used to obtain the averaged signal. Contralateral dominance was determined by subtracting the perisaccadic activity of ipsilateral saccades from that of contralateral saccades. Subtraction was performed using the z -scored spectrogram data. The estimation of p -values is described in the Statistical Analysis sub-section. Similarly, in Fig. 9 , we subsampled the saccades based on the duration of the subsequent fixation.
Activation/suppression driven by saccade or fixation onset
To determine whether the perisaccadic signal was better explained by saccade or fixation onset, we obtained two different average signal time courses aligned by saccade or fixation onset. We computed them with different saccade durations ranging from 20 to 60 ms (bin width = 3 ms, sliding step = 1 ms). To classify the channels to either type 1 (eye-movement type: activation by saccade onset and suppression by fixation onset) or type 2 (fixation type: suppression by saccade onset and activation by fixation onset) in Fig. 5 , we performed the following analysis. First, we obtained the activation peak in different conditions (saccade/fixation onset × saccade duration), and obtained the activation peak and suppression peak timing as local maximum/minimum. In each channel, we considered either only pre- or post-suppression whose signal modulation was larger than the other. Then, we performed regression of those latencies separately to the aligned event timing (saccade activation peak aligned to saccade onset, and fixation aligned to fixation onset, which are the same as for suppression). We obtained a saccade-fixation (s-f) bias by subtracting the regression coefficient for fixation onset from that of saccade onset for each activation and suppression regression coefficient. The s-f bias represents the extent to which degree perisaccadic modulation is better explained by saccade onset (a negative value indicates modulation is better explained by fixation onset). We considered an electrode as type 1 (eye-movement type) if the s-f bias for activation was greater than 0.4 and less than −0.4 for suppression and also that suppression was larger after activation. An electrode was defined as type 2 (fixation type) if the s-f bias for suppression was greater than 0.4 and less than −0.4 for activation and also that suppression occurred before activation. The original s-f values for activation and suppression are shown in Supplementary Fig. 2 . We considered only electrodes whose regression coefficient was obtained reliably, i.e., the residual peak latency time as compared to estimated timing by regression was less than 10 ms on average across different saccade durations.
The trajectory of the high-gamma signal
To visualize the trajectory of high-gamma activity, we used a 3D vertex of the standard marmoset brain model. We assigned the high-gamma activity of each electrode on the nearest vertex of the 3D brain surface derived from nonlinear registration across the atlas and subject MR images as described in the previous section. Signal amplitude was derived from average activity aligned by saccade onset as described earlier. Then, we smoothed the activity power along the cortical surface using the “SurfSmooth” function implemented in AFNI software 56 ( http://afni.nimh.nih.gov ) with 10 mm FWHM. To assess the center of gravity of high-gamma activity, we computed the Fréchet mean of high-gamma activity from all ECoG electrodes as the vertex p that was obtained by the following formula:
where x i is a location of an electrode, w is the high-gamma signal of the electrode, p is any vertex of the 3D brain model, and d(p,x i ) is the distance (geodesics) between p and the electrode x i . Geodesics between electrodes x to an arbitral vertex p on the 3D brain model were computed using the MATLAB (MathWorks) utility “Exact geodesic for triangular meshes.”
Phase-amplitude coupling (PAC) and the traveling wave of theta oscillations
PAC was computed as a circular-linear correlation 65 . When evaluating the time-evolving PAC of the perisaccadic period, we adjusted the size of the sliding window based on the phase frequency so that it contained one cycle, e.g., for 8 Hz theta oscillations, the sliding window size was 125 ms. PAC was computed trial-by-trial, i.e., without averaging phase or amplitude across trials; all time points within the window from all trials were concatenated to compute the circular-linear correlation. In the traveling wave analysis for Fig. 6 , we analyzed the phase of theta oscillations (8 Hz). To determine the propagation direction of the traveling wave, at time t and electrode i , we sought the electrode j that minimized:
where P represents the phase of electrode i at time t . Then, the direction of p i to p j was computed on the surface model. In this case, phase was obtained by signal time course averaged across multiple saccades for a reliable estimation of theta oscillation phase at each timing of each electrode.
Temporal dynamics of whole-brain activation and the effect of subsequent fixation duration
Figure 8b shows a simple summation of the positive part of high-gamma activity for all electrodes from all four animals. The high-gamma signal was averaged time course aligned by saccade onset obtained as described above. Here, we considered only the positive component over baseline activity from all electrodes because the primary focus of the present study was to see to what extent the cortical activity evoked by a saccade remained before the next saccade was likely to occur. In addition, the inclusion of the negative component may underestimate the ongoing activation in other electrodes (see Supplementary Fig. 8 in the case when the negative component was included).
Granger causality
We assessed the degree of signal interaction and its directionality by Granger causality analysis, which is a statistical measurement indicating the extent that one time series with a slight time delay can predict another time series 66 . First, we computed the Bayesian information criterion (BIC) to the autocorrelation of a single channel, which is the regression equation to predict the time series of high-gamma activity of channel i based on the past activity of the same channel i . Then, we performed the same process for regression-predicting channel i from the past activity of channel j . We computed ΔBIC as the subtraction of the BIC for the autoregression model ( i and past i ) from that of a pair of electrodes ( i and past j ). Lower ΔBIC values indicate a higher degree of Granger causality from channel j to channel i . We repeated this process for all combinations of electrodes for each animal.
Statistics and reproducibility
For Figs. 2 c and 3a , statistical significance was obtained by a randomization test in which saccade onset and ECoG signal were derived from different sessions of the same animal. Then, we obtained activation/suppression magnitude from the pseudo-randomly generated perisaccadic signal. We repeated this procedure 500 times, and the p -value was estimated based on the distribution of the resulting magnitude derived from randomization. We computed the P -value by fitting the Gaussian model to the resulting distribution, rather than directly estimating the P -value. This is because it was computationally too heavy to repeat randomization for the thousands of iterations that are required for the direct estimation of the P -value from the distribution. The false discovery rate for multiple comparisons was controlled by Storey’s method 67 . The resulting P -values are shown in Supplementary Fig. 1 .
For Fig. 4 , we performed a randomization test to obtain statistical significance, where labels of contralateral or ipsilateral saccade were randomized within the same session, so that the null hypothesis is the signal pattern should be the same regardless of saccade direction. The following procedure was the same as described above.
In Fig. 9d , the similarity of the spatial–temporal pattern of cortical-wide activity was compared across “time from fixation onset” and “time from fixation end.” The correlation value was computed from 96 × 4 channels from four animals in each time window with different fixation durations, and those values were compared across conditions (“time from fixation onset” and “time from fixation end”) by an unpaired two-tailed t test.
Reporting summary
Further information on research design is available in the Nature Research Reporting Summary linked to this article.
Data availability
Source data underlying Figs. 2 d, 3 c, 4c , and 9d is presented in Supplementary Data 1 . Other data acquired for this study is available upon reasonable request.
Code availability
The custom code used for data analysis in this study is available upon reasonable request.
Van Essen, D. C. & Maunsell, J. H. R. Hierarchical organization and functional streams in the visual cortex. Trends Neurosci. 6 , 370–375 (1983).
Article Google Scholar
Felleman, D. J. & Van Essen, D. C. Distributed hierarchical processing in the primate cerebral cortex. Cereb. Cortex 1 , 1–47 (1991).
Article CAS PubMed Google Scholar
Ungerleider, L. G. & Mishkin, M. Two cortical visual systems. in Analysis of Visual Behavior. 549–586 (eds Ingle, D. J. et al.) (The MIT Press, 1982).
Mishkin, M., Ungerleider, L. G. & Macko, K. A. Object vision and spatial vision: two cortical pathways. Trends Neurosci. 6 , 414–417 (1983).
Goodale, M. A. & Milner, A. D. Separate visual pathways for perception and action. Trends Neurosci. 15 , 20–25 (1992).
Kravitz, D. J., Saleem, K. S., Baker, C. I., Ungerleider, L. G. & Mishkin, M. The ventral visual pathway: an expanded neural framework for the processing of object quality. Trends Cogn. Sci. 17 , 26–49 (2013).
Article PubMed Google Scholar
Kravitz, D. J., Saleem, K. S., Baker, C. I. & Mishkin, M. A new neural framework for visuospatial processing. Nat. Rev. Neurosci. 12 , 217–230 (2011).
Article CAS PubMed PubMed Central Google Scholar
Cowey, A. & Stoerig, P. Blindsight in monkeys. Nature 373 , 247–249 (1995).
Isa, T. & Yoshida, M. Saccade control after V1 lesion revisited. Curr. Opin. Neurobiol. 19 , 608–614 (2009).
Leopold, D. A. Primary visual cortex: awareness and blindsight*. Annu Rev. Neurosci. 35 , 91–109 (2012).
Warner, C. E., Kwan, W. C. & Bourne, J. A. The early maturation of visual cortical area MT is dependent on input from the retinorecipient medial portion of the inferior pulvinar. J. Neurosci. 32 , 17073–17085 (2012).
Beltramo, R. & Scanziani, M. A collicular visual cortex: neocortical space for an ancient midbrain visual structure. Science 363 , 64–69 (2019).
Yu, H.-H. et al. Visually evoked responses in extrastriate area MT after lesions of striate cortex in early life. J. Neurosci. 33 , 12479–12489 (2013).
Warner, C. E., Goldshmit, Y. & Bourne, J. A. Retinal afferents synapse with relay cells targeting the middle temporal area in the pulvinar and lateral geniculate nuclei. Front. Neuroanatomy 4 , 8 (2010).
Kaneko, T. et al. Spatial organization of occipital white matter tracts in the common marmoset. Brain Struct. Funct. 225 , 1313–1326 (2020).
Article PubMed PubMed Central Google Scholar
Takemura, H. et al. A major human white matter pathway between dorsal and ventral visual cortex. Cereb. Cortex 26 , 2205–2214 (2016).
Leopold, D. A. & Park, S. H. Studying the visual brain in its natural rhythm. Neuroimage 216 , 116790 (2020).
Schroeder, C. E., Wilson, D. A., Radman, T., Scharfman, H. & Lakatos, P. Dynamics of active sensing and perceptual selection. Curr. Opin. Neurobiol. 20 , 172–176 (2010).
Komatsu, M., Kaneko, T., Okano, H. & Ichinohe, N. Chronic implantation of whole-cortical electrocorticographic array in the common marmoset. J. Vis. Exp. 144 , e58980 (2019).
Mitchell, J. F. & Leopold, D. A. The marmoset monkey as a model for visual neuroscience. Neurosci. Res. 93 , 20–46 (2015).
Solomon, S. G. & Rosa, M. G. P. A simpler primate brain: the visual system of the marmoset monkey. Front. Neural Circuits 8 , 96 (2014).
Schaeffer, D. J. et al. Task-based fMRI of a free-viewing visuo-saccadic network in the marmoset monkey. Neuroimage 202 , 116147 (2019).
Okano, H. Current status of and perspectives on the application of marmosets in neurobiology. Annu. Rev. Neurosci. 44 , 27–48 (2021).
Ray, S., Crone, N. E., Niebur, E., Franaszczuk, P. J. & Hsiao, S. S. Neural correlates of high-gamma oscillations (60–200 Hz) in macaque local field potentials and their potential implications in electrocorticography. J. Neurosci. 28 , 11526–11536 (2008).
Ray, S. & Maunsell, J. H. R. Different origins of gamma rhythm and high-gamma activity in macaque visual cortex. PLoS Biol. 9 , e1000610 (2011).
Bartoli, E. et al. Functionally distinct gamma range activity revealed by stimulus tuning in human visual cortex. Curr. Biol. 29 , 3345–3358 (2019).
Andersen, R. A., Brotchie, P. R. & Mazzoni, P. Evidence for the lateral intraparietal area as the parietal eye field. Curr. Opin. Neurobiol. 2 , 840–846 (1992).
Wurtz, R. H., McAlonan, K., Cavanaugh, J. & Berman, R. A. Thalamic pathways for active vision. Trends Cogn. Sci. 15 , 177–184 (2011).
Sun, L. D. & Goldberg, M. E. Corollary discharge and oculomotor proprioception: cortical mechanisms for spatially accurate vision. Annu. Rev. Vis. Sci. 2 , 61–84 (2016).
Lowe, K. A. & Schall, J. D. Functional categories of visuomotor neurons in macaque frontal eye field. eNeuro 5 , ENEURO.0131-0118.2018 (2018).
Krauzlis, R. J. The control of voluntary eye movements: new perspectives. Neuroscientist 11 , 124–137 (2005).
Krauzlis, R. J. Recasting the smooth pursuit eye movement system. J. Neurophysiol. 91 , 591–603 (2004).
Majka, P. et al. Open access resource for cellular-resolution analyses of corticocortical connectivity in the marmoset monkey. Nat. Commun. 11 , 1133 (2020).
Zhang, H., Watrous, A. J., Patel, A. & Jacobs, J. Theta and alpha oscillations are traveling waves in the human neocortex. Neuron 98 , 1269–1281 (2018). e1264.
Muller, L., Chavane, F., Reynolds, J. & Sejnowski, T. J. Cortical travelling waves: mechanisms and computational principles. Nat. Rev. Neurosci. 19 , 255 (2018).
Muller, L. et al. Rotating waves during human sleep spindles organize global patterns of activity that repeat precisely through the night. eLife 5 , e17267 (2016).
Davis, Z. W., Muller, L., Martinez-Trujillo, J., Sejnowski, T. & Reynolds, J. H. Spontaneous travelling cortical waves gate perception in behaving primates. Nature 587 , 432-436 (2020).
Guillery, R. W. & Sherman, S. M. The thalamus as a monitor of motor outputs. Philos. Trans. R. Soc. Lond. Ser. B: Biol. Sci. 357 , 1809–1821 (2002).
Article CAS Google Scholar
Thiele, A., Henning, P., Kubischik, M. & Hoffmann, K.-P. Neural mechanisms of saccadic suppression. Science 295 , 2460–2462 (2002).
Rosa, M. G. P. & Tweedale, R. Brain maps, great and small: lessons from comparative studies of primate visual cortical organization. Philos. Trans. R. Soc. B: Biol. Sci. 360 , 665–691 (2005).
Sincich, L. C., Park, K. F., Wohlgemuth, M. J. & Horton, J. C. Bypassing V1: a direct geniculate input to area MT. Nat. Neurosci. 7 , 1123–1128 (2004).
Wurtz, R. H., Joiner, W. M. & Berman, R. A. Neuronal mechanisms for visual stability: progress and problems. Philos. Trans. R. Soc. B: Biol. Sci. 366 , 492–503 (2011).
Inaba, N. & Kawano, K. Neurons in cortical area MST remap the memory trace of visual motion across saccadic eye movements. Proc. Natl Acad. Sci. USA 111 , 7825–7830 (2014).
Berg, R. W. & Kleinfeld, D. Rhythmic whisking by rat: retraction as well as protraction of the vibrissae is under active muscular control. J. Neurophysiol. 89 , 104–117 (2003).
Amit, R., Abeles, D., Bar-Gad, I. & Yuval-Greenberg, S. Temporal dynamics of saccades explained by a self-paced process. Sci. Rep. 7 , 886–886 (2017).
Wutz, A., Muschter, E., van Koningsbruggen, M. G., Weisz, N. & Melcher, D. Temporal integration windows in neural processing and perception aligned to saccadic eye movements. Curr. Biol. 26 , 1659–1668 (2016).
White, B. J., Kan, J. Y., Levy, R., Itti, L. & Munoz, D. P. Superior colliculus encodes visual saliency before the primary visual cortex. Proc. Natl Acad. Sci. USA 114 , 9451–9456 (2017).
Schall, J., Morel, A., King, D. & Bullier, J. Topography of visual cortex connections with frontal eye field in macaque: convergence and segregation of processing streams. J. Neurosci. 15 , 4464–4487 (1995).
Merigan a, W. H. & Maunsell, J. H. R. How parallel are the primate visual pathways? Annu. Rev. Neurosci. 16 , 369–402 (1993).
Ferrera, V. P., Nealey, T. A. & Maunsell, J. H. R. Mixed parvocellular and magnocellular geniculate signals in visual area V4. Nature 358 , 756–758 (1992).
Tootell, R. B. H. & Nasr, S. Columnar segregation of magnocellular and parvocellular streams in human extrastriate cortex. J. Neurosci . 37 , 8014–8032 (2017).
Bastos André, M. et al. Visual areas exert feedforward and feedback influences through distinct frequency channels. Neuron 85 , 390–401 (2015).
Nonomura, S. et al. Monitoring and updating of action selection for goal-directed behavior through the striatal direct and indirect pathways. Neuron 99 , 1302–1314 (2018).
Inoue, K.-I., Takada, M. & Matsumoto, M. Neuronal and behavioural modulations by pathway-selective optogenetic stimulation of the primate oculomotor system. Nat. Commun. 6 , 1–7 (2015).
Amita, H., Kim, H. F., Inoue, K.-I., Takada, M. & Hikosaka, O. Optogenetic manipulation of a value-coding pathway from the primate caudate tail facilitates saccadic gaze shift. Nat. Commun. 11 , 1876 (2020).
Cox, R. W. AFNI: software for analysis and visualization of functional magnetic resonance neuroimages. Comput. Biomed. Res. 29 , 162–173 (1996).
Fedorov, A. et al. 3D slicer as an image computing platform for the quantitative imaging network. Magn. Reson. Imaging 30 , 1323–1341 (2012).
Woodward, A. et al. The Brain/MINDS 3D digital marmoset brain atlas. Sci. Data 5 , 180009 (2018).
Avants, B. B. et al. A reproducible evaluation of ANTs similarity metric performance in brain image registration. Neuroimage 54 , 2033–2044 (2011).
Paxinos, G., Watson, C., Petrides, M., Rosa, M. & Tokuno, H. The Marmoset Brain in Stereotaxic Coordinates (Elsevier Science Publishing, 2012).
Pelli, D. G. The VideoToolbox software for visual psychophysics: transforming numbers into movies. Spat. Vis. 10 , 437–442 (1997).
Brainard, D. H. The psychophysics toolbox. Spat. Vis. 10 , 433–436 (1997).
Matsuda, K., Nagami, T., Sugase, Y., Takemura, A. & Kawano, K. A widely applicable real-time mono/binocular eye tracking system using a high frame-rate digital camera. in Human-Computer Interaction. User Interface Design, Development and Multimodality 593–608 (eds Kurosu, M.) (Springer, 2017).
Mitchell, J. F., Reynolds, J. H. & Miller, C. T. Active vision in marmosets: a model system for visual neuroscience. J. Neurosci. 34 , 1183–1194 (2014).
Kempter, R., Leibold, C., Buzsaki, G., Diba, K. & Schmidt, R. Quantifying circular-linear associations: hippocampal phase precession. J. Neurosci. Methods 207 , 113–124 (2012).
Granger, C. W. J. Investigating causal relations by econometric models and cross-spectral methods. Econometrica 37 , 424–438 (1969).
Storey, J. D. A direct approach to false discovery rates. J. R. Stat. Soc.: Ser. B (Stat. Methodol.) 64 , 479–498 (2002).
Download references
Acknowledgements
We thank Yuri Shinomoto for animal care, training, and awake recordings; Dr. Naomi Hasegawa for veterinary care of the animals; and Drs. Fumiko Seki and Junichi Hata for obtaining the CT images. This work was financially supported by the Brain/MINDS (Brain Mapping by Integrated Neurotechnologies for Disease Studies) project from the Japan Agency for Medical Research and Development (JP18dm0207001, JP19dm0207001, and JP20dm0207001 to H.O.; JP20dm0207069 to M.K.); by JSPS KAKENHI (JP19H04993 to M.K.; 19K20653 to T.K.), and by internal budgets from Keio University including the Program for the Advancement of Research in Core Projects on Longevity of the Keio University Global Research Institute from Keio University (to H.O.).
Author information
These authors contributed equally: Takaaki Kaneko, Misako Komatsu.
Authors and Affiliations
Laboratory for Marmoset Neural Architecture, RIKEN Center for Brain Science, Saitama, Japan
Takaaki Kaneko & Hideyuki Okano
Systems Neuroscience Section, Primate Research Institute, Kyoto University, Aichi, Japan
Takaaki Kaneko
Laboratory for Molecular Analysis of Higher Brain Function, Center for Brain Science, RIKEN, Saitama, Japan
Misako Komatsu & Tetsuo Yamamori
Department of Ultrastructural Research, National Institute of Neuroscience, National Center of Neurology and Psychiatry, Tokyo, Japan
Noritaka Ichinohe
Department of Physiology, Keio University School of Medicine, Tokyo, Japan
Hideyuki Okano
You can also search for this author in PubMed Google Scholar
Contributions
T.K and H.O. conceived the project. M.K., N.I., and T.Y. provided ECoG methodology. T.K developed the behavioral system. T.K and M.K. performed the surgery and the recording. T.K. analyzed the data. All authors discussed the results, and contributed to shape the final intellectual product. T.K wrote the manuscript with feedback from M.K., N.I., T.Y., and H.O.
Corresponding authors
Correspondence to Takaaki Kaneko or Hideyuki Okano .
Ethics declarations
Competing interests.
The authors declare no competing interests.
Peer review
Peer review information.
Communications Biology thanks the anonymous reviewers for their contribution to the peer review of this work. Primary Handling Editor: George Inglis. Peer reviewer reports are available.
Additional information
Publisher’s note Springer Nature remains neutral with regard to jurisdictional claims in published maps and institutional affiliations.
Supplementary information
Transparent peer review file, supplemental information, description of additional supplementary files, supplementary data 1, reporting summary, rights and permissions.
Open Access This article is licensed under a Creative Commons Attribution 4.0 International License, which permits use, sharing, adaptation, distribution and reproduction in any medium or format, as long as you give appropriate credit to the original author(s) and the source, provide a link to the Creative Commons license, and indicate if changes were made. The images or other third party material in this article are included in the article’s Creative Commons license, unless indicated otherwise in a credit line to the material. If material is not included in the article’s Creative Commons license and your intended use is not permitted by statutory regulation or exceeds the permitted use, you will need to obtain permission directly from the copyright holder. To view a copy of this license, visit http://creativecommons.org/licenses/by/4.0/ .
Reprints and permissions
About this article
Cite this article.
Kaneko, T., Komatsu, M., Yamamori, T. et al. Cortical neural dynamics unveil the rhythm of natural visual behavior in marmosets. Commun Biol 5 , 108 (2022). https://doi.org/10.1038/s42003-022-03052-1
Download citation
Received : 01 April 2021
Accepted : 13 January 2022
Published : 03 February 2022
DOI : https://doi.org/10.1038/s42003-022-03052-1
Share this article
Anyone you share the following link with will be able to read this content:
Sorry, a shareable link is not currently available for this article.
Provided by the Springer Nature SharedIt content-sharing initiative
This article is cited by
A novel micro-ecog recording method for recording multisensory neural activity from the parietal to temporal cortices in mice.
- Susumu Setogawa
- Ryota Kanda
- Noriaki Ohkawa
Molecular Brain (2023)
By submitting a comment you agree to abide by our Terms and Community Guidelines . If you find something abusive or that does not comply with our terms or guidelines please flag it as inappropriate.
Quick links
- Explore articles by subject
- Guide to authors
- Editorial policies
Sign up for the Nature Briefing newsletter — what matters in science, free to your inbox daily.

A computational examination of the two-streams hypothesis: which pathway needs a longer memory?
- Research Article
- Published: 10 August 2021
- Volume 16 , pages 149–165, ( 2022 )
Cite this article
- Abolfazl Alipour ORCID: orcid.org/0000-0002-9757-8823 1 , 2 ,
- John M. Beggs 2 , 3 ,
- Joshua W. Brown 1 , 2 &
- Thomas W. James 1 , 2
579 Accesses
3 Altmetric
Explore all metrics
The two visual streams hypothesis is a robust example of neural functional specialization that has inspired countless studies over the past four decades. According to one prominent version of the theory, the fundamental goal of the dorsal visual pathway is the transformation of retinal information for visually-guided motor behavior. To that end, the dorsal stream processes input using absolute (or veridical) metrics only when the movement is initiated, necessitating very little, or no, memory. Conversely, because the ventral visual pathway does not involve motor behavior (its output does not influence the real world), the ventral stream processes input using relative (or illusory) metrics and can accumulate or integrate sensory evidence over long time constants, which provides a substantial capacity for memory. In this study, we tested these relations between functional specialization, processing metrics, and memory by training identical recurrent neural networks to perform either a viewpoint-invariant object classification task or an orientation/size determination task. The former task relies on relative metrics, benefits from accumulating sensory evidence, and is usually attributed to the ventral stream. The latter task relies on absolute metrics, can be computed accurately in the moment, and is usually attributed to the dorsal stream. To quantify the amount of memory required for each task, we chose two types of neural network models. Using a long-short-term memory (LSTM) recurrent network, we found that viewpoint-invariant object categorization (object task) required a longer memory than orientation/size determination (orientation task). Additionally, to dissect this memory effect, we considered factors that contributed to longer memory in object tasks. First, we used two different sets of objects, one with self-occlusion of features and one without. Second, we defined object classes either strictly by visual feature similarity or (more liberally) by semantic label. The models required greater memory when features were self-occluded and when object classes were defined by visual feature similarity, showing that self-occlusion and visual similarity among object task samples are contributing to having a long memory. The same set of tasks modeled using modified leaky-integrator echo state recurrent networks (LiESN), however, did not replicate the results, except under some conditions. This may be because LiESNs cannot perform fine-grained memory adjustments due to their network-wide memory coefficient and fixed recurrent weights. In sum, the LSTM simulations suggest that longer memory is advantageous for performing viewpoint-invariant object classification (a putative ventral stream function) because it allows for interpolation of features across viewpoints. The results further suggest that orientation/size determination (a putative dorsal stream function) does not benefit from longer memory. These findings are consistent with the two visual streams theory of functional specialization.
This is a preview of subscription content, log in via an institution to check access.
Access this article
Subscribe and save.
- Get 10 units per month
- Download Article/Chapter or eBook
- 1 Unit = 1 Article or 1 Chapter
- Cancel anytime
Price includes VAT (Russian Federation)
Instant access to the full article PDF.
Rent this article via DeepDyve
Institutional subscriptions
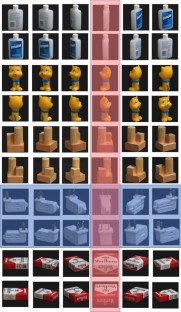
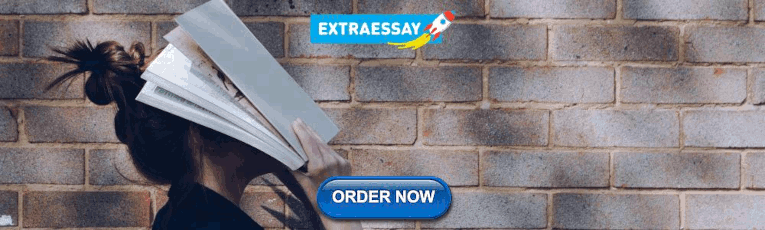
Similar content being viewed by others
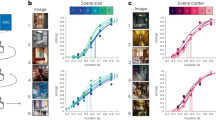
Memorability shapes perceived time (and vice versa)

Behavioral asymmetries in visual short-term memory occur in retinotopic coordinates
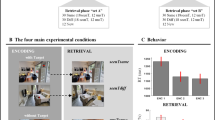
The lateral intraparietal sulcus takes viewpoint changes into account during memory-guided attention in natural scenes
Explore related subjects.
- Artificial Intelligence
Amedi A, Malach R, Hendler T, Peled S, Zohary E (2001) Visuo-haptic object-related activation in the ventral visual pathway. Nat Neurosci 4(3):324–330. https://doi.org/10.1038/85201
Article CAS PubMed Google Scholar
Amedi A, Jacobson G, Hendler T, Malach R, Zohary E (2002) Convergence of visual and tactile shape processing in the human lateral occipital complex. Cereb Cortex 12(11):1202–1212. https://doi.org/10.1093/cercor/12.11.1202
Article PubMed Google Scholar
Amedi A, Stern WM, Camprodon JA, Bermpohl F, Merabet L, Rotman S, Hemond C, Meijer P, Pascual-Leone A (2007) Shape conveyed by visual-to-auditory sensory substitution activates the lateral occipital complex. Nat Neurosci 10(6):687–689. https://doi.org/10.1038/nn1912
Automatic differentiation in PyTorch | OpenReview . (n.d.). Retrieved December 14, 2019, from https://openreview.net/forum?id=BJJsrmfCZ
Bao P, She L, McGill M, Tsao DY (2020) A map of object space in primate inferotemporal cortex. Nature 583(7814):103–108. https://doi.org/10.1038/s41586-020-2350-5
Article CAS PubMed PubMed Central Google Scholar
Bullier J, Nowak LG (1995) Parallel versus serial processing: new vistas on the distributed organization of the visual system. Curr Opin Neurobiol 5(4):497–503. https://doi.org/10.1016/0959-4388(95)80011-5
Bülthoff HH, Edelman S (1992) Psychophysical support for a two-dimensional view interpolation theory of object recognition. Proc Natl Acad Sci 89(1):60–64. https://doi.org/10.1073/pnas.89.1.60
Article PubMed PubMed Central Google Scholar
Bülthoff HH, Edelman SY, Tarr MJ (1995) How are three-dimensional objects represented in the brain? Cereb Cortex 5(3):247–260. https://doi.org/10.1093/cercor/5.3.247
Cadieu CF, Hong H, Yamins DLK, Pinto N, Ardila D, Solomon EA, Majaj NJ, DiCarlo JJ (2014) Deep neural networks rival the representation of primate IT cortex for core visual object recognition. PLoS Comput Biol 10(12):e1003963. https://doi.org/10.1371/journal.pcbi.1003963
Cant JS, Westwood DA, Valyear KF, Goodale MA (2005) No evidence for visuomotor priming in a visually guided action task. Neuropsychologia 43(2):216–226. https://doi.org/10.1016/j.neuropsychologia.2004.11.008
Connolly AC, Guntupalli JS, Gors J, Hanke M, Halchenko YO, Wu Y-C, Abdi H, Haxby JV (2012) The Representation of biological classes in the human brain. J Neurosci 32(8):2608–2618. https://doi.org/10.1523/JNEUROSCI.5547-11.2012
Desimone R, Schein SJ (1987) Visual properties of neurons in area V4 of the macaque: Sensitivity to stimulus form. J Neurophysiol 57(3):835–868. https://doi.org/10.1152/jn.1987.57.3.835
Dijkerman HC, de Haan EHF (2007) Somatosensory processes subserving perception and action. Behav Brain Sci 30(2):189–201. https://doi.org/10.1017/S0140525X07001392
Fedorenko E, Behr MK, Kanwisher N (2011) Functional specificity for high-level linguistic processing in the human brain. Proc Natl Acad Sci 108(39):16428–16433. https://doi.org/10.1073/pnas.1112937108
Flevaris AV, Robertson LC (2016) Spatial frequency selection and integration of global and local information in visual processing: a selective review and tribute to Shlomo Bentin. Neuropsychologia 83:192–200. https://doi.org/10.1016/j.neuropsychologia.2015.10.024
Gallicchio C, Micheli A, Pedrelli L (2017) Deep reservoir computing: a critical experimental analysis. Neurocomputing 268:87–99. https://doi.org/10.1016/j.neucom.2016.12.089
Article Google Scholar
Gallicchio C, Micheli A, Silvestri L (2018) Local Lyapunov exponents of deep echo state networks. Neurocomputing 298:34–45. https://doi.org/10.1016/j.neucom.2017.11.073
Goodale MA, Milner AD, Jakobson LS, Carey DP (1991) A neurological dissociation between perceiving objects and grasping them. Nature 349(6305):154–156. https://doi.org/10.1038/349154a0
Haxby JV, Hoffman EA, Gobbini MI (2000) The distributed human neural system for face perception. Trends Cogn Sci 4(6):223–233. https://doi.org/10.1016/S1364-6613(00)01482-0
Article CAS Google Scholar
Haxby JV, Connolly AC, Guntupalli JS (2014) Decoding neural representational spaces using multivariate pattern analysis. Annu Rev Neurosci 37(1):435–456. https://doi.org/10.1146/annurev-neuro-062012-170325
Hesse C, Schenk T (2014) Delayed action does not always require the ventral stream: a study on a patient with visual form agnosia. Cortex 54:77–91. https://doi.org/10.1016/j.cortex.2014.02.011
Hickok G, Poeppel D (2007) The cortical organization of speech processing. Nat Rev Neurosci 8(5):393–402. https://doi.org/10.1038/nrn2113
Himmelbach M, Nau M, Zündorf I, Erb M, Perenin M-T, Karnath H-O (2009) Brain activation during immediate and delayed reaching in optic ataxia. Neuropsychologia 47(6):1508–1517. https://doi.org/10.1016/j.neuropsychologia.2009.01.033
Hochreiter S, Schmidhuber J (1997) Long short-term memory. Neural Comput 9(8):1735–1780. https://doi.org/10.1162/neco.1997.9.8.1735
Howard MF, Reggia JA (2007) A theory of the visual system biology underlying development of spatial frequency lateralization. Brain Cogn 64(2):111–123. https://doi.org/10.1016/j.bandc.2007.01.004
Jaeger H (2001) The “echo state” approach to analysing and training recurrent neural networks-with an erratum note. Bonn, Germany: German Natl Res Center Inf Technol GMD Tech Rep 148(34):13
Google Scholar
Jaeger H (2004) Harnessing nonlinearity: predicting chaotic systems and saving energy in wireless communication. Science 304(5667):78–80. https://doi.org/10.1126/science.1091277
Jaeger H (2007) Echo state network. Scholarpedia 2(9):2330. https://doi.org/10.4249/scholarpedia.2330
Jager G, Postma A (2003) On the hemispheric specialization for categorical and coordinate spatial relations: a review of the current evidence. Neuropsychologia 41(4):504–515. https://doi.org/10.1016/S0028-3932(02)00086-6
James TW, Kim S (2010) Dorsal and Ventral cortical pathways for visuo-haptic shape integration revealed using fMRI. In: Kaiser J, Naumer MJ (eds) Multisensory object perception in the primate brain. Springer, New York, pp 231–250
Chapter Google Scholar
James TW, Humphrey GK, Gati JS, Servos P, Menon RS, Goodale MA (2002) Haptic study of three-dimensional objects activates extrastriate visual areas. Neuropsychologia 40(10):1706–1714. https://doi.org/10.1016/S0028-3932(02)00017-9
James TW, Stevenson RA, Kim S, VanDerKlok RM, James KH (2011) Shape from sound: Evidence for a shape operator in the lateral occipital cortex. Neuropsychologia 49(7):1807–1815. https://doi.org/10.1016/j.neuropsychologia.2011.03.004
Jax SA, Rosenbaum DA (2007) Hand path priming in manual obstacle avoidance: Evidence that the dorsal stream does not only control visually guided actions in real time. J Exp Psychol Hum Percept Perform 33(2):425–441. https://doi.org/10.1037/0096-1523.33.2.425
Kanezaki A, Matsushita Y, Nishida Y (2018) RotationNet: joint object categorization and pose estimation using multiviews from unsupervised viewpoints. IEEE/CVF Conf Comput Vis Pattern Recogn 2018:5010–5019. https://doi.org/10.1109/CVPR.2018.00526
Kanwisher N (2000) Domain specificity in face perception. Nat Neurosci 3(8):759–763. https://doi.org/10.1038/77664
Kanwisher N (2010) Functional specificity in the human brain: a window into the functional architecture of the mind. Proc Natl Acad Sci 107(25):11163–11170. https://doi.org/10.1073/pnas.1005062107
Kar K, Kubilius J, Schmidt K, Issa EB, DiCarlo JJ (2019) Evidence that recurrent circuits are critical to the ventral stream’s execution of core object recognition behavior. Nat Neurosci. https://doi.org/10.1038/s41593-019-0392-5
Konen CS, Kastner S (2008) Two hierarchically organized neural systems for object information in human visual cortex. Nat Neurosci 11(2):224–231. https://doi.org/10.1038/nn2036
Kravitz DJ, Saleem KS, Baker CI, Mishkin M (2011) A new neural framework for visuospatial processing. Nat Rev Neurosci 12(4):217–230. https://doi.org/10.1038/nrn3008
Lacey S, Tal N, Amedi A, Sathian K (2009) A putative model of multisensory object representation. Brain Topogr 21(3):269–274. https://doi.org/10.1007/s10548-009-0087-4
Lotter W, Kreiman G, Cox D (2016) Deep predictive coding networks for video prediction and unsupervised learning . https://arxiv.org/abs/1605.08104v5
Lotter W, Kreiman G, Cox D (2020) A neural network trained for prediction mimics diverse features of biological neurons and perception. Nat Mach Intell 2(4):210–219. https://doi.org/10.1038/s42256-020-0170-9
Mahon BZ, Cantlon JF (2011) The specialization of function: cognitive and neural perspectives. Cogn Neuropsychol 28(3–4):147–155. https://doi.org/10.1080/02643294.2011.633504
Merigan WH, Maunsell JHR (1993) How parallel are the primate visual pathways? Annu Rev Neurosci 16(1):369–402. https://doi.org/10.1146/annurev.ne.16.030193.002101
Milner AD, Goodale MA (2008) Two visual systems re-viewed. Neuropsychologia 46(3):774–785. https://doi.org/10.1016/j.neuropsychologia.2007.10.005
Murray JD, Bernacchia A, Freedman DJ, Romo R, Wallis JD, Cai X, Padoa-Schioppa C, Pasternak T, Seo H, Lee D, Wang X-J (2014) A hierarchy of intrinsic timescales across primate cortex. Nat Neurosci 17(12):1661–1663. https://doi.org/10.1038/nn.3862
Nene SA, Nayar SK, Murase H (1996) Object image library (COIL-100 .
O’Reilly RC, Wyatte DR, Rohrlich J (2017) Deep predictive learning: a comprehensive model of three visual streams. [q-Bio] . http://arxiv.org/abs/1709.04654
O’Reilly RC, Russin JL, Zolfaghar M, Rohrlich J (2020) Deep Predictive Learning in Neocortex and Pulvinar. [q-Bio] . http://arxiv.org/abs/2006.14800
Pascual-Leone A, Hamilton R (2001) The metamodal organization of the brain. Prog Brain Res 134:427–445
Rauschecker JP (2018) Where, when, and how: are they all sensorimotor? Towards a unified view of the dorsal pathway in vision and audition. Cortex 98:262–268. https://doi.org/10.1016/j.cortex.2017.10.020
Rogers G, Smith D, Schenk T (2009) Immediate and delayed actions share a common visuomotor transformation mechanism: a prism adaptation study. Neuropsychologia 47(6):1546–1552. https://doi.org/10.1016/j.neuropsychologia.2008.12.022
Schaetti N, Salomon M, Couturier R (2016) Echo state networks-based reservoir computing for mnist handwritten digits recognition. In: 2016 IEEE Intl conference on computational science and engineering (CSE) and IEEE Intl conference on embedded and ubiquitous computing (EUC) and 15th Intl symposium on distributed computing and applications for business engineering (DCABES) , pp. 484–491. https://doi.org/10.1109/CSE-EUC-DCABES.2016.229
Schenk T, Hesse C (2018) Do we have distinct systems for immediate and delayed actions? A selective review on the role of visual memory in action. Cortex 98:228–248. https://doi.org/10.1016/j.cortex.2017.05.014
Schrimpf M, Kubilius J, Hong H, Majaj NJ, Rajalingham R, Issa EB, Kar K, Bashivan P, Prescott-Roy J, Schmidt K, Yamins DLK, DiCarlo JJ (2018) Brain-score: which artificial neural network for object recognition is most brain-like? BioRxiv. https://doi.org/10.1101/407007
Siegle JH, Jia X, Durand S, Gale S, Bennett C, Graddis N, Heller G, Ramirez TK, Choi H, Luviano JA, Groblewski PA, Ahmed R, Arkhipov A, Bernard A, Billeh YN, Brown D, Buice MA, Cain N, Caldejon S, Koch C (2021) Survey of spiking in the mouse visual system reveals functional hierarchy. Nature 592(7852):86–92. https://doi.org/10.1038/s41586-020-03171-x
Singhal A, Monaco S, Kaufman LD, Culham JC (2013) Human fMRI reveals that delayed action re-recruits visual perception. PLoS ONE 8(9):e73629. https://doi.org/10.1371/journal.pone.0073629
Stewart CA, Welch V, Plale B, Fox G, Pierce M, Sterling T (2017) Indiana University Pervasive Technology Institute. Bloomington, Indiana .
Tan C, Sun F, Kong T, Zhang W, Yang C, Liu C (2018) A survey on deep transfer learning. In: Kůrková V, Manolopoulos Y, Hammer B, Iliadis L, Maglogiannis I (eds) Artificial neural networks and machine learning – ICANN 2018. Springer, Cham, pp 270–279
van Elk M, van Schie HT, Neggers SFW, Bekkering H (2010) Neural and temporal dynamics underlying visual selection for action. J Neurophysiol 104(2):972–983. https://doi.org/10.1152/jn.01079.2009
Yamins DLK, DiCarlo JJ (2016) Using goal-driven deep learning models to understand sensory cortex. Nat Neurosci 19(3):356–365. https://doi.org/10.1038/nn.4244
Yamins DL, Hong H, Cadieu C, DiCarlo JJ (2013) Hierarchical modular optimization of convolutional networks achieves representations similar to macaque IT and human ventral stream. In Burges CJC, Bottou L, Welling M, Ghahramani Z, Weinberger KQ (Eds.) Advances in Neural Information Processing Systems 26 (pp. 3093–3101). Curran Associates, Inc. http://papers.nips.cc/paper/4991-hierarchical-modular-optimization-of-convolutional-networks-achieves-representations-similar-to-macaque-it-and-human-ventral-stream.pdf
Zeki S (1980) The representation of colours in the cerebral cortex. Nature 284(5755):412–418. https://doi.org/10.1038/284412a0
Zerilli J (2017) Against the “system” module. Philos Psychol 30(3):235–250. https://doi.org/10.1080/09515089.2017.1280145
Zheng H, Yuan J, Chen L (2017) Short-term load forecasting using EMD-LSTM neural networks with a Xgboost algorithm for feature importance evaluation. Energies 10(8):1168. https://doi.org/10.3390/en10081168
Download references
Acknowledgements
This research was supported in part by Lilly Endowment, Inc., through its support for the Indiana University Pervasive Technology Institute and in part by the Indiana METACyt Initiative. The Indiana METACyt Initiative at IU was also supported in part by Lilly Endowment, Inc. The authors acknowledge the Indiana University Pervasive Technology Institute for providing Carbonate HPC resources that have contributed to the research results reported within this paper (Stewart et al. 2017 ).
Author information
Authors and affiliations.
Department of Psychological and Brain Sciences, Indiana University, Bloomington, IN, USA
Abolfazl Alipour, Joshua W. Brown & Thomas W. James
Program in Neuroscience, Indiana University, Bloomington, IN, USA
Abolfazl Alipour, John M. Beggs, Joshua W. Brown & Thomas W. James
Department of Physics, Indiana University, Bloomington, IN, USA
John M. Beggs
You can also search for this author in PubMed Google Scholar
Corresponding author
Correspondence to Thomas W. James .
Additional information
Publisher's note.
Springer Nature remains neutral with regard to jurisdictional claims in published maps and institutional affiliations.
Supplementary Information
Below is the link to the electronic supplementary material.
Supplementary file1 (DOCX 711 kb)
Rights and permissions.
Reprints and permissions
About this article
Alipour, A., Beggs, J.M., Brown, J.W. et al. A computational examination of the two-streams hypothesis: which pathway needs a longer memory?. Cogn Neurodyn 16 , 149–165 (2022). https://doi.org/10.1007/s11571-021-09703-z
Download citation
Received : 07 August 2020
Revised : 26 June 2021
Accepted : 14 July 2021
Published : 10 August 2021
Issue Date : February 2022
DOI : https://doi.org/10.1007/s11571-021-09703-z
Share this article
Anyone you share the following link with will be able to read this content:
Sorry, a shareable link is not currently available for this article.
Provided by the Springer Nature SharedIt content-sharing initiative
- Two-streams hypothesis
- Dorsal and ventral visual pathway
- Convolutional neural networks
- Memory span
- Viewpoint invariant object recognition
- Find a journal
- Publish with us
- Track your research

Member-only story
Deep Learning on Video (Part Two): The Rise of Two-Stream Architectures

Cameron R. Wolfe, Ph.D.
Towards Data Science
This post is the second in a series of blog posts exploring the topic of deep learning on video data. The goal of this series of blog posts is to both overview the history of deep learning on video and provide relevant context for researchers or practitioners looking to become involved in the field. In the first post of the series, I overviewed the earliest publications on the topic that used 3D convolutions to extract learnable features from video.
Within this post, I will overview the next major phase of video deep learning: the introduction and popularization of two-stream network architectures. Two-stream architectures for video recognition are composed of two separate convolutional neural networks (CNNs) — one to handle spatial features and one to handle temporal/motion features. These separate CNNs are typically referred to as the “spatial” and “temporal” networks within the two-stream architecture, and the output of these separate network components can be combined together to form a spatiotemporal video representation. Two-stream architectures yielded massively-improved performance in video action recognition, making them a standard approach to video deep learning for some time.
The post will begin by overviewing relevant preliminary information, such as the definition/formulation of…
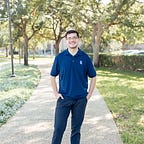
Written by Cameron R. Wolfe, Ph.D.
Director of AI @ Rebuy • Deep Learning Ph.D. • I make AI understandable
Text to speech
Two stream hypothesis of visual processing for navigation in mouse
- October 2020
- Current Opinion in Neurobiology 64:70-78
- This person is not on ResearchGate, or hasn't claimed this research yet.
Discover the world's research
- 25+ million members
- 160+ million publication pages
- 2.3+ billion citations
No full-text available

To read the full-text of this research, you can request a copy directly from the author.
- Andreas Burkhalter

- Paul Linton

- Joseph Del Rosario
- Stefano Coletta

- Navneet Sharma

- Edward A B Horrocks
- Isabelle Mareschal

- Martin Montmerle

- Loïc Magrou

- Guillaume Bresson

- BRAIN STRUCT FUNCT

- Arbora Resulaj

- Carl D Holmgren

- Michael J. Goard
- Tomaso Muzzu

- Emi Uchishiba
- Aman B Saleem
- CURR OPIN NEUROBIOL

- Mary Kate P. Joyce
- Sean Froudist-Walsh
- Amy F.T. Arnsten
- NEUROSCI BIOBEHAV R

- Shanshan Ke
- Pengcheng Li

- Jeffrey N. Stirman
- Christopher R. Dorsett
- Spencer L. Smith

- Joshua T. Trachtenberg

- TRENDS NEUROSCI
- Anderson Speed

- Dan Feldman
- Kristin Scott

- E. Mika Diamanti
- Matteo Carandini
- Lukas Fischer

- Friederike Buck
- Mark T. Harnett
- NAT NEUROSCI
- Saskia de Vries
- Jérôme A. Lecoq
- Michael A. Buice

- Charu Bai Reddy

- Laura Masullo

- Nicolas Alexandre

- Morio Hamada

- James J Knierim

- Giulio Casali
- Sarah Shipley
- Charlie Dowell
- Caswell Barry

- Pierrick Bordiga
- Etienne Save

- Amina A. Kinkhabwala

- David W. Tank
- Stephen P Currie

- Malcolm G. Campbell
- Samuel A. Ocko

- Lisa M Giocomo

- Kirstie Wailes-Newson

- Alexander Robert Patrick Wade

- Jack Waters

- Teppei Matsui

- Steffen Kandler

- Vincent Bonin

- VISUAL NEUROSCI

- Edward M Callaway
- PHILOS T R SOC B

- Bernard Willers

- P NATL ACAD SCI USA
- John O'Keefe
- Quanxin Wang

- Marco Tamietto
- David A Leopold
- Shawn R. Olsen

- David Mahringer

- Cliff Vuong
- J O'Keefe

- Emilio Kropff

- NEUROSCI RES

- Leticia Pedrido
- Carl C H Petersen
- Albert Tsao

- Recruit researchers
- Join for free
- Login Email Tip: Most researchers use their institutional email address as their ResearchGate login Password Forgot password? Keep me logged in Log in or Continue with Google Welcome back! Please log in. Email · Hint Tip: Most researchers use their institutional email address as their ResearchGate login Password Forgot password? Keep me logged in Log in or Continue with Google No account? Sign up
Two Streams hypothesis
The Two-Streams hypothesis is a widely accepted account of visual processing. As visual information exits the occipital lobe , it follows two main channels, or "streams." The ventral stream (also known as the "what pathway") travels to the temporal lobe and is involved with object identification. The dorsal stream (or, "where pathway") terminates in the parietal lobe and process spatial locations.
The hypothesis was originally proposed by L.G. Ungerleider and M. Mishkin in 1982 ( abstract ) and reviewed by G. Ettlinger in 1990 ( abstract ).
Template:WH Template:WS
- Visual system
- Cognitive neuroscience
Navigation menu

An official website of the United States government
The .gov means it’s official. Federal government websites often end in .gov or .mil. Before sharing sensitive information, make sure you’re on a federal government site.
The site is secure. The https:// ensures that you are connecting to the official website and that any information you provide is encrypted and transmitted securely.
- Publications
- Account settings
Preview improvements coming to the PMC website in October 2024. Learn More or Try it out now .
- Advanced Search
- Journal List

How do the two visual streams interact with each other?
A. d. milner.
1 Durham University, Durham, UK
2 Department of Psychology, Science Laboratories, Durham University, South Road, Durham, DH1 3LE UK
The current consensus divides primate cortical visual processing into two broad networks or “streams” composed of highly interconnected areas (Milner and Goodale 2006 , 2008 ; Goodale 2014 ). The ventral stream, passing from primary visual cortex (V1) through to inferior parts of the temporal lobe, is considered to mediate the transformation of the contents of the visual signal into the mental furniture that guides memory, recognition and conscious perception. In contrast the dorsal stream, passing from V1 through to various areas in the posterior parietal lobe, is generally considered to mediate the visual guidance of action, primarily in real time. The brain, however, does not work through mutually insulated subsystems, and indeed there are well-documented interconnections between the two streams. Evidence for contributions from ventral stream systems to the dorsal stream comes from human neuropsychological and neuroimaging research, and indicates a crucial role in mediating complex and flexible visuomotor skills. Complementary evidence points to a role for posterior dorsal-stream visual analysis in certain aspects of 3-D perceptual function in the ventral stream. A series of studies of a patient with visual form agnosia has been instrumental in shaping our knowledge of what each stream can achieve in isolation; but it has also helped us to tease apart the relative dependence of parietal visuomotor systems on direct bottom-up visual inputs versus inputs redirected via perceptual systems within the ventral stream.
Introduction
Otto Creutzfeldt, one of the pre-eminent European neuroscientists of the post-war period, was a major player in the birth and development of Experimental Brain Research , and indeed succeeded Sir John Eccles as the chief editor from 1976, his stewardship ending only with his premature death in January 1992. In the 1980s, Creutzfeldt published two papers ( 1981 , 1985 ), in which he recognized the crucial importance of output connections for a full understanding of the functional roles of different cortical visual areas. It is of course a truism that all visual processing systems ultimately serve to guide behaviour; otherwise they would never have evolved. Creutzfeldt, however, pointed out that quite different patterns of neural efferents had been observed in the then-known cortical visual areas, and presciently proposed that “not only different features of a stimulus are represented but also different behavioural responses to stimuli” (Creutzfeldt 1985 ). This insight has been amply confirmed in a broad array of subsequent research in visual neuroscience (summarized by Milner and Goodale 2006 ). In particular, we know that there are direct pathways from the occipito-parietal “dorsal stream” to subcortical structures such as the superior colliculus, and to other brainstem structures that control the eye muscles and parts of the spinal cord that control the limbs (Glickstein et al. 1985 ; Baizer et al. 1993 ; Borra et al. 2014 ). Areas in the occipito-temporal ventral stream have few or none of these direct connections with motor systems. Instead, the ventral stream interfaces with structures in the temporal and frontal lobes that have been implicated in memory, emotion, and social behaviour, including the amygdala and other mesial temporal structures (Iwai and Yukie 1987 ; Baizer et al. 1993 ). The Two Visual Systems model developed by Milner and Goodale ( 1995 , 2006 ; Goodale and Milner 1992 ; Jeannerod and Rossetti 1993 ) builds upon Creutzfeldt’s insight, conceiving “the functional role of the two streams as largely defined in terms of their outputs to other regions of the brain, what we might call the ‘consumers’ of those outputs, and the tasks those consumers serve” (Foley et al. 2016 ).
The existence of these two interconnected clusters of visual areas in the nonhuman primate neocortex is amply documented in anatomical studies, as collated pictorially by Felleman and Van Essen ( 1991 ) and mathematically by Young ( 1992 ). There may be some value in regarding the dorsal stream itself as being split into two interacting parts (Rizzolatti and Matelli 2003 ), and perhaps likewise the ventral stream (Aflalo and Graziano 2011 ). What is not in dispute, however, is that several of the early connectional studies showed—and new anatomical studies continue to show—clear, if less profuse, interconnections between the two visual streams themselves. For example, investigations have found bidirectional projections between temporal area TEO and the lateral intraparietal area, LIP (Distler et al. 1993 ; Webster et al. 1994 ). Projections to inferior temporal cortex (TE) in monkeys have been reported more recently from areas within the intraparietal sulcus including the anterior intraparietal area, AIP (Zhong and Rockland 2003 ; Borra et al. 2008 , 2010 ).
Clearly then, although anatomy tells us that visual areas may work within cooperative conglomerates to perform distinctive roles, these conglomerates also need to talk to each other. The most obvious role for this inter-stream communication would be to provide an integration between the two disparate systems, such that the animal is provided with a unitary visual life. The likelihood of such a role was recognized in the earliest formulations of Two Visual Systems theory (Goodale and Milner 1992 ; Milner and Goodale 1993 , 1995 ). For example, in the final section of the Milner and Goodale ( 1995 ) book, we wrote:
“efficiently programmed and coordinated behaviour requires that neither the ventral nor the dorsal stream should work in isolation: they should cooperate. It is, therefore, to be expected that there will be reciprocal cross-connections between areas in the two streams, and there is extensive anatomical evidence that this is so (Felleman and Van Essen 1991 ). Understanding these interactions would take us some way towards answering what is one of the central questions in modern neuroscience: how is sensory information transformed into purposeful acts?”
Cloutman ( 2013 ) outlines three potential forms of cross-stream interaction:
- computations along the two pathways proceed strictly independently and in parallel, reintegrating at some ‘terminal’ stage of processing within a shared target brain region (the ‘independent processing’ account);
- processing along the separate pathways is modulated by the existence of feedback loops which transmit information from ‘downstream’ brain regions, including information processed along the complementary stream (the ‘feedback’ account); and
- information is transferred between the two systems at multiple stages and locations along their processing pathways (the ‘continuous cross-talk’ account).
In the present article I will concentrate on possibility (3), though a resolution of the problems of visual integration and mental unification alluded to in the above quotation from Milner and Goodale ( 1995 ) is likely to involve Cloutman’s possibilities (1) and (2) (see Goodale and Milner 2004 ; Milner and Goodale 2006 ). The former may well operate via common projections to the lateral prefrontal cortex or superior temporal sulcus (Borra et al. 2008 , 2010 ); the latter via back-projections to early retinotopic cortical areas (Rockland and Van Hoesen 1994 ; Borra and Rockland 2011 ).
Visuomotor performance in patient D.F.
A major inspiration for Milner and Goodale’s original formulation of the Two Visual Systems model was provided by a series of behavioural observations carried out with a patient (D.F.) suffering from visual form agnosia. These studies showed that D.F. was able to perform manual actions guided by visual information that was not available to her for making perceptual reports. First, Milner et al. ( 1991 ) used a vertically mounted disc containing a large slot which was randomly set at different angles. They found that D.F.’s attempts to describe or otherwise report the orientation of the slot showed little or no relationship to its orientation. When asked to insert her hand or a hand-held card into the slot from a starting position an arm’s length away, however, she showed no difficulty. Video recordings showed that her hand began to rotate in the appropriate direction as soon as it left the start position. In short, although she could not report the orientation of the slot, she could direct her hand or a card into it without difficulty. The results were replicated by Goodale et al. ( 1991 ), who showed that D.F. was quite unable to match the orientation of a slot using a hand-held plaque while keeping her arm stationary, though she performed accurately when ‘posting’ the same plaque into the slot. A similar dissociation was later found with solid rectangular blocks when presented in front of her at different orientations: D.F.’s perceptual judgements of the stimuli were poor, yet her grasping movements to pick them up were accurate, and were preceded by normal anticipatory orientation of the wrist during the course of the reaching movement (Carey et al. 1996 ).
Goodale et al. ( 1991 ) observed similar dissociations between perceptual report and visuomotor control in D.F. when she had to deal with the intrinsic properties of objects such as their width and shape. Thus, D.F.’s hand exhibited normal anticipatory shaping as she reached out to pick up blocks of different width—ones that she could not distinguish perceptually. In one such test, solid blocks of matched surface area but different widths (based on a shape discrimination task devised by Efron 1969 ) were used. Healthy subjects adjust their fingerthumb separation in advance of arrival at the object during such reaching behaviour (Jeannerod 1986 ; Jakobson and Goodale 1991 ), reaching a maximum grip aperture at about 75% of the way toward the object. This maximum aperture is strongly related to the width of the object. D.F. showed this visual scaling of her grip size quite normally during reaching. Yet when she was asked to use her finger and thumb to make a perceptual judgement of the object’s width on a separate series of trials (in a manner analogous to the matching task she had carried out earlier with the card and slot), her responses were unrelated to the stimulus, and showed high variation from trial to trial.
Finally, D.F.’s sensitivity to the outline shape of irregular flat objects was tested. To pick up the object successfully, the fingers and thumb had to be placed at appropriate opposition points on the object’s perimeter. D.F.’s performance with these stimuli yielded another clear dissociation between perception and action: she was able to position her index finger and thumb in stable positions on either side of each object during grasping, but was quite unable to discriminate one object from another (Goodale et al. 1994a ). In contrast, the authors found that a patient with bilateral parietal damage and optic ataxia (R.V.) failed to place her fingers correctly on the objects, with the result that they would frequently slip out of her grasp. Yet R.V. could readily distinguish these objects from one another.
Taken together, these findings clearly indicate the preserved operation in D.F. of a system for visual control of manual actions on the basis of orientation, width, and shape, despite her profound visual form agnosia. Indeed in the study mentioned above by Carey et al. ( 1996 ), we further confirmed that orientation and width could work together in concert to guide D.F.’s hand and fingers, in that she was able to reach out and grasp solid plaques of different dimensions placed at varying orientations in front of her, with an accuracy equal to healthy controls. Following the early studies of D.F.’s performance, it was proposed that her lesion had critically damaged the ventral stream of cortical processing, but left the bulk of the dorsal stream intact (Goodale and Milner 1992 ; Milner and Goodale 1993 , 1995 ). That is, we proposed that her spared visuomotor capacities reflected a relatively intact dorsal stream, in the presence of a severely compromised ventral stream. Recent functional structural MRI studies of D.F. indicate that actually there is some bilateral (particularly right-hemisphere) posterior parietal damage present in her brain (James et al. 2003 ; Bridge et al. 2013 ). Yet the visuomotor area most concerned with grasping (AIP) is robustly and selectively activated during prehension in D.F., whereas her ventral stream area LOC (lateral occipital cortex) appears to be completely destroyed bilaterally, and no selective activation to visual shapes is detectable (James et al. 2003 ).
Given this pattern of brain damage, it is clear that the information that area LOC would normally share with the dorsal stream would not be available in D.F.: in other words, not only would she suffer the direct effects of bilateral LOC damage on shape perception, she would also suffer the indirect effects of losing ventral-stream influence on dorsal-stream processing. This has caused a number of specific problems in visuomotor tasks whose complexity exceeds the basic ones that are described above. Some of the ventral-to-dorsal stream interactions whose absence underlie these impairments were predicted on the basis of the model as first set out by Goodale and Milner ( 1992 ), for example in delayed action (Goodale et al. 1994c ); and others were predicted when the ramifications of the model were further thought out, such as in visual judgements of weight (Dijkerman et al. 2004 ). Yet other ventral-dorsal interactions, however, were not predicted, but became apparent as we sought to refine our understanding through empirical investigation, such as in D.F.’s responses to multiple visual orientations (Goodale et al. 1994b ); and also unpredicted were the dorsal-to-ventral interactions that are reviewed later in this paper, such as those involved in stereoscopic depth perception.
Evidence for ventral-to-dorsal traffic
Multiple orientations.
Although D.F. could use the orientation of a target to control the orientation of her hand in a posting or grasping task, the question arose as to whether she could use the orientation of a more complex target stimulus to control hand rotation during posting or grasping. We first explored this question by asking her to post a T-shaped object into a T-shaped aperture (Goodale et al. 1994b ). On different test trials, the target aperture was presented at different orientations, such that its principal axis was oriented at ±30° or ±60° from the vertical. We found that D.F. succeeded in smoothly inserting the T shape on about half of the trials; but on the other trials her errors were almost always made at approximately 90°. This result confirms that D.F. is able to use the orientation of one visible edge to determine her manual posting behaviour, but suggests that she cannot combine two visual orientations to form a composite shape to guide such actions. In a second study designed to test D.F.’s spared visuomotor abilities with multiple orientations, we found that her hand orientation en route towards grasping a cross-shaped object was insensitive to changes in orientation of the object, averaging the same default wrist posture whatever the stimulus orientation (Carey et al. 1996 ).
The behavioural variable being measured in these experiments was the orientation of the wrist, either directly (in cross grasping) or indirectly by virtue of turning the T-shaped object to ‘post’ it. Clearly this element of prehension has only one degree of freedom, and in the absence of ventral stream processing may be driven not by shape as such, but rather by a single dominant axis present in the display. Such a form of primitive visuomotor control would perhaps account for the results of the T-posting study. A clear principal axis, however, was not present in the cross experiment as the stimulus was doubly symmetrical: as a result it may have been incapable of controlling wrist orientation at all without the additional influence of ventral-stream shape processing. It may be hypothesized that in D.F.’s isolated dorsal stream, rotation of the wrist is only sensitive to one major visual axis at a time, rendering it limited to translating visual orientation into oriented action reliably only with stimuli where there is a single major axis. Recent psychophysical evidence supports this idea (Almeida et al. 2014 ). If this is so, then a healthy person’s performance of these two more visually complex tasks may depend upon input from shape processing systems in the ventral stream that are able to upgrade such a first-order orientation visuomotor channel in the dorsal stream into a more flexible one that can simultaneously handle multiple visual axes.
Delayed action
D.F.’s ability to scale her grasp to the size of a goal object is striking, but nevertheless has certain revealing limitations. In a seminal early study, Goodale et al. ( 1994c ) examined the effects of interposing a delay between briefly presenting an object to D.F. and then allowing her to reach out to perform a grasp as if the object were still there. In control subjects, grip size still correlated well with object width, even for delays as long as 30 s. In D.F., however, all evidence of grip scaling had disappeared after a delay of only 2 s. Kinematic analysis showed that even the grasping movements of healthy subjects in the delay condition took a very different form from those directed at objects that were physically present. It was inferred that when making such ‘pantomimed’ grasps, healthy subjects had to use a stored perceptual representation of the object generated in the ventral stream to supplement the direct dorsal-stream route dedicated to normal target-directed grasping. In other words, delayed action would require some form of cross-stream information transmission in the ventral-to-dorsal direction at the time of the action. This interpretation was borne out by the later discovery that transcranial magnetic stimulation (TMS applied to the dorsal stream (area AIP) in healthy subjects compromised both immediate and delayed grasping, whereas TMS to the ventral stream (area LOC) compromised only delayed grasping (Cohen et al. 2009 ). Clearly D.F’s brain damage would have precluded the use of this circuitous route to the dorsal steam via LOC. In a later study it was found using fMRI that both LOC and also early visual cortex (including V1) were re-activated at the end of the delay period—even though the participants remained in complete darkness with no visual stimulation at the time of the action (Singhal et al. 2013 ). (As an aside, the authors observed higher activation for grasping than reaching within early visual cortex, during both vision and subsequent action execution. This may indicate the existence of downstream priming that could affect both streams in the manner of Cloutman’s ( 2013 ) second putative cross-stream mechanism.)
D.F. performs accurately in reaching towards individual items distributed within her visual field, despite a severe deficit in perceiving spatial relationships among the items (Carey et al. 2006 ). Milner et al. ( 1999 ), however, found that here too the imposition of a delay impaired D.F.’s performance. Using laterally located targets (four LEDs spaced 2.5 deg apart on either side of a fixation point), they reported that D.F.’s errors were similar to those of healthy controls when she was allowed to respond immediately, but were 3 times greater than control values after a 10 s delay. Similar results have been reported recently in a patient with hemiagnosia caused by unilateral ventral-stream damage (Cornelsen et al. 2016 ). This patient performed considerably worse than controls for the most peripheral contralesional target during delayed reaching, but was proficient at immediate reaching. D.F. also showed a comparable dissociation when she was asked to make saccadic eye movements to a target location, either directly or after a delay when the target was no longer there (Milner et al. 1999 ; Rossit et al. 2010 ). In the latter case her accuracy dropped precipitously.
In summary, the behaviour of D.F. and of healthy subjects in delayed grasping and reaching is consistent with an assumption that visuomotor mechanisms within the dorsal stream, if left to their own devices, operate very much in the ‘here and now’. When movements have to be generated after even short delays, the brain has to make use of stored perceptual representations constructed within the ventral-stream via cross-stream inputs. Unfortunately of course this ventral-stream source of information is no longer available for D.F.’s dorsal stream to receive.
Grasping spatial relationships
Dijkerman et al. ( 1998 ) tested D.F. on a complex prehension task in which she was presented with transparent circular discs, each of which had circular holes cut in it. D.F. was asked to reach out and grasp the disc by placing her fingers through the holes. The discs either had three holes (for forefinger, middle finger, and thumb) or two holes (for forefinger and thumb). In the three-hole task, D.F. was quite unable to adjust her grip aperture with respect to the distance between the forefinger and thumb holes or her hand orientation with regard to their relative orientation of the holes. Although she was able to orient her hand appropriately for the two-hole disks, she still remained unable to adjust her grip aperture to the distance between the holes. McIntosh et al. ( 2004 ) subsequently clarified these findings. First, they replicated the earlier findings that D.F. was unable to produce normal prehension movements when attempting to grasp transparent stimuli by placing her digits into holes. However, they went on to show, using parallel pairs of elongated stimuli, that D.F. was perfectly able to scale her grip with respect to the separation between a pair of objects, just as well as with respect to the width of a single stimulus.
These findings are consistent with the proposal that allocentric processing of spatial information where three or more locations need to be combined requires access to a functioning ventral stream, whether the information is being used to guide a motor response (Dijkerman et al. 1998 ; McIntosh et al. 2004 ) or not (Murphy et al. 1998 ; Carey et al. 2006 , 2009 ). If this is correct, then clearly grasping a 3-hole disc would require ventral-to-dorsal crosstalk. In addition, there seems to be a separate problem when the task requires insertion of digits into particular holes in an object rather than the more natural grasping of outer surfaces of objects. Just as concluded above for multiple contour orientations, although simple objects may offer themselves directly to the dorsal stream for grasping, an intact ventral stream seems to be required to respond appropriately to complex stimuli. This limitation on the capacity of dorsal visuomotor channels again most probably demands the intercession of ventral-to-dorsal crosstalk.
Grasping orientation in depth
Dijkerman et al. ( 1996 ) devised a grasping task designed to investigate D.F.’s ability to use binocular and monocular information about the orientation of an object in the depth plane for perceptual and visuomotor purposes. A square plaque was presented at 7 different slants for subjects to reach out and grasp using a precision grip, under binocular and monocular viewing conditions. (In separate testing they were asked to match the slant of the target using a hand-held plaque: we will return to this later). D.F.’s scaling of her handgrip orientation was found to be normal under binocular conditions, but substantially impaired using monocular vision. This finding is consistent with reports that many neurons in the monkey’s intraparietal area CIP respond selectively to orientation in depth, and that many of these cells require binocular viewing of the target, becoming less responsive when one eye is occluded (Sakata et al. 1995 ; Shikata et al. 1996 ). Presumably when binocular vision is unavailable, the extraction of depth information for visuomotor control has to rely on pictorial cues like texture, illumination gradients, and (particularly relevant here) perspective. Other evidence indicates that perspective cues are not available to D.F. due to her ventral stream damage (Marotta et al. 1997 ; Mon-Williams et al. 2001 ), so that when deprived of binocular cues in Dijkerman et al.’s ( 1996 ) study, her performance inevitably deteriorated. Corroborative evidence was later obtained in another patient with visual form agnosia, S.B. (Lê et al. 2002 ), who showed similar results (Dijkerman et al. 2004 ). Taken together, this evidence strongly suggests that parietal visuomotor systems—unless informed by ventral stream crosstalk—are critically dependent on binocular input for processing orientation in depth.
Pursuing this logic further, Verhagen et al. ( 2008 ) argued that although both viewing conditions in Dijkerman et al.’s ( 1996 ) task are likely to engage both streams in healthy brains, the dorsal stream would need to rely more on inputs from the ventral stream as the relevance of pictorial depth cues increases. In particular, increasing the object slant increases the importance of pictorial cues like perspective whereas the presence of binocular cues decreases that need (Knill and Saunders 2003 ). Verhagen et al. ( 2008 ) used functional MRI to test for such dynamic cross-stream interactions and found that area AIP (in conjunction with ventral premotor cortex, PMv) and area LOC in the ventral visual stream showed differential slant-related responses, with activity increasing when monocular viewing conditions and increasing slant required the processing of pictorial depth cues. These conditions also increased the functional coupling of AIP with both LOC and PMv. They, therefore, argue that the trial-to-trial demands of the task modulate the extent to which the dorsal stream imports perceptual information into the prehension plan in an online fashion.
Control of grip force
While the data from D.F. indicate that ventral-stream information about width and shape is not required for the dorsal stream to mediate accurate scaling of finger-thumb grip size with simple objects, there have long been suggestions that an equally important aspect of grip control may not be so autonomous. The grip forces we exert when picking up an object are normally tailored to its expected weight, which other things being equal, will vary with its apparent size (see Johansson and Cole 1992 ). But such expectations depend on learned associations, rather than being directly specified in the visual information available as we look at an object, and therefore would be expected a priori to rely on the kinds of visual processing for which the ventral stream is specialized (Milner and Goodale 2006 ). Data consistent with this interpretation come from studies using pictorial illusions of size, which have been shown to have little or no effect on the scaling of grip aperture in flight (Aglioti et al. 1995 ; Ganel et al. 2008 ). Such illusions nevertheless show a strong effect not only on size perception but also on the calibration of grip forces used to pick up a target object (Brenner and Smeets 1996 ; Jackson and Shaw 2000 ). That the ventral stream plays an important role in judging weight also gains support from preliminary evidence that both of our patients with visual form agnosia and ventral-stream lesions, D.F. and S.B., fail to show a significant visual size-weight illusion, which in some sense also depends on visually generated expectations about weight (McIntosh 2000 ; Dijkerman et al. 2004 ; but see also; Flanagan and Beltzner 2000 ). Yet in a separate test they both experienced a strong kinaesthetic size-weight illusion, in which they simply felt the size and shape of the objects while blindfolded.
More direct evidence of the role of the ventral stream in weight perception has recently been reported using functional MRI: using multivoxel pattern analysis, Gallivan et al. ( 2014 ) have found that the weight of an object being lifted is represented in specific “visual” areas in occipito-temporal cortex. Even more interestingly, the pattern of response in ventral stream visual areas varied according to whether an object’s predicted weight was based on repeated experience of lifting a specific object, or from associations between the surface properties (colour and texture) of the object and its weight. In the former case, the activations were biased towards lateral occipital cortex (associated with shape perception), while in the latter they were biased towards posterior fusiform areas close to the anterior part of the collateral sulcus (associated with surface properties: Cavina-Pratesi et al. 2010a , b ). These results provide evidence that the ventral visual pathway is actively and flexibly engaged in processing object weight. Since it is known from TMS studies (Davare et al. 2007 ) that dorsal-stream area AIP is critically involved in grip-force control as well as in grip-size scaling, we may assume that there is constant direct and flexible traffic between this area and the ventral-stream areas representing shape and surface properties. That is, just as we saw in the previous section from Verhagen et al.’s ( 2008 ) work on the visuomotor control of grasping objects oriented in depth, we see here further evidence for inter-stream interactions being recruited dynamically according to the current behavioural demands.
Semantic influences on action
Advance knowledge of object function allows us to fine-tune our actions to suit the objects we may interact with in our daily life. For example, D.F. makes mistakes in picking up everyday objects like tools and cutlery, not in mis-scaling her grip or mis-orienting her hand, but in grasping the object in a manner appropriate to its use (Carey et al. 1996 ). Semantic knowledge about what an object is for evidently needs to be provided by the ventral stream for the object to be grasped correctly. This has been nicely illustrated in an experiment by Creem and Proffitt ( 2001 ), which showed that healthy subjects too can make ‘functional’ without ‘metric’ visuomotor errors, under conditions of cognitive overload from a concurrent verbal memory task (Creem and Proffitt 2001 ). Given the close association of apraxia with left-hemisphere lesions, it may be significant that a concurrent visuospatial task did not interfere with grip selection in this experiment (Creem and Proffitt 2001 ). These observations illustrate the obvious point that one’s acquired knowledge of a manufactured object’s function permits the brain to anticipate what likely use will be made of the object by a person grasping it.
The intimate collaboration between the visual streams when an observer is faced with tools is revealed by fMRI studies even when no act of grasping can take place (since the tools are presented as pictures). As Chao et al. ( 1999 ) and Chao and Martin ( 2000 ) showed some years ago, viewing tools selectively activates areas in both the ventral stream and the dorsal stream, chiefly in the left hemisphere in both cases (see Lewis 2006 for review). More recently, fMRI studies using functional connectivity analysis have shown that the two areas concerned (in left posterior middle temporal cortex and intraparietal sulcus, respectively) are mutually interconnected (Bracci et al. 2012 ; Hutchison et al. 2014 ), in agreement with an earlier DTI study by Ramayya et al. ( 2010 ). Evidence consistent with a ventral-to-dorsal direction of transmission comes from a study by Almeida et al. ( 2013 ), who have recently shown that increased neural responses to tool stimuli are still observed in the inferior parietal lobule even when the stimuli are transmitted visually only to the ventral stream. (The experimenters achieved this by presenting the tools as chromatically defined red/green isoluminant stimuli, thereby restricting inputs to parvocellular retinal channels).
Interestingly however, Mahon et al. ( 2007 ), using repetition MR suppression, have shown that responses to tools in both visual streams within the left hemisphere are coded according to action properties associated with the stimuli—not only the tool-responsive areas in the dorsal stream, where this might be more expected. This suggests a complementary dorsal-to-ventral interaction: that is, two-way traffic within a complex temporo-parietal “tool network”. Almeida et al. ( 2010 ) have presented supporting evidence for this using continuous flash suppression to one eye, a technique that effectively blocks ventral-stream processing of stimuli presented to the other eye, while allowing dorsal stream processing to proceed (cf. Fang and He 2005 ). They still found semantic priming effects from such “unseen” stimuli on the naming and categorization of pictures of tools (though not animals). They argue that information about tools extracted from the prime by the dorsal stream (e.g. “graspability”) can be transmitted to ventral stream processing to aid tool identification. Consistent with such dorsal-to-ventral recursive traffic, Gallivan et al. ( 2013 ) have found using fMRI and pattern classification methods that information about planned actions is coded to some degree in ventral-stream areas, including the tool-related area.
As an aside, it should be noted here that there is accumulating evidence that semantic knowledge can influence not just the selection of alternative actions, but even the parameters of the movements themselves. For example the known size of familiar objects such as different brands of matchboxes (McIntosh and Lashley 2008 ), and the use of meaningful as opposed to meaningless objects (Borchers and Himmelbach 2012 ) have been found to affect grip aperture during grasping. These effects may be attributable to the prior acquisition of visuomotor habits following repeated actions with the familiar objects in the past, rather than to any mis-scaling on the basis of current visual size processing, on the part of the healthy subjects used in the studies. But either way, any full understanding of everyday visuomotor acts must recognize these phenomena and allow that inter-stream communication is probably involved at some stage in their genesis.
Section summary: what has the ventral stream ever done for the dorsal stream?
The study of visual form agnosia has flagged up a number of visuomotor tasks that the dorsal stream can only perform with the help of crosstalk from the ventral stream. For example, patient D.F. can use simple visual information about shape, width, and orientation to guide her reaching and grasping as accurately as a healthy person, but when presented with more challenging tasks requiring more complex visual analysis her performance deteriorates markedly. We may assume that the brain’s visuomotor control systems rely on ventral-stream mediation to perform these various kinds of supplementary visual analysis. Likewise when a delay is interposed between a stimulus presentation and a reaching, grasping or saccadic response towards it, again D.F.’s performance deteriorates (as discussed earlier). We must infer here again that the ventral stream is required for us to perform this task; the dorsal stream appears to have no ‘memory’ of the stimulus that was presented, and depends on crosstalk from ventral areas. Similarly when asked to report manually a shape she is shown, or to ‘pantomime’ its size or orientation, D.F. is unable to do so: these capacities evidently depend on the mediation of ventral-stream processing. Use of pictorial depth cues in guiding grasping in depth also seems to rely on ventral stream inputs, particularly when binocular cues need to be supplemented or are absent; and the planning of how to grasp an object to optimize end state comfort likewise requires input from the ventral stream.
Evidence for dorsal-to-ventral traffic
Shape and orientation discrimination.
Although D.F. normally has severe difficulties in distinguishing among rectangular blocks of different aspect ratio, there have occasionally been instances where she performed somewhat better than would have been predicted. In one such experiment, a square and a rectangular block that she could not discriminate between verbally were presented together, and D.F. was asked to pick up one of them (e.g. the square) over a series of trials (Murphy et al. 1996 ). Although she achieved above-chance success in this task, closer examination revealed that rather than always reaching for the target object directly, as healthy subjects do, she often changed course mid-flight. It was surmised that she was able to monitor the aperture of her grasp as she reached towards one of the objects, and was then able to use this information either to continue her reach trajectory or to change it when she detected that her reach was directed at the wrong object.
D.F.’s ability to use self-cueing extends to the dimension of orientation. Dijkerman and Milner ( 1997 ) asked D.F. to copy a single line presented at one of a variety of orientations on a sheet of paper by drawing on an adjacent sheet. According to her performance with ‘slot-posting’ she should not have been able to do this task: but in practice she performed well. The authors observed that D.F. proceeded by first ‘tracing’ a line in the air above the line presented, and then making the same movement on paper with the pencil. But even when D.F. was required to stop tracing in the air, she continued to copy lines far better than chance. To achieve this she would look at the original line for a few seconds on each trial, with her pencil on the other piece of paper, before then quickly drawing her line. Afterwards she explained that instead of explicitly tracing in the air over the line, she imagined doing that, while keeping her pencil ready. She then drew her line quickly, before the imagined movement had faded from her mind. When prevented from doing this by having to copy the line as soon as it was presented, D.F. now drew randomly oriented lines bearing no systematic relationship to the line she was shown.
The above findings reflect forms of self-cueing that may not require direct neuronal cross connections. In a later study, however, we found evidence that this self-cueing could be completely internalized: the very act of picking up rectangular blocks raised D.F.’s ability to discriminate the form of the target object from chance to above-chance performance (Schenk and Milner 2006 ). The authors used a square and an oblong block equated for surface area like Murphy et al. ( 1996 ), presenting them one at once. They found that D.F. could name the object while concurrently grasping it at a level significantly higher than when she made judgements without grasping, which remained at chance. The results of control experiments ruled out proprioceptive and efferent cues, supporting the idea that internal cues derived from visuomotor processing could directly influence discriminative responses in D.F. A further test showed that the grasping-induced discrimination improvement disappeared when the target objects differed only with respect to their shape but not their width, suggesting that shape information per se did not underlie D.F.’s grasping in the task. While the results do not mean that D.F.’s conscious perception of the block’s geometry improved during concurrent grasping, it remains a possibility that dorsal-to-ventral signals might have biased her binary decisions to above-chance levels via spared temporal lobe systems.
Stereoscopic depth perception
In the Dijkerman et al. ( 1996 ) study discussed earlier, D.F. was able to perform well at adjusting her handgrip orientation to match the slant of an object, though monocular viewing reduced her performance. In contrast, her perception of slant, as indicated by her ability to match it using a hand-held object of the same dimensions, was poor, falling to chance under monocular viewing. This difference provides another example of the dissociation between perception and action that characterizes D.F.’s visual life. However, the question still arises as to how binocular viewing rendered her able to match object slant at an above-chance level. Given that there are dedicated mechanisms for computing orientation in depth in dorsal stream area AIP (Sakata et al. 1995 ), might it be that when binocular cues are available to her, D.F. can derive cross-stream benefit from those AIP neurons to inform her slant judgements? D.F. does have a surviving ability to judge depth as tested with Julesz stereograms (Milner et al. 1991 ; Read et al. 2010 ), and actually falls within the range of healthy controls when judging slant created with full-field stereograms (Read et al. 2010 ). 1 Although D.F. is unable to identify the shapes that she can see emerging in stereoscopic presentations, presumably due to her damage to area LOC, she does seem to have distinct percepts of an object located in depth. It is possible that these experiences of depth might be informed by dorsal-to ventral crosstalk.
In a remarkable series of related studies, the stereoscopic perception of curvature has been investigated in nonhuman primates. Srivastava et al. ( 2009 ) reported robust selectivity for disparity-defined curved surfaces as well as slanted ones in a high proportion of AIP neurons sampled in the monkey. They noted that this representation of 3D shape features in dorsal stream neurons would provide just the kinds of object parameters needed for programming grasping movements. However, Verhoef et al. ( 2015 ) have recently provided evidence that the activity of these curvature-selective neurons in AIP is also related to the monkey’s choice behaviour in a discrimination task between disparity-defined 3-D shapes. The same group had earlier shown that the activity of neurons in part of the anterior inferior temporal cortex (ITC) correlates with trial-by-trial judgements made by monkeys during 3-D shape categorization (Verhoef et al. 2010 ), and that micro-stimulation of these neurons strongly modulates those same judgements (Verhoef et al. 2012 ). In their most recent paper on this topic, the same researchers have demonstrated that there are clear causal links underlying these phenomena, with dorsal stream activity playing a determining role in both ventral stream activity and curvature discrimination judgements (Van Dromme et al. 2016 ). They report that reversible inactivation of the caudal intraparietal area (CIP) reduced fMRI activations elicited by curved surfaces in both AIP and ITC, and also caused a deficit in discrimination. These results provide the first clear causal evidence for the flow of visual 3D information from the dorsal stream to the ventral stream, and identify CIP as a key area for depth-structure processing. The results of this processing appear to be passed on to AIP to inform motor acts, or to the ventral stream to inform perceptual decisions, as and when the current task demands it.
Section summary: what has the dorsal stream ever done for the ventral stream?
As indicated earlier, these influences of dorsally processed visual features such as width, 2D orientation and figural depth upon the operations of the ventral stream were not predicted by the two-visuals-streams model as outlined almost a quarter-century ago by Goodale and Milner ( 1992 ; Milner and Goodale 1993 ). However, judging from the evidence gathered thus far, it should be noted that the dorsal-to-ventral traffic seems to carry somewhat primitive visual information, based on simple object features rather than anything of a more configural nature. It is the reverse traffic, from ventral to dorsal stream, that seems to carry visual and semantic complexity, thereby allowing us to bring meaning to our actions. This makes good sense within the framework of the Milner/Goodale model.
Indeed, notwithstanding the risks in making inferences based on the less-than-clean lesions in patient D.F., I would suggest that the processing of visual inputs in the dorsal stream appears to be restricted to relatively simple features rather than complex configurations. Support for this conclusion comes not only from neuropsychology, but also from a study using continuous flash suppression in healthy human subjects (Almeida et al. 2010 ). To quote those authors:
Our results indicate that the dorsal stream, in isolation from the ventral stream, is agnostic as to the identity of the objects that it processes. We suggest that structures within the dorsal visual processing stream compute motor-relevant information (e.g. graspability), which influences the identification of manipulable objects, and is not either about the function of the object or function-specific.
Contrary to this conclusion, a case has been made recently that independent computation of complex shape proceeds in parallel in both visual streams (Freud et al. 2015 , 2016 ). Their argument is based on the perception of images depicting possible and impossible objects in healthy and agnosic subjects. The two patients who were tested using fMRI were impaired at distinguishing possible from impossible objects, and evinced a lower differential activation in their damaged ventral streams, yet the two classes of objects still showed differential activations in the parietal cortex. While it is not impossible that this kind of complex spatial processing occurs independently in the dorsal stream, a perhaps more plausible interpretation would be that a signal is generated in the ventral stream (the right LOC was still differentially responsive in the two patients) that then informs the dorsal stream as to the depicted object’s graspability.
Concluding thoughts
The approach I have taken to the question of cross-stream interaction is perhaps a biased and idiosyncratic one, emphasizing as it does the value of neuropsychological evidence as a starting point. Excellent reviews in which a more balanced approach has been taken are those of van Polanen and Davare ( 2015 ) and Cloutman ( 2013 ), the latter of which compares possible similarities between different sensory modalities. What I hope that the present rather selective review offers is a corrective to the surprisingly common view that the original two-visual-systems model of Milner and Goodale postulated two independent non-interactive streams of processing. This is particularly ironic given that most of Milner and Goodale’s published research with patient D.F. over the past 25 years, which has provided the backbone of the subsequent development and refinement of the model, specifically documents the results of depleted inter-stream communication . The failures of her visuomotor ability under various experimental circumstances have consistently been explained by the authors as precisely the result of a loss of inputs to the dorsal stream from the ventral stream.
A model of the two visual streams as fully encapsulated has always been explicitly recognized as untenable by the model’s proponents. Indeed at a general level, a moment’s thought will reveal that the fact of different brain modules doing different jobs and processing information in different ways could never exclude the possibility (even likelihood) that those modules are interconnected and to varying degrees interdependent. Examples disproving such a naïve supposition abound in neuroscience. For example, it has long been known that different sensory modalities interact in the brain to some degree—yet nobody would claim that they should, therefore, be regarded as somehow part of a single system.
Acknowledgements
The author is grateful to Professors M A Goodale and H C Dijkerman for their comments on an earlier draft of this article. He also acknowledges numerous fruitful discussions with the late Dr C Cavina Pratesi prior to her recent untimely death.
1 D.F.’s stereo perception is however by no means fully normal. The control subjects all showed substantial significant improvements in a condition where the slant was represented only in a strip set between areas of zero disparity (no slant), whereas DF did not. This may result from D.F.’s having lost the scene-based processing mode that seems to characterize ventral-stream processing (Goodale et al. 2004 )
- Aflalo TN, Graziano MS. Organization of the macaque extrastriate visual cortex re-examined using the principle of spatial continuity of function. J Neurophysiol. 2011; 105 :305–320. [ PMC free article ] [ PubMed ] [ Google Scholar ]
- Aglioti S, Goodale MA, DeSouza JFX. Size-contrast illusions deceive the eye but not the hand. Curr Biol. 1995; 5 :679–685. [ PubMed ] [ Google Scholar ]
- Almeida J, Mahon BZ, Caramazza A. The role of the dorsal visual processing stream in tool identification. Psychol Sci. 2010; 21 :772–778. [ PMC free article ] [ PubMed ] [ Google Scholar ]
- Almeida J, Fintzi AR, Mahon BZ. Tool manipulation knowledge is retrieved by way of the ventral visual object processing pathway. Cortex. 2013; 49 :2334–2344. [ PMC free article ] [ PubMed ] [ Google Scholar ]
- Almeida J, Mahon BZ, Zapater-Raberov V, et al. Grasping with the eyes: the role of elongation in visual recognition of manipulable objects. Cognit Affect. Behav Neurosci. 2014; 14 :319–335. [ PubMed ] [ Google Scholar ]
- Baizer JS, Desimone R, Ungerleider LG. Comparison of subcortical connections of inferior temporal and posterior parietal cortex in monkeys. Vis Neurosci. 1993; 10 :59–72. [ PubMed ] [ Google Scholar ]
- Borchers S, Himmelbach M. The recognition of everyday objects changes grasp scaling. Vision Res. 2012; 67 :8–13. [ PubMed ] [ Google Scholar ]
- Borra E, Rockland KS. Projections to early visual areas V1 and V2 in the calcarine fissure from parietal association areas in the macaque. Front Neuroanat. 2011; 5 :35. [ PMC free article ] [ PubMed ] [ Google Scholar ]
- Borra E, Belmalih A, Calzavara R, et al. Cortical connections of the macaque anterior intraparietal (AIP) area. Cereb Cortex. 2008; 18 :1094–1111. [ PubMed ] [ Google Scholar ]
- Borra E, Ichinohe N, Sato T, et al. Cortical connections to area TE in monkey: hybrid modular and distributed organization. Cereb Cortex. 2010; 20 :257–270. [ PMC free article ] [ PubMed ] [ Google Scholar ]
- Borra E, Gerbella M, Rozzi S, et al. Projections to the superior colliculus from inferior parietal, ventral premotor, and ventrolateral prefrontal areas involved in controlling goal-directed hand actions in the macaque. Cereb Cortex. 2014; 24 :1054–1065. [ PubMed ] [ Google Scholar ]
- Bracci S, Cavina-Pratesi C, Ietswaart M, et al. Closely overlapping responses to tools and hands in left lateral occipitotemporal cortex. J Neurophysiol. 2012; 107 :1443–1456. [ PubMed ] [ Google Scholar ]
- Brenner E, Smeets JBJ. Size illusion influences how we lift but not how we grasp an object. Exp Brain Res. 1996; 111 :473–476. [ PubMed ] [ Google Scholar ]
- Bridge H, Thomas OM, Minini L, et al. Structural and functional changes across the visual cortex of a patient with visual form agnosia. J Neurosci. 2013; 33 :12779–12791. [ PMC free article ] [ PubMed ] [ Google Scholar ]
- Carey DP, Harvey M, Milner AD. Visuomotor sensitivity for shape and orientation in a patient with visual form agnosia. Neuropsychologia. 1996; 34 :329–338. [ PubMed ] [ Google Scholar ]
- Carey DP, Dijkerman CD, Murphy KJ, et al. Pointing to places and spaces in a patient with visual form agnosia. Neuropsychologia. 2006; 44 :1584–1594. [ PubMed ] [ Google Scholar ]
- Carey DP, Dijkerman HC, Milner AD. Pointing to two imaginary targets at the same time: bimanual allocentric and egocentric localization in visual form agnosic D.F. Neuropsychologia. 2009; 47 :1469–1475. [ PubMed ] [ Google Scholar ]
- Cavina-Pratesi C, Kentridge RW, Heywood CA, Milner AD. Separate channels for processing form, texture, and color: evidence from FMRI adaptation and visual object agnosia. Cereb Cortex. 2010; 20 :2319–2332. [ PubMed ] [ Google Scholar ]
- Cavina-Pratesi C, Kentridge RW, Heywood CA, Milner AD. Separate processing of texture and form in the ventral stream: evidence from FMRI and visual agnosia. Cereb Cortex. 2010; 20 :433–446. [ PubMed ] [ Google Scholar ]
- Chao LL, Martin A. Representation of manipulable man-made objects in the dorsal stream. Neuroimage. 2000; 12 :478–484. [ PubMed ] [ Google Scholar ]
- Chao LL, Haxby JV, Martin A. Attribute-based neural substrates in temporal cortex for perceiving and knowing about objects. Nat Neurosci. 1999; 2 :913–919. [ PubMed ] [ Google Scholar ]
- Cloutman LL. Interaction between dorsal and ventral processing streams: where, when and how? Brain Lang. 2013; 127 :251–263. [ PubMed ] [ Google Scholar ]
- Cohen NR, Cross ES, Tunik E, et al. Ventral and dorsal stream contributions to the online control of immediate and delayed grasping: a TMS approach. Neuropsychologia. 2009; 47 :1553–1562. [ PubMed ] [ Google Scholar ]
- Cornelsen S, Rennig J, Himmelbach M. Memory-guided reaching in a patient with visual hemiagnosia. Cortex. 2016; 79 :32–41. [ PubMed ] [ Google Scholar ]
- Creem SH, Proffitt DR. Grasping objects by their handles: a necessary interaction between cognition and action. J Exp Psychol. 2001; 27 :218228. [ PubMed ] [ Google Scholar ]
- Creutzfeldt O. Diversification and synthesis of sensory systems across the cortical link. In: Pompeiano O, Ajmone-Marsan C, editors. Brain mechanisms of perceptual awareness and purposeful behaviour. New York: Raven Press; 1981. pp. 153–165. [ Google Scholar ]
- Creutzfeldt O. Multiple visual areas: multiple sensori-motor links. In: Rose D, Dobson VG, editors. Models of the visual cortex. New York: Wiley; 1985. pp. 54–61. [ Google Scholar ]
- Davare M, Andres M, Clerget E, et al. Temporal dissociation between hand shaping and gripforce scaling in the anterior intraparietal area. J Neurosci. 2007; 27 :3974–3980. [ PMC free article ] [ PubMed ] [ Google Scholar ]
- Dijkerman HC, Milner AD. Copying without perceiving: Motor imagery in visual form agnosia. NeuroReport. 1997; 8 :729–732. [ PubMed ] [ Google Scholar ]
- Dijkerman HC, Milner AD, Carey DP. The perception and prehension of objects oriented in the depth plane: I. Effects of visual form agnosia. Exp Brain Res. 1996; 112 :442–451. [ PubMed ] [ Google Scholar ]
- Dijkerman HC, Milner AD, Carey DP. Grasping spatial relationships: failure to demonstrate allocentric visual coding in a patient with visual form agnosia. Consc Cognit. 1998; 7 :424–437. [ PubMed ] [ Google Scholar ]
- Dijkerman HC, Lê S, Démonet J-F, Milner AD. Visuomotor performance in a patient with visual agnosia due to an early lesion. Cogn Brain Res. 2004; 20 :12–25. [ PubMed ] [ Google Scholar ]
- Distler C, Boussaoud D, Desimone R, Ungerleider LG. Cortical connections of inferior temporal area TEO in macaque monkeys. J Comp Neurol. 1993; 334 :125–150. [ PubMed ] [ Google Scholar ]
- Efron R. What is perception? Boston Stud Philos Sci. 1969; 4 :137–173. [ Google Scholar ]
- Fang F, He S. Cortical responses to invisible objects in the human dorsal and ventral pathways. Nat Neurosci. 2005; 8 :1380–1385. [ PubMed ] [ Google Scholar ]
- Felleman DJ, Van Essen DC. Distributed hierarchical processing in the primate cerebral cortex. Cereb Cortex. 1991; 1 :1–47. [ PubMed ] [ Google Scholar ]
- Flanagan JR, Beltzner MA. Independence of perceptual and sensorimotor predictions in the size-weight illusion. Nat Neurosci. 2000; 3 :737–741. [ PubMed ] [ Google Scholar ]
- Foley RT, Whitwell RL, Goodale MA. The two-visual-systems hypothesis and the perspectival features of visual experience. Consc Cognit. 2015; 35 :225–233. [ PubMed ] [ Google Scholar ]
- Freud E, Ganel T, Shelef I, et al. Three-dimensional representations of objects in dorsal cortex are dissociable from those in ventral cortex. Cereb Cortex. 2015 [ PubMed ] [ Google Scholar ]
- Freud E, Plaut DC, Behrmann M. ‘What’ is happening in the dorsal visual pathway. Trends Cogn Sci. 2016; 20 :773–784. [ PubMed ] [ Google Scholar ]
- Gallivan JP, Chapman CS, McLean DA, et al. Activity patterns in the category-selective occipitotemporal cortex predict upcoming motor actions. Eur J Neurosci. 2013; 38 :2408–2424. [ PubMed ] [ Google Scholar ]
- Gallivan JP, Cant JS, Goodale MA, Flanagan JR. Representation of object weight in human ventral visual cortex. Curr Biol. 2014; 24 :1866–1873. [ PubMed ] [ Google Scholar ]
- Ganel T, Tanzer M, Goodale MA. A double dissociation between action and perception in the context of visual illusions: opposite effects of real and illusory size. Psychol Sci. 2008; 19 :221–225. [ PubMed ] [ Google Scholar ]
- Glickstein M, May JG, Mercier BE. Corticopontine projection in the macaque: the distribution of labelled cortical cells after large injections of horseradish peroxidase in the pontine nuclei. J Comp Neurol. 1985; 235 :343–359. [ PubMed ] [ Google Scholar ]
- Goodale MA. How (and why) the visual control of action differs from visual perception. Proc R Soc Lond B. 2014; 281 :20140337. [ PMC free article ] [ PubMed ] [ Google Scholar ]
- Goodale MA, Milner AD. Separate visual pathways for perception and action. Trends Neurosci. 1992; 15 :20–25. [ PubMed ] [ Google Scholar ]
- Goodale MA, Milner AD. Sight unseen: an exploration of conscious and unconscious vision. Oxford: Oxford University Press; 2004. [ Google Scholar ]
- Goodale MA, Milner AD, Jakobson LS, Carey DP. A neurological dissociation between perceiving objects and grasping them. Nature. 1991; 349 :154–156. [ PubMed ] [ Google Scholar ]
- Goodale MA, Meenan JP, Bülthoff HH, et al. Separate neural pathways for the visual analysis of object shape in perception and prehension. Curr Biol. 1994; 4 :604–610. [ PubMed ] [ Google Scholar ]
- Goodale MA, Jakobson LS, Milner AD, et al. The nature and limits of orientation and pattern processing supporting visuomotor control in a visual form agnosic. J Cogn Neurosci. 1994; 6 :46–56. [ PubMed ] [ Google Scholar ]
- Goodale MA, Jakobson LS, Keillor JM. Differences in the visual control of pantomimed and natural grasping movements. Neuropsychologia. 1994; 32 :1159–1178. [ PubMed ] [ Google Scholar ]
- Goodale MA, Westwood DA, Milner AD. Two distinct modes of control for object-directed action. Prog Brain Res. 2004; 144 :131–144. [ PubMed ] [ Google Scholar ]
- Hutchison RM, Culham JC, Everling S, et al. Distinct and distributed functional connectivity patterns across cortex reflect the domain-specific constraints of object, face, scene, body, and tool category-selective modules in the ventral visual pathway. Neuroimage. 2014; 96 :216236. [ PubMed ] [ Google Scholar ]
- Iwai E, Yukie M. Amygdalofugal and amygdalopetal connections with modality- specific visual cortical areas in the macaques (Macaca fuscata, M. mulatta, M. fascicularis) J Comp Neurol. 1987; 261 :362–387. [ PubMed ] [ Google Scholar ]
- Jackson SR, Shaw A. The Ponzo illusion affects grip-force but not grip-aperture scaling during prehension movements. J Exp Psycholv. 2000; 26 :418–423. [ PubMed ] [ Google Scholar ]
- Jakobson LS, Goodale MA. Factors affecting higher-order movement planning: a kinematic analysis of human prehension. Exp Brain Res. 1991; 86 :199–208. [ PubMed ] [ Google Scholar ]
- James TW, Culham J, Humphrey GK, et al. Ventral occipital lesions impair object recognition but not object-directed grasping: a fMRI study. Brain. 2003; 248 :2463–2475. [ PubMed ] [ Google Scholar ]
- Jeannerod M. The formation of finger grip during prehension: a cortically mediated visuomotor pattern. Behav Brain Res. 1986; 19 :99–116. [ PubMed ] [ Google Scholar ]
- Jeannerod M, Rossetti Y. Visuomotor coordination as a dissociable visual function: experimental and clinical evidence. In: Kennard C, editor. Visual perceptual defects. Baillière’s Clinical Neurology, vol. 2. no. (2). London: Baillière Tindall; 1993. pp. 439–460. [ PubMed ] [ Google Scholar ]
- Johansson RS, Cole KJ. Sensory-motor coordination during grasping and manipulative actions. Curr Opin Neurobiol. 1992; 2 :815–823. [ PubMed ] [ Google Scholar ]
- Knill DC, Saunders JA. Do humans optimally integrate stereo and texture information for judgments of surface slant? Vision Res. 2003; 43 :2539–2558. [ PubMed ] [ Google Scholar ]
- Lê S, Cardebat D, Boulanouar K, et al. Seeing, since childhood, without ventral stream: a behavioural study. Brain. 2002; 004 :58–74. [ PubMed ] [ Google Scholar ]
- Lewis JW. Cortical networks related to human use of tools. Neuroscientist. 2006; 12 :211–231. [ PubMed ] [ Google Scholar ]
- Mahon BZ, Milleville SC, Negri GA, et al. Action-related properties shape object representations in the ventral stream. Neuron. 2007; 55 :507–520. [ PMC free article ] [ PubMed ] [ Google Scholar ]
- Marotta JJ, Behrmann M, Goodale MA. The removal of binocular cues disrupts the calibration of grasping in patients with visual form agnosia. Exp Brain Res. 1997; 116 :113–121. [ PubMed ] [ Google Scholar ]
- McIntosh RD. Seeing size and weight. Trends Cogn Sci. 2000; 4 :442–444. [ PubMed ] [ Google Scholar ]
- McIntosh RD, Lashley G. Matching boxes: familiar size influences action programming. Neuropsychologia. 2008; 46 :2441–2444. [ PubMed ] [ Google Scholar ]
- McIntosh RD, Dijkerman HC, Mon-Williams M, Milner AD. Grasping what is graspable: evidence from visual form agnosia. Cortex. 2004; 40 :695–702. [ PubMed ] [ Google Scholar ]
- Milner AD, Goodale MA. Visual pathways to perception and action. In: Hicks TP, Molotchnikoff S, Ono T, editors. Progress in brain research, vol. 95. The visually responsive neuron: from basic neurophysiology to behaviour. Amsterdam: Elsevier; 1993. pp. 317–337. [ PubMed ] [ Google Scholar ]
- Milner AD, Goodale MA. The visual brain in action. 2 edn. Oxford: Oxford University Press; 1995. [ Google Scholar ]
- Milner AD, Goodale MA. The visual brain in action. Oxford: Oxford University Press; 2006. [ Google Scholar ]
- Milner AD, Goodale MA. Two visual systems re-viewed. Neuropsychologia. 2008; 46 :774–785. [ PubMed ] [ Google Scholar ]
- Milner AD, Perrett DI, Johnston RS, et al. Perception and action in ‘visual form agnosia’ Brain. 1991; 114 :405–428. [ PubMed ] [ Google Scholar ]
- Milner AD, Dijkerman HC, Carey DP. Visuospatial processing in a pure case of visual form agnosia. In: Burgess N, Jeffery KJ, O’Keefe J, editors. The hippocampal and parietal foundations of spatial cognition. Oxford: Oxford University Press; 1999. pp. 443–466. [ Google Scholar ]
- Mon-Williams M, Tresilian JR, McIntosh RD, Milner AD. Monocular and binocular distance cues: insights from visual form agnosia I (of III) Exp Brain Res. 2001; 139 :127–136. [ PubMed ] [ Google Scholar ]
- Murphy KJ, Racicot CI, Goodale MA. The use of visuomotor cues as a strategy for making perceptual judgments in a patient with visual form agnosia. Neuropsychology. 1996; 10 :396–401. [ Google Scholar ]
- Murphy KJ, Carey DP, Goodale MA. The perception of spatial relations in a patient with visual form agnosia. Cogn Neuropsychol. 1998; 15 :705–722. [ PubMed ] [ Google Scholar ]
- Ramayya AG, Glasser MF, Rilling JK. A DTI investigation of neural substrates supporting tool use. Cereb Cortex. 2010; 20 :507–516. [ PubMed ] [ Google Scholar ]
- Read JC, Phillipson GP, Serrano-Pedraza I, et al. Stereoscopic vision in the absence of the lateral occipital cortex. PLoS ONE. 2010; 5 :e12608. [ PMC free article ] [ PubMed ] [ Google Scholar ]
- Rizzolatti G, Matelli M. Two different streams form the dorsal visual system: anatomy and function. Exp Brain Res. 2003; 153 :146–157. [ PubMed ] [ Google Scholar ]
- Rockland KS, Van Hoesen GW. Direct temporal-occipital feedback connections to striate cortex (V1) in the macaque monkey. Cereb Cortex. 1994; 4 :300–313. [ PubMed ] [ Google Scholar ]
- Rossit S, Szymanek L, Butler SH, Harvey M. Memory-guided saccade processing in visual form agnosia (patient DF) Exp Brain Res. 2010; 200 :109–116. [ PubMed ] [ Google Scholar ]
- Sakata H, Taira M, Murata A, Mine S. Neural mechanisms of visual guidance of hand action in the parietal cortex of the monkey. Cereb Cortex. 1995; 5 :429–438. [ PubMed ] [ Google Scholar ]
- Schenk T, Milner AD. Concurrent visuomotor behaviour improves form discrimination in a patient with visual form agnosia. Eur J Neurosci. 2006; 24 :1495–1503. [ PubMed ] [ Google Scholar ]
- Shikata E, Tanaka Y, Nakamura H, et al. Selectivity of the parietal visual neurones in 3D orientation of surface of stereoscopic stimuli. NeuroReport. 1996; 7 :2389–2394. [ PubMed ] [ Google Scholar ]
- Singhal A, Monaco S, Kaufman LD, Culham JC. Human fMRI reveals that delayed action re-recruits visual perception. PLoS ONE. 2013; 8 :e73629. [ PMC free article ] [ PubMed ] [ Google Scholar ]
- Srivastava S, Orban GA, De Mazière PA, Janssen P. A distinct representation of three-dimensional shape in macaque anterior intraparietal area: fast, metric, and coarse. J Neurosci. 2009; 29 :10613–10626. [ PMC free article ] [ PubMed ] [ Google Scholar ]
- Van Polanen V, Davare M. Interactions between dorsal and ventral streams for controlling skilled grasp. Neuropsychologia. 2015; 79 :186–191. [ PMC free article ] [ PubMed ] [ Google Scholar ]
- Van Dromme IC, Premereur E, Verhoef BE, et al. Posterior parietal cortex drives inferotemporal activations during three-dimensional object vision. PLoS Biol. 2016; 14 :e1002445. [ PMC free article ] [ PubMed ] [ Google Scholar ]
- Verhagen L, Dijkerman HC, Grol MJ, Toni I. Perceptuo-motor interactions during prehension movements. J Neurosci. 2008; 28 :4726–4735. [ PMC free article ] [ PubMed ] [ Google Scholar ]
- Verhoef BE, Vogels R, Janssen P. Contribution of inferior temporal and posterior parietal activity to three-dimensional shape perception. Curr Biol. 2010; 20 :909–913. [ PubMed ] [ Google Scholar ]
- Verhoef BE, Vogels R, Janssen P. Inferotemporal cortex subserves three-dimensional structure categorization. Neuron. 2012; 73 :171–182. [ PubMed ] [ Google Scholar ]
- Verhoef BE, Michelet P, Vogels R, Janssen P. Choice-related activity in the anterior intraparietal area during 3-D structure categorization. J Cogn Neurosci. 2015; 27 :1104–1115. [ PubMed ] [ Google Scholar ]
- Webster MJ, Bachevalier J, Ungerleider LG. Connections of inferior temporal areas TEO and TE with parietal and frontal cortex in macaque monkeys. Cereb Cortex. 1994; 4 :470–483. [ PubMed ] [ Google Scholar ]
- Young MP. Objective analysis of the topological organization of the primate cortical visual system. Nature. 1992; 358 :152–155. [ PubMed ] [ Google Scholar ]
- Zhong YM, Rockland KS. Inferior parietal lobule projections to anterior inferotemporal cortex (area TE) in macaque monkey. Cereb Cortex. 2003; 13 :527–540. [ PubMed ] [ Google Scholar ]
Academia.edu no longer supports Internet Explorer.
To browse Academia.edu and the wider internet faster and more securely, please take a few seconds to upgrade your browser .
Enter the email address you signed up with and we'll email you a reset link.
- We're Hiring!
- Help Center
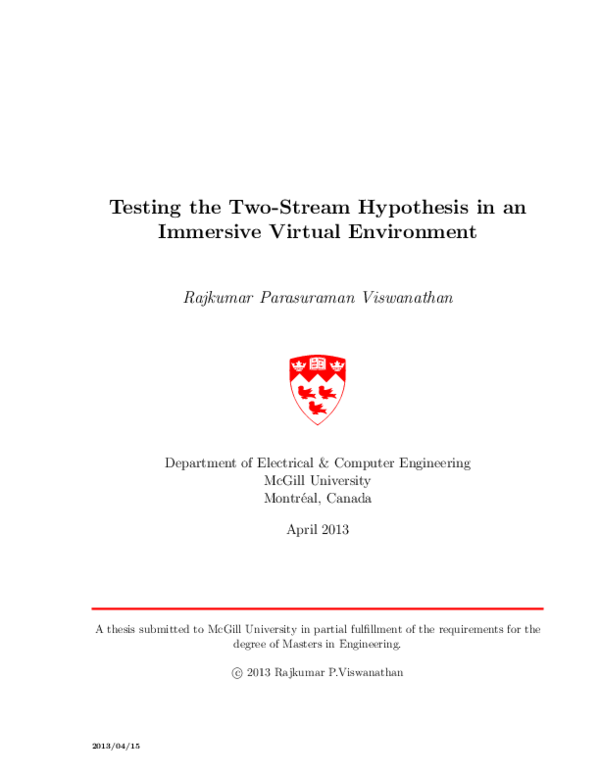
Testing the Two-Stream Hypothesis in an Immersive Virtual Environment

A great deal of behavioural research has gone into a proposed distinction between two separate streams for visual processing, vision for action and vision for perception. Research on perceptual and geometric illusions has gone a long way in determining this proposed dissociation in visual processing. These illusions fool the brain into misjudging object sizes but at the same time do not a ect ngers from scaling to the correct size while grabbing. This e ect is maintained even when the stimuli are three-dimensional. The mechanisms mediating the visual control of object-oriented actions are thought to operate in egocentric coordinates. We would therefore like to know whether this e ect is maintained when reaching for the illusion with a virtual arm, where there is an indirect pairing of visual and proprioceptive feedback, a process essential for pairing the external visual scene onto egocentric coordinates. Our research shows that while the two stream e ect is maintained in a real world, it is lost out in a virtual world where there is a lack of haptic feedback. It is also seen that participants underestimate depth unless given an external feedback, in our case, a change of colour in the virtual arm within a virtual environment with a depth grid.
Related Papers
Psychonomic Bulletin & Review
Oliver Herbort
Previous research has revealed changes in the perception of objects due to changes of object-oriented actions. In present study, we varied the arm and finger postures in the context of a virtual reaching and grasping task and tested whether this manipulation can simultaneously affect the perceived size and distance of external objects. Participants manually controlled visual cursors, aiming at reaching and enclosing a distant target object, and judged the size and distance of this object. We observed that a visual–proprioceptive discrepancy introduced during the reaching part of the action simultaneously affected the judgments of target distance and of target size (Experiment 1). A related variation applied to the grasping part of the action affected the judgments of size, but not of distance of the target (Experiment 2). These results indicate that perceptual effects observed in the context of actions can directly arise through sensory integration of multimodal redundant signals an...
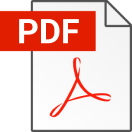
Jacqueline Fulvio
3D motion perception is of central importance to daily life. However, when tested in laboratory settings, sensitivity to 3D motion signals is found to be poor, leading to the view that heuristics and prior assumptions are critical for 3D motion perception. Here we explore an alternative: sensitivity to 3D motion signals is context-dependent and must be learned based on explicit visual feedback in novel environments. The need for action-contingent visual feedback is well-established in the developmental literature. For example, young kittens that are passively moved through an environment, but unable to move through it themselves, fail to develop accurate depth perception. We find that these principles also obtain in adult human perception. Observers that do not experience visual consequences of their actions fail to develop accurate 3D motion perception in a virtual reality environment, even after prolonged exposure. By contrast, observers that experience the consequences of their actions improve performance based on available sensory cues to 3D motion. Specifically, we find that observers learn to exploit the small motion parallax cues provided by head jitter. Our findings advance understanding of human 3D motion processing and form a foundation for future study of perception in virtual and natural 3D environments. The perception of 3D motion is fundamental to our interactions with the environment, but we have poor insight into the sensory cues that support its accuracy. Previous studies have reported poor sensitivity to 3D motion cues 1 , and some work has reported that observers will discount 3D motion cues altogether 2. Poor sensory sensitivity will inevitably lead to inconsistent behavioral performance, but may also lead to more systematic perceptual errors. Such errors have been reported in the judgment of 3D motion. Observers will judge that approaching objects will miss the head, even when they are on a collision course 3,4. Observers will also judge approaching objects to be receding and vice versa 5. This has led to the view that 3D motion perception relies in large part on heuristics and prior assumptions 6. Here we consider another possibility: that the sensitivity to 3D motion cues is context-dependent and needs to be learned for a given visual environment based on explicit visual feedback. Virtual reality (VR) provides the ideal tool to investigate sensitivity to 3D motion because it allows us to present sensory signals that closely approximate those in the real world, while maintaining tight experimental control. We manipulated the sensory cues thought to contribute to perception in VR environments and tested the role of experience and feedback on performance. We first explored the extent to which the virtual environment supported accurate sensory processing of 3D motion information " out of the box ". We asked observers to intercept targets that moved in a 3D environment, and found performance to be generally poor. One of the compelling features of VR-based viewing is that it can provide motion parallax cues, i.e., head-motion contingent updating of the visual display. Such cues are not available in most traditional visual experiments. We found that the addition of motion parallax cues produced by small naturally-occurring random head-motion (head jitter) did not improve performance. Observers were insensitive to the additional cues even after prolonged exposure to the stimuli. This result is consistent with the notion that head jitter-based cues are too small, or too noisy to have a meaningful impact. Second, we tested the hypothesis that observers require explicit visual feedback when immersed in a new (virtual) environment. Consistent with feedback-driven sensory recalibration 7–11 , rapid and significant improvements in performance were observed when visual feedback was provided following the observer's actions. In particular, the cues to motion and depth provided by head jitter that had no effect in the first part of the study became an important source of sensory information. In summary, we identified the sensory cues that contribute to 3D motion perception and the conditions under which they are employed. Our results advance understanding of human visual processing in 3D environments.
Psychological Research
Bernhard Riecke
Chiara Bozzacchi
Maria V. Sanchez-Vives
Frontiers in Psychology
Natale Stucchi
Behavioral and Brain Sciences
Simon Rushton
Consciousness and Cognition
Elisabeth Stoettinger
Proceedings of the European Conference on Cognitive Ergonomics
Glyn Lawson
Elisabeth Stöttinger
Loading Preview
Sorry, preview is currently unavailable. You can download the paper by clicking the button above.
RELATED PAPERS
Charles Lenay
Arquivos Brasileiros De Oftalmologia
Paul B Hibbard
ACM Transactions on …
Neuropsychologia
Edouard Gentaz
Frontiers in Bioengineering and Biotechnology
Experimental Brain Research
Proceedings of the SPIE
Takahiro Higuchi
Cognitive Neurodynamics
2009 Computation World: …
Chellali Ryad
Arthur Tang
Experimental Brain …
Jeroen Smeets
Heinrich Bülthoff
ACM Transactions on Applied Perception
John Rieser , Gayathri Narasimham
Fred W Mast
Artificial life and virtual reality
Vince Polito
Perception & Psychophysics
Umberto Castiello
Marcos Hilsenrat , Miriam Reiner
Frontiers in Human …
- We're Hiring!
- Help Center
- Find new research papers in:
- Health Sciences
- Earth Sciences
- Cognitive Science
- Mathematics
- Computer Science
- Academia ©2024

An official website of the United States government
The .gov means it’s official. Federal government websites often end in .gov or .mil. Before sharing sensitive information, make sure you’re on a federal government site.
The site is secure. The https:// ensures that you are connecting to the official website and that any information you provide is encrypted and transmitted securely.
- Publications
- Account settings
- My Bibliography
- Collections
- Citation manager
Save citation to file
Email citation, add to collections.
- Create a new collection
- Add to an existing collection
Add to My Bibliography
Your saved search, create a file for external citation management software, your rss feed.
- Search in PubMed
- Search in NLM Catalog
- Add to Search
Two stream hypothesis of visual processing for navigation in mouse
Affiliation.
- 1 UCL Institute of Behavioural Neurosciences, Department of Experimental Psychology, University College London, London, WC1H 0AP, UK. Electronic address: [email protected].
- PMID: 32294570
- DOI: 10.1016/j.conb.2020.03.009
Vision research has traditionally been studied in stationary subjects observing stimuli, and rarely during navigation. Recent research using virtual reality environments for mice has revealed that responses even in the primary visual cortex are modulated by spatial context - identical scenes presented in different positions of a room can elicit different responses. Here, we review these results and discuss how information from visual areas can reach navigational areas of the brain. Based on the observation that mouse higher visual areas cover different parts of the visual field, we propose that spatial signals are processed along two-streams based on visual field coverage. Specifically, this hypothesis suggests that landmark related signals are processed by areas biased to the central field, and self-motion related signals are processed by areas biased to the peripheral field.
Copyright © 2020 Elsevier Ltd. All rights reserved.
PubMed Disclaimer
Similar articles
- Stereosonic vision: Exploring visual-to-auditory sensory substitution mappings in an immersive virtual reality navigation paradigm. Massiceti D, Hicks SL, van Rheede JJ. Massiceti D, et al. PLoS One. 2018 Jul 5;13(7):e0199389. doi: 10.1371/journal.pone.0199389. eCollection 2018. PLoS One. 2018. PMID: 29975734 Free PMC article.
- Coherent encoding of subjective spatial position in visual cortex and hippocampus. Saleem AB, Diamanti EM, Fournier J, Harris KD, Carandini M. Saleem AB, et al. Nature. 2018 Oct;562(7725):124-127. doi: 10.1038/s41586-018-0516-1. Epub 2018 Sep 10. Nature. 2018. PMID: 30202092 Free PMC article.
- Disparity Sensitivity and Binocular Integration in Mouse Visual Cortex Areas. La Chioma A, Bonhoeffer T, Hübener M. La Chioma A, et al. J Neurosci. 2020 Nov 11;40(46):8883-8899. doi: 10.1523/JNEUROSCI.1060-20.2020. Epub 2020 Oct 13. J Neurosci. 2020. PMID: 33051348 Free PMC article.
- Spatial navigation signals in rodent visual cortex. Flossmann T, Rochefort NL. Flossmann T, et al. Curr Opin Neurobiol. 2021 Apr;67:163-173. doi: 10.1016/j.conb.2020.11.004. Epub 2020 Dec 25. Curr Opin Neurobiol. 2021. PMID: 33360769 Review.
- The contribution of virtual reality to the diagnosis of spatial navigation disorders and to the study of the role of navigational aids: A systematic literature review. Cogné M, Taillade M, N'Kaoua B, Tarruella A, Klinger E, Larrue F, Sauzéon H, Joseph PA, Sorita E. Cogné M, et al. Ann Phys Rehabil Med. 2017 Jun;60(3):164-176. doi: 10.1016/j.rehab.2015.12.004. Epub 2016 Mar 24. Ann Phys Rehabil Med. 2017. PMID: 27017533 Review.
- Multimodal Deep Learning Model Unveils Behavioral Dynamics of V1 Activity in Freely Moving Mice. Xu A, Hou Y, Niell CM, Beyeler M. Xu A, et al. Adv Neural Inf Process Syst. 2023 Dec;36:15341-15357. Adv Neural Inf Process Syst. 2023. PMID: 39005944 Free PMC article.
- The meso-connectomes of mouse, marmoset, and macaque: network organization and the emergence of higher cognition. Magrou L, Joyce MKP, Froudist-Walsh S, Datta D, Wang XJ, Martinez-Trujillo J, Arnsten AFT. Magrou L, et al. Cereb Cortex. 2024 May 2;34(5):bhae174. doi: 10.1093/cercor/bhae174. Cereb Cortex. 2024. PMID: 38771244 Free PMC article. Review.
- Modular horizontal network within mouse primary visual cortex. Burkhalter A, Ji W, Meier AM, D'Souza RD. Burkhalter A, et al. Front Neuroanat. 2024 Apr 8;18:1364675. doi: 10.3389/fnana.2024.1364675. eCollection 2024. Front Neuroanat. 2024. PMID: 38650594 Free PMC article.
- Interactions between rodent visual and spatial systems during navigation. Saleem AB, Busse L. Saleem AB, et al. Nat Rev Neurosci. 2023 Aug;24(8):487-501. doi: 10.1038/s41583-023-00716-7. Epub 2023 Jun 28. Nat Rev Neurosci. 2023. PMID: 37380885 Review.
- Walking humans and running mice: perception and neural encoding of optic flow during self-motion. Horrocks EAB, Mareschal I, Saleem AB. Horrocks EAB, et al. Philos Trans R Soc Lond B Biol Sci. 2023 Jan 30;378(1869):20210450. doi: 10.1098/rstb.2021.0450. Epub 2022 Dec 13. Philos Trans R Soc Lond B Biol Sci. 2023. PMID: 36511417 Free PMC article. Review.
Publication types
- Search in MeSH
Grants and funding
- 200501/Z/16/Z/WT_/Wellcome Trust/United Kingdom
- R004765/BB_/Biotechnology and Biological Sciences Research Council/United Kingdom
LinkOut - more resources
Full text sources.
- Elsevier Science
- Citation Manager
NCBI Literature Resources
MeSH PMC Bookshelf Disclaimer
The PubMed wordmark and PubMed logo are registered trademarks of the U.S. Department of Health and Human Services (HHS). Unauthorized use of these marks is strictly prohibited.
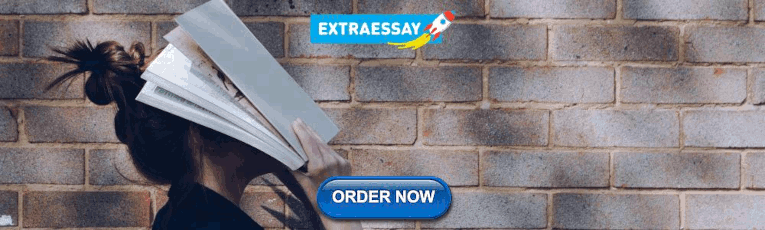
IMAGES
VIDEO
COMMENTS
The two-streams hypothesis is a model of the neural processing of vision as well as hearing. [1] The hypothesis, given its initial characterisation in a paper by David Milner and Melvyn A. Goodale in 1992, argues that humans possess two distinct visual systems. [2] Recently there seems to be evidence of two distinct auditory systems as well. As visual information exits the occipital lobe, and ...
The two-streams hypothesis is a widely accepted and influential model of the neural processing of vision as well as hearing. The hypothesis, given its most popular characterisation in a paper by David Milner and Melvyn A. Goodale in 1992, argues that humans possess two distinct visual systems. However, recently there seems to be evidence of two ...
The dorsal stream (green) and ventral stream (purple) are shown. They originate from a common source in the visual cortex. The two-streams hypothesis is a widely accepted and influential model of the neural processing of vision. The hypothesis, given its most popular characterisation in a paper by David Milner and Melvyn A. Goodale in 1992, argues that humans possess two distinct visual systems.
The two visual streams hypothesis is a robust example of neural functional specialization that has inspired countless studies over the past four decades. According to one prominent version of the theory, the fundamental goal of the dorsal visual pathway is the transformation of retinal information for visually-guided motor behavior. To that end, the dorsal stream processes input using absolute ...
A computational examination of the two-streams hypothesis: which pathway needs a longer memory? Abolfazl Alipour, 1, 2 John M. Beggs, 2, 3 Joshua W. Brown, 1, 2 and Thomas W. James 1, 2 ... To that end, the dorsal stream processes input using absolute (or veridical) metrics only when the movement is initiated, necessitating very little, or no ...
The two visual streams hypothesis is a robust framework that has inspired many studies in the past three decades. One of the well-studied claims of this hypothesis is the idea that the dorsal visual pathway is involved in visually guided motor behavior, and it is operating with a short memory. Conversely, this hypothesis claims that the ventral visual pathway is involved in object ...
98 part of the spectrum is the influential two visual streams hypothesis/theory 99 (TVSH). According to this hypothesis, visual information processing splits into 100 two cortical streams after its initial processing in early visual areas. In colloquial 101 terms, the function of the ventral visual stream is to shape our visual perception
The two visual streams hypothesis is a robust example of neural functional specialization that has inspired countless studies over the past four decades. According to one prominent version of the theory, the fundamental goal of the dorsal visual pathway is the transformation of retinal information for visually-guided motor behavior. To that end, the dorsal stream processes input using absolute ...
Based on the hypothesis of two-streams of visual processing for navigation, we can make a couple of predictions on their function. The first prediction is based on the fact that spatial representations in the place cells and head-direction cells are known to be strongly controlled by visual cues (or landmarks) [2-6].
The two visual streams hypothesis is a robust framework that has inspired many studies in the past three decades. One of the well-studied claims of this hypothesis is the idea that the dorsal ...
More than 30 cortical areas have visual functions and are organized hierarchically into complex feedforward and feedback connections 1,2. The dual-stream hypothesis models how visual information ...
38 hypothesis was proposed in a seminal paper by Goodale and Milner in 1992 39 (Melvyn A. Goodale & Milner, 1992). According to their two-streams hypothesis, 40 the ventral visual stream (from occipital to temporal cortex) is heavily involved 41 in object recognition while the dorsal visual stream (occipital to parietal) is
The results further suggest that orientation/size determination (a putative dorsal stream function) does not benefit from longer memory. These findings are consistent with the two visual streams theory of functional specialization. Keywords Two-streams hypothesis Dorsal and ventral visual pathway LSTM Convolutional neural networks
The two-stream network architecture [2] is motivated by the two-stream hypothesis for the human visual cortex in biology [4], which states that the brain has separate pathways for recognizing objects and motion. Attempting to mimic this this structure, the two-stream network architecture for video understanding utilizes two separate network ...
CHAPTER 1: TWO-STREAM HYPOTHESIS. When information from the external world reaches the visual cortex in the form of nerve impulses, it follows two streams for visual perception. This is commonly known as the two-stream hypothesis, which is widely accepted. This theory, proposed by Ungerleider and Mishkin (1982) posits a " where"
According to the two-streams hypothesis, the static visual system is traditionally segregated into two main processing streams, one the dorsal 'where' pathway which is responsible for processing ...
This new data provides new challenges to existing theories of visual processing and an opportunity to revisit them from a fresh perspective of navigation. We propose a new two-stream hypothesis — that visual information for navigation is processed along two streams that are based on visual field coverage.
The Two-Streams hypothesis is a widely accepted account of visual processing. As visual information exits the occipital lobe, it follows two main channels, or "streams."The ventral stream (also known as the "what pathway") travels to the temporal lobe and is involved with object identification. The dorsal stream (or, "where pathway") terminates in the parietal lobe and process spatial locations.
The current consensus divides primate cortical visual processing into two broad networks or "streams" composed of highly interconnected areas (Milner and Goodale 2006, 2008; Goodale 2014).The ventral stream, passing from primary visual cortex (V1) through to inferior parts of the temporal lobe, is considered to mediate the transformation of the contents of the visual signal into the mental ...
The idea that two pathways process visual information was first defined by Ungerleider and Mishkin [1] and is called the "two-stream hypothesis". However, this idea has been heavily contested by 2013/04/15 1 Introduction 2 the one-stream hypothesis where only one pathway processes visual information i.e., both perception and action.
Based on the observation that mouse higher visual areas cover different parts of the visual field, we propose that spatial signals are processed along two-streams based on visual field coverage. Specifically, this hypothesis suggests that landmark related signals are processed by areas biased to the central field, and self-motion related ...
Abstract. The two visual streams hypothesis is a robust example of neural functional specialization that has inspired countless studies over the past four decades. According to one prominent version of the theory, the fundamental goal of the dorsal visual pathway is the transformation of retinal information for visually-guided motor behavior.
Based on the observation that mouse higher visual areas cover different parts of the visual field, we propose that spatial signals are processed along two-streams based on visual field coverage. Specifically, this hypothesis suggests that landmark related signals are processed by areas biased to the central field, and self-motion related ...
The current consensus divides primate cortical visual processing into two broad networks or "streams" composed of highly interconnected areas (Milner and Goodale 2006, 2008; Goodale 2014). The ventral stream, passing from primary visual cortex (V1) through to inferior parts of the temporal lobe, is considered to mediate the transformation of the contents of the visual signal into the ...
dorsal stream function and dysfunction, inspired by the two-visual systems theory, is now extending beyond the limits of the model. Two Visual Pathways in the Cerebral Cortex Work in the 1960s by Trevarthen, Ingle, Schneider, and others anticipated the heavily cited two visual systems hypothesis of Ungerleider and Mishkin (1982) and its
This is inspired by two-stream hypothesis -- the human visual cortex contains two pathways: the ventral stream (which performs object recognition in the scene) and the dorsal stream (which recognizes motion). ... [2]. In addition to a spatial stream, which operates on original video frames, YOLO-based Adaptive Window Two-stream Convolutional ...