Thank you for visiting nature.com. You are using a browser version with limited support for CSS. To obtain the best experience, we recommend you use a more up to date browser (or turn off compatibility mode in Internet Explorer). In the meantime, to ensure continued support, we are displaying the site without styles and JavaScript.
- View all journals
- Explore content
- About the journal
- Publish with us
- Sign up for alerts
- Published: 18 February 2021
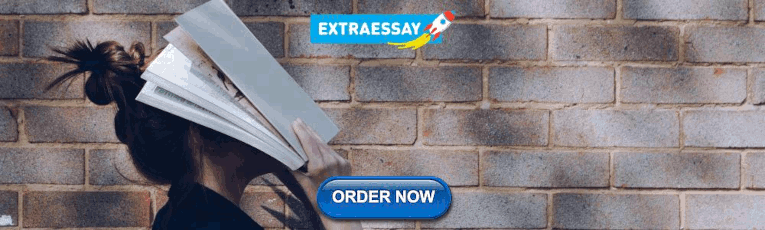
Essentials of data management: an overview
- Miren B. Dhudasia 1 , 2 ,
- Robert W. Grundmeier 2 , 3 , 4 &
- Sagori Mukhopadhyay 1 , 2 , 3
Pediatric Research volume 93 , pages 2–3 ( 2023 ) Cite this article
4037 Accesses
4 Citations
5 Altmetric
Metrics details
What is data management?
Data management is a multistep process that involves obtaining, cleaning, and storing data to allow accurate analysis and produce meaningful results. While data management has broad applications (and meaning) across many fields and industries, in clinical research the term data management is frequently used in the context of clinical trials. 1 This editorial is written to introduce early career researchers to practices of data management more generally, as applied to all types of clinical research studies.
Outlining a data management strategy prior to initiation of a research study plays an essential role in ensuring that both scientific integrity (i.e., data generated can accurately test the hypotheses proposed) and regulatory requirements are met. Data management can be divided into three steps—data collection, data cleaning and transformation, and data storage. These steps are not necessarily chronological and often occur simultaneously. Different aspects of the process may require the expertise of different people necessitating a team effort for the effective completion of all steps.
Data collection
Data source.
Data collection is a critical first step in the data management process and may be broadly classified as “primary data collection” (collection of data directly from the subjects specifically for the study) and “secondary use of data” (repurposing data that were collected for some other reason—either for clinical care in the subject’s medical record or for a different research study). While the terms retrospective and prospective data collection are occasionally used, 2 these terms are more applicable to how the data are utilized rather than how they are collected . Data used in a retrospective study are almost always secondary data; data collected as part of a prospective study typically involves primary data collection, but may also involve secondary use of data collected as part of ongoing routine clinical care for study subjects. Primary data collected for a specific study may be categorized as secondary data when used to investigate a new hypothesis, different from the question for which the data were originally collected. Primary data collection has the advantage of being specific to the study question, minimize missingness in key information, and provide an opportunity for data correction in real time. As a result, this type of data is considered more accurate but increases the time and cost of study procedures. Secondary use of data includes data abstracted from medical records, administrative data such as from the hospital’s data warehouse or insurance claims, and secondary use of primary data collected for a different research study. Secondary use of data offers access to large amounts of data that are already collected but often requires further cleaning and codification to align the data with the study question.
A case report form (CRF) is a powerful tool for effective data collection. A CRF is a paper or electronic questionnaire designed to record pertinent information from study subjects as outlined in the study protocol. 3 CRFs are always required in primary data collection but can also be useful in secondary use of data to preemptively identify, define, and, if necessary, derive critical variables for the study question. For instance, medical records provide a wide array of information that may not be required or be useful for the study question. A CRF with well-defined variables and parameters helps the chart reviewer focus only on the relevant data, and makes data collection more objective and unbiased, and, in addition, optimize patient confidentiality by minimizing the amount of patient information abstracted. Tools like REDCap (Research Electronic Data Capture) provide electronic CRFs and offer some advanced features like setting validation rules to minimize errors during data collection. 4 Designing an effective CRF upfront during the study planning phase helps to streamline the data collection process, and make it more efficient. 3
Data cleaning and transformation
Quality checks.
Data collected may have errors that arise from multiple sources—data manually entered in a CRF may have typographical errors, whereas data obtained from data warehouses or administrative databases may have missing data, implausible values, and nonrandom misclassification errors. Having a systematic approach to identify and rectify these errors, while maintaining a log of the steps performed in the process, can prevent many roadblocks during analysis.
First, it is important to check for missing data. Missing data are defined as values that are not available and that would be meaningful for analysis if they were observed. 5 Missing data can bias the results of the study depending on how much data is missing and what is the pattern of distribution of missing data in the study cohort. Many methods for handling missing data have been published. Kang 6 provide a practical review of methods for handling missing data. If missing data cannot be retrieved and is limited to only a small number of subjects, one approach is to exclude these subjects from the study. Missing data in different variables across many subjects often require more sophisticated approaches to account for the “missingness.” These may include creating a category of “missing” (for categorical variables), simple imputation (e.g., substituting missing values in a variable with an average of non-missing values in the variable), or multiple imputations (substituting missing values with the most probable value derived from other variables in the dataset). 7
Second, errors in the data can be identified by running a series of data validation checks. Some examples of data validation rules for identifying implausible values are shown in Table 1 . Automated algorithms for detection and correction of implausible values may be available for cleaning specific variables in large datasets (e.g., growth measurements). 8 After identification, data errors can either be corrected, if possible, or can be marked for deletion. Other approaches, similar to those for dealing with missing data, can also be used for managing data errors.
Data transformation
The data collected may not be in the form required for analysis. The process of data transformation includes recategorization and recodification of the data, which has been collected along with derivation of new variables, to align with the study analytic plan. Examples include categorizing body mass index collected as a continuous variable into under- and overweight categories, recoding free-text values such as “growth of an organism” or “no growth,” and into a binary “positive” or “negative,” or deriving new variables such as average weight per year from multiple weight values over time available in the dataset. Maintaining a code-book of definitions for all variables, predefined and derived, can help a data analyst better understand the data.
Data storage
Securely storing data is especially important in clinical research as the data may contain protected health information of the study subjects. 9 Most institutes that support clinical research have guidelines for safeguards to prevent accidental data breaches.
Data are collected in paper or electronic formats. Paper data should be stored in secure file cabinets inside a locked office at the site approved by the institutional review board. Electronic data should be stored on a secure approved institutional server, and should never be transported using unencrypted portable media devices (e.g., “thumb drives”). If all study team members do not require access to study data, then selective access should be granted to the study team members based on their roles.
Another important aspect of data storage is data de-identification. Data de-identification is a process by which identifying characteristics of the study participants are removed from the data, in order to mitigate privacy risks to individuals. 10 Identifying characteristics of a study subject includes name, medical record number, date of birth/death, and so on. To de-identify data, these characteristics should either be removed from the data or modified (e.g., changing the medical record number to study IDs, changing dates to age/duration, etc.). If feasible, study data should be de-identified when storing. If you anticipate that reidentification of the study participants may be required in future, then the data can be separated into two files, one containing only the de-identified data of the study participants, and one containing all the identifying information, with both files containing a common linking variable (e.g., study ID), which is unique for every subject or record in the two files. The linking variable can be used to merge the two files when reidentification is required to carry out additional analyses or to get further data. The link key should be maintained in a secure institutional server accessible only to authorized individuals who need access to the identifiers.
To conclude, effective data management is important to the successful completion of research studies and to ensure the validity of the results. Outlining the steps of the data management process upfront will help streamline the process and reduce the time and effort subsequently required. Assigning team members responsible for specific steps and maintaining a log, with date/time stamp to document each action as it happens, whether you are collecting, cleaning, or storing data, can ensure all required steps are done correctly and identify any errors easily. Effective documentation is a regulatory requirement for many clinical trials and is helpful for ensuring all team members are on the same page. When interpreting results, it will serve as an important tool to assess if the interpretations are valid and unbiased. Last, it will ensure the reproducibility of the study findings.
Krishnankutty, B., Bellary, S., Kumar, N. B. & Moodahadu, L. S. Data management in clinical research: an overview. Indian J. Pharm. 44 , 168–172 (2012).
Article Google Scholar
Weinger, M. B. et al. Retrospective data collection and analytical techniques for patient safety studies. J. Biomed. Inf. 36 , 106–119 (2003).
Avey, M. in Clinical Data Management 2nd edn. (eds Rondel, R. K., Varley, S. A. & Webb, C. F.) 47–73 (Wiley, 1999).
Harris, P. A. et al. Research electronic data capture (REDCap)—a metadata-driven methodology and workflow process for providing translational research informatics support. J. Biomed. Inf. 42 , 377–381 (2009).
Little, R. J. et al. The prevention and treatment of missing data in clinical trials. N. Engl. J. Med. 367 , 1355–1360 (2012).
Article CAS Google Scholar
Kang, H. The prevention and handling of the missing data. Korean J. Anesthesiol. 64 , 402 (2013).
Rubin, D. B. Inference and missing data. Biometrika 63 , 581–592 (1976).
Daymont, C. et al. Automated identification of implausible values in growth data from pediatric electronic health records. J. Am. Med. Inform. Assoc. 24 , 1080–1087 (2017).
Office for Civil Rights, Department of Health and Human Services. Health insurance portability and accountability act (HIPAA) privacy rule and the national instant criminal background check system (NICS). Final rule. Fed. Regist. 81 , 382–396 (2016).
Google Scholar
Office for Civil Rights (OCR). Methods for de-identification of PHI. https://www.hhs.gov/hipaa/for-professionals/privacy/special-topics/de-identification/index.html (2012).
Download references
Acknowledgements
This work was partially supported in part by the Eunice Kennedy Shriver National Institute of Child Health & Human Development of the National Institutes of Health grant (K23HD088753).
Author information
Authors and affiliations.
Division of Neonatology, Children’s Hospital of Philadelphia, Philadelphia, PA, USA
Miren B. Dhudasia & Sagori Mukhopadhyay
Center for Pediatric Clinical Effectiveness, Children’s Hospital of Philadelphia, Philadelphia, PA, USA
Miren B. Dhudasia, Robert W. Grundmeier & Sagori Mukhopadhyay
Department of Pediatrics, University of Pennsylvania Perelman School of Medicine, Philadelphia, PA, USA
Robert W. Grundmeier & Sagori Mukhopadhyay
Department of Biomedical and Health Informatics, Children’s Hospital of Philadelphia, Philadelphia, PA, USA
Robert W. Grundmeier
You can also search for this author in PubMed Google Scholar
Corresponding author
Correspondence to Sagori Mukhopadhyay .
Ethics declarations
Competing interests.
The authors declare no competing interests.
Additional information
Publisher’s note Springer Nature remains neutral with regard to jurisdictional claims in published maps and institutional affiliations.
Rights and permissions
Reprints and permissions
About this article
Cite this article.
Dhudasia, M.B., Grundmeier, R.W. & Mukhopadhyay, S. Essentials of data management: an overview. Pediatr Res 93 , 2–3 (2023). https://doi.org/10.1038/s41390-021-01389-7
Download citation
Received : 11 December 2020
Revised : 27 December 2020
Accepted : 06 January 2021
Published : 18 February 2021
Issue Date : January 2023
DOI : https://doi.org/10.1038/s41390-021-01389-7
Share this article
Anyone you share the following link with will be able to read this content:
Sorry, a shareable link is not currently available for this article.
Provided by the Springer Nature SharedIt content-sharing initiative
This article is cited by
Advancing clinical and translational research in germ cell tumours (gct): recommendations from the malignant germ cell international consortium.
- Adriana Fonseca
- Matthew J. Murray
British Journal of Cancer (2022)
Quick links
- Explore articles by subject
- Guide to authors
- Editorial policies

Clinical Trial Data Management and Professionals
By Andy Marker | January 16, 2020 (updated September 16, 2021)
- Share on Facebook
- Share on LinkedIn
Link copied
This guide provides professionals with everything they need to understand clinical data management, offering expert advice, templates, graphics, and a sample clinical data management plan.
Included on this page, you'll find information on how to become a clinical trial data manager , a clinical data management plan template , a clinical data validation plan template , and much more.
What Is Clinical Trial Management?
Clinical trial management refers to the structured, organized regulatory approach that managers take in clinical trial projects to produce timely and efficient project outcomes. It includes developing and maintaining specified or general software systems, processes, procedures, training, and protocols.
What Is a Clinical Trial Management System (CTMS)?
A clinical trial management system (CTMS) is a type of project management software specific to clinical research and clinical data management. It allows for centralized planning, reporting, and tracking of all aspects of clinical trials, with the end goal of ensuring that the trials are efficient, compliant, and successful, whether across one or several institutions.
Companies use CTMS for their clinical data management to ensure they build trust with regulatory agencies. Trust is earned as the companies collect, integrate, and validate their clinical trial data with integrity over time. A comprehensive system helps them do so.
In a 2017 paper, “ Artificial intelligence based clinical data management systems: A review ,” Gazali discusses CTMS and what makes it worthwhile for investigators — namely, that it helps to authenticate data. Accurate study results and a trail of data collection, as collected through a quality CTMS, lend credence to research study data. Clinical trial data management systems enable researchers to adhere to quality standards and provide assurance that they are appropriately collecting, cleaning, and managing the data.
A clinical data management system also offers remote data monitoring. The sponsor, or principal investigator, may want to monitor the trial from a distance, especially if the organization has many sites. Since the FDA mandates monitoring in clinical trials, and many studies generally consider it a large cost, remote monitoring offers a lower-priced option in which sponsors can identify issues and outliers and mitigate them quickly.
Many data management systems are also incorporating artificial intelligence (AI). AI-based clinical data management systems support process automation, data insights analysis, and critical decision making. All of this can happen as your staff inputs the research data. According to a review of clinical data management systems , researchers note that automating all dimensions of clinical data management in trials can take them from mere electronic data capture to something that helps with findings in clinical trials.
The most helpful strategies for implementing clinical data management systems balance risk reduction and lead time. All trial managers want to have their software deployed rapidly. However, it is best to set up the databases thoroughly before the trial. When staff must make software changes during the trial, it can be costly and have implications on the trial data’s validity.
Other strategies that help organizations implement a new system include making sure that, prior to deployment, the intended users give input. These users include entities such as the contract research organization (CRO), the sponsor, staff at the investigator site, and any onsite technical support. Staff should respond well to the graphical user interface (GUI). Additionally, depending on software support, the staff can gradually expand the modules to include more functionality, perform module-based programming, and duplicate the hardware. These actions give the staff the most functionality and the software the best chance at success.
How to Compare Clinical Data Management Systems
When deciding which clinical data management system to use, compare the program’s available features and those that your clinical sites need. Additionally, you can compare clinical data management systems by reviewing the installation platforms, pricing, technical support, and number of allowed users.
For programs that collect data on paper and send it to data entry staff, the data entry portal should be simple and allow for double entry and regular oversight.
In general, here are the main features to compare in a clinical data management system:
- 21 CFR Part 11 Compliance: Electronic systems must provide assurance of authentic records.
- Document Management: All documents should be in a centralized location, linked to their respective records.
- Electronic Data Capture (EDC): Direct clinical trial data collection, as opposed to paper forms.
- Enrollment Management: Research studies can use this data (from interactive web or voice response systems) to enroll, randomize, and manage patients.
- HIPAA compliance: Ensure compliance with the Health Insurance Portability and Accountability Act to protect patients’ information.
- Installation: Identify whether you want a cloud-based or on-premises solution and if you need mobile deployment (iOS or Android).
- Investigator and Site Profiling: Use this function to rapidly identify the feasibility of possible investigators and sites.
- Monitoring: The system should offer a calendar, scheduling capabilities, adverse and severe adverse event tracking, trip reporting, site communication, and triggers.
- Number of Users: How many users can the software can handle? Is there a minimum number of required users? Does the software provide levels of accessibility and price based on the number of users?
- Patient Database: Separate from recruitment and enrollment, the patient database is a record of previous contacts that you can potentially draw from for future trials.
- Payment Portal: Pay out stipends, contracts, and other finances related to the research project.
- Pricing: Check whether the software company offers free trials, free or premium options, monthly subscriptions, annual subscriptions, or a one-time license.
- Recruiting Management: This function helps streamline recruitment by targeting potential trial patients with web recruitment and prescreening.
- Scheduling: Use this feature to keep track of visits and events.
- Study Planning and Workflows: This function enables you to track all required study pieces from the beginning and optimize each piece with workflows.
- Support: Check if the software company offers 24-hour issue support and training on the software.
What Is Clinical Data Management?
Clinical data management (CDM) is the part of clinical trial management that deals specifically with information that comes out of the trials. In order to yield ethical, repeatable results, researchers must document their patients’ medical status — including everything relative to that status — and the trial’s interventions.
Clinical data management evolved from drug companies’ need for an honest path from their research to their findings; in short, their data had to be reproducible. CDM helps evolve a standards-based approach, and many regulators are continually imposing their requirements on it. For instance, paper is no longer favored as a collection method; most clinical trials prefer software systems that improve the timeliness and quality of data.
In one model for data management, the cycle begins when the clinical trial is in the planning stages and goes through the final analysis and lockdown of the data. The stages for data management are as follows:
- Plan: The data manager prepares the database, forms, and overall plan.
- Collect: Staff gathers data in the course of the trial.
- Assure: The data manager determines if the data plan and tools meet the requirements.
- Identify: Staff and the data manager identify any issues or risks.
- Preserve: The data manager preserves the data already collected and mitigates risks.
- Integrate: The data manager oversees different datasets and information mapped together for consistency.
- Analyze: The statisticians analyze the mapped data trends and outcomes.
- Lock: The data manager locks the database for integrity.
Model for Data Management in Clinical Trials
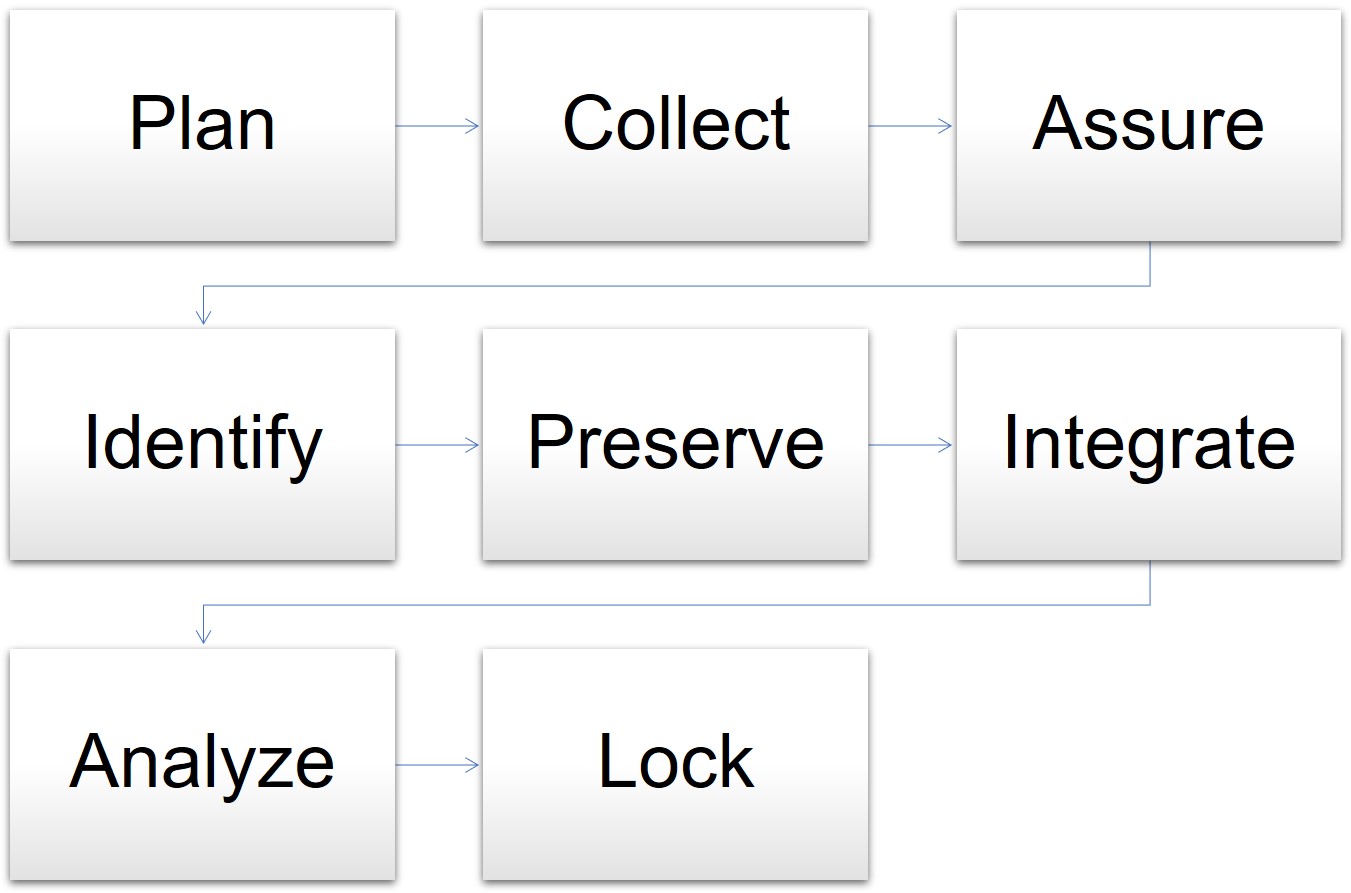
When it comes to data, clinical research has several areas of responsibility. Sponsors can split these functions among several staff or, in smaller studies, assign them to the main data manager. These functions include the following:
Clinical systems: Any software or technology used.
Data management: Data acquisition, coding, and standardization.
Data review and analytics: Quality management, auditing, and statistical analysis of the collected data.
Data standards: Checking against regulatory requirements.
Innovation: Using tools and theory that coordinate with the developing field. For more innovative templates to use in clinical trials, see “ Clinical Trial Templates to Start Your Clinical Research .”
Clinical Research Data Areas of Responsibility
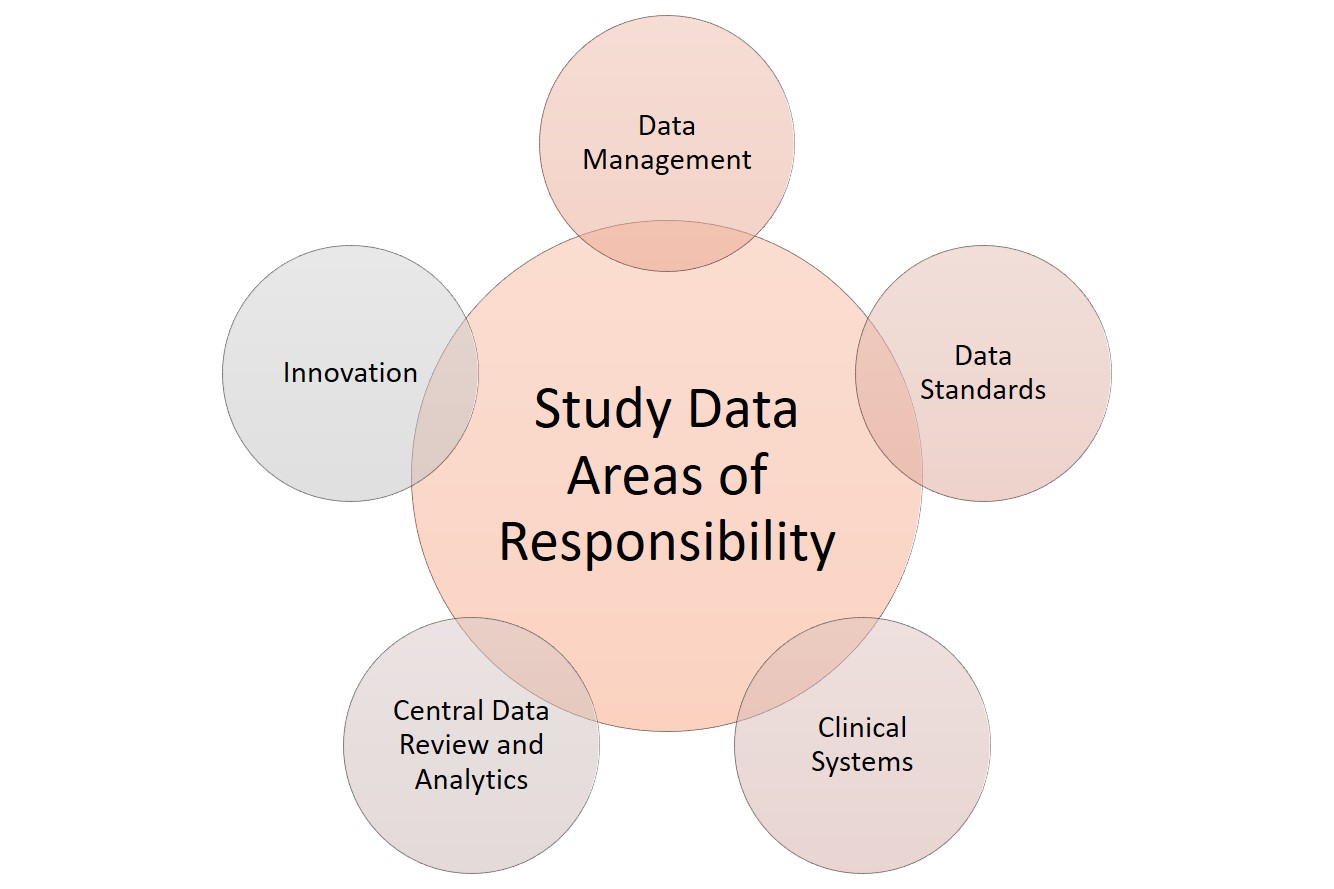
Clinical data management is one of the most critical functions in overall clinical trial management. Staff collects data from many different sources in a clinical trial — some will necessarily be from paper forms filled out by the patient, their representative, or a staff member on their behalf. However, instead of paper, some clinics may use devices such as tablets or iPads to fill out this direct-entry data electronically.
Clinical data management also includes top-line data , such as the demographic data summary, the primary endpoint data, and the safety data. Together, this constitutes the executive summary for clinical trials. Companies often issue this data as a part of press releases. Additional clinical trial data management activities include the following:
- Case report form (CRF) design, annotation, and tracking
- Central lab data
- Data abstraction and extraction
- Data archiving
- Data collection
- Data entry and validation
- Data extraction
- Data queries and analysis
- Data storage and privacy
- Data transmission
- Database design, build, and testing
- Database locking
- Discrepancy management
- Medical data coding and processing
- Patient recorded data
- Severe adverse event (SAE) reconciliation
- Study metrics and tracking
- Quality control and assurance
- User acceptance testing
- Validation checklist
Since there are many different types of data coming from many different sources, some data managers have become experts in hybrid data management — the synchronization required to not only make disparate data relate to each other, but also to adequately manage each type of data. For example, one study could generate data on paper from both the trial site and from a contract research organization, electronic data from the site, and clinical data measurements from a laboratory.
The Roles and Responsibilities in Clinical Data Management
Clinical data management software assigns database access limitations based on the assigned roles and responsibilities of the users. This coding ensures there is an audit trail and the users can only access their respective required functionalities, without the ability to make other changes.
All staff members, whether a manager, programmer, administrator, medical coder, data coordinator, quality control staff, or data entry person, have differing levels of access to the software system, as delineated in the protocol. The principle investigator can use the CDMS to restrict these access levels.
What Is Clinical Trial Data Management (CDM)?
Clinical trial data management (CDM) is the process of a program or study collecting, cleaning, and managing subject and study data in a way that complies with internal protocols and regulatory requirements. It is simultaneously the initial phase in a clinical trial, a field of study, and an aspirational model.
With properly collected data in clinical trials, the study can progress and result in reliable, high-quality, statistically appropriate conclusions. Proper data collection also decreases the time from drug development to marketing. Further, proper data collection involves a multidisciplinary team, such as the research nurses, clinical data managers, investigators, support personnel, biostatisticians, and database programmers. Finally, CDM enables high-quality, understandable research, which can be capitalized on in its field and across many disciplines, according to the National Institutes of Health (NIH).
In clinical trials, data managers perform setup during the trial development phase. Data comes from the primary sources, such as site medical records, laboratory results, and patient diaries. If the project uses paper-based CRFs, staff members must transcribe them, then enter this source data into a clinical trial database. They enter paper-based forms twice, known as double data entry, and compare them, per best practice. This process significantly decreases the error rate from data entry mistakes. Electronic CRFs (eCRFs) enable staff to enter source data directly into the database.
As with any project, the financial and human resources in clinical trials are finite. Coming up with and sticking to a solid data management plan is crucial — it should include structure for the research personnel, resources, and storage. A clinical trial is a huge investment of time, people, and money. It warrants expert-level management from its inception.
Clinical Data Management Plans
Clinical data management plans (DMPs) outline all the data management work needed in a clinical research project. This includes the timeline, any milestones, and all deliverables, as well as strategies for how the data manager will deal with disparate data sets.
Regulators do not require a DMP, but they expect and audit them in clinical research. Thus, the DMPs should be comprehensive and all stakeholders should agree on them. They should also be living documents that staff regularly updates as the study evolves and the various study pieces develop.
For example, during one study, the study manager might change the company used for laboratory work. This affects the DMP in two ways: First, staff needs to develop the data sharing agreement with the new company, and second, they need to integrate the data from both laboratories into one dataset at the end of the trial. The DMP should describe both.
When creating DMPs, you should also bear in mind any industry data standards, so the research can also be valuable outside of the discrete study. The Clinical Data Acquisitions Standards Harmonization (CDASH) recommends 16 standards for data collection fields for consistency in data across different studies.
The final piece of standardization in DMPs is the use of a template, which provides staff with a solid place to start developing a DMP specific to their study. Sponsors may have a standard template they use across their projects to help reduce the complexity inherent in clinical trials.
Data Management Plan Template for Clinical Trials
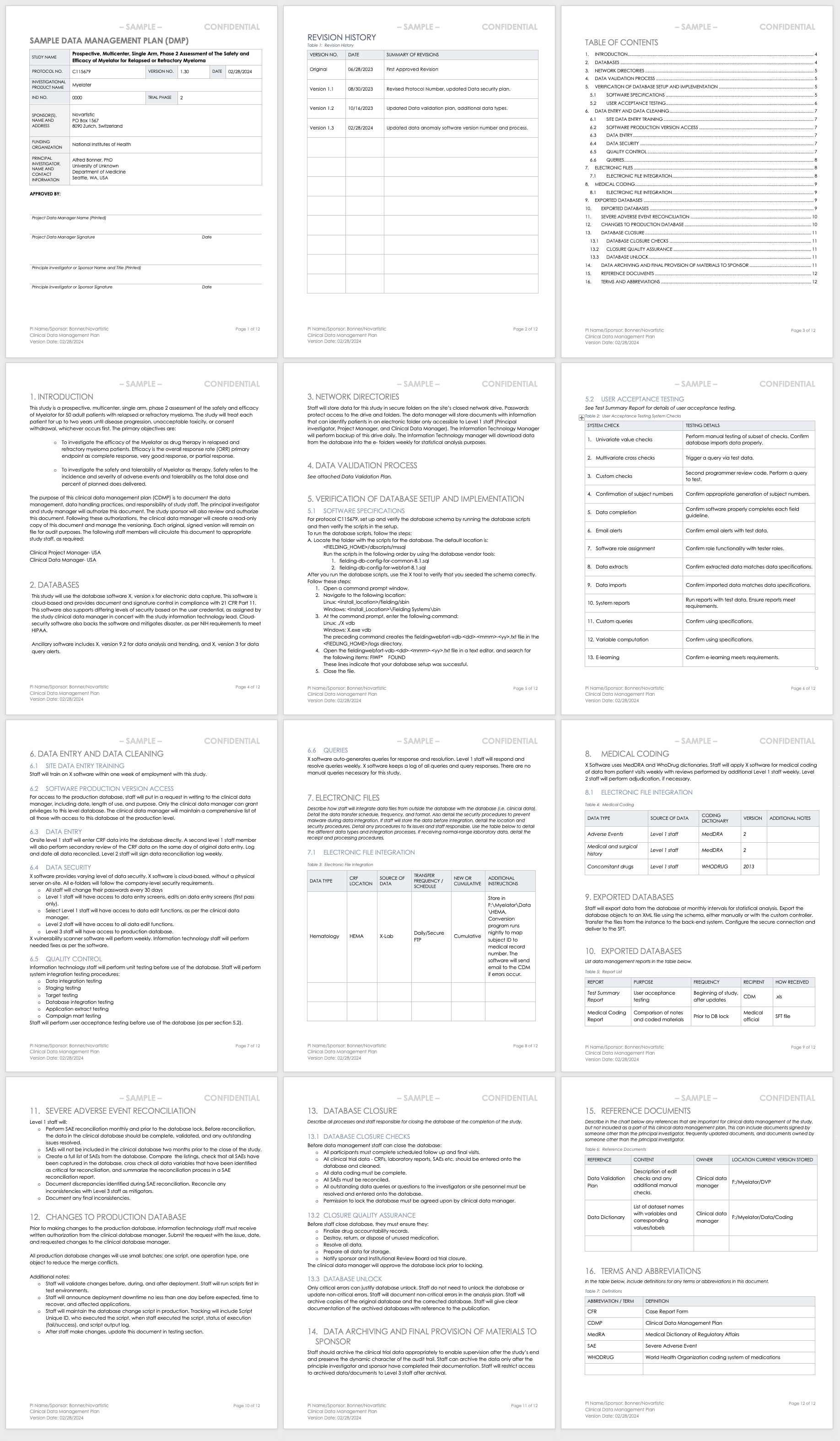
This data management plan template provides the required contents of a standard clinical trial data management plan, with space and instructions to input elements such as the data validation process, the verification of database setup and implementation processes, and the data archival process.
Download Data Management Plan Template - Word
Sample Data Management Plan for Clinical Trials
This sample data management plan shows a fictitious prospective, multicenter, single-arm study and its data management process needs. In two years of study, the data manager should regularly update this plan to demonstrate the study’s evolving needs, and document each change and update. Examples of sections include the databases used, how data will be entered and cleaned, and how staff will integrate different data sets collected in the study.
Download Sample Data Management Plan - Word
Clinical Trial Data Validation Plan
Data validation involves resolving database queries and inconsistencies by checking the data for accuracy, quality, and completeness. A data validation plan in clinical trials has all the variable calculations and checks that data managers use to identify any discrepancies in the dataset.
When the data is final, the database administrator locks it to ensure no further changes are made, as they could interrupt the integrity of the data. During reporting and analysis, experts may copy the data and reformat it into tables, lists, and graphs. Once the analysts complete their work, they report the results. When they have significant findings, they may create additional tables, lists, and graphs to present as part of the results. They then integrate these results into higher-level findings documentation. Examples of this type of documentation include investigator’s brochures or clinical case study reports (CSRs). Finally, the data manager archives the database.
The above steps are important because they preserve the integrity of the data in the database. However, managers do not need to perform them in a strict order. Some studies may need more frequent data validation, due to the high volume of data they produce, while other studies may produce intermediate analysis and reporting as part of their predetermined requirements. Finally, due to the complexity of some studies, the data manager or analyst may need to query , which means running a data request in a database and determining cursory results so that they may adjust the protocol.
Use this template to develop your own data validation plan. This Word template includes space and instructions for you to develop a data validation plan that you can include in your data management plan or use as a stand-alone document. Examples of sections include selecting and classifying the computer systems, validation protocol, and validation reporting.
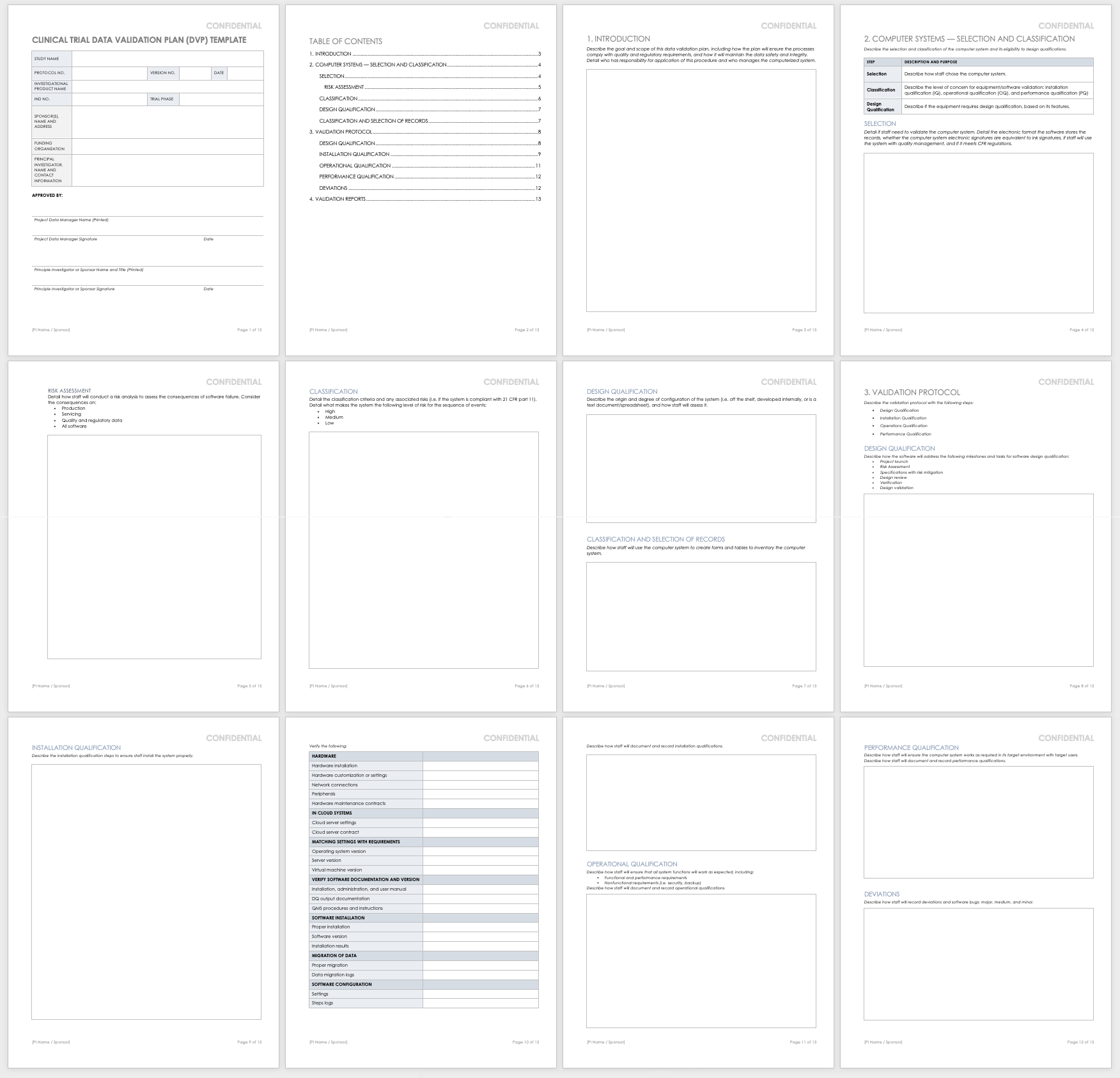
Download Data Validation Plan - Word
Data Management Workflow
A data management workflow is the process clinical research uses to deal with their data, from the data collection design to the electronic archival and findings presentation. This includes getting through the entry process, any batch validation, discrepancy management, coding, reconciliations, and quality control plans.
This workflow starts when researchers generate a CRF, whether manually or electronically, and continues through the final lock on the database. The data manager should perform quality checks and data cleaning throughout the workflow. The workflow steps for a data manager are as follows:
- CRF Design: This initial design step forms the basis of initial data collection.
- Database Design: The database should include space for all data collected in the study.
- Data Mapping: This step integrates data from different forms or formats so researchers can consistently report it.
- SAE Reconciliation: Data managers should regularly review and correct severe adverse events and potential events.
- Database Locking: Once a study is complete, the database manager should lock the database so that no one can change the data.
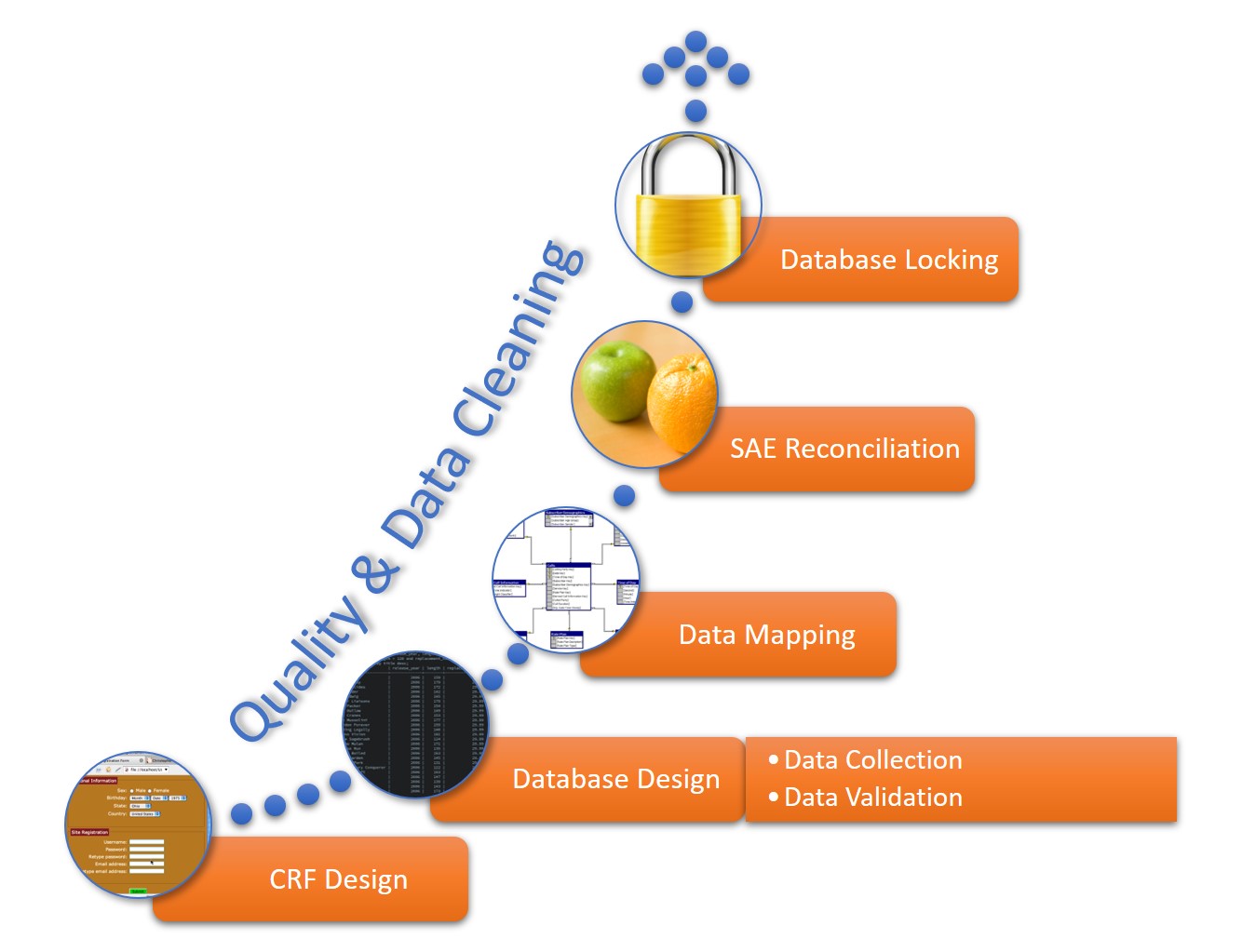
Clinical Trial Data Audits
A clinical trial data audit is a review of the information collected in order to ensure the quality, accuracy, and appropriateness for the stated research requirements, per the study protocol. Regulatory authorities, sponsors, and internal study staff can conduct two varieties of audit: overall and database-specific.
Regulators use database audits to ensure that no one has tampered with the data. In general, there must be an audit trail to know which user made changes to what and when in the database. For example, the auditors will look at record creation, modification, and deletion, noting the usernames, dates, and times. FDA 21 CFR Part 11 includes this as a part of fraud detection, and requires that there is a complete history of the recordkeeping system and clinical trial data transparency.
The data manager develops templates for auditing the study during the study development phase and performs their own internal audits as a part of its quality management.
This free clinical trial data management audit checklist template will help you develop your own checklist. This Excel template lets you show the status of your audit in an easy color-coded display, the category and tasks to review, and what criteria you require. It brings all your audit requirements and results together.
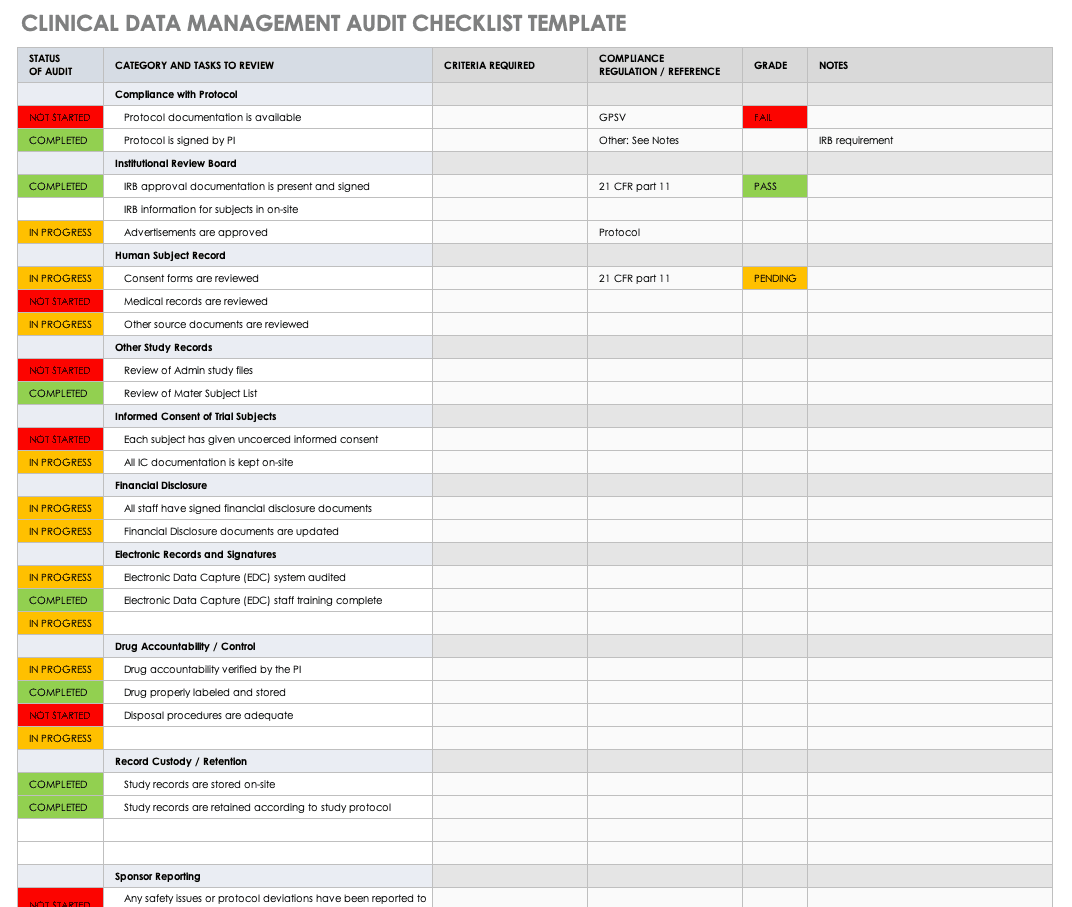
Download Clinical Data Management Audit Checklist - Excel
Quality Management in Clinical Trials
Data quality management (DQM) refers to the practices that ensure clinical information is of high value. In a clinical trial, DQM starts when staff first acquires the information and continues until the findings are distributed. DQM is critical in providing accurate outcomes.
The factors that influence the quality of clinical data include how well the study investigators develop and implement each of the following data pieces:
- Case Report Forms (CRF): Design the CRF in parallel with the protocol so that the data collected by staff is complete, accurate, and consistent.
- Data Conventions: Data conventions include dates, times, and acronyms. Data managers should set these conventions during study development, especially if there are multiple study locations and investigators.
- Guidelines for Monitoring: The overall data quality is contingent on the quality of the monitoring guidelines established.
- Missing Data: Missing data are those values not available that could change the analysis findings. During study development, investigators and analysts should determine how they will handle missing data.
- Verification of Source Data: Staff must verify that the source data is complete and accurate during data validation.
Regulations, Guidelines, and Standards in Clinical Data Management
Different regulations, guidelines, and standards govern clinical data management industry. The Clinical Data Interchange Standards Consortium (CDISC) is a global organization that holds clinical studies accountable to clinical trial data standards, international regulations, institutional and sponsor standard operating procedures (SOPs), and state laws.
There are standard operating procedures and best practices in clinical trial data management that are widespread. CDISC has two standards, the Study Data Tabulation Model Implementation Guide for Human Clinical Trials (SDTMIG), mandated by the U.S. Food and Drug Administration (FDA), and the Clinical Data Acquisition Standards Harmonization (CDASH). Also, in the industry, the Society for Clinical Data Management (SCDM) releases the Good Clinical Data Management Practices (GCDMP) guidelines and administers the International Association for Continuing Education and Training (IACET) credential for certified clinical data managers. The National Accreditations Board of Hospitals Health (NABH) provides additional guidance, such as pharmaceutical study auditing checklists. Finally, Good Clinical Practices (GCP) guidelines discuss ethical and quality standards in clinical research.
A trial conducted under the appropriate standards ensures that staff has followed the protocol and treated the patients according to that protocol. Ultimately, this shows the integrity and reproducibility of the study and acceptance in the industry.
Case Report Forms in Data Management
In data management, CRFs are the main tool researchers use to collect information from their participants. Researchers design CRFs based on the study protocol; in them, they document all patient information per the protocol for the duration of the study’s requirements.
CRFs should comply with all regulatory requirements and enable efficient analysis to decrease the need for data mapping during any data exchange. When longer than one page, the CRF is known as a CRF book, and each visit adds to the book. The main parts of a CRF are the header, the efficacy-related modules, and the safety-related modules:
- CRF Header: This portion includes the patient identification information and study information, such as the study number, site number, and subject identification number.
- Efficacy-Related Modules: This portion includes the baseline measurements, any diagnostics, the main efficacy endpoints of the trial, and any additional efficacy testing.
- Safety-Related Modules: The portion contains the patient’s demographic information, any adverse events, medical history, physical history, medications, confirmation of eligibility, and any reasons for release from the study.
What Is the Role of a Clinical Data Manager?
In a clinical trial, the data manager is the person who ensures that the research staff collects, manages, and prepares the resulting information accurately, comprehensively, and securely. This is a key role in clinical research, as the person is involved in the study setup, conduct, closeout, and some analysis and reporting.
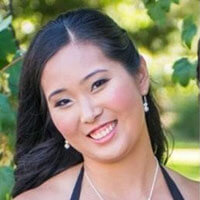
Melissa Peda , Clinical Data Manager at Fred Hutch Cancer Research Center , says, “Being a clinical data manager, you have to be very detail-oriented. We write up very specific instructions for staff. For example, the specifications to a program’s database include one document that could easily have 1,000 rows in Excel, and it needs to be perfect for queries to fire in real time. Code mistakes can put your project behind, so they must do their review with a close eye. You must also be logical and think through the project setup. A good clinical data manager must be detailed, so the programmers and other staff can do their thing.”
Krishnankutty, et al. , developed an overview of best practices for data management in clinical research. In their article, published in the Indian Journal of Pharmacology, they say that the need for strong clinical data management has sprung up from the pharmaceuticals industry wanting to fast-track drug development by having high-quality data, regardless of the type of data. Clinical data managers can expect to work with many different types of clinical data; the most common types include the following:
- Administrative data
- Case report forms (CRFs)
- Electronic health records
- Laboratory data
- Patient diaries
- Patient or disease registries
- Safety data
The clinical data managers often must oversee the analysis of the data as well. Data analysis conducted in clinical trial data management is very delicate: It requires a solid dataset and an analyst who can explain the findings. Regulatory agencies, along with other companies and professionals, check the findings and analysis, so they need to be accurate and understandable.
Education and Credentials of a Clinical Data Manager
Professionals in clinical data management receive data management in clinical trials training, and often have the Certified Clinical Data Manager (CCDM) credential. Their studies can have optimized outcomes since they are executed by a competent CDM team with validated skill sets and continued professional development.
To become certified, the applicant must have the appropriate education and experience, including the following:
- A bachelor’s degree and two or more years of full-time data management experience.
- An associate’s degree and three or more years of full-time data management experience.
- Four years of full-time data management experience.
- Part-time data management experience that adds up to the requirements above.
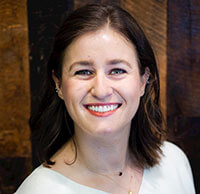
Raleigh Edelstein , a clinical data manager and EDC programmer, discusses the credentialing in this field. “Anyone can excel in this profession,” she says. “A CRA — a clinical research associate, also called a clinical monitor or a trial monitor — may need this credential more, as their profession is more competitive, and their experience is more necessary in trials. But if the credential makes you more confident, then I say go for it. Your experience and confidence matter.”
There are several degrees with an emphasis on clinical research that can also teach the necessary technical skills. In addition to many online options, these include the following, or a combination of the following:
- Associate of Science in biology, mathematics, or pharmacy.
- Bachelor of Science in one of the sciences.
- Post-Master's certificate in clinical data management, or a certificate related to medical device and drug development.
- Master of Science in clinical research, biotechnology, bioinformatics.
- Doctor of Nursing Practice.
- Doctor of Philosophy in any clinical research area.
These degree programs include concepts that help data managers understand what clinical studies need. They especially focus on survey design and data collection, but also include the following:
- Biostatistics
- Clinical research management and safety surveillance
- Compliance, ethics, and law
- New product business and strategic planning
- New product research and development
These degree programs offer coursework that improves the relevant clinical research skills. Many of the courses are introductory to clinical research, trials, and pharmacology, and others include the following:
- Business processes
- Clinical outsourcing
- Clinical research biostatistics
- Clinical trial design
- Compliance and monitoring
- Data collection strategies
- Data management
- Electronic data capture
- Epidemiology
- Ethics in research
- Federal regulatory issues
- Health policy and economics
- Human research protection
- Medical devices and product regulation
- Patient recruitment and informed consent
- Pharmaceutical law
- Review boards
- Worldwide regulations for submission
Clinical data managers can get involved with several professional organizations worldwide, including the following:
- The Association for Clinical Data Management (ACDM): This global professional organization supports the industry by providing additional resources and promoting best practices.
- The Association Française de Data Management Biomédicale (DMB): This French data management organization shares information and practices among professionals.
- International Network of Clinical Data Management Associations (INCDMA): Based in the United Kingdom, this professional network exchanges information and discusses relevant professional issues.
- The Society for Clinical Data Management (SCDM): This global organization awards CCDM credential to professionals, provides additional education, and facilitates conferences in clinical data management.
FAQs about Clinical Trial Managers
The field of clinical management is quickly expanding in many forms to support the need for new research. Below are some frequently asked questions.
How do I become a clinical trial manager?
To become a clinical trial manager, you must obtain the appropriate education, experience, and credentialing, as detailed above.
What is better: a Master’s in Health Administration or a Master’s in Health Sciences?
To work as a clinical data manager, either degree program is appropriate. Your choice depends on your interest.
What can you do with a degree in biotechnology or bioenterprise?
Biotechnology is involved in the technology that aids in biological research, and bioenterprise takes the products of biotechnology and markets and sells them.
What is a clinical application analyst?
A clinical application analyst is a professional who helps clinics evaluate software systems and vendors.
What is a clinical data analyst?
A clinical data analyst is a professional who analyzes data from clinical trials, and develops and maintains databases.
Contract Research Organizations for Data Management Services
Contract research organizations (CROs) are companies that provide outsourced research services to industries such as pharmaceutical, biotechnology, and research development. Designed to keep costs low, studies can hire them to perform everything from overall project management and data management to technical jobs.
Studies can hire CROs that specialize as clinical trial data management companies so they don’t have to worry about having all the necessary skills in-house. According to Melissa Peda, “A consultant may have the expertise that someone already working in the organization may not have, so they make sense to bring in.” Further, a contractor outside of the business can bring a lack of bias to the project.
According to Raleigh Edelstein, “A third-party person in charge of data management may be necessary because you don’t have to worry about the lack of company loyalty that the data may need.”
CROs can offer skills such as the following:
- Annotation and review
- Coding and validation
- Database export, transfer, and locking
- Database integration
- Database setup and validation
- Double data entry and third-party review of discrepancies
- Form design
- Planning, such as project management and data management plans
- Quality assessments and auditing
- Software implementation and training
- SAE reconciliation
Related Topics in Clinical Data Management
The following are related topics to clinical data management:
- Application Analyst: This position deals with the software side of clinical trials. Examples of their work include choosing software, designing databases, and writing queries.
- Clinical Data Analyst: A professional who examines and verifies that clinical study data is appropriate and means what it is supposed to mean.
- Clinical Research Academic Programs: Entry-level professional positions in clinical trials often require a minimum of a bachelor’s degree.
- Clinical Research Associate: This clinical trial staff member designs and performs clinical studies.
- Laboratory Informatics: The field of data and computational systems specialized for laboratory work.
- Laboratory Information Management System (LIMS): LIMS enables collection and analysis of data from laboratory work. LIMS is specialized to work in different environments, such as manufacturing and pharmaceuticals.
- Scientific Management: This management theory studies workflows, applying science to process engineering and management.
Improve Clinical Trial Data Management with Smartsheet for Healthcare
Empower your people to go above and beyond with a flexible platform designed to match the needs of your team — and adapt as those needs change.
The Smartsheet platform makes it easy to plan, capture, manage, and report on work from anywhere, helping your team be more effective and get more done. Report on key metrics and get real-time visibility into work as it happens with roll-up reports, dashboards, and automated workflows built to keep your team connected and informed.
When teams have clarity into the work getting done, there’s no telling how much more they can accomplish in the same amount of time. Try Smartsheet for free, today.
Any articles, templates, or information provided by Smartsheet on the website are for reference only. While we strive to keep the information up to date and correct, we make no representations or warranties of any kind, express or implied, about the completeness, accuracy, reliability, suitability, or availability with respect to the website or the information, articles, templates, or related graphics contained on the website. Any reliance you place on such information is therefore strictly at your own risk.
These templates are provided as samples only. These templates are in no way meant as legal or compliance advice. Users of these templates must determine what information is necessary and needed to accomplish their objectives.
Discover why over 90% of Fortune 100 companies trust Smartsheet to get work done.
Best Practices for Research Data Management
- First Online: 15 June 2023
Cite this chapter
- Anita Walden 4 ,
- Maryam Garza 5 &
- Luke Rasmussen 6
Part of the book series: Health Informatics ((HI))
620 Accesses
Data is one of the most valuable assets to answer vital questions, so careful planning is needed to ensure quality while maximizing resources and controlling costs. Mature research and clinical trial organizations create and use data management plans to guide their processes from data generation to archiving. Today, clinical research activities are more complex due to a myriad of data sources, the use of new technologies, linkages between health care, patient reported, and research data environments, and the availability of digital tools. It is important to invest in training and hiring skilled data managers and informaticists to manage this rapidly changing landscape and to integrate variable and large-scale data. For everyone in the research enterprise, being aware of and implementing end-to-end data management best practices early in the research design phase can have a positive impact on data analysis.
This is a preview of subscription content, log in via an institution to check access.
Access this chapter
- Available as PDF
- Read on any device
- Instant download
- Own it forever
- Available as EPUB and PDF
- Durable hardcover edition
- Dispatched in 3 to 5 business days
- Free shipping worldwide - see info
Tax calculation will be finalised at checkout
Purchases are for personal use only
Institutional subscriptions
Krishnankutty B, Bellary S, Kumar NB, Moodahadu LS. Data management in clinical research: an overview. Indian J Pharmacol. 2012;44(2):168.
Article PubMed PubMed Central Google Scholar
Society for Clinical Data Management. Good clinical data management practices(GCDMP) [Internet]. 2013 Oct [cited 2022 Jun 01]. https://scdm.org/wp-content/uploads/2019/05/Full-GCDMP-Oct-2013.pdf
Ulrich H, Kock-Schoppenhauer AK, Deppenwiese N, Gött R, Kern J, Lablans M, Majeed RW, Stöhr MR, Stausberg J, Varghese J, Dugas M. Understanding the nature of metadata: systematic review. J Med Internet Res. 2022;24(1):e25440.
Go Fair.(Meta)data are associated with detailed provenance [Internet]. [cited 2022 Jun 01]. https://www.go-fair.org/fair-principles/r1-2-metadata-associated-detailed-provenance/
U.S. Department of Health & Human Services (HHS). Human Research Protection Training [Internet]. [cited 2022 Jun 01]. https://www.hhs.gov/ohrp/education-and-outreach/online-education/human-research-protection-training/index.html
National Institutes of Health (NIH). Good Clinical Practice Training [Internet]. [cited 2022 Jun 01]. https://grants.nih.gov/policy/clinical-trials/good-clinical-training.htm
Zozus MN, Sanns W, Eisenstein E, Sanns B. Beyond EDC. J Soc Clin Data Manag. 2021;1(1):1–22.
Google Scholar
Park YR, Yoon YJ, Koo H, Yoo S, Choi CM, Beck SH, Kim TW. Utilization of a clinical trial management system for the whole clinical trial process as an integrated database: system development. J Med Internet Res. 2018;20(4):e9312.
Article Google Scholar
Michael W. Electronic Clinical Trial Management Systems: The Basics, Needs, and Outputs. SoCRA [Internet]. 2021 Jan 04 [cited 2022 Jun 01]. https://www.socra.org/blog/electronic-clinical-trial-management-systems-the-basics-needs-and-outputs/ .
United States Food and Drug Administration; Guidance for Industry Part 11, Electronic Records; Electronic Signatures. https://www.fda.gov/regulatory-information/search-fda-guidance-documents/part-11-electronic-records-electronic-signatures-scope-and-application
U.S. Department of Health & Human Services (HHS). Revised Common Rule Q&As [Internet]. [cited 2022 Jun 01]. https://www.hhs.gov/ohrp/education-and-outreach/revised-common-rule/revised-common-rule-q-and-a/index.html .
U.S. Food & Drug Administration (FDA). Use of Electronic Informed Consent in Clinical Investigations – Questions and Answers [Internet]. [cited 2022 Jun 01]. https://www.fda.gov/regulatory-information/search-fda-guidance-documents/use-electronic-informed-consent-clinical-investigations-questions-and-answers .
TransCelerate BioPharma. What is eConsent? [Internet]. [cited 2022 Jun 01]. https://www.transceleratebiopharmainc.com/assets/econsent-solutions/what-is-econsent/#implementation-guidance .
Research Electronic Data Capture (REDCap). https://www.project-redcap.org
National Institutes of Health (NIH). Use Common Data Elements for More FAIR Research Data [Internet]. Waltham, MA: USA. [cited 27 May 2022]. https://cde.nlm.nih.gov/home .
National Institutes of Health (NIH). BRIDG, ISO and DICOM [Internet]. [cited 27 May 2022]. https://bridgmodel.nci.nih.gov/bridg-iso-dicom .
Von Rosing M, Von Scheel H, Scheer AW. The complete business process handbook: body of knowledge from process modeling to BPM, vol. 1. Morgan Kaufmann; 2014.
Gane C. Structured systems analysis: tools and techniques. Prentice-Hall, NJ: Englewood Cliffs; 1978.
UNICOM Systems. Ward and Mellor data flow diagrams [Internet]. [cited 27 May 2022]. https://support.unicomsi.com/manuals/systemarchitect/11482/starthelp.html#page/Architecting_and_designing%2FStructuredAnalysisDesign.18.010.html%23
Visual Paradigm. DFD Using Yourdon and DeMarco Notation [Internet]. [cited 2022 Jun 01]. https://online.visual-paradigm.com/knowledge/software-design/dfd-using-yourdon-and-demarco
Lucidchart. What is a data flow diagram? [Internet]. [cited 2022 Jun 01]. https://www.lucidchart.com/pages/data-flow-diagram#section_0
Lucidchart. What is a data flow diagram? [Internet]. [cited 2022 Jun 01]. https://www.lucidchart.com/blog/data-flow-diagram-tutorial#:~:text=Data%20flow%20diagrams%20visually%20represent,a%20new%20system%20for%20implementation
Bellary S, Krishnankutty B, Latha MS. Basics of case report form designing in clinical research. Perspect Clin Res. 2014;5(4):159.
Guideline IH. Guideline for good clinical practice E6 (R1). ICH Harmon Tripart Guidel. 1996;1996(4)
Califf RM, Karnash SL, Woodlief LH. Developing systems for cost-effective auditing of clinical trials. Control Clin Trials. 1997;18(6):651–60.
Article CAS PubMed Google Scholar
Coons SJ, Eremenco S, Lundy JJ, O’Donohoe P, O’Gorman H, Malizia W. Capturing patient-reported outcome (PRO) data electronically: the past, present, and promise of ePRO measurement in clinical trials. Patient. 2015;8(4):301–9.
Article PubMed Google Scholar
Coons SJ, Gwaltney CJ, Hays RD, Lundy JJ, Sloan JA, Revicki DA, Lenderking WR, Cella D, Basch E. Recommendations on evidence needed to support measurement equivalence between electronic and paper-based patient-reported outcome (PRO) measures: ISPOR ePRO good research practices task force report. Value Health. 2009;12(4):419–29.
Denny JC. Chapter 13: mining electronic health records in the genomics era. PLoS Comput Biol. 2012;8(12):e1002823.
Article CAS PubMed PubMed Central Google Scholar
Robinson JR, Wei WQ, Roden DM, Denny JC. Defining phenotypes from clinical data to drive genomic research. Annu Rev Biomed Data Sci. 2018;1:69–92.
Westra BL, Christie B, Johnson SG, Pruinelli L, LaFlamme A, Sherman SG, Park JI, Delaney CW, Gao G, Speedie S. Modeling flowsheet data to support secondary use. Comput Inform Nurs. 2017;35(9):452.
Newton KM, Peissig PL, Kho AN, Bielinski SJ, Berg RL, Choudhary V, Basford M, Chute CG, Kullo IJ, Li R, Pacheco JA. Validation of electronic medical record-based phenotyping algorithms: results and lessons learned from the eMERGE network. J Am Med Inform Assoc. 2013;20(e1):e147–54.
Zozus MN, Young LW, Simon AE, Garza M, Lawrence L, Tounpraseuth S, Bledsoe M, Newman-Norlund S, Jarvis JD, McNally M, Harris KR. Training as an intervention to decrease medical record abstraction errors multicenter studies. Stud Health Technol Inform. 2019;257:526.
PubMed PubMed Central Google Scholar
National Institutes of Health Pragmatic Trials Collaboratory. Electronic Health Records Based Phenotyping [Internet]. [cited 2022 Jun 01]. https://rethinkingclinicaltrials.org/chapters/conduct/electronic-health-records-based-phenotyping/electronic-health-records-based-phenotyping-introduction/
Wei WQ, Denny JC. Extracting research-quality phenotypes from electronic health records to support precision medicine. Genome Med. 2015;7(1):1–4.
Article CAS Google Scholar
Peissig PL, Rasmussen LV, Berg RL, Linneman JG, McCarty CA, Waudby C, Chen L, Denny JC, Wilke RA, Pathak J, Carrell D. Importance of multi-modal approaches to effectively identify cataract cases from electronic health records. J Am Med Inform Assoc. 2012;19(2):225–34.
Richesson RL, Smerek MM, Cameron CB. A framework to support the sharing and reuse of computable phenotype definitions across health care delivery and clinical research applications. eGEMs. 2016;4(3):1232.
Levinson RT, Malinowski JR, Bielinski SJ, Rasmussen LV, Wells QS, Roger VL, Wiley LK Identifying heart failure from electronic health records: a systematic evidence review medRxiv. 2021.
Kirby JC, Speltz P, Rasmussen LV, Basford M, Gottesman O, Peissig PL, Pacheco JA, Tromp G, Pathak J, Carrell DS, Ellis SB. PheKB: a catalog and workflow for creating electronic phenotype algorithms for transportability. J Am Med Inform Assoc. 2016;23(6):1046–52.
HDR UK Caliber Phenotype Library [Internet]. HDR UK Phenotype Library. [cited 2022Aug3]. https://portal.caliberresearch.org/
Rasmussen LV, Brandt PS, Jiang G, Kiefer RC, Pacheco JA, Adekkanattu P, Ancker JS, Wang F, Xu Z, Pathak J, Luo Y. Considerations for improving the portability of electronic health record-based phenotype algorithms. In AMIA Annual Symposium Proceedings 2019 (Vol. 2019, p. 755). American Medical Informatics Association.
Mo H, Thompson WK, Rasmussen LV, Pacheco JA, Jiang G, Kiefer R, et al. Desiderata for computable representations of electronic health records-driven phenotype algorithms. J Am Med Inform Assoc. 2015;22(6):1220–30.
Richesson R, Wiley LK, Gold S, Rasmussen L. Electronic Health REcords-Based Phenotyping: Introduction. NIH Health Care Systems Research Collaboratory Electronic Health Records Core Working Group. Electronic health records based phenotyping in next-generation clinical trials: a perspective from the NIH Health Care Systems Collaboratory. J Am Med Inform Assoc. 2021;20:e226–31. https://doi.org/10.1136/amiajnl-2013-001926 .
Richesson RL, Rusincovitch SA, Wixted D, Batch BC, Feinglos MN, Miranda ML, Hammond W, Califf RM, Spratt SE. A comparison of phenotype definitions for diabetes mellitus. J Am Med Inform Assoc. 2013;20(e2):e319–26.
Haendel MA, Chute CG, Bennett TD, Eichmann DA, Guinney J, Kibbe WA, Payne PR, Pfaff ER, Robinson PN, Saltz JH, Spratt H. The national COVID cohort collaborative (N3C): rationale, design, infrastructure, and deployment. J Am Med Inform Assoc. 2021;28(3):427–43.
U.S. Department of Health & Human Services (HHS). Summary of the HIPAA Privacy Rule [Internet]. [cited 2022 Jun 01]. https://www.hhs.gov/hipaa/for-professionals/privacy/laws-regulations/index.html .
Hemming K, Kearney A, Gamble C, Li T, Jüni P, Chan AW, Sydes MR. Prospective reporting of statistical analysis plans for randomised controlled trials. Trials. 2020;21(1):1–2.
Yuan I, Topjian AA, Kurth CD, Kirschen MP, Ward CG, Zhang B, Mensinger JL. Guide to the statistical analysis plan. Pediatr Anesth. 2019;29(3):237–42.
Services H. National Institutes of Health. Clinical trials registration and results information submission. Final rule. Fed Regist. 2016;81(183):64981–5157.
Guideline IH. Statistical Principles for Clinical Trials. Geneva In International Conference on Harmonisation of Technical Requirements for Registration of Pharmaceuticals for Human Use 1998.
Chan AW, Tetzlaff JM, Gøtzsche PC, Altman DG, Mann H, Berlin JA, Dickersin K, Hróbjartsson A, Schulz KF, Parulekar WR, Krleža-Jerić K. SPIRIT 2013 explanation and elaboration: guidance for protocols of clinical trials. BMJ. 2013:346.
Gamble C, Krishan A, Stocken D, Lewis S, Juszczak E, Doré C, Williamson PR, Altman DG, Montgomery A, Lim P, Berlin J. Guidelines for the content of statistical analysis plans in clinical trials. JAMA. 2017;318(23):2337–43.
Weiskopf NG, Weng C. Methods and dimensions of electronic health record data quality assessment: enabling reuse for clinical research. J Am Med Inform Assoc. 2013;20(1):144–51.
European Medicines Agency. ICH Topic E 6 (R1). Guideline for Good Clinical Practice. Note for Guidance on Good Clinical Practice.
European Medicines Agency. ICH Topic E 6 (R2). Guideline for Good Clinical Practice.
Hines S. Targeting source document verification. Appl Clin Trials. 2011;20(2):38.
Dykes B. Reporting vs. analysis: What’s the difference. Digital Marketing Blog. 2010.
Walden A, Nahm M, Barnett ME, Conde JG, Dent A, Fadiel A, Perry T, Tolk C, Tcheng JE, Eisenstein EL. Economic analysis of centralized vs. decentralized electronic data capture in multi-center clinical studies. Stud Health Technol Inform. 2011;164:82.
Download references
Author information
Authors and affiliations.
University of Colorado Denver—Anschutz Medical Campus, Denver, CO, USA
Anita Walden
University of Arkansas for Medical Sciences, Little Rock, AR, USA
Maryam Garza
Northwestern University Feinberg School of Medicine, Chicago, IL, USA
Luke Rasmussen
You can also search for this author in PubMed Google Scholar
Corresponding author
Correspondence to Anita Walden .
Editor information
Editors and affiliations.
Learning Health Sciences, University of Michigan School of Medicin, Ann Arbor, MI, USA
Rachel L. Richesson
School of Information, University of South Florida, Tampa, FL, USA
James E. Andrews
Medical Informatics and Clinical Epidemiology, Oregon Health and Science University, Portland, OR, USA
Kate Fultz Hollis
Rights and permissions
Reprints and permissions
Copyright information
© 2023 The Author(s), under exclusive license to Springer Nature Switzerland AG
About this chapter
Walden, A., Garza, M., Rasmussen, L. (2023). Best Practices for Research Data Management. In: Richesson, R.L., Andrews, J.E., Fultz Hollis, K. (eds) Clinical Research Informatics. Health Informatics. Springer, Cham. https://doi.org/10.1007/978-3-031-27173-1_14
Download citation
DOI : https://doi.org/10.1007/978-3-031-27173-1_14
Published : 15 June 2023
Publisher Name : Springer, Cham
Print ISBN : 978-3-031-27172-4
Online ISBN : 978-3-031-27173-1
eBook Packages : Medicine Medicine (R0)
Share this chapter
Anyone you share the following link with will be able to read this content:
Sorry, a shareable link is not currently available for this article.
Provided by the Springer Nature SharedIt content-sharing initiative
- Publish with us
Policies and ethics
- Find a journal
- Track your research

An official website of the United States government
Here’s how you know

Official websites use .gov A .gov website belongs to an official government organization in the United States.

Secure .gov websites use HTTPS A lock ( Lock Locked padlock icon ) or https:// means you’ve safely connected to the .gov website. Share sensitive information only on official, secure websites.

Investigators should consider using this template when developing the Data and Safety Monitoring Plan (DSMP) for clinical studies funded by the National Institute of Arthritis and Musculoskeletal and Skin Diseases (NIAMS).
The goal of the DSMP is to provide a general description of a plan that you intend to implement for data and safety monitoring. The DSMP should specify the following:
- Primary and secondary outcome measures/endpoints
- Sample size and target population
- Inclusion and exclusion criteria
- A list of proposed participating sites and centers for multi-site trials
- Potential risks and benefits for participating in the study
- Procedures for data review and reportable events
- Project Director (PD)/Principal Investigator (PI) (required)
- Institutional Review Board (IRB) (required)
- Designated medical monitor
- Internal Committee or Board
- Independent, NIAMS-appointed Monitoring Body (MB) which can include a Data and Safety Monitoring Board (DSMB), an Observational Study Monitoring Board (OSMB), a Safety Officer (SO) or Dual SOs
- Content and format of the safety report
- Data Management, Quality Control and Quality Assurance
Note that all sample text should be replaced with the study specific text. There is no need to include sections that are not relevant to the particular study. Please do not use the sample text verbatim .
TABLE OF CONTENTS
1.0 Study Overview
- 1.1 Study Description
- 1.2 Study Management
2.0 Participant Safety
- 2.1.1 Potential Risks
- 2.1.2 Potential Benefits
- 2.2.1 Informed Consent Process
3.0 Reportable Events
- 3.1.1 Adverse Events (AEs)
- 3.1.2 Serious Adverse Events (SAEs)
- 3.1.3 Unanticipated Problems (UPs)
- 3.1.4 Protocol Deviations
- 3.2 Collection and Assessment of AEs, SAEs, UPs, and Protocol Deviations
- 3.3.1 AE Reporting Procedures
- 3.3.2 SAE Reporting Procedures
- 3.3.3 UP Reporting Procedures
- 3.3.4 Protocol Deviation Reporting Procedures
- 3.3.5 Serious or Continuing Noncompliance
- 3.3.6 Suspension or Termination of IRB Approval
4.0 Interim Analysis & Stopping Rules
5.0 Data and Safety Monitoring
- 5.1 Frequency of Data and Safety Monitoring
- 5.2 Content of Data and Safety Monitoring Report
- 5.3 Monitoring Body Membership and Affiliation
- 5.4 Conflict of Interest for Monitoring Bodies
- 5.5 Protection of Confidentiality
- 5.6 Monitoring Body Responsibilities
6.0 Data Management, Quality Control, and Quality Assurance
1.0 Study Overview
1.1 study description.
This section outlines the overall goal of this project. It also describes the study design, primary and secondary outcome measures/endpoints, sample size/power calculation and target population, inclusion and exclusion criteria.
1.2 Study Management
This section includes the proposed participating sites and their responsibilities. In addition, this section should include the planned enrollment timetable (i.e. projected enrollment).
2.0 Participant Safety
2.1 potential risks and benefits for participants.
This section outlines the potential risks and benefits of the research for the study participants and for society. It should include a description of all expected adverse events (AEs), the known side effects of the intervention, and all known risks or complications of the outcomes being assessed.
2.1.1 Potential Risks
Outline potential risks for study participants including a breach of confidentiality.
{Begin sample text}
{End sample text}
2.1.2 Potential Benefits
Outline potential benefits for study participants or if there are no direct benefits to the participants.
2.2 Protection Against Study Risks
This section provides information on how risks to participants will be managed. It should specify any events that would preclude a participant from continuing in the study. In general, the format and content of this section are similar to the Human Participants section of the grant application.
In addition, this section describes measures to protect participants against study specific risks including the data security to protect the confidentiality of the data.
2.2.1 Informed Consent Process
This section explains the informed consent process. It should include, but not be limited to, who will be consenting the participant, how and under what conditions will a participant be consented, and that participation is voluntary. The informed consent process should meet the revised Common Rule requirements for consenting. For further details on this requirement, please visit: https://www.ecfr.gov/cgi-bin/text-idx?SID=921afb2e7909a2cf08c5f3ce160a0c96&mc=true&node=se45.1.46_1116 .
3.0 Data and Safety Monitoring
3.1 definitions.
This section should describe how to identify AEs, SAEs and UPs. In the case where the intervention is a Food and Drug Administration (FDA) regulated drug, device or biologic, it should include the FDA definition, grading scale and “study relatedness” criteria of AEs.
3.1.1 Adverse Events (AEs)
The definition of adverse event here is drawn from the OHRP guidance ( https://www.hhs.gov/ohrp/regulations-and-policy/guidance/reviewing-unanticipated-problems/index.html ); for some studies, the ICH E6 definition may be more appropriate. Expected and unexpected AEs should be listed in this section.
3.1.2 Serious Adverse Events (SAEs)
SAEs are a subset of all AEs.
3.1.3 Unanticipated Problems (UPs)
The OHRP definition of UPs can be accessed using the link provided in Section 3.1.1 above.
{End sample text}
3.1.4 Protocol Deviations
This section should include the study definition of protocol deviations and define the events placing the participant at increased risk of harm or compromising the integrity of the safety data.
3.2 Collection and Assessment of AEs, SAEs, UPs, and Protocol Deviations
The section should include who is responsible for collecting these events, how the information will be captured, where the information will be collected from (e.g., medical records, self-reported), and what study form(s) will be used to collect the information (e.g., case report forms, direct data entry). This section should also include what type of information will be collected (e.g., event description, time of onset, assessment of seriousness, relationship to the study intervention, severity, etc.). Note that it is the NIAMS requirement to collect all AEs regardless of the expectedness or relatedness.
This section should also describe who is responsible for assessing these events. The individual(s) responsible should have the relevant clinical expertise to make such an assessment (e.g., physician, nurse practitioner, physician assistant, nurse). When assessing AEs and SAEs, the following information should be included:
- Possibly/Probably (may be related to the intervention)
- Definitely (clearly related to the intervention)
- Not Related (clearly not related to the intervention)
3.3 Reporting of AEs, SAEs, UPs, Protocol Deviations, Serious or Continuing Noncompliance, and Suspension or Termination of IRB Approval
This section should describe who is responsible for reporting these events and the roles and responsibilities of each person on the clinical study team who is involved in the safety reporting to the IRB, FDA (if applicable), Monitoring Body, and NIAMS (through the NIAMS Executive Secretary). It should also include the Office for Human Research Protections (OHRP) and FDA reporting requirements. See NIAMS Reportable Events Requirements and Guidelines for more details.
3.3.1 AE Reporting Procedures
All non-serious AEs (regardless of expectedness or relatedness) are reported to the Monitoring Body and NIAMS (through the NIAMS Executive Secretary) semi-annually or as determined by the NIAMS.
3.3.2 SAE Reporting Procedures
All SAEs (regardless of expectedness or relatedness ) must be reported in an expedited manner to the NIAMS and the Monitoring Body. There may be different timeline for reporting SAE to the IRBs, FDA (if applicable), Monitoring Body and the NIAMS. The timeline for reporting SAEs to the Monitoring Body and NIAMS (through the NIAMS Executive Secretary) is within 48 hours of the investigator becoming aware of the event so that a real time assessment can be conducted, and the outcome shared in a timely manner.
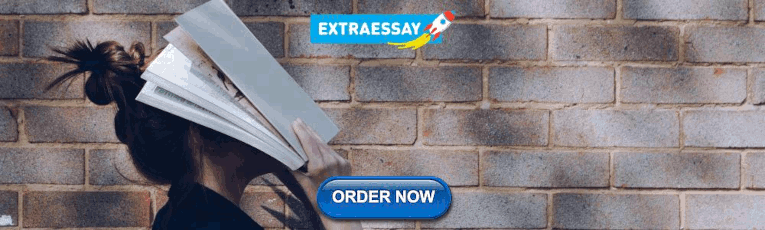
3.3.3 UP Reporting Procedures
All events that meet the criteria of a UP must be reported in an expedited manner to the NIAMS and the Monitoring Body. There may be different timeline for reporting UPs to the IRBs, OHRP/FDA (if applicable), Monitoring Body, and the NIAMS. The timeline for reporting UPs to the Monitoring Body and NIAMS (through the NIAMS Executive Secretary) is within 48 hours of the investigator becoming aware of the event so that a real time assessment can be conducted, and the outcome shared in a timely manner.
3.3.4 Protocol Deviation Reporting Procedures
Protocol deviations impacting participant safety are subject to expedited reporting to the Monitoring Body and NIAMS (through the NIAMS Executive Secretary) within 48 hours of the investigator becoming aware of the even t so that a real time assessment can be conducted, and the outcome shared in a timely manner. All other events should be reported at the time of the routine DSMB meeting or submission of the safety report.
3.3.5 Serious or Continuing Noncompliance
This section should include the process in place at your institution to capture and report serious or continuing noncompliance. It should include who is responsible for reporting. Serious or continuing noncompliance must be reported to the NIAMS Program Officer and Grants Management Specialist within 3 business days of IRB determination. A copy of the IRB submission and determination must be submitted along with the report to the NIAMS. The guidance on reporting incidents to OHRP should also be followed to provide the timeline of reporting to this regulatory body.
3.3.6 Suspension or Termination of IRB Approval
This section should include the process for reporting study suspension or termination by the IRB. It should also include who is responsible for reporting to the NIAMS, OHRP, and the timeline for reporting of these events. Suspension or termination of IRB approval must include a statement of the reason(s) for the action and must be reported promptly to the NIAMS Program Officer and Grants Management Specialist within 3 business days of receipt by the PI.
4.0 Interim Analysis & Stopping Rules
This section provides information on planned interim analysis. Interim analysis may be conducted either due to pre-specified stopping rules as outlined in the protocol and at predetermined intervals, or as determined necessary by the Monitoring Body to assess safety concerns or study futility based upon accumulating data. An interim analysis may be performed for safety, efficacy and/or futility, and the reports are prepared by the unmasked study statistician or data coordinating center responsible for generating such reports. Rules for stopping the study, based on interim analysis, should be described.
If no interim analysis is planned, this should be noted within this section.
5.0 Data and Safety Monitoring
This section identifies the name of the individual or entity responsible for data and safety monitoring, what information will be reviewed, and frequency of such reviews. A brief general introduction regarding data and safety monitoring oversight should be provided in section 5.0, and further details should be provided in the subsequent sections.
5.1 Frequency of Data and Safety Monitoring
This section describes the frequency of data and safety monitoring reviews. As the reviews of reportable events (AEs, SAEs, UPs, and protocol deviations) are included in Section 3, this section should focus on the routine and ad hoc review of the full data and safety monitoring reports. {Begin sample text}
5.2 Content of Data and Safety Monitoring Report
This section describes the content of the data and safety monitoring reports. The specifics of the study and the requests of the Monitoring Body will guide requirements for additional tables and listings. Tables for multi-site studies will present aggregated data as well as data by site. For studies with more than one intervention group, this section should indicate the plans for providing data stratified by masked intervention group (i.e., Group A vs. Group B) as part of the closed report to the Monitoring Body, while the open report should have data presented in aggregate without stratification by groups. The complete data and safety monitoring report template should be included as an appendix. Refer to the NIAMS Data and Safety Monitoring Board Report Templates ( https://www.niams.nih.gov/grants-funding/conducting-clinical-research/trial-policies-guidelines-templates/data-safety-monitoring-guidelines-policies/clinical-study-templates-forms ) for guidance.
5.3 Monitoring Body Membership and Affiliation
This section includes a roster of the Monitoring Body’s name(s) and affiliation(s). For studies with a NIAMS-appointed Monitoring Body, the NIAMS Executive Secretary will provide the name(s) and affiliation(s) of the individual(s) serving once the Monitoring Body has been assembled. However, if this is an Internally-appointed Monitoring Body (i.e., PI-appointed), the study team should enter the information in this section once the NIAMS has confirmed that no conflicts of interest with the Monitoring Body member(s) are identified.
5.4 Conflict of Interest for Monitoring Bodies
This section describes the conflict of interest procedure for Monitoring Body members. For studies with a NIAMS-appointed Monitoring Body, the NIAMS Executive Secretary will conduct a conflict of interest check on each member prior to beginning their service and on an annual basis thereafter. For studies with an Internally-appointed Monitoring Body (i.e., PI-appointed), the study team should provide the name, affiliation, and curriculum vitae (if available) of the proposed Monitoring Body member(s) to the NIAMS Executive Secretary for a conflict of interest check to be conducted. Once the conflict of interest check is complete, this section should be updated to indicate that the NIAMS did not identify any conflicts of interest for the Monitoring Body member(s).
5.5 Protection of Confidentiality
This section describes how confidentiality of data presented to the Monitoring Body will be protected.
5.6 Monitoring Body Responsibilities
A charter provides a detailed list of the Monitoring Body’s responsibilities. Listed in the sample text below are the responsibilities for a NIAMS-appointed Monitoring Body. Please ensure that all of the items are applicable for this study. For studies with an Internally-appointed Monitoring Body, the study team should ensure that a detailed list of the Monitoring Body’s responsibilities are provided in this section.
6.0 Data Management, Quality Control, and Quality Assurance
This section describes how the site will collect, document, and review the data. Who will be responsible for data entry and ensure they are accurate and complete? Which database will be used? Does it have audit tracking capabilities? What is the data query process and frequencies? Are there any planned mitigation strategies in the event of non-compliance? What is the process for locking the final study datasets? Are there any procedures on data access and sharing as appropriate? Is there a description of security measures in place? (If you have a separate Clinical Monitoring and Data Management Plan, please reference it and utilize that information to help populate this section).
Each study should have standard operating procedures (SOPs) and/or a quality management plan that describe the following (if this is a multi-site study, each site should have SOPs and a plan):
- Staff training methods and how such training will be tracked
- How data will be evaluated for compliance with the protocol and for accuracy in relation to source documents
- The documents to be reviewed (e.g., case report forms, clinic notes, product accountability records, specimen tracking logs, questionnaires), who is responsible, and the frequency for reviews
- Who will be responsible for addressing quality assurance (QA) issues (correcting procedures that are not in compliance with protocol) and quality control issues (correcting data entry errors). It is anticipated that QA review and data verification will be performed by someone other than the individual originally collecting the data, or by double-data entry. The frequency of internal QA review and measures to be taken for corrective action (e.g., for trends in errors) should be included
- QA measures for participant recruitment, enrollment, enrollment targets, and for the validity and integrity of the data. E6 Good Clinical Practice (R1): 1.46 defines quality assurance as “All those planned and systematic actions that are established to ensure that the trial is performed and the data are generated, documented (recorded), and reported in compliance with Good Clinical Practice (GCP) and the applicable regulatory requirement(s)”
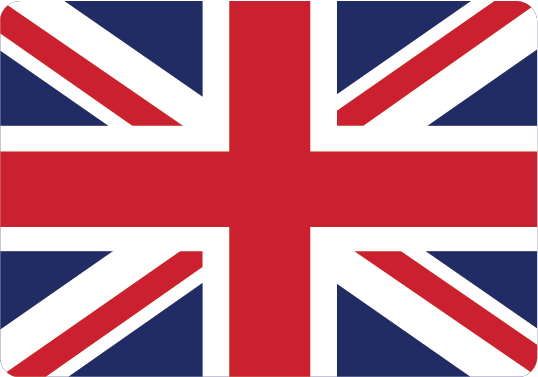
Latest News
How oct clinical completed patient recruitment…, completing recruitment in three countries…, resource library, why data management is essential for clinical trials.
CDM (Clinical Data Management) is essential to the success of clinical studies, including in multinational studies, where CDM is vital for the handling of vast amounts of data generated simultaneously from different sites across several countries. The process itself is considered the key to ensuring reliable, fast and flexible clinical trials. To accomplish this goal, all steps need to be fully planned with an integrated scheme, as CDM activities start from the beginning.
The success of clinical trials depends on the accurate gathering, generation, and verification of data. This is because pharmaceutical companies, device manufacturers, researchers, and other life science organizations rely on data management to improve the outcomes and speed of clinical trials.
Here is a brief explanation aimed at assessing why CDM is considered decisive for studies.
What is Clinical Data Management?
Clinical Data Management is intended to deliver quality data that support the purpose of a study and find answers to the research question and the conclusion to hypotheses.
CDM helps to obtain faster and better results with higher accuracy, as it allows the building of a valid, error-free and solid database.
Data management in clinical studies involves main two steps:
1. Data collection
2. Data cleaning
The main objective of these steps is to collect the maximum amount of information for statistical analysis and to ensure the data are of high integrity and quality by eliminating any errors and likelihood of missing data.
Our team at OCT is dedicated to delivering accurate and high-quality CDM solutions by selecting the most advanced and innovative approach to improve the efficiency of your trial.
Also we have an experienced team of biostatisticians collaborates, scientific experts and medical writers who will optimize data accuracy and integrity and enable you to deliver faster and more accurate results. Read more about our Clinical Data Management services here .
The Role of CTMS: Key Considerations for Selecting a Clinical Trial Ma...
The role of cras and the importance of effective research site managem..., world immunization week 2023: why we celebrate it at oct clinical as a....
© 2020 – 2024 OCT Group LLC. All rights reserved.
Clinical Trials in Eastern Europe, Russia, Ukraine, Georgia, Bulgaria, Latvia, Estonia, Lithuania, Belarus, Moldova, Serbia, Croatia, The Netherlands, Kazakhstan, Turkey, Brazil, Germany, Switzerland
CRO Services
Therapeutic areas, get in touch, thank you for your request our team will respond to you within 24 hours..
This Website uses cookies to offer and ensure better browser experience. Find out more how we use cookies.
- Sponsored Article
Advancing Clinical Research Through Effective Data Delivery
Novel data collection and delivery strategies help usher the clinical research industry into its next era..
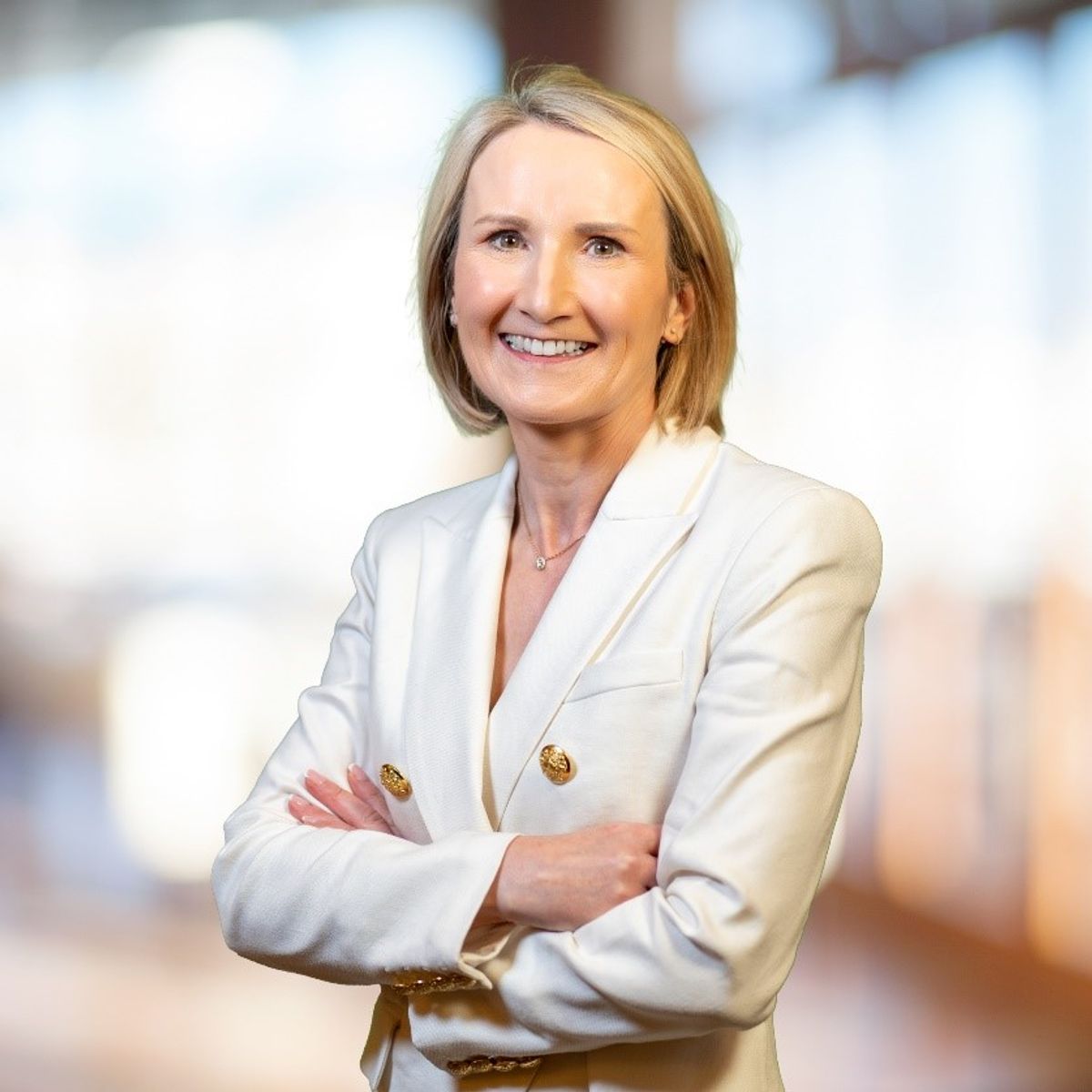
The clinical research landscape is rapidly transforming. Instead of viewing patients as subjects, sponsors now use the patients’ input to help reduce the burden they face during trials. This patient-centric approach is necessary to ensure that the clinical trial staff recruit and retain enough participants and it has led the industry to modify all stages of the clinical trial life cycle, from design to analysis. “What we are seeing is a lot more openness to innovations, digitization, remote visits for the patient, and telemedicine, for example,” said Rose Kidd, the president of Global Operations Delivery at ICON, who oversees a variety of areas including site and patient solutions, study start up, clinical data science, biostatistics, medical writing, and pharmacovigilance. “It is becoming a lot more decentralized in terms of how we collect clinical data, which is really constructive for the industry, and also hugely positive for patients.”
The Increasing Complexity of Clinical Trials
Accurate data is central to the success of a clinical trial. “Research results are only as reliable as the data on which they are based,” Kidd remarked. “If your data is of high quality, the conclusions of that data are trustworthy.” Sponsors are now collecting more data than ever through their trials. 1 This allows them to observe trends and make well-informed decisions about a drug’s or device’s development.
However, these changes in data volume complicate how clinicians design and run their clinical trials. They must capture enough data to fully assess the drug or device without severely disrupting a patient’s lifestyle. Additionally, the investigational sites must ensure that they have enough staff to collect the data in the clinic or through home visits and keep up with their country’s clinical trial regulations. They also must develop efficient data collection and delivery strategies to ensure a trial’s success. While poorly collected data can introduce noise, properly collected data allows clinical trial leads to quickly consolidate and analyze this information. 2 And they often require support with this process.
Innovative Solutions to Improve Data Collection and Delivery
Fortunately, sponsors can find that support with ICON, the healthcare intelligence and clinical research organization. “We essentially advance clinical research [by] providing outsourced services to the pharmaceutical industry, to the medical device industry, and also to government and public health organizations,” Kidd explained. With expertise in numerous therapeutic areas, such as oncology, cell and gene therapies, cardiovascular, biosimilars, vaccines, and rare diseases to mention just a few, ICON helps the pharmaceutical industry efficiently bring devices and drugs to the patients that need them, while ensuring patient safety and meeting local regulations.
One of the areas that Kidd’s team is specifically focused on is providing solutions to advance the collection, delivery, and analysis of clinical data.
The platform that ICON provides to support sponsors in this regard not only stores data directly entered into the system by clinicians during their site or home visits, but also serves as an electronic diary for patients to remotely record their symptoms as they happen. This makes it easier for patients to participate in clinical trials while maintaining their jobs and familial responsibilities. Moreover, this solution provides clinical trial staff with insights into their data as they emerge, such as adverse event profiles and the geographical spread of these events. However, this requires that the data is input into the system in the same manner at every participating site.
To address this problem, ICON’s solutions also include a site-facing web portal that helps to reduce the training burden by standardizing data capture and allowing site teams to learn key information about a drug or device. The portal also offers a visit-by-visit guide to ensure that clinicians are asking the necessary questions for a particular visit and helps them remember how to record the data correctly. “It is training at their fingertips when they need it most,” Kidd said. Solutions like these help sponsors obtain the high-quality clinical data that they need to progress from the trial to the market.
Clinical research is evolving and data strategies that support sites and patients alike must similarly evolve. With the right expertise, experience, and technology solutions, ICON is supporting better decision-making by sponsors.
- Crowley E, et al. Using systematic data categorisation to quantify the types of data collected in clinical trials: The DataCat project . Trials . 2020;21(1):535.
- McGuckin T, et al. Understanding challenges of using routinely collected health data to address clinical care gaps: A case study in Alberta, Canada . BMJ Open Qual . 2022;11(1):e001491.
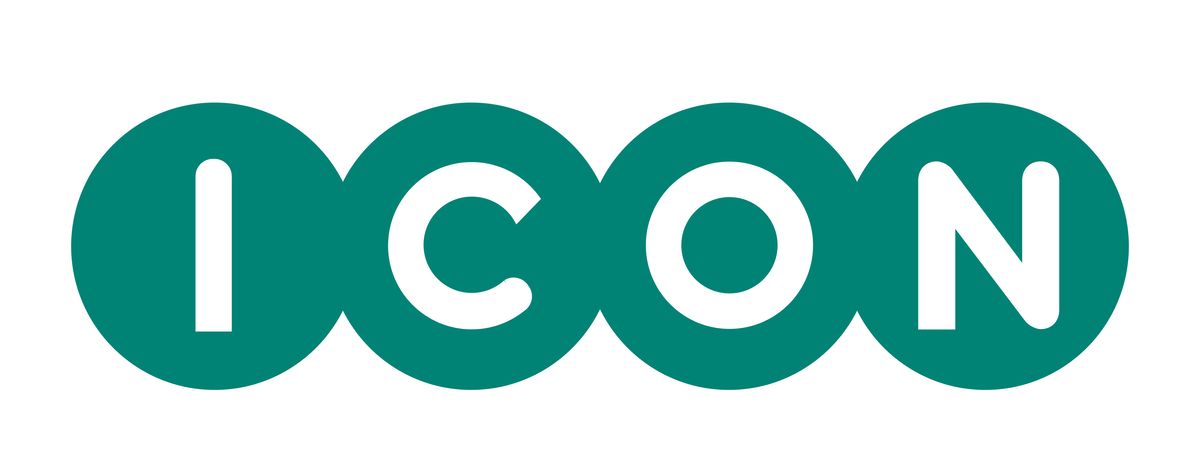
Related drug development Research Resources
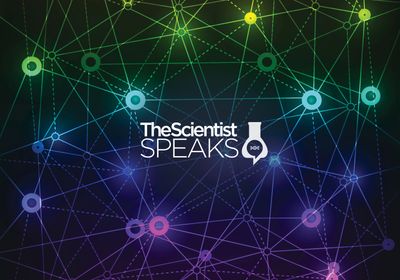
Explainable AI for Rational Antibiotic Discovery

Building Advanced Cell Models for Toxicity Testing

How Cloud Labs and Remote Research Shape Science

An official website of the United States government
The .gov means it’s official. Federal government websites often end in .gov or .mil. Before sharing sensitive information, make sure you’re on a federal government site.
The site is secure. The https:// ensures that you are connecting to the official website and that any information you provide is encrypted and transmitted securely.
- Publications
- Account settings
Preview improvements coming to the PMC website in October 2024. Learn More or Try it out now .
- Advanced Search
- Journal List
- J Med Libr Assoc
- v.107(1); 2019 Jan
Adapting data management education to support clinical research projects in an academic medical center
Associated data.
The workshop evaluation form, resulting data, and slide deck from the “Clinical Research Data Management” workshop are available in Figshare at DOI: http://dx.doi.org/10.6084/m9.figshare.7105817.v1 .
Librarians and researchers alike have long identified research data management (RDM) training as a need in biomedical research. Despite the wealth of libraries offering RDM education to their communities, clinical research is an area that has not been targeted. Clinical RDM (CRDM) is seen by its community as an essential part of the research process where established guidelines exist, yet educational initiatives in this area are unknown.
Case Presentation
Leveraging my academic library’s experience supporting CRDM through informationist grants and REDCap training in our medical center, I developed a 1.5 hour CRDM workshop. This workshop was designed to use established CRDM guidelines in clinical research and address common questions asked by our community through the library’s existing data support program. The workshop was offered to the entire medical center 4 times between November 2017 and July 2018. This case study describes the development, implementation, and evaluation of this workshop.
Conclusions
The 4 workshops were well attended and well received by the medical center community, with 99% stating that they would recommend the class to others and 98% stating that they would use what they learned in their work. Attendees also articulated how they would implement the main competencies they learned from the workshop into their work. For the library, the effort to support CRDM has led to the coordination of a larger institutional collaborative training series to educate researchers on best practices with data, as well as the formation of institution-wide policy groups to address researcher challenges with CRDM, data transfer, and data sharing.
For over ten years, data management training has been identified as a need by the biomedical research community and librarians alike. From the perspective of biomedical researchers, the lack of good quality information management for research data [ 1 , 2 ] and an absence of training for researchers to improve their data management skills are recurring issues cited in the literature and a cause for concern for research overall [ 1 , 3 , 4 ]. Similarly, librarians practicing data management have identified that researchers generally receive no formal training in data management [ 5 ] yet have a desire to learn [ 6 ] because they lack confidence in their skills.
To address this need, librarians from academic institutions have been working to provide data management education and support to their communities. By developing specific approaches to creating data management education, libraries have found successful avenues in implementing stand-alone courses and one-shot workshops [ 7 ], integrating research data management into an existing curriculum [ 8 ], and offering domain-specific training [ 9 ]. Libraries have offered these training programs by providing general data management training to undergraduate and graduate students [ 10 – 12 ], doctoral scholars [ 13 ], and the general research community [ 14 – 20 ], whereas domain-specific data management can be seen most prominently in the life sciences [ 21 ], earth and environmental sciences [ 22 , 23 ], social sciences [ 24 ], and the digital humanities [ 25 ].
While it is clear that libraries have made inroads into domain-specific areas to provide training in data management, the clinical research community—clinical faculty, project and research coordinators, postdoctoral scholars, medical residents and fellows, data analysts, and medical or doctoral degree (MD/PhD) students—is one that has not received much attention. Clinical research data management (CRDM), an integral part of the clinical research process, differs from the broader concept of research data management because it involves rigorous procedures for the standardized collection and careful management of patient data to protect patient privacy and ensure quality and accuracy in medical care. The clinical research community understands the importance of data standardization [ 26 – 29 ], data quality [ 30 – 33 ], and data collection [ 28 , 34 – 36 ] and has established good clinical data management practices (GCDMP) [ 37 ] to ensure that CRDM is conducted at the highest level of excellence.
Despite this community-driven goal toward CRDM excellence, there is a dearth of literature about data management training for clinical research, with the only evidence coming from nursing training programs [ 35 , 38 ], whose research practices are further afield in that they focus on quality improvement rather than clinical investigations. This lack of evidence is surprising considering that the need for CRDM training has been communicated [ 1 , 3 , 4 , 6 ].
My library, located in an academic medical center, has supported CRDM through National Library of Medicine informationist projects by collaborating with clinical research teams to improve data management practices [ 39 ] and, more recently, by serving as the front line of support for REDCap (an electronic data capture system for storing research data) by offering consultations and comprehensive training [ 40 ]. Through REDCap training, I identified a need to expand my knowledge of CRDM to better support the needs of our research community. While REDCap is a tool to help researchers collect data for their studies, the majority of issues that our clinical research community encountered were related to data management. These issues included developing data collection plans, assigning and managing roles and responsibilities throughout the research process, ensuring that the quality of data remains intact throughout the course of the study, and creating data collection instruments. As this recurring thread of issues expanded the learning needs of our community beyond those provided via our REDCap training, I decided to expand my knowledge to address the questions that our researchers asked, to develop a curriculum to support CRDM, and to offer and evaluate CRDM training for our community.
STUDY PURPOSE
This case study will discuss (a) the development and implementation of a 1.5-hour CRDM workshop for the medical center research community, (b) the results and outcomes from teaching the CRDM workshop, and (c) the next steps for the library in this area.
CASE PRESENTATION
Workshop development, gaining skills.
Beyond the experience I gained from working closely with researchers on their clinical research projects and through REDCap support, I took two particularly valuable training opportunities that improved my skills in CRDM: the “Data Management for Clinical Research” Coursera course [ 41 ] and “Developing Data Management Plans” course [ 42 ] offered through the online educational program sponsored by the Society for Clinical Data Management. These two courses provided me with the knowledge that I needed to teach a CRDM workshop but more importantly gave me the confidence to teach it because they provided a depth of knowledge I did not have before. These courses also served to reinforce that the issues and challenges encountered at my own institution were common data management concerns across the broader clinical research community.
Identifying core competencies and building workshop content
The primary focus for developing a 1.5-hour CRDM workshop was to use the GCDMP core guidelines [ 37 ] as the baseline structure for the workshop. The core guidelines are separated into chapters in the GCDMP, which were used as the foundation for the core competencies of the workshop. Once this baseline structure was established, my goal was to weave in answers to the common questions that our clinical research community has asked through our existing REDCap training. These questions related to how to create codebooks and data dictionaries for research projects, how to structure roles in a research team, how to use best practices for building data collection instruments, how to protect their data according to Health Insurance Portability and Accountability Act (HIPAA) regulations that they should be aware of, how to improve the quality of their data throughout a study, and how to best document procedures throughout a study.
The goal of the workshop was to tie as many examples back to REDCap as possible, because the use of REDCap was written into institutional policy as the recommended tool for research data collection, which made it essential to highlight its data management capabilities. The core competencies combined with the questions mentioned above served as the foundation for developing the learning objectives and interactive learning activities for the workshop ( Table 1 ).
Clinical research data management workshop core competencies
The core competencies and learning objectives were designed to make the workshop as practical as possible. While the theoretical components of CRDM are important and are emphasized in the workshop, the main focus was to consistently incorporate interactive learning throughout so that attendees could both apply and contextualize what they learned to their own research. Another goal of this workshop was to encourage communication between attendees to highlight common CRDM errors and provide avenues for attendees to learn about successful and unsuccessful approaches from their peers. To this end, after each core competency was taught, the workshop was designed to have attendees discuss their own experiences.
In addition to the core competencies listed in Table 1 , the overarching theme and intention applied across the workshop was the importance of maintaining good documentation throughout a clinical research project (e.g., data collection plan, roles and responsibilities documents, statistical analysis plan). By stressing the importance of documentation for each competency, I hoped that attendees would understand the value of and be able to develop their own detailed documentation at each stage of the research process. The time dedicated to developing this workshop—which included reviewing the GCDMP core competencies, outlining commonly asked questions from the research community, establishing learning objectives, building the slide deck, and creating the workshop activities—took between 80 and 100 hours to complete.
Workshop implementation
The CRDM workshop was offered broadly throughout the medical center three separate times in November 2017, January 2018, and February 2018. These workshops were promoted using our library’s email discussion list of attendees from previous data classes and the Office of Science and Research and Clinical and Translational Science Institute’s announcements emails. Direct outreach was also extended to residency directors and research coordinators, both of whom regularly attend the library’s REDCap training. A fourth workshop was offered in July 2018 as part of the library’s established Data Day to Day series [ 43 ], which the library has substantially marketed through posters, write-ups in institutional newsletters, and broadcast emails.
Workshop evaluation
The CRDM workshop evaluation consisted of both quantitative and qualitative methods using a questionnaire administered at the conclusion of each workshop ( supplemental Appendix ). This study was deemed exempt by our institutional review board (IRB). Using Likert scales, questions asked attendees to evaluate the difficulty level of the material presented in the workshop, their willingness to recommend the workshop to others, and their intention to use what they had learned in their work. Free-text questions asked attendees to specify how they would use what they learned in their current roles in the institution and what other course topics they would be interested in learning about. For the question that asked attendees to describe how they would use what they learned in their current roles, I hand coded responses in a spreadsheet using the emergent coding technique [ 44 ] to identify the competencies that attendees stated as the most applicable to their work.
Workshop results
Of the 145 attendees at the 4 workshops, 113 provided fully or partially completed evaluation forms. Overall registration to and attendance at all 4 workshops was very high, with substantial waitlists accumulating for each class offered ( Figure 1 ). In fact, the workshop offered in February 2018 was a direct result of having 60 people on the waitlist from the January session. Waitlists were useful for identifying communities that I had not reached through training to date as well as for understanding the popularity of the topic for the research community. If the waitlist was high in number, it provided another opportunity to offer the workshop or reach out to attendees to see if there was an opportunity to teach a smaller class in their departments.

Total attendance, registration, and waitlist numbers for the four clinical research data management (CRDM) workshops
There was a wide range of attendees at these workshops ( Figure 2 ), as there were no restrictions on who could attend. Project/research coordinators (n=38), faculty (n=18), and managers (n=13) were prominent attendees at the workshop, and their comments in the evaluation form reflected its value and the importance of someone from the library teaching this material.

Roles of attendees of the four CRDM workshops
Research coordinators and project managers specifically indicated that the CRDM workshop was helpful in multiple ways for their roles, including how to set up the organization of their data collection procedures, how to establish and clarify roles in a research team, and how to develop documentation for both data collection and the roles and responsibilities of their staff. Research coordinators also indicated that no other stakeholders in the institution taught this kind of material and that this type of training was essential for their work.
Faculty indicated that the workshop was beneficial for developing project management skills, gaining an awareness of the benefits of using REDCap to both collect and manage data, and clarifying the roles and responsibilities of statisticians on their team. They also mentioned the benefits of their study team taking a workshop of this kind at the beginning of a study.
Attendees more generally described the value of the resources presented in the workshop, specifically stating that using REDCap, locating resources for identifying relevant data collection standards, gaining awareness of institutional data storage options, and using the workshop slide deck to guide their CRDM processes were particularly helpful.
Overall, the evaluation data indicated positive results, with the majority of those who responded (94%) indicating the level of material was just right and almost all who responded stating they would recommend the class to others (99%) and would use what they learned in their work (98%). Additionally, responses from attendees who indicated how they would use what they learned and apply it to their current role helped provide additional context for the benefits of the CRDM workshop ( Figure 3 ) with improving documentation (37%), planning work flows (34%), using REDCap (22%), and assigning roles and responsibilities (17%) being the most prominent applications of the core competencies learned.

How attendees would use what they learned in their current roles
Finally, attendees expressed interest in many additional topics that they would like to see taught in future classes. These topics included statistics, research compliance, the legal implication of data sharing, and IRB best practices for study design. It is important to mention that attendees indicated that they would like to see these additional topics taught in tandem with the CRDM workshop so that they could gain a better understanding of CRDM from the perspective of an established institutional work flow for clinical research projects.
Considering that this was the first time that I had offered CRDM training to our research community, the overall attendance, high waitlist numbers, and percentage of attendees who said the course content was at the appropriate level validated the educational approach that I used. One major concern during the workshop development phase was that the content would be too rudimentary for our research community; however, the evaluations suggested that this was not the case. Furthermore, since one of the central goals of the CRDM workshop was to emphasize the importance of documentation for each core competency, the fact that this was the most commonly cited application of what attendees learned was further validation of the CRDM workshop’s course content.
While my approach was to utilize REDCap as a resource to demonstrate good CRDM practices because it served a direct purpose for our research community, this workshop can be taught without reference to it. The core competencies of this workshop ( Table 1 ) are based on fundamental guidelines of good CRDM practice, and these competencies and skills are applicable to any stakeholder who participates in clinical research, no matter what tool or format they decide to use to collect their data.
The positive reviews of the four broadly offered courses led to seven additional CRDM training sessions that were requested by specific departments and research teams, indicating a strong need from our research community for this material. Evaluation forms were not distributed during these seven sessions due to the consult-like nature of these requests. During these sessions, several research coordinators indicated that the CRDM workshop should be required for all clinical research teams before their studies begin. This call for additional training presents an opportunity for our library to incorporate CRDM education into existing institutional initiatives. Specifically, I identified our institutional education and training management system, residency research blocks, and principal investigator training as logical next steps for integrating CRDM education into institutional research work flows.
The evaluation data initiated the development of partnerships with other institutional stakeholders to better support clinical research training efforts. Our library has begun conversations with stakeholders from research compliance, general counsel, the IRB, the Office of Science and Research, and information technology (IT) to identify ways to better address the needs of clinical researchers. The CRDM workshop highlighted a level of uncertainty on the part of clinical researchers about how best to conduct research in the medical center and whom to contact when faced with certain questions or issues.
Subsequent discussions with the aforementioned stakeholders have emphasized a need to provide more clarity to our community about the research process. To this end, our library is leading the coordination of these groups to offer a comprehensive clinical data education series with representatives from each major department providing their own training to complement the library’s existing REDCap and CRDM workshops. This training series will likely be offered through our library’s existing “Data Day to Day” series so that the research community can take all of the classes within a short time span.
The lack of institutional clarity that attendees and the aforementioned stakeholders identified has also led to policy discussions related to data transfer, sharing, and compliance, as our current institutional procedures are unclear and poorly utilized. Through the development of new standard operating procedures and increased educational initiatives, our library is driving awareness of institutional best practices with the hopes of improving clinical research efficiency. Members from our library now sit on institutional policy working groups that are working to improve institutional data transfer and data sharing work flows.
Just as librarians at the University of Washington carved out a role for themselves in supporting clinical research efforts [ 45 ], we seized the opportunity to do the same by offering CRDM education. As the first line of defense for teaching researchers, identifying their data management issues, and hearing their concerns, our library is serving as the conduit for ensuring clinical research is conducted according to GCDM practices at our institution. Establishing partnerships with research compliance, general counsel, the Office of Science and Research, and IT provides us with additional knowledge of their institutional roles and subsequently enables us to send researchers in the right direction to receive the necessary expertise and support. As this service model develops, our library plans to monitor and assess referrals to these other departments to demonstrate the value of increasing compliance in the institution and to integrate CRDM education services into any newly developed policy (which we were successful in doing for the new institutional data storage policy and REDCap). With our library serving as the driving force behind the improvement of CRDM support, the ultimate goal is that these new partnerships will result in our research community being better trained, more compliant, and increasingly aware of established institutional work flows for clinical research
DATA AVAILABILITY STATEMENT
Supplemental file.
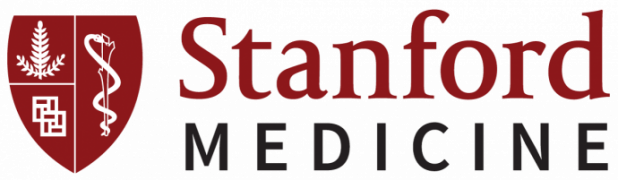
Stanford Cancer Institute
Search stanford cancer institute, new nih requirements for proposals in january 2023.
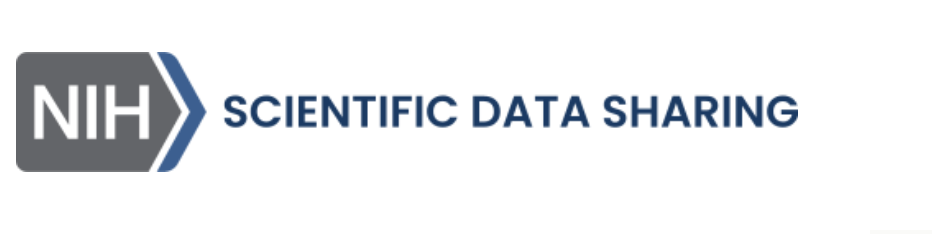
- Training (T)
- Fellowships (Fs)
- Construction (C06)
- Conference Grants (R13)
- Resource (Gs)
- Research-Related Infrastructure Programs (e.g., S06)
- Start planning now for the selection of the appropriate repository for data sharing and secondary uses.
- Note that non-compliance with this new requirement for a DMS plan could result in your proposal not moving forward for scientific review
- Resources and support are available through the Lane Library.
The information below is for NIH-sponsored PI and their research teams, and staff responsible for supporting sponsored research.
What are the new NIH requirements?
From January 25, 2023, the National Institutes of Health (NIH) will require all new proposals that involve the generation of scientific data to include a 2-page Data Management and Sharing Plan (DMSP or DMP). This information must be provided in a new “other Plan(s)” attachment field in the PHS 398 Research Plan form. Applicants must attach the DMSP/DMP in this new field in FORMS-H applications .
The plan must include six elements (as specified in NOT-OD-21-014 ):
- Related Tools, Software, and/or Code
- Data Preservation, Access, and Associated Timelines
- Data Access, Distribution, or Reuse Considerations
- Oversight of Data Management and Sharing
Getting Assistance
The VPDoR, School of Medicine, and a community of Stanford-led teams are partnering to coordinate support for principal investigators and research teams across campus who are preparing new proposals for the coming funding cycle.
Resources are available to Principal Investigators and research teams that will be affected by these changes.
How should I prepare?
Researchers who foresee submitting new proposals for data-generating studies should start planning now, by prioritizing the following:
- Selection of the appropriate repository for data sharing and secondary uses;
- Planning for curation , deposit, local management, preservation, and sharing of data (if applicable). This includes building the costs of these activities into project budgets. (Information regarding categories of allowable costs can be found here .)
- Preparing to draft a DSMP/DMP (Data Sharing and Management Plan/Data Management Plan) to be included in submissions on or after 1/25/23. DMPs must account for human subjects protections and privacy. If your project involves data derived from human participants, it is paramount that researchers uphold six operational privacy principles in developing the plan and throughout the project:
- Proactive assessment of protections
- Clear communication of data sharing and use in consent forms
- Considerations of justifiable limitations on data sharing
- Institutional review of the conditions for data sharing
- Protections for all data used in research
- Remaining vigilant regarding data misuse
Where do I find information to help me estimate repository costs?
Costs depend on the repository selected and the size of the data sets to be stored. NIH provides a list of approved domain-specific and generalist repositories. In addition, Stanford provides DMP consultation services to assist in creating a budget for your data management needs and free archival of data in the Stanford Digital Repository , which meets or exceeds NIH repository requirements.
The National Academies of Science Engineering and Medicine have posted resources to help researchers forecast biomedical data sharing costs .
Is there guidance on designating data for ‘controlled access’ v ‘openly without access restrictions?
Yes. The Policy provides points to consider factoring into controlled access decisions.
Are there tips and guidance for generating high-quality metadata, preparing files for sharing, and documentation?
Yes. Through the Generalist Repository Ecosystem Initiative (GREI), the NIH Office of Data Science Strategy has created a publicly available webinar series on repository sharing best practices.
What about data curation resources?
In addition to DMP data management services provided by the Stanford University Library and Lane Library , the following external links describe curation and archival services using various cost and delivery models including:
- Dryad (Stanford subscribes)
- Center for Open Science
- Elsevier Digital Commons
- Vivli (clinical trial data) (Stanford subscribes)
- Harvard Dataverse (includes curation and price table)
See this chart comparing generalist repositories .
What resources are available to help me?
- NIH maintains a website dedicated to scientific data sharing designed as a centralized landing page for resources.
- Visit NIH’s 12 tips for compliance with the new policy.
- If you have questions about the NIH requirements, data management, repository selection, or assistance with your Data management and Sharing Plan, please contact the new Stanford DMP Service .
- If you have questions about the policy’s provisions concerning privacy, security, or ELSI (ethical, legal, or social implications), please e-mail Scott Edmiston [email protected]
- The Lane Library in the School of Medicine has created a resource guide accessible to anyone interested in gaining better knowledge on the process.
- The Stanford Data Farm is a research tool built on the Redivis platform enabling a host of features aligned with NIH requirements, such as persistent identifiers, logging/auditing, governance, privacy, security, and secure analytics for a variety of structured and unstructured data types.
Additional sources of information and assistance:
- Read the final NIH Policy Data Management and Sharing and review NIH’s new Sharing.NIH.gov site to understand the policy changes further.
- Watch the recent School of Medicine Faculty Townhall presentation on the upcoming changes.
- Plan to attend The Stanford Program on Research Rigor and Reproducibility ( SPORR ), SoM colloquium and Help-a-Thon on January 23, 2023, with sign-up for onsite data consultations. Hosted in collaboration with the Lane Library and Quantitative Sciences Unit. Registration details are forthcoming.
We continue to monitor the changes and encourage you to stay connected to the Research Administrators in your schools for additional updates.
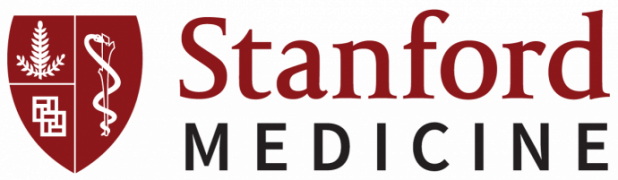
- Patient Care
- Clinical Trials
- Health Equity
- Shared Resources
Stanford Medicine
Health care.
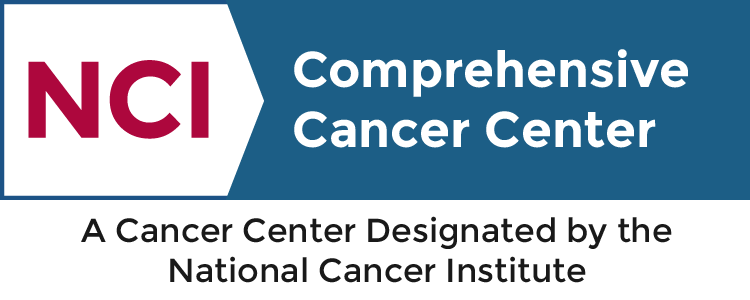
©2024 Stanford Medicine

An official website of the Department of Health & Human Services
- Search All AHRQ Sites
- Email Updates
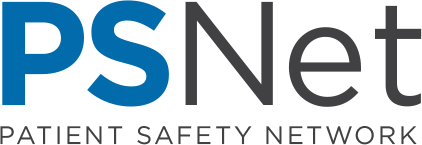
1. Use quotes to search for an exact match of a phrase.
2. Put a minus sign just before words you don't want.
3. Enter any important keywords in any order to find entries where all these terms appear.
- The PSNet Collection
- All Content
- Perspectives
- Current Weekly Issue
- Past Weekly Issues
- Curated Libraries
- Clinical Areas
- Patient Safety 101
- The Fundamentals
- Training and Education
- Continuing Education
- WebM&M: Case Studies
- Training Catalog
- Submit a Case
- Improvement Resources
- Innovations
- Submit an Innovation
- About PSNet
- Editorial Team
- Technical Expert Panel
Suicide Prevention in an Emergency Department Population: ED-SAFE
- Contact Innovator
Suicide is the 12 th leading cause of death in the United States, and the 3 rd leading cause of death for people ages 15-24. 1 More than 4% of all emergency department visits are attributed to psychiatric conditions 2 and 3–8% of all patients have suicidal ideation when screened in the ED. 3 In addition, there are approximately 420,000 ED visits every year for intentional self-harm. 4 The emergency department (ED) is an ideal place to implement interventions designed to reduce suicidal behavior. However, there have been few trials conducted in clinical settings to reduce suicidal behavior.
Brown University and Butler Hospital created the Emergency Department Safety Assessment and Follow-Up Evaluation (ED-SAFE) innovation to reduce suicidal behavior among patients who present to the ED with suicidal ideation. They published the results from the initial clinical trial, ED-SAFE 1, a multicenter study of eight EDs that assessed the ED-SAFE innovation. The ED-SAFE 1 innovation provided participants with a standard universal suicide risk screening (any standard universal screening tool can be applied) plus a secondary suicide risk screening by an ED physician. It also included discharge resources (including a self-administered safety plan) and post-ED telephone calls based on the Coping with Long Term Active Suicide Program (CLASP) 5 focused on reducing suicide risk. 6 In ED-SAFE 1, there was a 5% absolute reduction in suicide attempts between the treatment as usual and intervention phases. 6 During the intervention phase, participants had 30% fewer total suicide attempts than participants in the treatment as usual phase. 6 The study found that universal screening alone did not reduce suicide attempts, and therefore, the reduction is most likely tied to the innovation itself. 6
The ED-SAFE 2 trial implemented two key elements that built on ED-SAFE 1: a Lean continuous quality improvement (CQI) approach and collaborative safety planning between patients and caregivers. Data were collected from 2014 to 2018 and analyzed from April 2022 to December 2022. 3 The trial included three phases: baseline (retrospective), implementation, and maintenance. 3 During implementation, each of the eight EDs formed a Lean team consisting of staff, management, information technology (IT), patient safety, and quality assurance members. The teams attended a one-day training and monthly followup meetings on Lean principles with an industrial engineering Lean expert with doctoral training. 3 The teams evaluated their workflows, identified gaps in care, designed solutions to close these gaps, and oversaw the implementation of ED-SAFE 2. 3 Additionally, the innovators implemented collaborative safety planning. Collaborative safety planning involved six-step safety plans created by clinicians and patients to help patients manage their individual suicidal crises. 3 In addition to these changes, teams were expected to increase the number of suicide risk screenings for patients. 3 The primary outcome measured was a suicide composite measure. The measure included 1) an ED visit or hospitalization due to suicidal ideation/behavior or 2) death by suicide in the six months after the index visit. 3 The composite measure improved over the three phases (baseline by 21%; implementation by 22%; and maintenance by 15.3%; p=.001). 3
Innovation Patient Safety Focus
Although the National Action Alliance for Suicide Prevention and The Joint Commission both identify EDs as an essential setting for suicide prevention, suicide prevention interventions in EDs remain underdeveloped and understudied. 3 The Joint Commission identifies suicide within 72 hours of discharge from a healthcare setting that provides around-the-clock care, including the ED, as a sentinel event (a patient safety event that results in death, permanent harm, or severe temporary harm). 3
Resources Used and Skills Needed
When implementing this innovations, organizations should consider the following:
- Buy-in from hospital leadership and all staff involved in the continuum of care.
- Staff to conduct post-visit phone calls.
- Physicians willing to conduct secondary suicide prevention screenings.
- Physicians to serve as treatment advisers for the post-visit phone calls.
- Time and resources to train staff on the intervention.
- Staff to conduct data analysis.
- Leaders to train multidisciplinary teams on the Lean CQI strategy.
- Staff to participate in the Lean teams and create collaborative safety plans with patients.
- Clinicians with the bandwidth to create collaborative safety plans with patients. These clinicians must attend at least one training related to collaborative safety planning and they must demonstrate competency in collaborative safety planning through observation or other work samples.
- Staff to champion the maintenance stage of the innovation.
- Staff to measure and report results: at least one person with data related skills who can use the EHR for reporting per site; 40-80 hours are needed as an initial investment for setting up reports followed by one to two hours per month after the initial investment per site.
Use By Other Organizations
The innovator has received regular inquiries from other EDs about their ED-SAFE innovation. Much of the ED-SAFE innovation aligns with the Zero Suicide model, an emerging model for suicide prevention in healthcare. 7
Date First Implemented
Problem addressed.
The innovators created the Emergency Department Safety Assessment and Follow-Up Evaluation (ED-SAFE) 2 to build on the successes from ED-SAFE 1 (the reduction of suicidal behavior). The innovators wanted to sustain the suicide risk management that was achieved in ED-SAFE 1. 3 They deduced that a continuous quality improvement (CQI) strategy was the best way to ensure consistency, effectiveness, and the standard use of the new procedures and interventions from ED-SAFE 1. 3 This led to the development of the Lean CQI strategy, one of the key elements of ED-SAFE 2, and a focus on collaborative safety planning as a standard part of treating patients at risk for suicide who are discharged from the ED.
Description of the Innovative Activity
The ED-SAFE study was designed to assess the effect of universal screening for suicide risk and an intervention for people at risk for suicide in the ED setting. 6 ED-SAFE 1 included elements like standard universal screening, secondary risk screening, collaborative safety plans, and post-ED phone calls. Universal screening and secondary screening were carried over into the ED-SAFE 2 study. 3 The primary and secondary screener are both referred to as the Patient Safety Screener (PSS-3). 8 The first part of the PSS-3 is used to identify suicide risk for all patients who come to the acute care setting. 8 The second part of the PSS-3 takes those who have been identified with risk of suicide and guides risk stratification. 8 The second part of PSS-3 guides physicians through care pathways and mitigation procedures based on the patient’s risk level. The screeners also include other risk factors, like psychiatric hospitalization. This makes PSS-3 different than many other predominate screeners that focus entirely on suicidal ideation and behavior. When all elements of ED-SAFE 1 are implemented, the intervention costs around $1,000 per patient, per month. 9
For ED-SAFE 2, two key improvements were made: the implementation of the Lean CQI strategy and the introduction of collaborative safety planning with clinicians and patients. 3 Each of the eight EDs created a Lean CQI multidisciplinary team. 3 The team included members from all areas of patient care and support services, including frontline providers, patient safety professionals, information technology (IT) staff, and quality assurance staff. 3 Each team participated in a day-long training on Lean principles. 3 Following the training, the team attended monthly coaching calls to ensure that they had consistent knowledge of the Lean principles. 3 The Lean teams evaluated their workflows to find gaps that could be targeted with interventions. 3 The interventions to close these gaps focused on addressing the root causes associated with negative patient care outcomes. 3 In addition, the Lean teams oversaw the implementation of the interventions they established. 3 The eight ED Lean teams reported their metrics to a coordinating center each month to ensure continuity across the whole system. 3
Collaborative safety planning involved a six-step safety plan created by a clinician and a patient; the goal of the safety plan was to help manage individual suicidal crises for patients who screened positive for suicidal ideation but who were discharged from the ED. 3 When patients were in crisis states, they used the collaborative safety plans. The plans helped patients to cope with the crisis by providing collaborative interventions to curb suicidal thoughts. The plans included interventions that patients could do on their own and ideas for interventions they could do with others. The plans also directed patients on how and when to reach out for additional professional help. Although created collaboratively, the plans were written in the patient’s voice. The clinicians attended a training with a safety planning intervention trainer. The trainers followed up with the clinicians each month to provide additional training as needed, to review safety plans, and to provide feedback to the clinicians. 3
Context of the Innovation
Emergency departments treat many patients who are at risk for suicidal behavior. High-risk individuals are susceptible to suicide attempts after their ED visit. 10 In addition, a significant number of those who die by suicide received care in an ED in the period prior to death. 11 In a study using Medicaid data from 2008 to 2018, researchers looked at data from national cohorts of patients with mental health ED visits due to suicide attempts. Researchers wanted to determine the rate of suicide for these patients up to one year after discharge. 12 Among these patients, the suicide rate was 325.4 per 100,000 person years. 12 This finding contrasts with the rate of suicide in the general population as reported by the Centers for Disease Control and Prevention (CDC), which was 14.1 per 100,000 person years in 2021. 13 Because of these key patient safety factors, the innovators chose to conduct this intervention to improve patient safety for those at risk of suicide or suicidal behavior who are presenting to the ED.
The positive results from ED-SAFE 1 prompted the development of ED-SAFE 2. In ED-SAFE 1, compared with the treatment as usual phase, patients in the intervention phase showed a 5% absolute reduction in the risk of a suicide attempt (23% vs 18%; p=0.05). 6 Participants in the intervention phase in ED-SAFE 1 had 30% fewer total suicide attempts than participants in the treatment as usual phase. 3 For ED-SAFE 2, across all three phases (baseline, implementation, and maintenance), 2,761 patient encounters were included in the study analysis. 3 The intervention was based on a suicide composite measure. The measure included an ED visit or hospitalization due to suicidal ideation/behavior or death by suicide in the six months after the index visit. 3 The percentage of patient encounters with a suicide composite outcome decreased from 21% as baseline (216 of 1030) and 22% at implementation (213 of 967) to 15.3% during the maintenance phase (117 of 764; p=0.001). 3 The adjusted odds ratio of risk demonstrated a 43% reduction in the maintenance phase compared to the implementation phase and a 39% reduction in the maintenance phase compared to the baseline phase. 3
Planning and Development Process
When planning and developing this innovation, it is important to ensure that the innovating site is committed to change, there is support from senior leadership, a multidisciplinary team is convened, a standard definition of suicide risk is established, and a gap analysis is completed.
The innovators recommend convening a multidisciplinary team when planning and developing this innovation. The team must consist of members of the healthcare organization who are involved in the reduction of suicide and care pathways within the innovating organization, if possible. This may include senior leaders, frontline staff, patient safety officers, and nurse managers. The team will be instrumental in developing the innovation. Because there is no one-size-fits-all approach for EDs, the team should adapt ED-SAFE to fit their organization. Senior leadership should give the multidisciplinary team the authority to make changes in the organization that are necessary for the innovation’s success. For example, there must be a suicide risk screener administered with the innovation. However, an innovating organization should have the flexibility to pick which screener they would like to use, when they would like to screen patients, who they would like to administer the screener, and whether the screener is universal for all patients who present to the ED.
Before this innovation is implemented, it is critical that the innovation organization agree on a standard definition of suicide risk. This definition will be instrumental in determining who is eligible for the innovation.
In addition, the innovating organization should conduct a gap analysis. The gap analysis will evaluate the current state of managing risk of suicide among patients presenting to the ED versus what the innovating organization would like to achieve. The organization can determine the current state by convening meetings with management and frontline staff to reconcile the differences between the policies in place to reduce suicide risk and what is actually occurring at the organization. The organization will remedy the gaps identified in the analysis with targeted interventions. In addition, the organization will use data discovered in the gap analysis to monitor the success of the innovation.
If this innovation was used in a smaller emergency department, the smaller emergency department would likely need to adjust the scope and pace of the innovation to make it successful. However, if the ED has a strong champion, the innovation could be successful. For example, the smaller organization may need to establish who their quality improvement team will be, as they may not have a dedicated quality improvement department. A nurse manager could serve as the quality improvement lead. In addition, the smaller ED could conduct a modest amount of chart reviews rather than using EHR data reports to evaluate performance.
Funding Sources
The National Institute of Mental Health funded this innovation.
Getting Started with This Innovation
The innovators found that a deployment plan was necessary when getting started with this innovation. A deployment plan lays out the step-by-step process for implementing the innovation. It includes details like what to do first and includes the ways that every person involved in the innovation will be trained. The deployment plan also describes how the organization will gather and measure metrics and tracks implementation successes and challenges when implementing the innovation. For example, the deployment plan may include metrics that track whether the innovation is being adopted within the organization. If the innovator discovers that the organization is not adopting certain elements, they can make changes to rectify the situation. For example, the innovator discovered via their deployment plan that the screener was not being used as intended. Because of this, the innovator created targeted trainings to remedy the situation and improve the success of the innovation.
Sustaining This Innovation
The innovators found a continuous quality improvement (CQI) approach to be the primary investment in sustaining the innovation. The CQI approach identifies areas for improvement throughout the lifetime of the innovation. The CQI approach monitors the training of personnel, reviews performance, identifies gaps, creates ways to remediate gaps, implements, and evaluates remediation measures, and remeasures performance after remediation. The CQI approach should be iterative and provide flexibility for staff who take part in the innovation.
One component of the innovator’s CQI process were spot checks. The innovator conducted spot checks using the same measures that were created during the gap analysis. Spot checks help the innovators gauge whether the innovation is reaching the final target that was established during the gap analysis. If the spot checks find there are reoccurring gaps, an innovator may want to consider going back to a previous implementation phase to increase adoption of the innovation. The innovators found that conducting spot checks during suicide awareness month garnered strong support from leadership and frontline staff, as there was already a renewed focus on suicide prevention at that time.
The innovators found the minimum investment to maintain results included quarterly meetings with the innovation team (usually four to ten people for one hour), a core steering committee (usually three to five people for two hours a quarter, plus leading the quarterly meetings), and trainers focused on training new employees and current staff as needed (three to five hours a quarter). An annual review of current protocols to determine if the protocols should be updated due to new care expectations or evidence-based best practices is also beneficial when sustaining this innovation.
References/Related Articles
ClinicalTrials.gov. Emergency Department Safety Assessment and Follow-Up Evaluation (ED-SAFE). https://clinicaltrials.gov/study/NCT01150994
Miller I, Gaudiano B, Weinstock L. The Coping Long Term with Active Suicide Program (CLASP): description and pilot data. Suicide Life Threat Behav . 2016;46(6):752-761. doi:10.1111/sltb.12247
- Save.org. U.S.A. SUICIDE: 2020 OFFICIAL FINAL DATA. https://save.org/wp-content/uploads/2022/01/2020datapgsv1a-3.pdf
- Owens P, Mutter R, Stocks C. Mental Health and Substance Abuse-Related Emergency Department Visits Among Adults, 2007 . Statistical Brief #92. July 2010. Agency for Healthcare Research and Quality, Rockville, MD. https://www.hcup-us.ahrq.gov/reports/statbriefs/sb92.pdf
- Boudreaux ED, Larkin C, Vallejo Sefair A, et al. Effect of an emergency department process improvement package on suicide prevention: the ED-SAFE 2 cluster randomized clinical trial. JAMA Psychiatry 2023;80(7):665-674. doi:10.1001/jamapsychiatry.2023.1304
- Ting SA, Sullivan AF, Boudreaux ED, Miller I, Camargo CA Jr. Trends in US emergency department visits for attempted suicide and self-inflicted injury, 1993-2008. Gen Hosp Psychiatry . 2012;34(5):557-565. doi:10.1016/j.genhosppsych.2012.03.020
- Miller I, Gaudiano B, Weinstock L. The Coping Long Term with Active Suicide Program (CLASP: A Clinicians Guide to a Multi-Modal Intervention for Suicide Prevention. Oxford University Press; 2022.
- Miller IW, Camargo Jr CA, Arias SA, et al. Suicide prevention in an emergency department population: the ED-SAFE Study. JAMA Psychiatry . 2017;74(6):563-570. doi:10.1001/jamapsychiatry.2017.0678
- Education Development Center. Zero Suicide. Accessed February 12, 2024. https://zerosuicide.edc.org
- Boudreaux ED. The Patient Safety Screener: A Brief Tool to Detect Suicide Risk. Accessed March 18, 2024. https://sprc.org/micro-learning/the-patient-safety-screener-a-brief-tool-to-detect-suicide-risk/
- Dunlap L, Orme S, Zarkin G, Miller I. Screening and Intervention for Suicide Prevention: A Cost-Effectiveness Analysis of the ED-SAFE Interventions. Accessed March 19 2024. https://pubmed.ncbi.nlm.nih.gov/31451063/
- Olfson M, Marcus SC, Bridge JA. Focusing suicide prevention on periods of high risk. JAMA . 2014;311(11):1107-1108. doi:10.1001/jama.2014.501
- Ahmedani BK, Simon GE, Stewart C, et al. Health care contacts in the year before suicide death. J Gen Intern Med . 2014;29(6):870-877. doi:10.1007/s11606-014-2767-3
- Olfson M, Gao YN, Xie M, Wiesel Cullen S, Marcus SC. Suicide risk among adults with mental health emergency department visits with and without suicidal symptoms. J Clin Psychiatry . 2021;82(6):20m13833. doi:10.4088/JCP.20m13833
- Garnett MF, Curtin SC. Suicide mortality in the United States, 2001-2021 . NCHS Data Brief, no. 464. April 2023. National Center for Health Statistics, Hyattsville, MD. https://www.cdc.gov/nchs/data/databriefs/db464.pdf
The inclusion of an innovation in PSNet does not constitute or imply an endorsement by the U.S. Department of Health and Human Services, the Agency for Healthcare Research and Quality, or of the submitter or developer of the innovation.
Contact the Innovator
ED-SAFE 1: Dr. Ivan Miller, [email protected]
ED-SAFE 2: Dr. Edwin Boudreaux, [email protected]
Perspective
Perspectives on Safety
Annual Perspective
WebM&M Cases
Pathologists, patients and diagnostic errors—part 1 and part 2. August 10, 2016
A simple surgery with harrowing complications. August 8, 2012
Amid lack of accountability for bias in maternity care, a California family seeks justice. August 16, 2023
Improving Patient Safety: The Intersection of Safety Culture, Clinician and Staff Support, and Patient Safety Organizations. October 28, 2015
Why doctors should own up to their medical mistakes. February 13, 2013
A Tale of Two Stories: Contrasting Views of Patient Safety. March 27, 2005
Patient Safety Primers
Discharge Planning and Transitions of Care
Evaluating independent double checks in the pediatric intensive care unit: a human factors engineering approach. February 21, 2024
Pediatric safety incidents from an intensive care reporting system. May 27, 2009
Medicines-related harm in the elderly post-hospital discharge. March 27, 2019
A Crisis in Health Care: A Call to Action on Physician Burnout. January 30, 2019
Near-miss event analysis enhances the barcode medication administration process. January 17, 2018
Diagnostic Error in Medicine. October 7, 2009
Simulation in Maternal Fetal Medicine. June 26, 2013
The 2013 John M. Eisenberg Patient Safety and Quality Awards. April 30, 2014
Iatrogenic Disease. March 5, 2008
Making Healthcare Safer III. March 18, 2020
Patient Safety and Quality. March 3, 2010
2011 John M. Eisenberg Patient Safety and Quality Awards. June 27, 2012
The Second Society for Simulation in Healthcare Research Summit: Beyond Our Boundaries. August 1, 2018
Risk, Safety and Reliability Special Issue. May 20, 2009
Quality and Safety Competencies in Nursing Education. January 13, 2010
Contributions from Ergonomics and Human Factors. November 17, 2010
Innovation in Perioperative Patient Safety. February 27, 2013
Making Health Care Safer: A Critical Review of Modern Evidence Supporting Strategies to Improve Patient Safety. March 6, 2013
The Science of Simulation in Healthcare: Defining and Developing Clinical Expertise. November 19, 2008
Special Issue: Progress at the Intersection of Patient Safety and Medical Liability. December 14, 2016
Patient Safety Papers 3. April 23, 2008
Quality and Safety in Medicine. December 9, 2009
Patient Safety. November 21, 2018
Special Issue on Health Information Technology. April 16, 2008
Patient Safety Papers 4. September 2, 2009
Patient Safety Innovations
Risk Mitigation Using the Anesthesia Risk Alert Program: Applying a Proactive Approach With Data Review & Collaborating With a Second Practitioner
Should dignity preservation be a precondition for safety and a design priority for healing in inpatient psychiatry spaces? April 10, 2024
Patient and Family Centered I-PASS (Family-Centered Communication Program to Reduce Medical Errors and Improve Family Experience and Communication Processes)
The e-autopsy/e-biopsy: a systematic chart review to increase safety and diagnostic accuracy innovation.
Clinicians' insights on emergency department boarding: an explanatory mixed methods study evaluating patient care and clinician well-being. August 23, 2023
Ambulatory Safety Nets to Reduce Missed and Delayed Diagnoses of Cancer
Remote response team and customized alert settings help improve management of sepsis, preventing falls through patient and family engagement to create customized prevention plans.
Effect of an emergency department process improvement package on suicide prevention: the ED-SAFE 2 cluster randomized clinical trial. May 31, 2023
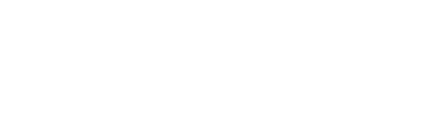
Connect With Us
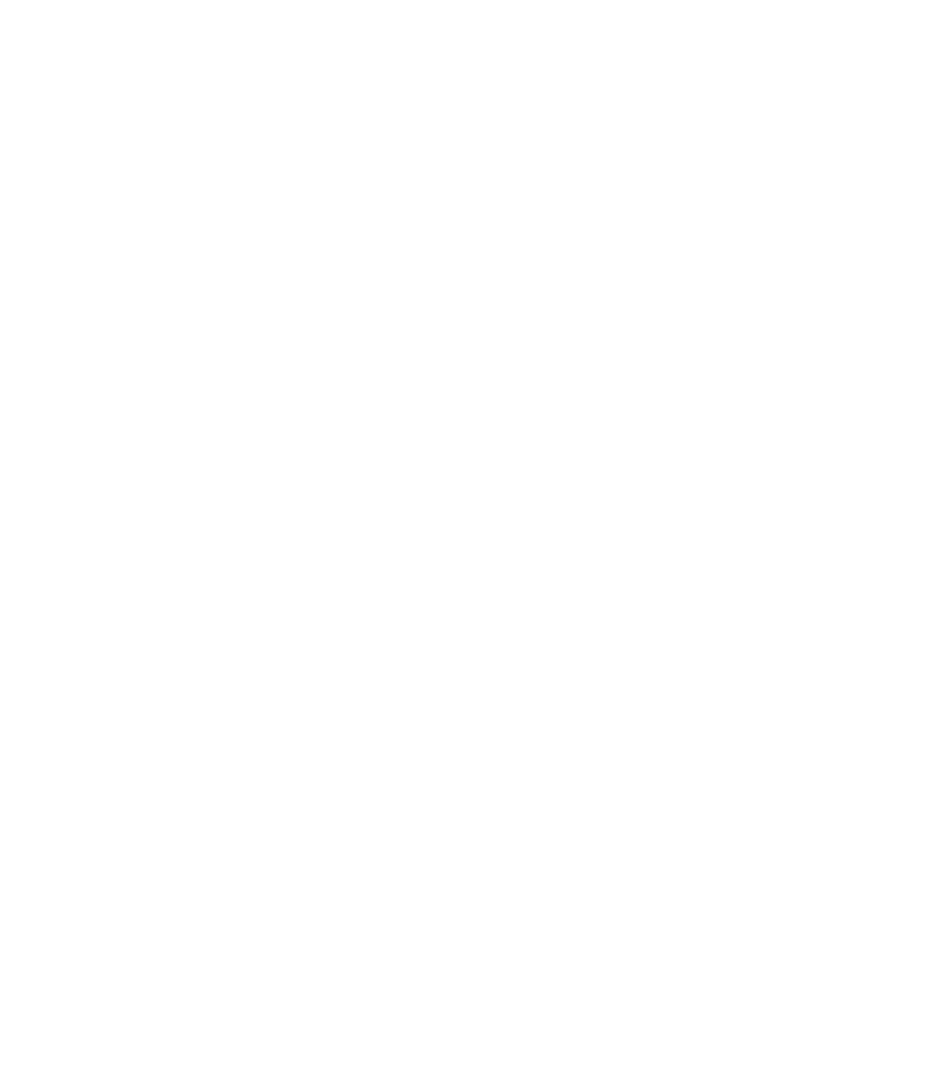
Sign up for Email Updates
To sign up for updates or to access your subscriber preferences, please enter your email address below.
Agency for Healthcare Research and Quality
5600 Fishers Lane Rockville, MD 20857 Telephone: (301) 427-1364
- Accessibility
- Disclaimers
- Electronic Policies
- HHS Digital Strategy
- HHS Nondiscrimination Notice
- Inspector General
- Plain Writing Act
- Privacy Policy
- Viewers & Players
- U.S. Department of Health & Human Services
- The White House
- Don't have an account? Sign up to PSNet
Submit Your Innovations
Please select your preferred way to submit an innovation.
Continue as a Guest
Track and save your innovation
in My Innovations
Edit your innovation as a draft
Continue Logged In
Please select your preferred way to submit an innovation. Note that even if you have an account, you can still choose to submit an innovation as a guest.
Continue logged in
New users to the psnet site.
Access to quizzes and start earning
CME, CEU, or Trainee Certification.
Get email alerts when new content
matching your topics of interest
in My Innovations.
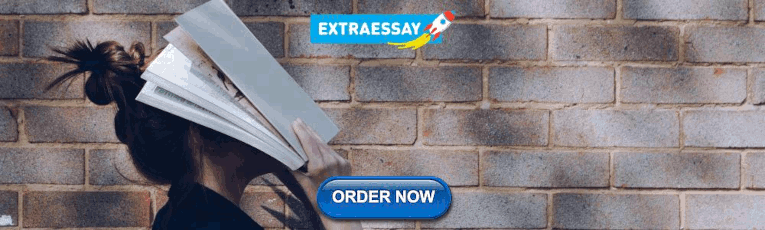
IMAGES
VIDEO
COMMENTS
Clinical Data Management (CDM) is a critical phase in clinical research, which leads to generation of high-quality, reliable, and statistically sound data from clinical trials. This helps to produce a drastic reduction in time from drug development to marketing. Team members of CDM are actively involved in all stages of clinical trial right ...
A data management plan, or DMP, is a formal document that outlines how data will be handled during and after a research project. Many funding agencies, especially government sources, require a DMP as part of their application processes. Even if you are not seeking funding for your research, documenting a plan for your research data is a best ...
7. Understand the reasons for performing research that is reproducible from data collection through publication of results. 9. Distinguish between variable types (e.g. continuous, binary, categorical) and understand the implications for selection of appropriate statistical methods. Extensively covered by required coursework.
Outlining a data management strategy prior to initiation of a research study plays an essential role in ensuring that both scientific integrity (i.e., data generated can accurately test the ...
A clinical trial management system (CTMS) is a type of project management software specific to clinical research and clinical data management. It allows for centralized planning, reporting, and tracking of all aspects of clinical trials, with the end goal of ensuring that the trials are efficient, compliant, and successful, whether across one or several institutions.
2. Provide a general explanation of data management planning and execution for a research study or clinical trial. 3. Describe the many data management responsibilities, the types of tools used to manage data activities, and the importance of documentation. 4.
There are 6 modules in this course. This course presents critical concepts and practical methods to support planning, collection, storage, and dissemination of data in clinical research. Understanding and implementing solid data management principles is critical for any scientific domain. Regardless of your current (or anticipated) role in the ...
Introduction to Clinical DataClinical data is either collected during patient care or as part of a clinical trial program. Funding agencies, publishers, and research communities are encouraging researchers to share data, while respecting Institutional Review Board (IRB) and federal restrictions against disclosing identifiers of human subjects.You should take initial steps to de-identify data for:
5.5.4 Clinical Data Management Plan. The design of the research data lifecycle should be strategized in the clinical data management plan (CDMP). The exact content of the CDMP will vary on the type of trial, the number of sites involved, and the sponsor's specifications. Among the recommended items to include are: Clinical data management ...
Data management plan (DMP) is a crucial document that outlines how clinical data will be collected, managed, analyzed, and shared during a clinical trial. DMP serves as a roadmap for clinical data ...
Compilation of necessary documentation in discipline-specific or functional plans is an approach to organizing and maintaining the essential documentation for a clinical study. A DMP comprehensively documents data and its handling from definition, collection and processing to final archival or disposal.
The Data Management Plan (DMP) has multiple purposes and is used to comprehensively document the collection and handling of the data. This should represent the accountability ... in clinical research (nih.gov) System Integrations System integrations being used for this study are listed below: • [Safety database (PV)
Data management is at the heart of the clinical research process. Although good data management practices cannot make up for poor study design, poor data management can render a perfectly executed trial useless. This book is about clinical research; the failure of a study to produce generalizable knowledge because of bad data management ...
Data Management Plan (Model) Template - version 06.10.2021 Author: Khaled Mostaguir Page 1 / 10 Data Management Plan (DMP) for clinical research projects A model for submissions to the Swiss National Science Foundation (FNS) and/or to Ethics Committees Important Notes:
Clinical data management (CDM) is a critical process in clinical research, which leads to generation of high-quality, reliable, and statistically sound data from clinical trials. Clinical data management ensures collection, integration and availability of data at appropriate quality and cost. It also supports the conduct, management and analysis of studies across the spectrum of clinical ...
Clinical Data Management (CDM) is a critical phase in clinical research, which leads to. generation of high-quality, reliable, and statistically sound data from clinical trials. This. helps to ...
The goal of the DSMP is to provide a general description of a plan that you intend to implement for data and safety monitoring. The DSMP should specify the following: A brief description of the study design. Primary and secondary outcome measures/endpoints. Sample size and target population.
Clinical Data Management is intended to deliver quality data that support the purpose of a study and find answers to the research question and the conclusion to hypotheses. CDM helps to obtain faster and better results with higher accuracy, as it allows the building of a valid, error-free and solid database. Data management in clinical studies ...
Through her 30-year career with ICON, Rose Kidd has watched the company and the clinical research industry evolve. Now her team provides the clinical data science, biostatistics, medical writing, pharmacovigilance, study start up, and site and patient solutions that sponsors need to improve their clinical trials.
The clinical research community understands the importance of data standardization [26-29], data quality [30-33], and data collection [28, 34-36] and has established good clinical data management practices (GCDMP) to ensure that CRDM is conducted at the highest level of excellence.
From January 25, 2023, the National Institutes of Health (NIH) will require all new proposals that involve the generation of scientific data to include a 2-page Data Management and Sharing Plan (DMSP or DMP). This information must be provided in a new "other Plan(s)" attachment field in the PHS 398 Research Plan form.
Monitors patient data & study-related information related to clinical study sites and clinical trial participation.. Ensures the investigator adheres to research protocols, regulatory requirements and good clinical practices and provides input into data validation plan. Provides timely and accurate monitoring of patient data and study-related information from source documents, research records ...
Summary. Suicide is the 12 th leading cause of death in the United States, and the 3 rd leading cause of death for people ages 15-24. 1 More than 4% of all emergency department visits are attributed to psychiatric conditions 2 and 3-8% of all patients have suicidal ideation when screened in the ED. 3 In addition, there are approximately 420,000 ED visits every year for intentional self-harm ...