
Snapsolve any problem by taking a picture. Try it in the Numerade app?

- Why Does Water Expand When It Freezes
- Gold Foil Experiment
- Faraday Cage
- Oil Drop Experiment
- Magnetic Monopole
- Why Do Fireflies Light Up
- Types of Blood Cells With Their Structure, and Functions
- The Main Parts of a Plant With Their Functions
- Parts of a Flower With Their Structure and Functions
- Parts of a Leaf With Their Structure and Functions
- Why Does Ice Float on Water
- Why Does Oil Float on Water
- How Do Clouds Form
- What Causes Lightning
- How are Diamonds Made
- Types of Meteorites
- Types of Volcanoes
- Types of Rocks
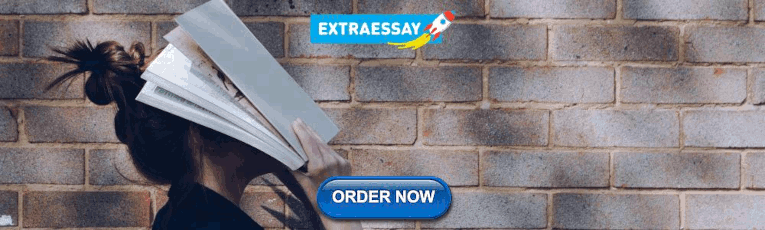
Global Wind Patterns and Wind Belts
There are several kinds of wind blowing across the surface of the Earth, depending on the origin, destination, and distance traveled, among other factors. These are called prevailing or planetary winds. Global winds are one such type of wind.
Global winds are winds that develop in belts distributed all around the world. Like local winds, the leading cause of global winds is unequal heating of the atmosphere, causing a difference in air pressure.
What Causes Global Wind Patterns
Earth is hottest at the Equator and cooler toward the poles. In the area near the Equator, the sun is overhead for most of the year. As a result, the warm air rises and moves towards the poles. This phenomenon creates a low-pressure zone near the equatorial area called the Equatorial Low-Pressure Zone, also known as the Intertropical Convergence Zone (ITCZ). At the polar front, the cooler air sinks and moves back towards the Equator.
Almost 30° north and 30° south of the Equator, this air cools and descends to create high-pressure zones called Subtropical High-Pressure Zones. Cold air at the poles descends and creates Polar High-Pressure Zones. The cold polar air moves at about 60° north and south towards the Equator and starts rising.
This unique, permanent movement of winds across the surface of the Earth due to permanent air pressure zones creates a distinct pattern known as global wind patterns or global wind systems.
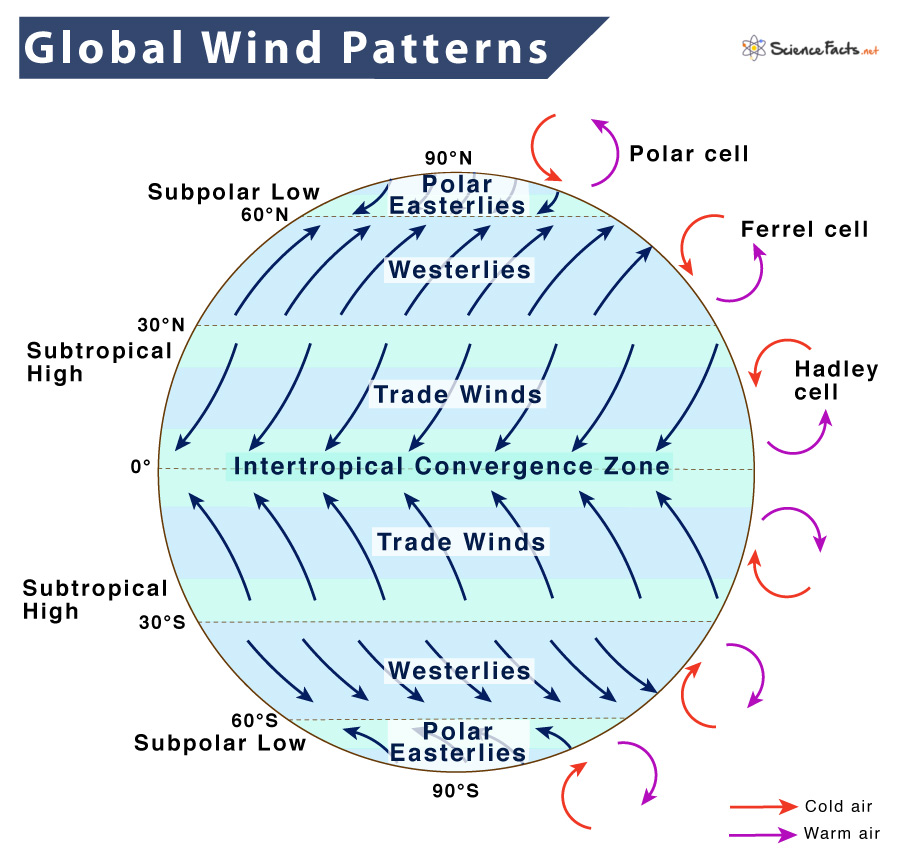
However, global winds do not move directly from north to south or south to north due to the rotation of the Earth. All winds in the Northern Hemisphere curves to the right as they move. Contrastingly, winds appear to curve to the left in the southern hemisphere. This apparent shift in the path of the wind moving about the Earth’s surface due to the Earth’s rotation is called the Coriolis Effect.
The global movement of air on the Earth in the three atmospheric convection cells is summarized below:
Hadley Cell | 0° to 30° latitude north and south | Rising at the Equator, sinking at 0° to 30° north and south | Towards the Equator | Tropical Easterlies (Trade Winds) |
Ferrel Cell | 30° to 60° latitude north and south | Rising at 60° north and south, sinking at 30° north and south | Towards the poles | Prevailing Westerlies |
Polar Cell | 60° to 90° latitude north and south | Rising at 60° north and south, sinking at 90° north and south | Towards the Equator | Polar Easterlies |
What Factors Contribute to Global Winds
Thus the pattern of global winds is affected by:
- Unequal heating of the Earth
- High and low-pressure areas (Pressure Gradients)
- Coriolis Effect
- Convection Cells
What are Global Wind Belts
As we now know, three types of prevailing winds on the Earth form three major global wind belts created due to the Coriolis Effect. They are named based on their origin and the area where they blow. We will now describe them in more detail.
1. Tropical Easterlies (Trade Winds)
Location – 0° to 30° Latitude
They are persistent winds that blow westward and toward the Equator from the subtropical high-pressure belt. Trade Winds finally converge at an area near the ITCZ, producing a narrow band of clouds, and thunderstorms encircle portions of the globe. It is more robust and consistent over the oceans than the land, often producing partly cloudy sky conditions characterized by shallow cumulus clouds or clear skies.
Most tropical storms, including hurricanes, cyclones, and typhoons, develop as trade winds.
2. Westerlies
Location – 30° to 60° Latitude
They are moist prevailing winds that blow from the subtropical high-pressure belts towards sub-polar low-pressure belts. Westerlies come from the southwest in the Northern Hemisphere and the northwest in the Southern Hemisphere.
Westerlies are essential in carrying the warm, equatorial winds to the western coasts of continents.
3. Polar Easterlies
Location – 60° to 90° Latitude
They are dry, cold prevailing winds that blow from the high-pressure areas of the polar highs in both hemispheres. They flow towards low-pressure areas within the Westerlies at high latitudes. Cold air subsides at the pole creating high pressure, creating a northward flow of wind towards the Equator in the southern hemisphere. The flow is then deflected westward by the Coriolis Effect. Thus, these prevailing winds blow from the east to the west.
Since the winds originate in the east, they are then known as easterlies.
How Do Global Winds Affect Climate
The global wind belts are associated with typical weather conditions, and the prevailing winds lead to seasonal climatic changes in many places on Earth. All low-pressure areas are associated with high precipitation (tropical rainforests), and all high-pressure areas are associated with arid climates (deserts).
Jet Streams and Global Wind Belts
The polar jet stream or the jet stream blows high up in the atmosphere where the two cells meet. They are fast-moving air currents at the boundary between the troposphere and the stratosphere.
They also develop due to the unequal heating of the Earth’s surface, creating a vast temperature difference between two air masses. Jet streams circle the planet, mainly from west to east, but the flow often shifts to the north and south. The polar jets are the strongest jet streams that blow between about 30°N and 50° to 75°N. They blow towards the south in the winter and north in the summer.
Ans . No. Deep currents are caused by the moon’s gravity, the Earth’s rotation, and the movement of the tectonic plates.
Ans . Since prevailing westerlies dominate most of the United States, they blow from the southwest.
- Global Wind Belts – Flexbooks.ck12.org
- Global Pressure Belts and Prevailing Winds – Study.com
- Global Wind Definition – Study.com
- Global Wind Patterns – Fau.edu
- Global Wind Explained – E-education.psu.edu
Article was last reviewed on Thursday, February 2, 2023
Related articles

Leave a Reply Cancel reply
Your email address will not be published. Required fields are marked *
Save my name, email, and website in this browser for the next time I comment.
Popular Articles
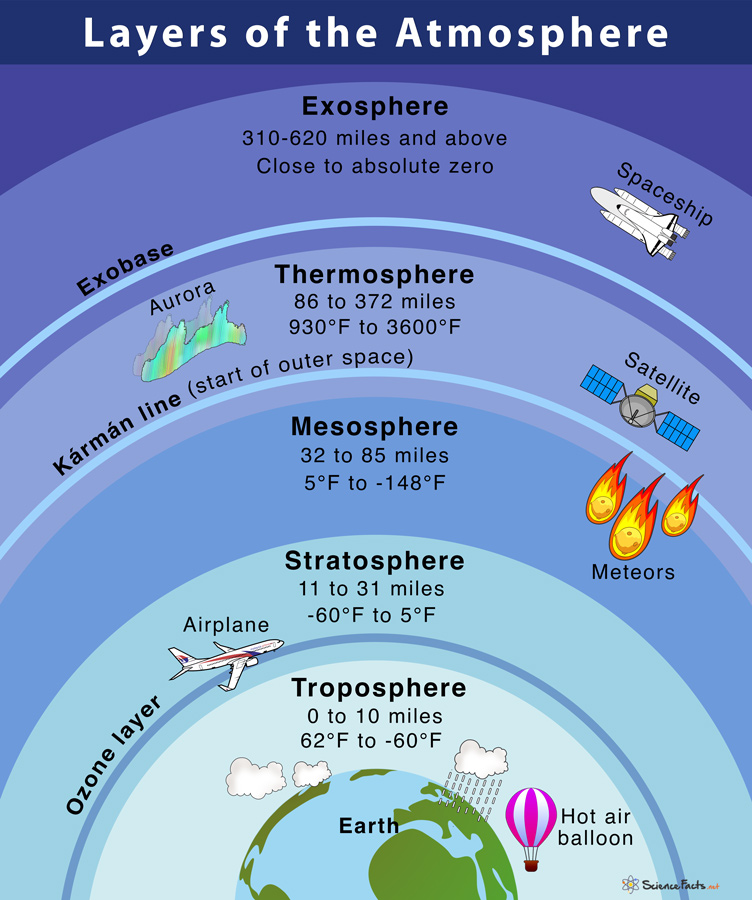
Join our Newsletter
Fill your E-mail Address
Related Worksheets
- Privacy Policy
© 2024 ( Science Facts ). All rights reserved. Reproduction in whole or in part without permission is prohibited.
SIMPLE - Rollovers -

Explanation
- U.S. Locations
- UMGC Europe
- Learn Online
- Find Answers
- 855-655-8682
- Current Students
Online Guide to Writing and Research Chapter 3: Thinking Strategies and Writing Patterns
Explore more of umgc.
- Online Guide to Writing
Introduction
Kinds of Assignments You Will Write
Developing a Paper Using Strategies
- General-to-Specific Pattern
- Specific-to-General Pattern
- Problem-Cause-Solution Pattern
- Discussing Raw Data
- Summaries and Abstracts
Supporting with Research and Examples
- Read and Understand the Question
- Organize Thinking Before Writing
- Make Your Answer Relevant and Complete
- Style Through Vocabulary and Diction
Mailing Address: 3501 University Blvd. East, Adelphi, MD 20783 This work is licensed under a Creative Commons Attribution-NonCommercial-ShareAlike 4.0 International License . © 2022 UMGC. All links to external sites were verified at the time of publication. UMGC is not responsible for the validity or integrity of information located at external sites.
Table of Contents: Online Guide to Writing
Chapter 1: College Writing
How Does College Writing Differ from Workplace Writing?
What Is College Writing?
Why So Much Emphasis on Writing?
Chapter 2: The Writing Process
Doing Exploratory Research
Getting from Notes to Your Draft
Prewriting - Techniques to Get Started - Mining Your Intuition
Prewriting: Targeting Your Audience
Prewriting: Techniques to Get Started
Prewriting: Understanding Your Assignment
Rewriting: Being Your Own Critic
Rewriting: Creating a Revision Strategy
Rewriting: Getting Feedback
Rewriting: The Final Draft
Techniques to Get Started - Outlining
Techniques to Get Started - Using Systematic Techniques
Thesis Statement and Controlling Idea
Writing: Getting from Notes to Your Draft - Freewriting
Writing: Getting from Notes to Your Draft - Summarizing Your Ideas
Writing: Outlining What You Will Write
Chapter 3: Thinking Strategies
A Word About Style, Voice, and Tone
A Word About Style, Voice, and Tone: Style Through Vocabulary and Diction
Critical Strategies and Writing
Critical Strategies and Writing: Analysis
Critical Strategies and Writing: Evaluation
Critical Strategies and Writing: Persuasion
Critical Strategies and Writing: Synthesis
Patterns for Presenting Information
Patterns for Presenting Information: Critiques
Patterns for Presenting Information: Discussing Raw Data
Patterns for Presenting Information: General-to-Specific Pattern
Patterns for Presenting Information: Problem-Cause-Solution Pattern
Patterns for Presenting Information: Specific-to-General Pattern
Patterns for Presenting Information: Summaries and Abstracts
Writing Essay Examinations
Writing Essay Examinations: Make Your Answer Relevant and Complete
Writing Essay Examinations: Organize Thinking Before Writing
Writing Essay Examinations: Read and Understand the Question
Chapter 4: The Research Process
Planning and Writing a Research Paper
Planning and Writing a Research Paper: Ask a Research Question
Planning and Writing a Research Paper: Cite Sources
Planning and Writing a Research Paper: Collect Evidence
Planning and Writing a Research Paper: Decide Your Point of View, or Role, for Your Research
Planning and Writing a Research Paper: Draw Conclusions
Planning and Writing a Research Paper: Find a Topic and Get an Overview
Planning and Writing a Research Paper: Manage Your Resources
Planning and Writing a Research Paper: Outline
Planning and Writing a Research Paper: Survey the Literature
Planning and Writing a Research Paper: Work Your Sources into Your Research Writing
Research Resources: Where Are Research Resources Found? - Human Resources
Research Resources: What Are Research Resources?
Research Resources: Where Are Research Resources Found?
Research Resources: Where Are Research Resources Found? - Electronic Resources
Research Resources: Where Are Research Resources Found? - Print Resources
Structuring the Research Paper: Formal Research Structure
Structuring the Research Paper: Informal Research Structure
The Nature of Research
The Research Assignment: How Should Research Sources Be Evaluated?
The Research Assignment: When Is Research Needed?
The Research Assignment: Why Perform Research?
Chapter 5: Academic Integrity
Academic Integrity
Giving Credit to Sources
Giving Credit to Sources: Copyright Laws
Giving Credit to Sources: Documentation
Giving Credit to Sources: Style Guides
Integrating Sources
Practicing Academic Integrity
Practicing Academic Integrity: Keeping Accurate Records
Practicing Academic Integrity: Managing Source Material
Practicing Academic Integrity: Managing Source Material - Paraphrasing Your Source
Practicing Academic Integrity: Managing Source Material - Quoting Your Source
Practicing Academic Integrity: Managing Source Material - Summarizing Your Sources
Types of Documentation
Types of Documentation: Bibliographies and Source Lists
Types of Documentation: Citing World Wide Web Sources
Types of Documentation: In-Text or Parenthetical Citations
Types of Documentation: In-Text or Parenthetical Citations - APA Style
Types of Documentation: In-Text or Parenthetical Citations - CSE/CBE Style
Types of Documentation: In-Text or Parenthetical Citations - Chicago Style
Types of Documentation: In-Text or Parenthetical Citations - MLA Style
Types of Documentation: Note Citations
Chapter 6: Using Library Resources
Finding Library Resources
Chapter 7: Assessing Your Writing
How Is Writing Graded?
How Is Writing Graded?: A General Assessment Tool
The Draft Stage
The Draft Stage: The First Draft
The Draft Stage: The Revision Process and the Final Draft
The Draft Stage: Using Feedback
The Research Stage
Using Assessment to Improve Your Writing
Chapter 8: Other Frequently Assigned Papers
Reviews and Reaction Papers: Article and Book Reviews
Reviews and Reaction Papers: Reaction Papers
Writing Arguments
Writing Arguments: Adapting the Argument Structure
Writing Arguments: Purposes of Argument
Writing Arguments: References to Consult for Writing Arguments
Writing Arguments: Steps to Writing an Argument - Anticipate Active Opposition
Writing Arguments: Steps to Writing an Argument - Determine Your Organization
Writing Arguments: Steps to Writing an Argument - Develop Your Argument
Writing Arguments: Steps to Writing an Argument - Introduce Your Argument
Writing Arguments: Steps to Writing an Argument - State Your Thesis or Proposition
Writing Arguments: Steps to Writing an Argument - Write Your Conclusion
Writing Arguments: Types of Argument
Appendix A: Books to Help Improve Your Writing
Dictionaries
General Style Manuals
Researching on the Internet
Special Style Manuals
Writing Handbooks
Appendix B: Collaborative Writing and Peer Reviewing
Collaborative Writing: Assignments to Accompany the Group Project
Collaborative Writing: Informal Progress Report
Collaborative Writing: Issues to Resolve
Collaborative Writing: Methodology
Collaborative Writing: Peer Evaluation
Collaborative Writing: Tasks of Collaborative Writing Group Members
Collaborative Writing: Writing Plan
General Introduction
Peer Reviewing
Appendix C: Developing an Improvement Plan
Working with Your Instructor’s Comments and Grades
Appendix D: Writing Plan and Project Schedule
Devising a Writing Project Plan and Schedule
Reviewing Your Plan with Others
By using our website you agree to our use of cookies. Learn more about how we use cookies by reading our Privacy Policy .
Have a language expert improve your writing
Run a free plagiarism check in 10 minutes, generate accurate citations for free.
- Knowledge Base
- Starting the research process
- Writing Strong Research Questions | Criteria & Examples
Writing Strong Research Questions | Criteria & Examples
Published on October 26, 2022 by Shona McCombes . Revised on November 21, 2023.
A research question pinpoints exactly what you want to find out in your work. A good research question is essential to guide your research paper , dissertation , or thesis .
All research questions should be:
- Focused on a single problem or issue
- Researchable using primary and/or secondary sources
- Feasible to answer within the timeframe and practical constraints
- Specific enough to answer thoroughly
- Complex enough to develop the answer over the space of a paper or thesis
- Relevant to your field of study and/or society more broadly
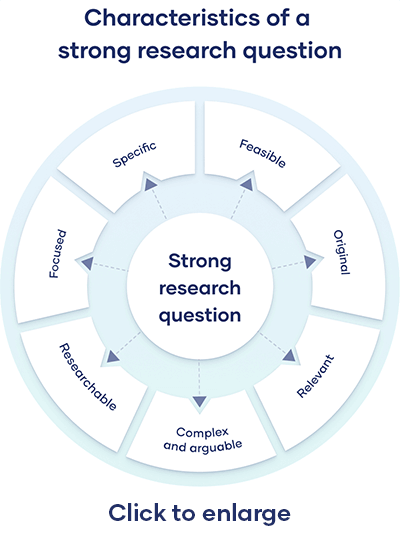
Table of contents
How to write a research question, what makes a strong research question, using sub-questions to strengthen your main research question, research questions quiz, other interesting articles, frequently asked questions about research questions.
You can follow these steps to develop a strong research question:
- Choose your topic
- Do some preliminary reading about the current state of the field
- Narrow your focus to a specific niche
- Identify the research problem that you will address
The way you frame your question depends on what your research aims to achieve. The table below shows some examples of how you might formulate questions for different purposes.
Research question formulations | |
---|---|
Describing and exploring | |
Explaining and testing | |
Evaluating and acting | is X |
Using your research problem to develop your research question
Example research problem | Example research question(s) |
---|---|
Teachers at the school do not have the skills to recognize or properly guide gifted children in the classroom. | What practical techniques can teachers use to better identify and guide gifted children? |
Young people increasingly engage in the “gig economy,” rather than traditional full-time employment. However, it is unclear why they choose to do so. | What are the main factors influencing young people’s decisions to engage in the gig economy? |
Note that while most research questions can be answered with various types of research , the way you frame your question should help determine your choices.
Prevent plagiarism. Run a free check.
Research questions anchor your whole project, so it’s important to spend some time refining them. The criteria below can help you evaluate the strength of your research question.
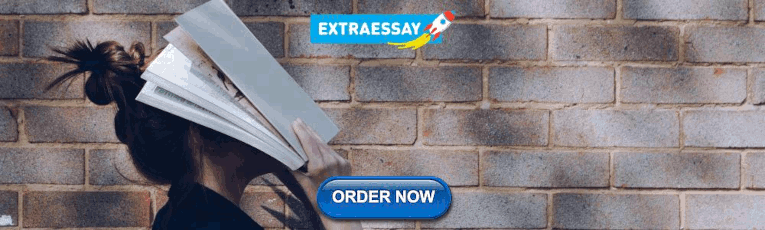
Focused and researchable
Criteria | Explanation |
---|---|
Focused on a single topic | Your central research question should work together with your research problem to keep your work focused. If you have multiple questions, they should all clearly tie back to your central aim. |
Answerable using | Your question must be answerable using and/or , or by reading scholarly sources on the to develop your argument. If such data is impossible to access, you likely need to rethink your question. |
Not based on value judgements | Avoid subjective words like , , and . These do not give clear criteria for answering the question. |
Feasible and specific
Criteria | Explanation |
---|---|
Answerable within practical constraints | Make sure you have enough time and resources to do all research required to answer your question. If it seems you will not be able to gain access to the data you need, consider narrowing down your question to be more specific. |
Uses specific, well-defined concepts | All the terms you use in the research question should have clear meanings. Avoid vague language, jargon, and too-broad ideas. |
Does not demand a conclusive solution, policy, or course of action | Research is about informing, not instructing. Even if your project is focused on a practical problem, it should aim to improve understanding rather than demand a ready-made solution. If ready-made solutions are necessary, consider conducting instead. Action research is a research method that aims to simultaneously investigate an issue as it is solved. In other words, as its name suggests, action research conducts research and takes action at the same time. |
Complex and arguable
Criteria | Explanation |
---|---|
Cannot be answered with or | Closed-ended, / questions are too simple to work as good research questions—they don’t provide enough for robust investigation and discussion. |
Cannot be answered with easily-found facts | If you can answer the question through a single Google search, book, or article, it is probably not complex enough. A good research question requires original data, synthesis of multiple sources, and original interpretation and argumentation prior to providing an answer. |
Relevant and original
Criteria | Explanation |
---|---|
Addresses a relevant problem | Your research question should be developed based on initial reading around your . It should focus on addressing a problem or gap in the existing knowledge in your field or discipline. |
Contributes to a timely social or academic debate | The question should aim to contribute to an existing and current debate in your field or in society at large. It should produce knowledge that future researchers or practitioners can later build on. |
Has not already been answered | You don’t have to ask something that nobody has ever thought of before, but your question should have some aspect of originality. For example, you can focus on a specific location, or explore a new angle. |
Chances are that your main research question likely can’t be answered all at once. That’s why sub-questions are important: they allow you to answer your main question in a step-by-step manner.
Good sub-questions should be:
- Less complex than the main question
- Focused only on 1 type of research
- Presented in a logical order
Here are a few examples of descriptive and framing questions:
- Descriptive: According to current government arguments, how should a European bank tax be implemented?
- Descriptive: Which countries have a bank tax/levy on financial transactions?
- Framing: How should a bank tax/levy on financial transactions look at a European level?
Keep in mind that sub-questions are by no means mandatory. They should only be asked if you need the findings to answer your main question. If your main question is simple enough to stand on its own, it’s okay to skip the sub-question part. As a rule of thumb, the more complex your subject, the more sub-questions you’ll need.
Try to limit yourself to 4 or 5 sub-questions, maximum. If you feel you need more than this, it may be indication that your main research question is not sufficiently specific. In this case, it’s is better to revisit your problem statement and try to tighten your main question up.
If you want to know more about the research process , methodology , research bias , or statistics , make sure to check out some of our other articles with explanations and examples.
Methodology
- Sampling methods
- Simple random sampling
- Stratified sampling
- Cluster sampling
- Likert scales
- Reproducibility
Statistics
- Null hypothesis
- Statistical power
- Probability distribution
- Effect size
- Poisson distribution
Research bias
- Optimism bias
- Cognitive bias
- Implicit bias
- Hawthorne effect
- Anchoring bias
- Explicit bias
The way you present your research problem in your introduction varies depending on the nature of your research paper . A research paper that presents a sustained argument will usually encapsulate this argument in a thesis statement .
A research paper designed to present the results of empirical research tends to present a research question that it seeks to answer. It may also include a hypothesis —a prediction that will be confirmed or disproved by your research.
As you cannot possibly read every source related to your topic, it’s important to evaluate sources to assess their relevance. Use preliminary evaluation to determine whether a source is worth examining in more depth.
This involves:
- Reading abstracts , prefaces, introductions , and conclusions
- Looking at the table of contents to determine the scope of the work
- Consulting the index for key terms or the names of important scholars
A research hypothesis is your proposed answer to your research question. The research hypothesis usually includes an explanation (“ x affects y because …”).
A statistical hypothesis, on the other hand, is a mathematical statement about a population parameter. Statistical hypotheses always come in pairs: the null and alternative hypotheses . In a well-designed study , the statistical hypotheses correspond logically to the research hypothesis.
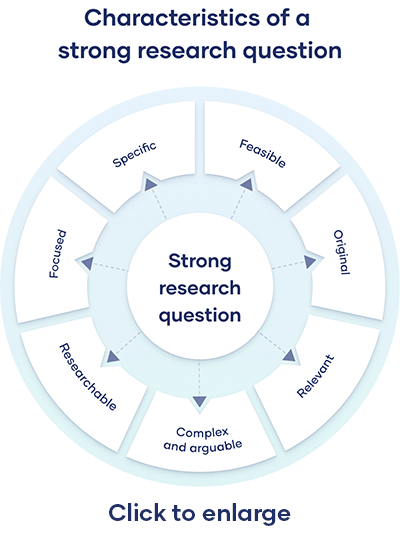
Formulating a main research question can be a difficult task. Overall, your question should contribute to solving the problem that you have defined in your problem statement .
However, it should also fulfill criteria in three main areas:
- Researchability
- Feasibility and specificity
- Relevance and originality
Cite this Scribbr article
If you want to cite this source, you can copy and paste the citation or click the “Cite this Scribbr article” button to automatically add the citation to our free Citation Generator.
McCombes, S. (2023, November 21). Writing Strong Research Questions | Criteria & Examples. Scribbr. Retrieved August 29, 2024, from https://www.scribbr.com/research-process/research-questions/
Is this article helpful?
Shona McCombes
Other students also liked, how to define a research problem | ideas & examples, how to write a problem statement | guide & examples, 10 research question examples to guide your research project, what is your plagiarism score.

There are no comments.

- Search Menu
- Sign in through your institution
- Author Guidelines
- Submission Site
- Open Access
- Why Submit?
- About Journal of Legal Analysis
- Editorial Board
- Advertising and Corporate Services
- Journals Career Network
- Self-Archiving Policy
- Journals on Oxford Academic
- Books on Oxford Academic
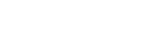
Article Contents
1. introduction, 2. dark patterns in the wild, 3. testing dark patterns’ effectiveness through experiments, 4. are dark patterns unlawful, 5. conclusion.
- < Previous
Shining a Light on Dark Patterns
- Article contents
- Figures & tables
- Supplementary Data
Jamie Luguri, Lior Jacob Strahilevitz, Shining a Light on Dark Patterns, Journal of Legal Analysis , Volume 13, Issue 1, 2021, Pages 43–109, https://doi.org/10.1093/jla/laaa006
- Permissions Icon Permissions
Dark patterns are user interfaces whose designers knowingly confuse users, make it difficult for users to express their actual preferences, or manipulate users into taking certain actions. They typically exploit cognitive biases and prompt online consumers to purchase goods and services that they do not want or to reveal personal information they would prefer not to disclose. This article provides the first public evidence of the power of dark patterns. It discusses the results of the authors’ two large-scale experiments in which representative samples of American consumers were exposed to dark patterns. In the first study, users exposed to mild dark patterns were more than twice as likely to sign up for a dubious service as those assigned to the control group, and users in the aggressive dark pattern condition were almost four times as likely to subscribe. Moreover, whereas aggressive dark patterns generated a powerful backlash among consumers, mild dark patterns did not. Less educated subjects were significantly more susceptible to mild dark patterns than their well-educated counterparts. The second study identified the dark patterns that seem most likely to nudge consumers into making decisions that they are likely to regret or misunderstand. Hidden information, trick question, and obstruction strategies were particularly likely to manipulate consumers successfully. Other strategies employing loaded language or generating bandwagon effects worked moderately well, while still others such as “must act now” messages did not make consumers more likely to purchase a costly service. Our second study also replicated a striking result in the first experiment, which is that where dark patterns were employed the cost of the service offered to consumers became immaterial. Decision architecture, not price, drove consumer purchasing decisions. The article concludes by examining legal frameworks for addressing dark patterns. Many dark patterns appear to violate federal and state laws restricting the use of unfair and deceptive practices in trade. Moreover, in those instances where consumers enter into contracts after being exposed to dark patterns, their consent could be deemed voidable under contract law principles. The article also proposes that dark pattern audits become part of the Federal Trade Commission (FTC)’s consent decree process. Dark patterns are presumably proliferating because firms’ proprietary A-B testing has revealed them to be profit maximizing. We show how similar A-B testing can be used to identify those dark patterns that are so manipulative that they ought to be deemed unlawful.
Everybody has seen them before and found them frustrating, but most consumers don’t know what to call them. They are what computer scientists and user-experience (UX) designers have (for the last decade) described as dark patterns , 1 and they are a proliferating species of sludge (to use a term preferred by behavioral economists) ( Sunstein 2019 , p. 1843; Thaler 2018 , p. 431) or market manipulation (the moniker preferred by some legal scholars) ( Calo 2014 , p. 995; Hanson & Kysar 1999 , p. 632). Dark patterns are user interfaces whose designers knowingly confuse users, make it difficult for users to express their actual preferences, or manipulate users into taking certain actions. They typically prompt users to rely on System 1 decision-making rather than more deliberate System 2 processes, exploiting cognitive biases like framing effects, the sunk cost fallacy, and anchoring. The goal of most dark patterns is to manipulate the consumer into doing something that is inconsistent with her preferences, in contrast to marketing efforts that are designed to alter those preferences. The first wave of academic research into dark patterns identified the phenomenon and developed a typology of dark pattern techniques ( Bösch et al. 2016 ; Gray et al. 2018 ).
Computer scientists at Princeton and the University of Chicago recently took a second step toward tackling the problem by releasing the first major academic study of the prevalence of dark patterns ( Mathur et al. 2019 ). Arunesh Mathur and six co-authors developed a semi-automated method for crawling more than 11,000 popular shopping websites. Their analysis revealed the presence of dark patterns on more than 11 percent of those sites, and the most popular sites were also most likely to employ dark patterns. Dark patterns appear to be especially prevalent in mobile apps, with recent research by Di Geronimo and co-authors identifying them on 95percent of the free Android apps they analyzed in the US Google Play Store ( Di Geronimo et al. 2020 ).
If the first wave of scholarship created a useful taxonomy and the second step in the scholarship established the growing prevalence of dark pattern techniques, it seems clear where the literature ought to go next. Scholars need to quantify the effectiveness of dark patterns in convincing online consumers to do things that they would otherwise prefer not to do. In short, the question we pose in this article is “how effective are dark patterns?” That is not a question that has been answered in academic research to date. 2 But it is a vital inquiry if we are to understand the magnitude of the problem and whether regulation is appropriate.
To be sure, the lack of published research does not mean that the effectiveness of these techniques is a complete mystery. On the contrary, we suspect that the kind of research results we report here have been replicated by social scientists working in-house for technology and e-commerce companies. Our hunch is that consumers are seeing so many dark patterns in the wild because the internal, proprietary research suggests dark patterns generate profits for the firms that employ them. But those social scientists have had strong incentives to suppress the results of their A-B testing of dark patterns, so as to preserve data about the successes and failures of the techniques as trade secrets and (perhaps) to stem the emergence of public outrage and legislative or regulatory responses.
Bipartisan legislation that would constrain the use of dark patterns is currently pending in the Senate ( Deceptive Experiences to Online Users Reduction Act (DETOUR Act), Senate Bill 1084, 116th Congress, introduced April 9, 2019 ), and investigative reporters are beginning to examine the problem of dark patterns ( Valentino-Devries 2019 ). E-commerce firms probably expect that, where the effectiveness of dark patterns is concerned, heat will follow light. So they have elected to keep the world in the dark for as long as possible. The strategy has worked so far.
The basic problem of manipulation in marketing and sales is not unique to interactions between computers and machines. The main factors that make this context interesting are its relative newness, scale, and the effectiveness of the techniques. In both traditional and online contexts, legal actors have to make tough decisions about where the precise line is between persuasion and manipulation, and which conduct is misleading enough to eliminate what might otherwise be constitutionally protected rights for sellers to engage in commercial speech. The law has long elected to prohibit certain strategies for convincing people to part with money or personal information. Laws prohibiting fraud have been around, seemingly forever, and more recently implemented laws proscribe pretexting. States and the federal government have given consumers special rights in settings characterized by high pressure, mild coercion, or vulnerability, such as door-to-door-sales, and transactions involving funeral services, timeshares, telemarketing, or home equity loans. Sometimes the law enacts outright prohibitions with substantial penalties. Other times it creates cooling-off periods that cannot be waived. A key question we address is what online tactics are egregious enough to warrant this kind of special skepticism.
Ours is a descriptive article, an empirical article, a normative article, and then a descriptive article again. That said, the new experimental data we reveal are the most important takeaway. Section 2 begins by describing dark patterns—what techniques they include and what some of the most prominent examples are. The description illuminates several real-world dark patterns and suites of dark patterns employed by major multinational corporations.
Section 3 provides the article’s core contribution. As scholars have seen the proliferation of dark patterns, many have assumed that dark patterns are efficacious. Why else would large, well-capitalized companies that are known to engage in A-B testing be rolling them out? Judges confronting dark patterns have for the most part shared these intuitions, though not universally. We report the results of two large-scale experiments on census-weighted samples of American adults. In the first experiment, we show that many widely employed dark patterns prompt consumers to do what they would not do in a more neutral decision-making environment. But beyond that, we provide the first comparative evidence that quantifies how well they work, shining some light on the question of which techniques work best. Our bottom line is that dark patterns are strikingly effective in getting consumers to do what they would not do when confronted with more neutral user interfaces . Relatively mild dark patterns more than doubled the percentage of consumers who signed up for a dubious identity theft protection service, which we told our subjects we were selling, and aggressive dark patterns nearly quadrupled the percentage of consumers signing up. In social science terms, the magnitudes of these treatment effects are enormous. We then provide powerful evidence that dosage matters—aggressive dark patterns generate a powerful customer backlash whereas mild dark patterns usually do not. Therefore, counterintuitively, the strongest case for regulation and other legal interventions concern subtle uses of dark patterns. We provide compelling evidence that less educated Americans are significantly more vulnerable to dark patterns than their more educated counterparts, and that trend is particularly pronounced where subtler dark patterns are concerned. This observation raises distributive issues and is also useful as we consider how the law might respond to dark patterns.
Our second experiment builds upon these initial results and allowed us to isolate those dark patterns that substantially alter consumer decisions to purchase a service from those that have no significant effect. We found that the most effective dark pattern strategies were hidden information (smaller print in a less visually prominent location), obstruction (making users jump through unnecessary hoops to reject a service), trick questions (intentionally confusing prompts), and social proof (efforts to generate a bandwagon effect). Other effective strategies included loaded questions and making acceptance the default. By contrast, countdown timers that sought to convince consumers that the ability to purchase a service was time-limited and would disappear shortly did not significantly increase consumer purchases, nor did labeling acceptance of the offered service as the “recommended” choice. The second experiment also showed that very substantial increases in the price of the service did not dampen the effects of the dark pattern. In many cases, consumers exposed to dark patterns did not understand that they had signed up for a costly service. These results confirm the problematic nature of dark patterns and can help regulators and other watchdogs establish enforcement priorities.
Section 4 looks at the existing law and asks whether it prohibits dark patterns. This is an important area for inquiry because pending bipartisan legislation proposes that the Federal Trade Commission (FTC) be given new authority to prohibit dark patterns. It turns out that with respect to a number of central dark pattern techniques, the FTC is already going after some kinds of dark patterns, and the federal courts have been happy to cheer the agency along. The most successful actions have nearly all fallen under the FTC’s Section five authority to regulate deceptive acts and practices in trade. To be sure, other important dark patterns fit less comfortably within the categories of deceptive or misleading trade practices, and there is lingering uncertainty as to how much the FTC’s authority to restrict unfair trade practices will empower the agency to restrict that behavior. The passage of federal legislation aimed squarely at dark patterns would provide useful new legal tools, but there is no reason to delay enforcement efforts directed at egregious dark patterns while waiting on Congress to do something.
Of course, the FTC lacks the resources to be everywhere, so a critical issue going forward will be whether contracts that are agreed to in large measure because of a seller’s use of dark patterns are deemed valid. This issue is just now starting to bubble up in the case law. In our view, the FTC and courts interpreting contract claims should view the most successful dark patterns as the most legally problematic ones, while also taking into account the social harms associated with the implementation of a dark pattern. Scholarship that helps identify which dark patterns are best at thwarting user preferences therefore can help legal institutions establish enforcement priorities and draw lines between permitted and prohibited conduct. As we explain in section 4, there is a plausible case to be made that agreements procured through the use of dark patterns are voidable as a matter of contract law under the undue influence doctrine.
We said at the outset that dark patterns are different from other forms of dodgy business practices because of the scale of e-commerce. There may be poetic justice in the fact that this very scale presents an opportunity for creative legal regulators. It is exceedingly difficult to figure out whether a door-to-door salesperson’s least savory tactics significantly affected the chances of a purchase—was the verbal sleight of hand material or incidental? Who knows? But with e-commerce, firms run thousands of consumers through identical interfaces at a reasonable cost and see how small software tweaks might alter user behavior. Social scientists working in academia or for the government can do this too; we just haven’t done so before today. Now that scholars can test dark patterns, we can isolate causation in a way that’s heretofore been impossible in the brick-and-mortar world. Unlike brick-and-mortar manipulation, dark patterns are hiding in plain sight, operate on a massive scale, and are relatively easy to detect. Those facts strengthen the case further for the legal system to address their proliferation.
So let us spend some time getting to know dark patterns.
Suppose you are getting commercial emails from a company and wish to unsubscribe. If the company is following the law they will include in their emails a link to the page that allows you to remove your email address. 3 Some companies make that process simple, automatically removing your address when you click on an unsubscribe link or taking you to a page that asks you to type in your email address to unsubscribe. Once you do so they will stop sending you emails.
Other companies will employ various tools to try to keep you on their lists. They may remind you that if you unsubscribe, you will lose valuable opportunities to save money on their latest products (dark patterns researchers call this practice “confirmshaming”). Or they will give you a number of options besides the full unsubscribe that most people presumably want, such as “receive emails from us once a week” or “receive fewer emails from us” while making users who want to receive no more emails click through to a subsequent page. 4 (These techniques are referred to as “obstruction” dark patterns) ( Gray et al. 2018 ; Strahilevitz et al. 2019 , pp. 22–23). The company is making it easy for you to do what it prefers (you continue to receive lots of marketing emails), and harder for you to do the thing it can live with (receiving fewer emails) or the thing you probably prefer and are entitled to by law (receiving no emails from the company).

Alexander Hamilton was generally depicted clean-shaven in portraits. Other than that, it is not clear what the connection is between the ticket purchase and a razor blade subscription. Notice further that besides bait and switch, there are two subtler aesthetic manipulation dark patterns embedded in the image above. In the ad, “Yes please” appears against a bright blue background while “No thanks” appears less legible against a gray one. Moreover, another even lighter gray font (barely legible in the pop-up ad) reveals important text about what a consumer has to click on to skip the several bait-and-switch ads that would follow, which in this case included further offers from Hotels.com, Priceline, and Hulu. The font appears much less prominently than the darker text above it about the razor blade offer.
Another common dark pattern is generating false or misleading messages about demand for products or testimonials. Arunesh Mathur and co-authors recently revealed that a number of popular shopping sites display information about recent sales activities that are driven by random number generators and similar techniques. For example, they caught thredup.com using a random number generator to display information about how many of a particular product were “just sold” in the last hour, and they found various sports jersey sales sites using identically phrased customer testimonials but with different customer names each time ( Mathur et al. 2019 , p. 19). When a site notes that Anna in Anchorage just purchased a jacket that a user is examining, the academic research suggests these high-demand messages may well be phony.
Having introduced a few vivid examples of dark patterns, it seems appropriate to identify a workable taxonomy of the techniques. Several have been developed in the existing literature. One problem is that as interest in dark patterns has grown, so has the ostensible list of what counts as one. Putting together the various taxonomies in the literature results in a rather lengthy list, with some techniques being very problematic and others less so. There have been four key taxonomies to emerge in the dark patterns literature, with each building on and tweaking what came before. The chart below reproduces the current aggregated taxonomy in the literature and identifies which types of dark patterns have been identified in multiple taxonomies against only some. 6 Our literature review reveals eight categories of dark patterns and twenty-seven variants. 7 After presenting this information we will propose a modified, streamlined taxonomy that appropriately focuses on the means (the manipulative techniques used) rather than the ends (getting users to provide sensitive information, cash, recruit others, etc.). It is worth noting at the outset that some of the choices different teams of scholars have made in presenting their taxonomies relate to their different objectives. For example, some scholars, like Bösch et al., are not trying to be comprehensive. Others, like Mathur et al., are focusing on the sorts of dark patterns that can be identified using a semi-automated, web-crawling process. Such processes lend themselves to flagging certain kinds of dark patterns (such as low-stock messages) more readily than others (such as toying with emotions).
A common theme is that dark patterns manipulate consumers by altering online choice architecture in ways that are designed to thwart users’ preferences for objectionable ends. They make it possible but asymmetrically difficult for a user to act in a manner consistent with her preferences, often by prompting impulsive System 1 decision-making and discouraging deliberative System 2 decision-making. Thus, a dark pattern is defined with respect to users’ actual preferences but may necessitate some judgment about social welfare. This latter feature helps differentiate dark patterns from nudges, which may use similar techniques to foster prosocial behavior like organ donation or retirement saving ( Thaler & Sunstein 2009 ).
A useful approach to characterizing dark patterns recently was developed in Mathur, Mayer & Kshirsagar 2020 . They note that dark patterns often employ strategies that render the ease of selecting different options asymmetric, cover up the mechanisms by which consumers are influenced, deceive users through acts or omissions, hide relevant information, or restrict choices likely to be popular among consumers ( id ., p. 3). They further examine the possible normative criteria for deeming dark patterns problematic, including that they may undermine individual welfare (prompting decisions that hurt consumers or will spark regret), reduce collective welfare (by, for example, promoting anticompetitive behavior or eroding trust in markets), or reduce autonomy by exploiting weaknesses and cognitive biases ( id ., pp. 6–8). Mathur, Mayer, and Kshirsagar helpfully suggest that what ultimately tie together dark patterns conceptually are Wittgensteinian familial resemblances, such that there are close connections but a crisp definition of the concept may prove elusive ( id ., p. 3). In their opinion, then, identifying dark patterns seems to depend on both means and ends. We adopt their framework here.
Now that we have identified ways to make progress on conceptual issues, we shall conclude this section with an examination of the state of the art dark patterns being employed by a single influential corporation. As gaming platforms have become a major source of revenue, the dominant platforms have sought to profit off the increased appeal of online gaming. Online gaming allows individuals to play over the Internet against friends or strangers, and both Sony and Microsoft have made major investments in this technology. One strategy that is widely employed in popular games makes it necessary for players to sign up for online platforms in order to earn the most appealing available rewards. One of the authors has a child who enjoys EA’s FIFA soccer games on the Sony PlayStation, and to that end, the author signed up for a short-term subscription to PlayStation Plus—Sony’s online gaming platform. In the next few paragraphs, we will use Sony’s user interface as a case study of dark patterns.

Several notable aspects of the user interface stand out. First, notice the visual prominence of the twelve-month subscription rather than the alternatives in the default view. This “false hierarchy” graphic design approach is a kind of dark pattern. Choice architects have long understood that contrasting visual prominence can be used to nudge choosers effectively into a choice the architect prefers. The visual contrast is one of the least subtle and presumably more benign dark patterns that can be encountered in the wild. Unlike many dark patterns identified above, it is almost certainly a legal marketing tactic when used in isolation. 8
Now let us consider Sony’s pricing strategy. It is no mystery what Sony is trying to do. It wants to minimize customer churn. Acquiring subscribers is quite costly, so Sony wants to retain them once they obtain them. Moreover, some customers may subscribe for a year because of the lower per-month rate ($5 per month versus $10 per month) and then grow bored with the service—these customers are good for Sony because they will be paying for network resources that they do not use, which will improve the experience for other paying customers. It is akin to people joining a gym as a New Year’s resolution and then never showing up after January to use the treadmills. One way to get customers to commit to a longer term is by substantially discounting the monthly cost for customers who are willing to sign up for a year’s membership. In this instance, Sony is willing to charge such customers half as much as customers who will only commit to subscribing for a month. To be clear, there is nothing legally wrong with Sony pursuing this pricing model itself and (at least from our perspective) there is not anything morally dubious about the practice either, at least not yet. The pricing model is not a dark pattern.

So customers who sign up for a one-month membership at $10 per month will have that membership automatically renewed, at twice the monthly cost of customers who sign up for a twelve-month membership. Presumably, a tiny fraction of one-month subscribers prefers autorenewal at a high monthly rate. But never fear, as the figure above shows, Sony will let those customers opt-out of automatic renewal, provided they click through … at least five screens—Settings, Account Managements, Account Information, Wallet, and Purchase Settings, where they will see a button that lets them toggle off autorenewal. 9 A user who neither writes down the precise opt-out instructions nor takes a digital photograph of the screen above will be lost at sea—the different steps a user must go through are far from intuitive.
A cynical observer might view Sony as furthering two objectives here. First, Sony knows that a number of their one-month subscribers will be auto-renewed at a high monthly rate, and that is a lucrative source of revenue for the company. Second, Sony knows that some of its customers will grasp immediately how difficult opting out of automatic renewal is, think it through a bit, and then press cancel. Presumably most will then sign up for the twelve-month subscription that Sony probably prefers, whose automatic renewal feature is less blatantly problematic. Either way, Sony comes out ahead.

The initial set-up and very difficult process for opting out of autorenewal at the outset seem to be a conscious and intentional choice by Sony. If we examine what Sony is doing through the lens of existing taxonomies we can see that it is combining several tactics that have been identified as dark patterns.
In this instance, Sony is combining a false hierarchy (the different sizes of the buttons on the initial screen), the bait and switch (the one-month subscription looks like it offers an easy way to decline the product after the user experiences it, but given user inertia it is often an indefinite subscription with a higher monthly rate), preselection (the default choice is good for the company and bad for most one-month subscription consumers) 12 , a roach motel (opting out of automatic renewal is far more difficult and time-consuming than keeping automatic renewal), and forced continuity (many users will wind up paying for the service for a lengthy period of time despite their initial intent not to do so). These dark patterns are used in combination, seemingly in an effort to manipulate users into either a long-term subscription or an automatically renewing indefinite subscription at a high monthly rate.
To review, there are a variety of dark patterns that are designed to nudge consumers into contractual arrangements that they presumably would not otherwise prefer, and these techniques appear to be employed by a variety of different e-commerce firms, from start-up apps to well-capitalized platforms like Ticketmaster and Sony. Ticketmaster and Sony have a lot of smart people who work for them, so presumably they are doing what they are doing because it is good for the firms’ bottom lines. But beyond that intuition we lack reliable information about the effectiveness of these dark patterns in nudging consumers to behave in ways that maximize firm profits. Turning to Section 2 of our article, which is the heart of the project, we will now attempt to fill that gap in the literature. In order to do that, we created a classic “bait-and-switch” scenario with a large sample of Americans online.
Let us suppose Amazon or Microsoft was interested in testing the effectiveness of dark patterns. It would be easy to do so using their existing platform. They have ongoing relationships with millions of customers, and many of them have already stored their credit card information to enable one-click purchasing. So they could beta-test different dark patterns on subsets of their user-base, exploiting randomization and then track purchases and revenue to see what works. The risks of customer/employee blowback or legal liability would be the main constraints on what the companies could do.
For academics seeking to test the efficacy of dark patterns, the challenge is much more significant. Academic researchers generally do not have established relationships with customers (students aside, and that relationship is heavily regulated where financial aid and tuition payment are concerned). The point of a dark pattern typically is to manipulate people to pay for something they otherwise would not purchase or surrender personal information they would otherwise keep confidential. There has been a little bit of academic work that has studied how different user interfaces can encourage the latter ( Brandimarte, Acquisti & Loewensteinl 2012 , p. 340; John, Acquisti & Loewenstein 2011 , p. 858; Junger, Montoya & Overink, 2017 , p. 75), and none on the former. Because we are most interested in understanding how effective dark patterns are at parting consumers with their money, we wanted to situate ourselves in Amazon or Microsoft’s shoes to the fullest extent possible. Alas, setting up a new e-commerce platform to run those experiments was prohibitively expensive.
To that end, we designed a bait-and-switch scenario that would strike consumers as plausible. We would use an existing survey research firm to recruit large populations of American adults to participate in research studies that would evaluate their attitudes about privacy. Then we would deceive those adults into believing, at the end of the survey, that because they expressed a strong interest in privacy (as respondents typically do in surveys), we had signed them up for a costly identity theft protection service and would give them the opportunity to opt-out. We would structure the experiment in a way so as to make experimental subjects believe that their own money was at stake and they would need to pay for the service if they did not opt-out. Then we would randomly vary whether the opportunity to opt-out was impeded by different dosages or types of dark patterns. This manipulation would plausibly generate information about consumers’ revealed preferences, and it would allow us to do so without actually selling any goods or services to consumers. Our host university’s IRB approved our proposals to engage in the deceptive experiment after we explained, among other things: (i) that we would not actually store any of the information that we were purportedly collecting to uniquely identify our experimental subjects, and (ii) that we would promptly debrief participants at the end of the survey so they understood they would not be charged any money for our non-existent identity theft protection service.
3.1 Study One: Aggressive versus Mild Dark Patterns
To put that research plan in motion, we first administered an online survey to a nationally representative (census weighted) sample of American participants recruited by Dynata, a professional survey research firm. We removed respondents who took too long or too little time to complete the survey from the sample, as well as those who failed an attention check. 13 This left a final sample of 1,963 participants. 14 Participants were compensated by Dynata for their time, and we compensated Dynata. We pre-registered the experiment with AsPredicted.Org. 15
To begin, study participants answered various demographic questions including age, gender, race, education, income, employment, political orientation, and region of the country. Included with these basic demographic questions were additional questions aimed to bolster the later cover story that we had pinpointed their mailing address. Specifically, participants were asked their zip code, how long they had lived at their current residence, their telephone number, and where they were completing the survey (home, work, or other). In order to preserve confidentiality, these responses were deleted from the dataset and were not analyzed.
Next, we assessed subjects’ attitudes and opinions on data privacy. Though not the focus of the present article, this section consisted of us asking participants about what companies either should be allowed to do or are allowed to do with consumer data. These questions focused on data collection, retention, third-party sharing, and encryption. We collected responses to a battery of information privacy questions. This data collection allowed us to create a cover story for offering the participant identity theft protection.
Answering these questions took up most of respondents’ time. In the last few minutes of the survey, they were exposed to a manipulation designed to assess the effectiveness of dark patterns. The first part of the survey provided us with an appropriate pretext for what followed. Respondents were told to wait while the software calculated their “privacy propensity score.” All respondents were then told that based on their responses, our system had identified them as someone with a heightened concern about privacy. As such, we would automatically sign them up to receive a data and identity theft protection plan offered by our corporate partner, the largest and most experienced identity theft prevention and credit monitoring company in the USA. This was our bait and switch.
We told participants that by using the demographic information they had provided at the beginning of the survey, along with their IP address, we had pinpointed their mailing address. Our corporate partner would now provide them with six months of free data protection and credit history monitoring. After the six-month period, they would be billed monthly (though they could cancel at any time). The amount they would be billed varied by condition. Participants in the low stakes condition were told that the monthly fee would be $2.99, and participants in the high-stakes condition were told the fee would be $8.99 per month.
Participants were then allowed to either accept or decline the data protection program. But the steps that were required to do so varied by the level of the dark pattern manipulation. In the control group condition, we did not include any dark patterns. As such, this condition serves as a baseline to help us establish a ceiling for what percentage of the sample was inherently interested in receiving the identity theft protection. 16 Participants could thus either click “Accept” or “Decline” on the first screen. Regardless of which option they selected, they proceeded to the final stage of the experiment, which is described below.
In the mild dark patterns condition, subjects could either click “Accept and continue (recommended)” or “Other options,” and the button that accepted the program was selected by default. We made it easier for users to accept the program (because they did not have to select the button themselves) and harder to decline it (because there was not a straightforward and immediate way to decline, only to see other options). Adding a “recommended” parenthetical is a form of false hierarchy. The parenthetical plausibly triggers a heuristic where consumers encounter recommendations made by a neutral fiduciary elsewhere and may be uncertain as to who is making the recommendation and what the basis for that recommendation is ( Gray et al. 2018 , p. 7).
If subjects selected “Other options,” they were directed to the next screen, which asked them to choose between “I do not want to protect my data or credit history” and “After reviewing my options, I would like to protect my privacy and receive data protection and credit history monitoring.” This question uses confirmshaming as a dark pattern to nudge respondents to accept the program (i.e. their decision to decline the program is framed as not wanting to protect their data).
Next, if subjects did not accept the program, they were asked to tell us why they declined the valuable protection. Several non-compelling options were listed, including “My credit rating is already bad,” “Even though 16.7 million Americans were victimized by identity theft last year, I do not believe it could happen to me or my family,” “I’m already paying for identity theft and credit monitoring services,” and “I’ve got nothing to hide so if hackers gain access to my data I won’t be harmed.” They also could choose “Other” and type in their reason, or choose “On second thought, please sign me up for 6 months of free credit history monitoring and data protection services.” This is another confirmshaming strategy. Additionally, it makes it more onerous for many users to decline rather than accept (because if they did not select one of the sub-optimal options provided, they were asked to type out their reason for declining). Subjects who rejected the data protection plan on this screen were treated as having declined the service, and they advanced to the same final screens that those in the control group also saw.
In the aggressive dark pattern condition, the first and second screens were identical to those in the mild dark pattern condition. Participants attempting to decline the identity theft protection were then told that since they indicated they did not want to protect their data, we would like to give them more information so that they could make an informed choice. We asked them to read a paragraph of information about what identity theft is. Participants could either choose “Accept data protection plan and continue” or “I would like to read more information.” They were forced to remain on the page for at least ten seconds before being able to advance, and they were shown a countdown timer during this period. This screen created a significant roach motel. Namely, it obstructed respondents’ ability to decline the program by making it more onerous to decline than accept. It also toyed with respondents’ emotions by using vivid, frightening language in the text. For example, participants read that identity theft “can damage your credit status, and cost you time and money to restore your good name.”
If respondents chose to read more information (rather than accept the program), the next screen had information about why identity theft matters and what a thief could do with their personal information. The options and countdown timer were the same as the previous screen. A third information screen explained how common identity theft is, with the same options and countdown timer displayed before they could advance. The cumulative effect of these screens amounted to a nagging dark pattern.
If participants endured all three information screens and chose “I would like to read more information,” they were then directed to a question designed to confuse them. They were asked, “If you decline this free service, our corporate partner won’t be able to help you protect your data. You will not receive identity theft protection, and you could become one of the millions of Americans who were victimized by identity theft last year. Are you sure you want to decline this free identity theft protection?” The two options were “No, cancel” and “Yes.” This trick question intentionally tried to confuse participants about which option they should select to decline the program. 17 Checking the box that includes the word “cancel” counterintuitively accepts the identity theft program. Participants choosing “Yes” were directed to the same last screen as in the mild dark pattern condition, which asked them to indicate their reason for declining the program. After that, they were sent to the same final screens that all subjects saw.
At the conclusion of the study, all participants were asked to indicate their current mood on a scale from 1 (“Happy and relaxed”) to 7 (“Aggravated and annoyed”). They were then asked whether they would be interested in potentially participating in follow-up research studies by the same researchers, again on a 7-point scale ranging from “not at all” to “extremely interested.” Next, they were asked on another 7-point scale how free they felt they were to refuse the offered plan. These questions aimed to assess whether companies that employ dark patterns face any negative repercussions for their use. By comparing the responses of mild and aggressive dark pattern participants to those of the control group, we could estimate the size of the good-will loss that a company employing dark patterns would suffer. Lastly, participants were asked how seriously they took the survey, and then were given a text box to write any questions, comments, or concerns they had about the survey. They were then thoroughly debriefed.
3.1.1. Rates of Acceptance
The results of the study offer striking empirical support for the proposition that dark patterns are effective in bending consumers’ will. As expected, in the control group condition, respondents opted to accept the identity theft protection program at very low rates. Only 11.3 percent of respondents accepted the program when they were allowed to accept or decline the program on the first screen. 18 This acceptance rate likely overestimates the demand for a product of this kind. 19
When mild dark pattern tactics were deployed, the acceptance rate more than doubled. Now 25.8 percent of participants accepted the data protection program, which corresponds to a 228 percent increase compared to the control group condition. When participants were exposed to aggressive dark patterns aggressive, the acceptance rate shot up further, with 41.9 percent of the sample accepting the program. 20 So the aggressive dark pattern condition nearly quadrupled the rate of acceptance, with a 371 percent increase in rates of acceptance compared to the control group condition. These results are statistically significant. Indeed, the effect sizes are enormous by the standards of social science research. Manipulative tactics widely employed in the world of brick-and-mortar sales are evidently much less powerful in comparison ( Hanson & Kysar 1999 , pp. 1447–1448).
In both conditions, the initial screen (which offered a choice between “Accept and continue (recommended)” and “Other options,” with the former choice pre-selected) accounted for the majority of acceptances. In the mild condition, more than three-quarters of participants who accepted did so on this first screen (75.5 percent, 117 out of 155). In the aggressive condition, this screen accounted for 65 percent of acceptances (141 out of 217). 21 The second screen (which offered a choice between “I do not want to protect my data or credit history” and “After reviewing my options, I would like to protect my privacy and receive data protection and credit history monitoring”) accounted for thirty-five more acceptances in the mild condition (23 percent of overall acceptances) and twenty-two more in the aggressive condition (10 percent of acceptances). For those in the aggressive condition, when participants were forced to read three screens of information on identity theft for at least ten seconds per screen, this roach motel and nagging strategy accounted for 19 percent of acceptances overall. The confusing trick question (offering an “Are you sure you want to decline this free identity theft protection?” prompt with the options “No, cancel” and “Yes.”) was responsible for another 11 percent of acceptances. Finally, nearly no one who made it to the final, confirmshaming screen (the list of largely bad reasons for declining the service, with one final chance to accept) ended up accepting, either in the mild or aggressive conditions.
Of course, participants in Study 1 only advanced to new screens in the mild and aggressive conditions if they did not “fall” for the dark pattern on an earlier screen. As soon as they accepted the program, the dark patterns ceased (as is often the case in the real world). This means that it is not appropriate to infer in this experiment the relative strengths of the different dark patterns deployed from the number of acceptances each caused. Dark patterns that were used later in the manipulation are less likely to work by the very fact that people who were most susceptible to dark patterns were no longer in the sample. This limitation on the first study was one of the factors that motivated us to launch a second experiment, which is described below.
That said, the information from our first experiment about when people accepted is informative for two reasons. First, it demonstrates the substantial cumulative power that different kinds of dark patterns can have. Some people who were able to resist certain dark patterns (like roach motels) are still susceptible to falling for others (like confusingly worded questions). Second, this data demonstrates that seemingly minor dark patterns can have relatively large effects on consumer choices. In the control group condition, participants were able to choose “Accept” or “Decline.” Changing these options to “Accept and continue (recommended)” and “Other options,” with the former pre-selected, all by itself, nearly doubled the percentage of respondents accepting the program—the acceptance rate increased from 11.3 percent to 20.7 percent in the combined dark patterns conditions (counting only those users who accepted on the first dark pattern screen).
3.1.2. The Influence of Stakes
Across the dark pattern conditions, we varied the price point of the program ($2.99 vs. $8.99) to see whether higher monetary stakes influenced rates of acceptance. The neoclassical model of economics generally predicts that consumers will be willing to jump over more hurdles in order to save themselves more money. On this account, consumers face a tradeoff between out-of-pocket expenses to be incurred later and annoying wasted time costs to be incurred now. Impatient consumers should therefore be more likely to relent and accept the program when the costs of acceptance are lower. 22 Moreover, rational consumers should be more attentive on average when they are asked to pay a higher price for a good or service, and this might make them less prone to mistakes or impulsive decision-making.
Based on these neoclassical assumptions, one of the authors (who has produced a fair bit of law & economics scholarship) hypothesized that in the high-stakes condition, overall acceptance rates would be lower. Additionally, he predicted that when respondents had more money at stake, they would be less likely to “fall” for the dark patterns employed in the mild and aggressive conditions. The other author (a psychologist) expressed her consistent skepticism about this hypothesis. The predictions suggested by the neoclassical model were not borne out by the data, and the psychologist’s skepticism proved well-founded. Rates of acceptance were not related to stakes ( χ (1, N = 1,762) = 0.76, p = 0.38). There were no significant differences between the high- and low-stakes conditions across any of the dark pattern conditions (see Appendix B for acceptance rates broken down by stakes and level of dark pattern). Tripling the cost of a service had no effect on uptake in this domain. You read that right.
3.1.3. Potential Repercussions of Deploying Dark Patterns
The rates of acceptance in the mild and aggressive conditions show that dark patterns are effective at swaying consumer choices. Though only a small percentage of participants were truly interested in the data protection program for its own sake, a much larger percentage decided to accept the program after we exposed them to dark patterns. These results illustrate why dark patterns are becoming more common—because companies know that they are effective in nudging consumers to act against their own preferences. But it is possible that companies may experience a backlash by consumers when they use dark patterns. If so, then there would be less concern that dark patterns are the result of market failure, weakening the case for legal intervention. The questions asked immediately after the experiment were designed to get at this question.
First, participants were asked about their mood to assess whether exposure to dark patterns elicited negative emotions. There was an overall effect of the dark pattern manipulation ( F (2, 1740) = 323.89, p < 0.001). While participants in the control group ( M = 2.96, SD = 1.61) and mild ( M = 3.05, SD = 1.73) conditions reported similar levels of negative affect, participants in the aggressive condition were significantly more upset ( M = 3.94, SD = 2.06). Post-hoc Tukey HSD (honestly significant difference) tests confirmed that the control group and mild conditions were not significantly different ( p = 0.63), but both differed significantly from the aggressive condition ( p < 0.001). These results suggest that if companies go too far and present customers with a slew of blatant dark patterns designed to nudge them, they might experience backlash and the loss of goodwill. Yet it is notable that the mild dark pattern condition more than doubled the acceptance rate and did not prompt discernable emotional backlash.
At the end of the study, participants had another chance to express their emotions; they were given a box to type any questions, concerns, or comments they might have. We decided to code these responses to see whether, similar to the explicit mood question mentioned above, participants were more likely to spontaneously express anger after having been exposed to the mild or aggressive dark patterns. 23 The pattern of results mirrored those of the explicit mood measure. Participants in the control group and mild conditions did not express anger at different rates. However, participants in the aggressive dark pattern condition were significantly more likely to express anger. 24
Taken together, these two mood measures suggest that overexposure to dark patterns can irritate people. Respondents in the aggressive dark pattern condition reported being more aggravated and were more likely to express anger spontaneously. It is notable that those respondents exposed to the mild dark patterns did not show this same affective response. Though the mild condition very substantially increased the percentage of respondents accepting the data protection program, there were no corresponding negative mood repercussions.
Even though respondents in the aggressive dark pattern condition overall expressed more negative affect (thereby indicating a potential backlash), it is important to understand what is driving this aggravation. Are people who end up accepting or declining the program equally angered by the use of dark patterns? To answer this question, we compared the moods of people who accepted or declined across the dark pattern conditions. There was an overall main effect, such that people who declined the program reported more displeasure ( M = 3.50, SD = 1.99) than those who accepted the program ( M = 3.21, SD = 1.78; F (1, 1741) = 8.21, p = 0.004). This effect is driven by the aggressive dark pattern condition. Specifically, among people who accepted the program, there were no significant differences in mood across the control group, mild, and aggressive dark pattern conditions. 25 However, among those who declined, respondents in the aggressive dark pattern condition were more aggravated than those in the control group and mild conditions. The latter two conditions did not differ. This suggests that when dark patterns are effective at leading people to a certain answer, there is no affective backlash. Only when participants are forced to resist a slew of dark patterns in order to express their preference do we observe increased aggravation.
In addition to mood, another potential kind of backlash that dark patterns might elicit is disengagement. People might negatively react because they feel pressured, leading them to want to avoid the dark patterns either in the moment or be hesitant to interact with the entity that employed the dark patterns in the future. In the current study, we have two measures that capture this potential disengagement.
First, participants were able to exit the survey at any time, though if they failed to complete the survey they forfeited the compensation to which they would otherwise be entitled. We therefore can examine whether participants were more likely to drop out of the study in the aggressive versus mild conditions (because the control group condition only contained one question, there was no opportunity for participants to drop out in this condition). We found that respondents were much more likely to drop out and disengage with the study in the aggressive condition ( χ (1, N = 1,192) = 47.85, p < 0.001). Only nine participants dropped out in the mild condition, while sixty-five dropped out at some point during the aggressive condition. The latter is an unusual, strikingly high dropout rate in our experience, made all the more meaningful by the sunk costs fallacy. Respondents had typically devoted ten minutes or more to the survey before encountering the dark pattern, and by exiting the survey during the dark pattern portion of the experiment they were forfeiting money they may well have felt like they had already earned. 26
Second, participants were told that some of them might be contacted to do a follow up survey with the same researchers. They were asked if they were potentially interested in participating. We expected participants to be less interested in the follow-up study if they had been exposed to the mild or aggressive dark pattern conditions. The results supported this hypothesis. Dark pattern condition was significantly related to interest in participating in a follow-up survey ( F (2, 1740) = 6.99, p = 0.001). Post-hoc Tukey tests revealed that participants in the control group condition indicated significantly more interest ( M = 4.46, SD = 2.31) than participants in the mild ( M = 4.11, SD = 2.32, p = 0.02) and aggressive ( M = 3.97, SD = 2.39, p = 0.001) conditions. However, here the difference between those in the mild and aggressive conditions was not significant ( p = 0.57). This is the one measure of customer sentiment where significant differences were observed between the control group and subjects exposed to mild dark patterns.
One potential reason for the disengagement found above is that the more participants were exposed to dark patterns, the more likely they were to feel coerced into accepting the data protection program. To assess this, we asked participants how free they felt to refuse the data protection program. As expected, condition significantly influenced feelings of freedom ( F (2, 1739) = 96.63, p < 0.001). Post-hoc Tukey tests show that participants in the control group condition felt freer to refuse ( M = 6.21, SD = 1.44) compared to those in the mild ( M = 5.81, SD = 1.75) and aggressive ( M = 4.74, SD = 2.26) conditions ( p s < 0.001). Interestingly, as the median scores suggest, most respondents felt more free than unfree to refuse the program, even in the aggressive dark pattern condition.
3.1.4 Predicting Dark Pattern Susceptibility
Given the strong influence that dark patterns seem to exert on consumer choice, it is important to understand what individual differences might predict susceptibility. Put another way, what kinds of people are more vulnerable to being manipulated by dark patterns? 27 To answer this question, we analyzed whether demographic differences predicted acceptance rates across dark pattern conditions.
We first analyzed whether education predicts acceptance of the program and found that it does. A logistic regression was performed to ascertain the effects of education on the likelihood that participants accepted the data protection program. The less educated participants were, the more likely they were to accept the program ( b = −0.15, SE = 0.04, p < 0.001). The key question, though, is whether the relationship between level of education and likelihood of acceptance varies by dark pattern condition. In the control group condition, education is not significantly related to whether participants accepted or declined ( b = −.11, SE = 0.08, p = 0.17). This means that in the absence of dark patterns, participants with high and low levels of education do not differentially value the offered program. However, when they are exposed to mild dark patterns, participants with less education become significantly more likely to accept the program ( b = −.19, SE = 0.06, p = 0.002). A similar pattern of results emerged in the aggressive dark pattern condition ( b = −0.17, SE = 0.06, p = 0.003).
When controlling for income, the relationship between education and acceptance varies slightly. The results are similar, except that less education no longer predicts acceptance in the aggressive dark pattern condition ( b = −0.07, SE = 0.07, p = 0.27). The relationship persists in the mild dark pattern condition ( b = −0.17, SE = 0.07, p = 0.01). When additional demographic variables are controlled for—including age, gender, and race (white vs. non-white)—this pattern of results endures. Education predicts acceptance rates in the mild dark pattern condition ( b = −0.18, SE = 0.07, p = 0.01) but not control ( b = −0.05, SE = 0.10, p = 0.57) or aggressive conditions ( b = −0.08, SE = 0.07, p = 0.24).This result further illustrates the insidiousness of relatively mild dark patterns. They are effective, engender little or no backlash, and exert a stronger influence on more vulnerable populations.
3.2 Study Two: Isolating the Effects of Different Dark Patterns
While our first experiment provided tantalizing answers to a number of questions, it left many important questions open. First and foremost, it was uncertain which dark patterns were responsible for our results. Because dark patterns were presented in an intuitive but non-random order, experimental subjects who rejected the service represented increasingly hardened targets as the experiment proceeded. Moreover, we wondered whether the experimenters’ affiliation with a renowned research university might have caused consumers to be more susceptible to dark patterns than they otherwise would be, and whether our results about indifference to stakes would replicate if the offer presented were more obviously a bad deal for consumers. To address these and other issues, we administered a second online study to a new census-weighted sample of American participants recruited by Dynata in 2020. We removed respondents who took too long or too little time to complete the survey from the sample, as well as those who indicated they did not take the survey seriously. 28 Participants were compensated by Dynata for their time, and we compensated Dynata.
To begin, study participants viewed a consent form. In the description of the study, participants either read that the study was being completed by researchers at the University of Chicago or a fictitious company called Ydentity Incorporated. At the end of the survey, participants who viewed the consent form including the fictitious company were asked if they googled Ydentity Incorporated. After removing participants who indicated they had googled the company, 29 this left a final sample of 3,777. 30
After consenting to the survey, participants answered the same demographic questions as Study 1, including age, gender, race, education, income, employment, political orientation, and region of the country. As in the first study, included with the demographics questions were additional questions aimed to bolster the later cover story that we had pinpointed their mailing address. 31 Participants were asked their zip code, how long they had lived at their current residence, the area code of their primary telephone number, and where they were completing the survey (home, work, or other). To reinforce the cover story, participants were also asked whether they had a credit card. If they answered yes, they were asked what kind of credit card it was and who issued it. If they reported they did not have a credit card, they were asked to indicate their primary bank. 32
Participants then filled out several measures to assess whether certain worldviews or personality traits would predict susceptibility to dark pattern manipulation: risk propensity, right-wing authoritarianism, and political ideology. We then assessed participants’ attitudes about data privacy, as in the first study. The set up to the dark pattern manipulation was the same as in Study 1.
3.2.1. Dark Pattern Conditions
Using the demographic information you provided at the beginning of the survey and your IP address, we have pinpointed your mailing address. We have partnered with the nation’s largest and most experienced data security and identity theft protection company. They will be provided with your answers on this survey. After identifying you, you will receive one month of data protection and credit history monitoring free of charge . After the one month period, you will be billed $8.99 per month for continued data protection and credit history monitoring. You can cancel this service at any time (emphasis in original).
There were five different “content” conditions that varied the information presented to participants: control, hidden information, social proof, scarcity, and confirmshaming. Participants in the control condition read the text above as is.
Hidden Information: Those in this condition read largely the same text, but were told that they would “receive one month of data protection and credit history monitoring free of charge*”, and that “[t]erms and conditions apply.” At the bottom of the page, the price information was included in small, gray font.
Social Proof: Those in the social proof condition read the control text, and then read two additional sentences: “1,657 other participants have accepted this free month of data protection and credit history monitoring in the last three weeks. Would you like to accept the program and join them?”
Scarcity: Those in this condition read the control condition, but were first told “Congratulations! You have been selected to receive one month of free data protection and credit history monitoring. But you must ACT FAST ! We only have three trial memberships left and this offer will expire in the next 60 seconds.” There was a one-minute timer included on the screen that counted down.
Confirmshaming: Those in this condition read the control condition, but then the option to decline the program was phrased as “I don’t care about protecting my data or credit history.”
Additionally, on the same screen, there were four different conditions as to how participants accepted or declined the program: control, default, recommendation, and obstruction. Participants in the control condition could either choose “Accept” or “Decline.” Those in the default condition had the “accept” answer preselected. Those in the recommendation condition could choose either “Accept (recommended)” or “Decline.” Those in the obstruction condition saw the choices as “Accept and continue” or “Other options.”
Participants were randomly assigned to both content and form conditions, such that the survey had a 5 (content condition: control, hidden information, social proof, scarcity, confirmshaming) x 4 (form condition: control, default, recommendation, obstruction) design. The control conditions were oversampled in order to ensure an adequately powered control group.
For those participants who were assigned to the obstruction condition, they were assigned to one of two follow-up scenarios if they selected “other options.” In the short follow-up condition, they were simply asked whether they would like to decline the free month of data protection and credit history monitoring by answering “Yes” or “No.” In the long obstruction condition, they read that because they indicated they may not want to protect their data or credit history, we would give them a little more information so that they can make an informed decision. On the first screen, they were told information about what identity theft is. They were forced to stay on the screen for at least fifteen seconds. After that time elapsed, they could select “Accept data protection plan and continue” or “I would like to read more information.” For those that selected they would like to read more information, a second screen gave them information about how common identity theft is. After fifteen seconds, they were able to accept or decline the data protection plan. This condition was somewhat similar to parts of the aggressive dark pattern condition in Study 1.
Half of all participants were then assigned to a trick question that ostensibly asked them to confirm their decision. The question read, “Would you prefer not to decline this free data protection and credit history monitoring?” and participants could either select yes or no. The “correct” answer to this double-negative question if a participant wanted to decline the data protection plan was “no.”
At the conclusion of the study, all participants were asked to indicate their current mood and whether they would be interested in potentially participating in follow-up research by the same researchers, as in Study 1. They were also asked new questions about whether they accepted or declined the plan, and how much (on a seven-point scale) they regret their decision. If they indicated they accepted the plan, they were asked how likely they were to cancel it after the free month, again on a seven-point scale. Next, they were asked on another seven-point scale how free they felt they were to refuse the offered plan. Lastly, participants were asked how seriously they took the survey, and then were given a text box to write any questions, comments, or concerns they had about the survey. Subjects were then thoroughly debriefed.
3.2.2 Rates of Acceptance
The results of the study offer empirical support for the notion that not all dark patterns are equally effective—while some of the dark patterns tested had a striking effect on consumer choice, others had little to no effect.
Which content condition participants were exposed to had a significant effect on acceptance rates after the initial dark pattern screen ( χ (4, N = 3,777) = 76.04, p < 0.001). Collapsing across form condition, 14.8 percent of participants in the content control condition accepted. The hidden information condition doubled acceptance rates, with 30.1 percent of participants accepting the data protection plan ( χ (1, N = 1,896) = 61.25, p < 0.001). The confirmshaming and social proof conditions also significantly increased acceptance rates, but to a more modest degree ( χ (1, N = 1,901) = 6.96, p = 0.008 and χ (1, N = 1,923) = 15.74, p < 0.001, respectively). The scarcity condition, on the other hand, did not have any significant impact on acceptance rates ( χ (1, N = 1,924) = 0.08, p = 0.78).
How participants were able to accept or decline the plan also had a significant effect on acceptance rates ( χ (3, N = 3,777) = 15.98, p < 0.001). Making “accept” the default choice, and indicating participants would have to do more work to decline significantly increased acceptance rates ( χ (1, N = 2,145) = 4.02, p = 0.045 and χ (1, N = 2,065) = 14.84, p < 0.001, respectively). Marking the choice to “accept” as recommended, however, did not have a significant impact ( χ (1, N = 2,155) = 0.74, p = 0.39). In Study 1, there were three differences between the control condition and the mild dark pattern condition on the first screen: the “accept” option was recommended, selected by default, and the option of “decline” was changed to “other options.” The results of the second study suggest that both setting “accept” as the default answer and indicating to participants they would need to do more work to decline than accept the program both contributed to the higher rates of acceptance in the mild dark pattern condition in Study 1. For a full breakdown of acceptance rates by content and form condition, see Appendix C.
The trick question had a striking effect on whether participants accepted or declined the program. For half of the sample that saw the trick question, 19.2 percent had accepted the program. However, after being exposed to the trick question that asked them to “confirm” their answer, 33.4 percent accepted the plan by answering “yes.” This difference is highly significant ( χ (1, N = 1,857) = 275.02, p < 0.001).
As in Study 1, across dark pattern conditions, we varied the price point of the program. Unlike Study 1, however, the difference between the “low” and “high” price points in Study 2 differed more dramatically. In the “low”-stakes condition, participants were told that after the free month, the plan would cost $8.99 per month. In the “high”-stakes condition, it was $38.99 a month. This $38.99 figure is substantially higher than the monthly costs for established competitors’ data protection plans ( CNET.com 2020) . The one-month free trial period also was substantially less generous than Study 1’s six-month free trial.
Replicating Study 1’s surprising results, the price point again had little effect on whether people accepted or declined the program. Looking at participants’ decision after the initial screen, in the low-stakes condition, 19.8 percent of participants accepted. In the high-stakes condition, only slightly less—17.3 percent—accepted. This difference is not significant ( χ (1, N = 3,777) = 2.82, p = 0.09). This difference remains non-significant if participants’ ultimate choice (after the trick question) is analyzed ( χ (1, N = 3,777) = 0.18, p = 0.67). There, 26.7 percent of participants accept in the low-stakes condition, and 26 percent accept in the high-stakes condition.
At the end of the study, participants who reported that they accepted the program were asked how likely they were to cancel the plan after the free month. In total, 73.7 percent of people who accepted reported a 4 or higher on the scale, and 21.1 percent of participants reported a 7 on the scale—that they “definitely will cancel.” This suggests that participants may be discounting the price point of the plan because they anticipate they will cancel it before they have to pay.
3.2.3. Influence of Researcher
Participants saw one of two consent forms at the beginning of the study. Half of the participants were told the study was being conducted by “University of Chicago researchers” and the other half were told it was being conducted by “Ydentity Incorporated.” Participants were also asked at the end of the survey if they googled Ydentity Incorporated.
Before excluding participants who indicated they googled Ydentity Incorporated, those who saw the corporate versus university consent forms did not differ in their overall acceptance rates ( χ (1, N = 3,932) = 0.87, p = 0.77). In total, 21.8 percentage of those in the university condition and 21.4 percent of those in the corporate condition accepted the data protection plan. However, after removing participants in the corporate condition who indicated they googled Ydentity Incorporated—which presumably includes both participants who googled the company and those who answered the question inattentively, as discussed above—consent condition had a significant effect on acceptance rates ( χ (1, N = 3,777) = 17.17, p < 0.001). In total, 16.4 percent of participants in the corporate condition accepted the program. (Subjects in the University of Chicago condition were not asked whether they googled the school.) As illustrated below, though overall acceptance rates were higher in the university consent condition, in general, consent condition did not meaningfully change the relative strength of the different dark patterns.
In other words, once participants who reported googling Ydentity were removed, there was a main effect such that more participants accepted the program in the University of Chicago condition than the Ydentity condition. Table 5 shows that acceptance rates decreased by approximately 2 to 7 percentage points as a result of the removal. This is likely due, at least in part, to the fact that those in the Ydentity condition were asked an additional question that functioned as an extra attention check: whether they had googled the company. The fact that participants who reported googling the company spent less time on the consent screen suggests participants who were answering the survey quickly or inattentively were more likely to (inaccurately) answer “yes” to the question of whether they had googled. Thus, removing these participants from the Ydentity condition means that, on average, the remaining participants in that condition were more attentive. Given that overall acceptance of the program were low (and certainly below 50 percent), we would expect acceptance rates among inattentive participants to be higher because they are more likely to accept or decline the program at random.
Summary of existing dark pattern taxonomies
Category . | Variant . | Description . | Source . |
---|---|---|---|
Repeated requests to do something the firm prefers | |||
Activity messages | False/misleading Notice that others are purchasing, contributing | ||
Testimonials | False/misleading positive statements from customers | ||
Roach motel | Asymmetry between signing up and canceling | , | |
Price comparison prevention | Frustrates comparison shopping | , , | |
Intermediate currency | Purchases in virtual currency to obscure cost | ||
Immortal accounts | Account and consumer info cannot be deleted | ||
Sneak into basket | Item consumer did not add is in cart | , , | |
Hidden costs | Costs obscured/disclosed late in transaction | , , | |
Hidden subscription/forced continuity | Unanticipated/undesired automatic renewal | , , | |
Bait and switch | Customer sold something other than what’s originally advertised | ||
Hidden information/aesthetic manipulation | Important information visually obscured | ||
Preselection | Firm-friendly default is preselected | , | |
Toying with emotion | Emotionally manipulative framing | ||
False hierarchy/pressured selling | Manipulation to select more expensive version | , | |
Trick questions | Intentional or obvious ambiguity | , | |
Disguised ad | Consumer induced to click on something that isn’t apparent ad | , | |
Confirmshaming | Choice framed in a way that makes it seem dishonorable, stupid | , | |
Cuteness | Consumers likely to trust attractive robot | ) | |
Friend spam/social pyramid/address book leeching | Manipulative extraction of information about other users | , , | |
Privacy Zuckering | Consumers tricked into sharing personal info | , , | |
Gamification | Features earned through repeated use | ||
Forced Registration | Consumer tricked into thinking registration necessary | ||
Low stock message | Consumer informed of limited quantities | ||
High demand message | Consumer informed others are buying remaining stock | ||
Countdown timer | Opportunity ends soon with blatant visual cue | ||
Limited time message | Opportunity ends soon |
Category . | Variant . | Description . | Source . |
---|---|---|---|
Repeated requests to do something the firm prefers | |||
Activity messages | False/misleading Notice that others are purchasing, contributing | ||
Testimonials | False/misleading positive statements from customers | ||
Roach motel | Asymmetry between signing up and canceling | , | |
Price comparison prevention | Frustrates comparison shopping | , , | |
Intermediate currency | Purchases in virtual currency to obscure cost | ||
Immortal accounts | Account and consumer info cannot be deleted | ||
Sneak into basket | Item consumer did not add is in cart | , , | |
Hidden costs | Costs obscured/disclosed late in transaction | , , | |
Hidden subscription/forced continuity | Unanticipated/undesired automatic renewal | , , | |
Bait and switch | Customer sold something other than what’s originally advertised | ||
Hidden information/aesthetic manipulation | Important information visually obscured | ||
Preselection | Firm-friendly default is preselected | , | |
Toying with emotion | Emotionally manipulative framing | ||
False hierarchy/pressured selling | Manipulation to select more expensive version | , | |
Trick questions | Intentional or obvious ambiguity | , | |
Disguised ad | Consumer induced to click on something that isn’t apparent ad | , | |
Confirmshaming | Choice framed in a way that makes it seem dishonorable, stupid | , | |
Cuteness | Consumers likely to trust attractive robot | ) | |
Friend spam/social pyramid/address book leeching | Manipulative extraction of information about other users | , , | |
Privacy Zuckering | Consumers tricked into sharing personal info | , , | |
Gamification | Features earned through repeated use | ||
Forced Registration | Consumer tricked into thinking registration necessary | ||
Low stock message | Consumer informed of limited quantities | ||
High demand message | Consumer informed others are buying remaining stock | ||
Countdown timer | Opportunity ends soon with blatant visual cue | ||
Limited time message | Opportunity ends soon |
Acceptance rates by condition
Condition . | Acceptance rate (%) among subjects completing experiment . | Acceptance rate (%)—treating drop-outs as rejecting data protection plan . | Number of respondents accepting data protection plan . |
---|---|---|---|
Control Group | 11.3 | 11.3 | 73 |
Mild | 25.8 | 25.4 | 155 |
Aggressive | 41.9 | 37.2 | 217 |
Condition . | Acceptance rate (%) among subjects completing experiment . | Acceptance rate (%)—treating drop-outs as rejecting data protection plan . | Number of respondents accepting data protection plan . |
---|---|---|---|
Control Group | 11.3 | 11.3 | 73 |
Mild | 25.8 | 25.4 | 155 |
Aggressive | 41.9 | 37.2 | 217 |
Acceptance rates by content condition
Condition . | Acceptance rate (%) . | Number of respondents accepting . |
---|---|---|
Control group | 14.8 | 191 (out of 1,289) |
Scarcity | 14.3 | 91 (out of 635) |
Confirmshaming | 19.6 | 120 (out of 612) |
Social proof | 22.1 | 140 (out of 634) |
Hidden information | 30.1 | 183 (out of 607) |
Condition . | Acceptance rate (%) . | Number of respondents accepting . |
---|---|---|
Control group | 14.8 | 191 (out of 1,289) |
Scarcity | 14.3 | 91 (out of 635) |
Confirmshaming | 19.6 | 120 (out of 612) |
Social proof | 22.1 | 140 (out of 634) |
Hidden information | 30.1 | 183 (out of 607) |
Acceptance rates by form condition
Condition . | Acceptance rate (%) . | Number of respondents accepting . |
---|---|---|
Control group | 16.7 | 216 (out of 1,294) |
Recommended | 18.1 | 156 (out of 861) |
Default | 20.1 | 171 (out of 851) |
Obstruction | 23.6 | 182 (out of 771) |
Condition . | Acceptance rate (%) . | Number of respondents accepting . |
---|---|---|
Control group | 16.7 | 216 (out of 1,294) |
Recommended | 18.1 | 156 (out of 861) |
Default | 20.1 | 171 (out of 851) |
Obstruction | 23.6 | 182 (out of 771) |
Acceptance rates by university or corporate consent condition
Condition . | University condition acceptance rate (%) . | Corporate condition acceptance rate (googlers excluded) (%) . | Corporate condition acceptance rate (googlers included) (%) . |
---|---|---|---|
Content condition | |||
Control group | 17.7 | 11.7 | 17 |
Scarcity | 16.4 | 12 | 17.3 |
Confirmshaming | 20.8 | 18.3 | 23.2 |
Social proof | 25.5 | 18.8 | 22.3 |
Hidden information | 33.3 | 26.6 | 32 |
Form condition | |||
Control group | 19.9 | 13.3 | 18.9 |
Recommended | 21.2 | 14.5 | 18.2 |
Default | 20.3 | 19.9 | 23.1 |
Obstruction | 27.1 | 19.9 | 26.8 |
Condition . | University condition acceptance rate (%) . | Corporate condition acceptance rate (googlers excluded) (%) . | Corporate condition acceptance rate (googlers included) (%) . |
---|---|---|---|
Content condition | |||
Control group | 17.7 | 11.7 | 17 |
Scarcity | 16.4 | 12 | 17.3 |
Confirmshaming | 20.8 | 18.3 | 23.2 |
Social proof | 25.5 | 18.8 | 22.3 |
Hidden information | 33.3 | 26.6 | 32 |
Form condition | |||
Control group | 19.9 | 13.3 | 18.9 |
Recommended | 21.2 | 14.5 | 18.2 |
Default | 20.3 | 19.9 | 23.1 |
Obstruction | 27.1 | 19.9 | 26.8 |
Acceptance rates by educational attainment
Education level . | Acceptance rate in control condition (%) . | Acceptance rate in treatment conditions (%) . |
---|---|---|
High school diploma or less | 7.2 | 17.8 |
Some college or associate’s degree | 16.3 | 22.2 |
Bachelor degree or higher | 17.8 | 22 |
Education level . | Acceptance rate in control condition (%) . | Acceptance rate in treatment conditions (%) . |
---|---|---|
High school diploma or less | 7.2 | 17.8 |
Some college or associate’s degree | 16.3 | 22.2 |
Bachelor degree or higher | 17.8 | 22 |
Despite the fact that overall rates of acceptance differed between the two consent conditions, the table below illustrates that the relative effectiveness of the dark patterns tested did not differ by condition. Regardless of whether participants saw the University of Chicago or Ydentity consent form, the rank ordering of the dark patterns by effectiveness did not meaningfully vary.
3.2.4. Potential Repercussions of Deploying Dark Patterns
Studies 1 and 2 offer empirical support for the striking effectiveness of dark patterns in shaping consumer choice. One potential repercussion is that not only are consumers unaware of how these sludges may affect their behavior, but they also might not even be aware of the actual choice that they made. In Study 2, some participants were shown a trick question asking them to “confirm” their choice, but it was worded in a confusing manner that was cognitively taxing to unravel. At the end of the survey, participants were asked to indicate whether they accepted or declined the program. Thus, participants’ actual choice (whether they accepted or declined the program when asked the trick question) can be compared to the choice they think they made. For those that saw the trick question, 33.4 percent of participants accepted the program. However, when asked about their choice, only 16.7 percent of participants reported accepting the program. Put another way, 310 participants signed up for the data protection plan lacking awareness of that choice. That is a significant social cost.
As in Study 1, at the end of the survey, participants were asked to indicate their mood and how interested they were in doing follow up research with the researchers. Unlike in Study 1, there was no aggressive dark pattern condition in Study 2 in which participants were exposed forced to interact with numerous dark patterns in order to decline the data protection program. Therefore, in order to examine whether there might be negative repercussions to deploying certain dark patterns, we examined participants' interaction with the trick question and how it was related to their mood and interest in doing follow-up research.
First, we analyzed the amount of time participants spent on the trick question screen. Presumably, the more time they spent reading and answering the question, the more aware they are of how cognitively taxing the question was. On average, participants spent 12.18 seconds on the trick question, with a standard deviation of 11.78 seconds. We created two groups of participants: those who spent relatively less and more time on the screen. 33 We then tested whether these two groups differed in their mood and willingness to re-engage with the researchers. Participants who spent relatively less time on the trick question screen had similar mood levels ( M = 3.71, SD = 1.90) to those who spent more time on the screen ( M = 3.70, SD = 1.99; F (1, 716) = 0.006, p = 0.94). However, those who spent more time on the trick question were significantly less interested in being contacted about doing follow-up research ( M = 4.11, SD = 2.30) than those who spent less time on the question ( M = 4.50, SD = 2.18; F (1, 716) = 4.96, p = 0.023).
Second, we analyzed whether understanding the trick question (as evidenced by a participant’s choice to accept or decline the program remaining the same before and after the trick question) predicted participants’ mood and interest. As with time spent on the question, if participants were able to decode the trick question and confirm their prior answer, it is more likely they were aware of the cognitively taxing nature of the question. Participants who got the trick question correct were in a worse mood ( M = 3.79, SD = 1.91) than those who were tricked ( M = 3.44, SD = 1.76; F (1, 1855) = 12.11, p < 0.001). Additionally, those who answered the trick question correctly were significantly less interested in doing follow-up research ( M = 4.25, SD = 2.27) than those who answered it incorrectly ( M = 4.50, SD = 2.16; F (1, 1855) = 4.17, p = 0.04). This suggests that the use of intentionally ambiguous questions generates a backlash that is limited to users who recognize the manipulation.
Next, we examined whether the initial dark patterns participants were exposed to produced any backlash, either for their mood or likelihood of doing follow-up research with the same researchers. 34 Which content conditions participants were exposed to had a significant effect on mood ( F (4, 2112) = 6.57, p < 0.001). Post-hoc Tukey tests reveal that participants in the control condition ( M = 3.72, SD= 1.91) reported similar mood levels as those in the confirmshaming ( M = 3.82, SD = 2.05) and social proof ( M = 3.60, SD = 1.81) conditions. Notably, those in the hidden information condition ( M = 3.20, SD = 1.73) — the content condition that had the greatest effect on overall acceptance rates — were in a significantly better mood than those in the control condition ( p < 0.001). Further, those in the scarcity condition were also in a better mood ( p = 0.04). Which content condition participants were in had no effect on their interest in doing follow-up research ( F (4, 2112) = 0.96, p = 0.43). Similarly, form condition (control, recommended, default, or obstruction) did not significantly affect mood ( F (3, 2113) = 0.90, p = 0.44) or interest in doing follow up research ( F (3, 2113) = 2.08, p = 0.10).
3.2.5. Predicting Dark Pattern Susceptibility
As in Study 1, Study 2 analyzed whether education level predicted susceptibility to dark patterns. In order to do so, we divided the sample into those who were not shown a dark pattern—in other words, those who were in both the control condition for both content and form—and those who viewed at least one dark pattern for either content or form. We then analyzed whether education predicted acceptance levels among these two groups. For those in the control condition, education significantly predicts acceptance levels, such that those with less education were more likely to decline the data protection plan ( b = −0.25, SE = 0.07, p < 0.001). This remains the case when demographic controls (including age, gender, race, and income) are included in the model ( b = −0.27, SE = 0.08, p = 0.001). This indicates that, without any intervention, those with less education had less of a preference for accepting the data protection plan. 35 However, for those exposed to at least one dark pattern, education no longer predicts acceptance rates (b = −0.002, SE = 0.03, p = 0.94). As illustrated in the table below, there is a larger difference between the control and treatment groups amongst those with a high school diploma or less, compared to the other two groups. While, at baseline, those with a high school diploma or less are much less likely to accept the data protection plan, this difference narrows when participants are exposed to dark patterns. In short, although the results of Study 1 and 2 were not identical, both studies show that less education increases vulnerability to small- or moderate-dose dark patterns.
3.3. Summary of Results
To summarize the data we have collected and analyzed here, it appears that several frequently employed dark patterns can be very effective in prompting consumers to select terms that substantially benefit firms. These dark patterns might involve getting consumers to sign up for expensive goods or services they do not particularly want, as in our study and several real-world examples discussed in the previous part, or they might involve efforts to get consumers to surrender personal information—a phenomenon we did not test but that also is prevalent in e-commerce.
From our perspective, it is the mild dark patterns tested—like selecting an option that is good for a company’s bottom line but maybe not for consumers by default or by providing initial choices between “Yes” and “Not Now”—that are most insidious. In Study 1, this kind of decision architecture, combined with the burden of clicking through an additional screen, managed to more than double the percentage of respondents who agreed to accept a data protection plan of dubious value, and it did so without alienating customers in the process. As a result, consumers were manipulated into signing up for a service that they probably did not want and almost certainly did not need. In Study 2, slight alterations to the content of the message or form of acceptance had profound effects on consumer behavior—obscuring the price information or using confusing wording nearly doubled the rate of acceptance, leading consumers to pay for a product they did not desire, and (in the case of the trick question) obscuring that choice from the consumer. Notably, both studies show that deploying dark patterns to shape a consumer’s behavior does not necessarily lead to backlash—for example, when the dark patterns are subtle or trick the consumer. More broadly, we can say the same things about the kinds of dark patterns that are proliferating on digital platforms. These techniques are harming consumers by convincing them to surrender cash or personal data in deals that do not reflect consumers’ actual preferences and may not serve their interests. There appears to be a substantial market failure where dark patterns are concerned—what is good for e-commerce profits is bad for consumers, and plausibly the economy as a whole. Legal intervention is justified.
We now know that dark patterns are becoming prevalent and they can be powerful. Knowing these things raises the question of whether they are also unlawful (as unfair or deceptive practices in trade). It also implicates the related question of whether consumer assent secured via dark pattern manipulations ought to be regarded as consent by contract law. Finally, if readers conclude that dark patterns ought to be unlawful or ought not to count as valid consumer consent, that conclusion raises a host of implementation issues. We consider those issues in Section 4.
There are several plausible legal hooks that could be used to curtail the use of dark patterns by U.S. firms in e-commerce. 36 First, the Federal Trade Commission Act restricts the use of unfair or deceptive practices in interstate trade, providing the Commission with a mandate to regulate and restrict such conduct. Second, state unfair competition laws include similar frameworks. Finally, there is a broad question about whether consumer consent that is procured in a process that employs highly effective dark patterns should be voidable, which would entitle consumers to various remedies available under contract law and which could open up liability for firms that engage in various activities (for example, engaging in surveillance or processing biometric information) without having first obtained appropriate consumer consent.
4.1. Laws Governing Deceptive and Unfair Practices in Trade
The FTC, with its power to combat unfair and deceptive acts and practices under section 5 of the FTC Act, is the most obvious existing institution that can regulate dark patterns. The scope of the FTC’s investigation and enforcement authority covers “any person, partnership or corporation engaged in or whose business affects commerce,” (15 U.S.C. § 46(a)) with some minor exceptions. As such, the FTC has the necessary reach to restrict the use of dark patterns across a wide range of industries. Since 1938 the FTC Act has included language prohibiting “unfair or deceptive acts or practices in or affecting commerce” ( Sawchak & Nelson 2012 , p. 2057). The scope of the FTC’s reach and the language of the provision remain broad, reflecting Congress’s view that it would be challenging to specify ex ante all the different forms of behavior in trade that might be problematic. The Judiciary has consistently deferred to the FTC’s interpretation of its mandate, with the Supreme Court holding in FTC v. Sperry & Hutchinson Co. that the FTC Act allows, “the Commission to define and proscribe practices as unfair or deceptive in their effect upon consumers” (405 U.S. 233 (1972)).
In using its authority to restrict deceptive acts or practices affecting commerce, the FTC treats as deceptive any “representation, omission, or practice” that is (i) material, and (ii) likely to mislead consumers who are acting reasonably under the circumstances. (In the Matter of Cliffdale Assoc., Inc., 103 FTC 110, 1984 WL 565319 ( FTC, March 23, 1984) . Materiality involves whether the information presented “is important to consumers and, hence, likely to affect their choice of, or conduct regarding, a product” ( FTC v. Cyberspace.com LLC , 453 F.3d 1196, 1201 (9th Cir. 2006)). Any express product claims made by a company are presumptively material ( FTC v. Pantron 1 Corp. , 33 F.3d 1088 (9th Cir. 1994)). As for the second prong, “the Commission need not find that all, or even a majority, of consumers found a claim implied” a false or misleading statement. Rather, liability “may be imposed if at least a significant minority of reasonable consumers would be likely to take away the misleading claim” ( Fanning v. FTC , 821 F.3d 164, 170–171 (1st Cir. 2016)). When enforcing the law, the FTC need not show that the defendants intended to deceive consumers. Rather, it will be adequate for the agency to show that the “overall net impression” of the defendant’s communication is misleading ( FTC v. E.M.A. Nationwide, Inc., 767 F.3d 611, 631 (6th Cir. 2014)). Thus, a company cannot make an initial series of misstatements and then bury the corrections of those misstatements in a subsequent communication.
Because lawyers have written very little about dark patterns, and because computer scientists writing in the field are largely unaware of developments in the case law, the existing literature has missed the emergence in recent years of numerous FTC enforcement actions that target dark patterns, albeit without using that term. Indeed, many of the key published opinions postdate Ryan Calo’s survey of the law from 2014, in which he found hardly any relevant FTC enforcement actions ( Calo 2014 , p. 1002).
Federal Trade Commission v. AMG Capital Management is the most important of the dark patterns cases, but because it’s very recent and flew under the radar when it was decided it rarely has been discussed in the legal scholarship (910 F.3d 417 (9th Cir. 2018)). 37 The dispute involved the FTC’s enforcement action against a payday lender that was using various dodgy tactics to lure customers. The primary defendant, Scott Tucker, ran a series of companies that originated more than $5 million in payday loans, typically for amounts less than $1,000 ( id. , p. 420). Tucker’s websites included Truth in Lending Act (TILA) statements explaining that customers would be charged a finance rate of, say, 30 percent for these loans. But the fine print below the TILA disclosures mentioned an important caveat. Amidst “densely packed text” especially diligent readers were informed that customers could choose between two repayment options – a “decline to renew” option and a “renewal” option ( id. , pp. 422–423). Customers who wanted to decline to renew would pay off the payday loan at the first opportunity, provided they gave Tucker’s company notice of their intention to do so at least three business days before the loan was due. On the other hand, customers who opted for “renewal” would accrue additional finance charges, such as an additional 30 percent premium on the loan. After three such renewals, Tucker would impose an additional $50 per month penalty on top of the accumulated premiums. As the Ninth Circuit explained, a typical customer who opted for the renewal option could expect to pay more than twice as much for the loan as a typical “decline to renew” customer. So, of course, Tucker’s companies made “renewal” the default option and buried information about how to switch to the “decline to renew” option in a wall of text. That was the case even though the TILA disclosures provided the repayment terms under the assumption that a customer opted to decline to renew.
Judge O’Scannlain, writing for the court, was not impressed with Tucker’s protestations that his disclosures were “technically correct.” In the Court’s view, “the FTC Act’s consumer-friendly standard does not require only technical accuracy…. Consumers acting reasonably under the circumstances – here, by looking to the terms of the Loan Note to understand their obligations – likely could be deceived by the representations made here. Therefore, we agree with the Commission that the Loan Note was deceptive” ( id. , p. 424). Tucker’s websites employed numerous dark patterns. Renewal option customers were subjected to forced continuity (a costly subscription by default) and a roach motel (avoiding the onerous default is more taxing than submitting to it). And all customers had to overcome hidden costs (the burial of the renewal option’s onerous terms in a long wall of text), preselection (making renewal the default), and trick question text (hard-to-understand descriptions of their options) in order to avoid paying substantially higher fees. Each of these problematic aspects of the website design was emphasized by the circuit court ( id. , pp. 422–424). The court did not need a dark patterns label or experimental data to see how deceptive the individual strategies and their cumulative effect could be. The circuit court affirmed a $1.27 billion award against Tucker after he lost on summary judgment.
AMG Capital Management is not the only recent appellate court opinion in which the courts have regarded dark pattern techniques as deceptive trade practices. In Federal Trade Commission v. LeadClick Media , the Second Circuit confronted “disguised ad” behavior and false testimonials ( FTC v. LeadClick Media , LLC, 838 F.3d 158 (2d. Cir. 2016)). LeadClick was an internet advertising company, and its key customer was LeanSpa, an internet retailer that sold weight-loss and colon-cleanse products ( id. , p. 163). LeadClick’s strategy was to place much of its advertising on websites that hosted fake news. Many of the advertisements it placed purported to be online news articles but they were in fact ads for LeanSpa’s products. The supposed articles included photos and bylines of the phony journalists who had produced the stories extolling the virtues of LeanSpa’s products. As the court explained, these “articles generally represented that a reporter had performed independent tests that demonstrated the efficacy of the weight loss products. The websites also frequently included a ‘consumer comment’ section where purported ‘consumers’ praised the products. But there were no consumers commenting—this content was invented” ( id. , pp. 163–164). The Second Circuit thought it was self-evident that these techniques were unlawfully deceptive, reaching that conclusion after articulating the applicable legal standard ( id. , p. 168). Again, the court lacked the vocabulary of dark patterns, and also lacked data about their efficacy, but it still regarded the issue as straightforward. The Second Circuit’s decision echoed a First Circuit decision from the same year, Fanning v. Federal Trade Commission , in which that court treated a defendant’s incorrect implication that content was user-generated as a deceptive practice in trade (Fanning , 821 F.3d, 171–173).
A recent deceptive conduct in FTC action against Office Depot is instructive as to the agency’s current thinking. In that complaint, the FTC alleged that Office Depot and its corporate partner, Support.com, were falsely informing consumers that their computers were infected with malware and then selling them various fixes for non-existent problems ( Federal Trade Commission v. Office Depot , Complaint for Permanent Injunction and Other Equitable Relief, Case No. 9-19-cv-80431 (S.D. Fla. March 27, 2019 )). Office Depot and Support.com were apparently employing misleading software that convinced consumers to pay money for virus and malware removal services they did not need.
Advertisements and in-store sales associates encouraged customers to bring their computers to Office Depot for free “PC Health Checks.” When a customer did so, Office Depot employees would ask consumers whether they had any of the following four problems with their computer: (i) frequent pop-up ads, (ii) a computer that was running slowly, (iii) warnings about virus infections, or (iv) a computer that crashed frequently. If the answer to any of those questions was yes, the employees were to check a corresponding box on the first screen of the Health Check software. The computers then had their systems scanned by Office Depot employees using the Support.com software. Customers were led to believe that the process of scanning the computers was what generated subsequent recommendations from Office Depot employees about necessary fixes, such as virus and malware removal services. In fact, the scanning process was irrelevant for the purposes of generating such recommendations. The only relevant factors for generating recommendations were the responses to the first four questions that the employee asked the customer. 38
Office Depot strongly encouraged its affiliated stores to push customers toward the PC Health Checks and allegedly expected a high percentage (upwards of 50 percent) of these Health Checks to result in subsequent computer repairs. Various store employees raised internal alarms about the software, noting that it was flagging as compromised computers that were working properly. These internal complaints evidently were ignored at the C-suite level. Eventually, a whistle-blower called reporters at a local Seattle television station. The station had its investigative reporters purchase brand new computers straight from the manufacturers and then bring those computers into Office Depot for PC Health Checks. In several cases, the Support.com software indicated that virus and malware removal was needed. Oops! The journalists’ revelation resulted in an FTC investigation and Office Depot quickly pulled the plug on its PC Health Check software. The companies settled with the FTC, agreeing to pay $25 million (in the case of Office Depot) and $10 million (in the case of Support.com) to make the case go away, albeit with no admission of wrongdoing on the part of either company ( Federal Trade Commission v. Office Depot , Stipulated Order for Permanent Injunction and Monetary Judgment, Case No. 9-19-cv-80431-RLR (S.D. Fla. March 28, 2019 ); Singletary 2019 ).
Several aspects of the deception in Office Depot resemble dark patterns. The entire computer scanning process was an example of aesthetic manipulation/hidden information designed to make the customer think that something other than their answers to the first four questions (yes, I see annoying pop-up ads) were driving the company’s recommendations about necessary repairs. There is also a clear bait-and-switch component to the allegations against Office Depot—customers thought they were getting a helpful and free diagnostic from a respected retailer. Instead, they were opening themselves up to a deceitful way for Office Depot to upsell services that many customers did not need. This was done via a mediated online interface employed in brick-and-mortar retail outlets.
Critically, in deciding what constitutes a deceptive practice in trade, the fact that many consumers wind up with terms, goods, or services they do not want strongly suggests that the seller has engaged in deception. That is a key take-away from another Ninth Circuit case, Cyberspace.com (453 F.3d at 1196). In that case, a company mailed personal checks to potential customers, and the fine print on the back of those checks indicated that by cashing the check the consumers were signing up for a monthly subscription that would entitle them to internet access. Hundreds of thousands of consumers and small businesses cashed the checks, but less than one percent of them ever utilized the defendant’s internet access service ( id. , p. 1199). That so many consumers had been stuck with something they did not desire and were not using was “highly probative,” indicating that most consumers “did not realize they had contracted for internet service when the cashed or deposited the solicitation check” ( id. , p. 1201). Courts considering FTC section 5 unfairness suits, discussed below, embrace the same kind of evidence and reasoning ( FTC v. Direct Benefits Group , LLC, 2013 WL 3771322, Case No. 6:11–cv–1186–Orl–28TBS, at *14 (M.D. Fla. July 18, 2013)). By the same logic, if it appears that a large number of consumers are being dark patterned into a service they do not want (as occurred in our experiment), then this evidence strongly supports a conclusion that the tactics used to produce this assent are deceptive practices in trade.
There is less clear case law surrounding the FTC’s use of section 5 from which to construct a profile of what conduct is “unfair.” In the overwhelming majority of enforcement actions, companies choose to settle with the Commission, entering into binding settlement agreements, rather than challenge the commission in court or administrative proceedings ( Solove & Hartzog 2014 , 583). In the absence of judicial decisions, however, consent decrees and other FTC publications have guided companies in interpreting the expected standards of behavior and ensuring their continued compliance with the law.
In 1980, the FTC laid out the test that is still currently utilized to find an act or practice “unfair.” Under this test, an unfair trade practice is one that (i) causes or is likely to cause substantial injury to consumers, (ii) is not reasonably avoidable by consumers themselves and (iii) is not outweighed by countervailing benefits to consumers or competition (FTC Policy Statement on Unfairness, 104 FTC 949 (1984)). This three-part test is now codified in section 5(n) of the FTC Act.
Generally, the “substantial injury” prong focuses on whether consumers have suffered a pecuniary loss. Monetary harm can come from the coercion of consumers into purchasing unwanted goods, or other incidental injuries that come as a result of the unfair action such as financial harm from identity theft. Notably, a harm’s substantiality can derive from its collective effect on consumers, as the FTC notes “an injury may be sufficiently substantial, however, if it does a small harm to a large number of people” ( id. ).
The next prong of the three-part unfairness test is that the injury must not be one that the consumer could have reasonably avoided. This prong is grounded in the belief that the market will be self-correcting and that consumers will learn to avoid companies that utilize unfair practices. Those practices that “prevent consumers from effectively making their own decisions,” run afoul of this prong, even if they merely hinder free market decisions, and fall short of depriving a consumer of free choice. For reasonable consumers to avoid harm, particularly in the case of a nonobvious danger, they must also be aware of the possible risk.
The cost–benefit analysis prong of the unfairness test ensures that companies are only punished for behaviors that produce “injurious net effects.” There are, as the Commission notes, inevitable trade-offs in business practices between costs and benefits for consumers, and as such certain costs may be imposed on consumers, provided they are balanced by legitimate benefits. Broader societal burdens are also accounted for in this equation, as are the potential costs that a remedy would entail. Additionally, the Commission looks to public policy considerations as part of this analysis to help establish the existence and weight of injuries and benefits that are not easily quantified. The Eleventh Circuit held in LabMD that the FTC needs to demonstrate a violation of constitutional, statutory, or common law constraints in order for an unfairness claim under section 5 to prevail ( LabMD, Inc. v. Federal Trade Comm’n , 894 F.3d 1221, 1231 (11th Cir. 2018)).
A few cases that resemble dark pattern conduct were brought on unfairness grounds as well as deception. A number of these FTC cases involve unsavory billing practices. One example is FTC v. Bunzai Media Group, Inc (2016 WL 3922625, at *5, Case No. CV 15-4527-GW(PLAx) (C.D. Cal. July 19, 2016)), a case in which the FTC secured a settlement of upwards of $73 million after alleging both deceptive and unfair practices. In that case the FTC asserted that the defendants’ skin-care companies were using a host of dark patterns, including deceptive pop-up ads that stopped consumers from navigating away from a website without accepting an offer, small print at the very end of a transaction that were in tension with marketing claims used in larger, bold print, and pricing plans that quickly converted “risk-free trials” into renewing monthly subscriptions and were onerous to cancel ( FTC v. Bunzai Media Group , Case No. CV 15-4527-GW(PLAx), First Amended Complaint for Permanent Injunction and Other Equitable Relief, (C.D. Cal. October 9, 2015) ). The FTC’s more recent suit against Triangle Media involved some similar sales tactics, plus a nasty surprise—at the end of the transaction to set up the “free trial,” the defendants used misleading website text to create the false impression that the transaction was not complete until customers signed up for a second free trial for an entirely different product, and they would be signed up for costly monthly subscriptions to both by clicking on the “complete checkout” button ( FTC v. Triangle Media Corp ., 2018 WL 4051701, Case No. 18cv1388-MMA (NLS) (S.D. Cal. August 24, 2018)). This case too was brought under both prongs of section 5—deception and unfairness.
FTC v. FrostWire, LLC , is another case involving alleged unfairness as well as deception, this time with respect to the default settings of a peer-to-peer file sharing service that caused users to share more media than they were led to believe ( FTC v. Frostwire, LLC , Complaint for Permanent Injunction and Other Equitable Relief, Oct. 7, 2011 , available at 2011 WL 9282853). The FTC pointed to the obstructionist defaults of the program, which made it exceptionally burdensome for a consumer to prevent all of her files from being shared. As described in the complaint “a consumer with 200 photos on her mobile device who installed the application with the intent of sharing only ten of those photos first had to designate all 200 … as shared, and then affirmatively unshare each of the 190 photos that she wished to keep private.” This user interface presents a classic roach motel employing preselection.
These cases notwithstanding, there is little case law discussing unfairness and dark patterns in depth, especially in comparison to the development of the deceptive acts and practices precedents. Worse still, the leading appellate unfairness case is a Ninth Circuit unpublished disposition that lacks precedential value. The court concluded in that case, for example, that it was unfair conduct for material language to appear in blue font against a blue background on an “otherwise busy” web page ( FTC v. Commerce Planet, Inc. , 642 Fed.Appx. 680, 682 (9th Cir. March 3, 2016)). 39
Many of the dark patterns discussed earlier could be characterized in a manner to frame the injury as a consumer entering into a transaction they otherwise would have avoided, therefore falling squarely into the current conception of substantial injury. That said, there may be hurdles in conceptualizing dark patterns in a way that fulfills the “unavoidability” prong. When the use of dark patterns is extreme, capitalizing on consumer cognitive bias to the extent that it can be shown to overwhelm their ability to make a free decision, there should be no problem satisfying this prong. At first blush, the milder the use of dark patterns, the more difficult it will be to characterize the harm as unavoidable, particularly when not applied to any exceptionally vulnerable subsets of consumers. On the other hand, our data suggest that milder dark patterns are—if anything—harder to avoid, because of their potent combination of subtlety and persuasive ability.
To summarize, there is an emerging body of precedent in which the federal courts have viewed the FTC as well within its rights to pursue companies that deploy dark patterns online. Among the techniques identified in the taxonomy, false testimonials, roach motels, hidden costs, forced continuity, aesthetic manipulation, preselection, trick questions, and disguised ads have already formed the basis for violations of the FTC’s prohibition on deceptive acts in trade. Techniques that also employ deception, such as false activity messages, sneaking into the basket, bait and switch, forced registration, and scarcity techniques would seem to fall straightforwardly within the parameters of the existing law. Other techniques, like nagging, price comparison prevention, intermediate currency, toying with emotion, or confirmshaming would probably need to be challenged under unfairness prong in section 5. We were not able to find cases that shed light on whether nagging, toying with emotion, and confirmshaming are lawful. In any event, this survey of the existing precedents suggests that the law restricting dark patterns does not need to be invented; to a substantial degree it is already present.
State unfair competition laws largely track their federal counterpart. There has been far less enforcement activity under these laws targeting dark patterns than there has been under the applicable federal regime. As a result, the law is underdeveloped, and few state cases have broken new ground. These kinds of cases are especially important to the extent that courts are reluctant to find unfairness in section 5 in the absence of a violation of some independent law. One relevant precedent is Kulsea v. PC Cleaner, Inc. , a case brought under California’s unfair competition law that predated, and in many ways anticipated, the FTC’s suit against Office Depot (2014 WL 12581769, NO. CV 12-0725 FMO (ANx), (C.D. Cal. February 10, 2014)). The allegations against PC Cleaner were that the firm’s software indicated that there were harmful bugs on the machine that could be addressed via the purchase of the full version of the software.
Another instructive state law case is In re Lenovo Adware Litigation (2016 WL 6277245 (N.D. Cal. October 27, 2016)). That class action case is a sort of split-decision where dark patterns are concerned. Lenovo pre-installed adware on computers that it sold to customers, hiding the software deep within the computers’ operating system so it would be difficult to detect and remove. Consumers were given just one chance to remove the software the first time they opened their internet browser, and retaining the software was the default option. Lenovo thus employed preselection, alongside arguable bait-and-switch and hidden costs. A claim brought under New York’s consumer protection law, which prohibits deceptive trade practices, was dismissed because the plaintiffs failed to show that they suffered an actual injury, such as a pecuniary harm ( id. , *10). In the court’s view, this lack of pecuniary harm did not justify dismissing the plaintiffs’ claims under California state unfair competition law, given that the adware negatively affected the performance of the laptops, and that the installation of the adware was peculiarly within Lenovo’s knowledge, material, and a fact that went undisclosed to consumers ( id. , *11–*14). The case ultimately settled for more than $8 million ( In re Lenovo Adware Litigation , 2019 WL 1791420, at *6 Case No. 15-md-02624-HSG (N.D. Cal. April 24, 2019)).
4.2 Other Relevant Federal Frameworks
Some enforcement efforts that target dark patterns could be done through the Consumer Financial Protection Bureau (CFPB), which has the authority to regulate “abusive conduct,” at least within the banking and financial services sector. The abusive conduct definition by the CFPB is arguably more expansive than the unfair conduct that can be regulated by the FTC. An abusive practice, per 12 U.S.C. § 5531 is one that:
materially interferes with the ability of a consumer to understand a term or condition of a consumer financial product or service; or
takes unreasonable advantage of -
a lack of understanding on the part of the consumer of the material risks, costs, or conditions of the product or service;
the inability of the consumer to protect the interests of the consumer in selecting or using a consumer financial product or service; or
the reasonable reliance by the consumer on a covered person to act in the interests of the consumer.
This provision would seemingly cover the exploitation of the cognitive biases of consumers in order to manipulate them into making a decision that may not be in their best interests.
Another relevant federal law is the Restore Online Shoppers’ Confidence Act (ROSCA) (15 U.S.C. §§8401–05). ROSCA makes it unlawful for a third-party seller to charge customers absent a clear and conspicuous disclosure of the transaction’s material terms, informed consent, and an affirmative step by the consumer indicating willingness to enter into the transaction with the third party (15 U.S.C. § 8402). This law was aimed at the problem of consumers unwittingly being signed up for a subscription to a third party’s good or service immediately after entering into a desired transaction with a vendor, where the third party would use the payment information that the consumer had already inputted. The FTC enforces ROSCA in a manner similar to its section 5 enforcement, and ROSCA squarely addresses certain types of bait-and-switch dark patterns, which often employed hidden costs and forced continuity schemes.
4.3 Contracts and Consent
In his 2018 book, Woodrow Hartzog advanced the argument that contractual consent secured via pernicious forms of dark patterns or other deceptive designs should be deemed invalid as a matter of law ( Hartzog, 2018 , pp. 212–213). Hartzog’s argument is built on a series of powerful anecdotes, and the eye-opening data we present here buttresses his bottom line. In our view, Hartzog has it mostly right. The hard part, however, is determining how to tell whether a dark pattern is egregious enough to disregard a consumer’s clicking of an “I agree” button. Hartzog’s book spends just a few pages developing that particular argument, so there is more theoretical and doctrinal work to be done.
The law’s deference to contractual arrangements is premised on a belief that private ordering that commands the mutual assent of the parties makes them better off than the alternative of mandatory rules whose terms are set by the government. The more confidence we have that a contractual arrangement is misunderstood by one of the parties and does not serve the expressed interests of that party, the less reason there is to let the terms of a relationship be set by contract law. Assent procured mostly via the use of dark patterns doesn’t form contracts; it forms pseudo-contracts ( Kar & Radin 2019 , pp. 1192–1201). Those should not bind the signatories. Some courts, such as the Seventh Circuit in Sgouros v. TransUnion Corp ., have embraced the idea that material information displayed on a screen that a reasonable consumer almost certainly did not see is inadequate to create contractual assent (817 F.3d 1029, 1035–1036 (7th Cir. 2016)).
To snare members, Trilegiant allegedly designs its enrollment screens to appear as confirmation pages for the legitimate, just-completed transaction, so that the customer is unaware of having registered to buy and new and completely different product. Trilegiant’s cancellation and billing process allegedly prolongs the fraud. To cancel a subscription, the customer must first discover the monthly billing on a credit card statement and call Trilegiant’s customer service; Trilegiant’s representatives then attempt to keep members enrolled as long as possible, either through promotion of the program’s benefits or delay in the cancellation process. ( id. , p. 120)
To be clear, not everything described above is a dark pattern, but some of those steps—the disguised ad, the roach motel, the forced continuity, and the nagging—would qualify. The district court’s opinion helpfully reproduced the text of Trilegiant’s user interface, albeit with much of the text too small to read (In re Trilegiant Corp., 2016 WL 8114194, at *2 (D. Conn. August 23, 2016)). From that text and the lower court opinion, it appears that the plaintiffs were arguing that the deceptive conduct was evident from a glance at the screenshots.
To the Williams court, there was insufficient evidence that this conduct vitiated consent. The plaintiffs produced an expert witness, a marketing scholar, who testified that the user interface “was designed to result in purchases of Trilegiant’s services without awareness of those purchases” ( Williams , 889 F.3d, 123), and that the disclosures were designed “so that they would not be seen or understood” ( id. , p. 122) The plaintiff’s also argued that the relevant terms of the program were buried in “miniscule fine print” ( id. ).
The plaintiff made two key mistakes that, from the Second Circuit’s perspective, warranted the district court’s decision to grant the defendant’s summary judgment motion. First, the expert witness does not appear to have presented any data about consumer confusion—his statements about the interface design and Trilegiant’s likely intentions were conclusory and not supported by evidence in the record ( In re Trilegiant Corp ., 2016 WL 8114194, *11 n.3). Second, the plaintiffs did not argue that the plaintiffs were confused as a result of ambiguous language or design ( Williams , 889 F.3d, 123). In short, the Williams opinion leaves the door ajar for class action suits against e-commerce firms that employ dark patterns, provided the proof of consumers being confused or tricked into paying for goods and services they do not want employs the kind of rigorous randomization-based testing that we present here. Indeed, the data that we gathered in Study 2 shows that some dark patterns, like trick questions, can cause large numbers of consumers to not grasp that they have just signed up for a service. Relatedly, the results of our hidden information experiment, especially the consumer mood data, suggest that dark patterns might cause consumers to misunderstand material terms of a contractual offer they have just accepted.
The contract doctrine of undue influence provides the most promising existing framework for efforts to curtail dark patterns. Under the Restatement (Second) of Contracts, “undue influence is unfair persuasion of a party who is under the domination of the person exercising the persuasion or who by virtue of the relation between them is justified in assuming that that person will not act in a manner inconsistent with his welfare” ( Restatement (Second) of Contracts (1981) , § 177). Comment b of section 177 of the Restatement emphasizes further that the “law of undue influence … affords protection in situations where the rules on duress and misrepresentation give no relief. The degree of persuasion that is unfair depends on a variety of circumstances. The ultimate question is whether the result was produced by means that seriously impaired the free and competent exercise of judgment. Such factors as the unfairness of the resulting bargain, the unavailability of independent advice, and the susceptibility of the person persuaded are circumstances to be taken into account in determining whether there was unfair persuasion, but they are not in themselves controlling.” Undue influence renders a contract voidable by the influenced party ( Rich v. Fuller , 666 A.2d 71, 76 (Maine 1995)).
Applying this rubric, it should not be controversial to assert that some packages of dark patterns like the ones employed in our experiments seriously impaired the free and competent exercise of judgment. That seems to be their purpose and effect, as our data show. The more effective a dark pattern is at overcoming user preferences, and the greater the social harm that results, the more troubling the dark pattern should be as a legal matter. The harder doctrinal question is whether a consumer and the typical firm that employs dark patterns satisfies either the domination or relationship part of the Restatement test.
The case law suggests that some courts construe the relationship language broadly. In one prominent case, a chiropractor convinced his patient to sign a form indicating that the patient would pay for the services in full even if her insurance company elected not to cover them ( Gerimonte v. Case , 712 P.2d 876 (Wash. App. 1986)). When the patient objected, saying that she could not afford to pay out of pocket, the chiropractor told her “that if her insurance company said they would take care of her, they would. He told her not to worry” ( id. , p. 877). These statements induced the patient to sign. The court granted summary judgment to the chiropractor against the patient’s undue influence claim, and the appellate court reversed. From the appellate court’s perspective, these statements uttered in the context of this medical treatment relationship were enough for a reasonable jury to conclude that undue influence had occurred ( id. , p. 879). The majority brushed aside the concerns of a dissenting judge, who accused the majority of invalidating a contract over “nothing more than the urging, encouragement, or persuasion that will occur routinely in everyday business transactions” ( id. , p. 880 (Scholfield, C.J., dissenting)). Another leading case where the court similarly reversed a summary judgment motion involved a relationship between a widow and her long-time friend who was also an attorney ( Goldman v. Bequai , 19 F.3d 666, 669 (D.C. Cir. 1994)).
In influential publications, Jack Balkin and Jonathan Zittrain have proposed that digital platforms like Facebook, Google, Microsoft, and Amazon should owe fiduciary duties to their customers ( Balkin 2016 , p. 1183; Balkin & Zittrain 2016 ). If such a proposal were implemented, then the use of effective dark patterns by these platforms would render any consent procured thereby voidable by the customer. This result follows because the law generally presumes undue influence in those instances where a fiduciary owes a duty to a client and the fiduciary benefits from a transaction with its client ( Matlock v. Simpson , 902 S.W.2d 385 (Tenn. 1995)). Fiduciaries must demonstrate the substantive fairness of the underlying transaction to defeat a claim of undue influence ( id. , p. 386).
Even to skeptics of Balkin and Zittrain’s information fiduciary theory ( Khan & Pozen 2019 , p. 497), dark patterns could be voidable under the domination theory referenced in the Restatement. There is some fuzziness around the precise meaning of domination in the case law. Circumstantial evidence is plainly adequate to prove undue influence ( Nichols v. Estate of Tyler , 910 N.E.2d 221 (Ind. App. 2009); In re Cheryl E., 207 Cal. Rptr. 728, 737 (Cal. App. 1984)). A classic undue influence case describes domination as a kind of “overpersuasion” that applies pressure that “works on mental, moral, or emotional weakness to such an extent that it approaches the boundaries of coercion” ( Odorizzi v. Bloomfield Sch. Dist. , 54 Cal. Rptr. 533, 539 (Cal. App. 1966)). As the court emphasized, “a confidential or authoritative relationship between the parties need not be present when the undue influence involves unfair advantage taken of another’s weakness or distress” ( id. ). In the court’s judgment, undue influence could arise when “a person of subnormal capacities has been subjected to ordinary force or a person of normal capacities subjected to extraordinary force” ( id. , p. 540). None of the cases suggest that domination requires a certainty that the dominated party will do the dominant party’s bidding.
Doubling or tripling the percentage of consumers who surrender and agree to waive their rights through non-persuasive tactics like nagging, confusion, hidden costs, or roach motels could satisfy the domination test, particularly when those tactics are unleashed against relatively unsophisticated users. Indeed, in trying to determine whether a tactic amounts to undue influence, courts have emphasized factors such as “limited education and business experience” ( Delaney v. Delaney , 402 N.W.2d 701. 705 (S.D. 1987)) as well as the uneven nature of the exchange in terms of what the party exercising influence gave and received ( Goldman , 19 F.3d 675). Similarly, the Restatement identifies “the unfairness of the resulting bargain, the unavailability of independent advice, and the susceptibility of the person persuaded” as the relevant considerations ( Restatement (Second) of Contracts 1981 , § 177 comment b). Treating highly effective dark patterns as instances of domination-induced undue influence would amount to an extension of the doctrine, but it is an extension consistent with the purpose of the doctrine. Furthermore, the availability of quantifiable evidence about the effects of particular dark patterns addresses lingering problems of proof that might otherwise make judges skeptical of the doctrine’s application. In short, there are sensible reasons to think that the use of dark patterns to secure a consumer’s consent can render that consent voidable by virtue of undue influence.
To push the argument further, there are a number of instances in which the existence of consent is necessary in order for the sophisticated party to a transaction to engage in conduct that would otherwise be unlawful. We identify three such statutory frameworks here. The first is electronic communications law. It is unlawful to intercept an electronic communication (such as a phone call or an email) without the consent of the parties to a communication (18 U.S.C. § 2511(2)(d)). Failure to secure consent has given rise to civil suits under this provision of the Electronic Communications Privacy Act and its state law equivalents ( Deal v. Spears ; In re Yahoo Mail Litigation, 7 F. Supp.3d 1016 (N.D. Cal. 2014); In re Google Inc. Gmail Litigation, 2013 WL 5423918 (N.D. Cal. September 26, 2013) (No. 13-MD-02430-LHK)). There is a strong argument to be made that consent secured via dark patterns is not adequate consent under these statutes, thereby opening up parties that intercept such communications to substantial liability, especially in cases where large numbers of communications have been intercepted, such as controversies involving automated content analysis of emails.
Illinois’ unique Biometric Identification Privacy Act (BIPA) places similar emphasis on the consent requirement. It requires firms that process the biometric information of consumers to obtain their explicit consent before doing so. The Illinois law sets a high threshold for what counts as adequate consent—firms must inform customers of the fact that biometric information is being collected and stored, the reason for collection, use, and storage, and the duration of storage (740 Ill. Comp. Stat. 14/20(2)). The law has produced an avalanche of class action litigation, directed at firms that analyze fingerprints, facial geometry in photos, voiceprints, or other biometric information. In the first half of 2019, new class action suits under BIPA were being filed at a rate of approximately one per day ( Seyferth Shaw LLP 2019 ). This rate of new class actions is driven in part by the availability of minimum statutory damages under the statute and the determination by the Illinois Supreme Court that it is not necessary to demonstrate an actual injury in order to have standing to sue under the statute in state court ( Rosenbach v. Six Flags Entertainment Corp ., 129 N.E.3d 1197 (Ill. 2019)). As e-commerce firms increasingly recognize the scope of their potential exposure to BIPA damages, many have done more to provide the disclosure boxes required by the statute. To the extent that they do so via a disclosure or consent-extracting mechanism that employs dark patterns, the courts could well deem those interfaces (and the “consent” produced thereby) inadequate as a matter of law, opening up the firms that employ those mechanisms subject to very significant liability ( Strahilevitz & Kugler 2016 ).
A relevant, but not heavily utilized, law exists in California as well. That state enacted a law in 2009 that can be used to aim squarely at forced continuity dark patterns. The law would “end the practice of ongoing charging of consumer credit or debit cards or third party payment accounts without the consumers’ explicit consent for ongoing shipments of a product or ongoing deliveries of service” (Cal. Bus. & Prof. Code § 17600). Recall Sony’s use of a roach motel to substantially thwart the wishes of PlayStation Plus users who wish to avoid a renewing subscription. There is a very plausible argument that Sony’s obstruction scheme, and ones like it, fall short of the explicit consumer consent standard required by California law. Without stretching the meaning of the statute’s words it is easy to imagine significant class action exposure for Sony.
4.4 Line Drawing
We expect that most readers will have some sympathy for the idea that dark patterns could be so pervasive in a particular context as to obviate consent. But the hard question, and the one readers have probably had on their minds as they read through the preceding pages, is “where does one draw the line?” We would readily concede that some dark patterns are too minor to warrant dramatic remedies like contractual rescission, and some do not warrant a regulatory response of any sort. Study 2 suggests that scarcity messages can be counterproductive for sellers, and small dosages of nagging or toying with emotion may be relatively benign. 40 Policing these dark patterns aggressively is unlikely to be cost-justified.
Our combination of experiments, however, suggests that certain kinds of dark patterns, such as trick questions, hidden information, and obstruction can be so powerful in and of themselves, when compared with more neutral choice architectures, to deserve special scrutiny. Indeed, the data presented here could well suffice to cause the FTC to conclude that the most potent dark patterns (in terms of affecting purchase or disclosure decisions and their propensity to spark ex post consumer regret or confusion) are presumptively deceptive or unfair.
Admittedly, one challenge here is to develop a neutral baseline against which the A/B testing can occur. With respect to straightforward linguistic choices, that sometimes will be easy. It should not be hard to generate consensus around the idea that a simple Yes/No or Accept/Decline prompt is neutral, provided the choices are presented with identical fonts, colors, font sizes, and placement. Things get more challenging when legal decision-makers must determine whether two, three, four, or five options are neutral, and that is an inquiry that is easier to answer with the benefit of data than it is in the abstract. Experiments like ours can be highly instructive, but there will always be legitimate questions about external validity. A manipulative survey like ours is not precisely identical to the online interface of an e-commerce site with which a consumer regularly interacts.
Here the FTC has an important tool at its disposal. The Commission regularly enters into twenty-year consent decrees after an initial violation of section 5. A standard feature of these consent decrees is the company’s agreement to permit independent audits of the firms’ compliance with its legal obligations arising under the decree and the law ( McGeveran 2016 , p. 999–1003). A muscular FTC could start insisting that these audits also empower regulators or independent auditors to conduct limited A/B testing on the sites operated by consent decree signatories to ensure that user interface design choices are not unduly manipulative. In other words, auditors should be able to randomly vary aspects of, say, the Facebook or Ticketmaster user interface for trial runs and then track user responses to ensure that consumers' choices are broadly consistent with their preferences. Invigorating FTC consent decrees to permit this kind of auditing could become a powerful institutional check on dark patterns. Companies are already doing the kind of beta-testing that reveals how effective their interfaces are at changing consumer behavior. To the extent that there is any doubt about a new technique, they can always examine their own design choices ex ante and see whether any cross the line ( Ben-Shahar & Strahilevitz 2017 , pp. 1822–1824).
A multi-factor test for dark patterns that looks to considerations such as (i) evidence of a defendant’s malicious intent or knowledge of detrimental aspects of the user interface’s design, (ii) whether vulnerable populations—like less educated consumers, the elderly, or people suffering from chronic medical conditions—are particularly susceptible to the dark pattern, and (iii) the magnitude of the costs and benefits produced by the dark pattern would be a good starting point for a doctrinal framework. Evidence about the ex post regret experienced by consumers who found themselves influenced by a dark pattern, like the data we generated in Study 2, might be a particularly revealing indicium of the costs. The greater the number of consumers who complained and sought cancellation of a term they did not realize they agreed to, or who did not utilize a service they found themselves paying for (as the Cyberspace.com court indicated), the greater the presumptive magnitude of the associated harm would be. By the same token, if it turned out that consumers were happy ex post with a good or service that a dark pattern manipulated them into obtaining, this would be revealing evidence cutting against liability for the seller. The ends could justify the means for a firm that genuinely was trying to trick consumers for their own good.
4.5 Persuasion
A final tricky challenge for a systematic effort to regulate dark patterns is to confront the issue of how to deal with variants of dark patterns that may be constitutionally protected. For most types of dark patterns, this is relatively easy—false and misleading commercial speech is not protected by the First Amendment ( Central Hudson Gas & Electric Corp. v. Public Serv. Comm’n of N.Y ., 447 U.S. 557, 563 (1980)). Returning to our taxonomy of dark patterns, then, this means that regulating several categories of dark patterns (social proof, sneaking, forced action, and urgency) is constitutionally unproblematic. In our revised taxonomy we have been more careful than the existing literature to indicate that social proof (activity messages and testimonials) and urgency (low stock/high demand/limited time messages) are only dark patterns insofar as the information conveyed is false or misleading. If a consumer is happy with a product and provides a favorable quote about it, it is not a dark pattern to use that quote in online marketing, absent a showing that it is misleadingly atypical. Similarly, Amazon can indicate that quantities of an item are limited if there really are unusually low quantities available and if the restocking process could take long enough to delay the customer’s order. But the First Amendment’s tolerance for the imposition of sanctions on commercial speech is premised on the false character of the defendant’s representations, such as by making false representations about the source of content on the defendant’s website ( Fanning v. FTC , 821 F.3d 164, 174–175 (1st Cir. 2016)). This is an important point, one that the existing writing on dark patterns sometimes misses.
Obstruction and interface interference present marginally harder issues. That said, in a leading case relatively blatant examples of these tactics have been deemed deceptive practices in trade. As such, the conduct would not receive First Amendment protection ( FTC v. AMG Capital Mgmt ., LLC, 910 F.3d 417 (9th Cir. 2018)). But strategies like toying with emotion, as well as confirmshaming, may be hard to restrict under current doctrine given firms’ speech interests. 41 There is virtually no legal authority addressing the question of whether commercial speech that satisfies the FTC’s test for unfairness, but is neither misleading nor deceptive, is protected by the First Amendment ( Pomeranz 2011 , pp. 550–552). The appellate cases that have been litigated recently tend to involve truthful but incomplete disclosures that create a misimpression among consumers, and FTC action in those cases has generally been deemed constitutionally permissible ( POM Wonderful LLC v. FTC , 777 F.3d 478 (D.C. Cir. 2015); Fanning , 821 F.3d at 164; ECM BioFilms, Inc. v. FTC , 831 F.3d 599 (6th Cir. 2017)).
Nagging presents perhaps the thorniest type of dark pattern from a First Amendment perspective. CNN’s website employs a nagging dark pattern, one that regularly asks users whether they wish to turn on notifications. There is no question that CNN’s core business is protected by the First Amendment. Would a regulation that prevented them from asking consumers to turn on notifications more than once a month, or once a year, infringe on the company’s rights as an organization? It would seem not, so long as the rule was implemented as a broadly applicable, content-neutral rule. Here a helpful analogy is to the Federal Do Not Call registry, which applies to newspapers and other speech-oriented entities, but which has withstood First Amendment challenges ( Mainstream Marketing Servs. Inc. v. FTC , 358 F.3d 1228, 1236–1246 (10th Cir. 2004); National Coalition of Prayer, Inc. v. Carter , 455 F.3d 783, 787-92 (7th Cir. 2006)). Limits on requests to reconsider previous choices seem likely to survive similar challenges, provided they establish default rules rather than mandatory ones. 42 On the other hand, the do-not-call cases involve communications by firms to individuals with whom they do not have existing relationships. In the case of nagging restrictions, the government would be limiting what firms can say to their customers in an effort to persuade them to waive existing rights, and it could be that this different dynamic alters the legal bottom line.
Given the potential uncertainty over whether nagging and other forms of annoying-but-nondeceptive forms of dark patterns can be punished, the most sensible strategy for people interested in curtailing these dark patterns is to push on the contractual lever. That is, the First Amendment may be implicated by the imposition of sanctions on firms that nag consumers into agreeing to terms and conditions that do not serve their interests. But there is no First Amendment problem whatsoever with a court or legislature deciding that consent secured via those tactics is voidable. At least in the American legal regime, then, while there is a lot to be gained from considering dark patterns as a key conceptual category, there are some benefits to disaggregation and context-sensitivity, at least in terms of thinking about ideal legal responses.
More broadly, the contractual lever may be the most attractive one for reasons that go far beyond First Amendment doctrine. The FTC has brought some important cases, but neither the federal agency nor enforcers of similar state laws can be everywhere. Public enforcement resources are necessarily finite. But consumers, and attorneys willing to represent them in contract disputes, are numerous. The widespread use of dark patterns could open up firms to substantial class action exposure. As a result, for even a few courts to hold that the use of unfair or deceptive dark patterns obviates consumer consent would significantly deter that kind of conduct.
Finally, and on a more encouraging note, we are starting to see the emergence of light patterns in user interfaces. For example, Apple’s most recent iOS interface alerts users to location tracking periodically and requires them to opt into it ( Albergotti 2019 ). An even more clever feature of iOS asks users whether they wish to authorize a particular smartphone app to access their phone’s camera roll. If the user says no, and then subsequently decides to change their mind, iOS will enable them to do so but now requires the user to go through several screens in order to undo their earlier, privacy-protective choice. The intuition appears to be that when a possibly impulsive decision is in tension with an earlier (possibly considered) judgment, the current preference should prevail only if the user is certain about her desire to undo a privacy-protective choice. The distinction between dark patterns and light patterns necessarily entails a value judgment, and we can imagine some readers who value efficiency over privacy would view this kind of obstruction as undesirable. But we endorse the intuition behind Apple’s UX design choices, given the plausible manner in which their operating system architecture is likely to nudge users to reflect on choices they have made and privilege deliberative System 2 decision-making.
Computer scientists and user experience designers discovered dark patterns about a decade ago, and there is a sense in which what they have found is the latest manifestation of something very old—sales practices that test the limits of law and ethics. There is a lot to be learned from looking backwards, but the scale of dark patterns, their rapid proliferation, the possibilities of using algorithms to detect them, and the breadth of the different approaches that have already emerged means this is a realm where significant legal creativity is required.
That is not to say that legal scholars concerned about dark patterns and the harms they can impose on consumers are writing on a blank slate. In a series of unheralded FTC deception cases, and in a few unfairness enforcement actions to boot, the regulator best positioned to address dark patterns has successfully shut down some of the most egregious ones. Courts have generally been sympathetic to these efforts, intuiting the dangers posed by these techniques for consumers’ autonomy and their pocketbooks. But an observer of the court cases comes away with an impression that the judges in these cases are like the blind men in the parable of the elephant. They do not understand the interconnectedness of the emerging strategies, nor does the nature of judging allow them to make comparisons about the most pressing problems and needs. As a result, they have not given serious thought to the hardest problem facing the legal system—how to differentiate tolerable from intolerable dark patterns.
We think of this article as making two important contributions to a literature that is growing beyond the human–computer interactions field. First and foremost, there is now an academic article that demonstrates the efffectiveness of various dark patterns. That was not true yesterday, even if part of our bottom line is an empirical assessment that has been presupposed by some courts and regarded skeptically by others. The apparent proliferation of dark patterns in e-commerce suggests that they were effective in getting consumers to do things they might not otherwise do, and we now have produced rather solid evidence that this is the case. Paradoxically, it appears that relatively subtle dark patterns are most dangerous, because they sway large numbers of consumers without provoking the level of annoyance that will translate into lost goodwill. We have begun to establish which dark pattern techniques are most effective and most likely to undermine consumer autonomy. This data can inform regulatory priorities and the development of doctrine and agency guidance. Obviously, there is a lot more experimental work to do, but this is a critical first step. We hope other social scientists follow us into this body of experimental research.
Second, though legal commentators have largely failed to notice, the FTC is beginning to combat dark patterns with some success, at least in court. The courts are not using the terminology of dark patterns, and they have been hamstrung by the absence of data similar to what we report here. But they have established some key and promising benchmarks already, with the prospect of more good work to come. Developing a systemic understanding of the scope of the problem, the magnitude of the manipulation that is occurring, and the legal landmarks that constrain what the government can do will only aid that new and encouraging effort.
The problem we identify here, then, is both an old problem and a new one. Companies have long manipulated consumers through vivid images, clever turns of phrase, attractive spokesmodels, or pleasant odors and color schemes in stores. This behavior should worry us a little, but not enough to justify aggressive legal responses. Regulating this conduct is expensive, and the techniques are limited in their effectiveness, especially when consumers have the opportunity to learn from previous mistakes.
The online environment is different. It is perhaps only a difference of degree, but the degrees are very large. Through A-B testing, firms now have opportunities to refine and perfect dark patterns that their Mad Men -era counterparts could have never imagined. By running tens of thousands of consumers through interfaces that were identical in every respect but one, firms can determine exactly which interface, which text, which juxtapositions, and which graphics maximize revenues. What was once an art is now a science. As a result, consumers’ ability to defend themselves has degraded. The trend toward personalization could make it even easier to weaponize dark patterns against consumers ( Strahilevitz et al. 2019 , pp. 34–36).
Today the law faces a new technology that presents challenges and opportunities. An analogous dynamic has developed recently with partisan gerrymandering and cell tower geolocation. Partisan gerrymandering has been around for a long time, but computing advances in the last several years have made the state-of-the-art techniques precise at a level entirely without precedent, permitting parties to create much greater partisan advantages than they used to be able to. Once the computers became powerful enough, scholars argued that new legal regimes were warranted ( Stephanopoulos & McGhee 2015 , pp. 899–900). But a bitterly divided Supreme Court ultimately disagreed, at least where the federal Constitution is concerned ( Rucho v. Common Cause , 139 S. Ct. 2484 (2019)). A similar challenge arose with geolocation, albeit with different results. It had long been settled that police officers could physically tail suspects without a warrant, but when doing just that became trivially expensive, because cell tower records revealed nearly every person’s historic whereabouts, scholars said that legal innovation was necessary ( Tokson 2016 , p. 139). And this time the Supreme Court majority agreed with the scholars ( Carpenter v. United States , 138 S Ct 2206 (2018)).
The technology of dark patterns has taken a quantum leap forward, rendering cheap and effective corporate tactics that used to be costly and clunky. So we are making a similar kind of argument to those who suggested that gerrymandering and geolocation technologies had upset status quo assumptions in fundamental ways. Manipulation in the marketplace is a longstanding problem, but recent events have made the problem much worse, and the data presented here give the strongest hint yet of how large the mismatch is between what consumers want and what they are supposedly consenting to. Dark patterns will proliferate in the absence of a muscular government response. Judges, legislators, and regulators now have the data they need to decide whether and how to help.
For helpful comments on earlier drafts and conversations the authors thank Ronen Avraham, Omri Ben-Shahar, Sebastian Benthall, Ryan Calo, Marshini Chetty, Neil Chilson, Adam Chilton, Danielle Citron, Lorrie Faith Cranor, Sheldon Evans, Brett Frischmann, Michael Froomkin, Meirav Furth-Matkin, Assaf Hamdani, Woody Hartzog, Todd Henderson, William Hubbard, Margot Kaminski, Anita Krishnakumar, Matthew Kugler, Filippo Lancieri, Anup Malani, Florencia Marotta-Wurgler, Jonathan Masur, Arunesh Mathur, Jonathan Mayer, Richard McAdams, Terrell McSweeney, Paul Ohm, Jeremy Sheff, Dan Simon, Roseanna Sommers, Geof Stone, Kathy Strandburg, Cass Sunstein, Blase Ur, Lukas Vermeer, Mark Verstraete, Lauren Willis, Felix Wu, and Luigi Zingales, along with workshop participants at the University of Chicago’s PALS Lab, the University of Chicago Works in Progress Workshop, St. John’s University, Tel Aviv University’s Law and Psychology Conference and Law and Economics Workshop, the University of Colorado Law School’s Faculty Colloquium, the Stigler Center’s 2019 Antitrust and Competition Conference, and the 2020 Privacy Law Scholars Conference. The authors thank the Carl S. Lloyd Faculty Fund for research support and Jess Clay, Tyler Downing, Daniel Jellins, and Quinn Underriner for excellent research assistance. The views expressed herein are solely those of the authors.
User interface designer Harry Brignull coined the phrase in 2009 and maintains a website that documents them in an effort to shame the programmers behind them. See Brignull 2020 .
After our paper was posted on SSRN, some computer scientists began to use similar methodologies to test dark patterns. Nouwens et al. (2020) employed small ( n = 40) convenience samples drawn from the authors’ personal contacts rather than representative samples. But other work builds on our own work and examines consumer awareness of dark patterns through survey methods with larger, albeit unrepresentative, samples ( Di Geronimo et al. 2020 , pp. 474, 480).
This is required by the CAN-SPAM Act of 2003, 15 U.S.C. § 103.
As of July 2019, Best Buy’s unsubscribe link in commercial emails followed this pattern. If a user clicked on the unsubscribe hyperlink at the bottom of a marketing email, she would be taken to a screen that provided three options: “Receive all General Marketing emails from Best Buy.” [This box is checked by default, so a user who clicks “unsubscribe” and then “submit” will not stop receiving any emails from Best Buy.] The second option says, “Receive no more than one General Marketing email per week.” And the third option is “Receive no General Marketing emails (unsubscribe).”
Google Maps does essentially the same thing. When a user repeatedly travels to a particular location and uses the app's directions, the app will display a “Go here often?” pop-up window that asks whether the location is her “Home,” “Work,” or “Other” (school, gym, etc.) approximately once a week. A user can close the window each time but there is evidently no way to prevent the queries from reappearing short of deleting all location history. The pop-up window notes that users’ labels for locations “will be used across Google products, for personalized recommendations, and for more useful ads.”
Most of the dark patterns literature is co-authored. Due to space constraints, we include in the table only the surname of the first listed author of such work.
We apologize for the small font size necessary to squeeze the table onto a page. We promise the table is not intended to be a dark pattern—we actually want you to read the categories and examples closely.
Most of the brick-and-mortar equivalent of dark pattern techniques on our list are either very uncommon or are widely believed to be dubious or unlawful when practiced in brick and mortar establishments. For example, telemarketers are prohibited from engaging in various forms of nagging, such as continuing to call someone who has said she does not wish to receive such calls (10 C.F.R. 310.4(b)(1)(iii)(A)). To take another example, the FTC has issued a policy statement making it clear that the use of disguised ads is actionable under section 5 (FTC, Enforcement Policy Statement on Deceptively Formatted Advertisements (December 22, 2015)). And some brick-and-mortar equivalents of dark patterns are so obviously unlawful that reputable firms do not even try them. For example, suppose Trader Joe’s instructed its cashiers to start charging their customers for granola bars they did not purchase and slipping those bars into their shopping carts surreptitiously. Surely some customers who did not notice what happened in the check-out aisle will not wind up returning the granola bars because of the inconvenience involved, but that hardly means they do not suffer from the conduct, nor would we be comfortable describing the transaction as one in which the customers consented to purchase the granola bars. The online equivalent of that conduct is “sneak into basket.” It is hard to imagine what a coherent defense of the tactic at a grocery store would look like.
It is actually even more cumbersome. When one of the authors opted to turn off automatic renewal, the author was required to re-log into the system with a username and password, even though the author was already logged in.
Even though some legal schemes, such as section 5 of the FTC Act, render intent irrelevant as a formal doctrinal matter, it remains a highly relevant consideration when the FTC has to decide how to spend its scarce enforcement resources.
The connection between the majority sentiment among consumers and the identification of dark patterns is explored more explicitly in Strahilevitz et al. (2019 , p. 44). In this article’s companion piece, we devote more time and experimental energy toward identifying the expectations and preferences that most consumers share ( Strahilevitz & Luguri 2019 , p. 139). Snyder & Mirabito (2016) somewhat similarly report the results of survey research into consumer preferences in other sales contexts.
Numerous studies examine the stickiness of default rules in various settings involving consumers and employees ( Pichert & Katsikopoulos 2008 , p. 63; Cronqvist & Thaler 2004 , p. 424).
After removing two participants who started and ended the survey on different days, the average completion time was computed. Participants took 11.5 minutes on average to complete the survey. We removed participants who took less than 4 minutes and more than 47.5 minutes (two standard deviations above the survey completion time). Additionally, participants were asked an attention check question that asked them to “Please select “Strongly agree” for this question below to show that you are paying attention.” Those that failed to answer accordingly were removed from the sample. At the end of the survey participants were asked to indicate how seriously they took the survey on a scale from 1 (“not at all seriously”) to 5 (“extremely seriously”). Participants who answered 1 were also removed from the sample.
Males comprised 47.1 percent of the sample. 76.2 percent of the sample self-identified as White, 13.2 percent as Black, 1.2 percent as American Indian, 4.4 percent as Asian, and 4.9 percent as “other.” On a separate question, 14 percent indicated they are Hispanic or Latino. 6 percent of the sample had not completed high school, 29.8 percent had high school diplomas, 29.8 percent had some college or an associate’s degree, 20.9 percent had bachelor’s degrees, and 13.6 percent had advanced degrees. 10.8 percent of the sample was between eighteen and twenty-four years, 18 percent was between twenty-five and thirty-four years, 17.6 percent was between thirty-five and forty-four years, 17.2 percent was between forty-five and fifty-four years, 19.3 percent was between fifty-five and sixty-four years, and 17 percent was sixty-five years or older. In total, 1773 participants (90.3 percent) fully completed the survey from start to finish.
See https://aspredicted.org/see_one.php (Experiment # 19680) (submitted February 17, 2019). On the value of pre-registration in social science research, see Nosek et al. (2018) .
We refer to this figure as a ceiling in the sense that it likely overestimates demand for the service subjects told our corporate partners were selling. This overestimation arises for at least two reasons. First, respondents were told that they already had been signed up for the service (potentially triggering loss aversion at the prospect of its removal) and second, subjects were told that they would pay nothing for the service for the first six months (potentially triggering hyperbolic discounting and optimism bias about whether their future selves would remember to cancel the service once the free trial period ended). We had also primed them to think a lot about privacy, though it is not clear which way that cuts, given our setup. Because we are more interested in comparing the control group to the dark pattern conditions than we are in estimating the actual unmet demand for an identity theft protection service, this potential overestimation presents no problems for our study.
For a discussion of similar dark pattern strategies in Apple’s iOS 6, see Hartzog (2018 , p. 208).
Participants were counted as accepting the program if, in any question, they selected the option to accept. They were counted as declining if they indicated they declined in the control group condition, or if they reached the last screen in the mild and aggressive conditions and selected an option other than accepting the program.
See note 16.
A chi-square test of independence was performed to examine the relationship between dark pattern condition and acceptance rates. The relation between these variables was significant ( χ (2, N = 1,762) = 142.16, p < 0.001). Table 2 also presents the data if subjects who closed their browsers to exit the survey during the dark pattern manipulation are treated as declining the plan. Counting drop-outs as no’s has a negligible effect on the mild dark pattern acceptance rate and a small effect on the aggressive dark pattern acceptance rate.
Because the aggressive dark pattern subjects had more opportunities to relent and accept the data protection plan later in the survey it makes sense that this percentage is lower. The higher dropout rate of those in the aggressive dark patterns condition, discussed below, is another contributing factor. Counting dropouts as people who declined the data protection plan, 19.2 percent of subjects in the mild dark pattern condition and 24.2 percent of subjects in the aggressive dark pattern condition accepted the offer on the first screen. Another 5.7 percent of subjects accepted the offer on the second screen in the mild condition and 3.7 percent accepted on the second screen in the aggressive condition. Thus, 24.9 percent of respondents in the mild dark pattern condition and 27.9 percent of respondents in the aggressive dark pattern condition accepted on one of the first two screens, which were identical across the mild and aggressive dark pattern conditions. For a full breakdown of acceptance rate by question, see Appendix A.
One countervailing force consistent with the neoclassical model is that high price can function as a signal of quality ( Gneezy, Gneezy & Lauga 2014 , p. 154). There is obviously a limit to this signaling dynamic, however, which constrains price increases.
Participants who did not write anything, wrote something neutral, or wrote something positive were coded as 0. Participants who either expressed general anger or anger specifically at the offer of the data protection program were coded as 1.
In the control group condition, 36 out of 632 (5.70 percent) were coded as expressing anger. In the mild condition, the rate was 36 out of 591 (6.09 percent). In the aggressive condition, it was 66 out of 515 (12.82 percent). A chi-square test of independence was performed to examine the relationship between dark pattern condition and whether anger was expressed (Yes/No). The relation between these variables was significant ( χ (1, N = 1,738) = 23.86, p < 0.001). The control group and mild conditions did not differ significantly from each other ( χ (1, N = 1151) = 0.09, p < 0.77) but both differed significantly from the aggressive condition (control group vs. aggressive: χ (1, N = 1,045) = 17.75, p < 0.001; mild vs. aggressive: χ (1, N = 1,004) = 14.86, p < 0.001) .
There was a significant interaction between dark pattern manipulation and outcome, F (5, 1737) = 15.12, p < 0.001. Among people who accepted, there was no main effect of dark pattern condition, F (2, 434) = 0.62, p = 0.54. However, among those who declined, there was a main effect, F (2, 1303) = 67.02, p < 0.001. Post-hoc Tukey tests revealed that respondents who declined after being exposed to the aggressive dark pattern condition were significantly more aggravated than those in the control group and mild conditions ( p s < 0.001). Respondents who declined in the control group and mild conditions did not differ significantly ( p = 0.81).
The dropout rates observed provide highly relevant information about the social welfare costs of dark patterns. A reasonably high percentage of respondents were willing to forfeit real money rather than continuing to incur the costs of declining an unwanted service or running the risk that they would be signed up for a service they did not want. Of course, by closing their browser and stopping the experiment, there was no guarantee that they would avoid the unwanted subscription. We told respondents at the beginning of the experiment that we had already signed them up for the data protection plan using information they had provided at the beginning of the survey.
In other contexts, scholars have found that people with fewer financial resources have more difficulty overcoming administrative burdens than people with more resources ( Herd & Moynihan 2019 , pp. 7–8, 57–60).
After removing five participants who started and ended the survey on different days, the average completion time was computed. Participants took 9.8 minutes on average to complete the survey. We removed participants who took less than 3 minutes and more than 34.8 minutes (two standard deviations above the survey completion time), as well as participants who did not finish (n=53). Additionally, we removed participants who answered 1 (“not at all seriously”) when asked how seriously they took the survey. The remaining sample comprised 3,932 participants.
Out of 1,979 participants who viewed the consent form from Ydentity Incorporated, 155 (7.8 percent) indicated they had googled the company. The most likely point at which they googled the company would have been when viewing the consent screen, because participants could not go back to that screen and check the name of the company once they advanced to the next screen. However, those who reported googling the company spent less time on the consent screen before advancing ( M = 12.90 seconds) compared to those who indicated they did not google ( M = 18.79). This indicates that some participants who indicated they had googled the company did not; rather, some participants were answering the survey questions quickly and incorrectly indicated they had googled the company. Thus, two types of participants answered “yes” to whether they googled the company: (i) those that actually had tried to search for the company, and (ii) those answering the survey quickly and inattentively. Given the inability to distinguish between these two groups, we removed all participants who indicated they had googled the company. Whether these participants were included did not meaningfully alter the main results of study. For a breakdown of the descriptive statistics with these participants included, see Appendix D.
Males comprised 45.2 percent of the sample. 77.3 percent of the sample self-identified as White, 12.3 percent as Black, 1.3 percent as American Indian, 5.2 percent as Asian, and 3.8 percent as “other.” On a separate question, 13.1 percent indicated they are Hispanic or Latino. 9.5 percent of the sample had not completed high school, 30.8 percent had high school diplomas, 28.9 percent had some college or an associate’s degree, 19.7 percent had bachelor’s degrees, and 11.2 percent had advanced degrees. 13 percent of the sample was between eighteen and twenty-four years, 18.6 percent was between twenty-five and thirty-four years, 19.1 percent was between thirty-five and forty-four years, 13.1 percent was between forty-five and fifty-four years, 17.8 percent was between fifty-five and sixty-four years, and 18.4 percent was sixty-five years or older.
Also embedded in the demographic section was an attention check question. Participants were asked to select a specific answer to a question about their primary mode of transportation, and those who did not select the requested answer were not selected to continue the survey after the demographics section.
Dynata permitted us to ask subjects for their cell phone numbers in Study 1 but changed their practices to prohibit the collection of that data in Study 2.
The participants who took half a standard deviation or less than the average time were coded as having spent less time on the screen, and those who took a half a standard deviation or more were coded as having spent more time on the screen.
Given that subsequent exposure to the trick question or the long obstruction questions may have influenced these variables, we analyzed those participants who were only exposed to the initial dark patterns.
This result differed from what we found in Study 1. A possible explanation is the timing of Study 2, as it occurred during the start of the COVID-19 lockdown in the USA, at a time when less educated / lower wage workers may have felt especially vulnerable and therefore less likely to incur non-essential expenses. Indeed, among those participants who were not exposed to any dark patterns, employment status predicted rates of acceptance. Among those employed full time, 21.1 percent accepted the program. Among those employed part-time, only 12.2 percent accepted. And 17.1 percent of people who reported being unemployed accepted the program; 2.9 percent of those who reported being retired accepted.
Under European data protection law, Article 25 of the GDPR requires data controllers to implement privacy by design and by default (European Union General Data Protection Regulation art. 25 §§ 1–2). These obligations may well prohibit privacy unfriendly default settings, the use of small print, and other dark pattern strategies that are designed to prompt consumers to disclose personal information. Because a lot of dark patterns are designed to purchase a product or service, however, Article 25 is not a comprehensive legal tool that can control dark patterns.
There were five law review citations to the case as of July 12, 2020.
Note the similarity between Office Depot’s computer scans and our bogus calculation of each subject’s “privacy propensity score” in the experiment.
The district court’s opinion, which is published, and which was affirmed in this respect by the Ninth Circuit, provides more detail. ( FTC v. Commerce Planet, Inc ., 878 F. Supp.2d 1048, 1066 (C.D. Cal. 2012)) (“As placed, the disclosure regarding OnlineSupplier's negative option plan is difficult to read because it is printed in the smallest text size on the page and in blue font against a slightly lighter blue background at the very end of the disclosure. The disclosure is also not placed in close proximity to the ‘Ship My Kit!’ button and placed below the fold. It is highly probable that a reasonable consumer using this billing page would not scroll to the bottom and would simply consummate the transaction by clicking the ‘Ship My Kit!’ button, as the consumer is urged to do by the message at the top left: ‘You are ONE CLICK AWAY from receiving the most up-to-date information for making money on eBay!’”).
Take the nagging example. As any parent of verbal kids can attest, a modicum of nagging is entirely tolerable. When kids nag their parents it conveys an intensity of preferences to parents, who may appropriately choose to relent after realizing (on the basis of the persistent nagging) that the requested food, activity, or toy really is very important to the child. That said, the legal system has long recognized that nagging should have its limits. The college student who asks a classmate out on a date once or maybe twice, only to be rebuffed, is behaving within acceptable bounds. As the requests mount in the face of persistent rejection, the questions become harassment. It is plausible that after the fifth or sixth request to turn on notifications is declined, a commercial free speech claim lodged against a policy that prevents further requests becomes weak enough for the restriction to survive Central Hudson scrutiny.
For a competing view, one that rejects the idea that the First Amendment presents a significant obstacle to these sorts of efforts, see Wu (2019 , p. 631).
That is, if a customer wants to be contacted more than the law provides, they would have the right to permit a commercial speaker to do so. This proviso is important to the constitutional analysis, as Mainstream Marketing emphasized that the Do Not Call registry merely established a default.
Albergotti Reed. 2019 . Apple Says Recent Changes to Operating System Improve User Privacy, but Some Lawmakers See Them as an Effort to Edge out Its Rivals, Washington Post , November 26.
Balkin Jack M. 2016 . Information Fiduciaries and the First Amendment . 49 U.C. Davis L. Rev . 1183 – 1234 .
Google Scholar
Balkin Jack M. , Zittrain Jonathan . 2016 . A Grand Bargain to Make Tech Companies Trustworthy, The Atlantic , October 3. https://www.theatlantic.com/technology/archive/2016/10/information-fiduciary/502346/ .
Ben-Shahar Omri , Strahilevitz Lior . 2017 . Interpreting Contracts via Surveys and Experiments . 92 N.Y.U. L. Rev . 1753 – 1827 .
Bösch Christoph , Erb Benjamin, Kargl Frank, Kopp Henning & Pfatteicher Stefan. 2016 . Tales from the Dark Side: Privacy Dark Strategies and Privacy Dark Patterns . 4 Proc. Priv. Enh. Technol . 237 – 254 .
Brandimarte Laura , Acquisti Alessandro & Loewenstein Georgeet al. . 2012 . Misplaced Confidences: Privacy and the Control Paradox . 4 Soc. Psychol. Pers. Sci. 340 – 347 .
Brignull, Harry. 2020 . Types Of Dark Pattern . https://darkpatterns.org/types-of-dark-pattern.html .
Calo Ryan. 2014 . Digital Market Manipulation . 82 Geo. Wash. L. Rev . 995 – 1051 .
Cherie Lacey & Catherine Caudwell. Cuteness as a Dark Pattern in Home Robots. 2019 . 14th ACM/IEEEInternational Conference on Human-Robot Interaction (HRI). https://ieeexplore.ieee.org/document/8673274.
CNET.com. 2020 . Best Identity Theft Protection and Monitoring Services in 2020 . https://www.cnet.com/news/best-identity-theft-protection-monitoring-services-in-2020/ (visited June 28, 2020).
Complaint for Permanent Injunction and Other Equitable Relief, October 7, 2011 , available at 2011 WL 9282853.
Cronqvist Henrick , Thaler Richard H. 2004 . Design Choices in Privatized Social-Security Systems: Learning from the Swedish Experience . 94 Amer. Econ. Rev. Papers & Proceedings 424 – 428 .
Deceptive Experiences to Online Users Reduction Act (DETOUR Act), Senate Bill 1084, 116th Congress, introduced April 9, 2019 .
Di Geronimo Linda, Braz Larissa, Fregnan Enrico, Palomba Fabio & Bacchelli Alberto 2020 . UI Dark Patterns and Where to Find Them: A Study on Mobile Applications and User Perception, CHI 2020 Conference Proceedings 473 . https://dl.acm.org/doi/10.1145/3313831.3376600 .
Federal Trade Commission v. Office Depot , Stipulated Order for Permanent Injunction and Monetary Judgment, Case No. 9-19-cv-80431-RLR (S.D. Fla. March 28, 2019 ). https://www.FTC.gov/system/files/documents/cases/office_depot_stipulated_order_3-29-19.pdf (Office Depot settlement) and https://www.FTC.gov/system/files/documents/cases/office_depot_-_support.com_stipulated_order_3-29-19.pdf (Support.com settlement).
Federal Trade Commission v. Office Depot , Complaint for Permanent Injunction and Other Equitable Relief, Case No. 9-19-cv-80431 (S.D. Fla. March 27, 2019 ). https://www.FTC.gov/system/files/documents/cases/office_depot_complaint_3-27-19.pdf .
Federal Trade Commission, Enforcement Policy Statement on Deceptively Formatted Advertisements (December 22, 2015 ). https://www.FTC.gov/system/files/documents/public_statements/896923/151222deceptiveenforcement.pdf .
Federal Trade Commission v. Bunzai Media Group , Case No. CV 15-4527-GW(PLAx), First Amended Complaint for Permanent Injunction and Other Equitable Relief, (C.D. Cal. October 9, 2015 ). https://www.FTC.gov/system/files/documents/cases/151009bunzaicmpt.pdf
Federal Trade Commission Policy Statement on Unfairness. 1984 . 104 FTC 949.
Gneezy Ayelet, Gneezy Uri & Dominque Olie Lauga et al. 2014 . A Reference-Dependent Model of the Price-Quality Heuristic . 51 J. Marketing Res . 153 – 164 .
Gray Colin M., Kou Yubo, Battles Bryan, Hogatt Joseph & Toombs Austin L et al. . 2018 . The Dark (Patterns) Side of UX Design, Proceedings of the 2018 CHI Conference on Human Factors in Computing Systems , Paper 534 . https://dl.acm.org/doi/10.1145/3173574.3174108 .
Hanson Jon D. , Kysar Douglas A. . 1999 . Taking Behavioralism Seriously: The Problem of Market Manipulation . 74 NYU L. Rev . 632 – 749 .
Hartzog Woodrow. 2018 . Privacy's Blueprint: The Battle to Control the Design of New Technologies . Cambridge, Mass.: Harvard University Press.
Herd Pamela , Moynihan Donald P. . 2019 . Administrative Burden: Policymaking by Other Means . New York : Russell Sage Foundation .
Google Preview
John Leslie K., Acquisti Alessandro & Loewenstein George 2011 . Strangers on a Plane: Context-Dependent Willingness to Disclose Sensitive Information . 37 J. Consumer Res . 858 – 873 .
Junger Marianne, Montoya Lorena & Overink Floris-Jan et al. . 2017. Priming and Warnings Are Not Effective to Prevent Social Engineering Attacks . 66 Computers in Hum. Behav . 75 – 87 .
Kar Robin Bradley , Radin Margaret Jane . 2019 . Pseudo-Contract and Shared Meaning Analysis . 132 Harv. L. Rev . 1135 – 1219 .
Khan Lina , Pozen David . 2019 . A Skeptical View of Information Fiduciaries . 133 Harv. L. Rev . 497 – 541 .
Mathur Arunesh, Acar Gunes, Friedman Michael J., Lucherini Elena, Mayer Jonathan, Chetty Marshini & Narayan Arvind. 2019 . Dark Patterns at Scale: Findings from a Crawl of 11K Shopping Websites , 3 Proc. ACM Hum.-Comput. Interact ., No. CSCW, Article 81.
Mathur Arunesh, Mayer Jonathan & Kshirsagar Mihir 2020 . What Makes a Dark Pattern … Dark?, tUnpublished Draft, presented at Privacy Law Scholars Conference 2020 (on file with author).
McGeveran William. 2016 . Friending the Privacy Regulators . 58 Ariz. L. Rev. 959 – 1025 .
Nosek Brian A., Ebersole Charles R., DeHaven Alexander C. & Mellor David T. et al. . 2018 . The Preregistration Revolution . 115 Proc. Natl. Acad. Sci. U S A 2600 – 2606 .
Nouwens Midas, Liccardi Ilaria, Veale Michael, Karger David & Kagal Lalana et al. . 2020 . Dark Patterns after the GDPR: Scraping Consent Pop-Ups and Demonstrating their Influence, January 8, 2020, presented at 2020 Conference on Human Factors in Computing Systems. https://arxiv.org/pdf/2001.02479.pdf
Pichert Daniel , Katsikopoulos Knonstainos V. 2008 . Green Defaults: Information Presentation and Pro-Environmental Behavior . 28 J. Envtl. Psych . 63 – 73 .
Pomeranz Jennifer L. 2011 . Federal Trade Commission’s Authority to Regulate Marketing to Children: Deceptive vs. Unfair Rulemaking . 21 Health Matrix 521 – 553 .
Restatement (Second) of Contracts. 1981 . Philadelphia: American Law Institute.
Sawchak Matthew , Nelson Kip . 2012 . Defining Unfairness in “Unfair Trade Practices” . 90 N.C.L. Rev . 2033 – 2082 .
Seyferth Shaw LLP. 2019 . Biometric Privacy Class Actions by the Numbers: Analyzing Illinois’ Hottest Class Action Trend , July 2, 2019. https://www.jdsupra.com/legalnews/biometric-privacy-class-actions-by-the-48938/ .
Singletary Michelle. 2019 . Office Depot and Support.com to Pay $35 Million to Settle Charges of Tech Support Scam, Washington Post , March 28.
Snyder Franklin G. , Mirabito Ann M. 2016 . Consumer Preferences for Performance Defaults . 6 Mich. Bus. & Entrepreneurial L. Rev . 35 – 60 .
Solove Daniel J. , Hartzog Woodrow . 2014 . The FTC and the New Common Law of Privacy . 114 Col. L. Rev . 583 – 676 .
Stephanopoulos Nicholas O. , McGhee Eric M. . 2015 . Partisan Gerrymandering and the Efficiency Gap . 82 U. Chi. L. Rev . 831 – 900 .
Strahilevitz Lior , Cranor Lorrie Faith, Marotta-Wurgler Florencia, Mayer Jonathan, Ohm Paul, Strandburg Katherine, Ur Blase, Benthall Sebastian, Lancieri Filippo Maria, Luguri Jamie & Verstraete Mark. 2019 . Subcommittee Report: Privacy and Data Protection, Stigler Center Committee for the Study of Digital Platforms (2019).
Strahilevitz Lior Jacob , Luguri Jamie . 2019 . Consumertarian Default Rules . 82 Law & Contemp. Prob. 139 – 161 .
Strahilevitz Lior Jacob , Kugler Mathew B. . 2016 . Is Privacy Policy Language Irrelevant to Consumers? 45 J. Legal Stud. S69–S95 .
Sunstein Cass R. 2019 . Sludge and Ordeals . 68 Duke L.J . 1843 – 1883 .
Thaler Richard H. , Sunstein Cass. R. . 2009 . Nudge: Improving Decisions about Health, Wealth, and Happiness . New York : Penguin Books .
Thaler Richard H. 2018 . Nudge, Not Sludge . 361 Science 431 .
Tokson Matthew. 2016 . Knowledge and the Fourth Amendment . 111 Nw. U. L. Rev . 139 – 204 .
Valentino-Devries Jennifer. 2019 . How E-Commerce Sites Manipulate You into Buying Things You May Not Want, New York Times , June 24.
Wu Felix T. 2019 . Commercial Speech Protection as Consumer Protection . 90 U. Col. L. Rev . 631 – 651 .
Study 1 - Number of respondents accepting on each screen
Condition . | Total . | Q1 . | Q2 . | Q3 . | Q4 . | Q5 . | Q6 . | Q7 . |
---|---|---|---|---|---|---|---|---|
Description . | . | Accept/ Options . | Other options . | Info 1 . | Info 2 . | Info 3 . | Trick . | Reason . |
Control group | 73 | 73 | n/a | n/a | n/a | n/a | n/a | n/a |
Mild | 155 | 117 | 35 | n/a | n/a | n/a | n/a | 3 |
Aggressive | 217 | 141 | 22 | 11 | 10 | 8 | 24 | 1 |
Condition . | Total . | Q1 . | Q2 . | Q3 . | Q4 . | Q5 . | Q6 . | Q7 . |
---|---|---|---|---|---|---|---|---|
Description . | . | Accept/ Options . | Other options . | Info 1 . | Info 2 . | Info 3 . | Trick . | Reason . |
Control group | 73 | 73 | n/a | n/a | n/a | n/a | n/a | n/a |
Mild | 155 | 117 | 35 | n/a | n/a | n/a | n/a | 3 |
Aggressive | 217 | 141 | 22 | 11 | 10 | 8 | 24 | 1 |
Study 1 - Acceptance rates by service cost and dark pattern dosage
Condition . | Overall (% accept) . | Low stakes (%) . | High stakes (%) . |
---|---|---|---|
Control group | 11.3 | 9.3 | 13.3 |
Mild | 25.8 | 26.8 | 24.9 |
Aggressive | 41.9 | 40.9 | 42.8 |
Condition . | Overall (% accept) . | Low stakes (%) . | High stakes (%) . |
---|---|---|---|
Control group | 11.3 | 9.3 | 13.3 |
Mild | 25.8 | 26.8 | 24.9 |
Aggressive | 41.9 | 40.9 | 42.8 |
All pairwise comparisons of acceptance rates across dark pattern condition are significant at p < 0.001. Chi-square tests for independence were run separately for each of the dark pattern conditions. There was no significant relationship between stakes and acceptance rates in the control group ( χ (1, N = 644) = 2.52, p = 0.11), mild ( χ (1, N = 600) = 0.27, p = 0.61), or aggressive ( χ (1, N = 518) = 0.19, p = 0.66) conditions.
Study 2 - Acceptance rates by content and form condition
. | Control . | Recommended . | Default . | Obstruction . |
---|---|---|---|---|
Control | 13.2% | 15.1% | 15.0% | 19.5% |
= 0.46 | = 0.49 | = 0.03 | ||
Scarcity | 10.6% | 10.8% | 18.9% | 17.4% |
= 0.39 | = .41 | = .061 | = 0.19 | |
Confirmshaming | 20.5% | 16.4% | 21.0% | 20.4% |
= 0.02 | = .29 | = 0.012 | = 0.03 | |
Social proof | 19.0% | 21.0% | 21.4% | 27.9% |
= 0.053 | = .01 | = 0.009 | < 0.001 | |
Hidden information | 30.8% | 28.7% | 26.7% | 34.5% |
< 0.001 | < 0.001 | < 0.001 | < 0.001 |
. | Control . | Recommended . | Default . | Obstruction . |
---|---|---|---|---|
Control | 13.2% | 15.1% | 15.0% | 19.5% |
= 0.46 | = 0.49 | = 0.03 | ||
Scarcity | 10.6% | 10.8% | 18.9% | 17.4% |
= 0.39 | = .41 | = .061 | = 0.19 | |
Confirmshaming | 20.5% | 16.4% | 21.0% | 20.4% |
= 0.02 | = .29 | = 0.012 | = 0.03 | |
Social proof | 19.0% | 21.0% | 21.4% | 27.9% |
= 0.053 | = .01 | = 0.009 | < 0.001 | |
Hidden information | 30.8% | 28.7% | 26.7% | 34.5% |
< 0.001 | < 0.001 | < 0.001 | < 0.001 |
Acceptance rates by content and form condition, with p -values comparing each condition to the full control condition.
Study 2 - Results with respondents who reported googling Ydentity, Inc. included
Condition . | Acceptance rate (%) . | |
---|---|---|
Content condition | ||
Control group | 17.3 | |
Scarcity | 16.8 | |
Confirmshaming | 22.1 | |
Social proof | 23.8 | |
Hidden information | 32.6 | |
Form condition | ||
Control group | 19.4 | |
Recommended | 19.8 | |
Default | 21.7 | |
Obstruction | 27.0 | |
Stakes condition | ||
Low stakes: After initial screen | 21.9 | |
Final choice | 28.5 | |
High stakes: After initial screen | 20.4 | |
Final choice | 28.5 | |
Education | Control (%) | Treatment (%) |
High school diploma or less | 9.2 | 18.9 |
Some college or associate’s degree | 20.2 | 24.2 |
Bachelor degree or higher | 21.6 | 25.7 |
Condition . | Acceptance rate (%) . | |
---|---|---|
Content condition | ||
Control group | 17.3 | |
Scarcity | 16.8 | |
Confirmshaming | 22.1 | |
Social proof | 23.8 | |
Hidden information | 32.6 | |
Form condition | ||
Control group | 19.4 | |
Recommended | 19.8 | |
Default | 21.7 | |
Obstruction | 27.0 | |
Stakes condition | ||
Low stakes: After initial screen | 21.9 | |
Final choice | 28.5 | |
High stakes: After initial screen | 20.4 | |
Final choice | 28.5 | |
Education | Control (%) | Treatment (%) |
High school diploma or less | 9.2 | 18.9 |
Some college or associate’s degree | 20.2 | 24.2 |
Bachelor degree or higher | 21.6 | 25.7 |
The main results from Study 2, including participants who indicated they had googled Ydentity Incorporated.
Month: | Total Views: |
---|---|
March 2021 | 797 |
April 2021 | 1,198 |
May 2021 | 768 |
June 2021 | 866 |
July 2021 | 546 |
August 2021 | 665 |
September 2021 | 671 |
October 2021 | 811 |
November 2021 | 917 |
December 2021 | 638 |
January 2022 | 830 |
February 2022 | 834 |
March 2022 | 918 |
April 2022 | 924 |
May 2022 | 1,322 |
June 2022 | 783 |
July 2022 | 615 |
August 2022 | 1,042 |
September 2022 | 2,254 |
October 2022 | 1,398 |
November 2022 | 1,153 |
December 2022 | 935 |
January 2023 | 872 |
February 2023 | 867 |
March 2023 | 1,173 |
April 2023 | 1,045 |
May 2023 | 1,044 |
June 2023 | 732 |
July 2023 | 774 |
August 2023 | 1,365 |
September 2023 | 2,499 |
October 2023 | 1,459 |
November 2023 | 1,513 |
December 2023 | 1,242 |
January 2024 | 1,545 |
February 2024 | 1,709 |
March 2024 | 1,955 |
April 2024 | 1,653 |
May 2024 | 1,256 |
June 2024 | 891 |
July 2024 | 1,140 |
August 2024 | 1,221 |
Email alerts
Citing articles via, affiliations.
- Online ISSN 1946-5319
- Print ISSN 2161-7201
- Copyright © 2024
- About Oxford Academic
- Publish journals with us
- University press partners
- What we publish
- New features
- Open access
- Institutional account management
- Rights and permissions
- Get help with access
- Accessibility
- Advertising
- Media enquiries
- Oxford University Press
- Oxford Languages
- University of Oxford
Oxford University Press is a department of the University of Oxford. It furthers the University's objective of excellence in research, scholarship, and education by publishing worldwide
- Copyright © 2024 Oxford University Press
- Cookie settings
- Cookie policy
- Privacy policy
- Legal notice
This Feature Is Available To Subscribers Only
Sign In or Create an Account
This PDF is available to Subscribers Only
For full access to this pdf, sign in to an existing account, or purchase an annual subscription.
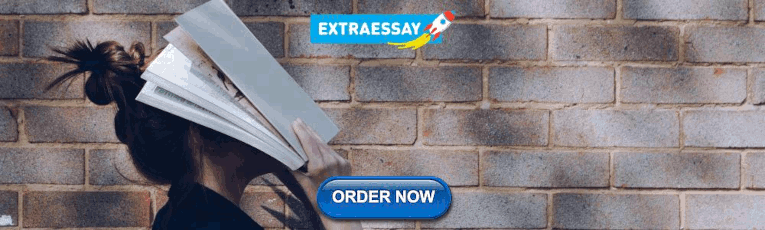
IMAGES
VIDEO
COMMENTS
Study with Quizlet and memorize flashcards containing terms like Jamie is writing a research paper about wind patterns. Which digital resource should he consult?, Match each statement to the type of behavior it describes., _____ is an important discipline of Earth and space science that focuses on events in the atmosphere. Scientists of this discipline also research Earth's changing _____.
Jamie is writing a research paper about wind patterns. Which digital resource should he consult? A. an article titled "Shifting Winds" published by the US government B. a Wikipedia page dedicated to the study of global wind patterns C. an environmental enthusiast's blog page titled "Winds of Change" D. a middle school student's research paper ...
Jamie is writing a research paper about wind patterns. Which digital resource should he consult? A. an article titled "Shifting Winds" published by the US government B. a Wikipedia page dedicated to the study of global wind patterns C. an environmental enthusiast's blog page titled "Winds of Change" D.
Jamie is writing a research paper about wind patterns. Which digital resource should he consult? Get the answers you need, now! ... There are many digital resources that offer help in the research paper for wind patterns.Google apps for education, NC wise owl, SAS curriculum pathways , geography apps can be used for the research.Google search ...
Jamie is writing a research paper about wind patterns. Which digital resource should he consult? Click the card to flip. A. an article titled "Shifting Winds" published by the US government ... Jamie is writing a research paper about wind patterns. Which digital resource should he consult?
Jamie is writing a research paper about wind patterns. Which digital resource should he consult? A. an article titled "Shifting Winds" published by the US government B. a Wikipedia page dedicated to the study of global wind patterns C. an environmental enthusiast's blog page titled "Winds of Change" D.
2. Determine the type of resource needed: Since Jamie is writing a research paper, he needs a reliable and credible digital resource. Answer 3. Suggest a suitable digital resource: A suitable digital resource for researching wind patterns could be a scientific journal, a government website, or a reputable organization's website.
Question: jamie is writing a research paper about wind patterns which digital resource should he consult. Here's the best way to solve it. Identify key reliable sources that focus on meteorological data and wind patterns, like government research papers and international organizations' websites. There are n number of digital resources that ...
As we now know, three types of prevailing winds on the Earth form three major global wind belts created due to the Coriolis Effect. They are named based on their origin and the area where they blow. We will now describe them in more detail. 1. Tropical Easterlies (Trade Winds) Location - 0° to 30° Latitude.
This section develops an integrative framework for quanti- fying the day-to-day and spatial variability in wind's diurnal pattern at non-observational locations. The underlying idea is as follows. We formulate the wind model using trigonometric functions to characterize a nonstationary pattern.
Wind power generation is a subject that has been widely analyzed in the last 20 years and much attention has been given by researchers around the world to short-run forecasting and related issues, leaving a gap especially in review studies and analysis focused on medium- and long-term forecasting.
Jamie is writing a research paper about wind patterns. Which digital resource should he consult? an article titled "Shifting Winds" published by the US government. ... a middle school student's research paper exploring wind patterns. 5 of 29. Term. Select the correct answer.
Select the correct answer. Jamie is writing a research paper about wind patterns. Which digital resource should he consult? A. an article titled "Shifting Winds" published by the US government B. a Wikipedia page dedicated to the study of global wind pattems C. an environmental enthusiast's blog page titled "Winds of Change" D. a middle school student's research paper exploring wind patterns
The general-to-specific pattern is a common pattern in college writing. Although you can use it as an overall essay structure, writers find it most useful when writing introductions. Here are some examples. Introduce an essay or other, larger work. Introduce essay examination answers. Introduce or transition into a discussion of synthesized ...
Key Takeaways. The problem-cause-solution approach will first describe the problem, then analyze the cause or responses to the problem, and then will lead to a solution. We practice this approach daily in our interactions with others, whether at work or home. Mailing Address: 3501 University Blvd. East, Adelphi, MD 20783.
Select the correct answer. Jamie is writing a research paper about wind patterns. Which digital resource should he consult? A. an article titled "Shifting Winds" published by the US government B. a Wikipedia page dedicated to the study of global wind patterns C. an environmental enthusiast's blog page titled "Winds of Change" D. a middle school student's research paper exploring ...
Falsifiable Fallibility is the capacity for some proposition, statement, theory or hypothesis to be proven wrong.
Kinds of Assignments You Will Write. Critical Strategies and Writing. Analysis. Synthesis. Evaluation. Persuasion. Developing a Paper Using Strategies. Patterns for Presenting Information. General-to-Specific Pattern.
A good research question is essential to guide your research paper, dissertation, or thesis. All research questions should be: Focused on a single problem or issue. Researchable using primary and/or secondary sources. Feasible to answer within the timeframe and practical constraints. Specific enough to answer thoroughly.
Jamie is writing a research paper about wind patterns. Which digital resource should he consult? an article titled "Shifting Winds" published by the US government a Wikipedia page dedicated to the study of global wind patterns an environmental enthusiast's blog page titled "Winds of Change" a middle school student's research paper exploring wind patterns
In the word search below are the names of several pieces of lab equipment. As you find each piece of equipment, record its name on the list. There are only 13 words out of the listBunsen burner,Pipestem triangle, Evaporating dish, Beaker, Utility clamp,Iron ring, Mortar and pestle, Crucible and cover, Gas bottle, Saftey goggles,Corks, Watch glass, Erlenmeyer flask, Wire gauze, Pipet, Buret ...
Drag the tiles to the correct boxes to complete the pairs. Match each earth and space science discipline to its description . The study of space around earth, and within the universe - astronomy. The study of earths rocky surface - geology. The study of the complex interactions - environmental science. Drag each statement to the correct location.
Arunesh Mathur and six co-authors developed a semi-automated method for crawling more than 11,000 popular shopping websites. Their analysis revealed the presence of dark patterns on more than 11 percent of those sites, and the most popular sites were also most likely to employ dark patterns.