- Resources Home 🏠
- Try SciSpace Copilot
- Search research papers
- Add Copilot Extension
- Try AI Detector
- Try Paraphraser
- Try Citation Generator
- April Papers
- June Papers
- July Papers

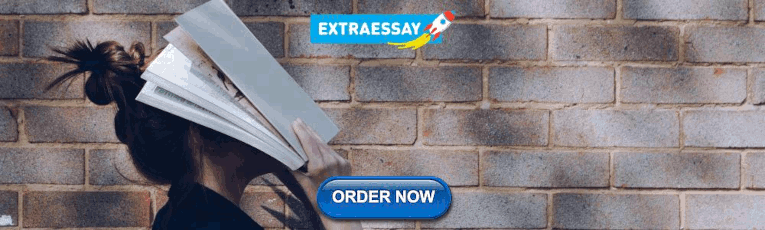
How To Write A Research Summary

It’s a common perception that writing a research summary is a quick and easy task. After all, how hard can jotting down 300 words be? But when you consider the weight those 300 words carry, writing a research summary as a part of your dissertation, essay or compelling draft for your paper instantly becomes daunting task.
A research summary requires you to synthesize a complex research paper into an informative, self-explanatory snapshot. It needs to portray what your article contains. Thus, writing it often comes at the end of the task list.
Regardless of when you’re planning to write, it is no less of a challenge, particularly if you’re doing it for the first time. This blog will take you through everything you need to know about research summary so that you have an easier time with it.
What is a Research Summary?
A research summary is the part of your research paper that describes its findings to the audience in a brief yet concise manner. A well-curated research summary represents you and your knowledge about the information written in the research paper.
While writing a quality research summary, you need to discover and identify the significant points in the research and condense it in a more straightforward form. A research summary is like a doorway that provides access to the structure of a research paper's sections.
Since the purpose of a summary is to give an overview of the topic, methodology, and conclusions employed in a paper, it requires an objective approach. No analysis or criticism.
Research summary or Abstract. What’s the Difference?
They’re both brief, concise, and give an overview of an aspect of the research paper. So, it’s easy to understand why many new researchers get the two confused. However, a research summary and abstract are two very different things with individual purpose. To start with, a research summary is written at the end while the abstract comes at the beginning of a research paper.
A research summary captures the essence of the paper at the end of your document. It focuses on your topic, methods, and findings. More like a TL;DR, if you will. An abstract, on the other hand, is a description of what your research paper is about. It tells your reader what your topic or hypothesis is, and sets a context around why you have embarked on your research.
Getting Started with a Research Summary
Before you start writing, you need to get insights into your research’s content, style, and organization. There are three fundamental areas of a research summary that you should focus on.
- While deciding the contents of your research summary, you must include a section on its importance as a whole, the techniques, and the tools that were used to formulate the conclusion. Additionally, there needs to be a short but thorough explanation of how the findings of the research paper have a significance.
- To keep the summary well-organized, try to cover the various sections of the research paper in separate paragraphs. Besides, how the idea of particular factual research came up first must be explained in a separate paragraph.
- As a general practice worldwide, research summaries are restricted to 300-400 words. However, if you have chosen a lengthy research paper, try not to exceed the word limit of 10% of the entire research paper.
How to Structure Your Research Summary
The research summary is nothing but a concise form of the entire research paper. Therefore, the structure of a summary stays the same as the paper. So, include all the section titles and write a little about them. The structural elements that a research summary must consist of are:
It represents the topic of the research. Try to phrase it so that it includes the key findings or conclusion of the task.
The abstract gives a context of the research paper. Unlike the abstract at the beginning of a paper, the abstract here, should be very short since you’ll be working with a limited word count.
Introduction
This is the most crucial section of a research summary as it helps readers get familiarized with the topic. You should include the definition of your topic, the current state of the investigation, and practical relevance in this part. Additionally, you should present the problem statement, investigative measures, and any hypothesis in this section.
Methodology
This section provides details about the methodology and the methods adopted to conduct the study. You should write a brief description of the surveys, sampling, type of experiments, statistical analysis, and the rationality behind choosing those particular methods.
Create a list of evidence obtained from the various experiments with a primary analysis, conclusions, and interpretations made upon that. In the paper research paper, you will find the results section as the most detailed and lengthy part. Therefore, you must pick up the key elements and wisely decide which elements are worth including and which are worth skipping.
This is where you present the interpretation of results in the context of their application. Discussion usually covers results, inferences, and theoretical models explaining the obtained values, key strengths, and limitations. All of these are vital elements that you must include in the summary.
Most research papers merge conclusion with discussions. However, depending upon the instructions, you may have to prepare this as a separate section in your research summary. Usually, conclusion revisits the hypothesis and provides the details about the validation or denial about the arguments made in the research paper, based upon how convincing the results were obtained.
The structure of a research summary closely resembles the anatomy of a scholarly article . Additionally, you should keep your research and references limited to authentic and scholarly sources only.
Tips for Writing a Research Summary
The core concept behind undertaking a research summary is to present a simple and clear understanding of your research paper to the reader. The biggest hurdle while doing that is the number of words you have at your disposal. So, follow the steps below to write a research summary that sticks.
1. Read the parent paper thoroughly
You should go through the research paper thoroughly multiple times to ensure that you have a complete understanding of its contents. A 3-stage reading process helps.
a. Scan: In the first read, go through it to get an understanding of its basic concept and methodologies.
b. Read: For the second step, read the article attentively by going through each section, highlighting the key elements, and subsequently listing the topics that you will include in your research summary.
c. Skim: Flip through the article a few more times to study the interpretation of various experimental results, statistical analysis, and application in different contexts.
Sincerely go through different headings and subheadings as it will allow you to understand the underlying concept of each section. You can try reading the introduction and conclusion simultaneously to understand the motive of the task and how obtained results stay fit to the expected outcome.
2. Identify the key elements in different sections
While exploring different sections of an article, you can try finding answers to simple what, why, and how. Below are a few pointers to give you an idea:
- What is the research question and how is it addressed?
- Is there a hypothesis in the introductory part?
- What type of methods are being adopted?
- What is the sample size for data collection and how is it being analyzed?
- What are the most vital findings?
- Do the results support the hypothesis?
Discussion/Conclusion
- What is the final solution to the problem statement?
- What is the explanation for the obtained results?
- What is the drawn inference?
- What are the various limitations of the study?
3. Prepare the first draft
Now that you’ve listed the key points that the paper tries to demonstrate, you can start writing the summary following the standard structure of a research summary. Just make sure you’re not writing statements from the parent research paper verbatim.
Instead, try writing down each section in your own words. This will not only help in avoiding plagiarism but will also show your complete understanding of the subject. Alternatively, you can use a summarizing tool (AI-based summary generators) to shorten the content or summarize the content without disrupting the actual meaning of the article.
SciSpace Copilot is one such helpful feature! You can easily upload your research paper and ask Copilot to summarize it. You will get an AI-generated, condensed research summary. SciSpace Copilot also enables you to highlight text, clip math and tables, and ask any question relevant to the research paper; it will give you instant answers with deeper context of the article..
4. Include visuals
One of the best ways to summarize and consolidate a research paper is to provide visuals like graphs, charts, pie diagrams, etc.. Visuals make getting across the facts, the past trends, and the probabilistic figures around a concept much more engaging.
5. Double check for plagiarism
It can be very tempting to copy-paste a few statements or the entire paragraphs depending upon the clarity of those sections. But it’s best to stay away from the practice. Even paraphrasing should be done with utmost care and attention.
Also: QuillBot vs SciSpace: Choose the best AI-paraphrasing tool
6. Religiously follow the word count limit
You need to have strict control while writing different sections of a research summary. In many cases, it has been observed that the research summary and the parent research paper become the same length. If that happens, it can lead to discrediting of your efforts and research summary itself. Whatever the standard word limit has been imposed, you must observe that carefully.
7. Proofread your research summary multiple times
The process of writing the research summary can be exhausting and tiring. However, you shouldn’t allow this to become a reason to skip checking your academic writing several times for mistakes like misspellings, grammar, wordiness, and formatting issues. Proofread and edit until you think your research summary can stand out from the others, provided it is drafted perfectly on both technicality and comprehension parameters. You can also seek assistance from editing and proofreading services , and other free tools that help you keep these annoying grammatical errors at bay.
8. Watch while you write
Keep a keen observation of your writing style. You should use the words very precisely, and in any situation, it should not represent your personal opinions on the topic. You should write the entire research summary in utmost impersonal, precise, factually correct, and evidence-based writing.
9. Ask a friend/colleague to help
Once you are done with the final copy of your research summary, you must ask a friend or colleague to read it. You must test whether your friend or colleague could grasp everything without referring to the parent paper. This will help you in ensuring the clarity of the article.
Once you become familiar with the research paper summary concept and understand how to apply the tips discussed above in your current task, summarizing a research summary won’t be that challenging. While traversing the different stages of your academic career, you will face different scenarios where you may have to create several research summaries.
In such cases, you just need to look for answers to simple questions like “Why this study is necessary,” “what were the methods,” “who were the participants,” “what conclusions were drawn from the research,” and “how it is relevant to the wider world.” Once you find out the answers to these questions, you can easily create a good research summary following the standard structure and a precise writing style.

You might also like

Consensus GPT vs. SciSpace GPT: Choose the Best GPT for Research
Literature Review and Theoretical Framework: Understanding the Differences

Types of Essays in Academic Writing - Quick Guide (2024)
The Sheridan Libraries
- Write a Literature Review
- Sheridan Libraries
- Find This link opens in a new window
- Evaluate This link opens in a new window

Not every source you found should be included in your annotated bibliography or lit review. Only include the most relevant and most important sources.
Get Organized
- Lit Review Prep Use this template to help you evaluate your sources, create article summaries for an annotated bibliography, and a synthesis matrix for your lit review outline.
Summarize your Sources
Summarize each source: Determine the most important and relevant information from each source, such as the findings, methodology, theories, etc. Consider using an article summary, or study summary to help you organize and summarize your sources.
Paraphrasing
- Use your own words, and do not copy and paste the abstract
- The library's tutorials about plagiarism are excellent, and will help you with paraphasing correctly
Annotated Bibliographies
Annotated bibliographies can help you clearly see and understand the research before diving into organizing and writing your literature review. Although typically part of the "summarize" step of the literature review, annotations should not merely be summaries of each article - instead, they should be critical evaluations of the source, and help determine a source's usefulness for your lit review.
Definition:
A list of citations on a particular topic followed by an evaluation of the source’s argument and other relevant material including its intended audience, sources of evidence, and methodology
- Explore your topic.
- Appraise issues or factors associated with your professional practice and research topic.
- Help you get started with the literature review.
- Think critically about your topic, and the literature.
Steps to Creating an Annotated Bibliography:
- Find Your Sources
- Read Your Sources
- Identify the Most Relevant Sources
- Cite your Sources
- Write Annotations
Annotated Bibliography Resources
- Purdue Owl Guide
- Cornell Annotated Bibliography Guide
- << Previous: Evaluate
- Next: Synthesize >>
- Last Updated: Sep 26, 2023 10:25 AM
- URL: https://guides.library.jhu.edu/lit-review

How To Write The Results/Findings Chapter
For quantitative studies (dissertations & theses).
By: Derek Jansen (MBA). Expert Reviewed By: Kerryn Warren (PhD) | July 2021
So, you’ve completed your quantitative data analysis and it’s time to report on your findings. But where do you start? In this post, we’ll walk you through the results chapter (also called the findings or analysis chapter), step by step, so that you can craft this section of your dissertation or thesis with confidence. If you’re looking for information regarding the results chapter for qualitative studies, you can find that here .
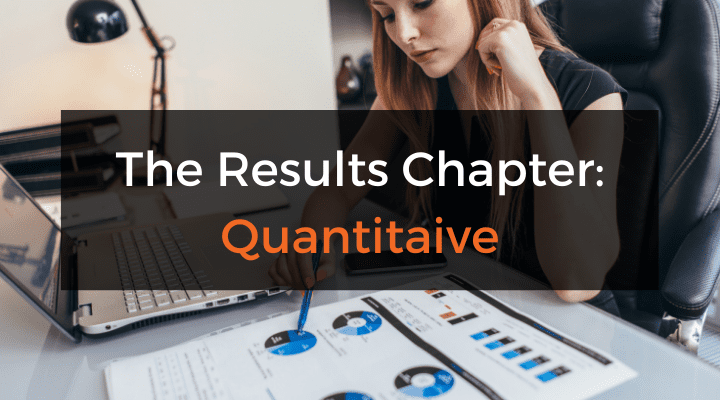
Overview: Quantitative Results Chapter
- What exactly the results/findings/analysis chapter is
- What you need to include in your results chapter
- How to structure your results chapter
- A few tips and tricks for writing top-notch chapter
What exactly is the results chapter?
The results chapter (also referred to as the findings or analysis chapter) is one of the most important chapters of your dissertation or thesis because it shows the reader what you’ve found in terms of the quantitative data you’ve collected. It presents the data using a clear text narrative, supported by tables, graphs and charts. In doing so, it also highlights any potential issues (such as outliers or unusual findings) you’ve come across.
But how’s that different from the discussion chapter?
Well, in the results chapter, you only present your statistical findings. Only the numbers, so to speak – no more, no less. Contrasted to this, in the discussion chapter , you interpret your findings and link them to prior research (i.e. your literature review), as well as your research objectives and research questions . In other words, the results chapter presents and describes the data, while the discussion chapter interprets the data.
Let’s look at an example.
In your results chapter, you may have a plot that shows how respondents to a survey responded: the numbers of respondents per category, for instance. You may also state whether this supports a hypothesis by using a p-value from a statistical test. But it is only in the discussion chapter where you will say why this is relevant or how it compares with the literature or the broader picture. So, in your results chapter, make sure that you don’t present anything other than the hard facts – this is not the place for subjectivity.
It’s worth mentioning that some universities prefer you to combine the results and discussion chapters. Even so, it is good practice to separate the results and discussion elements within the chapter, as this ensures your findings are fully described. Typically, though, the results and discussion chapters are split up in quantitative studies. If you’re unsure, chat with your research supervisor or chair to find out what their preference is.
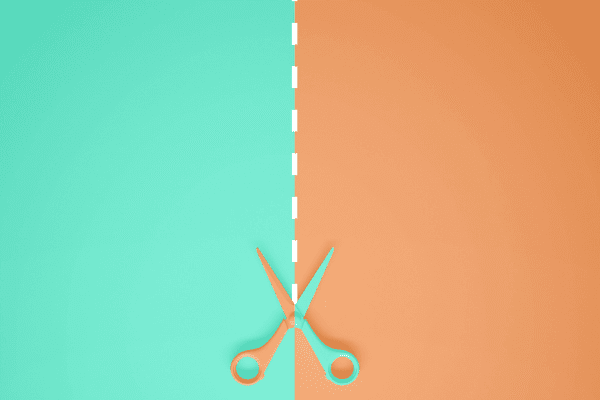
What should you include in the results chapter?
Following your analysis, it’s likely you’ll have far more data than are necessary to include in your chapter. In all likelihood, you’ll have a mountain of SPSS or R output data, and it’s your job to decide what’s most relevant. You’ll need to cut through the noise and focus on the data that matters.
This doesn’t mean that those analyses were a waste of time – on the contrary, those analyses ensure that you have a good understanding of your dataset and how to interpret it. However, that doesn’t mean your reader or examiner needs to see the 165 histograms you created! Relevance is key.
How do I decide what’s relevant?
At this point, it can be difficult to strike a balance between what is and isn’t important. But the most important thing is to ensure your results reflect and align with the purpose of your study . So, you need to revisit your research aims, objectives and research questions and use these as a litmus test for relevance. Make sure that you refer back to these constantly when writing up your chapter so that you stay on track.
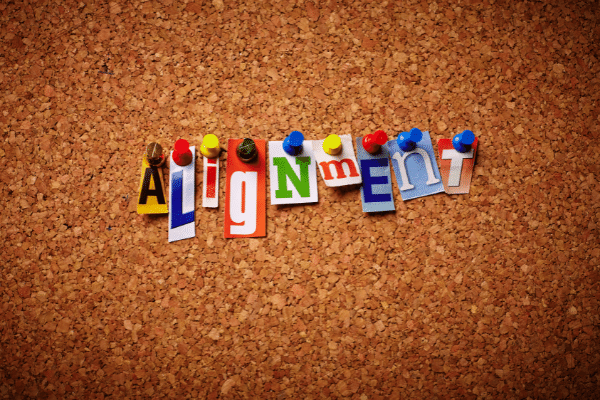
As a general guide, your results chapter will typically include the following:
- Some demographic data about your sample
- Reliability tests (if you used measurement scales)
- Descriptive statistics
- Inferential statistics (if your research objectives and questions require these)
- Hypothesis tests (again, if your research objectives and questions require these)
We’ll discuss each of these points in more detail in the next section.
Importantly, your results chapter needs to lay the foundation for your discussion chapter . This means that, in your results chapter, you need to include all the data that you will use as the basis for your interpretation in the discussion chapter.
For example, if you plan to highlight the strong relationship between Variable X and Variable Y in your discussion chapter, you need to present the respective analysis in your results chapter – perhaps a correlation or regression analysis.
Need a helping hand?
How do I write the results chapter?
There are multiple steps involved in writing up the results chapter for your quantitative research. The exact number of steps applicable to you will vary from study to study and will depend on the nature of the research aims, objectives and research questions . However, we’ll outline the generic steps below.
Step 1 – Revisit your research questions
The first step in writing your results chapter is to revisit your research objectives and research questions . These will be (or at least, should be!) the driving force behind your results and discussion chapters, so you need to review them and then ask yourself which statistical analyses and tests (from your mountain of data) would specifically help you address these . For each research objective and research question, list the specific piece (or pieces) of analysis that address it.
At this stage, it’s also useful to think about the key points that you want to raise in your discussion chapter and note these down so that you have a clear reminder of which data points and analyses you want to highlight in the results chapter. Again, list your points and then list the specific piece of analysis that addresses each point.
Next, you should draw up a rough outline of how you plan to structure your chapter . Which analyses and statistical tests will you present and in what order? We’ll discuss the “standard structure” in more detail later, but it’s worth mentioning now that it’s always useful to draw up a rough outline before you start writing (this advice applies to any chapter).
Step 2 – Craft an overview introduction
As with all chapters in your dissertation or thesis, you should start your quantitative results chapter by providing a brief overview of what you’ll do in the chapter and why . For example, you’d explain that you will start by presenting demographic data to understand the representativeness of the sample, before moving onto X, Y and Z.
This section shouldn’t be lengthy – a paragraph or two maximum. Also, it’s a good idea to weave the research questions into this section so that there’s a golden thread that runs through the document.
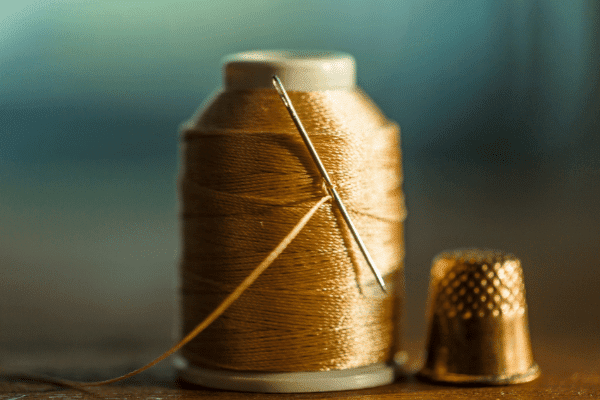
Step 3 – Present the sample demographic data
The first set of data that you’ll present is an overview of the sample demographics – in other words, the demographics of your respondents.
For example:
- What age range are they?
- How is gender distributed?
- How is ethnicity distributed?
- What areas do the participants live in?
The purpose of this is to assess how representative the sample is of the broader population. This is important for the sake of the generalisability of the results. If your sample is not representative of the population, you will not be able to generalise your findings. This is not necessarily the end of the world, but it is a limitation you’ll need to acknowledge.
Of course, to make this representativeness assessment, you’ll need to have a clear view of the demographics of the population. So, make sure that you design your survey to capture the correct demographic information that you will compare your sample to.
But what if I’m not interested in generalisability?
Well, even if your purpose is not necessarily to extrapolate your findings to the broader population, understanding your sample will allow you to interpret your findings appropriately, considering who responded. In other words, it will help you contextualise your findings . For example, if 80% of your sample was aged over 65, this may be a significant contextual factor to consider when interpreting the data. Therefore, it’s important to understand and present the demographic data.
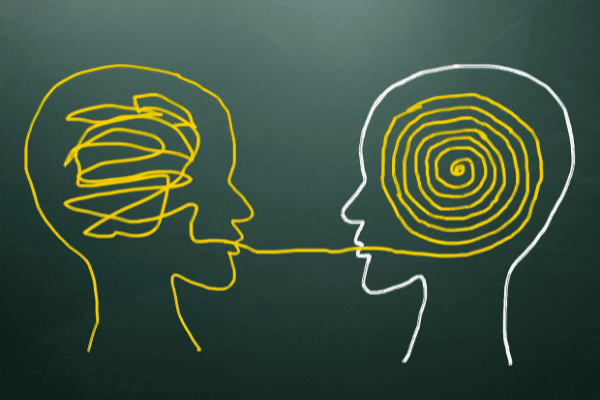
Step 4 – Review composite measures and the data “shape”.
Before you undertake any statistical analysis, you’ll need to do some checks to ensure that your data are suitable for the analysis methods and techniques you plan to use. If you try to analyse data that doesn’t meet the assumptions of a specific statistical technique, your results will be largely meaningless. Therefore, you may need to show that the methods and techniques you’ll use are “allowed”.
Most commonly, there are two areas you need to pay attention to:
#1: Composite measures
The first is when you have multiple scale-based measures that combine to capture one construct – this is called a composite measure . For example, you may have four Likert scale-based measures that (should) all measure the same thing, but in different ways. In other words, in a survey, these four scales should all receive similar ratings. This is called “ internal consistency ”.
Internal consistency is not guaranteed though (especially if you developed the measures yourself), so you need to assess the reliability of each composite measure using a test. Typically, Cronbach’s Alpha is a common test used to assess internal consistency – i.e., to show that the items you’re combining are more or less saying the same thing. A high alpha score means that your measure is internally consistent. A low alpha score means you may need to consider scrapping one or more of the measures.
#2: Data shape
The second matter that you should address early on in your results chapter is data shape. In other words, you need to assess whether the data in your set are symmetrical (i.e. normally distributed) or not, as this will directly impact what type of analyses you can use. For many common inferential tests such as T-tests or ANOVAs (we’ll discuss these a bit later), your data needs to be normally distributed. If it’s not, you’ll need to adjust your strategy and use alternative tests.
To assess the shape of the data, you’ll usually assess a variety of descriptive statistics (such as the mean, median and skewness), which is what we’ll look at next.
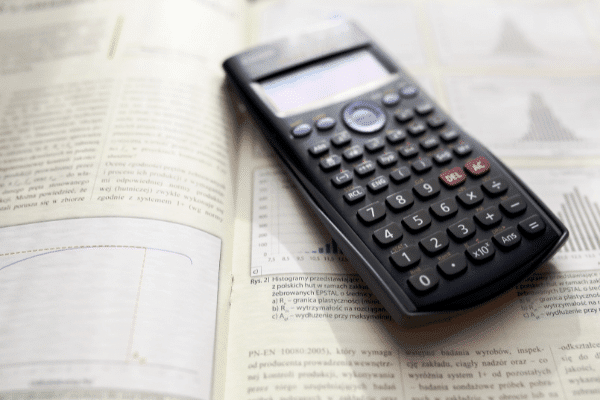
Step 5 – Present the descriptive statistics
Now that you’ve laid the foundation by discussing the representativeness of your sample, as well as the reliability of your measures and the shape of your data, you can get started with the actual statistical analysis. The first step is to present the descriptive statistics for your variables.
For scaled data, this usually includes statistics such as:
- The mean – this is simply the mathematical average of a range of numbers.
- The median – this is the midpoint in a range of numbers when the numbers are arranged in order.
- The mode – this is the most commonly repeated number in the data set.
- Standard deviation – this metric indicates how dispersed a range of numbers is. In other words, how close all the numbers are to the mean (the average).
- Skewness – this indicates how symmetrical a range of numbers is. In other words, do they tend to cluster into a smooth bell curve shape in the middle of the graph (this is called a normal or parametric distribution), or do they lean to the left or right (this is called a non-normal or non-parametric distribution).
- Kurtosis – this metric indicates whether the data are heavily or lightly-tailed, relative to the normal distribution. In other words, how peaked or flat the distribution is.
A large table that indicates all the above for multiple variables can be a very effective way to present your data economically. You can also use colour coding to help make the data more easily digestible.
For categorical data, where you show the percentage of people who chose or fit into a category, for instance, you can either just plain describe the percentages or numbers of people who responded to something or use graphs and charts (such as bar graphs and pie charts) to present your data in this section of the chapter.
When using figures, make sure that you label them simply and clearly , so that your reader can easily understand them. There’s nothing more frustrating than a graph that’s missing axis labels! Keep in mind that although you’ll be presenting charts and graphs, your text content needs to present a clear narrative that can stand on its own. In other words, don’t rely purely on your figures and tables to convey your key points: highlight the crucial trends and values in the text. Figures and tables should complement the writing, not carry it .
Depending on your research aims, objectives and research questions, you may stop your analysis at this point (i.e. descriptive statistics). However, if your study requires inferential statistics, then it’s time to deep dive into those .
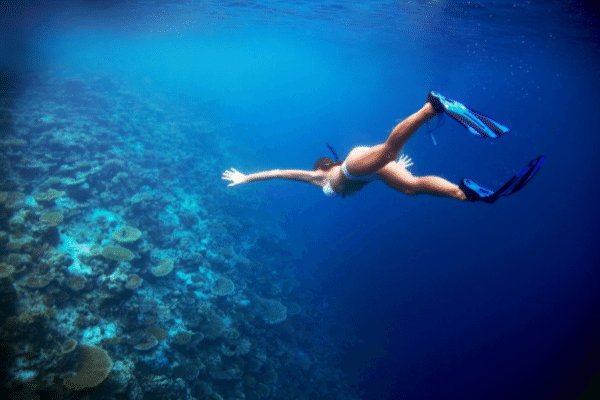
Step 6 – Present the inferential statistics
Inferential statistics are used to make generalisations about a population , whereas descriptive statistics focus purely on the sample . Inferential statistical techniques, broadly speaking, can be broken down into two groups .
First, there are those that compare measurements between groups , such as t-tests (which measure differences between two groups) and ANOVAs (which measure differences between multiple groups). Second, there are techniques that assess the relationships between variables , such as correlation analysis and regression analysis. Within each of these, some tests can be used for normally distributed (parametric) data and some tests are designed specifically for use on non-parametric data.
There are a seemingly endless number of tests that you can use to crunch your data, so it’s easy to run down a rabbit hole and end up with piles of test data. Ultimately, the most important thing is to make sure that you adopt the tests and techniques that allow you to achieve your research objectives and answer your research questions .
In this section of the results chapter, you should try to make use of figures and visual components as effectively as possible. For example, if you present a correlation table, use colour coding to highlight the significance of the correlation values, or scatterplots to visually demonstrate what the trend is. The easier you make it for your reader to digest your findings, the more effectively you’ll be able to make your arguments in the next chapter.
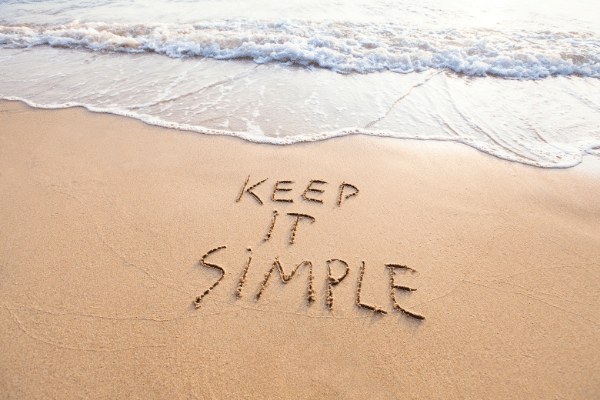
Step 7 – Test your hypotheses
If your study requires it, the next stage is hypothesis testing. A hypothesis is a statement , often indicating a difference between groups or relationship between variables, that can be supported or rejected by a statistical test. However, not all studies will involve hypotheses (again, it depends on the research objectives), so don’t feel like you “must” present and test hypotheses just because you’re undertaking quantitative research.
The basic process for hypothesis testing is as follows:
- Specify your null hypothesis (for example, “The chemical psilocybin has no effect on time perception).
- Specify your alternative hypothesis (e.g., “The chemical psilocybin has an effect on time perception)
- Set your significance level (this is usually 0.05)
- Calculate your statistics and find your p-value (e.g., p=0.01)
- Draw your conclusions (e.g., “The chemical psilocybin does have an effect on time perception”)
Finally, if the aim of your study is to develop and test a conceptual framework , this is the time to present it, following the testing of your hypotheses. While you don’t need to develop or discuss these findings further in the results chapter, indicating whether the tests (and their p-values) support or reject the hypotheses is crucial.
Step 8 – Provide a chapter summary
To wrap up your results chapter and transition to the discussion chapter, you should provide a brief summary of the key findings . “Brief” is the keyword here – much like the chapter introduction, this shouldn’t be lengthy – a paragraph or two maximum. Highlight the findings most relevant to your research objectives and research questions, and wrap it up.
Some final thoughts, tips and tricks
Now that you’ve got the essentials down, here are a few tips and tricks to make your quantitative results chapter shine:
- When writing your results chapter, report your findings in the past tense . You’re talking about what you’ve found in your data, not what you are currently looking for or trying to find.
- Structure your results chapter systematically and sequentially . If you had two experiments where findings from the one generated inputs into the other, report on them in order.
- Make your own tables and graphs rather than copying and pasting them from statistical analysis programmes like SPSS. Check out the DataIsBeautiful reddit for some inspiration.
- Once you’re done writing, review your work to make sure that you have provided enough information to answer your research questions , but also that you didn’t include superfluous information.
If you’ve got any questions about writing up the quantitative results chapter, please leave a comment below. If you’d like 1-on-1 assistance with your quantitative analysis and discussion, check out our hands-on coaching service , or book a free consultation with a friendly coach.
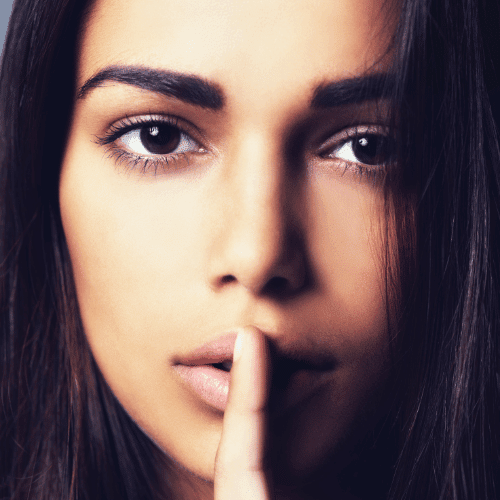
Psst... there’s more!
This post was based on one of our popular Research Bootcamps . If you're working on a research project, you'll definitely want to check this out ...
You Might Also Like:
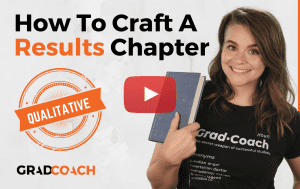
Thank you. I will try my best to write my results.
Awesome content 👏🏾
this was great explaination
Submit a Comment Cancel reply
Your email address will not be published. Required fields are marked *
Save my name, email, and website in this browser for the next time I comment.
- Print Friendly

An official website of the United States government
The .gov means it’s official. Federal government websites often end in .gov or .mil. Before sharing sensitive information, make sure you’re on a federal government site.
The site is secure. The https:// ensures that you are connecting to the official website and that any information you provide is encrypted and transmitted securely.
- Publications
- Account settings
Preview improvements coming to the PMC website in October 2024. Learn More or Try it out now .
- Advanced Search
- Journal List
- Malays Fam Physician
- v.2(1); 2007
Summarizing Research Findings: Systematic Review and Meta-Analysis
MSc, PhD, Lecturer, Health Research Development Unit (HeRDU), Faculty of Medicine, University of Malaya
The explosion of biomedical publishing makes keeping up with the primary studies an impossible task. The often disparate, confusing and contradicting findings of individual studies makes healthcare professionals turn to review articles where knowledge has been collated and published in summaries. Narrative reviews lack rigorous, systematic and reproducible synthesis. In contrast, systematic reviews are conducted using systematic and explicit methods to identify, select and critically appraise relevant research, and to collect and analyse data from the studies that are included in the review. The final pathway for systematic review is a statistical summary of the results of primary studies, or meta-analysis. This article provides some guidelines to health care providers in understanding the key aspects of systematic review and meta-analysis. Steps involved in systematic review are discussed. The potential pitfall of meta-analysis was also explored.
THE NEED FOR REVIEWS
The huge amount of medical information available and its exponential growth have become common problem in the literature of biomedical information. Health care practitioners face the explosion in biomedical knowledge which makes keeping up with the primary research an impossible feat. There are approximately 17,000 new biomedical books published every year along with 30,000 biomedical journals, with an annual increase of 7%. For instance, MEDLINE alone contains more than eleven million citations, and more than 400,000 articles are added to the file each year. 1 Majority health care providers noted that the current volume of scientific literature is unmanageable 2 and often do not have sufficient time for reading medical journals as the information explosion continues. 3 Further, some of these studies could be unclear, confusing or may also have contradicting results.
THE NARRATIVE REVIEWS
To make this task easier and manageable for health care providers as well as decision makers, reviews are often among their information resources. Reviews have always been a part of the medical literature. Traditionally, medical research has been integrated in the narrative or nonsystematic form. An expert in a particular field will review studies, decided on the relevance, and highlight the findings, both in terms of results and, to a lesser degree the methodology. 4 Such narrative reviews tend to be unsystematic and susceptible to many biases. Firstly, no systematic approach is prescribed to obtain the primary data and to integrate the data. Often, subjective judgment of the reviewer was used. There were often no explicit standards exist to assess the quality of review. Moreover, narrative reviewer also does not synthesize data quantitatively.
A CALL FOR SYSTEMATIC REVIEWS
A systematic review is defined by the Cochrane Handbook as ‘A review of a clearly formulated question that uses systematic and explicit methods to identify, select and critically appraise relevant research, and to collect and analyse data from the studies that are included in the review’. In contrast to narrative review, systematic review allows readers to appraise how the review was conducted and synthesized. It is of particular value in bringing together a number of separately conducted studies, sometimes with conflicting findings, and synthesizing their results. Systematic reviews have been proven to be able to yield valid, precise, and widely applicable answers to clinical questions. 5 In short, systematic reviews summarise large amounts of information and are more likely than individual trials to describe the true clinical effect of an intervention. Thus, systematic reviews have come to play a central role in informing clinical decisions and guidelines. A systematic review is also often called an ‘overview’.
A meta-analysis takes a systematic review one step further by statistically pooling the results of combinable studies. Since its introduction, meta-analysis has established itself as an influential branch of clinical epidemiology and health services research, with hundreds of meta-analyses published in the medical literature each year. 6
THE PROCESS OF SYSTEMATIC REVIEW
Systematic review should be carefully planned with a detailed written protocol prepared in advance as any other search project. Systematic review involves several discrete steps and the steps are summarised below.
Step 1: Formulate review question . This requires the formulation of a clear statement of relevant patient groups, intervention of interest, as well as outcomes. The details are used to select studies for inclusion in the review.
Step 2: Locate studies . Systematic review must be undertaken in accordance with a predefined search strategy that would allow the completeness of the search to be assessed. Search strategies should consider the following sources: The Cochrane Controlled Trials Register (CCTR), other electronic databases and trials registered not covered by CCTR, checking reference lists, hand searching of key journals and personal communication with experts in the fields. The selection of primary studies is governed by inclusion and exclusion criteria that are initially specified when the protocol is defined.
Step 3: Appraising the quality of studies . After an exhaustive search, all possible primary studies that have been identified need to be assessed for eligibility for inclusion. Application of stringent inclusion/exclusion criteria should be addressed for example types of participants, interventions, outcomes, study designs and methodological quality. Independent assessment by more than one observer is desirable.
Step 4: Combining the results. The findings from combinable individual primary studies are then pooled to produce an ‘overall estimate’ on the clinical effectiveness of the intervention. The aggregation can be qualitative, or more appropriate, by statistically combining the data produced by individual studies into a single summary estimate. The statistical pooling of data is termed meta-analysis ( Figure 1 ). In meta-analysis, results from studies are combined using ‘inverse variance method’, whereby larger studies and studies with less random variation are given greater weight than smaller studies.

Meta-analysis: Data from several different studies are combined and produce a single estimate
Meta-analysis can only be undertaken when studies address the same question, administer the intervention in a similar manner or measure the same outcomes. When studies differ in one or more of these components, meta-analysis is not appropriate. Therefore, systematic review may or may not include meta-analysis.
In meta-analysis, for outcomes measured on a continuous scale, the weighted mean difference is commonly used. For outcomes measured on a dichotomous scale, common approaches include the use of odds ratio or relative risk. There are two approaches for combining the data: fixed-effects model assume that an intervention has a single true effect whereas random-effects models assume that an effect may vary across studies. 7 The results of meta-analysis can be displayed graphically (Forest plot) to allow a visual comparison of findings of individual studies ( Figure 2 ).

Forest plot of meta-analysis
Systematic review should continue with an investigation of the reasons for heterogeneity. Subgroup analysis, sensitivity analysis and meta-regression are frequently used to investigate heterogeneity of individual studies in meta-analysis. One of the major drawbacks to using meta-analysis is the possibility of publication bias. One way to investigate whether a review is subject to publication bias is to prepare a ‘funnel plot’ ( Figure 3 ) and examine this for signs of asymmetry. 8

Funnel plot showing evidence of publication bias
Step 5: Interpret results. The findings from systematic review and statistical pooling of the studies then need to be interpreted, discussed and set out the implications for practice or further research. Issues such as the quality and heterogeneity of the included studies plus the possible impact of bias need to be discussed.
PITFALLS AND PROBLEMS
Meta-analyses have received mixed receptions. Some see meta-analysis as an exercise of ‘mega-silliness’, 9 ‘a tool has become a weapon’ 10 and a number of statisticians think that meta-analysis represents the unacceptable face of statisticism. 11 There are also those that still prefer the conventional narrative review article. 12 The mixed receptions were due to opposite conclusions observed in some systematic reviews that address the same issue. 13 , 14 Also, meta-analyses of small trials were discovered to contradict by a single large randomized trial. 15
Publication bias could be a serious problem for meta-analyses, secondly, studies may be of varying quality. Clearly the quality of trials included in a systematic review and meta-analysis is of crucial importance and should be of high methodological quality as well as free from biases. Meta-analysis should therefore be considered only within the framework of systematic review that has been prepared using a systematic approach to mitigate all kinds of biases and explicitly address the issue of the completeness of the evidence identified, the quality of component studies and the combinability of studies. 15 The Cochrane Collaboration has been established to overcome this problem by providing high quality and authoritative systematic reviews and meta-analyses. 16 , 17 The collaboration not only ensures that high-quality reviews are conducted but also update reviews when new evidence becomes available.
Systematic review is an invaluable resource for both clinicians and researchers. However, not all reviews are systematic and even those that are described as systematic may be methodologically flawed. Nonetheless, a high quality systematic review provides the best available evidence. The usefulness of a systematic review can further be enhanced by statistical summary of the results by meta-analytical technique. Pooling individual studies may reduce the risk of random error, increase statistical power and allow for a more accurate estimate of effect size.
SOME TERMINOLOGIES
Bias (synonym: systematic error): the distortion of the outcome, as a result of a known or unknown variable other than intervention (i.e. the tendency to produce results that depart from the “true” result).
Cochrane Collaboration : The Cochrane Collaboration is an international organization that aims to help people make well-informed decisions about healthcare by preparing, maintaining & promoting the accessibility of systematic reviews of the effects of healthcare interventions.
Cochrane Controlled Trials Register (CCTR): CCTR is a database of references to controlled trials in health care.
Critical appraisal : systematically finding, appraising and interpreting evidence of effectiveness. It is aimed to examine research evidence to assess its validity, results and relevance before using it to inform a decision.
Cumulative meta-analysis : the repeated performance of meta-analysis whenever a new trial becomes available for inclusion. In cumulative meta-analysis studies are added one at a time in a specified order.
Effect size : refers to the size of a relationship between an expose and an outcome. The term is applied to measurement of the differences in the outcome between the study groups. Relative risk, odds ratio, and risk differences can be defined as effect sizes for dichotomous scale. Effect size of continuous variable is the standardized mean differences.
Fixed-effect model : a mathematical model that combines the results of studies that assume the effect of the intervention is constant in all subject population studied. Only within study variation is included when assessing the uncertainty of results.
Forest plot : a forest plot presents the means and variance for the difference for each pooled primary study. The line represents the standard error of the difference, the box represents the mean difference and its size proportional to the number of subjects in the study. The bottom entry in a forest plot is the summary estimate of the treatment difference and confidence interval for the summary difference ( Figure 2 ).
Funnel plot : a graphical method of assessing bias; the effect size of each study is plotted against some measure of study information. If the shape of the plot resembles an inverted funnel, it can be stated that there is no evidence of publication bias within the systematic review ( Figure 3 ).
Heterogeneity : the variability between studies in terms of key characteristics (i.e. ecological variables) quality (i.e. methodology) or effect (i.e. results). Statistical tests of heterogeneity may be used to assess whether the observed variability in effect size (i.e. study results) is greater than that expected to occur purely by chance.
Meta-regression : a multivariable model investigating effect size from individual studies, generally weighted by sample size, as a function of various study characteristics (i.e. to investigate whether study characteristics are influencing effect size).
Outlier : an outlier study in meta-analysis is study that results very different from the rest of the studies. Outlier could alter the conclusions of a meta-analysis.
Overall estimate : is the pooled estimate from a meta-analysis. The overall estimate from a meta-analysis is always displayed with its confidence interval.
Primary studies : Individual studies contributing to a systematic review are called primary studies whereas a systematic review is a form of a secondary study.
Publication bias : publication bias refers to the problem that positive results are more likely to be published than negative results and this may therefore give a misleading assessment of the impact of an intervention. Publication bias can be examined via a funnel plot.
Random-effects model : a mathematical model for combining the results of studies that allow for variation in the effect of the intervention amongst the subject populations studied. Both within-study variation and between-study variation is included when assessing the uncertainty of results.
Review : article that summarizes a number of primary studies and discusses the effectiveness of a particular intervention. It may not be a systematic review.
Search strategy : a description of the methodology used to locate and identify research articles pertinent to a systematic review, as specified within the relevant protocol. It includes a list of search terms, based on the subject, intervention and outcome of the review, to be used when searching electronic databases, websites, reference lists and when engaging with personal contacts. If required, the strategy may be modified once the search has commenced.
Sensitivity analysis : repetition of the analysis using different sets of assumptions in order to determine the impact of variation arising from these assumptions, or uncertain decisions, on the results of a systematic review.
Subgroup analysis : used to determine if the effects of an intervention vary between subgroups in the systematic review.
Weighted mean difference : a method used to combine measures on continuous scales (where the mean, standard deviation and sample size in each group are known) and the weight given to each study is determined by the precision of its estimate of effect.
Cochrane Systematic Review and SEA-ORCHID Project
Cochrane systematic reviews combine the results of the best medical research using rigorous methods, and are regarded as the gold standard of reference for health care professionals. Malaysia has relatively minor involvement in Cochrane Collaboration despite its economic growth and the fast improving standard of medical care. It is likely that clinical questions with high relevance to Malaysia are therefore not being addressed in Cochrane reviews.
The SEA-ORCHID project, which stands for South East Asia Optimising Reproductive and Child Health Outcomes in Developing Countries Project, is a five-year project (2003 to 2008) aiming to promote the synthesis and application of high level clinical evidence on issues relevant to this region, focusing on maternal and child health but also involving other related disciplines. Jointly funded by the Wellcome Trust and the Australian National Health and Medical Research Council and supported by the Cochrane Australasian Centre, the project activities include regular Cochrane Systematic Review Workshop and work-in sessions throughout the country. This is a good opportunity for the pool of clinical and research talents in our country to contribute in synthesizing the best clinical evidence and making a significant impact on evidence-based health care.
If you are interested in authoring or co-authoring a Cochrane review, you will be guided at every step by experienced reviewers leading to its publication in the Cochrane Library. In this workshop, you will also hear the experiences of people who are in the process of developing a protocol or review.
For further information please contact:
- Professor Jackie Ho [email protected]
- Dr Lai Nai Ming [email protected]

- PRO Courses Guides New Tech Help Pro Expert Videos About wikiHow Pro Upgrade Sign In
- EDIT Edit this Article
- EXPLORE Tech Help Pro About Us Random Article Quizzes Request a New Article Community Dashboard This Or That Game Popular Categories Arts and Entertainment Artwork Books Movies Computers and Electronics Computers Phone Skills Technology Hacks Health Men's Health Mental Health Women's Health Relationships Dating Love Relationship Issues Hobbies and Crafts Crafts Drawing Games Education & Communication Communication Skills Personal Development Studying Personal Care and Style Fashion Hair Care Personal Hygiene Youth Personal Care School Stuff Dating All Categories Arts and Entertainment Finance and Business Home and Garden Relationship Quizzes Cars & Other Vehicles Food and Entertaining Personal Care and Style Sports and Fitness Computers and Electronics Health Pets and Animals Travel Education & Communication Hobbies and Crafts Philosophy and Religion Work World Family Life Holidays and Traditions Relationships Youth
- Browse Articles
- Learn Something New
- Quizzes Hot
- This Or That Game New
- Train Your Brain
- Explore More
- Support wikiHow
- About wikiHow
- Log in / Sign up
- Education and Communications
- College University and Postgraduate
- Academic Writing
- Research Papers
How to Write a Summary of a Research Paper
Last Updated: July 10, 2020 References
This article was co-authored by wikiHow staff writer, Hannah Madden . Hannah Madden is a writer, editor, and artist currently living in Portland, Oregon. In 2018, she graduated from Portland State University with a B.S. in Environmental Studies. Hannah enjoys writing articles about conservation, sustainability, and eco-friendly products. When she isn’t writing, you can find Hannah working on hand embroidery projects and listening to music. This article has been viewed 28,163 times. Learn more...
Writing a summary of an academic research paper is an important skill, and it shows that you understand all of the relevant information presented to you. However, writing a summary can be tough, since it requires you to be completely objective and keep any analysis or criticisms to yourself. By keeping your goal in mind as you read the paper and focusing on the key points, you can write a succinct, accurate summary of a research paper to prove that you understood the overall conclusion.
Reading the Research Paper

- For instance, if you’re supporting an argument in your own research paper, focus on the elements that are similar to yours.
- Or, if you’re comparing and contrasting methodology, focus on the methods and the significance of the results.

- You can also read the abstract of the paper as a good example of what the authors find to be important in their article.

- Depending on how long and dense the paper is, your initial reading could take you up to an hour or more.

- The important information will usually be toward the end of the paper as the authors explain their findings and conclusions.

- Writing a summary without plagiarizing, or copying the paper, is really important. Writing notes in your own words will help you get into the mindset of relaying information in your own way.
Including Relevant Information

- For example, “The methods used in this paper are not up to standards and require more testing to be conclusive.” is an analysis.
- ”The methods used in this paper include an in-depth survey and interview session with each candidate.” is a summary.

- If you’re writing a summary for class, your professor may specify how long your summary should be.
- Some summaries can even be as short as one sentence.

- ”Environmental conditions in North Carolina pose a threat to frogs and toads.”

- For example: “According to the climate model, frog and toad populations have been decreasing at a rapid rate over the past 10 years, and are on track to decrease even further in the coming years.”

- For example: “Smith and Herman (2008) argue that by decreasing greenhouse gases, frog and toad populations could reach historical levels within 20 years, and the climate model projections support that statement.”
- You can add in the authors and year of publication at any time during your summary.

- If you have time, try reading your summary to someone who hasn’t read the original paper and see if they understand the key points of the article.
Expert Q&A
- Make sure you fully understand the paper before you start writing the summary. Thanks Helpful 2 Not Helpful 0

- Plagiarism can have serious consequences in the academic world, so make sure you’re writing your summary in your own words. [12] X Research source Thanks Helpful 0 Not Helpful 0
You Might Also Like

- ↑ https://writingcenter.uconn.edu/wp-content/uploads/sites/593/2014/06/How_to_Summarize_a_Research_Article1.pdf
- ↑ https://www.ufv.ca/media/assets/academic-success-centre/handouts/Summarizing-a-Scholarly-Journal-Article-rev2018.pdf
- ↑ https://integrity.mit.edu/handbook/academic-writing/summarizing
- ↑ https://writingcenter.unc.edu/tips-and-tools/summary-using-it-wisely/
- ↑ https://davidson.libguides.com/c.php?g=349327&p=2361763
About This Article

- Send fan mail to authors
Did this article help you?

Featured Articles

Trending Articles

Watch Articles

- Terms of Use
- Privacy Policy
- Do Not Sell or Share My Info
- Not Selling Info
Don’t miss out! Sign up for
wikiHow’s newsletter
Jump to navigation

Cochrane Training
Chapter 14: completing ‘summary of findings’ tables and grading the certainty of the evidence.
Holger J Schünemann, Julian PT Higgins, Gunn E Vist, Paul Glasziou, Elie A Akl, Nicole Skoetz, Gordon H Guyatt; on behalf of the Cochrane GRADEing Methods Group (formerly Applicability and Recommendations Methods Group) and the Cochrane Statistical Methods Group
Key Points:
- A ‘Summary of findings’ table for a given comparison of interventions provides key information concerning the magnitudes of relative and absolute effects of the interventions examined, the amount of available evidence and the certainty (or quality) of available evidence.
- ‘Summary of findings’ tables include a row for each important outcome (up to a maximum of seven). Accepted formats of ‘Summary of findings’ tables and interactive ‘Summary of findings’ tables can be produced using GRADE’s software GRADEpro GDT.
- Cochrane has adopted the GRADE approach (Grading of Recommendations Assessment, Development and Evaluation) for assessing certainty (or quality) of a body of evidence.
- The GRADE approach specifies four levels of the certainty for a body of evidence for a given outcome: high, moderate, low and very low.
- GRADE assessments of certainty are determined through consideration of five domains: risk of bias, inconsistency, indirectness, imprecision and publication bias. For evidence from non-randomized studies and rarely randomized studies, assessments can then be upgraded through consideration of three further domains.
Cite this chapter as: Schünemann HJ, Higgins JPT, Vist GE, Glasziou P, Akl EA, Skoetz N, Guyatt GH. Chapter 14: Completing ‘Summary of findings’ tables and grading the certainty of the evidence. In: Higgins JPT, Thomas J, Chandler J, Cumpston M, Li T, Page MJ, Welch VA (editors). Cochrane Handbook for Systematic Reviews of Interventions version 6.4 (updated August 2023). Cochrane, 2023. Available from www.training.cochrane.org/handbook .
14.1 ‘Summary of findings’ tables
14.1.1 introduction to ‘summary of findings’ tables.
‘Summary of findings’ tables present the main findings of a review in a transparent, structured and simple tabular format. In particular, they provide key information concerning the certainty or quality of evidence (i.e. the confidence or certainty in the range of an effect estimate or an association), the magnitude of effect of the interventions examined, and the sum of available data on the main outcomes. Cochrane Reviews should incorporate ‘Summary of findings’ tables during planning and publication, and should have at least one key ‘Summary of findings’ table representing the most important comparisons. Some reviews may include more than one ‘Summary of findings’ table, for example if the review addresses more than one major comparison, or includes substantially different populations that require separate tables (e.g. because the effects differ or it is important to show results separately). In the Cochrane Database of Systematic Reviews (CDSR), all ‘Summary of findings’ tables for a review appear at the beginning, before the Background section.
14.1.2 Selecting outcomes for ‘Summary of findings’ tables
Planning for the ‘Summary of findings’ table starts early in the systematic review, with the selection of the outcomes to be included in: (i) the review; and (ii) the ‘Summary of findings’ table. This is a crucial step, and one that review authors need to address carefully.
To ensure production of optimally useful information, Cochrane Reviews begin by developing a review question and by listing all main outcomes that are important to patients and other decision makers (see Chapter 2 and Chapter 3 ). The GRADE approach to assessing the certainty of the evidence (see Section 14.2 ) defines and operationalizes a rating process that helps separate outcomes into those that are critical, important or not important for decision making. Consultation and feedback on the review protocol, including from consumers and other decision makers, can enhance this process.
Critical outcomes are likely to include clearly important endpoints; typical examples include mortality and major morbidity (such as strokes and myocardial infarction). However, they may also represent frequent minor and rare major side effects, symptoms, quality of life, burdens associated with treatment, and resource issues (costs). Burdens represent the impact of healthcare workload on patient function and well-being, and include the demands of adhering to an intervention that patients or caregivers (e.g. family) may dislike, such as having to undergo more frequent tests, or the restrictions on lifestyle that certain interventions require (Spencer-Bonilla et al 2017).
Frequently, when formulating questions that include all patient-important outcomes for decision making, review authors will confront reports of studies that have not included all these outcomes. This is particularly true for adverse outcomes. For instance, randomized trials might contribute evidence on intended effects, and on frequent, relatively minor side effects, but not report on rare adverse outcomes such as suicide attempts. Chapter 19 discusses strategies for addressing adverse effects. To obtain data for all important outcomes it may be necessary to examine the results of non-randomized studies (see Chapter 24 ). Cochrane, in collaboration with others, has developed guidance for review authors to support their decision about when to look for and include non-randomized studies (Schünemann et al 2013).
If a review includes only randomized trials, these trials may not address all important outcomes and it may therefore not be possible to address these outcomes within the constraints of the review. Review authors should acknowledge these limitations and make them transparent to readers. Review authors are encouraged to include non-randomized studies to examine rare or long-term adverse effects that may not adequately be studied in randomized trials. This raises the possibility that harm outcomes may come from studies in which participants differ from those in studies used in the analysis of benefit. Review authors will then need to consider how much such differences are likely to impact on the findings, and this will influence the certainty of evidence because of concerns about indirectness related to the population (see Section 14.2.2 ).
Non-randomized studies can provide important information not only when randomized trials do not report on an outcome or randomized trials suffer from indirectness, but also when the evidence from randomized trials is rated as very low and non-randomized studies provide evidence of higher certainty. Further discussion of these issues appears also in Chapter 24 .
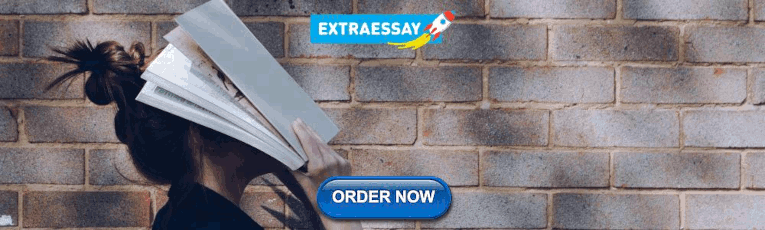
14.1.3 General template for ‘Summary of findings’ tables
Several alternative standard versions of ‘Summary of findings’ tables have been developed to ensure consistency and ease of use across reviews, inclusion of the most important information needed by decision makers, and optimal presentation (see examples at Figures 14.1.a and 14.1.b ). These formats are supported by research that focused on improved understanding of the information they intend to convey (Carrasco-Labra et al 2016, Langendam et al 2016, Santesso et al 2016). They are available through GRADE’s official software package developed to support the GRADE approach: GRADEpro GDT (www.gradepro.org).
Standard Cochrane ‘Summary of findings’ tables include the following elements using one of the accepted formats. Further guidance on each of these is provided in Section 14.1.6 .
- A brief description of the population and setting addressed by the available evidence (which may be slightly different to or narrower than those defined by the review question).
- A brief description of the comparison addressed in the ‘Summary of findings’ table, including both the experimental and comparison interventions.
- A list of the most critical and/or important health outcomes, both desirable and undesirable, limited to seven or fewer outcomes.
- A measure of the typical burden of each outcomes (e.g. illustrative risk, or illustrative mean, on comparator intervention).
- The absolute and relative magnitude of effect measured for each (if both are appropriate).
- The numbers of participants and studies contributing to the analysis of each outcomes.
- A GRADE assessment of the overall certainty of the body of evidence for each outcome (which may vary by outcome).
- Space for comments.
- Explanations (formerly known as footnotes).
Ideally, ‘Summary of findings’ tables are supported by more detailed tables (known as ‘evidence profiles’) to which the review may be linked, which provide more detailed explanations. Evidence profiles include the same important health outcomes, and provide greater detail than ‘Summary of findings’ tables of both of the individual considerations feeding into the grading of certainty and of the results of the studies (Guyatt et al 2011a). They ensure that a structured approach is used to rating the certainty of evidence. Although they are rarely published in Cochrane Reviews, evidence profiles are often used, for example, by guideline developers in considering the certainty of the evidence to support guideline recommendations. Review authors will find it easier to develop the ‘Summary of findings’ table by completing the rating of the certainty of evidence in the evidence profile first in GRADEpro GDT. They can then automatically convert this to one of the ‘Summary of findings’ formats in GRADEpro GDT, including an interactive ‘Summary of findings’ for publication.
As a measure of the magnitude of effect for dichotomous outcomes, the ‘Summary of findings’ table should provide a relative measure of effect (e.g. risk ratio, odds ratio, hazard) and measures of absolute risk. For other types of data, an absolute measure alone (such as a difference in means for continuous data) might be sufficient. It is important that the magnitude of effect is presented in a meaningful way, which may require some transformation of the result of a meta-analysis (see also Chapter 15, Section 15.4 and Section 15.5 ). Reviews with more than one main comparison should include a separate ‘Summary of findings’ table for each comparison.
Figure 14.1.a provides an example of a ‘Summary of findings’ table. Figure 15.1.b provides an alternative format that may further facilitate users’ understanding and interpretation of the review’s findings. Evidence evaluating different formats suggests that the ‘Summary of findings’ table should include a risk difference as a measure of the absolute effect and authors should preferably use a format that includes a risk difference .
A detailed description of the contents of a ‘Summary of findings’ table appears in Section 14.1.6 .
Figure 14.1.a Example of a ‘Summary of findings’ table
Summary of findings (for interactive version click here )
a All the stockings in the nine studies included in this review were below-knee compression stockings. In four studies the compression strength was 20 mmHg to 30 mmHg at the ankle. It was 10 mmHg to 20 mmHg in the other four studies. Stockings come in different sizes. If a stocking is too tight around the knee it can prevent essential venous return causing the blood to pool around the knee. Compression stockings should be fitted properly. A stocking that is too tight could cut into the skin on a long flight and potentially cause ulceration and increased risk of DVT. Some stockings can be slightly thicker than normal leg covering and can be potentially restrictive with tight foot wear. It is a good idea to wear stockings around the house prior to travel to ensure a good, comfortable fit. Participants put their stockings on two to three hours before the flight in most of the studies. The availability and cost of stockings can vary.
b Two studies recruited high risk participants defined as those with previous episodes of DVT, coagulation disorders, severe obesity, limited mobility due to bone or joint problems, neoplastic disease within the previous two years, large varicose veins or, in one of the studies, participants taller than 190 cm and heavier than 90 kg. The incidence for the seven studies that excluded high risk participants was 1.45% and the incidence for the two studies that recruited high-risk participants (with at least one risk factor) was 2.43%. We have used 10 and 30 per 1000 to express different risk strata, respectively.
c The confidence interval crosses no difference and does not rule out a small increase.
d The measurement of oedema was not validated (indirectness of the outcome) or blinded to the intervention (risk of bias).
e If there are very few or no events and the number of participants is large, judgement about the certainty of evidence (particularly judgements about imprecision) may be based on the absolute effect. Here the certainty rating may be considered ‘high’ if the outcome was appropriately assessed and the event, in fact, did not occur in 2821 studied participants.
f None of the other studies reported adverse effects, apart from four cases of superficial vein thrombosis in varicose veins in the knee region that were compressed by the upper edge of the stocking in one study.
Figure 14.1.b Example of alternative ‘Summary of findings’ table
14.1.4 Producing ‘Summary of findings’ tables
The GRADE Working Group’s software, GRADEpro GDT ( www.gradepro.org ), including GRADE’s interactive handbook, is available to assist review authors in the preparation of ‘Summary of findings’ tables. GRADEpro can use data on the comparator group risk and the effect estimate (entered by the review authors or imported from files generated in RevMan) to produce the relative effects and absolute risks associated with experimental interventions. In addition, it leads the user through the process of a GRADE assessment, and produces a table that can be used as a standalone table in a review (including by direct import into software such as RevMan or integration with RevMan Web), or an interactive ‘Summary of findings’ table (see help resources in GRADEpro).
14.1.5 Statistical considerations in ‘Summary of findings’ tables
14.1.5.1 dichotomous outcomes.
‘Summary of findings’ tables should include both absolute and relative measures of effect for dichotomous outcomes. Risk ratios, odds ratios and risk differences are different ways of comparing two groups with dichotomous outcome data (see Chapter 6, Section 6.4.1 ). Furthermore, there are two distinct risk ratios, depending on which event (e.g. ‘yes’ or ‘no’) is the focus of the analysis (see Chapter 6, Section 6.4.1.5 ). In the presence of a non-zero intervention effect, any variation across studies in the comparator group risks (i.e. variation in the risk of the event occurring without the intervention of interest, for example in different populations) makes it impossible for more than one of these measures to be truly the same in every study.
It has long been assumed in epidemiology that relative measures of effect are more consistent than absolute measures of effect from one scenario to another. There is empirical evidence to support this assumption (Engels et al 2000, Deeks and Altman 2001, Furukawa et al 2002). For this reason, meta-analyses should generally use either a risk ratio or an odds ratio as a measure of effect (see Chapter 10, Section 10.4.3 ). Correspondingly, a single estimate of relative effect is likely to be a more appropriate summary than a single estimate of absolute effect. If a relative effect is indeed consistent across studies, then different comparator group risks will have different implications for absolute benefit. For instance, if the risk ratio is consistently 0.75, then the experimental intervention would reduce a comparator group risk of 80% to 60% in the intervention group (an absolute risk reduction of 20 percentage points), but would also reduce a comparator group risk of 20% to 15% in the intervention group (an absolute risk reduction of 5 percentage points).
‘Summary of findings’ tables are built around the assumption of a consistent relative effect. It is therefore important to consider the implications of this effect for different comparator group risks (these can be derived or estimated from a number of sources, see Section 14.1.6.3 ), which may require an assessment of the certainty of evidence for prognostic evidence (Spencer et al 2012, Iorio et al 2015). For any comparator group risk, it is possible to estimate a corresponding intervention group risk (i.e. the absolute risk with the intervention) from the meta-analytic risk ratio or odds ratio. Note that the numbers provided in the ‘Corresponding risk’ column are specific to the ‘risks’ in the adjacent column.
For the meta-analytic risk ratio (RR) and assumed comparator risk (ACR) the corresponding intervention risk is obtained as:

As an example, in Figure 14.1.a , the meta-analytic risk ratio for symptomless deep vein thrombosis (DVT) is RR = 0.10 (95% CI 0.04 to 0.26). Assuming a comparator risk of ACR = 10 per 1000 = 0.01, we obtain:

For the meta-analytic odds ratio (OR) and assumed comparator risk, ACR, the corresponding intervention risk is obtained as:

Upper and lower confidence limits for the corresponding intervention risk are obtained by replacing RR or OR by their upper and lower confidence limits, respectively (e.g. replacing 0.10 with 0.04, then with 0.26, in the example). Such confidence intervals do not incorporate uncertainty in the assumed comparator risks.
When dealing with risk ratios, it is critical that the same definition of ‘event’ is used as was used for the meta-analysis. For example, if the meta-analysis focused on ‘death’ (as opposed to survival) as the event, then corresponding risks in the ‘Summary of findings’ table must also refer to ‘death’.
In (rare) circumstances in which there is clear rationale to assume a consistent risk difference in the meta-analysis, in principle it is possible to present this for relevant ‘assumed risks’ and their corresponding risks, and to present the corresponding (different) relative effects for each assumed risk.
The risk difference expresses the difference between the ACR and the corresponding intervention risk (or the difference between the experimental and the comparator intervention).
For the meta-analytic risk ratio (RR) and assumed comparator risk (ACR) the corresponding risk difference is obtained as (note that risks can also be expressed using percentage or percentage points):

As an example, in Figure 14.1.b the meta-analytic risk ratio is 0.41 (95% CI 0.29 to 0.55) for diarrhoea in children less than 5 years of age. Assuming a comparator group risk of 22.3% we obtain:

For the meta-analytic odds ratio (OR) and assumed comparator risk (ACR) the absolute risk difference is obtained as (percentage points):

Upper and lower confidence limits for the absolute risk difference are obtained by re-running the calculation above while replacing RR or OR by their upper and lower confidence limits, respectively (e.g. replacing 0.41 with 0.28, then with 0.55, in the example). Such confidence intervals do not incorporate uncertainty in the assumed comparator risks.
14.1.5.2 Time-to-event outcomes
Time-to-event outcomes measure whether and when a particular event (e.g. death) occurs (van Dalen et al 2007). The impact of the experimental intervention relative to the comparison group on time-to-event outcomes is usually measured using a hazard ratio (HR) (see Chapter 6, Section 6.8.1 ).
A hazard ratio expresses a relative effect estimate. It may be used in various ways to obtain absolute risks and other interpretable quantities for a specific population. Here we describe how to re-express hazard ratios in terms of: (i) absolute risk of event-free survival within a particular period of time; (ii) absolute risk of an event within a particular period of time; and (iii) median time to the event. All methods are built on an assumption of consistent relative effects (i.e. that the hazard ratio does not vary over time).
(i) Absolute risk of event-free survival within a particular period of time Event-free survival (e.g. overall survival) is commonly reported by individual studies. To obtain absolute effects for time-to-event outcomes measured as event-free survival, the summary HR can be used in conjunction with an assumed proportion of patients who are event-free in the comparator group (Tierney et al 2007). This proportion of patients will be specific to a period of time of observation. However, it is not strictly necessary to specify this period of time. For instance, a proportion of 50% of event-free patients might apply to patients with a high event rate observed over 1 year, or to patients with a low event rate observed over 2 years.

As an example, suppose the meta-analytic hazard ratio is 0.42 (95% CI 0.25 to 0.72). Assuming a comparator group risk of event-free survival (e.g. for overall survival people being alive) at 2 years of ACR = 900 per 1000 = 0.9 we obtain:

so that that 956 per 1000 people will be alive with the experimental intervention at 2 years. The derivation of the risk should be explained in a comment or footnote.
(ii) Absolute risk of an event within a particular period of time To obtain this absolute effect, again the summary HR can be used (Tierney et al 2007):

In the example, suppose we assume a comparator group risk of events (e.g. for mortality, people being dead) at 2 years of ACR = 100 per 1000 = 0.1. We obtain:

so that that 44 per 1000 people will be dead with the experimental intervention at 2 years.
(iii) Median time to the event Instead of absolute numbers, the time to the event in the intervention and comparison groups can be expressed as median survival time in months or years. To obtain median survival time the pooled HR can be applied to an assumed median survival time in the comparator group (Tierney et al 2007):

In the example, assuming a comparator group median survival time of 80 months, we obtain:

For all three of these options for re-expressing results of time-to-event analyses, upper and lower confidence limits for the corresponding intervention risk are obtained by replacing HR by its upper and lower confidence limits, respectively (e.g. replacing 0.42 with 0.25, then with 0.72, in the example). Again, as for dichotomous outcomes, such confidence intervals do not incorporate uncertainty in the assumed comparator group risks. This is of special concern for long-term survival with a low or moderate mortality rate and a corresponding high number of censored patients (i.e. a low number of patients under risk and a high censoring rate).
14.1.6 Detailed contents of a ‘Summary of findings’ table
14.1.6.1 table title and header.
The title of each ‘Summary of findings’ table should specify the healthcare question, framed in terms of the population and making it clear exactly what comparison of interventions are made. In Figure 14.1.a , the population is people taking long aeroplane flights, the intervention is compression stockings, and the control is no compression stockings.
The first rows of each ‘Summary of findings’ table should provide the following ‘header’ information:
Patients or population This further clarifies the population (and possibly the subpopulations) of interest and ideally the magnitude of risk of the most crucial adverse outcome at which an intervention is directed. For instance, people on a long-haul flight may be at different risks for DVT; those using selective serotonin reuptake inhibitors (SSRIs) might be at different risk for side effects; while those with atrial fibrillation may be at low (< 1%), moderate (1% to 4%) or high (> 4%) yearly risk of stroke.
Setting This should state any specific characteristics of the settings of the healthcare question that might limit the applicability of the summary of findings to other settings (e.g. primary care in Europe and North America).
Intervention The experimental intervention.
Comparison The comparator intervention (including no specific intervention).
14.1.6.2 Outcomes
The rows of a ‘Summary of findings’ table should include all desirable and undesirable health outcomes (listed in order of importance) that are essential for decision making, up to a maximum of seven outcomes. If there are more outcomes in the review, review authors will need to omit the less important outcomes from the table, and the decision selecting which outcomes are critical or important to the review should be made during protocol development (see Chapter 3 ). Review authors should provide time frames for the measurement of the outcomes (e.g. 90 days or 12 months) and the type of instrument scores (e.g. ranging from 0 to 100).
Note that review authors should include the pre-specified critical and important outcomes in the table whether data are available or not. However, they should be alert to the possibility that the importance of an outcome (e.g. a serious adverse effect) may only become known after the protocol was written or the analysis was carried out, and should take appropriate actions to include these in the ‘Summary of findings’ table.
The ‘Summary of findings’ table can include effects in subgroups of the population for different comparator risks and effect sizes separately. For instance, in Figure 14.1.b effects are presented for children younger and older than 5 years separately. Review authors may also opt to produce separate ‘Summary of findings’ tables for different populations.
Review authors should include serious adverse events, but it might be possible to combine minor adverse events as a single outcome, and describe this in an explanatory footnote (note that it is not appropriate to add events together unless they are independent, that is, a participant who has experienced one adverse event has an unaffected chance of experiencing the other adverse event).
Outcomes measured at multiple time points represent a particular problem. In general, to keep the table simple, review authors should present multiple time points only for outcomes critical to decision making, where either the result or the decision made are likely to vary over time. The remainder should be presented at a common time point where possible.
Review authors can present continuous outcome measures in the ‘Summary of findings’ table and should endeavour to make these interpretable to the target audience. This requires that the units are clear and readily interpretable, for example, days of pain, or frequency of headache, and the name and scale of any measurement tools used should be stated (e.g. a Visual Analogue Scale, ranging from 0 to 100). However, many measurement instruments are not readily interpretable by non-specialist clinicians or patients, for example, points on a Beck Depression Inventory or quality of life score. For these, a more interpretable presentation might involve converting a continuous to a dichotomous outcome, such as >50% improvement (see Chapter 15, Section 15.5 ).
14.1.6.3 Best estimate of risk with comparator intervention
Review authors should provide up to three typical risks for participants receiving the comparator intervention. For dichotomous outcomes, we recommend that these be presented in the form of the number of people experiencing the event per 100 or 1000 people (natural frequency) depending on the frequency of the outcome. For continuous outcomes, this would be stated as a mean or median value of the outcome measured.
Estimated or assumed comparator intervention risks could be based on assessments of typical risks in different patient groups derived from the review itself, individual representative studies in the review, or risks derived from a systematic review of prognosis studies or other sources of evidence which may in turn require an assessment of the certainty for the prognostic evidence (Spencer et al 2012, Iorio et al 2015). Ideally, risks would reflect groups that clinicians can easily identify on the basis of their presenting features.
An explanatory footnote should specify the source or rationale for each comparator group risk, including the time period to which it corresponds where appropriate. In Figure 14.1.a , clinicians can easily differentiate individuals with risk factors for deep venous thrombosis from those without. If there is known to be little variation in baseline risk then review authors may use the median comparator group risk across studies. If typical risks are not known, an option is to choose the risk from the included studies, providing the second highest for a high and the second lowest for a low risk population.
14.1.6.4 Risk with intervention
For dichotomous outcomes, review authors should provide a corresponding absolute risk for each comparator group risk, along with a confidence interval. This absolute risk with the (experimental) intervention will usually be derived from the meta-analysis result presented in the relative effect column (see Section 14.1.6.6 ). Formulae are provided in Section 14.1.5 . Review authors should present the absolute effect in the same format as the risks with comparator intervention (see Section 14.1.6.3 ), for example as the number of people experiencing the event per 1000 people.
For continuous outcomes, a difference in means or standardized difference in means should be presented with its confidence interval. These will typically be obtained directly from a meta-analysis. Explanatory text should be used to clarify the meaning, as in Figures 14.1.a and 14.1.b .
14.1.6.5 Risk difference
For dichotomous outcomes, the risk difference can be provided using one of the ‘Summary of findings’ table formats as an additional option (see Figure 14.1.b ). This risk difference expresses the difference between the experimental and comparator intervention and will usually be derived from the meta-analysis result presented in the relative effect column (see Section 14.1.6.6 ). Formulae are provided in Section 14.1.5 . Review authors should present the risk difference in the same format as assumed and corresponding risks with comparator intervention (see Section 14.1.6.3 ); for example, as the number of people experiencing the event per 1000 people or as percentage points if the assumed and corresponding risks are expressed in percentage.
For continuous outcomes, if the ‘Summary of findings’ table includes this option, the mean difference can be presented here and the ‘corresponding risk’ column left blank (see Figure 14.1.b ).
14.1.6.6 Relative effect (95% CI)
The relative effect will typically be a risk ratio or odds ratio (or occasionally a hazard ratio) with its accompanying 95% confidence interval, obtained from a meta-analysis performed on the basis of the same effect measure. Risk ratios and odds ratios are similar when the comparator intervention risks are low and effects are small, but may differ considerably when comparator group risks increase. The meta-analysis may involve an assumption of either fixed or random effects, depending on what the review authors consider appropriate, and implying that the relative effect is either an estimate of the effect of the intervention, or an estimate of the average effect of the intervention across studies, respectively.
14.1.6.7 Number of participants (studies)
This column should include the number of participants assessed in the included studies for each outcome and the corresponding number of studies that contributed these participants.
14.1.6.8 Certainty of the evidence (GRADE)
Review authors should comment on the certainty of the evidence (also known as quality of the body of evidence or confidence in the effect estimates). Review authors should use the specific evidence grading system developed by the GRADE Working Group (Atkins et al 2004, Guyatt et al 2008, Guyatt et al 2011a), which is described in detail in Section 14.2 . The GRADE approach categorizes the certainty in a body of evidence as ‘high’, ‘moderate’, ‘low’ or ‘very low’ by outcome. This is a result of judgement, but the judgement process operates within a transparent structure. As an example, the certainty would be ‘high’ if the summary were of several randomized trials with low risk of bias, but the rating of certainty becomes lower if there are concerns about risk of bias, inconsistency, indirectness, imprecision or publication bias. Judgements other than of ‘high’ certainty should be made transparent using explanatory footnotes or the ‘Comments’ column in the ‘Summary of findings’ table (see Section 14.1.6.10 ).
14.1.6.9 Comments
The aim of the ‘Comments’ field is to help interpret the information or data identified in the row. For example, this may be on the validity of the outcome measure or the presence of variables that are associated with the magnitude of effect. Important caveats about the results should be flagged here. Not all rows will need comments, and it is best to leave a blank if there is nothing warranting a comment.
14.1.6.10 Explanations
Detailed explanations should be included as footnotes to support the judgements in the ‘Summary of findings’ table, such as the overall GRADE assessment. The explanations should describe the rationale for important aspects of the content. Table 14.1.a lists guidance for useful explanations. Explanations should be concise, informative, relevant, easy to understand and accurate. If explanations cannot be sufficiently described in footnotes, review authors should provide further details of the issues in the Results and Discussion sections of the review.
Table 14.1.a Guidance for providing useful explanations in ‘Summary of findings’ (SoF) tables. Adapted from Santesso et al (2016)
14.2 Assessing the certainty or quality of a body of evidence
14.2.1 the grade approach.
The Grades of Recommendation, Assessment, Development and Evaluation Working Group (GRADE Working Group) has developed a system for grading the certainty of evidence (Schünemann et al 2003, Atkins et al 2004, Schünemann et al 2006, Guyatt et al 2008, Guyatt et al 2011a). Over 100 organizations including the World Health Organization (WHO), the American College of Physicians, the American Society of Hematology (ASH), the Canadian Agency for Drugs and Technology in Health (CADTH) and the National Institutes of Health and Clinical Excellence (NICE) in the UK have adopted the GRADE system ( www.gradeworkinggroup.org ).
Cochrane has also formally adopted this approach, and all Cochrane Reviews should use GRADE to evaluate the certainty of evidence for important outcomes (see MECIR Box 14.2.a ).
MECIR Box 14.2.a Relevant expectations for conduct of intervention reviews
For systematic reviews, the GRADE approach defines the certainty of a body of evidence as the extent to which one can be confident that an estimate of effect or association is close to the quantity of specific interest. Assessing the certainty of a body of evidence involves consideration of within- and across-study risk of bias (limitations in study design and execution or methodological quality), inconsistency (or heterogeneity), indirectness of evidence, imprecision of the effect estimates and risk of publication bias (see Section 14.2.2 ), as well as domains that may increase our confidence in the effect estimate (as described in Section 14.2.3 ). The GRADE system entails an assessment of the certainty of a body of evidence for each individual outcome. Judgements about the domains that determine the certainty of evidence should be described in the results or discussion section and as part of the ‘Summary of findings’ table.
The GRADE approach specifies four levels of certainty ( Figure 14.2.a ). For interventions, including diagnostic and other tests that are evaluated as interventions (Schünemann et al 2008b, Schünemann et al 2008a, Balshem et al 2011, Schünemann et al 2012), the starting point for rating the certainty of evidence is categorized into two types:
- randomized trials; and
- non-randomized studies of interventions (NRSI), including observational studies (including but not limited to cohort studies, and case-control studies, cross-sectional studies, case series and case reports, although not all of these designs are usually included in Cochrane Reviews).
There are many instances in which review authors rely on information from NRSI, in particular to evaluate potential harms (see Chapter 24 ). In addition, review authors can obtain relevant data from both randomized trials and NRSI, with each type of evidence complementing the other (Schünemann et al 2013).
In GRADE, a body of evidence from randomized trials begins with a high-certainty rating while a body of evidence from NRSI begins with a low-certainty rating. The lower rating with NRSI is the result of the potential bias induced by the lack of randomization (i.e. confounding and selection bias).
However, when using the new Risk Of Bias In Non-randomized Studies of Interventions (ROBINS-I) tool (Sterne et al 2016), an assessment tool that covers the risk of bias due to lack of randomization, all studies may start as high certainty of the evidence (Schünemann et al 2018). The approach of starting all study designs (including NRSI) as high certainty does not conflict with the initial GRADE approach of starting the rating of NRSI as low certainty evidence. This is because a body of evidence from NRSI should generally be downgraded by two levels due to the inherent risk of bias associated with the lack of randomization, namely confounding and selection bias. Not downgrading NRSI from high to low certainty needs transparent and detailed justification for what mitigates concerns about confounding and selection bias (Schünemann et al 2018). Very few examples of where not rating down by two levels is appropriate currently exist.
The highest certainty rating is a body of evidence when there are no concerns in any of the GRADE factors listed in Figure 14.2.a . Review authors often downgrade evidence to moderate, low or even very low certainty evidence, depending on the presence of the five factors in Figure 14.2.a . Usually, certainty rating will fall by one level for each factor, up to a maximum of three levels for all factors. If there are very severe problems for any one domain (e.g. when assessing risk of bias, all studies were unconcealed, unblinded and lost over 50% of their patients to follow-up), evidence may fall by two levels due to that factor alone. It is not possible to rate lower than ‘very low certainty’ evidence.
Review authors will generally grade evidence from sound non-randomized studies as low certainty, even if ROBINS-I is used. If, however, such studies yield large effects and there is no obvious bias explaining those effects, review authors may rate the evidence as moderate or – if the effect is large enough – even as high certainty ( Figure 14.2.a ). The very low certainty level is appropriate for, but is not limited to, studies with critical problems and unsystematic clinical observations (e.g. case series or case reports).
Figure 14.2.a Levels of the certainty of a body of evidence in the GRADE approach. *Upgrading criteria are usually applicable to non-randomized studies only (but exceptions exist).
14.2.2 Domains that can lead to decreasing the certainty level of a body of evidence
We now describe in more detail the five reasons (or domains) for downgrading the certainty of a body of evidence for a specific outcome. In each case, if no reason is found for downgrading the evidence, it should be classified as 'no limitation or not serious' (not important enough to warrant downgrading). If a reason is found for downgrading the evidence, it should be classified as 'serious' (downgrading the certainty rating by one level) or 'very serious' (downgrading the certainty grade by two levels). For non-randomized studies assessed with ROBINS-I, rating down by three levels should be classified as 'extremely' serious.
(1) Risk of bias or limitations in the detailed design and implementation
Our confidence in an estimate of effect decreases if studies suffer from major limitations that are likely to result in a biased assessment of the intervention effect. For randomized trials, these methodological limitations include failure to generate a random sequence, lack of allocation sequence concealment, lack of blinding (particularly with subjective outcomes that are highly susceptible to biased assessment), a large loss to follow-up or selective reporting of outcomes. Chapter 8 provides a discussion of study-level assessments of risk of bias in the context of a Cochrane Review, and proposes an approach to assessing the risk of bias for an outcome across studies as ‘Low’ risk of bias, ‘Some concerns’ and ‘High’ risk of bias for randomized trials. Levels of ‘Low’. ‘Moderate’, ‘Serious’ and ‘Critical’ risk of bias arise for non-randomized studies assessed with ROBINS-I ( Chapter 25 ). These assessments should feed directly into this GRADE domain. In particular, ‘Low’ risk of bias would indicate ‘no limitation’; ‘Some concerns’ would indicate either ‘no limitation’ or ‘serious limitation’; and ‘High’ risk of bias would indicate either ‘serious limitation’ or ‘very serious limitation’. ‘Critical’ risk of bias on ROBINS-I would indicate extremely serious limitations in GRADE. Review authors should use their judgement to decide between alternative categories, depending on the likely magnitude of the potential biases.
Every study addressing a particular outcome will differ, to some degree, in the risk of bias. Review authors should make an overall judgement on whether the certainty of evidence for an outcome warrants downgrading on the basis of study limitations. The assessment of study limitations should apply to the studies contributing to the results in the ‘Summary of findings’ table, rather than to all studies that could potentially be included in the analysis. We have argued in Chapter 7, Section 7.6.2 , that the primary analysis should be restricted to studies at low (or low and unclear) risk of bias where possible.
Table 14.2.a presents the judgements that must be made in going from assessments of the risk of bias to judgements about study limitations for each outcome included in a ‘Summary of findings’ table. A rating of high certainty evidence can be achieved only when most evidence comes from studies that met the criteria for low risk of bias. For example, of the 22 studies addressing the impact of beta-blockers on mortality in patients with heart failure, most probably or certainly used concealed allocation of the sequence, all blinded at least some key groups and follow-up of randomized patients was almost complete (Brophy et al 2001). The certainty of evidence might be downgraded by one level when most of the evidence comes from individual studies either with a crucial limitation for one item, or with some limitations for multiple items. An example of very serious limitations, warranting downgrading by two levels, is provided by evidence on surgery versus conservative treatment in the management of patients with lumbar disc prolapse (Gibson and Waddell 2007). We are uncertain of the benefit of surgery in reducing symptoms after one year or longer, because the one study included in the analysis had inadequate concealment of the allocation sequence and the outcome was assessed using a crude rating by the surgeon without blinding.
(2) Unexplained heterogeneity or inconsistency of results
When studies yield widely differing estimates of effect (heterogeneity or variability in results), investigators should look for robust explanations for that heterogeneity. For instance, drugs may have larger relative effects in sicker populations or when given in larger doses. A detailed discussion of heterogeneity and its investigation is provided in Chapter 10, Section 10.10 and Section 10.11 . If an important modifier exists, with good evidence that important outcomes are different in different subgroups (which would ideally be pre-specified), then a separate ‘Summary of findings’ table may be considered for a separate population. For instance, a separate ‘Summary of findings’ table would be used for carotid endarterectomy in symptomatic patients with high grade stenosis (70% to 99%) in which the intervention is, in the hands of the right surgeons, beneficial, and another (if review authors considered it relevant) for asymptomatic patients with low grade stenosis (less than 30%) in which surgery appears harmful (Orrapin and Rerkasem 2017). When heterogeneity exists and affects the interpretation of results, but review authors are unable to identify a plausible explanation with the data available, the certainty of the evidence decreases.
(3) Indirectness of evidence
Two types of indirectness are relevant. First, a review comparing the effectiveness of alternative interventions (say A and B) may find that randomized trials are available, but they have compared A with placebo and B with placebo. Thus, the evidence is restricted to indirect comparisons between A and B. Where indirect comparisons are undertaken within a network meta-analysis context, GRADE for network meta-analysis should be used (see Chapter 11, Section 11.5 ).
Second, a review may find randomized trials that meet eligibility criteria but address a restricted version of the main review question in terms of population, intervention, comparator or outcomes. For example, suppose that in a review addressing an intervention for secondary prevention of coronary heart disease, most identified studies happened to be in people who also had diabetes. Then the evidence may be regarded as indirect in relation to the broader question of interest because the population is primarily related to people with diabetes. The opposite scenario can equally apply: a review addressing the effect of a preventive strategy for coronary heart disease in people with diabetes may consider studies in people without diabetes to provide relevant, albeit indirect, evidence. This would be particularly likely if investigators had conducted few if any randomized trials in the target population (e.g. people with diabetes). Other sources of indirectness may arise from interventions studied (e.g. if in all included studies a technical intervention was implemented by expert, highly trained specialists in specialist centres, then evidence on the effects of the intervention outside these centres may be indirect), comparators used (e.g. if the comparator groups received an intervention that is less effective than standard treatment in most settings) and outcomes assessed (e.g. indirectness due to surrogate outcomes when data on patient-important outcomes are not available, or when investigators seek data on quality of life but only symptoms are reported). Review authors should make judgements transparent when they believe downgrading is justified, based on differences in anticipated effects in the group of primary interest. Review authors may be aided and increase transparency of their judgements about indirectness if they use Table 14.2.b available in the GRADEpro GDT software (Schünemann et al 2013).
(4) Imprecision of results
When studies include few participants or few events, and thus have wide confidence intervals, review authors can lower their rating of the certainty of the evidence. The confidence intervals included in the ‘Summary of findings’ table will provide readers with information that allows them to make, to some extent, their own rating of precision. Review authors can use a calculation of the optimal information size (OIS) or review information size (RIS), similar to sample size calculations, to make judgements about imprecision (Guyatt et al 2011b, Schünemann 2016). The OIS or RIS is calculated on the basis of the number of participants required for an adequately powered individual study. If the 95% confidence interval excludes a risk ratio (RR) of 1.0, and the total number of events or patients exceeds the OIS criterion, precision is adequate. If the 95% CI includes appreciable benefit or harm (an RR of under 0.75 or over 1.25 is often suggested as a very rough guide) downgrading for imprecision may be appropriate even if OIS criteria are met (Guyatt et al 2011b, Schünemann 2016).
(5) High probability of publication bias
The certainty of evidence level may be downgraded if investigators fail to report studies on the basis of results (typically those that show no effect: publication bias) or outcomes (typically those that may be harmful or for which no effect was observed: selective outcome non-reporting bias). Selective reporting of outcomes from among multiple outcomes measured is assessed at the study level as part of the assessment of risk of bias (see Chapter 8, Section 8.7 ), so for the studies contributing to the outcome in the ‘Summary of findings’ table this is addressed by domain 1 above (limitations in the design and implementation). If a large number of studies included in the review do not contribute to an outcome, or if there is evidence of publication bias, the certainty of the evidence may be downgraded. Chapter 13 provides a detailed discussion of reporting biases, including publication bias, and how it may be tackled in a Cochrane Review. A prototypical situation that may elicit suspicion of publication bias is when published evidence includes a number of small studies, all of which are industry-funded (Bhandari et al 2004). For example, 14 studies of flavanoids in patients with haemorrhoids have shown apparent large benefits, but enrolled a total of only 1432 patients (i.e. each study enrolled relatively few patients) (Alonso-Coello et al 2006). The heavy involvement of sponsors in most of these studies raises questions of whether unpublished studies that suggest no benefit exist (publication bias).
A particular body of evidence can suffer from problems associated with more than one of the five factors listed here, and the greater the problems, the lower the certainty of evidence rating that should result. One could imagine a situation in which randomized trials were available, but all or virtually all of these limitations would be present, and in serious form. A very low certainty of evidence rating would result.
Table 14.2.a Further guidelines for domain 1 (of 5) in a GRADE assessment: going from assessments of risk of bias in studies to judgements about study limitations for main outcomes across studies
Table 14.2.b Judgements about indirectness by outcome (available in GRADEpro GDT)
Intervention:
Comparator:
Direct comparison:
Final judgement about indirectness across domains:
14.2.3 Domains that may lead to increasing the certainty level of a body of evidence
Although NRSI and downgraded randomized trials will generally yield a low rating for certainty of evidence, there will be unusual circumstances in which review authors could ‘upgrade’ such evidence to moderate or even high certainty ( Table 14.3.a ).
- Large effects On rare occasions when methodologically well-done observational studies yield large, consistent and precise estimates of the magnitude of an intervention effect, one may be particularly confident in the results. A large estimated effect (e.g. RR >2 or RR <0.5) in the absence of plausible confounders, or a very large effect (e.g. RR >5 or RR <0.2) in studies with no major threats to validity, might qualify for this. In these situations, while the NRSI may possibly have provided an over-estimate of the true effect, the weak study design may not explain all of the apparent observed benefit. Thus, despite reservations based on the observational study design, review authors are confident that the effect exists. The magnitude of the effect in these studies may move the assigned certainty of evidence from low to moderate (if the effect is large in the absence of other methodological limitations). For example, a meta-analysis of observational studies showed that bicycle helmets reduce the risk of head injuries in cyclists by a large margin (odds ratio (OR) 0.31, 95% CI 0.26 to 0.37) (Thompson et al 2000). This large effect, in the absence of obvious bias that could create the association, suggests a rating of moderate-certainty evidence. Note : GRADE guidance suggests the possibility of rating up one level for a large effect if the relative effect is greater than 2.0. However, if the point estimate of the relative effect is greater than 2.0, but the confidence interval is appreciably below 2.0, then some hesitation would be appropriate in the decision to rate up for a large effect. Another situation allows inference of a strong association without a formal comparative study. Consider the question of the impact of routine colonoscopy versus no screening for colon cancer on the rate of perforation associated with colonoscopy. Here, a large series of representative patients undergoing colonoscopy may provide high certainty evidence about the risk of perforation associated with colonoscopy. When the risk of the event among patients receiving the relevant comparator is known to be near 0 (i.e. we are certain that the incidence of spontaneous colon perforation in patients not undergoing colonoscopy is extremely low), case series or cohort studies of representative patients can provide high certainty evidence of adverse effects associated with an intervention, thereby allowing us to infer a strong association from even a limited number of events.
- Dose-response The presence of a dose-response gradient may increase our confidence in the findings of observational studies and thereby enhance the assigned certainty of evidence. For example, our confidence in the result of observational studies that show an increased risk of bleeding in patients who have supratherapeutic anticoagulation levels is increased by the observation that there is a dose-response gradient between the length of time needed for blood to clot (as measured by the international normalized ratio (INR)) and an increased risk of bleeding (Levine et al 2004). A systematic review of NRSI investigating the effect of cyclooxygenase-2 inhibitors on cardiovascular events found that the summary estimate (RR) with rofecoxib was 1.33 (95% CI 1.00 to 1.79) with doses less than 25mg/d, and 2.19 (95% CI 1.64 to 2.91) with doses more than 25mg/d. Although residual confounding is likely to exist in the NRSI that address this issue, the existence of a dose-response gradient and the large apparent effect of higher doses of rofecoxib markedly increase our strength of inference that the association cannot be explained by residual confounding, and is therefore likely to be both causal and, at high levels of exposure, substantial. Note : GRADE guidance suggests the possibility of rating up one level for a large effect if the relative effect is greater than 2.0. Here, the fact that the point estimate of the relative effect is greater than 2.0, but the confidence interval is appreciably below 2.0 might make some hesitate in the decision to rate up for a large effect
- Plausible confounding On occasion, all plausible biases from randomized or non-randomized studies may be working to under-estimate an apparent intervention effect. For example, if only sicker patients receive an experimental intervention or exposure, yet they still fare better, it is likely that the actual intervention or exposure effect is larger than the data suggest. For instance, a rigorous systematic review of observational studies including a total of 38 million patients demonstrated higher death rates in private for-profit versus private not-for-profit hospitals (Devereaux et al 2002). One possible bias relates to different disease severity in patients in the two hospital types. It is likely, however, that patients in the not-for-profit hospitals were sicker than those in the for-profit hospitals. Thus, to the extent that residual confounding existed, it would bias results against the not-for-profit hospitals. The second likely bias was the possibility that higher numbers of patients with excellent private insurance coverage could lead to a hospital having more resources and a spill-over effect that would benefit those without such coverage. Since for-profit hospitals are likely to admit a larger proportion of such well-insured patients than not-for-profit hospitals, the bias is once again against the not-for-profit hospitals. Since the plausible biases would all diminish the demonstrated intervention effect, one might consider the evidence from these observational studies as moderate rather than low certainty. A parallel situation exists when observational studies have failed to demonstrate an association, but all plausible biases would have increased an intervention effect. This situation will usually arise in the exploration of apparent harmful effects. For example, because the hypoglycaemic drug phenformin causes lactic acidosis, the related agent metformin was under suspicion for the same toxicity. Nevertheless, very large observational studies have failed to demonstrate an association (Salpeter et al 2007). Given the likelihood that clinicians would be more alert to lactic acidosis in the presence of the agent and over-report its occurrence, one might consider this moderate, or even high certainty, evidence refuting a causal relationship between typical therapeutic doses of metformin and lactic acidosis.
14.3 Describing the assessment of the certainty of a body of evidence using the GRADE framework
Review authors should report the grading of the certainty of evidence in the Results section for each outcome for which this has been performed, providing the rationale for downgrading or upgrading the evidence, and referring to the ‘Summary of findings’ table where applicable.
Table 14.3.a provides a framework and examples for how review authors can justify their judgements about the certainty of evidence in each domain. These justifications should also be included in explanatory notes to the ‘Summary of Findings’ table (see Section 14.1.6.10 ).
Chapter 15, Section 15.6 , describes in more detail how the overall GRADE assessment across all domains can be used to draw conclusions about the effects of the intervention, as well as providing implications for future research.
Table 14.3.a Framework for describing the certainty of evidence and justifying downgrading or upgrading
14.4 Chapter information
Authors: Holger J Schünemann, Julian PT Higgins, Gunn E Vist, Paul Glasziou, Elie A Akl, Nicole Skoetz, Gordon H Guyatt; on behalf of the Cochrane GRADEing Methods Group (formerly Applicability and Recommendations Methods Group) and the Cochrane Statistical Methods Group
Acknowledgements: Andrew D Oxman contributed to earlier versions. Professor Penny Hawe contributed to the text on adverse effects in earlier versions. Jon Deeks provided helpful contributions on an earlier version of this chapter. For details of previous authors and editors of the Handbook , please refer to the Preface.
Funding: This work was in part supported by funding from the Michael G DeGroote Cochrane Canada Centre and the Ontario Ministry of Health.
14.5 References
Alonso-Coello P, Zhou Q, Martinez-Zapata MJ, Mills E, Heels-Ansdell D, Johanson JF, Guyatt G. Meta-analysis of flavonoids for the treatment of haemorrhoids. British Journal of Surgery 2006; 93 : 909-920.
Atkins D, Best D, Briss PA, Eccles M, Falck-Ytter Y, Flottorp S, Guyatt GH, Harbour RT, Haugh MC, Henry D, Hill S, Jaeschke R, Leng G, Liberati A, Magrini N, Mason J, Middleton P, Mrukowicz J, O'Connell D, Oxman AD, Phillips B, Schünemann HJ, Edejer TT, Varonen H, Vist GE, Williams JW, Jr., Zaza S. Grading quality of evidence and strength of recommendations. BMJ 2004; 328 : 1490.
Balshem H, Helfand M, Schünemann HJ, Oxman AD, Kunz R, Brozek J, Vist GE, Falck-Ytter Y, Meerpohl J, Norris S, Guyatt GH. GRADE guidelines: 3. Rating the quality of evidence. Journal of Clinical Epidemiology 2011; 64 : 401-406.
Bhandari M, Busse JW, Jackowski D, Montori VM, Schünemann H, Sprague S, Mears D, Schemitsch EH, Heels-Ansdell D, Devereaux PJ. Association between industry funding and statistically significant pro-industry findings in medical and surgical randomized trials. Canadian Medical Association Journal 2004; 170 : 477-480.
Brophy JM, Joseph L, Rouleau JL. Beta-blockers in congestive heart failure. A Bayesian meta-analysis. Annals of Internal Medicine 2001; 134 : 550-560.
Carrasco-Labra A, Brignardello-Petersen R, Santesso N, Neumann I, Mustafa RA, Mbuagbaw L, Etxeandia Ikobaltzeta I, De Stio C, McCullagh LJ, Alonso-Coello P, Meerpohl JJ, Vandvik PO, Brozek JL, Akl EA, Bossuyt P, Churchill R, Glenton C, Rosenbaum S, Tugwell P, Welch V, Garner P, Guyatt G, Schünemann HJ. Improving GRADE evidence tables part 1: a randomized trial shows improved understanding of content in summary of findings tables with a new format. Journal of Clinical Epidemiology 2016; 74 : 7-18.
Deeks JJ, Altman DG. Effect measures for meta-analysis of trials with binary outcomes. In: Egger M, Davey Smith G, Altman DG, editors. Systematic Reviews in Health Care: Meta-analysis in Context . 2nd ed. London (UK): BMJ Publication Group; 2001. p. 313-335.
Devereaux PJ, Choi PT, Lacchetti C, Weaver B, Schünemann HJ, Haines T, Lavis JN, Grant BJ, Haslam DR, Bhandari M, Sullivan T, Cook DJ, Walter SD, Meade M, Khan H, Bhatnagar N, Guyatt GH. A systematic review and meta-analysis of studies comparing mortality rates of private for-profit and private not-for-profit hospitals. Canadian Medical Association Journal 2002; 166 : 1399-1406.
Engels EA, Schmid CH, Terrin N, Olkin I, Lau J. Heterogeneity and statistical significance in meta-analysis: an empirical study of 125 meta-analyses. Statistics in Medicine 2000; 19 : 1707-1728.
Furukawa TA, Guyatt GH, Griffith LE. Can we individualize the 'number needed to treat'? An empirical study of summary effect measures in meta-analyses. International Journal of Epidemiology 2002; 31 : 72-76.
Gibson JN, Waddell G. Surgical interventions for lumbar disc prolapse: updated Cochrane Review. Spine 2007; 32 : 1735-1747.
Guyatt G, Oxman A, Vist G, Kunz R, Falck-Ytter Y, Alonso-Coello P, Schünemann H. GRADE: an emerging consensus on rating quality of evidence and strength of recommendations. BMJ 2008; 336 : 3.
Guyatt G, Oxman AD, Akl EA, Kunz R, Vist G, Brozek J, Norris S, Falck-Ytter Y, Glasziou P, DeBeer H, Jaeschke R, Rind D, Meerpohl J, Dahm P, Schünemann HJ. GRADE guidelines: 1. Introduction-GRADE evidence profiles and summary of findings tables. Journal of Clinical Epidemiology 2011a; 64 : 383-394.
Guyatt GH, Oxman AD, Kunz R, Brozek J, Alonso-Coello P, Rind D, Devereaux PJ, Montori VM, Freyschuss B, Vist G, Jaeschke R, Williams JW, Jr., Murad MH, Sinclair D, Falck-Ytter Y, Meerpohl J, Whittington C, Thorlund K, Andrews J, Schünemann HJ. GRADE guidelines 6. Rating the quality of evidence--imprecision. Journal of Clinical Epidemiology 2011b; 64 : 1283-1293.
Iorio A, Spencer FA, Falavigna M, Alba C, Lang E, Burnand B, McGinn T, Hayden J, Williams K, Shea B, Wolff R, Kujpers T, Perel P, Vandvik PO, Glasziou P, Schünemann H, Guyatt G. Use of GRADE for assessment of evidence about prognosis: rating confidence in estimates of event rates in broad categories of patients. BMJ 2015; 350 : h870.
Langendam M, Carrasco-Labra A, Santesso N, Mustafa RA, Brignardello-Petersen R, Ventresca M, Heus P, Lasserson T, Moustgaard R, Brozek J, Schünemann HJ. Improving GRADE evidence tables part 2: a systematic survey of explanatory notes shows more guidance is needed. Journal of Clinical Epidemiology 2016; 74 : 19-27.
Levine MN, Raskob G, Landefeld S, Kearon C, Schulman S. Hemorrhagic complications of anticoagulant treatment: the Seventh ACCP Conference on Antithrombotic and Thrombolytic Therapy. Chest 2004; 126 : 287S-310S.
Orrapin S, Rerkasem K. Carotid endarterectomy for symptomatic carotid stenosis. Cochrane Database of Systematic Reviews 2017; 6 : CD001081.
Salpeter S, Greyber E, Pasternak G, Salpeter E. Risk of fatal and nonfatal lactic acidosis with metformin use in type 2 diabetes mellitus. Cochrane Database of Systematic Reviews 2007; 4 : CD002967.
Santesso N, Carrasco-Labra A, Langendam M, Brignardello-Petersen R, Mustafa RA, Heus P, Lasserson T, Opiyo N, Kunnamo I, Sinclair D, Garner P, Treweek S, Tovey D, Akl EA, Tugwell P, Brozek JL, Guyatt G, Schünemann HJ. Improving GRADE evidence tables part 3: detailed guidance for explanatory footnotes supports creating and understanding GRADE certainty in the evidence judgments. Journal of Clinical Epidemiology 2016; 74 : 28-39.
Schünemann HJ, Best D, Vist G, Oxman AD, Group GW. Letters, numbers, symbols and words: how to communicate grades of evidence and recommendations. Canadian Medical Association Journal 2003; 169 : 677-680.
Schünemann HJ, Jaeschke R, Cook DJ, Bria WF, El-Solh AA, Ernst A, Fahy BF, Gould MK, Horan KL, Krishnan JA, Manthous CA, Maurer JR, McNicholas WT, Oxman AD, Rubenfeld G, Turino GM, Guyatt G. An official ATS statement: grading the quality of evidence and strength of recommendations in ATS guidelines and recommendations. American Journal of Respiratory and Critical Care Medicine 2006; 174 : 605-614.
Schünemann HJ, Oxman AD, Brozek J, Glasziou P, Jaeschke R, Vist GE, Williams JW, Jr., Kunz R, Craig J, Montori VM, Bossuyt P, Guyatt GH. Grading quality of evidence and strength of recommendations for diagnostic tests and strategies. BMJ 2008a; 336 : 1106-1110.
Schünemann HJ, Oxman AD, Brozek J, Glasziou P, Bossuyt P, Chang S, Muti P, Jaeschke R, Guyatt GH. GRADE: assessing the quality of evidence for diagnostic recommendations. ACP Journal Club 2008b; 149 : 2.
Schünemann HJ, Mustafa R, Brozek J. [Diagnostic accuracy and linked evidence--testing the chain]. Zeitschrift für Evidenz, Fortbildung und Qualität im Gesundheitswesen 2012; 106 : 153-160.
Schünemann HJ, Tugwell P, Reeves BC, Akl EA, Santesso N, Spencer FA, Shea B, Wells G, Helfand M. Non-randomized studies as a source of complementary, sequential or replacement evidence for randomized controlled trials in systematic reviews on the effects of interventions. Research Synthesis Methods 2013; 4 : 49-62.
Schünemann HJ. Interpreting GRADE's levels of certainty or quality of the evidence: GRADE for statisticians, considering review information size or less emphasis on imprecision? Journal of Clinical Epidemiology 2016; 75 : 6-15.
Schünemann HJ, Cuello C, Akl EA, Mustafa RA, Meerpohl JJ, Thayer K, Morgan RL, Gartlehner G, Kunz R, Katikireddi SV, Sterne J, Higgins JPT, Guyatt G, Group GW. GRADE guidelines: 18. How ROBINS-I and other tools to assess risk of bias in nonrandomized studies should be used to rate the certainty of a body of evidence. Journal of Clinical Epidemiology 2018.
Spencer-Bonilla G, Quinones AR, Montori VM, International Minimally Disruptive Medicine W. Assessing the Burden of Treatment. Journal of General Internal Medicine 2017; 32 : 1141-1145.
Spencer FA, Iorio A, You J, Murad MH, Schünemann HJ, Vandvik PO, Crowther MA, Pottie K, Lang ES, Meerpohl JJ, Falck-Ytter Y, Alonso-Coello P, Guyatt GH. Uncertainties in baseline risk estimates and confidence in treatment effects. BMJ 2012; 345 : e7401.
Sterne JAC, Hernán MA, Reeves BC, Savović J, Berkman ND, Viswanathan M, Henry D, Altman DG, Ansari MT, Boutron I, Carpenter JR, Chan AW, Churchill R, Deeks JJ, Hróbjartsson A, Kirkham J, Jüni P, Loke YK, Pigott TD, Ramsay CR, Regidor D, Rothstein HR, Sandhu L, Santaguida PL, Schünemann HJ, Shea B, Shrier I, Tugwell P, Turner L, Valentine JC, Waddington H, Waters E, Wells GA, Whiting PF, Higgins JPT. ROBINS-I: a tool for assessing risk of bias in non-randomised studies of interventions. BMJ 2016; 355 : i4919.
Thompson DC, Rivara FP, Thompson R. Helmets for preventing head and facial injuries in bicyclists. Cochrane Database of Systematic Reviews 2000; 2 : CD001855.
Tierney JF, Stewart LA, Ghersi D, Burdett S, Sydes MR. Practical methods for incorporating summary time-to-event data into meta-analysis. Trials 2007; 8 .
van Dalen EC, Tierney JF, Kremer LCM. Tips and tricks for understanding and using SR results. No. 7: time‐to‐event data. Evidence-Based Child Health 2007; 2 : 1089-1090.
For permission to re-use material from the Handbook (either academic or commercial), please see here for full details.
Get science-backed answers as you write with Paperpal's Research feature
How to Write a Research Paper Summary
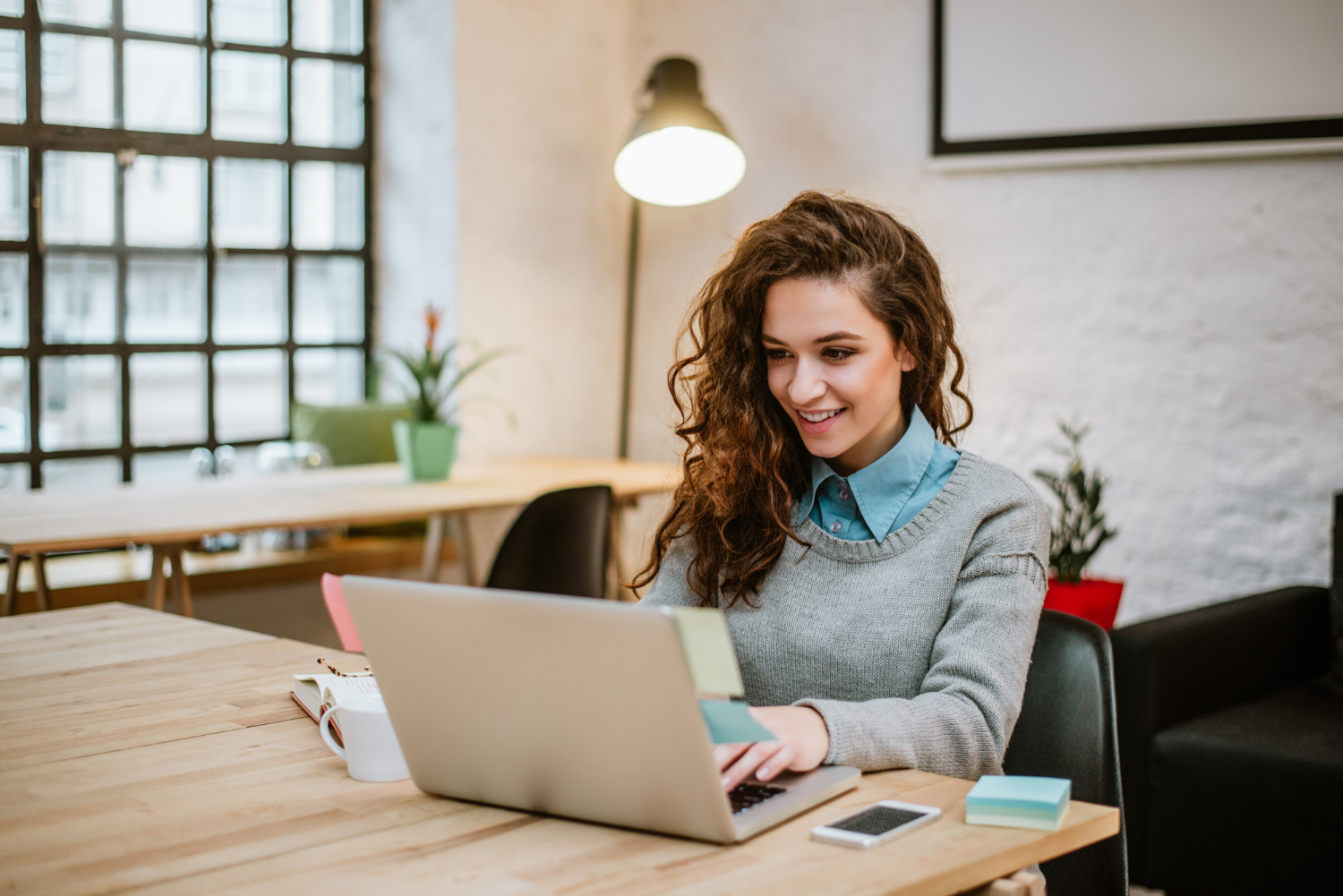
One of the most important skills you can imbibe as an academician is to know how to summarize a research paper. During your academic journey, you may need to write a summary of findings in research quite often and for varied reasons – be it to write an introduction for a peer-reviewed publication , to submit a critical review, or to simply create a useful database for future referencing.
It can be quite challenging to effectively write a research paper summary for often complex work, which is where a pre-determined workflow can help you optimize the process. Investing time in developing this skill can also help you improve your scientific acumen, increasing your efficiency and productivity at work. This article illustrates some useful advice on how to write a research summary effectively. But, what is research summary in the first place?
A research paper summary is a crisp, comprehensive overview of a research paper, which encapsulates the purpose, findings, methods, conclusions, and relevance of a study. A well-written research paper summary is an indicator of how well you have understood the author’s work.
Table of Contents
Draft a research paper summary in minutes with paperpal. click here to start writing.
- 2. Invest enough time to understand the topic deeply
Use Paperpal to summarize your research paper. Click here to get started!
- Mistakes to avoid while writing your research paper summary
Let Paperpal do the heavy lifting. Click here to start writing your summary now!
Frequently asked questions (faq), how to write a research paper summary.
Writing a good research paper summary comes with practice and skill. Here is some useful advice on how to write a research paper summary effectively.
1. Determine the focus of your summary
Before you begin to write a summary of research papers, determine the aim of your research paper summary. This will give you more clarity on how to summarize a research paper, including what to highlight and where to find the information you need, which accelerates the entire process. If you are aiming for the summary to be a supporting document or a proof of principle for your current research findings, then you can look for elements that are relevant to your work.
On the other hand, if your research summary is intended to be a critical review of the research article, you may need to use a completely different lens while reading the paper and conduct your own research regarding the accuracy of the data presented. Then again, if the research summary is intended to be a source of information for future referencing, you will likely have a different approach. This makes determining the focus of your summary a key step in the process of writing an effective research paper summary.
2. Invest enough time to understand the topic deeply
In order to author an effective research paper summary, you need to dive into the topic of the research article. Begin by doing a quick scan for relevant information under each section of the paper. The abstract is a great starting point as it helps you to quickly identify the top highlights of the research article, speeding up the process of understanding the key findings in the paper. Be sure to do a careful read of the research paper, preparing notes that describe each section in your own words to put together a summary of research example or a first draft. This will save your time and energy in revisiting the paper to confirm relevant details and ease the entire process of writing a research paper summary.
When reading papers, be sure to acknowledge and ignore any pre-conceived notions that you might have regarding the research topic. This will not only help you understand the topic better but will also help you develop a more balanced perspective, ensuring that your research paper summary is devoid of any personal opinions or biases.
3. Keep the summary crisp, brief and engaging
A research paper summary is usually intended to highlight and explain the key points of any study, saving the time required to read through the entire article. Thus, your primary goal while compiling the summary should be to keep it as brief, crisp and readable as possible. Usually, a short introduction followed by 1-2 paragraphs is adequate for an effective research article summary. Avoid going into too much technical detail while describing the main results and conclusions of the study. Rather focus on connecting the main findings of the study to the hypothesis , which can make the summary more engaging. For example, instead of simply reporting an original finding – “the graph showed a decrease in the mortality rates…”, you can say, “there was a decline in the number of deaths, as predicted by the authors while beginning the study…” or “there was a decline in the number of deaths, which came as a surprise to the authors as this was completely unexpected…”.
Unless you are writing a critical review of the research article, the language used in your research paper summaries should revolve around reporting the findings, not assessing them. On the other hand, if you intend to submit your summary as a critical review, make sure to provide sufficient external evidence to support your final analysis. Invest sufficient time in editing and proofreading your research paper summary thoroughly to ensure you’ve captured the findings accurately. You can also get an external opinion on the preliminary draft of the research paper summary from colleagues or peers who have not worked on the research topic.
Mistakes to avoid while writing your research paper summary
Now that you’ve understood how to summarize a research paper, watch out for these red flags while writing your summary.
- Not paying attention to the word limit and recommended format, especially while submitting a critical review
- Evaluating the findings instead of maintaining an objective , unbiased view while reading the research paper
- Skipping the essential editing step , which can help eliminate avoidable errors and ensure that the language does not misrepresent the findings
- Plagiarism, it is critical to write in your own words or paraphrase appropriately when reporting the findings in your scientific article summary
We hope the recommendations listed above will help answer the question of how to summarize a research paper and enable you to tackle the process effectively.
Summarize your research paper with Paperpal
Paperpal, an AI academic writing assistant, is designed to support academics at every step of the academic writing process. Built on over two decades of experience helping researchers get published and trained on millions of published research articles, Paperpal offers human precision at machine speed. Paperpal Copilot, with advanced generative AI features, can help academics achieve 2x the writing in half the time, while transforming how they research and write.
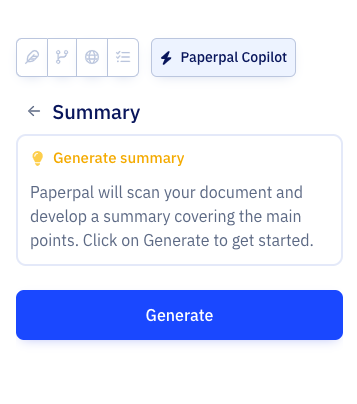
How to summarize a research paper with Paperpal?
To generate your research paper summary, simply login to the platform and use the Paperpal Copilot Summary feature to create a flawless summary of your work. Here’s a step-by-step process to help you craft a summary in minutes:
- Paste relevant research articles to be summarized into Paperpal; the AI will scan each section and extract key information.
- In minutes, Paperpal will generate a comprehensive summary that showcases the main paper highlights while adhering to academic writing conventions.
- Check the content to polish and refine the language, ensure your own voice, and add citations or references as needed.
The abstract and research paper summary serve similar purposes but differ in scope, length, and placement. The abstract is a concise yet detailed overview of the research, placed at the beginning of a paper, with the aim of providing readers with a quick understanding of the paper’s content and to help them decide whether to read the full article. Usually limited to a few hundred words, it highlights the main objectives, methods, results, and conclusions of the study. On the other hand, a research paper summary provides a crisp account of the entire research paper. Its purpose is to provide a brief recap for readers who may want to quickly grasp the main points of the research without reading the entire paper in detail.
The structure of a research summary can vary depending on the specific requirements or guidelines provided by the target publication or institution. A typical research summary includes the following key sections: introduction (including the research question or objective), methodology (briefly describing the research design and methods), results (summarizing the key findings), discussion (highlighting the implications and significance of the findings), and conclusion (providing a summary of the main points and potential future directions).
The summary of a research paper is important because it provides a condensed overview of the study’s purpose, methods, results, and conclusions. It allows you to quickly grasp the main points and relevance of the research without having to read the entire paper. Research summaries can also be an invaluable way to communicate research findings to a broader audience, such as policymakers or the general public.
When writing a research paper summary, it is crucial to avoid plagiarism by properly attributing the original authors’ work. To learn how to summarize a research paper while avoiding plagiarism, follow these critical guidelines: (1) Read the paper thoroughly to understand the main points and key findings. (2) Use your own words and sentence structures to restate the information, ensuring that the research paper summary reflects your understanding of the paper. (3) Clearly indicate when you are paraphrasing or quoting directly from the original paper by using appropriate citation styles. (4) Cite the original source for any specific ideas, concepts, or data that you include in your summary. (5) Review your summary to ensure it accurately represents the research paper while giving credit to the original authors.
Paperpal is a comprehensive AI writing toolkit that helps students and researchers achieve 2x the writing in half the time. It leverages 21+ years of STM experience and insights from millions of research articles to provide in-depth academic writing, language editing, and submission readiness support to help you write better, faster.
Get accurate academic translations, rewriting support, grammar checks, vocabulary suggestions, and generative AI assistance that delivers human precision at machine speed. Try for free or upgrade to Paperpal Prime starting at US$19 a month to access premium features, including consistency, plagiarism, and 30+ submission readiness checks to help you succeed.
Experience the future of academic writing – Sign up to Paperpal and start writing for free!
Related Reads:
- 5 Reasons for Rejection After Peer Review
- Ethical Research Practices For Research with Human Subjects
- How to Write a Conclusion for Research Papers (with Examples)
- Publish or Perish – Understanding the Importance of Scholarly Publications in Academia
PhD Dissertation Outline: Creating a Roadmap to Success
How ai can improve the academic writing experience, you may also like, measuring academic success: definition & strategies for excellence, is it ethical to use ai-generated abstracts without..., what are journal guidelines on using generative ai..., should you use ai tools like chatgpt for..., 9 steps to publish a research paper, how to make translating academic papers less challenging, self-plagiarism in research: what it is and how..., 6 tips for post-doc researchers to take their..., presenting research data effectively through tables and figures, 8 most effective ways to increase motivation for....
- Affiliate Program

- UNITED STATES
- 台灣 (TAIWAN)
- TÜRKIYE (TURKEY)
- Academic Editing Services
- - Research Paper
- - Journal Manuscript
- - Dissertation
- - College & University Assignments
- Admissions Editing Services
- - Application Essay
- - Personal Statement
- - Recommendation Letter
- - Cover Letter
- - CV/Resume
- Business Editing Services
- - Business Documents
- - Report & Brochure
- - Website & Blog
- Writer Editing Services
- - Script & Screenplay
- Our Editors
- Client Reviews
- Editing & Proofreading Prices
- Wordvice Points
- Partner Discount
- Plagiarism Checker
- APA Citation Generator
- MLA Citation Generator
- Chicago Citation Generator
- Vancouver Citation Generator
- - APA Style
- - MLA Style
- - Chicago Style
- - Vancouver Style
- Writing & Editing Guide
- Academic Resources
- Admissions Resources
How to Write the Results/Findings Section in Research
What is the research paper Results section and what does it do?
The Results section of a scientific research paper represents the core findings of a study derived from the methods applied to gather and analyze information. It presents these findings in a logical sequence without bias or interpretation from the author, setting up the reader for later interpretation and evaluation in the Discussion section. A major purpose of the Results section is to break down the data into sentences that show its significance to the research question(s).
The Results section appears third in the section sequence in most scientific papers. It follows the presentation of the Methods and Materials and is presented before the Discussion section —although the Results and Discussion are presented together in many journals. This section answers the basic question “What did you find in your research?”
What is included in the Results section?
The Results section should include the findings of your study and ONLY the findings of your study. The findings include:
- Data presented in tables, charts, graphs, and other figures (may be placed into the text or on separate pages at the end of the manuscript)
- A contextual analysis of this data explaining its meaning in sentence form
- All data that corresponds to the central research question(s)
- All secondary findings (secondary outcomes, subgroup analyses, etc.)
If the scope of the study is broad, or if you studied a variety of variables, or if the methodology used yields a wide range of different results, the author should present only those results that are most relevant to the research question stated in the Introduction section .
As a general rule, any information that does not present the direct findings or outcome of the study should be left out of this section. Unless the journal requests that authors combine the Results and Discussion sections, explanations and interpretations should be omitted from the Results.
How are the results organized?
The best way to organize your Results section is “logically.” One logical and clear method of organizing research results is to provide them alongside the research questions—within each research question, present the type of data that addresses that research question.
Let’s look at an example. Your research question is based on a survey among patients who were treated at a hospital and received postoperative care. Let’s say your first research question is:
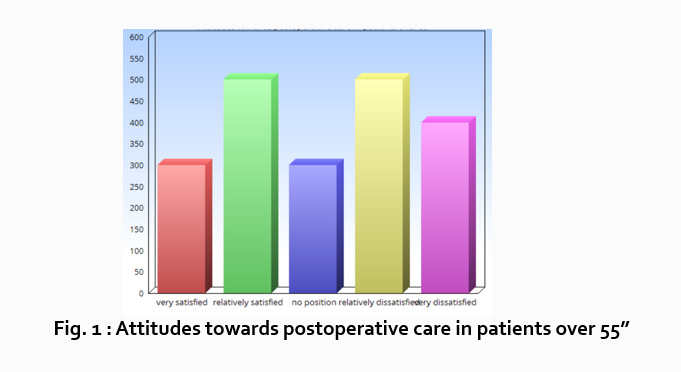
“What do hospital patients over age 55 think about postoperative care?”
This can actually be represented as a heading within your Results section, though it might be presented as a statement rather than a question:
Attitudes towards postoperative care in patients over the age of 55
Now present the results that address this specific research question first. In this case, perhaps a table illustrating data from a survey. Likert items can be included in this example. Tables can also present standard deviations, probabilities, correlation matrices, etc.
Following this, present a content analysis, in words, of one end of the spectrum of the survey or data table. In our example case, start with the POSITIVE survey responses regarding postoperative care, using descriptive phrases. For example:
“Sixty-five percent of patients over 55 responded positively to the question “ Are you satisfied with your hospital’s postoperative care ?” (Fig. 2)
Include other results such as subcategory analyses. The amount of textual description used will depend on how much interpretation of tables and figures is necessary and how many examples the reader needs in order to understand the significance of your research findings.
Next, present a content analysis of another part of the spectrum of the same research question, perhaps the NEGATIVE or NEUTRAL responses to the survey. For instance:
“As Figure 1 shows, 15 out of 60 patients in Group A responded negatively to Question 2.”
After you have assessed the data in one figure and explained it sufficiently, move on to your next research question. For example:
“How does patient satisfaction correspond to in-hospital improvements made to postoperative care?”
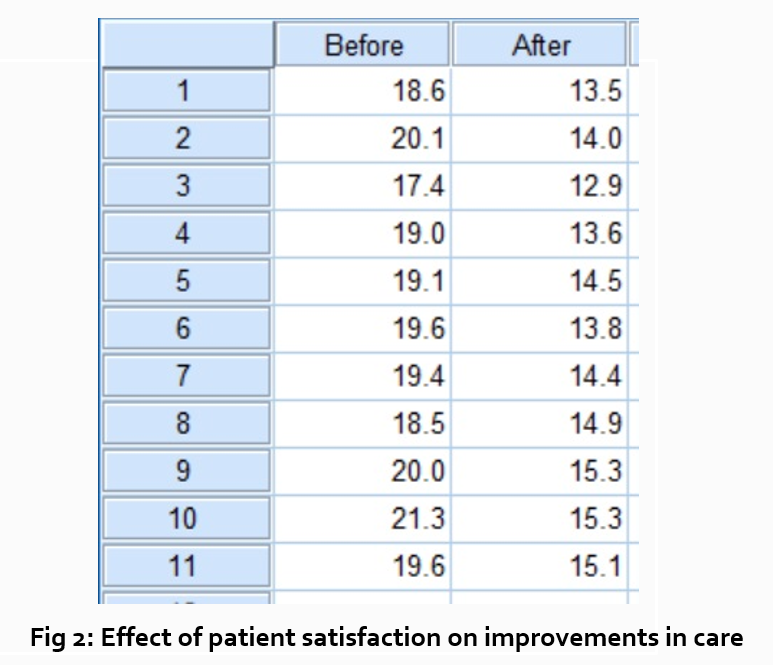
This kind of data may be presented through a figure or set of figures (for instance, a paired T-test table).
Explain the data you present, here in a table, with a concise content analysis:
“The p-value for the comparison between the before and after groups of patients was .03% (Fig. 2), indicating that the greater the dissatisfaction among patients, the more frequent the improvements that were made to postoperative care.”
Let’s examine another example of a Results section from a study on plant tolerance to heavy metal stress . In the Introduction section, the aims of the study are presented as “determining the physiological and morphological responses of Allium cepa L. towards increased cadmium toxicity” and “evaluating its potential to accumulate the metal and its associated environmental consequences.” The Results section presents data showing how these aims are achieved in tables alongside a content analysis, beginning with an overview of the findings:
“Cadmium caused inhibition of root and leave elongation, with increasing effects at higher exposure doses (Fig. 1a-c).”
The figure containing this data is cited in parentheses. Note that this author has combined three graphs into one single figure. Separating the data into separate graphs focusing on specific aspects makes it easier for the reader to assess the findings, and consolidating this information into one figure saves space and makes it easy to locate the most relevant results.
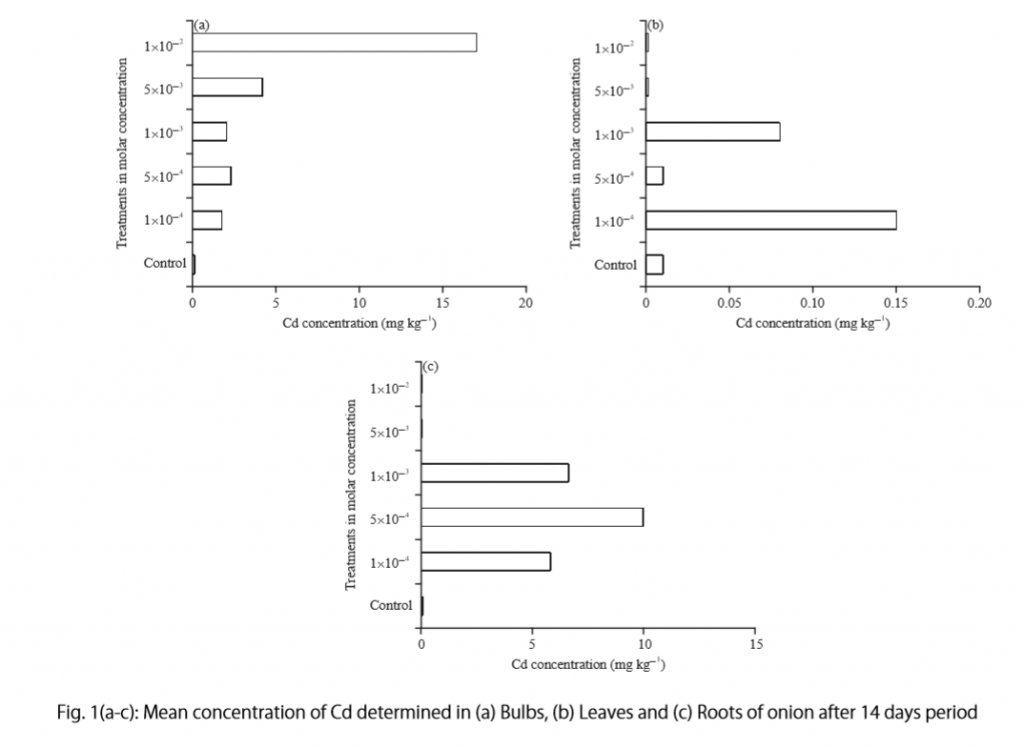
Following this overall summary, the relevant data in the tables is broken down into greater detail in text form in the Results section.
- “Results on the bio-accumulation of cadmium were found to be the highest (17.5 mg kgG1) in the bulb, when the concentration of cadmium in the solution was 1×10G2 M and lowest (0.11 mg kgG1) in the leaves when the concentration was 1×10G3 M.”
Captioning and Referencing Tables and Figures
Tables and figures are central components of your Results section and you need to carefully think about the most effective way to use graphs and tables to present your findings . Therefore, it is crucial to know how to write strong figure captions and to refer to them within the text of the Results section.
The most important advice one can give here as well as throughout the paper is to check the requirements and standards of the journal to which you are submitting your work. Every journal has its own design and layout standards, which you can find in the author instructions on the target journal’s website. Perusing a journal’s published articles will also give you an idea of the proper number, size, and complexity of your figures.
Regardless of which format you use, the figures should be placed in the order they are referenced in the Results section and be as clear and easy to understand as possible. If there are multiple variables being considered (within one or more research questions), it can be a good idea to split these up into separate figures. Subsequently, these can be referenced and analyzed under separate headings and paragraphs in the text.
To create a caption, consider the research question being asked and change it into a phrase. For instance, if one question is “Which color did participants choose?”, the caption might be “Color choice by participant group.” Or in our last research paper example, where the question was “What is the concentration of cadmium in different parts of the onion after 14 days?” the caption reads:
“Fig. 1(a-c): Mean concentration of Cd determined in (a) bulbs, (b) leaves, and (c) roots of onions after a 14-day period.”
Steps for Composing the Results Section
Because each study is unique, there is no one-size-fits-all approach when it comes to designing a strategy for structuring and writing the section of a research paper where findings are presented. The content and layout of this section will be determined by the specific area of research, the design of the study and its particular methodologies, and the guidelines of the target journal and its editors. However, the following steps can be used to compose the results of most scientific research studies and are essential for researchers who are new to preparing a manuscript for publication or who need a reminder of how to construct the Results section.
Step 1 : Consult the guidelines or instructions that the target journal or publisher provides authors and read research papers it has published, especially those with similar topics, methods, or results to your study.
- The guidelines will generally outline specific requirements for the results or findings section, and the published articles will provide sound examples of successful approaches.
- Note length limitations on restrictions on content. For instance, while many journals require the Results and Discussion sections to be separate, others do not—qualitative research papers often include results and interpretations in the same section (“Results and Discussion”).
- Reading the aims and scope in the journal’s “ guide for authors ” section and understanding the interests of its readers will be invaluable in preparing to write the Results section.
Step 2 : Consider your research results in relation to the journal’s requirements and catalogue your results.
- Focus on experimental results and other findings that are especially relevant to your research questions and objectives and include them even if they are unexpected or do not support your ideas and hypotheses.
- Catalogue your findings—use subheadings to streamline and clarify your report. This will help you avoid excessive and peripheral details as you write and also help your reader understand and remember your findings. Create appendices that might interest specialists but prove too long or distracting for other readers.
- Decide how you will structure of your results. You might match the order of the research questions and hypotheses to your results, or you could arrange them according to the order presented in the Methods section. A chronological order or even a hierarchy of importance or meaningful grouping of main themes or categories might prove effective. Consider your audience, evidence, and most importantly, the objectives of your research when choosing a structure for presenting your findings.
Step 3 : Design figures and tables to present and illustrate your data.
- Tables and figures should be numbered according to the order in which they are mentioned in the main text of the paper.
- Information in figures should be relatively self-explanatory (with the aid of captions), and their design should include all definitions and other information necessary for readers to understand the findings without reading all of the text.
- Use tables and figures as a focal point to tell a clear and informative story about your research and avoid repeating information. But remember that while figures clarify and enhance the text, they cannot replace it.
Step 4 : Draft your Results section using the findings and figures you have organized.
- The goal is to communicate this complex information as clearly and precisely as possible; precise and compact phrases and sentences are most effective.
- In the opening paragraph of this section, restate your research questions or aims to focus the reader’s attention to what the results are trying to show. It is also a good idea to summarize key findings at the end of this section to create a logical transition to the interpretation and discussion that follows.
- Try to write in the past tense and the active voice to relay the findings since the research has already been done and the agent is usually clear. This will ensure that your explanations are also clear and logical.
- Make sure that any specialized terminology or abbreviation you have used here has been defined and clarified in the Introduction section .
Step 5 : Review your draft; edit and revise until it reports results exactly as you would like to have them reported to your readers.
- Double-check the accuracy and consistency of all the data, as well as all of the visual elements included.
- Read your draft aloud to catch language errors (grammar, spelling, and mechanics), awkward phrases, and missing transitions.
- Ensure that your results are presented in the best order to focus on objectives and prepare readers for interpretations, valuations, and recommendations in the Discussion section . Look back over the paper’s Introduction and background while anticipating the Discussion and Conclusion sections to ensure that the presentation of your results is consistent and effective.
- Consider seeking additional guidance on your paper. Find additional readers to look over your Results section and see if it can be improved in any way. Peers, professors, or qualified experts can provide valuable insights.
One excellent option is to use a professional English proofreading and editing service such as Wordvice, including our paper editing service . With hundreds of qualified editors from dozens of scientific fields, Wordvice has helped thousands of authors revise their manuscripts and get accepted into their target journals. Read more about the proofreading and editing process before proceeding with getting academic editing services and manuscript editing services for your manuscript.
As the representation of your study’s data output, the Results section presents the core information in your research paper. By writing with clarity and conciseness and by highlighting and explaining the crucial findings of their study, authors increase the impact and effectiveness of their research manuscripts.
For more articles and videos on writing your research manuscript, visit Wordvice’s Resources page.
Wordvice Resources
- How to Write a Research Paper Introduction
- Which Verb Tenses to Use in a Research Paper
- How to Write an Abstract for a Research Paper
- How to Write a Research Paper Title
- Useful Phrases for Academic Writing
- Common Transition Terms in Academic Papers
- Active and Passive Voice in Research Papers
- 100+ Verbs That Will Make Your Research Writing Amazing
- Tips for Paraphrasing in Research Papers
Numbers, Facts and Trends Shaping Your World
Read our research on:
Full Topic List
Regions & Countries
- Publications
- Our Methods
- Short Reads
- Tools & Resources
Read Our Research On:
A record-high share of 40-year-olds in the U.S. have never been married
As of 2021, 25% of 40-year-olds in the United States had never been married. This was a significant increase from 20% in 2010, according to a new Pew Research Center analysis of Census Bureau data.
Pew Research Center conducted this analysis to look at changing marriage rates among 40-year-olds in the United States from 1850 to 2021. This analysis uses decennial census data and the American Community Survey (ACS). The ACS is the largest household survey in the U.S., with a sample of more than 3 million addresses. Collected by the Census Bureau since 2001, it covers the topics previously included in the long form of the decennial census. The ACS is designed to provide estimates of the size and characteristics of the nation’s resident population.
These large datasets allow for reliable estimates of outcomes for people at a given age.
The data on cohabitation comes from the Census Bureau’s Current Population Survey (CPS) 2022 Annual Social and Economic Supplement.
The microdata files used for this analysis were provided by the Integrated Public Use Microdata Series (IPUMS) from the University of Minnesota. IPUMS standardizes variable names and coding across years as much as possible, making it easier to analyze the data over time. The first census after the American Revolution occurred in 1790. IPUMS has decennial census samples from 1850 on. The 1850 and 1860 census samples only include the free population.
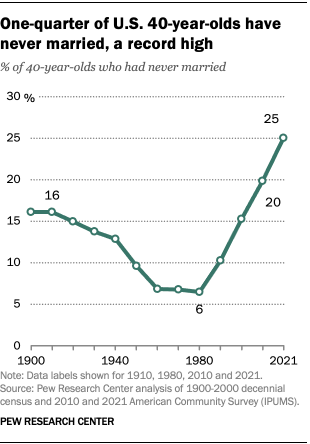
Marriage has long been a central institution in the lives of Americans. In 1980, just 6% of 40-year-olds had never been married. But people born from the 1960s onward have been increasingly delaying marriage , and a growing share are forgoing it altogether.
The 2021 data marks a new milestone in that decadeslong trend .
While many unmarried 40-year-olds are living with a romantic partner, most are not. In 2022, 22% of never-married adults ages 40 to 44 were cohabiting.
The share of 40-year-olds in 2021 who had never married varied by the following demographic characteristics:
- Gender: A higher share of men than women had never married.
- Race and ethnicity: Black 40-year-olds were much more likely to have never married than Hispanic, White and Asian 40-year-olds.
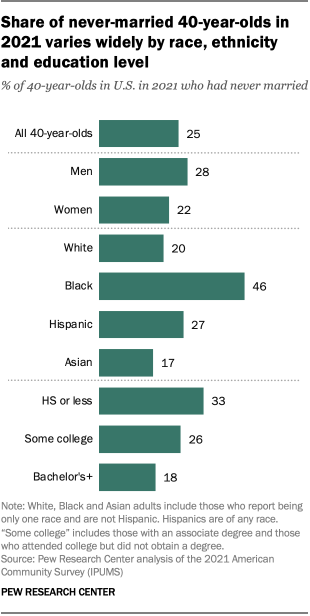
- Education level: 40-year-olds without a four-year college degree were more likely to have never married than those who had completed at least a bachelor’s degree. One-third of those with a high school diploma or less had never married, compared with 26% of those with some college education and 18% of those with a bachelor’s degree or more education.
The overall decrease in the share of 40-year-olds who have married is especially notable because the share of 40-year-olds who had completed at least a bachelor’s degree was much higher in 2021 than in 1980 (39% vs. 18%). More-highly educated 40-year-olds are more likely to have married, but the growth of this group has not reversed the overall trend of delaying or forgoing marriage.
To be sure, we can’t assume that if someone has not married by age 40, they never will. In fact, about one-in-four 40-year-olds who had not married in 2001 had done so by age 60. If that pattern holds, a similar share of today’s never-married 40-year-olds will marry in the coming decades.
- Family & Relationships
- Marriage & Divorce
- Older Adults & Aging
- Unmarried Adults
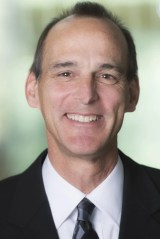
Richard Fry is a senior researcher focusing on economics and education at Pew Research Center
Few East Asian adults believe women have an obligation to society to have children
Among parents with young adult children, some dads feel less connected to their kids than moms do, how teens and parents approach screen time, most east asian adults say men and women should share financial and caregiving duties, among young adults without children, men are more likely than women to say they want to be parents someday, most popular.
1615 L St. NW, Suite 800 Washington, DC 20036 USA (+1) 202-419-4300 | Main (+1) 202-857-8562 | Fax (+1) 202-419-4372 | Media Inquiries
Research Topics
- Age & Generations
- Coronavirus (COVID-19)
- Economy & Work
- Gender & LGBTQ
- Immigration & Migration
- International Affairs
- Internet & Technology
- Methodological Research
- News Habits & Media
- Non-U.S. Governments
- Other Topics
- Politics & Policy
- Race & Ethnicity
- Email Newsletters
ABOUT PEW RESEARCH CENTER Pew Research Center is a nonpartisan fact tank that informs the public about the issues, attitudes and trends shaping the world. It conducts public opinion polling, demographic research, media content analysis and other empirical social science research. Pew Research Center does not take policy positions. It is a subsidiary of The Pew Charitable Trusts .
Copyright 2024 Pew Research Center
Terms & Conditions
Privacy Policy
Cookie Settings
Reprints, Permissions & Use Policy
- Open access
- Published: 26 April 2024
Associations of the circulating levels of cytokines with the risk of myeloproliferative neoplasms: a bidirectional mendelian-randomization study
- Hao Xiong 1 , 2 ,
- Huitao Zhang 2 ,
- Jun Bai 1 ,
- Yanhong Li 1 ,
- Lijuan Li 1 &
- Liansheng Zhang 1
BMC Cancer volume 24 , Article number: 531 ( 2024 ) Cite this article
47 Accesses
Metrics details
In the pathogenesis of myeloproliferative neoplasms (MPN), inflammation plays an important role. However, it is unclear whether there is a causal link between inflammation and MPNs. We used a bidirectional, two-sample Mendelian randomization (MR) approach to investigate the causal relationship between systemic inflammatory cytokines and myeloproliferative neoplasms.
A genome-wide association study (GWAS) of 8293 European participants identified genetic instrumental variables for circulating cytokines and growth factors. Summary statistics of MPN were obtained from a GWAS including 1086 cases and 407,155 controls of European ancestry. The inverse-variance-weighted method was mainly used to compute odds ratios (OR) and 95% confidence intervals (Cl).
Our results showed that higher Interleukin-2 receptor, alpha subunit ( IL-2rα ) levels, and higher Interferon gamma-induced protein 10 ( IP-10 ) levels were associated with an increased risk of MPN (OR = 1.36,95%CI = 1.03–1.81, P = 0.032; OR = 1.55,95%CI = 1.09–2.22, P = 0.015; respectively).In addition, Genetically predicted MPN promotes expression of the inflammatory cytokines interleukin-10 ( IL-10 ) (BETA = 0.033, 95% CI = 0.003 ~ 0.064, P = 0.032) and monokine induced by interferon-gamma ( MIG ) (BETA = 0.052, 95% CI = 0.002–0.102, P = 0.043) and, on activation, normal T cells express and secrete RANTES (BETA = 0.055, 95% CI = 0.0090.1, P = 0.018).
Our findings suggest that cytokines are essential to the pathophysiology of MPN. More research is required if these biomarkers can be used to prevent and treat MPN.
Peer Review reports
Introduction
The chronic hematological malignancies known as myeloproliferative neoplasms (MPN), which include polycythemia vera (PV), essential thrombocythemia (ET), and myelofibrosis (MF), advance at varying rates [ 1 ]. The incidence rates of PV, ET, and PMF are estimated to be 0.5 to 4.0, 1.1 to 2.0, and 0.3 to 2.0 per 100,000 people, respectively. It is reported that nearly 10–15% of patients with MPN progress to AML [ 2 ], more than 20% will develop thrombosis during the disease, and approximately 6.2% of newly diagnosed patients will suffer hemorrhage [ 3 , 4 ]. The presence of these symptoms mentioned above raises the rate of disability and mortality in MPN patients [ 5 ] and imposes a huge economic burden on the family and society. The most common feature of MPN is hyperactivation of Janus kinase 2 ( JAK2 ) signaling, which is caused by acquired mutations in JAK2 , MPL , and CALR [ 6 ]. However, clinically used JAK2 inhibitors such as Ruxolitinib and Fedratinib have limited efficacy, high toxicity, and are prone to drug resistance [ 6 , 7 , 8 ]. Therefore, increased awareness of the pathogenic components may offer clues for halting the disease's course and creating novel treatments.
The chronic inflammatory environment is one of the typical features of myeloproliferative neoplasms, where inflammation is tightly intertwined with tumor clones, providing a permissive micro-environment for disease progression [ 9 , 10 , 11 ]. Inflammatory cytokines are essential immune mediators in the physiology and disease process of MPN and not only play a significant role in inflammatory pathology but are also inextricably linked to the development of the disease [ 9 , 12 ]. GM-CSF , IL-1 , IL-4 , IL-5 , IL-6 , IL-10 , IFN-2 , MIP-1 , IL-12 , and TNF-α were shown to have higher cytokine levels in treatment-naive patients in all three MPN groups when compared to age-matched control participants, according to an observational study [ 9 ]. In addition, serum IL-2 and soluble IL-2 receptor alpha ( sIL-2rα ) increased as patients with MPNs progressed to advanced clinical stages [ 13 ], and serum IL-2 , sIL-2rα , and IL-6 levels were positively correlated with bone marrow neovascularization, indicating that increased inflammatory responses may be connected to the course of MPN disease [ 14 ], suggesting that MPN patients may benefit from using cytokines as a tool for illness monitoring [ 15 ]. However, little is known about the mechanisms and duration of inflammation in MPNs [ 16 ]. The origins of the increased cytokine production in MPNs (alterations, others?) and whether inflammation may occur before the development of JAK2/CALR/MPL gene mutations are still up for dispute. Observational studies are prone to common biases such as reverse causality and residual confounding [ 17 ] and have limitations such as small sample sizes and short follow-up periods. These studies, however, only addressed a small subset of inflammatory cytokines and did not take into account how other physical factors can affect changes in inflammatory cytokine levels. Determining whether variations in inflammatory cytokines cause the development of MPN or whether MPN development influences the microenvironment and causes variations in inflammatory cytokines is crucial. Investigating the precise nature of the connection between inflammatory cytokines and MPN is crucial from a therapeutic standpoint given the lack of knowledge regarding the etiology of MPN.
To establish a link between inflammatory cytokines and MPN, we applied Mendelian randomization (MR).MR has the advantage of reducing confounding variables and measurement error, as well as addressing the limitations of traditional observational studies mentioned above. This approach can effectively avoid bias caused by reverse causality [ 18 ]. The greatest level of evidence hierarchy outside of randomized controlled trials is provided by MR, which uses genetic variation as an instrumental variable (IV), which has been a dependable tool for getting reliable estimates of the causal influence of numerous risk variables on health [ 19 ]. In the current investigation, we used a two-sample MR design to methodically evaluate the potential causal link between inflammatory cytokines and MPN risk. Additionally, reverse MR analysis was done to determine how MPN affected cytokines.
Study design
The Mendelian randomization design method uses publicly available datasets from extensive genome-wide association studies (GWAS) for risk factors and disease to examine whether exposure has a causal effect on disease emergence. As a genetic instrumental variable analysis, MR Uses single nucleotide polymorphisms (SNPs) as instrumental variables for the risk factor of interest. SNP S are randomly assigned at meiosis and are not subject to reverse causality bias, so the Mendelian randomization approach can overcome unmeasured confounders and lead to more reliable causal inferences [ 20 ].
To determine the relationship between inflammatory cytokine levels and the risk of MPN, we conducted a bidirectional Mendelian randomized trial. As the summary statistics from published research are made available to the public, the institutional review board did not need to approve our study's ethics further. In Supplementary Table 1 , the features of the data used in this investigation are displayed.
Data sources and instruments
The data on inflammatory cytokines was obtained from a meta-analysis published in 2017 that summarized data from genome-wide association studies (GWAS) carried out with three Finnish cohorts (YFS and FINRISK, 1997 and 2002), totaling 8,293 Finns. The original publication has information about the cytokine assays, inclusion standards, etc. [ 21 ]. In MR analysis, P -values are used to measure whether there is an association between genetic variants and exposure factors. We set the P value to find the genetic variant loci associated with the trait. The independence of the selected instrumental variables was further ensured by removing all SNPs with linkage disequilibrium (LD) to avoid biased results (parameters kb = 250, r 2 = 0.001). Calculate the F-statistic [using the formula: F = (N-2)*R2/(1-R2), N is the sample size]to assess the extent of weak instrument bias, F > 10 suggests that full instrumental SNPs are sufficiently strong to lessen any potential bias, while an F-statistic ≤ 10 implies weak instruments [ 22 ].Initially, we used P < 5 × 10 –8 as a criterion to look for instrumental factors, and we discovered that the majority of cytokines had either no SNPs or only a few SNPs [ 3 ]. To get as many cytokines as possible into the study. Furthermore, we chose IVs using a permissive significance criterion ( P < 5 × 10 –6 ). Further, we use the parameter kb = 250, r 2 = 0.001 to eliminate the linkage disequilibrium among variables. Supplementary Tables 2 and 3 provide extensive details on the features of the IVs.
- Myeloproliferative neoplasms
The MPN Patients' data were obtained from publicly available GWAS data, which we downloaded from open GWAS ( https://gwas.mrcieu.ac.uk/ ). The data come from UK Biobank, which is a cohort study conducted between 2006 and 2010. The study collected in-depth genetic and phenotypic data on approximately 500,000 people across the United Kingdom. The data we downloaded for analysis included 1086 MPN patients and 407,155 controls [ 23 ]. The original article describes the criteria and procedures for the data's quality control [ 23 ]. Erythrocytosis, primary thrombocythemia, myelofibrosis, chronic myeloid leukemia, and chronic myeloproliferative illness are all included in the UKBB's description of the MPN phenotype. Those who had polycythemia vera, essential thrombocythemia, myelofibrosis, chronic myeloid leukemia, or malignant mastocytosis were additionally labeled as cases if they had self-reported cancer, self-reported sickness code, or histology of cancer tumor code. In the MPN dataset, we screened SNPs as instrumental variables according to the following criteria: ( P < 5 × 10 –8 , r 2 = 0.001, kb = 250 kb). Five SNPs were kept as separate MPN IVs. Inverse MR analyses were performed using these SNPs to examine the genetic influence of MPN on the amount of cytokines.
Bioinformatics analysis
We use the data set from the GEO database for gene expression spectrum analysis ( https://www.ncbi.nlm.nih.gov/geo/query/acc.cgi ). The GSE103237 dataset is based on the GPL13667 platform and consists of 26 polycythemia vera, 24 essential thrombocythemia, and 15 normal bone marrow samples. The GSE136335 dataset is based on the GPL17586 platform consisting of 8 myelofibrosis patients and 6 normal bone marrow samples. We used the "Ggpubr" package to create violin plots to visualize the expression of IP-10 , and IL-2ra in the three subtypes of MPN. The diagnostic value of IP-10 and IL-2ra was analyzed by the receiver operating characteristic (ROC) curve. The expression of genes was analyzed using the "pROC" package (v1.18.2), and genes with the area under the curve (AUC) > 0.7 were considered potential diagnostic markers.
Statistical analysis
We harmonized the effect of SNPs on inflammatory cytokines and MPNs before the Mendelian randomization analysis. Furthermore, we used the two-sample MR software package for MR analysis. The inverse variance weighted(IVW) methods were used to assess potential causality, while weighted median and MR-Egger regression methods were used as complementary methods for causality between inflammatory cytokines and MPN [ 24 ]. The MR-Egger method was used for multiple validity testing. We used Cochran's Q test to assess heterogeneity in IVW [ 25 ]. MR-PRESSO was used to detect the presence of outliers [ 26 ], and leave-one-out analyses were used to verify whether the causal effect depended on a single variant. We also performed instrumental strength tests using the F statistic, with F > 10 indicating sufficient strength [ 27 ]. Eventually, if the IVW method result is significant ( P < 0.05), even if the findings of other techniques are not significant and there is no pleiotropy or heterogeneity discovered, it may be regarded as a good result, given that the beta values of the other methods are in the same direction [ 28 ].
Based on the number of cytokines, we used the Bonferroni approach to compensate for multiple comparisons and set statistical significance at a P -value < 1.22 × 10 −3 (0.05/41) level. If a P -value was between 1.22 × 10 −3 and 0.05, we considered suggestive evidence for a potential causal association. The majority of the work mentioned above was completed using the R analysis program (version 4.3.0), which was applied to the relevant R package, including Two-sample MR, data array, etc.
Instrumental variables
Figure 1 offers a flowchart of the full-text logic. In the study of the effect of inflammatory factors on MPN, we screened instrumental variables according to the criteria of P < 5 × 10 –6 and r 2 < 0.001. A total of 354 SNPs linked to 41 cytokines were used for MR analysis after being harmonized with the outcome variable MPN (Supplementary Table 1 ).To analyze the effect of MPN on inflammatory factors, we used MPN as an exposure factor, By using such criteria ( P < 5 × 10 –8 , r 2 < 0.001), a total of five SNPs were obtained for subsequent analyses, and specific SNP information can be found in Supplementary Table 2 . These SNPs' F statistics, which ranged from 20.77 to 781.85(Table 1 ), showed that the instrument was sufficiently reliable to rule out the possibility of a null relationship brought on by instrument bias.
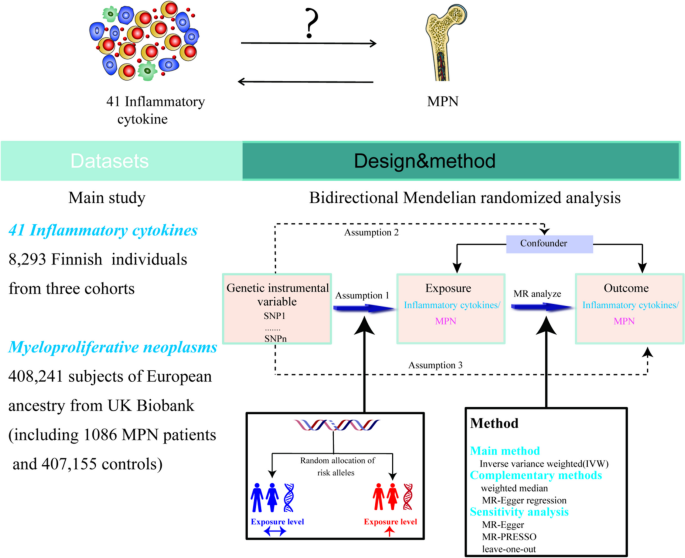
Study design overview and assumptions for MR Design. Assumption 1: IVs were not related to Confounders; Assumntion2: a strong correlation between iVs and exposure; Assumption 3: IVs can affect outcomes only through exposure and not through other pathways
Effect of inflammatory cytokines on MPN
We explored the effects of 41 cytokines on MPN sequentially using a two-sample Mendelian randomization analysis (Supplementary Table 3 ). Only two cytokines (Interleukin-2 receptor, alpha subunit ( IL − 2rα ), and interferon gamma-induced protein 10 ( IP-10 ) revealed suggestive associations with MPN risk after the Bonferroni correction. Genetically determined higher levels of circulating IL-2rα are suggestively positively associated with MPN risk [odds ratio (OR): 1.365,95% confidence interval (CI): 1.029–1.814, P = 0.032]. The other two complementary analytical methods Weighted median and MR Egger also obtained similar but not statistically significant results (Fig. 2 A). Furthermore, Cochran's Q test did not reveal any heterogeneity ( P = 0.268). A directional pleiotropy was also not discovered (MR egger-intercept = 0.021, P for MR egger-intercept = 0.710; P for MR PRESSO global test = 0.528) (Supplementary Table 5 ). Removing one SNP did not significantly change the results in the leave-one-out sensitivity analysis (Fig. 3 A). Sensitivity analyses were conducted using the leave-one-out analyses to assess the reliability and stability of the results. The overall effect of the remaining instrumental variables was calculated by removing each SNP stepwise and observing whether the results changed after removing a single SNP. The results showed that removing individual SNPs did not significantly change the results of the exclusion sensitivity analyses (Fig. 3 A). We did not find secondary phenotypes associated with SNPs that were used as instrumental variables on Pheno-Scanner's website.
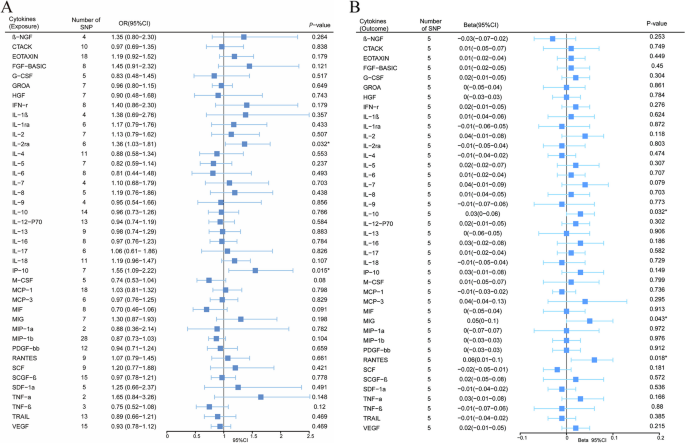
Forest plot of Mendelian randomization analysis of the correlation between inflammatory cytokines and risk of myeloproliferative neoplasms (MR analysis method using IVW). A : Effects of 41 inflammatory cytokines on myeloproliferative tumors. B :Effects of myeloproliferative neoplasms on 41 inflammatory cytokines(* P < 0.05)
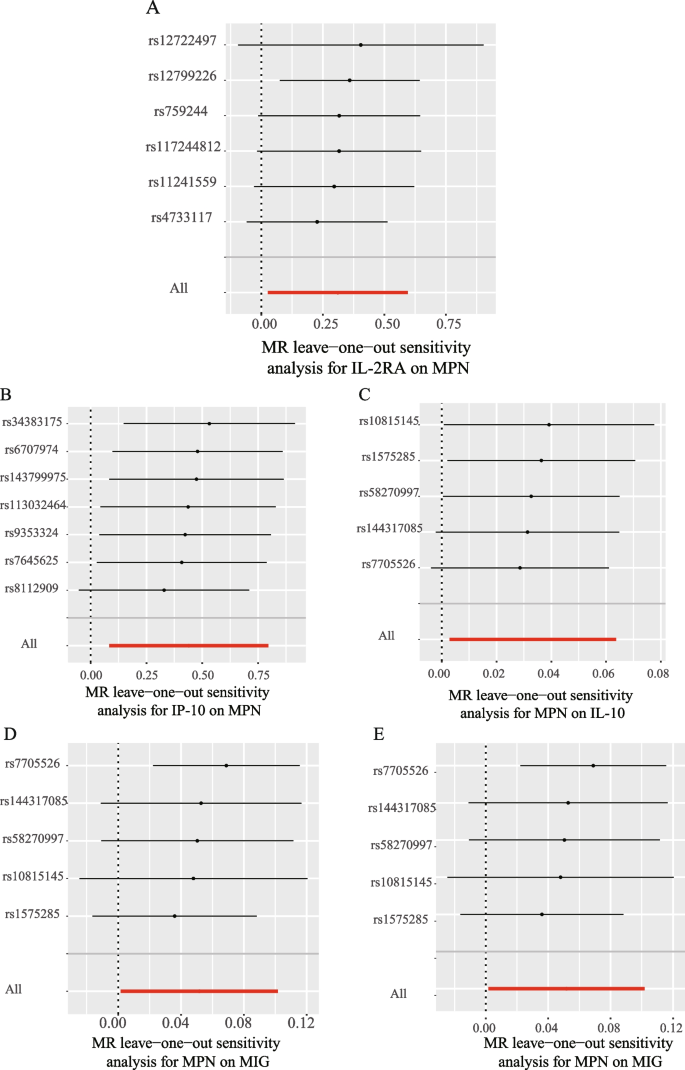
Leave-one-out analysis of bidirectional mendelian randomization in cytokines and MPN.The left side of the forest plot represents the SNPs for which the leave-one-out analysis was performed, and the short line parallel to the x-axis represents the 95% confidence interval for the OR/beta value of the MR analysis after excluding the corresponding SNPs. As shown in the figure, the overall error line does not change much after excluding each SNP, and all OR/beta values are on the 0 side, indicating that the results are reliable ( A ):Forest plots for the exposure of IL-2ra . B Forest plots for the exposure of IP-10 . C Forest plots for the outcome of IL-10 . D Forest plots for the outcome of MIG . E Forest plots for the outcome of RANTES
We also observed a suggestive association between genetically determined higher circulating IP-10 and a 55.3% increased risk of MPN (OR:1.553, 95% CI:1.088–2.216, P = 0.015), and it did not show heterogeneity (Cochrane Q test, P = 0.706)(Fig. 2 A); nor did it show directional pleiotropy (MR egger-intercept = 0.085, P for MR egger-intercept = 0.305, P for MR PRESSO global test = 0.668) (Supplementary Table 5 ). Sensitivity analysis was conducted using leave-one-out studies, and the results showed that no individual study had any impact (Fig. 3 B). We did not identify the SNP associated with other phenotypes on the Pheno-Scanner website, indicating that it does not increase the risk of MPN through the non-exposure pathway.
Effect of MPN on inflammatory cytokines
In analyzing the effect of MPN on inflammatory factors, We found a suggestive association between genetically predicted MPN and levels of the cytokines IL-10 , MIG , and RANTES . Genetically predicted MPN were suggestively associated with levels of interleukin-10 ( IL-10 ) (BETA = 0.033,95% CI = 0.003 ~ 0.064, P = 0.032) and Monokine induced by interferon-gamma ( MIG ) (BETA = 0.052,95% CI = 0.002–0.102, P = 0.043) and Regulated on activation, normal T Cell expressed and secreted ( RANTES ) (BETA = 0.055,95% CI = 0.009 − 0.1, P = 0.018) using IVW methods. It is worth paying attention to the fact that in the RANTES analysis, although the MR-egger results were not statistically significant, the direction of the MR-egger results was inconsistent with the IVW results, suggesting that the RANTES results may be unreliable (Fig. 2 B). In these findings, there was no indication of pleiotropy or heterogeneity. Supplementary Tables 4 and 6 provides a summary of the abovementioned findings. The leave-one-out analysis, meanwhile, revealed that all SNPs contributed to consistent causal estimates. (Fig. 3 C-E). The analysis mentioned above demonstrated the validity of the results.
The expression of IP-10 and IL-2ra in various subtypes of MPN and their diagnostic value
By analysing the effect of inflammatory cytokines on MPN, we found that higher levels of IP-10 , IL-2ra were associated with increased risk of MPN. However, due to data limitations, we did not have the opportunity to further analyse the effect of IP-10 , IL-2ra on the risk of developing each subtype of MPN using the MR approach. Therefore, in order to investigate the expression of IP-10 and IL-2ra in each subtype of MPN and their diagnostic value, we analyzed their expression in healthy individuals and the three subtypes of MPN and their diagnostic value based on data from the GEO database, as shown in Fig. 4 . Our results showed that IP-10 and IL-2ra were elevated in ET, PV, and MF compared with normal subjects. The highest diagnostic efficacy for MF was based on the expression of IP-10 and IL-2ra (IP10: AUC = 0.958, IL-2ra : AUC = 0.938); the areas under the ROC curves of IP-10 and IL-2ra in ET, PV, and MF were greater than 0.7, suggesting that IP-10 and IL-2ra may be used as potential diagnostic markers of MPN.
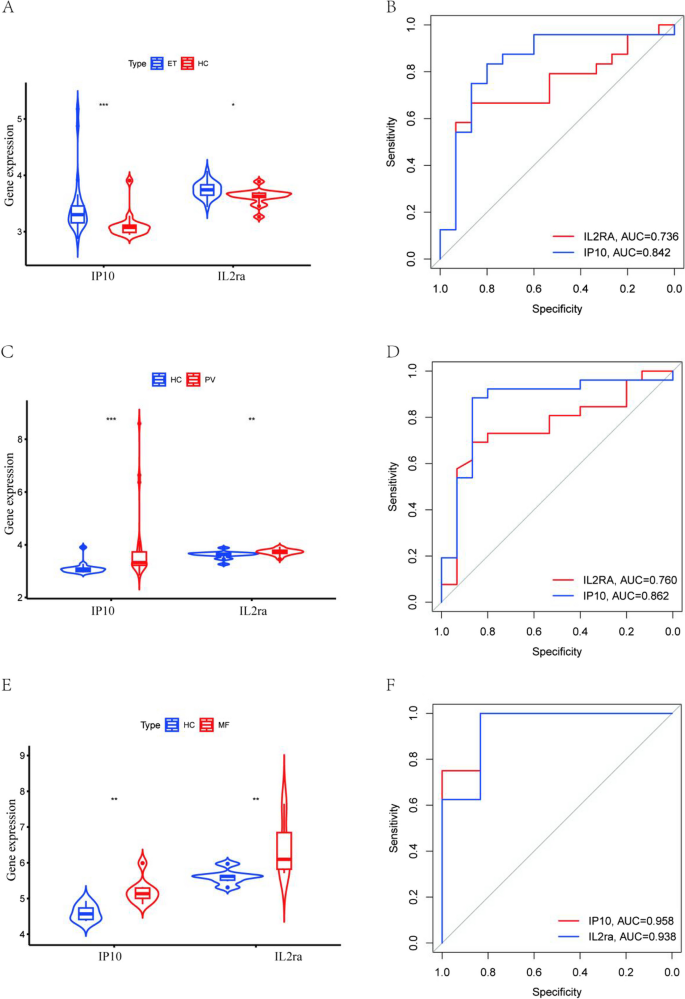
The GEO dataset analyses the expression of IP10 and IL2ra in each subtype of MPN and their diagnostic value.A: Expression of IP10 and IL2ra in ET and healthy donors; B: ROC curve of the prediction model based on IP10 and IL2ra to distinguish ET from healthy donors; C:IP10 and IL2ra expression in PV and healthy donors; D: ROC curve of the prediction model based on IP10 and IL2ra to distinguish PV from healthy donors; E:IP10 and IL2ra expression in MF and healthy donors; F: ROC curve of the prediction model based on IP10 and IL2ra to distinguish MF from healthy donors; *** P < 0.001, ** P < 0.01,* P < 0.05,HC: Healthy donors
Using publicly available pooled data from GWAS, we conducted a bidirectional two-sample MR analysis of the potential causative relationship between inflammatory cytokines and MPNs, and our study supported a causal association between inflammatory cytokines and MPNs. We found suggestive evidence that levels of the genetically predicted circulating cytokines IL-2rα , and IP-10 have a risk effect on MPNs. Reverse MR analysis found suggestive evidence of a positive causal effect of MPN on levels of the circulating cytokines IL-10 , MIG , and RANTES . These findings passed sensitivity analyses and were not affected by heterogeneity or horizontal pleiotropy. To our knowledge, this investigation is anticipated to be the broadest and most thorough MR evaluation of links between genetically inflammatory cytokines and MPN risk to date.
The CXC chemokine family member interferon gamma-induced protein 10 ( IP-10 ) is crucial for cell growth and proliferation [ 29 ]. IP-10 combines with the CXCR3 receptor, being a key driver in cancer and autoimmune regulation [ 30 ]. Several observational studies have demonstrated the presence of aberrant IP-10 expression in MPN patients, especially in PMF and PV, where IP-10 expression is significantly elevated [ 31 , 32 ]. Meanwhile, the serum level of IP-10 was also correlated with the disease progression of MPN [ 32 ]. Our MR analysis suggests that elevated IP-10 levels may contribute to MPN disease progression, which is consistent with results derived from observational studies. Previous basic research can explain our findings and the phenomena of observational studies in terms of pathogenesis. IP-10 expression is reported to be required for the activation of the JAK signaling pathway [ 33 ]and its level correlates with JAK2V617F status [ 9 , 34 ]. Therefore, JAK inhibition can reduce downstream chemokine IP-10 production by disrupting T cell-induced macrophage activation [ 35 ]. However, stromal cells in the microenvironment can protect MPN clonal cells from JAK2 inhibitors by secreting IP-10 , which can promote disease progression. These discoveries underscore the importance of researching IP-10 as a potential therapeutic target in the MPN tumor microenvironment and highlight the necessity of further studies on the exact mechanism of its role in MPN oncogenesis.
Our study also reveals a potential association between IL2rα and increased risk of MPN disease. IL2rα is an important component of IL-2R , a high-affinity receptor molecule highly expressed by activated T lymphocytes [ 36 ], and plays an important role in the regulation of T cell differentiation. Increasing IL-2rα expression on antigen-presenting cells (APCs) enhances the formation of memory T cells [ 37 ], and mutations in IL-2rα lead to decreased T cell function [ 38 ]. Accordingly, IL-2rα levels are associated with T-cell, B-cell, and immune system activation [ 36 ]. It has been demonstrated that conditions linked to cellular immune activation correlate with increased IL-2rα [ 39 , 40 ]. Additionally, a few observational studies have shown a connection between IL2r and MPN. Katerina et al. found that serum levels of IL-2rα were significantly elevated in patients with MPN compared to normal individuals [ 14 ], which was confirmed by further studies, where IL2rα levels were correlated with overall survival in patients with MF in MPN [ 41 ], and levels of IL-2ra in patients with MPN were positively correlated with disease progression and bone marrow angiogenesis [ 42 ]. According to the findings of our MR investigation, elevated levels of IL-2rα in the circulatory system may accelerate the development of MPN disease. This finding is not only consistent with the results of observational studies but also compensates for the shortcomings of small sample sizes and potential confounders in the observational studies mentioned above and provides more reliable evidence for the association between IL2rα and MPN at the level of genetics, emphasizing the importance and necessity of further investigating the role of IL2rα in the development of MPN disease.
Both our analyses and previous studies suggest that IP-10 and IL2rα may play an important role in MPN disease development. Considering the heterogeneity of the three subtypes of MPN, we sought to explore the effects of IP-10 and IL2rα on the disease risk of each subtype of MPN. Unfortunately, due to the limitation of the dataset, we had no way to further explore the relationship between IP-10 and IL2rα and different subtypes of MPN. Therefore, we initially analyzed the expression and diagnostic value of IP-10 and IL2rα in each subtype of MPN using the GEO database. We were surprised to find that IP-10 and IL2rα not only had elevated expression in the three subtypes of MPN compared to healthy individuals, but also had the potential to serve as independent biomarkers. This is consistent with our MR analysis that high expression of IP-10 , IL2rα increases the risk of MPN disease. This greatly encourages our confidence in further exploring the role of IL2rα , IP-10 in MPN at a later stage.
Positive MR analysis has revealed the role of inflammatory cytokines, particularly IP-10 and IL-2rα , in MPN disease progression. Indeed, MPN cells can also release large amounts of pro-inflammatory products, which in turn cause genomic instability and drive clonal myeloproliferation [ 43 , 44 ].To explore the effect of MPN disease on inflammatory cytokines, we performed a reverse MR analysis. The inverse MR analysis showed a potential positive correlation between genetically predicted MPN and the levels of cytokines IL-10 , MIG , and RANTES , and that MPN could promote slightly increased levels of the above cytokines. Inverse MR analysis revealed a potential positive correlation between genetically predicted MPN and levels of the cytokines IL-10 , MIG , and RANTES , with MPN promoting slightly elevated levels of the aforementioned cytokines, which is consistent with observational findings [ 9 , 45 , 46 , 47 ]. Our review of the literature revealed that aberrantly expressed IL-10 , MIG , and RANTES are all associated with premature atherosclerosis, a devastating consequence of chronic inflammation in the MPN [ 48 , 49 , 50 ].MIG binds to the receptor CXCR3 and not only participates in the recruitment of T cells to peripheral sites of inflammation [ 51 ]but also chemotactically recruits monocytes/macrophages to sites of inflammation. Activated inflammatory cells release pro-inflammatory factors to induce an inflammatory response [ 52 ], which promotes atherosclerosis. RANTES is one of the chemokines highly expressed upon platelet activation, and RANTES released by activated platelets facilitates the formation of atherosclerotic lesions by platelet-monocyte aggregation [ 53 , 54 ], and RANTES also regulates local inflammatory processes and atherosclerosis progression by mediating CD4 + T-cell homing [ 55 ]. It is interesting to note that IL-10 appears to be a protective factor against atherosclerosis, a common clinical symptom of MPN, and that, as an anti-inflammatory cytokine, IL-10 can attenuate atherosclerotic lesions by preventing dilation of inflamed areas, decreasing the size of plaques, and other mechanisms [ 56 ]. Specifically, IL-10 attenuates atherosclerotic lesions by inhibiting macrophage activation, as well as inhibiting the expression of matrix metalloproteinases, proinflammatory cytokines, and cyclooxygenase-2 in lipid-loaded and activated macrophage foam cells [ 57 , 58 ]. Therefore, it is necessary to investigate the correlation and mechanism between the elevated circulating levels of MPN-promoting inflammatory cytokines IL-10 , MIG , and RANTES and the common clinical complications of MPN, and to provide the possibility of targeting the above cytokines to alleviate the clinical complications.
Our study has several advantages. (1) The link between inflammatory cytokines and MPN risk is explained for the first time in magnetic resonance research. (2) Unlike observational studies, our study minimized confounders and reverse causality, providing a reliable causal relationship between MPN and inflammatory cytokines. (3) Our research data were sourced from the openly available GWAS database, which houses a significant volume of original research data, and thus gives this study a solid guarantee.
There are also some limitations to our study. In the first place, all participants in the dataset we used were of European ethnicity, which limits our ability to generalise our findings to other ethnicities. It is well known that Mendelian randomisation investigates the effect of genotypic variation (exposure) on phenotype (outcome) from a genetic perspective. Bias caused by confounding variables or reverse causality is avoided [ 18 ].In reality, however, it is well known that the level of gene expression determines the unique characteristics of a cell, that differences in disease prevalence between populations are associated with the frequency of alleles that regulate polymorphisms, and that differences in allele frequencies between racial groups have highly significant phenotypic consequences [ 59 ].Significant differences in gene expression phenotypes have been reported for at least 25 percent of genes between Europeans and Asians, and specific genetic variants (allele frequencies) between populations are the main cause of these differences [ 59 ].Therefore, in Mendelian randomisation analyses, genetic differences in quantitative phenotypes between different ethnic groups may be functionally equally important. Environmental, genetic, dietary, and lifestyle factors in different racial groups may influence phenotypic results [ 60 ], so we think it is unavoidable that the results of MR may differ between races due to residual confounding and selection bias [ 61 , 62 ].This is our limitation in this study. Therefore, whether elevated IP-10 and IL-2ra increase the risk of MPN prevalence in other populations requires specific analyses of gene expression variation for particular populations. Future studies will also need to enhance the analysis of gene expression variation between populations to improve understanding of the underlying genetics and population differences observed in complex genetic diseases. In the second, there are three subtypes of MPN, and due to the limitations of the GWAS dataset, we have not specifically stratified to explore the relationship between cytokines and the different subtypes of MPN. Finally, after Bonferroni correction, no cytokines showed statistically significant associations with MPN risk, and only two of them (IP-10, IL-2rα) showed suggestive associations.
In conclusion, our study suggests that elevated circulating levels of IP-10 and IL-2rα are associated with a high risk of MPN. Potential positive correlation between genetically predicted MPN and levels of the cytokines IL-10 , MIG , and RANTES . Our results show that cytokines play a significant role in the pathophysiology of MPN. Further research is required on the potential use of these biomarkers for the prevention and treatment of MPN.
Availability of data and materials
The datasets analyzed during the current study are available in the FinnGen repository, More details in FinnGen are described at https://r9.finngen.fi .
Grinfeld J, et al. Classification and Personalized Prognosis in Myeloproliferative Neoplasms. N Engl J Med. 2018;379(15):1416–30.
Article CAS PubMed PubMed Central Google Scholar
Tefferi A. Myeloproliferative neoplasms: A decade of discoveries and treatment advances. Am J Hematol. 2016;91(1):50–8.
Article CAS PubMed Google Scholar
Rungjirajittranon T, et al. A systematic review and meta-analysis of the prevalence of thrombosis and bleeding at diagnosis of Philadelphia-negative myeloproliferative neoplasms. BMC Cancer. 2019;19(1):184.
Article PubMed PubMed Central Google Scholar
Abdul-Rahim AH, et al. National institutes of health stroke scale item profiles as predictor of patient outcome: external validation on independent trial data. Stroke. 2015;46(2):395–400.
Article PubMed Google Scholar
Papageorgiou L, et al. Thrombotic and Hemorrhagic Issues Associated with Myeloproliferative Neoplasms. Clin Appl Thromb Hemost. 2022;28:10760296221097968.
Brkic S, Meyer SC. Challenges and Perspectives for Therapeutic Targeting of Myeloproliferative Neoplasms. Hemasphere. 2021;5(1):e516.
Vannucchi AM, et al. Ruxolitinib versus standard therapy for the treatment of polycythemia vera. N Engl J Med. 2015;372(5):426–35.
Verstovsek S, et al. Long-term treatment with ruxolitinib for patients with myelofibrosis: 5-year update from the randomized, double-blind, placebo-controlled, phase 3 COMFORT-I trial. J Hematol Oncol. 2017;10(1):55.
Cacemiro MDC, et al. Philadelphia-negative myeloproliferative neoplasms as disorders marked by cytokine modulation. Hematol Transfus Cell Ther. 2018;40(2):120–31.
Braun LM, Zeiser R. Immunotherapy in Myeloproliferative Diseases. Cells. 2020;9(6):1559.
Mantovani A, et al. Cancer-related inflammation. Nature. 2008;454(7203):436–44.
Mantovani A, et al. Interleukin-1 and Related Cytokines in the Regulation of Inflammation and Immunity. Immunity. 2019;50(4):778–95.
Nachbaur DM, et al. Serum levels of interleukin-6 in multiple myeloma and other hematological disorders: correlation with disease activity and other prognostic parameters. Ann Hematol. 1991;62(2–3):54–8.
Panteli KE, et al. Serum interleukin (IL)-1, IL-2, sIL-2Ra, IL-6 and thrombopoietin levels in patients with chronic myeloproliferative diseases. Br J Haematol. 2005;130(5):709–15.
Ramanathan G, Fleischman AG. The Microenvironment in Myeloproliferative Neoplasms. Hematol Oncol Clin North Am. 2021;35(2):205–16.
Hasselbalch HC. Chronic inflammation as a promotor of mutagenesis in essential thrombocythemia, polycythemia vera and myelofibrosis. A human inflammation model for cancer development? Leuk Res. 2013;37(2):214–20.
Smith GD, Ebrahim S. “Mendelian randomization”: can genetic epidemiology contribute to understanding environmental determinants of disease? Int J Epidemiol. 2003;32(1):1–22.
Davey Smith G, Hemani G. Mendelian randomization: genetic anchors for causal inference in epidemiological studies. Hum Mol Genet. 2014;23(R1):R89-98.
Davies N.M, Holmes M.V, Davey Smith G. Reading Mendelian randomisation studies: a guide, glossary, and checklist for clinicians. BMJ. 2018;362:k601.
Sun Y, et al. Assessing the causal role of hypertension on left atrial and left ventricular structure and function: A two-sample Mendelian randomization study. Front Cardiovasc Med. 2022;9:1006380.
Ahola-Olli A, et al. Genome-wide Association Study Identifies 27 Loci Influencing Concentrations of Circulating Cytokines and Growth Factors. Am J Hum Genet. 2017;100(1):40–50.
Zhang Y, et al. Causal Association Between Tea Consumption and Kidney Function: A Mendelian Randomization Study. Front Nutr. 2022;9:801591.
Bycroft C, et al. The UK Biobank resource with deep phenotyping and genomic data. Nature. 2018;562(7726):203–9.
Larsson SC, et al. Type 2 diabetes, glucose, insulin, BMI, and ischemic stroke subtypes: Mendelian randomization study. Neurology. 2017;89(5):454–60.
Bowden J, Hemani G, Davey Smith G. Invited Commentary: Detecting Individual and Global Horizontal Pleiotropy in Mendelian Randomization-A Job for the Humble Heterogeneity Statistic? Am J Epidemiol. 2018;187(12):2681–5.
PubMed PubMed Central Google Scholar
Verbanck M, et al. Detection of widespread horizontal pleiotropy in causal relationships inferred from Mendelian randomization between complex traits and diseases. Nat Genet. 2018;50(5):693–8.
Pierce BL, Ahsan H, Vanderweele TJ. Power and instrument strength requirements for Mendelian randomization studies using multiple genetic variants. Int J Epidemiol. 2011;40(3):740–52.
Chen X, et al. Depression and prostate cancer risk: A Mendelian randomization study. Cancer Med. 2020;9(23):9160–7.
Liu M, et al. CXCL10/IP-10 in infectious diseases pathogenesis and potential therapeutic implications. Cytokine Growth Factor Rev. 2011;22(3):121–30.
CAS PubMed PubMed Central Google Scholar
Karin N, Razon H. Chemokines beyond chemo-attraction: CXCL10 and its significant role in cancer and autoimmunity. Cytokine. 2018;109:24–8.
Obro NF, et al. Longitudinal Cytokine Profiling Identifies GRO-alpha and EGF as Potential Biomarkers of Disease Progression in Essential Thrombocythemia. Hemasphere. 2020;4(3):e371.
Tefferi A, et al. Circulating interleukin (IL)-8, IL-2R, IL-12, and IL-15 levels are independently prognostic in primary myelofibrosis: a comprehensive cytokine profiling study. J Clin Oncol. 2011;29(10):1356–63.
Schnoder TM, et al. Cell autonomous expression of CXCL-10 in JAK2V617F-mutated MPN. J Cancer Res Clin Oncol. 2017;143(5):807–20.
Allain-Maillet S, et al. Anti-Glucosylsphingosine Autoimmunity, JAK2V617F-Dependent Interleukin-1beta and JAK2V617F-Independent Cytokines in Myeloproliferative Neoplasms. Cancers (Basel). 2020;12(9):2446.
Nyirenda MH, et al. JAK inhibitors disrupt T cell-induced proinflammatory macrophage activation. RMD Open. 2023;9(1):e002671.
Waldmann TA. The structure, function, and expression of interleukin-2 receptors on normal and malignant lymphocytes. Science. 1986;232(4751):727–32.
Jones MC, et al. CD4 Effector TCR Avidity for Peptide on APC Determines the Level of Memory Generated. J Immunol. 2023;210(12):1950–61.
Picard C, Casanova JL. Inherited disorders of cytokines. Curr Opin Pediatr. 2004;16(6):648–58.
Semenzato G, et al. High serum levels of soluble interleukin 2 receptor in patients with B chronic lymphocytic leukemia. Blood. 1987;70(2):396–400.
Steis RG, et al. Serum soluble IL-2 receptor as a tumor marker in patients with hairy cell leukemia. Blood. 1988;71(5):1304–9.
Wang JC, Wang A. Plasma soluble interleukin-2 receptor in patients with primary myelofibrosis. Br J Haematol. 1994;86(2):380–2.
Bourantas KL, et al. Serum beta-2-microglobulin, TNF-alpha and interleukins in myeloproliferative disorders. Eur J Haematol. 1999;63(1):19–25.
Masselli E, et al. Protein kinase Cvarepsilon inhibition restores megakaryocytic differentiation of hematopoietic progenitors from primary myelofibrosis patients. Leukemia. 2015;29(11):2192–201.
Lussana F, Rambaldi A. Inflammation and myeloproliferative neoplasms. J Autoimmun. 2017;85:58–63.
Pourcelot E, et al. Cytokine profiles in polycythemia vera and essential thrombocythemia patients: clinical implications. Exp Hematol. 2014;42(5):360–8.
Gangemi S, et al. Evaluation of interleukin-23 plasma levels in patients with polycythemia vera and essential thrombocythemia. Cell Immunol. 2012;278(1–2):91–4.
Kleppe M, et al. JAK-STAT pathway activation in malignant and nonmalignant cells contributes to MPN pathogenesis and therapeutic response. Cancer Discov. 2015;5(3):316–31.
Li Q, et al. Activation of macrophage TBK1-HIF-1alpha-mediated IL-17/IL-10 signaling by hyperglycemia aggravates the complexity of coronary atherosclerosis: An in vivo and in vitro study. FASEB J. 2021;35(5):e21609.
Koenen RR, et al. Disrupting functional interactions between platelet chemokines inhibits atherosclerosis in hyperlipidemic mice. Nat Med. 2009;15(1):97–103.
Shi H, et al. CRISPR/Cas9 based blockade of IL-10 signaling impairs lipid and tissue homeostasis to accelerate atherosclerosis. Front Immunol. 2022;13:999470.
Park MK, et al. The CXC chemokine murine monokine induced by IFN-gamma (CXC chemokine ligand 9) is made by APCs, targets lymphocytes including activated B cells, and supports antibody responses to a bacterial pathogen in vivo. J Immunol. 2002;169(3):1433–43.
Koper OM, et al. CXCL9, CXCL10, CXCL11, and their receptor (CXCR3) in neuroinflammation and neurodegeneration. Adv Clin Exp Med. 2018;27(6):849–56.
von Hundelshausen P, et al. RANTES deposition by platelets triggers monocyte arrest on inflamed and atherosclerotic endothelium. Circulation. 2001;103(13):1772–7.
Article Google Scholar
Huo Y, et al. Circulating activated platelets exacerbate atherosclerosis in mice deficient in apolipoprotein E. Nat Med. 2003;9(1):61–7.
Ley K. Role of the adaptive immune system in atherosclerosis. Biochem Soc Trans. 2020;48(5):2273–81.
Xu S, et al. The role of interleukin-10 family members in cardiovascular diseases. Int Immunopharmacol. 2021;94:107475.
Hansson GK. Immune mechanisms in atherosclerosis. Arterioscler Thromb Vasc Biol. 2001;21(12):1876–90.
Han X, Boisvert WA. Interleukin-10 protects against atherosclerosis by modulating multiple atherogenic macrophage function. Thromb Haemost. 2015;113(3):505–12.
Spielman RS, et al. Common genetic variants account for differences in gene expression among ethnic groups. Nat Genet. 2007;39(2):226–31.
Zhao Y, et al. Associations between type 2 diabetes mellitus and chronic liver diseases: evidence from a Mendelian ranldomization study in Europeans and East Asians. Front Endocrinol (Lausanne). 2024;15:1338465.
Au Yeung SL, et al. Evaluating the role of non-alcoholic fatty liver disease in cardiovascular diseases and type 2 diabetes: a Mendelian randomization study in Europeans and East Asians. Int J Epidemiol. 2023;52(3):921–31.
Ford I, et al. The inverse relationship between alanine aminotransferase in the normal range and adverse cardiovascular and non-cardiovascular outcomes. Int J Epidemiol. 2011;40(6):1530–8.
Download references
Acknowledgements
We appreciate all investigators for sharing these data in our study.
This study was supported by Commissioned by the National Clinical Medical Research Center for Hematological Diseases (grant no. 2021WWA01) and the Construction of the clinical medical research center of the Gansu Science and Technology project (grant no. 21JR7RA435) and the Gansu Province Science and technology project natural science foundation (grant no. 21JR11RA104) and Lanzhou science and technology development plan project(grant no. 2020-ZD-99) and The Second Hospital of Lanzhou University "Cui-ying Science and Technology Innovation" Program (grant no. 2020QN-13).
Author information
Authors and affiliations.
Department of Hematology, The Second Hospital of Lanzhou University, Lanzhou, China
Hao Xiong, Jun Bai, Yanhong Li, Lijuan Li & Liansheng Zhang
Department of Hematology, The Affiliated Hospital of Southwest Medical University, Luzhou, China
Hao Xiong & Huitao Zhang
You can also search for this author in PubMed Google Scholar
Contributions
LSZ and LJL conceived, designed, and supervised the whole study. HX,HTZ performed the analyses. JB and YHL interpreted the results, and contributed in this work in study design, data interpretation, and manuscript writing.HX and HTZ wrote the manuscript. All authors provided critical comments and approved the final manuscript.
Corresponding authors
Correspondence to Lijuan Li or Liansheng Zhang .
Ethics declarations
Ethics approval and consent for participate.
Not applicable.
Consent for publication
The authors have given consent for publication.
Competing interests
The authors declare no competing interests.
Additional information
Publisher’s note.
Springer Nature remains neutral with regard to jurisdictional claims in published maps and institutional affiliations.
Supplementary Information
Supplementary material 1, supplementary material 2, supplementary material 3, supplementary material 4, supplementary material 5, supplementary material 6, rights and permissions.
Open Access This article is licensed under a Creative Commons Attribution 4.0 International License, which permits use, sharing, adaptation, distribution and reproduction in any medium or format, as long as you give appropriate credit to the original author(s) and the source, provide a link to the Creative Commons licence, and indicate if changes were made. The images or other third party material in this article are included in the article's Creative Commons licence, unless indicated otherwise in a credit line to the material. If material is not included in the article's Creative Commons licence and your intended use is not permitted by statutory regulation or exceeds the permitted use, you will need to obtain permission directly from the copyright holder. To view a copy of this licence, visit http://creativecommons.org/licenses/by/4.0/ . The Creative Commons Public Domain Dedication waiver ( http://creativecommons.org/publicdomain/zero/1.0/ ) applies to the data made available in this article, unless otherwise stated in a credit line to the data.
Reprints and permissions
About this article
Cite this article.
Xiong, H., Zhang, H., Bai, J. et al. Associations of the circulating levels of cytokines with the risk of myeloproliferative neoplasms: a bidirectional mendelian-randomization study. BMC Cancer 24 , 531 (2024). https://doi.org/10.1186/s12885-024-12301-x
Download citation
Received : 17 August 2023
Accepted : 22 April 2024
Published : 26 April 2024
DOI : https://doi.org/10.1186/s12885-024-12301-x
Share this article
Anyone you share the following link with will be able to read this content:
Sorry, a shareable link is not currently available for this article.
Provided by the Springer Nature SharedIt content-sharing initiative
- Mendelian randomization
- Inflammatory
ISSN: 1471-2407
- Submission enquiries: [email protected]
- General enquiries: [email protected]
The Federal Register
The daily journal of the united states government, request access.
Due to aggressive automated scraping of FederalRegister.gov and eCFR.gov, programmatic access to these sites is limited to access to our extensive developer APIs.
If you are human user receiving this message, we can add your IP address to a set of IPs that can access FederalRegister.gov & eCFR.gov; complete the CAPTCHA (bot test) below and click "Request Access". This process will be necessary for each IP address you wish to access the site from, requests are valid for approximately one quarter (three months) after which the process may need to be repeated.
An official website of the United States government.
If you want to request a wider IP range, first request access for your current IP, and then use the "Site Feedback" button found in the lower left-hand side to make the request.
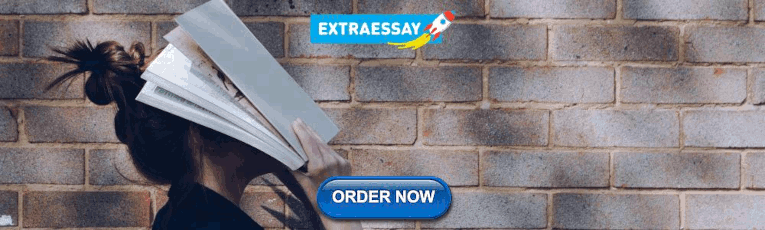
COMMENTS
So, follow the steps below to write a research summary that sticks. 1. Read the parent paper thoroughly. You should go through the research paper thoroughly multiple times to ensure that you have a complete understanding of its contents. A 3-stage reading process helps.
Research Summary. Definition: A research summary is a brief and concise overview of a research project or study that highlights its key findings, main points, and conclusions. It typically includes a description of the research problem, the research methods used, the results obtained, and the implications or significance of the findings.
The better you understand a subject, the easier it is to explain it thoroughly and briefly. Write a first draft. Use the same order as in the article itself. Adjust the length accordingly depending on the content of your particular article and how you will be using the summary. • State the research question and explain why it is interesting.
Table of contents. When to write a summary. Step 1: Read the text. Step 2: Break the text down into sections. Step 3: Identify the key points in each section. Step 4: Write the summary. Step 5: Check the summary against the article. Other interesting articles. Frequently asked questions about summarizing.
The results section of a quantitative research paper is where you summarize your data and report the findings of any relevant statistical analyses. The APA manual provides rigorous guidelines for what to report in quantitative research papers in the fields of psychology, education, and other social sciences.
Here are a few best practices: Your results should always be written in the past tense. While the length of this section depends on how much data you collected and analyzed, it should be written as concisely as possible. Only include results that are directly relevant to answering your research questions.
Draft Summary of Findings: Draft a paragraph or two of discussion for each finding in your study. Assert the finding. Tell the reader how the finding is important or relevant to your studies aim and focus. Compare your finding to the literature. Be specific in the use of the literature. The link or connection should be clear to the reader.
A summary should be written objectively and in a way that covers the article in sufficient detail—accurately yet briefly—to allow a reader to quickly absorb its significance. 3.1 Do some groundwork. Skim the article to get a rough idea of each section and the significance of the content. Read the paper in more depth.
Summarize each source: Determine the most important and relevant information from each source, such as the findings, methodology, theories, etc. Consider using an article summary, or study summary to help you organize and summarize your sources. ... Appraise issues or factors associated with your professional practice and research topic. Help ...
The results chapter (also referred to as the findings or analysis chapter) is one of the most important chapters of your dissertation or thesis because it shows the reader what you've found in terms of the quantitative data you've collected. It presents the data using a clear text narrative, supported by tables, graphs and charts.
Traditionally, medical research has been integrated in the narrative or nonsystematic form. An expert in a particular field will review studies, decided on the relevance, and highlight the findings, both in terms of results and, to a lesser degree the methodology.4 Such narrative reviews tend to be unsystematic and susceptible to many biases ...
Some summaries can even be as short as one sentence. 3. State the research question and hypothesis. To begin your summary, start by summarizing what the authors aim to answer and what their hypothesis was. You can combine both the question and the hypothesis into one short sentence to open up your summary.
'Summary of findings' tables present the main findings of a review in a transparent, structured and simple tabular format. In particular, they provide key information concerning the certainty or quality of evidence (i.e. the confidence or certainty in the range of an effect estimate or an association), the magnitude of effect of the ...
Empirical paper: Summarize your findings. In an empirical paper, this is the time to summarize your key findings. Don't go into great detail here (you will have presented your in-depth results and discussion already), but do clearly express the answers to the research questions you investigated.
Research findings can be used in a variety of situations, depending on the context and the purpose. Here are some examples of when research findings may be useful: Decision-making: Research findings can be used to inform decisions in various fields, such as business, education, healthcare, and public policy.
Microsoft Word - summarizing.doc. Summarizing a Research Article. Research articles use a standard format to clearly communicate information about an experiment. A research article usually has seven major sections: Title, Abstract, Introduction, Method, Results, Discussion, and References. Sometimes there are minor variations, such as a ...
One of the most important skills you can imbibe as an academician is to know how to summarize a research paper. During your academic journey, you may need to write a summary of findings in research quite often and for varied reasons - be it to write an introduction for a peer-reviewed publication, to submit a critical review, or to simply create a useful database for future referencing.
Basics of summarizing research findings . Science is based on many different pillars that combine to provide the methods of reasoning, logic, and ethics to conduct research.
Step 1: Consult the guidelines or instructions that the target journal or publisher provides authors and read research papers it has published, especially those with similar topics, methods, or results to your study. The guidelines will generally outline specific requirements for the results or findings section, and the published articles will ...
In the conclusion, you should summarize the key findings you have taken from the literature and emphasize their significance. Tip Be sure to show how your research addresses gaps and contributes new knowledge, or discuss how you have drawn on existing theories and methods to build a framework for your research.
Pew Research Center conducted this analysis to look at changing marriage rates among 40-year-olds in the United States from 1850 to 2021. This analysis uses decennial census data and the American Community Survey (ACS). The ACS is the largest household survey in the U.S., with a sample of more than 3 million addresses.
Don't make the reader do the analytic work for you. Now, on to some specific ways to structure your findings section. 1). Tables. Tables can be used to give an overview of what you're about to present in your findings, including the themes, some supporting evidence, and the meaning/explanation of the theme.
As the summary statistics from published research are made available to the public, the institutional review board did not need to approve our study's ethics further. ... Supplementary Tables 4 and 6 provides a summary of the abovementioned findings. The leave-one-out analysis, meanwhile, revealed that all SNPs contributed to consistent causal ...
Summary of Key Findings . April 2024 . Introduction . In April 2020, Cornell suspended the requirement for SAT/ACT exam scores for undergraduate applications for admission. This was largely a response to the logistical difficulties accessing testing locations with the onset of the COVID pandemic, but the decision also eased some concerns
Step 1: Summarize your key findings. Start this section by reiterating your research problem and concisely summarizing your major findings. To speed up the process you can use a summarizer to quickly get an overview of all important findings. Don't just repeat all the data you have already reported—aim for a clear statement of the overall result that directly answers your main research ...
SUMMARY: Notice is hereby given of the availability of final evaluation findings for seven State and territory coastal management programs ( i.e., Alabama, Connecticut, Guam, Michigan, Ohio, Texas, and Virginia), and four national estuarine research reserves ( i.e.,