- Translation
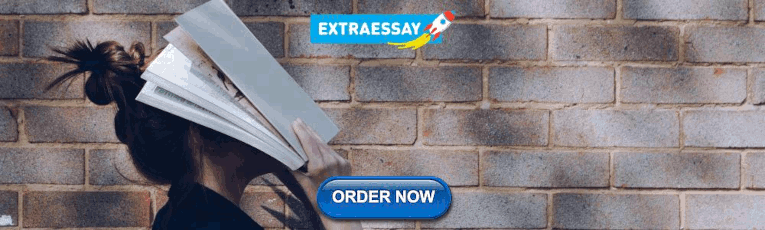
Novelty effect: How to ensure your research ideas are original and new
By charlesworth author services.
- Charlesworth Author Services
- 12 January, 2022
What has been is what will be, and what has been done is what will be done, and there is nothing new under the sun. — Ecclesiastes
Novelty can be described as the quality of being new, original or unusual . Novelty in scientific publishing is crucial, because journal editors and peer reviewers greatly prize novel research over and above confirmatory papers or research with negative results . After all, why give precious and limited journal space to something previously reported when authors submit novel, unreported discoveries?
How do you know what constitutes as novel? How can you as an academic author enhance the novelty effect with your research submissions ? Below we explore ideas that will help you maximise the novelty effect in your submissions.
a. New discovery
This comprises research on and reports of completely new discoveries. These can be new chemical elements, planets or other astrological phenomena, new species of flora or fauna, previously undiagnosed diseases, viruses etc. These are things never seen or reported before. Often such new discoveries serve as a seedbed for multiple reports or even completely new avenues of research. Journals prize submissions on new discoveries and often tout them in media reports.
b. The exceptionally rare
Not quite as exciting as new discoveries are reports on things not new, but seen or encountered exceptionally rarely, or not for a long time. An example is the sighting of the rare pink handfish, recently spotted in Australia for the first time in decades. In biomedical publishing , rare case reports of a near-unique condition (such as the separation of conjoined twins) are occasionally published and make the nightly news.
c. New theories
Typically, these papers provide substantial data which supports the novel thesis. Reports of new theories must have rigorous logic and need to stand on clear and well-documented foundations. They can’t be simple flights of theoretical fancy. As with new discoveries, new theories can spawn whole new branches of scientific inquiry.
d. New or significantly improved diagnostic/laboratory techniques
Reports on novel techniques don’t usually receive coverage from the mass media, but can often garner huge numbers of references if the new technique is adopted by the scientific community. Publication-worthy techniques include those which are more efficient, less time-consuming or more reliable than currently existing techniques or diagnostic procedures. Anything that is truly new or improves significantly on an established technique is potentially worthy of publication. In medicine, new surgical techniques are very important, but here’s a tip : try to provide a large prospective case series with long-term follow-up instead of a just a single case report.
e. Existing data combined into new knowledge
There is a profound novelty effect when researchers combine existing data/knowledge into something new. Ideas from disparate, previously unrelated fields of research can lead to completely novel discoveries with untold potential applications. Translational or applied research (particularly in the biomedical sciences) has borne abundant fruit over the last many decades. Translational applications of chemistry and physics to medicine have seen enormous advances in the diagnosis and treatment of numerous diseases.
f. Incremental additions to the literature
Not all research or publications will report on truly novel discoveries; in fact, very few will. But that doesn’t necessarily diminish the novelty effect of your work. The vast majority of published research adds incrementally to what is already known, nudging scientific knowledge forward. The accumulation of incremental discovery leads, over time, to large gains in understanding and knowledge.
How to ensure and verify the novelty effect
Whether your research reports something completely new or furthers an existing field in a new way, you need to make sure the contribution is indeed new.
- Do your homework : Pore through the literature (in as many languages as possible) to make sure your idea is indeed new, or significantly different enough to be considered new.
- To the degree possible, provide the ‘idea genealogy’ for your concept : Reference the major sources of those who have come before you. Through references and by describing your thought processes, describe clearly how you came up with the new idea or combination of ideas.
- Disclose your sources of inspiration and new application : Doing so constitutes academic honesty, gives credit to those upon whose shoulders your research rests and provides intellectual fertiliser for other scientists who may, in turn, be able to build upon your own ideas.
All the best for your (novel) submission!
Maximise your publication success with Charlesworth Author Services.
Charlesworth Author Services, a trusted brand supporting the world’s leading academic publishers, institutions and authors since 1928.
To know more about our services, visit: Our Services
Share with your colleagues
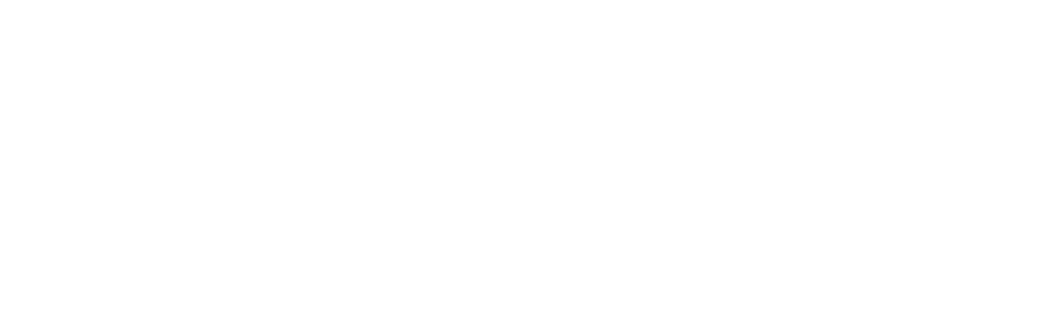
Scientific Editing Services
Sign up – stay updated.
We use cookies to offer you a personalized experience. By continuing to use this website, you consent to the use of cookies in accordance with our Cookie Policy.

An official website of the United States government
The .gov means it’s official. Federal government websites often end in .gov or .mil. Before sharing sensitive information, make sure you’re on a federal government site.
The site is secure. The https:// ensures that you are connecting to the official website and that any information you provide is encrypted and transmitted securely.
- Publications
- Account settings
Preview improvements coming to the PMC website in October 2024. Learn More or Try it out now .
- Advanced Search
- Journal List

Measuring novelty in science with word embedding
Sotaro shibayama.
1 School of Economics and Management, Lund University, Lund, Sweden
2 Institute for Future Initiative, The University of Tokyo, Tokyo, Japan
3 National Institute of Science and Technology Policy, Tokyo, Japan
4 School of Economics and Management, Harbin Institute of Technology, Shenzhen, China
5 World Intellectual Property Organization, Geneva, Switzerland
Kuniko Matsumoto
Associated data.
All relevant data are within the paper and its Supporting Information files .
Novelty is a core value in science, and a reliable measurement of novelty is crucial. This study proposes a new approach of measuring the novelty of scientific articles based on both citation data and text data. The proposed approach considers an article to be novel if it cites a combination of semantically distant references. To this end, we first assign a word embedding –a vector representation of each vocabulary–to each cited reference on the basis of text information included in the reference. With these vectors, a distance between every pair of references is computed. Finally, the novelty of a focal document is evaluated by summarizing the distances between all references. The approach draws on limited text information (the titles of references) and publicly shared library for word embeddings, which minimizes the requirement of data access and computational cost. We share the code, with which one can compute the novelty score of a document of interest only by having the focal document’s reference list. We validate the proposed measure through three exercises. First, we confirm that word embeddings can be used to quantify semantic distances between documents by comparing with an established bibliometric distance measure. Second, we confirm the criterion-related validity of the proposed novelty measure with self-reported novelty scores collected from a questionnaire survey. Finally, as novelty is known to be correlated with future citation impact, we confirm that the proposed measure can predict future citation.
Introduction
Novelty constitutes a core value in science, as new discoveries shape the basis of scientific advancement [ 1 , 2 ] and has broader impact on technological innovation [ 3 ]. Accordingly, novelty serves as a key criterion for the evaluation of scientific output as well as decision makings such as funding allocation, employment, and scientific awards [ 1 , 4 – 6 ]. It is therefore critical that scientific novelty can be reliably measured. In practice, novelty is usually assessed through peer review on a small scale [ 7 ], while evaluating novelty on a larger scale remains to be a challenge. Though recent bibliometric techniques have enabled us to measure various qualities of scientific discoveries, including novelty [ 8 – 11 ], the validity and practical utility of the extant measures are debatable [ 12 , 13 ].
Previous bibliometric measures for the novelty of scientific documents draw on roughly two data sources, either citation data or text data. Text data are of obvious use, in that once a scientific discovery is documented, its novelty should be revealed in text information. Nonetheless, due to the ambiguity and complexity of natural languages, previous measures use text data rather superficially without sufficiently exploiting the semantic information [e.g., 14 ]. It is relatively recently that such semantic information got extracted from text data and translated into bibliometric indices [e.g., 15 ]. To circumvent the technical challenges in extracting semantic information from text data, citation data have been extensively utilized in previous novelty measures. As a citation represents information flow from a cited document to a citing document, it can be used to infer certain qualities, including novelty, of a document without scrutinizing the content [ 10 , 16 ]. However, the validity of this approach has been occasionally questioned [ 12 ]. In fact, insufficient validation has been a limitation common to most novelty measures [ 17 ]. Furthermore, a practical limitation common to previous measures is that they require access to a large-scale bibliometric database (often the whole universe of scientific documents), which are usually proprietary and expensive, and high computational power, which potential users of the measures do not always have.
To address previous limitations, we propose a new approach to compute the novelty of scientific documents by combining both citation and text data (see Fig 1 ). Our approach features recombinant novelty [ 18 – 21 ], considering a document to be novel if it cites a combination of semantically distant documents. This is in line with the previous measures based on citation data [e.g., 8 ]. Unlike previous measures, however, we use text data to quantify the distances between cited documents. Specifically, based on the text information included in cited documents, we map each document to a word embedding –a high-dimensional vector assigned to each vocabulary [ 22 ]–with which to compute distances between cited documents. To the best of our knowledge, this is the first to use the word-embedding technique to measure the novelty of scientific documents.

For text information, we test three sources–the abstract, keywords, and the title of cited documents–finding all satisfactory performance. Of the three sources, titles of cited documents are often included in the focal document itself, and the burden of data access is minimized. As a library of word embeddings, we draw on scispaCy [ 23 ], which is publicly available and thus significantly reduces the computational cost. We publicly share the code [ 24 ], with which one can compute the novelty score of a document only with the focal document’s reference list.
We validate the proposed measure in three exercises. First, we confirm that word embeddings from the selected library can be used to quantify semantic distances between documents by comparing with an established bibliometric distance measure. Second, we test the criterion-related validity of the proposed novelty measure based on self-reported novelty scores collected from a questionnaire survey. Third, as novelty is known to be a predictor of future citation impact [ 8 , 11 ], we test whether the proposed measure is correlated with future citation.
This paper is structured as follows. In the next section, we categorize previous novelty measures and discuss their characteristics and limitations. The following section describes our proposed measure and outlines its operationalization. Then, we present the methods and data for the validation exercises. Finally, we present the results and conclude.
Literature review
Previous bibliometric measures for novelty can be categorized based on their conceptualization and operationalization ( Table 1 ). Conceptually, some measures aim to represent the uniqueness of a certain knowledge element (Groups 1 and 4)–for example, a discovery of a new molecule and development of a new material. In contrast, other measures aim to capture a recombination of knowledge elements (Groups 2 and 3), in which a new or rare combination of knowledge is considered to be a sign of novelty. The notion of recombination as a source of novelty has been widely discussed in the literature. The creativity literature argues that associating remote elements is a path to creative solution in general as well as in science [ 18 , 19 ], and the management literature suggests that combining components is a major route to technological innovation [ 20 , 21 ].
Group | Description | Concept | Data | ||
---|---|---|---|---|---|
Recombination | Uniqueness | Citation | Text | ||
1 | A new word [14, 25 , 26 ] | X | X | ||
2 | A new combination of words [9, 25 , 27 ] | X | X | ||
3 | A new combination of cited references [8, 10, 11, 16 , 17] | X | X | ||
4 | A distant text [15 ] | X | X |
*patent measures
For operationalization, a group of measures exploits citation information to assess novelty indirectly (Group 3), and the other draws on text analysis to assess the content of documents (Groups 1, 2, and 4). Among the latter, the majority uses text information only superficially without using the semantic information of the text (Groups 1 and 2), but recent measures attempt to extract semantic information (Group 4). Studies on novelty measures have been relatively advanced in technology management, in which a patent is used as a unit of document [e.g., 16 , 25 ]. We also refer to these measures because the key idea behind the measures is applicable to scientific documents. In what follows, we discuss four groups of previous measures.
(1) A new word
The first group of novelty measures is based on the first appearance of a word(s) that appears in a document [ 14 , 25 ]. If a document includes or is associated with a certain word or a sequence of words that is new to the world, it can be inferred that the document delivers novel information. For example, if a document contains a previously unknown chemical compound, it suggests that the document is novel. In this category, Azoulay et al . [ 14 ] drew on Medical Subject Heading (MeSH), a controlled keyword dictionary, and operationalized the novelty of a journal article based on the average age of keywords (the number of years since its first appearance). Balsmeier et al . [ 26 ] and Arts et al . [ 25 ] also identified novel inventions based on the first occurrence of a word as well as a sequence of words (bigram and trigram) in patent documents.
(2) Recombination of words
The second group is technically similar to the first group but conceptually different as it is to measure "recombinant" novelty [ 19 , 20 ]. When a document includes a rare combination of knowledge elements, even if each element has been known, the document can be considered to be novel. In this category, Boudreau et al . [ 9 ] measured the novelty of a research grant proposal based on a new combination of MeSH keywords. Similarly, drawing on a controlled dictionary of patent classifications, Verhoeven et al . [ 27 ] measured recombinant novelty by a new combination of IPC codes assigned to the patent. Arts et al . [ 25 ] also measured the novelty of a patent based on a new combination of two words that appeared in the patent.
The first and second groups are intuitively straightforward but have some limitations. Among others, these measures largely disregard semantic information included in text data. For example, the first group may consider a new synonym of an existing concept to be novel, unless controlled dictionaries are available. Similarly, the second group may consider any recombination equally novel regardless of the semantic distance between combined elements.
(3) Recombination of cited documents
The third group also measures recombinant novelty, but instead of using text information, it draws on citation information. A document citing another document implies that knowledge in the latter is used by the former [ 28 ]. Thus, a document can be characterized by its cited documents, by considering each of cited documents to be a knowledge element that is incorporated into the citing document. Based on the recombinant novelty concept [ 18 , 19 ], a document citing a set of documents that have rarely been cited together can be considered as a sign of novelty. In contrast to the first and second groups, in which a single word is considered a representation of knowledge, considering a cited document as a knowledge element adds semantic richness, making this approach popular in previous studies.
In this group, Dahlin and Behrens [ 16 ] proposed a novelty measure of patents based on a rare combination of cited references. Trapido [ 10 ] applied the same approach to journal articles, specifically in the field of electrical engineering. This approach is extended by Matsumoto et al . [ 17 ] so that it is applicable in any scientific field. A variation of this approach is to draw on journals in which cited documents are published [ 8 , 11 ]. That is, if a focal document cites documents in two journals that have rarely been cited together, it is considered as a sign of novelty. This approach thus consolidates the unit of knowledge further at the journal level. Though considering a document or a journal as a unit of knowledge, without needing to scrutinize the content of documents, is convenient, its validity is under dispute [ 12 , 13 ].
(4) A distant text
The last group quantifies the uniqueness of a document based on text analysis, and relies on more recent development of natural language processing (NLP) to extract semantic information. In particular, drawing on the word embedding technique, Hain et al . [ 15 ] proposed a measure of patent novelty. Word embeddings map each word to a high-dimensional vector (i.e., a list of numbers). It allows us to quantify a semantic relationship between a pair of words by calculating the distance between the vectors–i.e., similar words have close vectors while dissimilar words have remote vectors. Hain et al . [ 15 ] assigned a vector to each patent by aggregating the vectors for a set of words that appear in the patent. Then, they calculated a distance between every pair of patents, with which a patent remote from any other patent is considered to be novel.
Proposed measure of novelty
Measuring novelty with word embedding.
As a new approach, we propose to measure recombinant novelty of scientific documents by applying the combination of the word embedding technique and citation analysis. We consider a cited document as an appropriate unit of knowledge input, as in Group 3. Unlike the previous measures, which disregard the content of cited documents, we draw on the word embedding technique to extract semantic information in cited documents.
The word embedding technique often draws on machine learning algorithms (e.g., word2vec) to calculate a vector representation for each word based on the co-occurrences of words in a text corpus [ 22 ]. The approach is gaining confidence as the performance of machine learning has been improving, and has been recently applied to scientific documents for various purposes. For example, Tshitoyan et al . [ 29 ] captures the knowledge structure in the extant literature in material sciences with which they predict future scientific discoveries in the field. Still, to the best of our knowledge, the technique has not been used to measure the novelty of scientific documents.
Although computing word embeddings is demanding, some algorithms are publicly available, and some well-trained word embedding models (a list of vectors for a set of vocabularies) are also publicly accessible [ 30 ]. In this study, we use scispaCy as an established and publicly available library of word embeddings. ScispaCy builds on a popular spaCy model [ 30 ] and offers vector representations in a 200-dimensional vector space for 600,000 vocabularies specializing in biomedical texts [ 23 , 31 ].
Operationalization
With the selected word embedding library and citation information, the novelty of a document is computed through the following steps ( Fig 1 ). Suppose that a focal document cites N references, and that each of the cited references has some text information. One can use various sources of text information, such as the full text and the abstract. In the following analysis, we construct respective measures from three text sources: the abstract, keywords, and the title of cited documents. Of the three sources, we intend to propose primarily using the title to minimize data requirement and maximize the utility of the measure.
Step 1. First, we vectorize the text information of the i -th reference as v i ∈ℝ 200 ( i ∈{1,…, N }). Since the text information includes multiple words, v i is calculated as the mean of word embeddings of all words included.
Step 2. Second, we compute the distance of each pair of cited documents. The cosine distance between i -th and j -th references (1≤ i < j ≤ N ) is given by:
The cosine distance ranges from 0 to 2, where a larger value indicates a larger distance.
Step 3. Finally, we aggregate the distance scores over all pairs of cited references. In our dataset, one document has 32 cited references on average, which gives approximately 500 reference pairs. As a novelty measure of a focal document, we take the q -percentile value of the distance scores ( Novel q ), where q ∈[0,100] and the 100-percentile value is defined as the maximum. Hence,
where R ( d ij ) is the ordinal rank of d ij of all the distances of N ( N −1)/2 reference pairs.
Computational cost
The aforementioned previous measures of novelty require extensive data access and processing. Text-based approaches ( Table 1 , Groups 1, 2, and 4) require the entire history of word uses, and citation-based approaches ( Table 1 , Group 3) need comprehensive citation network data. This poses two practical challenges for potential users of the novelty measures. First, the required data are usually proprietary, and thus, literally expensive. Second, processing the massive data takes high computational power. Not all users have such rich resources, compromising the utility of the measures.
Our proposed approach addresses these issues and aims to allow anyone to compute and use the novelty measures. Our measure requires only limited data access and little need for proprietary data. The measure can be computed only with the titles of a focal document’s cited references, which is often included in the focal document itself, and a publicly available library of word embeddings. The approach requires only small data processing. Unlike previous measures, our approach does not require extensive citation network analysis unlike Group 3, nor comparison with the whole document universe unlike Group 4. With the publicly shared code, anyone can compute the measure.
Methods and data
Previous novelty measures have been rarely validated with a few exceptions [ 17 ]. To confirm the validity of our proposed measure, we carry out three exercises. The primary analysis is to test the criterion-related validity based on self-reported novelty scores for selected documents. As a preparatory step to this main analysis, we test whether scispaCy word embeddings can be indeed used to measure distances between documents (corresponding to Step 2). Finally, since novelty is known as a predictor of future citation impact [ 8 , 11 ], we run regression analyses to test whether our proposed measure is positively associated with future citation.
To compute the proposed measures, we downloaded bibliometric information from Web of science (WoS). Since scispaCy specializes in the vocabularies in biomedicine, we focus on documents within relevant Subject Categories [ 32 ]. We focus on "article" as a document type and documents written in "English" [ 33 ]. We employ different sets of random samples for each analysis as detailed below.
Validation of distance
Before validating the novelty measure itself, we test if scispaCy word embeddings convey semantic information of a text, and that they can assess the distance between a pair of documents. To this end, we compute distances of pairs of documents in two approaches–one based on scispaCy word embeddings and the other with a previously established approach–and confirm that the two are sufficiently correlated.
As a previously established approach, we compute the co-citation distance between a pair of documents i and j :
where ref i is the number of references cited by i and coref ij is the number of references cited by both i and j . Co-citation distance has been previously used to measure the distance of scientific documents without a need to look into the content of the documents [ 10 , 17 ]. A basic assumption is that a pair of documents should include a similar content if they cite a similar set of documents. We do not consider that the co-citation distance is superior to the word-embedding distance, but the two distances are expected to be correlated if scispaCy word embeddings do convey semantic information.
Second, using scispaCy word embeddings, we assign vectors respectively to the same pair of documents i and j (see Step 1 in Fig 1 ) and compute their distance ( Eq 1 ). As text data for vectorization, we draw respectively on three sources (the title, the abstract, and keywords) from the pair of documents, preparing three distance measures ( d i j T , d i j A , and d i j K ). Note that the word-embedding distance between a pair of focal documents is computed in this analysis, and this is applied to pairs of references cited by focal documents when we compute novelty.
For this analysis, we employed the following sampling strategy. First, we randomly sampled 100 authors in the field of biomedicine. Then, we collected all documents authored by these authors [ 34 ]. Finally, we filtered out documents outside of the biomedical field as well as documents missing reference information, resulting in 1,600 documents (16 documents per author on average). We compute the distance measures between documents written by the same author (i.e., we do not compare documents written by different authors). This is because co-citation is rare between a randomly chosen pair of documents written by different authors, which spuriously inflates the correlation.
Validation of novelty
After confirming that the scispaCy word embeddings carry semantic information of text, we test the criterion-related validity of the proposed novelty measure ( Eq 2 ). To this end, we draw on self-reported novelty scores, which we obtained from a questionnaire survey we conducted in 2009–2010 [ 35 , 36 ]. The survey was responded by 2,081 scientists from various scientific fields, of whom this study draws on a subset of 321 respondents in biomedical fields.
The survey included a wide range of questionnaire items, one section of which asked the respondents to assess a randomly selected journal article that they published in 2001–2006. This section includes eight items to characterize the finding reported in the article ( Table 2 ). As novelty is a multifaceted concept [ 37 ], the survey incorporated four aspects (theory, phenomenon, method, and material) in which the article may make scientific contribution. For each aspect, the survey further included two items, one indicating newness and the other indicating improvement over existing literature. We expect that the proposed measure should be correlated more with the newness items but less with the improvement items. Each item was responded in a 5-point scale (1: not relevant at all—5: highly relevant).
Aspect | New vs. Improvement | Questionnaire item |
---|---|---|
Theory / Hypothesis | New | (1) Developing a new hypothesis or theory |
Improvement | (2) Supporting or rejecting an existing hypothesis or theory | |
Phenomenon | New | (3) Discovering an unknown phenomenon or material |
Improvement | (4) Understanding a phenomenon | |
Method | New | (5) Developing a new research method |
Improvement | (6) Improving an existing research method | |
Material / Function / Mechanism | New | (7) Creating a new function, mechanism, or material |
Improvement | (8) Improving on an existing function, mechanism, or material |
Note. Responded in a 5-point scale (1: not relevant at all—5: highly relevant).
For the selected articles, we computed the proposed novelty measures ( Eq 2 ), based on the title, the abstract, and keywords respectively, which generates three series of novelty measures ( N o v e l q T , N o v e l q A , and N o v e l q K ) where q ∈{100, 99, 95, 90, 80, 50}.
Prediction of future citation
Previous studies consistently indicate a positive association between novelty and future citation impact of scientific documents [ 8 , 11 ]. Thus, we test whether the proposed novelty measure can predict future citation effectively. For this analysis, we use "top-1% cited" ( TC ) in the respective field as the dependent variable and regress it on the proposed novelty measures. TC is a dummy variable coded 1 if the citation count of the article is within top 1% and 0 otherwise. Three sets of novelty measures are calculated with the title, the abstract, and keywords respectively ( N o v e l q T , N o v e l q A , and N o v e l q K ) where q ∈{100, 99, 95, 90, 80, 50}. Since the dependent variable is a dummy variable, we draw on logistic regressions:
where f is the logistic function.
For this analysis, we randomly sampled 2,000 articles published in biomedicine fields in 2010, and evaluated their citation impact as of 2020 (10 years after publication). We oversampled top-1% cited articles, so that the final sample consists of approximately 1,000 top-1% cited articles and 1,000 non-top-1% cited articles.
Description of the measure
To illustrate the distribution of the proposed measures, we computed the novelty of randomly selected documents ( Fig 2B ) and the distances of cited references of the documents ( Fig 2A ). Comparing distances based on three text data sources, Fig 2A shows that the abstract-based measure ( d i j A ) takes lower values. This is because abstracts include longer text information, which increases the chance that two cited documents share something in common. Based on the distances, novelty measures ( Novel q ) with various q ’s are computed (see S1 Appendix ). Fig 2B presents Novel 100 , which takes the maximum value of all reference pairs.

The same sample for the third validation study (prediction of future citation) is used, except that oversampled highly-cited documents are excluded. The 947 selected documents include in total approximately 230,000 combinations of cited references, for which the distance ( Eq 1 ) is computed (A). The distances are summarized at the focal document level ( Eq 2 ), and Novel 100 is displayed as an example (B). Novelty measures with different q values are illustrated in S1 Appendix . Since abstracts and keywords are not available for all documents, the sample sizes are smaller.
Table 3 presents the result of the validation of the distance measures. The sampled 1,600 articles authored by 100 authors yield 21,908 article pairs to compute the distances for. As the abstract and author keywords are not always available, the sample sizes are smaller for the analyses of the distances based on abstracts ( d i j A ) and keywords ( d i j K ).
Co-citation ( ) | Title ( ) | Abstract ( ) | |
Title ( ) | .231 (21,908) | ||
Abstract ( ) | .310 (16,706) | .337 (16,706) | |
Keyword ( ) | .318 (8,781) | .450 (8,781) | .407 (8,481) |
) and title distance ( ) by title length | |||
Title word count | Correlation coefficient | ||
1–10 | .440 (1,395) | ||
11–13 | .246 (1,151) | ||
14–17 | .197 (1,827) | ||
18- | .259 (1,867) |
Note. Pearson’s correlation coefficient (the number of observations in parentheses).
***p<0.001. (B) Subsamples of document pairs are selected based on the title word count of both paired documents.
Table 3A shows that three word-embedding distances ( d i j T , d i j A , and d i j K ) are all strongly positively correlated with the co-citation distance ( d i j C ). Given that the co-citation distance is an accepted measure for the distance of scientific documents, this result supports our idea that scispaCy word embeddings can be used as a basis of novelty measurement. Compared to the title distance ( d i j T ), the abstract distance ( d i j A ) and the keyword distance ( d i j A ) indicate greater correlations with the co-citation distance ( d i j C ). Still, the title distance ( d i j T ) has a strongly significant correlation.
As we propose to use titles as the main source of text data for practical utility, we further examine the validity of the title distance ( d i j T ). We anticipated that short titles may carry insufficient semantic information and may not allow us to compute the distance reliably. We thus carry out correlation analyses with documents with different title lengths (word counts). Table 3B shows that the title distance ( d i j T ) is significantly correlated with the co-citation distance ( d i j C ) regardless of the word count. In fact, the correlation is strongest when the title length is shortest (10 words or shorter), contrary to our expectation. Thus, longer titles might bring more noise than information.
Table 4 reports the correlation between the series of the proposed bibliometric measures (on the vertical axis) and the self-reported questionnaire scores (on the horizontal axis). On top of the eight scores from the questionnaire, we added two summary scores by taking the mean of the four newness scores (Column 9) and the mean of the four improvement scores (Column 10) respectively. We expect that our proposed measure should be correlated with the newness scores (Columns 1, 3, 5, 7, and 9) rather than the improvement scores (Columns 2, 4, 6, 8, and 10). Focusing on the newness summary score (Column 9), Fig 3 illustrates the correlation coefficients with novelty measures from three different text sources and with different q values.

Pearson’s correlation coefficient. Novel q ( q ∈{100,99,95,90,80,50}) is correlated with the mean of four self-reported newness scores (Column 9 in Table 4 ). † p<0.1, *p<0.05, **p<0.01, ***p<0.001.
Self-reported measure | ||||||||||||||||||||||
---|---|---|---|---|---|---|---|---|---|---|---|---|---|---|---|---|---|---|---|---|---|---|
Theory | Phenomenon | Method | Material | Summary | ||||||||||||||||||
(1) New | (2) Impr. | (3) New | (4) Impr. | (5) New | (6) Impr. | (7) New | (8) Impr. | (9) New | (10) Impr. | |||||||||||||
Bibliometric measures | Title ( ) | = 100 | .126 | -.012 | .137 | .066 | .038 | -.002 | .187 | .014 | .170 | .024 | ||||||||||
= 99 | .096 | -.021 | .097 | .034 | .017 | -.015 | .173 | .016 | .135 | .004 | ||||||||||||
= 95 | .086 | -.038 | .076 | .031 | .021 | -.009 | .174 | .021 | .125 | .002 | ||||||||||||
= 90 | .073 | -.065 | .060 | .030 | .028 | -.010 | .147 | .004 | .108 | -.016 | ||||||||||||
= 80 | .066 | -.079 | .048 | .027 | .022 | -.017 | .127 | -.003 | .092 | -.027 | ||||||||||||
= 50 | .051 | -.082 | .027 | .024 | .008 | -.072 | .098 | -.019 | .065 | -.057 | ||||||||||||
Abstract ( ) | = 100 | .159 | -.049 | .130 | .067 | .038 | -.034 | .212 | -.038 | .188 | -.022 | |||||||||||
= 99 | .131 | -.074 | .085 | .040 | .017 | -.041 | .184 | -.043 | .146 | -.045 | ||||||||||||
= 95 | .109 | -.094 | .058 | .021 | -.007 | -.051 | .169 | -.050 | .116 | -.066 | ||||||||||||
= 90 | .108 | -.116 | .059 | .042 | -.002 | -.053 | .173 | -.066 | .119 | -.074 | ||||||||||||
= 80 | .080 | -.145 | .053 | .039 | -.043 | -.077 | .142 | -.089 | .083 | -.104 | ||||||||||||
= 50 | .019 | -.162 | .079 | .041 | -.074 | -.104 | .119 | -.088 | .055 | -.120 | ||||||||||||
Keyword ( ) | = 100 | .186 | ** | .068 | .144 | .136 | .065 | -.030 | .129 | .022 | .180 | .070 | ||||||||||
= 99 | .188 | .059 | .137 | .139 | .057 | -.032 | .125 | .024 | .175 | .068 | ||||||||||||
= 95 | .183 | .043 | .124 | .128 | .056 | -.041 | .118 | .027 | .165 | .055 | ||||||||||||
= 90 | .198 | .022 | .112 | .117 | .051 | -.044 | .103 | .011 | .158 | .036 | ||||||||||||
= 80 | .194 | .009 | .123 | .087 | .072 | -.043 | .104 | .006 | .168 | .020 | ||||||||||||
= 50 | .180 | -.019 | .164 | .087 | .118 | -.025 | .120 | .020 | .200 | .022 |
Note. Pearson’s correlation coefficient.
† p<0.1
*p<0.05
**p<0.01
***p<0.001. N = 321 (Title), 318 (Abstract), and 293 (Keyword).
The result presents a few findings mostly consistent with our expectation. First, Column 9 shows significant correlations between the proposed measures and the self-reported newness score, while Column 10 shows insignificant or negatively significant correlations with the self-reported improvement score. This suggests that our proposed approach does measure the newness of a scientific document and can distinguish novel discoveries from mere improvements. Second, comparing different q values, the result shows more positive correlation coefficients for the title and abstract measures ( N o v e l q T and N o v e l q A ) with greater q ’s. This suggests that a small number of distant recombination (even a single new combination), rather than many recombinations, is sufficient for a document to be novel. Interestingly, however, correlation coefficients for the keyword-based measures ( N o v e l q K ) are rather constant over a range of q values. Third, comparing the three sources of text information, the result overall shows somewhat larger correlation coefficients for the keyword-based measures ( N o v e l q K ) than for the abstract-based ( N o v e l q A ) and the title-based measures ( N o v e l q T ). Nonetheless, the difference is not substantial when we focus on the measures with the highest q ( Novel 100 ). The title-based novelty ( N o v e l 100 T ), which has the smallest correlation, is still strongly correlated with the self-reported newness summary score ( r = .170, p < .01). Finally, looking into different aspects of newness and improvement (Columns 1–8), the result shows that newness in terms of theory, phenomenon, and material (Columns 1, 3, and 7) are correlated with the proposed measures but newness in terms of method (Column 5) is not. This may be attributed to a specificity of the biomedical field and needs further investigation.
Table 5 reports the result of logistic regressions to test if our proposed novelty measures predict future citation impact. It presents the odds ratios that a document falls within the top one percentile of citation counts. For example, the odds of a document with N o v e l 100 T = 1 to be in the top one percentile is 154 times the odds of a document with N o v e l 100 T = 0 . Overall, the result shows significantly positive correlations between most variations of the novelty measures and citation impact, supporting the construct validity of our novelty measures.
100 | 154.02 | 27.89 | 5.91 | |||
99 | 53.26 | 7.98 | 4.42 | |||
95 | 29.27 | 3.17 | 3.46 | |||
90 | 20.30 | 2.26 | 2.89 | |||
80 | 14.97 | 1.04 | 2.04 | |||
50 | 5.87 | 0.08 | 1.31 | |||
N | 1,921 | 1,903 | 1,814 |
Note. Logistic regressions. Two-tailed test.
***p<0.001. The sampling weight is incorporated in the regression analysis.
The result also shows that the measures with greater q ’s (e.g., Novel 100 ) have higher odds ratios with greater statistical significance. This suggests that documents with a small number of distant recombination (even a single new combination) is sufficient to attract citations. Further to compare different text sources, Fig 4A graphically illustrates the regression result for Novel 100 . The graph shows that the title-based measure ( N o v e l 100 T ) has steeper curves than those based on abstracts ( N o v e l 100 A ) and keywords ( N o v e l 100 K ), thus best distinguishing highly-cited documents from less cited documents.

The probability of a focal document falling within the top 1 percentile is predicted. For easier interpretation and comparison, the horizontal axis takes the percentile of the novelty measures. (A) based on Row 1 in Table 5 . (B) and (C) based on curvilinear models incorporating the quadratic term of the novelty measures ( S1 Appendix ).
Since previous studies occasionally reported that novelty and citation impact have an inverted-U shaped relationship [ 38 ], we regress the citation impact on the quadratic term of the novelty measures on top of the linear term:
We find that adding the quadratic term increases a model fit for the novelty measures with smaller q ’s. Fig 4B and 4C illustrate the curvilinear associations for Novel 90 and Novel 50 , showing that the optimal level of novelty scores decreases for lower q ’s. This also suggests that a document with too many recombinations does not attract citation.
Alternative measure of recombination within a document
Although the proposed measure utilizes recombination between cited documents, it is plausible to find recombination within a focal document itself. By decomposing the text information (the title, the abstract, or keywords) of a focal document into words, assigning word embeddings to them, and measuring the distance of every pair of words, we additionally constructed similar sets of novelty measures. This is in line with a category of previous measures [ 25 ] except that we use word embeddings to compute word distances.
We tested the validity of this additional set of measures for the correlation with self-reported novelty as well as for the prediction of future citation ( S1 Appendix ). The result is overall unsatisfactory. Correlations with the self-reported scores are mostly insignificant and sometimes negatively significant. Similarly, correlations with future citation impact are insignificant or negatively significant. Thus, the proposed approach to quantify recombinant novelty does not work with the text information within a focal document itself. This contrasts with the previous measures of recombination within a document [ 9 , 25 ], which may be attributable to a different operationalization that the previous measures are based on the first appearance of a combined use of two words rather than their distance.
Discussion and conclusion
Novelty is a core value in science [ 1 , 2 ], and thus, a reliable approach to measure the novelty of scientific documents in a large scale is crucial. This study is the first to propose measuring the recombinant novelty of scientific documents based on the word-embedding technique. Most previous measures for recombinant novelty in science have been based solely on citation data [ 8 , 10 , 11 , 16 , 17 ]. Although citation network data is an effective tool to indirectly retrieve semantic information, recent advancement in text analysis allows us to extract it more directly and possibly more accurately [ 39 , 40 ]. Combining citation data and text data, we provide a well-validated and user-friendly measure of scientific novelty.
One limitation common to most previous measures is insufficient validation [ 17 ]. To address this issue, we investigated our proposed measure from multiple angles. First, we show that the word embeddings, with which the novelty measure is computed, can be used to gauge the distance between scientific documents. Second, the novelty measures are significantly positively correlated with self-reported scores for various dimensions of newness but not with those for improvement, suggesting that the proposed measure can distinguish novel discoveries from mere improvements. Third, the novelty measure is found to be a significant predictor of citation impact in 10 years. Overall, these results confirm the validity of the proposed measure.
We examined several variations of novelty measures. First, we tested different percentile values ( q ) in aggregating the distance scores across all pairs of cited references. The result shows greater performance with higher q ’s both in the correlation with self-reported novelty measures and in the prediction of future citation. Thus, the novelty of scientific documents is determined by a small number of distant recombination. This contrasts with the previous recombinant novelty measures based on more average distances [ 9 ].
Second, we use three different sources of text data, the abstract, keywords, and the title of cited references, to which the word-embedding technique is applied. The three text sources have different advantages. Abstracts offer rich information and keywords may be beneficial for conciseness, while titles are easiest to access. Based on the validation exercises, we find that the abstract-based measure ( N o v e l 100 A ), if we focus on the highest q = 100, demonstrates slightly higher performance in the correlation with the self-reported novelty scores, though the difference is only marginal. In the prediction of future citation, the title-based measure ( N o v e l 100 T ) presents highest performance. Overall, we recommend the title-based measure for data accessibility and reasonable validation results.
Another limitation common to previous measures is their computational cost for expensive data access as well as processing of massive data. Many potential users of the novelty measure cannot afford to it, which has substantially compromised the utility of the measures and delayed the progress of studies on scientific novelty. Our proposed approach overcomes these challenges. Drawing on limited text information (titles of cited references) and publicly shared library of word embeddings (scispaCy), our approach minimizes data access requirement as well as computational cost. Using the shared code, one can compute the novelty score of a document of interest only with the reference list of the document. Thus, we encourage the application of the approach for various purposes.
The approach has two limitations that future work needs to address. First, it depends on publicly available word-embedding libraries. ScispaCy specializes in biomedicine. Similar libraries are available in some fields but not in others, in which one needs to start with computing word embeddings. When a different library is used, the external validity of our approach needs to be tested. Second, we disregard the time dependency of word embeddings. The semantic distances between words change over time. Iterated computation of word embeddings may be required, for example, when novelty scores across different time points are to be compared.
Supporting information
S1 appendix, funding statement.
S.S. received a research grant from Lars Erik Lundberg Foundation ( https://www.lundbergsstiftelserna.se ) and Japan Society for the Promotion of Science (19K01830, https://www.jsps.go.jp/english/index.html ). The funder had no role in study design, data collection and analysis, decision to publish, or preparation of the manuscript.
Data Availability
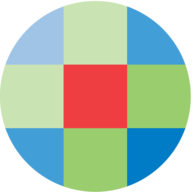
- Get new issue alerts Get alerts
- Submit a Manuscript
Secondary Logo
Journal logo.
Colleague's E-mail is Invalid
Your message has been successfully sent to your colleague.
Save my selection
Novelty in research: A common reason for manuscript rejection!
Kumar, Nishant; Ali, Zulfiqar 1 ; Haldar, Rudrashish 2
Department of Anaesthesia, Lady Hardinge Medical College and Associated Hospitals, New Delhi, India
1 Department of Anesthesiology, Sher-i-Kashmir Institute of Medical Sciences, Srinagar, Jammu and Kashmir, India
2 Department of Anesthesiology, Sanjay Gandhi Post Graduate Institute of Medical Science, Lucknow, Uttar Pradesh, India
Address for correspondence: Dr. Nishant Kumar, A 103, Urja Towers, Sector 47, Gurgaon, Haryana, India. E-mail: [email protected]
This is an open-access article distributed under the terms of the Creative Commons Attribution-Noncommercial-Share Alike 4.0 Unported, which permits unrestricted use, distribution, and reproduction in any medium, provided the original work is properly cited.
We often hear back from reviewers and editors of scientific journals that a particular manuscript (original research, case report, series or letter to the editor) has not been accepted because it lacks novelty. Though disheartening, the reason for such a response from said reviewers needs proper elucidation, as a moral obligation from the editorial board towards the authors of the manuscripts.
Research, as defined by the Cambridge Dictionary is ‘a detailed study of a subject, especially in order to discover (new) information or reach a (new) understanding’. [ 1 ] Novelty on the other hand is defined as ‘the quality of being new, original, or unusual’ or a ‘new or unfamiliar thing or experience’. Therefore, adding the adjective novel along with research is actually one of the most common redundancies that is similar to ‘return back’ or ‘revert back’ and denotes one and the same thing! [ 1 ]
Without delving into the nitty-gritty of the English language, novel research can be best described as one or more elements of research that are unique, such as a new methodology or a new observation that leads to the acquisition of new knowledge. It is this novelty that contributes to scientific progress. Since the main aim of research is to unravel what is unknown or to challenge views or ideas that may or may not be based on sound scientific principles, this exclusivity of novel research therefore allows us to expand our horizon beyond the realms of known domains. [ 2 ]
Having defined novelty in research, one of the most common mistakes that researchers commit is confusing novelty with originality. These terms are often used interchangeably. Originality implies the genuineness of the work and signifying that the said work has not been copied from any other source. Originality can always be examined by plagiarism checkers, and data is often analysed for duplication or fabrication only if there exists a certain doubt regarding its factuality. A study, therefore, can be mutually exclusive i.e. novel, but not original, or it can be original but not novel. It is the latter that reviewers and editors encounter most often.
The most common scenario encountered in anaesthesia related manuscripts that lacks novelty is the substitution of the same anaesthetic technique to different surgical procedures or patient populations (based on gender or age), with no expected change in the result. Here, the hypothesis and study designs are almost identical; however, the agents are replaced with different ones. A classic example is the comparison of the duration of analgesia with a longer acting analgesic or that of a local anaesthetic with a shorter one. The intrinsic properties of a drug are already well known, and, irrespective of it being an abdominal surgery or a limb surgery, the drugs are going to behave according to their pharmacological properties. Similarly, modern airway devices, such as video laryngoscopes, have conclusively been proven to be better aids than the conventional ones. A comparison of any new laryngoscope would definitely be a novel idea, in terms of whether it outperforms the existing device. If a certain number of studies, systematic reviews, or metanalyses have already been published on that particular device or drug, the study undertaken cannot be considered novel unless the results of the aforementioned study, utilising sound scientific principles, actually challenge or contradict the existing ideas.
Another common scenario faced by the reviewers or editors is the anaesthetic management of common or uncommon syndromes or diseases. They are often well described in literature, but when managed as per the existing guidelines and expected challenges they do not constitute novelty. A case report is novel and worth publishing if an unforeseen or unanticipated event has occurred or the case has been managed in a unique or unconventional manner or significant innovative skills or equipment have been employed. However, due caution has to be exercised as this should not lead the researcher to be overtly adventurous or show undue bravado by going against the principles of patient safety.
Now here lies the contradiction. We have been harping on novelty, introducing new ideas, and challenging old fixed ideas when conducting research and reporting cases. However, at the same time, due caution must be exercised, and one must not to be adventurous, unconventional, or bold. There is a fine line of distinction between these two. Herein comes the role of ethics, a separate topic of discussion altogether.
Research or advancement may not always be novel just by intervention or experimentation. Theoretical or hypothesis testing may also contribute paradigm-changing findings. Some of these may include thought-based experiments, rectifying or logical rearrangement of existing knowledge, re-evaluating space and time, utilising principles of philosophy, and analysing already existing data from a new and different perspective. [ 3 ] A thorough literature search is pivotal for designing a novel research project as it helps to understand known facts and gaps. An attempt at bridging identified research gaps adds to the novelty of the study. [ 2 ]
Another aspect of novel research is technological advancement. Most research starts from an idea, a thought, or an observation that further leads to hypothesis building, experimentation, data collection, analysis, and, finally, principle building. Technological advancement may stem from any of these phases. Novelty in research propels the industry to excel and outdo itself. [ 4 ]
Can novelty in research be measured? The answer is a resounding yes. Traditionally, it has been measured through peer reviews and by applying bibliometric measures such as citation or text data, keeping in mind their inherent limitations. However, word embedding is a new technique that can reliably measure novelty and even predict future citations. However, this is currently limited by publicly available word-embedding libraries and its high costs. [ 5 ]
To the average author and reader, novelty adds to their knowledge and makes them aware of complications that they may encounter. It offers a way out by conventional or different measures, within the realm of scientific, ethical and principles of social justice, should they get stuck, keeping in mind the quote of Hippocrates: ‘ Primum non nocere’ ( First, do no harm ).
- Cited Here |
- Google Scholar
- + Favorites
- View in Gallery
Readers Of this Article Also Read
Evaluation of nebulised dexmedetomidine in blunting haemodynamic response to..., a comparative study of 10% lidocaine spray versus eutectic mixture of 2.5%..., recent advances in obstetric anaesthesia and critical care, recent advances in paediatric anaesthesia, update on preoperative evaluation and optimisation.
- DOI: 10.24147/1990-5173.2020.17(4).107-110
- Corpus ID: 234377492
Scientific Novelty in the Dissertation by E.V. Krotova
- E. Nevzgodina , N. Temnikova
- Published 28 December 2020
Related Papers
Showing 1 through 3 of 0 Related Papers
Does science disrupt technology? Examining science intensity, novelty, and recency through patent-paper citations in the pharmaceutical field
- Open access
- Published: 09 August 2024
Cite this article
You have full access to this open access article
- Keye Wu 1 , 2 ,
- Ziyue Xie 2 , 3 &
- Jia Tina Du ORCID: orcid.org/0000-0002-3243-5768 3 , 4
79 Accesses
Explore all metrics
The role of scientific knowledge in advancing technology is widely recognized, but its impact in generating disruptive ideas and catalyzing technological change is less well known. To fill this gap, this study addresses a new research question about whether and how prior scientific knowledge contributes to technological disruptiveness. Specifically, our study focused on the pharmaceutical field, which has a frequent interaction between science and technology, and employed the patent-paper citations to explore the disruptive impact of science on technology. Drawing on the 1,883,593 granted patents in pharmaceuticals and their 1,546,960 cited papers prior to 2018, we found patents with scientific references appear to be more disruptive than those without scientific citations and such effect has gradually pronounced in recent decades, even though technological disruptiveness is generally declining over time. For each granted patent, we further developed three scientific characteristics including science intensity, science novelty and science recency and estimated their effects on technological disruptiveness. The regression analysis showed science intensity and science novelty both have an inverted U-shaped relationship with technological disruptiveness, suggesting intermediate-level novel scientific knowledge input can inspire the generation of disruptive ideas for pharmaceutical technological innovation. While science recency presents a negative association, underlying that recent scientific knowledge could offer advanced theoretical insights that may destabilize the existing technological trajectory. Moreover, collaboration is another important factor in enhancing the disruptive impact of science on technology. Our study contributes to the existing literature by introducing the disruptive impact of science on technology.
Similar content being viewed by others
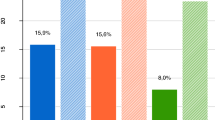
The case of sleeping beauties in nanotechnology: a study of potential breakthrough inventions in emerging technologies
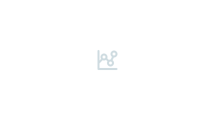
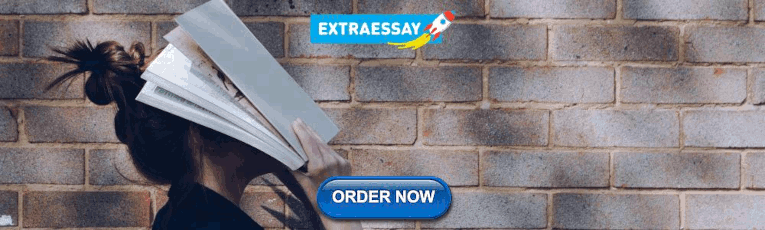
Basic science as a prescription for breakthrough inventions in the pharmaceutical industry
Analyzing the value of technology based on the differences of patent citations between applicants and examiners.
Avoid common mistakes on your manuscript.
Introduction
There is abundant evidence showing scientific advancement plays a crucial role in driving technology development (Toole, 2012 ). Scientific research has been well accepted as the “prior art” of practical inventions, which could provide innovative ideas and novel knowledge for technological development and finally progress into economic growth (Klitkou & Gulbrandsen, 2010 ). Numerous important technical inventions were the result of advances in scientific research. For example, the invention of the atomic bomb relied on a major scientific discovery in the field of quantum physics (Rhodes, 2012 ); the advent of nanomaterials is sourced from the development of computational biology and materials science (Finardi, 2011 ).
However, in an increasingly complex innovation environment, the interaction of science and technology is far from simply applying advanced scientific theory to develop new technologies (Arthur, 2007 ). With a deeper understanding of science-technology linkages, researchers found there are two different knowledge transfer pathways from science to technology. Traditional knowledge theory holds that technology is a type of applied science which is sourced from basic research (Tijssen & Winnink, 2016 ). It suggests knowledge interaction between science and technology could solve technological issues and enhance the performance and efficiency of existing technologies (Cassiman & Veugelers, 2006 ). From this perspective, science serves as a complementary role in technological development and stretches the existing technological trajectory. In contrast, another research stream of emerging technologies and innovation literature supposes that new technologies, introducing new methods, new products and new processes, could also be inspired by marginality and cross-cutting of science, e.g. interdisciplinary (Ebadi et al., 2022 ; Zhou et al., 2019 ). They suggest scientific participation in technological development can raise up the atypical recombination between science and technology, increase the novelty of technological outcomes (Verhoeven et al., 2016 ) and thus disrupt the existing technological trajectory (Xu et al., 2020 ). It is important to clarify that the consolidating and disrupting roles of science are not in conflict, two kinds of impact could co-exist in the technological development (Chen et al., 2021 ). Since the prompting/consolidating role of science on technology is well-documented, our research aims to answer another important question that ① Whether scientific participation in technological development may have a disruptive impact? If the first question is well-addressed, we also want to know ② How does scientific knowledge disrupt the existing technological knowledge? The answer to these questions can help us theoretically grasp the S&T innovation laws, understand the complex knowledge transfer pattern, promote the transformation of scientific outcomes, and optimize S&T innovation policies (Chen et al., 2023 ).
To address our research questions, this paper focused on the granted patents in the pharmaceutical field which has a well-established S&T linkage (Ahmadpoor & Jones, 2017 ). We employ patent-paper citations to track the knowledge flow from science to technology (Narin et al., 1997 ), and combine patent forward and backward citations to quantify the disruptiveness of focal patent. By statistically comparing the patents with and without scientific references, we found the disruptive effect of science upon technology is gradually pronounced in recent years, along with the declining trend of patent disruptiveness. More specifically, we also investigate three important scientific characteristics and their disruptive impact on technology: science intensity, science novelty and science recency. These indicators are developed from the scientific publications cited by the technological patents, revealing how and to which extent scientific knowledge participated into technological development. By using ordinary least square (OLS) models to estimate their effects, we learned that intermediate science intensity and moderate science novelty make great contributions to technological disruptiveness. This suggests that scientific knowledge needs appropriate coordination with technological knowledge to generate disruptive technological outcomes, otherwise scientific knowledge is difficult to be absorbed by the focal technical field. Science recency has a negative relationship with technological disruptiveness, indicating that fresh and cutting-edge scientific knowledge is one of the sources of disruptive technologies. Further robustness analysis gives us new insights that collaboration could enhance the disruptive effect of science upon technology. The possible explanation is that collaboration could increase knowledge diversity and improve the efficiency of knowledge transfer from science to technology.
This study makes contributions to both existing literature and R&D practice. First, this study enriches our understanding of the knowledge flow from science to technology. Most previous literature has only examined that science has a cross-domain impact on technology and could lead to higher innovation performance (Veugelers & Wang, 2019 ). This study demonstrates that science also has a disruptive effect upon technology, which is a totally new pathway from science to technology. Appropriate knowledge convergence between science and technology is the best option, rather than comprising unrelated scientific knowledge as much as possible. Second, our study also provides new insights for practitioners to improve their technological outcomings’ disruptiveness. According to our results, practitioners need to collaborate with scientists from relevant fields who possess the frontier scientific knowledge for acquiring disruptive ideas. Our research is of great interest in breaking through technological bottlenecks, especially in the context of a general decline in technological destructiveness (Park et al., 2023 ).
This article is organized as follows. In “ Related works ” section, we review the related literature about the transformation from science to technology and the technological impact of three science-based indicators. Next, in “ Data and method ” section, we introduce the data set, variables and their measurements for empirically answering our questions. In “ Results ” section, we present the results of our empirical tests and, in “ Discussion and implication ” section, we discuss the implications and limitations of this study.
Related works
Science and technological impact.
How science contributes to technology is a long-standing question in the innovation literature. According to Bush’s linear model (Bush, 1990 ), innovation obeys an idealized sequential progress starting with basic research, followed by applied research and development, and ends with production and diffusion. In this process, scientific knowledge can help inventors understand the essences and principles of technological problems, supporting their search for promising solutions in the inventive space. Fleming and Sorenson ( 2004 ) found that patents that have scientific references are more innovative and impactful, evidenced by receiving more citations from other patents, but only for relatively difficult inventions, that is, those seeking to combine highly coupled components. Cassiman et al. ( 2008 ) found patents have broader impact scopes rather than higher forward citation counts when they cite science. Subsequent research took the perspective of scientific research and found that basicness, interdisciplinary and knowledge recombination of papers are more likely to have technological impact on both patent-level (Ke, 2020 ; Wang & Verberne, 2021 ) and company-level (Leten et al., 2022 ). The above literature all agreed with the same underlying theory that scientific developments precede technological advances and play as a complementary role in overcoming technological bottlenecks and guiding technological innovation.
However, recent literature portrayed that science may not only play a promoting role in technological development but also disrupt technology by proposing entirely new technical questions or opening up new solutions for the existing technical questions. For example, Xu et al. ( 2020 ) argue that advanced scientific knowledge can open up a broad set of technological opportunities by detecting the topic linkage between science and technology. The cross-disciplinary knowledge convergence is also demonstrated to be informative in the early identification of emerging technologies (Ebadi et al., 2022 ; Zhou et al., 2019 ), which may disrupt the existing technology trajectory due to the radical novelty, relatively fast growth, coherence, prominent impact, and uncertainty and ambiguity characteristics (Rotolo et al., 2015 ). Previous studies conveyed the idea that science may have a disruptive impact on technological development, but how to organize scientific knowledge and to what extent science could disrupt technology is still unclear and needs further exploration.
In order to empirically track the knowledge flow from science to technology, patent-paper citation is often employed at a large scale, that is, using the scientific references in patents as relevant prior art for the patented inventions (Narin et al., 1997 ). A number of studies have examined the validity of using scientific publications in patent references for tracing such knowledge flow. For example, Nagaoka and Yamauchi ( 2015 ) compared cited scientific papers in patents with survey results of Japanese inventions, found scientific papers do not necessarily mean the direct or essential input for the patented invention but play a more indirect role as a source of relevant background information. Meyer and Persson ( 1998 ) and Callaert et al. ( 2014 ) reached a similar conclusion that scientific publication serves as the source of inspiration for invention rather than the direct links between science and technology. Roach and Cohen ( 2013 ) confirmed that patent-paper citation can be viewed as a comprehensive measure of knowledge flow from public research to technology.
Science intensity and technological impact
In order to figure out whether and how science disrupts technology, our study focuses on three scientific characteristics and their relationships with technological disruptiveness by summarizing the existing literature. The contribution of scientific intensity to technological innovation is well-documented. According to Liu et al. ( 2020 ), scientific intensity reflects the converging level between scientific knowledge and technological knowledge. In the research stream of innovation studies, the connection with public research, evidenced by references to scientific articles contained in patent documents, can positively improve technological productivity (Van Looy et al., 2002 ), technological impact (Hohberger, 2016 ) and even economic performance (Hiadlovsky et al., 2018 ). The intensity of science in different levels has different influence patterns upon technology development.
On the one hand, less science-based inventions are expected to have a more clearly defined but a more limited set of possible technological applications (Pezzoni et al., 2022 ). Tussen et al. ( 2000 ) acclaimed that science acts as exploratory action in R&D, it functions as an effective recombination of existing knowledge and its practical application. That is, due to the mapping role of scientific research in R&D, appropriate knowledge convergence between science and technology can efficiently address the technological problems encountered in innovation processes. Hohberger ( 2016 ) demonstrates that intermediate levels of science intensity played the highest citation propensity. Du et al. ( 2019 ) suggested convergence of science and technology at moderate levels (around 1/3) can achieve successful pharmaceutical innovation.
On the other hand, when science intensity is accumulated to a certain point, its impact on innovation performance could totally be different. A high level of science intensity, revealing the origin and essence of natural phenomena, could raise new technical problems that have never been observed, or come up with new problem-solving strategies and eliminate unpromising technological paths (Fleming & Sorenson, 2004 ). Lee et al. ( 2018 ) showed that an increase in the proportion of scientific knowledge in convergence has a positive but curvilinear relationship with innovation impact. As high scientific intensity disrupts the technology trajectory, the resulting innovation performance also becomes uncertain. Malva et al. ( 2015 ) suggested that basic science intensity does not increase the likelihood of technology breakthroughs, but it is significant at the firm level and could reap in other areas of the technology portfolio of the firm. In terms of economic performance, Hiadlovsky et al. ( 2018 ) found increasing the share of employment in science and technology beyond a certain turning point would not lead to any further positive effects on regional economic performance.
Science novelty and technological impact
From the combinational perspective, novel science/technology is widely accepted as the recombination of pre-existing knowledge components in an unprecedented fashion (Simonton, 2003 ). Within scientific areas, novel research is more likely to deliver scientific breakthroughs and generate extraordinary academic impact (Stephan et al., 2017 ; Wang et al., 2017 ). When it comes to technical innovation, Veugelers and Wang ( 2019 ) found scientific research characterized by higher combination novelty can not only raise scientific impact but also lead to higher technological impact, evidenced by receiving more patent citations than non-novel scientific research. Taking the perspective of individual technological invention, Verhoeven et al. ( 2016 ) developed another type of novel, namely novelty in the knowledge origins, which denotes the rareness of prior scientific/technological knowledge in the patent citation. This type of novelty is essentially similar to combinational novelty, denoting new scientific knowledge or technological knowledge that has never been combined with the existing knowledge. Following this conception, our study defines science novelty as novel connections made between new scientific fields and focal technical classes (Schoenmakers & Duysters, 2010 ).
Such input of novel scientific knowledge may disrupt the existing technological trajectories and meanwhile open up new pathway solutions for the complex technical challenges (Fleming & Sorenson, 2004 ). Relying on the theory of technological radicalness, several works suggested that a radical invention occurs when a new combination of components, especially those sourced from a scientific area (Gittelman & Kogut, 2003 ), creates a departure from the current situation (Shane, 2001 ). Arthur ( 2007 ) believed that knowledge recombination between science and technology increases the probability of making breakthroughs. For instance, the idea of autonomous driving using computer vision and vehicle control is a revolutionary idea and originates from a scientific perspective.
However, even though science novelty has the opportunity to destabilize the technology trajectory, it may also face a higher level of uncertainty (Stephan et al., 2017 ). Foster et al. ( 2015 ) suggested that innovative publications are more likely to achieve a higher impact than conservative ones but can also fail more frequently than they succeed. Applying firm level rather than patent level, researchers have demonstrated that cross-domain scientific knowledge would decrease the corporation innovation performance (Ferguson & Carnabuci, 2017 ).
Science recency and technological impact
As prior knowledge is crucial to technological innovation, the recency of prior knowledge, which refers to the age of prior knowledge input in the focal publication, has been recognized as another important predictor of the birth of important discoveries (Colavizza & Franceschet, 2016 ; Mukherjee et al., 2017 ; Petruzzelli et al., 2018 ). When scientific knowledge is recent, it takes time to be acknowledged by the scientific community, and even takes more time to observe these new ideas codified in educational material available to scientists and engineers employed in industrial R&D (Kuhn, 2012 ).
Some scholars argued that recent knowledge can provide advanced insights and trigger radical innovation (Schoenmakers & Duysters, 2010 ), since knowledge value decreases as time goes by (Gu et al., 2017 ; Kong et al., 2017 ). In the scientific area, science recency plays an important role in producing significant scientific breakthroughs. For example, Liang et al. ( 2020 ) observed over half of Nobel Prize-winning articles have cited at least 68% recent literature published within 5 years. Costas et al. ( 2012 ) found that top-performance scientists commonly use a higher number of references which are more recent and more frequently covered by Web of Science. Furthermore, science recency can also generate profound technological impact. Many companies employ recently published scientific knowledge to develop new techniques and tools offering greater value to their clients and meanwhile save costs (Birkinshaw & Sheehan, 2002 ).
In contrast, other scholars argued that mature knowledge benefiting from the test of time can reduce unnecessary inputs and is also likely to stimulate important discoveries (Nerkar, 2003 ; Rose-Wiles & Marzabadi, 2018 ). Due to the fact that cross-domain knowledge transfer from science to technology intrinsically has a time delay, scientific knowledge usually experiences sufficient discussion in the scientific areas before it develops as useful input to technical fields (Ahmadpoor & Jones, 2017 ; Finardi, 2011 ). Such adoption of mature scientific knowledge in technological development is equivalent to the “standing on the shoulders of giants” and can significantly reduce its risk of failure. Furthermore, some studies suggest that papers do not achieve citation recognition until a few years after publications, so-called “sleeping beauties”, may be hard to be accepted by the audience immediately due to their novelty characteristics (Costas et al., 2010 ; Li & Shi, 2016 ). Once these “sleeping beauties” are awakened, they show the highest impact within their fields (Li & Shi, 2016 ). Van Raan and Winnink ( 2018 ) made a further step and found “Sleeping Beauties” are increasingly discovered earlier by a “technological prince” rather than a “scientific prince”, manifesting their technological importance.
Data and method
In order to empirically investigate the disruptive impact of science upon technology, we take each patent as the analysis unit and employ patent-paper citations to trace the knowledge flow from science to technology. Our explanatory variables are science-related indicators including science intensity, science novelty and science recency which are measured through the scientific documents in the references of focal patent, and the explained variable is technological disruptiveness which is developed based on the subsequent usage of focal patent. The dataset collection process, measurement of variables and methodology are as follows.
Data collection
The dataset of this study is collected from three sources as shown in Fig. 1 . First, we collect the granted patents in the pharmaceutical field as the focal patents, as this technical sector has relatively high frequency of science-technology linkages (Ahmadpoor & Jones, 2017 ) allowing us to conduct a deep analysis. Specifically, we retrieve relevant patents from the Worldwide Patent Statistical Database (PATSTAT) by constraining “TECHNICA_FIELD_NR” as 16 which represents pharmaceuticals in the Chemistry sector. Moreover, we only take patents published before 2018 into consideration for two reasons. On the one hand, there is a 2–3 years lag in patent disclosure (Finardi, 2011 ), which means patents applied in recent years have not yet been completely published. On the other hand, our dependent variables, namely technological disruptiveness, depend on the fixed length of citation windows. Taking this into account, we follow the operation of Park et al. ( 2023 ) and retain a 5-year observation window. To this end, 1,883,593 focal patents in pharmaceuticals field before 2018 have been retrieved.

Three steps of data collection
Second, in order to capture the knowledge flow from science to technology, focal patents’ scientific citations are extracted from the patent cite science (PCS) dataset, which is a systematic paper-patent citation dataset constructed by Marx and Fuegi ( 2020 ). To accurately capture the knowledge flow from science to technology, we only select the citation pairs with the highest confidence score at 10, which is assigned by Marx and Fuegi ( 2020 ) to represent the accuracy of each citation relationship. Thus, 9,982,244 patent-paper citation pairs have been collected.
Third, for the purpose of examining the characteristics of referenced science, we further retrieve more bibliometric information of 1,546,960 cited papers from Microsoft Academic Graph (MAG), which includes more than 83 million papers with their citations, authors and affiliations (Sinha et al., 2015 ). In this study, we primarily focus on the WoS-defined subject and publication time of the cited scientific references, which can be used to measure the extent to which scientific knowledge participates in the process of patenting.
Measurement for technological disruptiveness
For measuring technological disruptiveness, we followed the operationalization of Chen et al. ( 2021 ) which employs forward citation and backward references simultaneously. It is worth noting that, unlike the CD index proposed by Wu et al. ( 2019 ), this indicator only focuses on the disruptiveness and calculates the D index for each prior art by taking the citations to the focal patent and/or the prior art as the denominator.
Taking Fig. 2 as an example, there are three types of patents in the citation network involving focal patent f , references R = [r 1 … r i ] and subsequent patents S = [s 1 …s j ]. According to previous literature (Park et al., 2023 ), we only include patents which cited f or cited its prior arts R 5 years after the focal patent has published in the subsequent set S . For each prior art ri , if subsequent work sj cites both focal patent f and prior art ri , then f consolidates the prior art ri . In contrast, if sj cites focal patent f but not ri , then f destabilizes the prior art ri . Specifically, the formula for measuring disruptiveness for the focal patent is defined as follow: \(\text{D}=\frac{1}{N}\sum_{i=1}^{N}\frac{{n}_{f}^{i}}{{n}_{f}^{i}+{n}_{fr}^{i}+{n}_{r}^{i}}\) , where N denotes the number of prior arts of the focal patent f. For each prior art ri, \({n}_{f}^{i}\) counts patents that cite the focal patent f but not its prior arts ri , \({n}_{fr}^{i}\) counts subsequent patents that cite both f and ri and \({n}_{r}^{i}\) counts patents that only cite ri but not f .
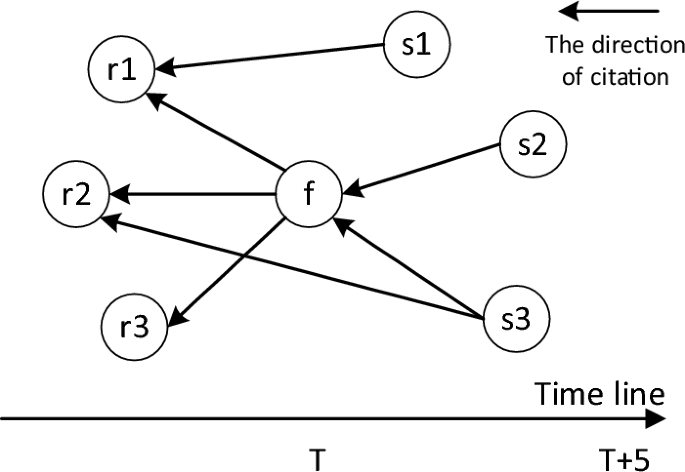
Calculation technological disruptiveness for a focal patent
Measurement for scientific characteristics in technology development
To quantify scientific participation in technology, we develop three variables, namely science novelty, science intensity and science recency, using the scientific documents in the patent references.
- Science novelty
The first independent variable is science novelty. From the perspective of novelty in knowledge origins (Verhoeven et al., 2016 ), science novelty refers to the rareness of scientific knowledge flowing to the focal technical field (Rizzo et al., 2020 ), which is calculated based on previous scientific references. If a patent cites papers from a particular scientific area that have never been cited in previous inventions belonging to its technical field, it has a relatively high degree of science novelty. According to previous operations (Stringer et al., 2010 ), this study employs web-of-science (WOS) defined disciplinaries to proxy for different areas of scientific knowledge. To guarantee the comparativeness of patents applied in different years, we apply the Uzzi et al. ( 2013 ) method of using Z-score to normalize citation frequency to the specific scientific area before the observed year. The operations are split into two steps as follow:
Calculate novelty of each cited scientific area in an individual year
In the above formula, we conduct the reversal operation upon citation frequency before the observed year, ensuring scientific area with fewer citation frequency has higher science novelty. Specifically, \({cf}_{i}^{t}\) counts the number of patents that cited papers from area i before the observed year t , \({cf}_{max}^{t}\) and \({cf}_{min}^{t}\) denote the maximum and minimum number of patents cited specific scientific areas before the observed year t .
Calculate the weighted average the novelty in distinct cited scientific areas
For each focal patent, M denotes the number of referenced scientific areas, and \(np\) denotes the number of all cited papers while \({np}_{i}\) counts the number of cited papers that only belong to area i . In this operation, we calculate the proportion for each cited area i as the weight of its scientific novelty. Then sum up the novelty of all cited areas as the final measurement.
- Science intensity
The second one is scientific intensity, which is designed to measure the extent to which scientific knowledge participates in technology development. Following the previous operations, we simply calculate the ratio of scientific references accounting for all references of focal patent as the measurement (Breschi & Catalini, 2010 ), denotes as the formula: \(Science intensity =np/nr\) in which, \(nr\) counts the number of all references in the focal patent including patent references and non-patent references. This operation has been commonly employed in innovation studies (Lee et al., 2018 ) and proved to be robust for science and technology interaction (Hohberger, 2016 ). With the higher science intensity, technological development is much more sourced from science.
- Science recency
The third one is science recency, which represents the freshness of scientific knowledge flowing to the technical field. Various measurements for knowledge recency are based on the age of references, such as “citation half-life”, and the “Price index” (Milojevic, 2012 ; Petruzzelli et al., 2018 ). Following this, our study employs the most common indicator which averages ages of all referenced scientific papers as the measurement: \(Science\, recency ={\sum }_{j=1}^{np}{t}_{i,j}/np\) , in which \({t}_{i,j}\) denotes the time interval between each scientific reference i and focal patent j that cite it, which is computed by the focal patent j ’s application year minus the publication year of the reference i . This indicator comprehensively depicts the extent to which technology is relied on the recent researches.
Control variables
To correctly identify the association, we control several other confounders which may be related to technological disruptiveness at the patent level. Firstly, the reference number of patent or paper has a non-negligible effect on its disruption. Funk and Owen-Smith ( 2017 ) found that patents are more consolidated when they have more references, that is reference number has a negative relationship with disruptive in terms of patents. While Ruan et al. ( 2021 ) reached a similar conclusion when the analysis units are scientific papers in the lower reference number group, but the reference number has a converse effect upon paper disruption when it comes to higher reference number papers. Therefore, we included references num variable to eliminate such biases. Secondly, patent family size refers to the value of patent rights (Dechezleprêtre et al., 2017 ). The existing literature argued that patents with larger family size have higher welfare effects (Wu et al., 2015 ) and broader technological impact (Kabore & Park, 2019 ) which may probably because of the unconventional characteristics of the patent itself. Thus, we comprise patent family size as another control variable. Thirdly, team size also has a higher corelation with patent/patent disruption. To this topic, a representative work is Wu et al. ( 2019 ) found that smaller teams have tended to disrupt science and technology with new ideas and opportunities, whereas larger teams have tended to develop existing ones. Therefore, we control the number of applicants ( nb_applicants ) and number of inventors ( nb_inventors ) to take the team size into account. Last but not least, we include application filing year dummies ( Year FE ), so that our estimated coefficients can eliminate the year differences, especially in terms of d_index (Bornmann & Tekles, 2019 ). The authority for granting application is also converted as dummy variables ( Appln_auth FE ) for covering regional differences.
In order to investigate the impact of scientific knowledge on technological disruptiveness, we applied two steps to illustrate their relationships. To address the first question about whether scientific knowledge disrupts technological development, we employed all of the granted patents in the pharmaceutical field and used statistical methods to compare the technological disruptiveness between patents with and without scientific references. Correlation and descriptive analysis are also conducted to depict the general relationship between three scientific characteristics and technological disruptiveness. To answer the question of how does science disrupt technology, we further focused on the patents with scientific references and employed Ordinary Least Square (OLS) models to investigate nonlinear relationships between scientific characteristics and technological disruptiveness. In this analysis, we take each technological patent as the analysis unit.
Description analysis for disruptive impact of science upon technology
To illustrate the technological impact of scientific participation, we first distinguish two groups of patents according to whether they report scientific references. We observe the fraction of patents citing prior scientific art is quite small, corresponding to 19% (363,635/1,883,593 patents) of the whole sample, but they can generate tremendous technological impact. Figure 1 shows the forward citation and disruptiveness of these two groups of patents at an average level over the entire timeline. The shaded areas represent 95% confidence interval. Both indicators fluctuated before the 1980s, which is the period of pharmaceutical technology was still stagnant in the laboratory and had no access to industry. When it comes to the 2000s, the market and legitimation of intellectual property has been complete and the differences between the two groups can be clearly observed.
In terms of forward citations, patents with scientific references have apparently higher technological impact than those without scientific references ( t = 218.43, p < 0.01). Especially in the recent two decades, patents with scientific references can receive about 2.67 subsequent citations on average over 5 years after their publication, while this value of the patents without scientific references is only 0.75. This implicates patents sourced from scientific knowledge are more likely to be impactful for future technological development. Moreover, Fig. 1 b compares the technological disruptiveness between the two groups. Even though the general trend of d_index is decreasing since the 1990s, which corresponds to recent work (Park et al., 2023 ), patents with scientific references still show a slightly higher average d_index than that of patents without scientific references ( t = 50.01, p < 0.01). This suggests patents citing scientific papers gain a wider technological impact by destabilizing prior art rather than consolidating them (Fig. 3 ).
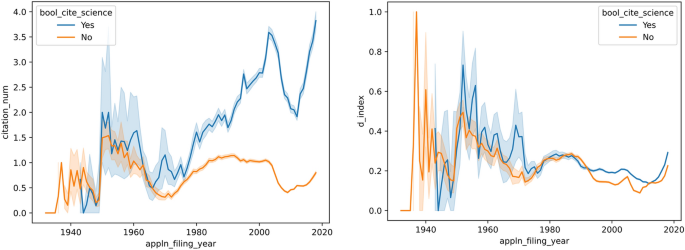
Comparison between patents with and without scientific references in terms of forward citation and d_index
In order to deeply investigate how scientific knowledge disrupts technology, we focus on the patents with scientific references and estimate the effects of three scientific characteristics. The descriptive statistics of related variables are reported in Table 2 . The maximum value of d_index is 1 when the focal patent totally disrupts its prior art, and the minimum value is 0 when the focal patent has not received the subsequent citations. The median and mean value of d_index are 0.19 and 0.21 respectively indicating the majority of patents showing lower disruptiveness. The mean and median value of science_intensity are 049 and 0.50 indicating a deep participation of scientific knowledge in technological development. The mean value of science_novelty is 0.56 and the median value is 0.57, suggesting patents are more inclined to follow the established science-technology linkage rather than refer to the scientific knowledge from new scientific areas. The mean value of science recency is 10.33 (years), representing the average time lag of knowledge transfer from science to technology. Excluding the delay of patent publication, scientific knowledge needs about 7–8 years to become accessible to technological fields (Fig. 4 ).
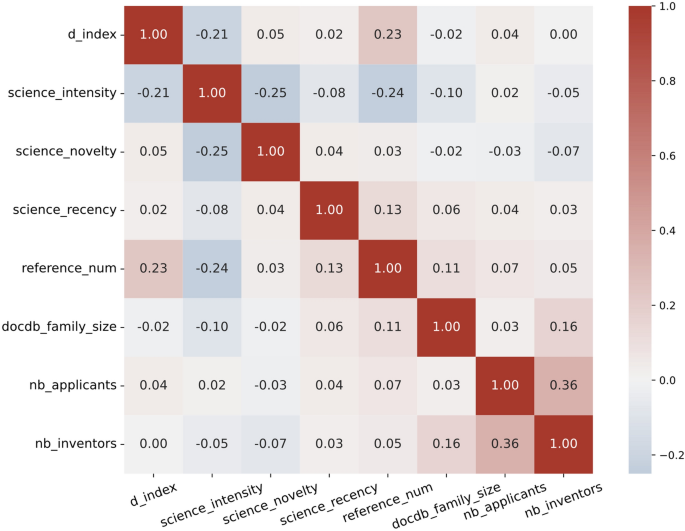
Pairwise Pearson correlation efficient between variables
Moreover, Fig. 5 depicts the Pearson correlation efficient of dependent variables, independent variables and control variables for further regression analysis. Most correlations are at acceptable levels, except for the relationship between two control variables, the number of inventors and the number of applicants. To examine potential multicollinearity issues, we calculated the variance inflation factor (VIF). All VIFs are relatively low (< 2), even the highest one, science_intensity, is only up to 1.41 while nb_applicants and nb_inventors both have an acceptable VIF value of 1.11. It is also worth noting that d_index is negatively correlated with science_intensity , while it is positively related to science_novelty and science_recency . This gives us the first insight that prior knowledge sourced from recent and novel scientific advancement is more likely to boost technological disruptiveness.
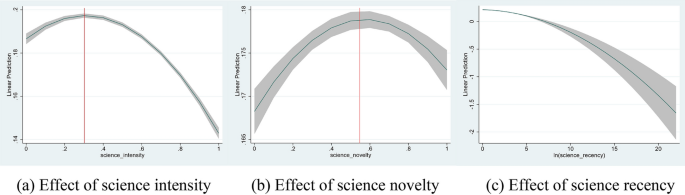
The linear predictions of three science-based variables
Regression analysis for effects of individual scientific characteristic
In this sector, we employ a series of regression analysis to estimate the curvilinear effect of three scientific characteristics upon technological disruptiveness. Specifically, we performed logarithmic operations on variables with maximum values exceeding 1 to reduce outliers and data fluctuations. Table 2 displays the regression estimations.
Model 1 only includes the control variables. Several effects of the control variables are worth mentioning. For example, the patent’s reference num is marginally positively related to its disruptiveness ( β = 0.081, p < 0.001) which is in line with the arguments of Bornmann et al. ( 2020 ) that the disruption index should be used only for patents with sufficient references. Patent disruptiveness is also higher for those inventions applied by multiple applicants ( β = 0.013, p < 0.001) and inventors ( β = 0.003, p < 0.001). In contrast, ln(patent_family_size) shows a significant negative relationship with the patent’s disruptiveness ( β = − 0.012, p < 0.001), indicating that destabilized inventions are less likely to have business value and are hard to be protected by applicants. These control variables stay robust in the most subsequent regression models, except for the significance of the positive effect of nb_inventors weakens in model 4 and model 5 which consider the effect of science novelty.
Model 2 and 3 provide statistical supports for an inverted U-shape relationship between science intensity and technological disruptiveness. The main effect of science intensity is negative and significant at 0.1% level ( β = − 0.042, p < 0.001) in Model 2, while the quadratic term is added in Model 3, the main effect still remains significant ( β = 0.070, p < 0.001) but turns to be positive. The negative and significant effect of the quadratic term ( β = − 0.113, p < 0.001) in Model 3 confirms the curvilinear relationship between science intensity and technological disruptiveness. To be specific, Fig. 5 a depicts the estimated marginal effects of science intensity , including the turning point (0.308) before which science intensity increases the technological disruptiveness ( slope = 0.069) while thereafter decreases it ( slope = − 0.157). It suggests that the convergence of science with technology at the range of 0% to 30% could provide new insights and disrupt the existing technology, but too much participation of scientific knowledge could hard to be accepted by the technological audience.
Model 4 and 5 suggest that science novelty also has a U-shaped relationship with technological disruptiveness. The main effect of science novelty is weakly positive in model 4 ( β = 0.004, p < 0.001) and significantly positive in model 4 ( β = 0.037, p < 0.001). Meanwhile, the quadratic coefficient of science novelty ( β = − 0.032, p < 0.001) is significantly negative in model 5. Figure 5 b intuitively demonstrates the quadratic relationship by plotting the estimation results with 95% confidence intervals for knowledge novelty for scientific input. It is important to note that turning point 0.57 is well within the data range from 0 to 1, with 48.9% of the observations being associated with a negative impact of science novelty, highlighting the relevance of our observations. These results suggest science novelty can effectively increase technological disruptiveness ( slope = 0.037) when the novelty is at a lower level, while above this point, scientific knowledge is too novel to the focal technical field and has the risk to be failure ( slope = − 0.027).
Model 6 and 7 demonstrate that publishing time lag ( science_recency ) between paper and patent is negatively associated with technological disruptiveness. The main effect of science recency is negative at 0.1% significance in model 6 ( β = − 0.020, p < 0.001), as well as in model 7 ( β = − 0.004, p = 0.021). Model 7 also confirms that the quadratic coefficient of science recency is also significantly negative ( β = − 0.004, p < 0.001). However, Fig. 5 c shows that the extreme point of this relationship (− 0.576) is outside data range, thus there is no inverted U-shape relationship but only a negative association between science recency and technological disruptiveness. These results suggest that the more recent the scientific knowledge input, the more likely it is to be the impetus for technological disruption. In addition, model 8 comprises all the variables and confirms the above-mentioned relationships. The maximum coefficients of science novelty and its quadratic term in model 8 demonstrate science novelty plays a more important role in disrupting technological compared to the science recency and science intensity.
Robustness analysis in different sub-groups
In order to check the robustness of our result, we repeat the experiments in several subgroups. In the first place, since technological disruptiveness is highly correlated to time (Bornmann & Tekles, 2019 ), all our econometric analyses include publication year dummies to account for the time differences. We additionally divide our data into three groups with different time durations to examine whether the relationship stands over time. The results shown in columns 1–3 of Table 3 demonstrate that the disruptive effect of science upon technology is robust and even more pronounced over time especially in recent years (in group of 2000–2007 and 2008–2015). The exception is that the U-shaped effect of science intensity upon technological disruptiveness is originally became negative in the group of patents published before 2000 ( β = -0.063, p < 0.001, column 3 of Table 3 ). It suggests that in the early stage of technological development, the effect of science participation in technology is not disruptive but consolidating which contributes to the formation of technological trajectory and reduces technological uncertainty.
Prior studies argue that successful knowledge transfer from science to technology is highly related to collaboration between different institutions, e.g. universities and industrial corporations (Li & Zhu, 2021 ). In consideration of this issue, we test the disruptive impact of science on technology in two groups of patents filed by one applicant or more applicants respectively. Results are significantly robust in both groups. More interestingly, the size of the estimated coefficients of most science-related variables are larger in collaborative patents than that in non-collaborating patents. This may be due to the fact that collaboration enhances knowledge exchange and absorptive capacity, thus making the disruptive effect of science on technology more pronounced.
Discussion and implication
Based on empirical analysis, we summarize the main findings and their implications as follows.
Main findings
(1) The disruptive impact of science upon technology is gradually non-negligible in the pharmaceutical field
According to descriptive analysis, we found that there are only a small proportion of pharmaceutical patents directly cite scientific references, accounting for 19% of all patents in the pharmaceutical field, which remains consistent with patents citing science across all fields at 21% as suggested in Ahmadpoor and Jones ( 2017 ). This figure illustrates that pharmaceuticals is a typical field about how technology interacts with science which may have general implications to other technical fields. More importantly, patents with scientific references have a higher level of forward citation and technological disruptiveness than their counterparts. Especially in terms of patent forward citation, this difference in the pharmaceutical field is higher than the average level across all fields (Ahmadpoor & Jones, 2017 ), indicating that the technological impact of science is much more pronounced in the pharmaceutical field.
Furthermore, scientific knowledge input cannot only enlarge the impact of technological invention but also disrupt technological development. Even though the technological disruptiveness is diminishing over time (“ Description analysis for disruptive impact of science upon technology ” section), pharmaceutical patents with scientific references always perform better than those without scientific references in recent decades in terms of technological disruptiveness (“ Description analysis for disruptive impact of science upon technology ” section). This result extends the conclusion of Park et al. ( 2023 ) by adding the disruptive impact of science in the context that patents are increasingly less likely to break with the past in ways that push science and technology in new directions. To this contribution, scientific knowledge participation could break the bottlenecks of the stagnant technology system and help technology development shift into new solutions, new insights and even new technological problems.
(2) Moderate knowledge convergence between science and technology contributes more to technological disruption
Based on the results of regression analysis, we find science intensity and science novelty both have a U-shaped relationship with technology disruptiveness. The nonlinear relationship indicates that only when scientific knowledge moderately combined with technological knowledge, science could make its contribution to disrupting technology. The moderate level not only reflected in the strength of knowledge convergence which is measured by science intensity, but also manifested in the quality of knowledge integration which is measured by science novelty.
In terms of science intensity, our results show that the initial increase in the scientific intensity has a positive effect on technological disruptiveness, but the effect turns negative after the scientific intensity reaches a threshold at about 33% (Tables 2 , 3 ). There are two mechanisms that can explain such results. Moderate connection with scientific knowledge can help technical staff quickly find new solutions for the focal technical challenges, but too much participation of science would reverse technical staff’s concentration on the basic research rather than technical problems, therefore impedes their R&D efficiency. That is, only the moderate convergence of scientific knowledge with technological knowledge shows the strongest impetus to technological disruption. This conclusion is similar to past literature (Du et al., 2019 ; Hohberger, 2016 ) which finds that moderate science participation could raise innovation performance, while conflicted with the conclusion of Della Malva et al. ( 2015 ) which suggests that basic science intensity does not increase the likelihood of technology breakthroughs. This difference may rely on different explanatory variables: technological breakthrough usually refers to the most impactful inventions which is essentially measured by forward patent citations, while disruption is more focused on how the subsequent patents use the focal patent.
In terms of science novelty, our results show that a lower level of science novelty under 0.55 could be helpful in disrupting technology but a higher level of science novelty would decrease the technological disruptiveness (Tables 2 , 3 ). The reasons for this U-shaped relationship can also be two-fold. On the one hand, searching for knowledge from a relatively unconventional scientific area could raise the opportunity to find some novel ideas and insights which can be useful in the focal domain, which corresponds with the insights in the literature regarding recombination novelty (Kok et al., 2020 ; Verhoeven et al., 2016 ). On the other hand, if technical staff search solutions from the scientific area with less relativeness or long distance, knowledge transformation could not only need a lot of effort but also has a high level of risk of being unadoptable to the focal problems. Furthermore, compared with science intensity and science recency, science novelty contributes the most to technological disruptiveness which underlines that technological disruption is more dependent on the quality of the input knowledge.
(3) Fresh scientific knowledge provides disruptive insights to technological development
We also find the linear negative impact of science recency upon technological disruptiveness. The results of description and regression analysis show time lag between the cited paper and the citing patent is approximately 6–7 years (Table 1 ) and more recent scientific advancement is more likely to disrupt technology development (Tables 2 , 3 ). These results are similar to the scientific impact of recent published scientific works. Scholars widely accepted that works citing literature with a low mean age have a higher potential to become scientific breakthroughs (Liang et al., 2020 ) or citation hotspots (Mukherjee et al., 2017 ). When it comes to the technological domain, fresh scientific knowledge could also provide new insights or new solutions and guide directional alternation for technological development, even though the time assume for knowledge transfer from science to technology is much longer.
(4) Collaboration makes the disruptive impact of science more pronounced
The robustness test shows that collaboration could significantly enlarge the disruptive impact of science on technology, evidenced by the increased size of science-related coefficients (Table 3 ). This result can be explained by the statement that collaboration provides access to distinct and novel knowledge not available in a single institution and prevents institutions from becoming locked-in, thus increasing the ability to develop new ideas and implement change (Boschma, 2005 ). Past literature demonstrates that collaboration can lead to, higher degrees of trust, and greater capacities for information sharing and mutual problem solving for individual inventors (Albats et al., 2022 ; Almeida et al., 2011 ). Our study argues that collaboration can improve the efficiency of knowledge transfer from science to technology, make prior scientific knowledge more applicable to technological development and thus facilitate the disruptive impact of science upon technology. This conclusion enhances our understanding of the impact of collaboration on innovation performance at the patent level.
Theoretical implications
Our work contributes to the ongoing understanding of the interaction between science and technology and contrasts with many earlier studies that examined the promoting role of science upon technology. Previous literature suggests that scientific knowledge could tackle technical issues and prompt technology development in a pre-defined trajectory (Cassiman & Veugelers, 2006 ; Tijssen & Winnink, 2016 ). This study makes a difference and finds out that scientific knowledge can destruct the technological system. Such difference is quite important as the disruptive impact of scientific knowledge inputs on technology has become more pronounced in the last decades, while the disruptive nature of technology is generally declining. Our study enriches the cross-domain knowledge transfer channel from science to technology by investigating the relationship between scientific input and technological disruptiveness, which complements the literature that only consider patent forward citation as the technological outcome of science (Cassiman et al., 2008 ; Fleming & Sorenson, 2004 ).
Furthermore, our study unpacks the disruptive impact upon technology at the patent level by introducing three scientific-related indicators. Much prior work in the innovation domain has only concentrated on the extent to which scientific knowledge participation would influence technological development (Du et al., 2019 ; Hohberger, 2016 ). Our study finds that the novelty of the input scientific knowledge plays a more significant role in disrupting technology rather than merely increasing the amount of scientific knowledge. In addition, the importance of science recency is also highlighted as it provides fresh and cutting-edge insights for developing disruptive technologies.
Practical implications
Our results provide practical implications for both practitioners and team leaders. First of all, we recommend that practitioners could expand the knowledge search scope in scientific areas to break technical bottlenecks. In this process, practitioners need to focus on the cutting-edge scientific theory and carefully select novel scientific knowledge, meanwhile appropriately accompanied with expertise technological knowledge to gain disruptive ideas. Particularly, practitioners should take care of the relevance, applicability and freshness of scientific knowledge upon the focal technical field, otherwise, costly knowledge transfer could lead to low profit.
Secondly, collaboration is crucial to improve the disruptiveness of technological invention. As collaboration could bring up knowledge diversity and increase the chances of knowledge recombination, team leaders should recruit more talents with different knowledge or educational backgrounds. Specifically, recruiting scientists in the R&D development team could increase the disruptive nature of technological products, while recruiting practitioners in the scientific community could also enhance the efficiency of the transformation of scientific achievements. It is also important to notice that a suitable coordinated strategy between scientists and practitioners should be carefully designed to guarantee successful knowledge recombination.
Given that science participation in technological development is becoming much more frequent in recent years, it is of great importance to understand by which pathways science knowledge will influence technology. Particularly, this study concerns about the disruptive impact of science on technology which is ignored by previous literature.
In order to trace the knowledge flow from science to technology, we employ patent-paper citations in the pharmaceutical field and compare the disruptive performance between two groups of patents with and without scientific references. The results show even though a general declining trend of patent disruptiveness, science always plays a crucial role in promoting technological disruptiveness at patent level. This result answers our first research question about whether or not science disrupt technology. To deeply investigate how does science disrupt technology, we develop science intensity, science novelty and science recency to qualify the degree to which science participates in technology development. The empirical results indicate that appropriate (moderate science intensity) and reasonable (moderate science novelty) knowledge coordination between science and technology could contribute more to technological disruptiveness. Recent published scientific knowledge always provides novel insights and open new solutions for technological development, therefore promote technological disruptiveness. Further robustness analysis in different time-span groups suggests the disruptive effect of science is gradually pronounced in recent years. The larger size of effects in collaboration group also demonstrates the knowledge diversity in a R&D team could improve the knowledge transfer efficiency from science to technology. This study not only contributes to the discussion of the interaction between science and technology by introducing the disruptive effect of science upon technology, but also provide practical implications for the R&D practitioners and leaders to facilitate their technological disruptive performance.
Our work is also subject to some limitations, which highlight avenues for further research. In the first place, the analysis is only limited in the pharmaceutical field which is a typical example with well-established science-technology linkages. The impact of science participation on technological disruptiveness may be different across technical fields. Further research could take fields differences into account and conclude more robust impact mechanisms from science to technological disruptiveness. In the second place, due to the disruptiveness is demonstrated as fluctuated with the citation windows (Bornmann & Tekles, 2019 ), but we only reserve 5 years to measure the technological disruptiveness. In the next research, we would test the association between scientific knowledge and technological disruptiveness in terms of different observed years.
Ahmadpoor, M., & Jones, B. F. (2017). The dual frontier: Patented inventions and prior scientific advance. Science, 357 (6351), 583–587.
Article Google Scholar
Albats, E., Alexander, A. T., & Cunningham, J. A. (2022). Traditional, virtual, and digital intermediaries in university-industry collaboration: Exploring institutional logics and bounded rationality. Technological Forecasting and Social Change, 177 , 121470.
Almeida, P., Hohberger, J., & Parada, P. (2011). Individual scientific collaborations and firm-level innovation. Industrial and Corporate Change, 20 (6), 1571–1599.
Arthur, W. B. (2007). The Structure of Invention. Research Policy, 36 (2), 274–287.
Birkinshaw, J., & Sheehan, T. (2002). Managing the knowledge life cycle. MIT Sloan Management Review, 2022 , 10.
Google Scholar
Bornmann, L., Devarakonda, S., Tekles, A., & Chacko, G. (2020). Disruptive papers published in scientometrics: Meaningful results by using an improved variant of the disruption index originally proposed by Wu, Wang, and Evans (2019). Scientometrics, 123 (2), 1149–1155.
Bornmann, L., & Tekles, A. (2019). Disruptive papers published in scientometrics. Scientometrics, 120 , 331–336.
Boschma, R. (2005). Proximity and innovation: A critical assessment. Regional Studies, 39 (1), 61–74.
Breschi, S., & Catalini, C. (2010). Tracing the links between science and technology: An exploratory analysis of scientists’ and inventors’ networks. Research Policy, 39 (1), 14–26.
Bush, V. (1990). Science–—the endless frontier: a report to the President on a program for postwar scientific research . National Science Foundation.
Callaert, J., Pellens, M., & Van Looy, B. (2014). Sources of inspiration? Making sense of scientific references in patents. Scientometrics, 98 , 1617–1629.
Cassiman, B., & Veugelers, R. (2006). In search of complementarity in innovation strategy: Internal R&D and external knowledge acquisition. Management Science, 52 (1), 68–82.
Cassiman, B., Veugelers, R., & Zuniga, P. (2008). In search of performance effects of (in) direct industry science links. Industrial and Corporate Change, 17 (4), 611–646.
Chen, J., Shao, D., & Fan, S. (2021). Destabilization and consolidation: Conceptualizing, measuring, and validating the dual characteristics of technology. Research Policy, 50 (1), 104115.
Chen, X., Ye, P., Huang, L., Wang, C., Cai, Y., Deng, L., & Ren, H. (2023). Exploring science-technology linkages: A deep learning-empowered solution. Information Processing & Management, 60 (2), 103255.
Colavizza, G., & Franceschet, M. (2016). Clustering citation histories in the Physical Review. Journal of Informetrics, 10 (4), 1037–1051.
Costas, R., van Leeuwen, T. N., & Bordons, M. (2012). Referencing patterns of individual researchers: Do top scientists rely on more extensive information sources? Journal of the American Society for Information Science and Technology, 63 (12), 2433–2450.
Costas, R., Van Leeuwen, T. N., & Van Raan, A. F. (2010). Is scientific literature subject to a ‘Sell-By-Date’? A general methodology to analyze the ‘durability’of scientific documents. Journal of the American Society for Information Science and Technology, 61 (2), 329–339.
Dechezleprêtre, A., Ménière, Y., & Mohnen, M. (2017). International patent families: From application strategies to statistical indicators. Scientometrics, 111 , 793–828.
Du, J., Li, P., Guo, Q., & Tang, X. (2019). Measuring the knowledge translation and convergence in pharmaceutical innovation by funding-science-technology-innovation linkages analysis. Journal of Informetrics, 13 (1), 132–148.
Ebadi, A., Auger, A., & Gauthier, Y. (2022). Detecting emerging technologies and their evolution using deep learning and weak signal analysis. Journal of Informetrics, 16 (4), 101344.
Ferguson, J. P., & Carnabuci, G. (2017). Risky recombinations: Institutional gatekeeping in the innovation process. Organization Science, 28 (1), 133–151.
Finardi, U. (2011). Time relations between scientific production and patenting of knowledge: The case of nanotechnologies. Scientometrics, 89 (1), 37–50.
Fleming, L., & Sorenson, O. (2004). Science as a map in technological search. Strategic Management Journal, 25 (8–9), 909–928.
Foster, J. G., Rzhetsky, A., & Evans, J. A. (2015). Tradition and innovation in scientists’ research strategies. American Sociological Review, 80 (5), 875–908.
Funk, R. J., & Owen-Smith, J. (2017). A dynamic network measure of technological change. Management Science, 63 (3), 791–817.
Gittelman, M., & Kogut, B. (2003). Does good science lead to valuable knowledge? Biotechnology firms and the evolutionary logic of citation patterns. Management Science, 49 (4), 366–382.
Gu, D., Li, J., Li, X., & Liang, C. (2017). Visualizing the knowledge structure and evolution of big data research in healthcare informatics. International Journal of Medical Informatics, 98 , 22–32.
Hiadlovsky, V., Hunady, J., Orviska, M., & Pisar, P. (2018). Research activities and their relation to economic performance of regions in the European Union. Business Systems Research: International Journal of the Society for Advancing Innovation and Research in Economy, 9 (1), 44–54.
Hohberger, J. (2016). Diffusion of science-based inventions. Technological Forecasting and Social Change, 104 , 66–77.
Kabore, F. P., & Park, W. G. (2019). Can patent family size and composition signal patent value? Applied Economics, 51 (60), 6476–6496.
Ke, Q. (2020). Technological impact of biomedical research: The role of basicness and novelty. Research Policy, 49 (7), 104071.
Klitkou, A., & Gulbrandsen, M. (2010). The relationship between academic patenting and scientific publishing in Norway. Scientometrics, 82 (1), 93–108.
Kok, H., Faems, D., & de Faria, P. (2020). Ties that matter: The impact of alliance partner knowledge recombination novelty on knowledge utilization in R&D alliances. Research Policy, 49 (7), 104011.
Kong, X., Jiang, H., Wang, W., Bekele, T. M., Xu, Z., & Wang, M. (2017). Exploring dynamic research interest and academic influence for scientific collaborator recommendation. Scientometrics, 113 , 369–385.
Kuhn, T. S. (2012). The structure of scientific revolutions . University of Chicago Press.
Book Google Scholar
Lee, C., Park, G., & Kang, J. (2018). The impact of convergence between science and technology on innovation. The Journal of Technology Transfer, 43 , 522–544.
Leten, B., Kelchtermans, S., & Belderbos, R. (2022). How does basic research improve innovation performance in the world’s major pharmaceutical firms? Industry and Innovation, 29 (3), 396–424.
Li, J., & Shi, D. (2016). Sleeping beauties in genius work: When were they awakened? Journal of the Association for Information Science and Technology, 67 (2), 432–440.
Li, Z., & Zhu, G. (2021). Knowledge transfer performance of industry-university-research institute collaboration in China: The moderating effect of partner difference. Sustainability, 13 (23), 13202.
Liang, G., Hou, H., Ding, Y., & Hu, Z. (2020). Knowledge recency to the birth of Nobel Prize-winning articles: Gender, career stage, and country. Journal of Informetrics, 14 (3), 101053.
Liu, N., Mao, J., & Guan, J. (2020). Knowledge convergence and organization innovation: The moderating role of relational embeddedness. Scientometrics, 125 (3), 1899–1921.
Malva, A. D., Kelchtermans, S., Leten, B., & Veugelers, R. (2015). Basic science as a prescription for breakthrough inventions in the pharmaceutical industry. The Journal of Technology Transfer, 40 , 670–695.
Marx, M., & Fuegi, A. (2020). Reliance on science: Worldwide front-page patent citations to scientific articles. Strategic Management Journal, 41 (9), 1572–1594.
Meyer, M., & Persson, O. (1998). Nanotechnology-interdisciplinarity, patterns of collaboration and differences in application. Scientometrics, 42 , 195–205.
Mukherjee, S., Romero, D. M., Jones, B., & Uzzi, B. (2017). The nearly universal link between the age of past knowledge and tomorrow’s breakthroughs in science and technology: The hotspot. Science Advances, 3 (4), e1601315.
Nagaoka, S., & Yamauchi, I. (2015). The use of science for inventions and its identification: Patent level evidence matched with survey . Research Institute of Economy, Trade and Industry (RIETI).
Narin, F., Hamilton, K. S., & Olivastro, D. (1997). The increasing linkage between US technology and public science. Research Policy, 26 (3), 317–330.
Nerkar, A. (2003). Old is gold? The value of temporal exploration in the creation of new knowledge. Management Science, 49 (2), 211–229.
Milojević, S. (2012). How are academic age, productivity and collaboration related to citing behavior of researchers?. PloS one, 7(11), e49176.
Park, M., Leahey, E., & Funk, R. J. (2023). Papers and patents are becoming less disruptive over time. Nature, 613 (7942), 138–144.
Petruzzelli, A. M., Ardito, L., & Savino, T. (2018). Maturity of knowledge inputs and innovation value: The moderating effect of firm age and size. Journal of Business Research, 86 , 190–201.
Pezzoni, M., Veugelers, R., & Visentin, F. (2022). How fast is this novel technology going to be a hit? Antecedents Predicting Follow-on Inventions. Research Policy, 51 (3), 104454.
Rhodes, R. (2012). The making of the atomic bomb . Simon and Schuster.
Rizzo, U., Barbieri, N., Ramaciotti, L., & Iannantuono, D. (2020). The division of labour between academia and industry for the generation of radical inventions. The Journal of Technology Transfer, 45 , 393–413.
Roach, M., & Cohen, W. M. (2013). Lens or prism? Patent citations as a measure of knowledge flows from public research. Management Science, 59 (2), 504–525.
Rose-Wiles, L. M., & Marzabadi, C. (2018). What do chemists cite? A 5-year analysis of references cited in American Chemical Society journal articles. Science & Technology Libraries, 37 (3), 246–273.
Rotolo, D., Hicks, D., & Martin, B. R. (2015). What is an emerging technology? Research Policy, 44 (10), 1827–1843.
Ruan, X., Lyu, D., Gong, K., Cheng, Y., & Li, J. (2021). Rethinking the disruption index as a measure of scientific and technological advances. Technological Forecasting and Social Change, 172 , 121071.
Schoenmakers, W., & Duysters, G. (2010). The technological origins of radical inventions. Research Policy, 39 (8), 1051–1059.
Shane, S. (2001). Technological opportunities and new firm creation. Management Science, 47 (2), 205–220.
Simonton, D. K. (2003). Scientific creativity as constrained stochastic behavior: The integration of product, person, and process perspectives. Psychological Bulletin, 129 (4), 475.
Sinha, A., Shen, Z., Song, Y., Ma, H., Eide, D., Hsu, B. J., & Wang, K. (2015). An overview of microsoft academic service (mas) and applications. In Proceedings of the 24th international conference on world wide web (pp. 243–246).
Stephan, P., Veugelers, R., & Wang, J. (2017). Reviewers are blinkered by bibliometrics. Nature, 544 (7651), 411–412.
Stringer, M. J., Sales-Pardo, M., & Amaral, L. A. N. (2010). Statistical validation of a global model for the distribution of the ultimate number of citations accrued by papers published in a scientific journal. Journal of the American Society for Information Science and Technology, 61 (7), 1377–1385.
Tijssen, R. J., & Winnink, J. (2016). Twenty-first century macro-trends in the institutional fabric of science: Bibliometric monitoring and analysis. Scientometrics, 109 , 2181–2194.
Toole, A. A. (2012). The impact of public basic research on industrial innovation: Evidence from the pharmaceutical industry. Research Policy, 41 (1), 1–12.
Article MathSciNet Google Scholar
Tussen, R. J. W., Buter, R. K., & Van Leeuwen, T. N. (2000). Technological relevance of science: An assessment of citation linkages between patents and research papers. Scientometrics, 47 , 389–412.
Uzzi, B., Mukherjee, S., Stringer, M., & Jones, B. (2013). Atypical combinations and scientific impact. Science, 342 (6157), 468–472.
Van Looy, B., Zimmermann, E., Veugelers, R., Verbeek, A., & Debackere, K. (2002). Do science-technology interactions pay off when developing technology? An exploratory investigation of 10 science-intensive technology domains. DTEW Research Report, 0244 , 1–14.
van Raan, A. F., & Winnink, J. J. (2018). Do younger Sleeping Beauties prefer a technological prince? Scientometrics, 114 , 701–717.
Verhoeven, D., Bakker, J., & Veugelers, R. (2016). Measuring technological novelty with patent-based indicators. Research Policy, 45 (3), 707–723.
Veugelers, R., & Wang, J. (2019). Scientific novelty and technological impact. Research Policy, 48 (6), 1362–1372.
Wang, J., & Verberne, S. (2021). Two tales of science technology linkage: Patent in-text versus front-page references. Preprint retrieved from https://arxiv.org/abs/2103.08931
Wang, J., Veugelers, R., & Stephan, P. (2017). Bias against novelty in science: A cautionary tale for users of bibliometric indicators. Research Policy, 46 (8), 1416–1436.
Wu, L., Wang, D., & Evans, J. A. (2019). Large teams develop and small teams disrupt science and technology. Nature, 566 (7744), 378–382.
Wu, M. F., Chang, K. W., Zhou, W., Hao, J., Yuan, C. C., & Chang, K. C. (2015). Patent deployment strategies and patent value in LED industry. PLoS ONE, 10 (6), e0129911.
Xu, H., Winnink, J., Yue, Z., Liu, Z., & Yuan, G. (2020). Topic-linked innovation paths in science and technology. Journal of Informetrics, 14 (2), 101014.
Zhou, Y., Dong, F., Kong, D., & Liu, Y. (2019). Unfolding the convergence process of scientific knowledge for the early identification of emerging technologies. Technological Forecasting and Social Change, 144 , 205–220.
Download references
Open Access funding enabled and organized by CAUL and its Member Institutions. Funding was provided by China Scholarship Council (Grant No. 202306190108).
Author information
Authors and affiliations.
Laboratory of Data Intelligence and Interdisciplinary Innovation, Nanjing University, Nanjing, China
School of Information Management, Nanjing University, Nanjing, China
Keye Wu & Ziyue Xie
School of Information and Communication Studies, Charles Sturt University, Bathurst, Australia
Ziyue Xie & Jia Tina Du
UniSA STEM, University of South Australia, Adelaide, Australia
Jia Tina Du
You can also search for this author in PubMed Google Scholar
Contributions
Keye Wu prepared for the first draft. Material preparation, data collection and analysis were performed by Keye Wu, Ziyue Xie and Jia Tina Du. All authors contributed to the writing of the manuscript.
Corresponding author
Correspondence to Jia Tina Du .
Ethics declarations
Competing interests.
All authors certify that they have no affiliations with or involvement in any organization or entity with any financial interest or non-financial interest in the subject matter or materials discussed in this manuscript.
Additional information
Publisher's note.
Springer Nature remains neutral with regard to jurisdictional claims in published maps and institutional affiliations.
Rights and permissions
Open Access This article is licensed under a Creative Commons Attribution 4.0 International License, which permits use, sharing, adaptation, distribution and reproduction in any medium or format, as long as you give appropriate credit to the original author(s) and the source, provide a link to the Creative Commons licence, and indicate if changes were made. The images or other third party material in this article are included in the article's Creative Commons licence, unless indicated otherwise in a credit line to the material. If material is not included in the article's Creative Commons licence and your intended use is not permitted by statutory regulation or exceeds the permitted use, you will need to obtain permission directly from the copyright holder. To view a copy of this licence, visit http://creativecommons.org/licenses/by/4.0/ .
Reprints and permissions
About this article
Wu, K., Xie, Z. & Du, J.T. Does science disrupt technology? Examining science intensity, novelty, and recency through patent-paper citations in the pharmaceutical field. Scientometrics (2024). https://doi.org/10.1007/s11192-024-05126-9
Download citation
Received : 02 January 2024
Accepted : 26 July 2024
Published : 09 August 2024
DOI : https://doi.org/10.1007/s11192-024-05126-9
Share this article
Anyone you share the following link with will be able to read this content:
Sorry, a shareable link is not currently available for this article.
Provided by the Springer Nature SharedIt content-sharing initiative
- Technological disruptiveness
- Patent-paper citation
- Find a journal
- Publish with us
- Track your research
- College of Information and Communications
- Location Location
- Contact Contact
- Colleges and Schools
- School of Information Science
- Information Science News
Dreeszen Bowman named a Biliniski Dissertation Fellow
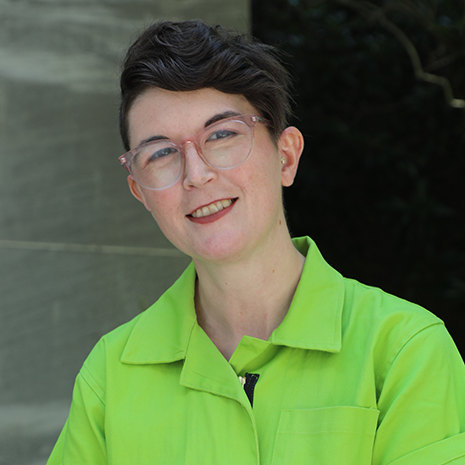
Rhys Dreeszen Bowman has been selected as a Biliniski Dissertation Fellow for the 2024-2025 academic year. During the fellowship, Dreeszen Bowman, who uses the pronoun they, will focus on completing their dissertation — From Margins to Center: Community-Based Action Research with Transgender Communities in South Carolina .
The College of Arts and Sciences manages the Russell J. and Dorothy S. Bilinski Fellowships which provide $40,000 to graduate students in the humanities and social sciences to support completion of their doctoral degrees.
Dreeszen Bowman is the first doctoral student from the School of Information Science to receive the award.
“Receiving this fellowship is a clear indication of the importance of Rhys’s dissertation work. It is timely and relevant to communities throughout the state of South Carolina,” says Lyda Fontes McCartin , director of the School of Information Science.
The main goal of Dreeszen Bowman’s dissertation is to understand how transgender individuals in South Carolina are able to find reliable information and what role libraries play in their ability to do so.
“I’m curious how this climate is impacting trans people’s ability to find reliable information and survive in this state,” Dreeszen Bowman says.
Dreeszen Bowman went on to explain how the Fellowship will allow them to fully focus on their dissertation, easing the financial strain of being a doctoral student.
“I’ve taught every year since I started the program and so being able to not have to teach — as much I love teaching and love working as a graduate assistant — but just being able to focus on my dissertation makes it feel possible to finish within the time that I’m funded by my program,” Dreeszen Bowman says.
Dreeszen Bowman plans to conduct community-based action research by recruiting an advisory board of five transgender people in Columbia to assist with the project. The committee will review Dreeszen Bowman’s research questions and collaborate in the creation of an informational health care resource to be used by transgender communities across the state.
By interviewing people across the state to get their viewpoints on their transgender healthcare ban for minors, Dreeszen Bowman hopes to make their dissertation reflect the issues faced by transgender people in both urban and rural communities.
“The interviews will be with people all across the state of South Carolina, and I’m really hoping that I can represent a broad racial diversity and also find rural folks. We know that people in rural communities in the South are facing a lot more barriers than people living in the cities, in these really regressive, political and religious climates where it can be very difficult to be trans, so I’m really hoping to be able to represent a rural perspective as well,” Dreeszen Bowman says.
After completing their dissertation, Dreeszen Bowman hopes to find employment in academia.
“I’m going to be applying for jobs , and I’m hoping to secure a job as a tenure track assistant professor at a university,” they say. “I’m hoping that being able to focus full time on the dissertation will give me the time I need to finish and also allow time for me to submit job applications.”
Challenge the conventional. Create the exceptional. No Limits.
- Request Info
- Browse Degrees
- Student Resources
- Faculty Resources
- Diversity, Equity & Inclusion
- Give to SLU
- Search & Directory
Field Exams and Thesis Information
M.A. students have special program requirements and opportunities, including sitting for a field exam and completing a master's thesis.
Political Science Field Exam
All students in Saint Louis University’s Master of Arts in Political Science program must sit for a field exam early during their last semester of coursework. The field exam has two parts. During the first three-hour segment, students choose one question to answer from a list of questions drawn from key debates in the literature of the student's concentration or track. During the second three-hour segment, students will choose one question to answer from a list provided by the exam committee. The exam committee is comprised of a chair and two other committee members. The chair must be in the student's examination field; ordinarily, the two committee members will be in the same field, as well. Students should choose committee members with the advice of the committee chair. Students should be prepared to engage explicitly with the relevant scholarly literature during their field exams. Students may have access to notes and other sources during the field exam but they are unlikely to do well on the exam if they rely heavily on those resources due to time limits. We advise that students develop effective strategies to retain and integrate the information learned in all their classes early on in their master’s program. All students must pass the written portion of the exam. An additional oral exam will be required at the discretion of the exam committee. If two members of the committee determine that the student has passed the field exam, a passing grade is warranted.
Cognate Field
Students in the Master of Arts in Political Science program may choose to complete some of their course work in a cognate field. A cognate field is made up of three courses (nine credit hours) in departments other than political science that are relevant to the student's areas of interests. Some possibilities for cognate fields include:
- Coursework toward a graduate certificate program, such as women's studies
- Graduate classes in another discipline, such as economics
- Three courses in different disciplines all touching on the same subject area, such as Asian studies
Political Science Master’s Thesis
The M.A. thesis is only an option for students in good standing, with no incompletes. All students must have the permission of the graduate committee and the thesis director before registering for M.A. thesis work. A master's thesis:
- Proposes an argument that addresses an important question to the discipline or area of study in question
- Grounds the argument thoroughly in the literature of this question
- Articulates what effect or contribution the argument of the thesis has on this literature
- Presents supporting evidence
The M.A. thesis is a two-semester project. Students complete a thesis proposal in the first semester and the thesis itself during the second semester. Students who are not able to defend their proposal successfully during the first semester will not be allowed to continue to the second semester of thesis work. The length of the thesis varies according to the nature of the project, but most theses fall between 40 and 75 pages. Students who write master's theses will work closely with a faculty director and a committee of two other faculty members chosen with the director's guidance. The oral defense of the M.A. thesis will be advertised and open to the public. All three members of the committee must approve the thesis for the student to pass.
Amgen PhD fellows gain industry experience while completing dissertations

University of Arizona Amgen Fellows clockwise from left: Danielle Johnson (’22), Marc Zempare (’23), Lauren Reyes (’22), Selina Kindelay (’23)
—Tucson, AZ—
University of Arizona PhD students Selina Kindelay and Marc Zempare hope more of their peers will benefit from the Amgen PhD Fellowship program, which enabled them to work in the company's world-class research laboratories while finishing their doctoral degrees. They agreed that the opportunity provided by Amgen, one of the early pioneers of the biotech industry, is helping to diversify and strengthen the nation's biotechnology workforce.
Internship opens doors to new fields for Selina Kindelay .
A Cellular & Molecular Medicine PhD student in the College of Medicine Tucson, Selina Kindelay joined a lab studying targeted protein degradation at Amgen's Thousand Oaks campus in October 2023. Her six-month project used mass spectrometry-based proteomics to study how proteins change when exposed to small molecule degraders.
The project required Kindelay to delve into computational biology and proteomics, fields she says she probably wouldn't have explored otherwise. "Coming in with no background in proteomics, or even computational biology, was a real stretch," she said, "but I wanted to challenge myself, and Amgen seemed happy to help me do that!" She described the experience as "a whole new education" that inspired her to pursue postdoctoral studies in precision medicine, focusing on using bioinformatics to understand disease variability and improve therapeutic design.
The structure of the Amgen PhD fellowship program compelled Kindelay to develop new skills and receive professional mentorship. Her Amgen mentor, Bo Zhou, a Senior Principal Scientist and Proteomics Group Leader, met with her weekly, offering invaluable input and career advice. Kindelay, who grew up on the San Carlos Apache reservation in southeastern Arizona, said many Indigenous young people would thrive with better access to resources and opportunities to interact with individuals who can broaden their horizons and introduce them to diverse career paths. "The mentoring I've received at the University of Arizona and now with Amgen has changed everything for me," she said.
In May 2024, Kindelay successfully defended her dissertation on developmental genetics in Drosophila , particularly the dynamics of ribosomal DNA.

University of Arizona Amgen Fellow: Selina Kindelay (’23)
Amgen welcomes Marc Zempare's passion for serving others .
Marc Zempare discovered neuroscience as an undergrad at the University of Arizona. "I took an animal behavior class in my junior year and loved everything about it," he said. Following graduation, he worked on campus as a technician for Carol Barnes, Regent's Professor, Psychology, Neurology & Neuroscience. Dr. Barnes's mentorship was invaluable, he said. "She pushed me to challenge myself and grow as a scientist." Barnes's guidance solidified his interest in research and led him to pursue his PhD in neuroscience through the University's Graduate Interdisciplinary Program (GIDP).
Zempare's doctoral work centers on the cognitive processes of learning and memory in normative aging and Alzheimer's disease. From January to July 2024, at Amgen's facilities in San Francisco, Zempare is complementing his campus research by using human pluripotent stem cells to screen interactions of various genes using CRISPR technology.
Like Kindelay, Zempare is thriving with the mentorship Amgen provides its fellows. Wilford Wang, PhD, Senior Scientist, Kaylee Choi, PhD, Senior Principal Scientist, and Peter Hodder, PhD, Executive Director and Head of Lead Discovery and Characterization, have all been generous in their time, said Zempare, meeting with him one-on-one regularly. "I feel extremely lucky to be part of this team, learning from such amazing scientists and incredible leaders," he said.
Zempare's internship has taken him out of the lab and into the community. For example, he was invited to speak to master's students from Cal State East Bay to encourage their pursuit of careers in STEM fields. "It's good to know that companies like Amgen will allow me to pursue my passion for service," he said. "Whatever I do and wherever I work, I want to be mentoring and helping others."
Zempare is already seeing results from Amgen's program to support members of communities underrepresented in STEM. "When I first started going to neuroscience conferences, I wouldn't see a lot of African-American men like me," he said. "Nowadays, I see so many young scientists of African-American background and different backgrounds at those events. The field is changing, and it's exciting and encouraging."

University of Arizona Amgen Fellow: Marc Zempare (’23)
The synergy of industry-academia partnerships.
Frans Tax, University of Arizona Associate Dean of Student Affairs and Professor of Molecular & Cellular Biology, is familiar with the generous returns that come when institutions collaborate to help underrepresented students with robust funding and wise mentoring. As director for the Initiative for Maximizing Student Diversity (IMSD) program , dozens of IMSD students are now among the leading ranks of industry, government and academia. “The relationship with Amgen has been everything we might have asked for, and then some! Amgen has been a great partner in giving our students a taste of conducting cutting-edge biotech research, and the mentorship experience for the students has been amazing. Our students receive training, advice and connections that will benefit them for the rest of their professional careers.”
Brian Adair, Executive Director for Industry Engagement in U of A’s Research Development Services unit, said, “I’m pleased to announce that Amgen recently funded a third year of the fellowship program. U of A PhD student Hannah Ortiz will join Amgen’s state-of-the-art R&D site in South San Francisco for 6-month fellowship starting in September 2024. We couldn’t be more pleased that Arizona students get access to world-class mentors, resources, and training from an industry leader as they prepare for careers in biotech.”

Catalysis Science & Technology
New mononuclear cu( i ) compounds: synthesis, characterization, and application to the electroreduction of co 2 †.

* Corresponding authors
a Facultad de Química, Universidad Nacional Autónoma de México, Circuito Interior, Ciudad Universitaria, Mexico City 04510, Mexico E-mail: [email protected]
This report includes the preparation of a new set of well-defined Cu( I ) catalytic precursors of the type [Cu(diphosphine)(PPh 3 )NO 3 ] and [Cu(diphosphine)NO 3 ], fully characterized by regular analytical methods, including single-crystal XRD (X-ray diffraction). The new compounds were assessed to activate CO 2 in an electrocatalytic process to yield oxalate selectively and with a relatively low overpotential. Some mechanistic insights into this process are also provided; oxalate is a valuable product for further chemical applications.
Supplementary files
- Supplementary information PDF (8413K)
- Crystal structure data CIF (2491K)
Article information

Download Citation
Permissions.

New mononuclear Cu( I ) compounds: synthesis, characterization, and application to the electroreduction of CO 2
A. Arévalo, E. Juárez-Francisco, D. A. Roa, M. Flores-Alamo and J. J. García, Catal. Sci. Technol. , 2024, Advance Article , DOI: 10.1039/D4CY00759J
This article is licensed under a Creative Commons Attribution-NonCommercial 3.0 Unported Licence . You can use material from this article in other publications, without requesting further permission from the RSC, provided that the correct acknowledgement is given and it is not used for commercial purposes.
To request permission to reproduce material from this article in a commercial publication , please go to the Copyright Clearance Center request page .
If you are an author contributing to an RSC publication, you do not need to request permission provided correct acknowledgement is given.
If you are the author of this article, you do not need to request permission to reproduce figures and diagrams provided correct acknowledgement is given. If you want to reproduce the whole article in a third-party commercial publication (excluding your thesis/dissertation for which permission is not required) please go to the Copyright Clearance Center request page .
Read more about how to correctly acknowledge RSC content .
Social activity
Search articles by author.
This article has not yet been cited.
Advertisements
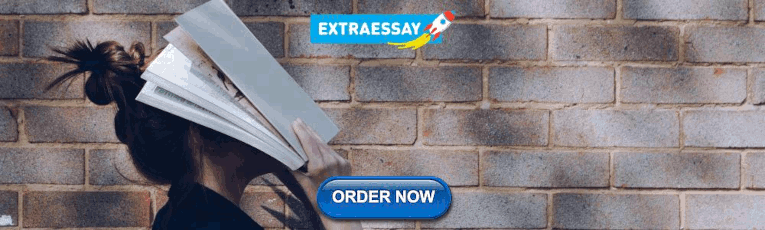
IMAGES
COMMENTS
A full understanding of the points of scientific papers is necessary and important for students and young researchers to prepare high-quality manuscripts. Herein, the three key points of novelty, accuracy, and standardization are focused on to help unlock the potential of every scientific study. Previousarticlein issue.
The scientific novelty score of each doctoral thesis is then quantified as the fraction of novel entity pairs out of the total number of entity pairs within the thesis. (2) We utilize Welch's t -tests and linear regression analyses to explore the temporal pattern of scientific novelty in doctoral theses over the previous decades.
Fig. 1 shows the overall process of the proposed analytical framework. The framework is designed to be executed in four discrete steps: (1) data collection and pre-processing using data scraping and parsing techniques; (2) construction of a paper (and word) landscape using fastText; (3) measurement of the novelty of scientific knowledge implied in the papers using the LOF; and (4) validation.
To measure the novelty of individual scientific papers, this study adopts a novelty indicator based on the combination-based novelty measure proposed by Dahlin and Behrens ().To assess the novelty of patents, Dahlin and Behrens proposed quantifying the degree of citation similarity between a focal patent and prior arts in the same technological domain to capture unusual knowledge recombination.
Novelty is a core value in science, and a reliable measurement of novelty is crucial. This study proposes a new approach of measuring the novelty of scientific articles based on both citation data and text data. The proposed approach considers an article to be novel if it cites a combination of semantically distant references. To this end, we first assign a word embedding-a vector ...
tion between team size and novelty, while there is a continuous increasing relation between team size and impact. In addition, novelty is likely to be driven by knowledge variety, while impact is affected by team size. Novelty in scientific publications is involved with new ideas and contributions that often come across disciplines [23].
Publications are an important means of sharing scientific results, which are enhanced by the peer review process. By providing context for evaluations of novelty and significance, the empty review can be prevented and bring a satisfying peer review experience to editors, authors, and the scientific community in its entirety.
1. INTRODUCTION. Scientific novelty advances knowledge frontier and drives technological innovation. One of the key issues in the science of science is how scientific novelty origins and develops (Fortunato et al., 2018; Uzzi et al., 2013).Driven by the outbreak of COVID‐19, a particular issue of interest is the evolution of scientific novelty during unexpected crises beyond a more ...
Novelty in research: A common reason for manuscript rejection! We often hear back from reviewers and editors of scientific journals that a particular manuscript (original research, case report, series or letter to the editor) has not been accepted because it lacks novelty. Though disheartening, the reason for such a response from said reviewers ...
Answer: The best way to highlight the novelty in your study is by comparing it with the work that was done by others and pointing out the things that your study does which was never done before. To do this, you should first c onduct a thorough literature search to identify what is already known in your field of research and what are the gaps to ...
Novelty is a very important aspect of research. It is true that research has progressed tremendously in the past two decades due to the advent and accessibility of new technologies that enable goods and data sharing. Consequently, it might be difficult to find a topic about which nothing is known or no literature is available.
Below we explore ideas that will help you maximise the novelty effect in your submissions. a. New discovery. This comprises research on and reports of completely new discoveries. These can be new chemical elements, planets or other astrological phenomena, new species of flora or fauna, previously undiagnosed diseases, viruses etc.
The novelty of scientific or technological knowledge has a paradoxical dual implication. Highly novel ideas are subject to a higher risk of rejection by their evaluating audiences than incremental, "normal science" contributions. ... The ProQuest Dissertations and Theses database was then used to expand the sample of information theorists ...
demonstrating critically how and in what way their. research makes a meaningful contribution to the. body of knowledge. Conclusion The concept of originality in the. PhD is complex and multi ...
Abstract. Novelty is a core value in science, and a reliable measurement of novelty is crucial. This study proposes a new approach of measuring the novelty of scientific articles based on both citation data and text data. The proposed approach considers an article to be novel if it cites a combination of semantically distant references.
It is this novelty that contributes to scientific progress. Since the main aim of research is to unravel what is unknown or to challenge views or ideas that may or may not be based on sound scientific principles, this exclusivity of novel research therefore allows us to expand our horizon beyond the realms of known domains.
The dissertation is an actual independent scientific research, which has a complete form, is based on a sufficient empirical base, the conclusions and proposals contained in it have scientific novelty and practical significance. The content of the abstract corresponds to the content of the dissertation text.
original or unusual. Social: Novelty may be the shared experience of a new cultural. phenomenon or the subje ctive perception of an individual. Return to T echnology: Actually, Novelty is very ...
Scientific novelty is the essential driving force for research breakthroughs and innovation. However, little is known about how early-career scientists pursue novel research paths, and the gender disparities in this process. To address this research gap, this study investigates a comprehensive dataset of 279,424 doctoral theses in biomedical sciences authored by US Ph.D. graduates. Spanning ...
The mean value of science_novelty is 0.56 and the median value is 0.57, suggesting patents are more inclined to follow the established science-technology linkage rather than refer to the scientific knowledge from new scientific areas. The mean value of science recency is 10.33 (years), representing the average time lag of knowledge transfer ...
The scientific novelty score of each doctoral thesis is then quantified as the fraction of novel entity pairs relative to the total number of entity pairs within the thesis. (2) We utilize Welch's t-tests and linear regression analyses to explore the temporal pattern of scientific novelty in doctoral theses over the previous decades.
The dissertation is an actual independent scientific research, which has a complete form, is based on a sufficient empirical base, the conclusions and proposals contained in it have scientific ...
The observed strong positive association between scientific novelty and the existence of a direct and indirect technological impact raises the question whether, within the set of publications directly cited by patents, novel science still differs from non-novel science in the nature of its technological impact. To this end, we restrict the ...
Rhys Dreeszen Bowman has been selected as a Biliniski Dissertation Fellow for the 2024-2025 academic year. During the fellowship, Dreeszen Bowman, who uses the pronoun they, will focus on completing their dissertation — From Margins to Center: Community-Based Action Research with Transgender Communities in South Carolina.
Political Science Master's Thesis. The M.A. thesis is only an option for students in good standing, with no incompletes. All students must have the permission of the graduate committee and the thesis director before registering for M.A. thesis work. A master's thesis: Proposes an argument that addresses an important question to the discipline ...
University of Arizona Amgen Fellow: Marc Zempare ('23) The synergy of industry-academia partnerships. Frans Tax, University of Arizona Associate Dean of Student Affairs and Professor of Molecular & Cellular Biology, is familiar with the generous returns that come when institutions collaborate to help underrepresented students with robust funding and wise mentoring. As director for the ...
Spanning from 1980 to 2016, the data originates from the ProQuest Dissertations & Theses Database. This study aims to shed light on Ph.D. students' pursuit of scientific novelty in their doctoral theses and assess gender-related differences in this process. ... Liu, Bu et al., 2022a; Uzzi et al., 2013). Scientific novelty possesses the ...
This report includes the preparation of a new set of well-defined Cu(I) catalytic precursors of the type [Cu(diphosphine)(PPh 3)NO 3] and [Cu(diphosphine)NO 3], fully characterized by regular analytical methods, including single-crystal XRD (X-ray diffraction).The new compounds were assessed to activate CO 2 in an electrocatalytic process to yield oxalate selectively and with a relatively low ...