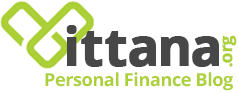
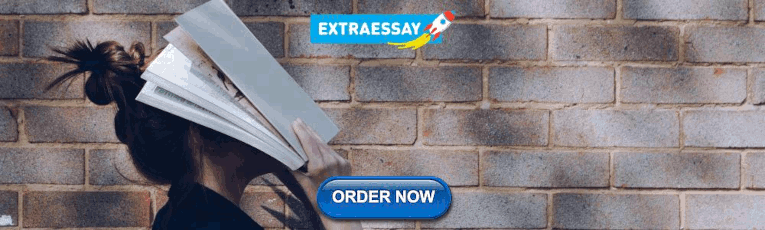
16 Advantages and Disadvantages of Experimental Research
How do you make sure that a new product, theory, or idea has validity? There are multiple ways to test them, with one of the most common being the use of experimental research. When there is complete control over one variable, the other variables can be manipulated to determine the value or validity that has been proposed.
Then, through a process of monitoring and administration, the true effects of what is being studied can be determined. This creates an accurate outcome so conclusions about the final value potential. It is an efficient process, but one that can also be easily manipulated to meet specific metrics if oversight is not properly performed.
Here are the advantages and disadvantages of experimental research to consider.
What Are the Advantages of Experimental Research?
1. It provides researchers with a high level of control. By being able to isolate specific variables, it becomes possible to determine if a potential outcome is viable. Each variable can be controlled on its own or in different combinations to study what possible outcomes are available for a product, theory, or idea as well. This provides a tremendous advantage in an ability to find accurate results.
2. There is no limit to the subject matter or industry involved. Experimental research is not limited to a specific industry or type of idea. It can be used in a wide variety of situations. Teachers might use experimental research to determine if a new method of teaching or a new curriculum is better than an older system. Pharmaceutical companies use experimental research to determine the viability of a new product.
3. Experimental research provides conclusions that are specific. Because experimental research provides such a high level of control, it can produce results that are specific and relevant with consistency. It is possible to determine success or failure, making it possible to understand the validity of a product, theory, or idea in a much shorter amount of time compared to other verification methods. You know the outcome of the research because you bring the variable to its conclusion.
4. The results of experimental research can be duplicated. Experimental research is straightforward, basic form of research that allows for its duplication when the same variables are controlled by others. This helps to promote the validity of a concept for products, ideas, and theories. This allows anyone to be able to check and verify published results, which often allows for better results to be achieved, because the exact steps can produce the exact results.
5. Natural settings can be replicated with faster speeds. When conducting research within a laboratory environment, it becomes possible to replicate conditions that could take a long time so that the variables can be tested appropriately. This allows researchers to have a greater control of the extraneous variables which may exist as well, limiting the unpredictability of nature as each variable is being carefully studied.
6. Experimental research allows cause and effect to be determined. The manipulation of variables allows for researchers to be able to look at various cause-and-effect relationships that a product, theory, or idea can produce. It is a process which allows researchers to dig deeper into what is possible, showing how the various variable relationships can provide specific benefits. In return, a greater understanding of the specifics within the research can be understood, even if an understanding of why that relationship is present isn’t presented to the researcher.
7. It can be combined with other research methods. This allows experimental research to be able to provide the scientific rigor that may be needed for the results to stand on their own. It provides the possibility of determining what may be best for a specific demographic or population while also offering a better transference than anecdotal research can typically provide.
What Are the Disadvantages of Experimental Research?
1. Results are highly subjective due to the possibility of human error. Because experimental research requires specific levels of variable control, it is at a high risk of experiencing human error at some point during the research. Any error, whether it is systemic or random, can reveal information about the other variables and that would eliminate the validity of the experiment and research being conducted.
2. Experimental research can create situations that are not realistic. The variables of a product, theory, or idea are under such tight controls that the data being produced can be corrupted or inaccurate, but still seem like it is authentic. This can work in two negative ways for the researcher. First, the variables can be controlled in such a way that it skews the data toward a favorable or desired result. Secondly, the data can be corrupted to seem like it is positive, but because the real-life environment is so different from the controlled environment, the positive results could never be achieved outside of the experimental research.
3. It is a time-consuming process. For it to be done properly, experimental research must isolate each variable and conduct testing on it. Then combinations of variables must also be considered. This process can be lengthy and require a large amount of financial and personnel resources. Those costs may never be offset by consumer sales if the product or idea never makes it to market. If what is being tested is a theory, it can lead to a false sense of validity that may change how others approach their own research.
4. There may be ethical or practical problems with variable control. It might seem like a good idea to test new pharmaceuticals on animals before humans to see if they will work, but what happens if the animal dies because of the experimental research? Or what about human trials that fail and cause injury or death? Experimental research might be effective, but sometimes the approach has ethical or practical complications that cannot be ignored. Sometimes there are variables that cannot be manipulated as it should be so that results can be obtained.
5. Experimental research does not provide an actual explanation. Experimental research is an opportunity to answer a Yes or No question. It will either show you that it will work or it will not work as intended. One could argue that partial results could be achieved, but that would still fit into the “No” category because the desired results were not fully achieved. The answer is nice to have, but there is no explanation as to how you got to that answer. Experimental research is unable to answer the question of “Why” when looking at outcomes.
6. Extraneous variables cannot always be controlled. Although laboratory settings can control extraneous variables, natural environments provide certain challenges. Some studies need to be completed in a natural setting to be accurate. It may not always be possible to control the extraneous variables because of the unpredictability of Mother Nature. Even if the variables are controlled, the outcome may ensure internal validity, but do so at the expense of external validity. Either way, applying the results to the general population can be quite challenging in either scenario.
7. Participants can be influenced by their current situation. Human error isn’t just confined to the researchers. Participants in an experimental research study can also be influenced by extraneous variables. There could be something in the environment, such an allergy, that creates a distraction. In a conversation with a researcher, there may be a physical attraction that changes the responses of the participant. Even internal triggers, such as a fear of enclosed spaces, could influence the results that are obtained. It is also very common for participants to “go along” with what they think a researcher wants to see instead of providing an honest response.
8. Manipulating variables isn’t necessarily an objective standpoint. For research to be effective, it must be objective. Being able to manipulate variables reduces that objectivity. Although there are benefits to observing the consequences of such manipulation, those benefits may not provide realistic results that can be used in the future. Taking a sample is reflective of that sample and the results may not translate over to the general population.
9. Human responses in experimental research can be difficult to measure. There are many pressures that can be placed on people, from political to personal, and everything in-between. Different life experiences can cause people to react to the same situation in different ways. Not only does this mean that groups may not be comparable in experimental research, but it also makes it difficult to measure the human responses that are obtained or observed.
The advantages and disadvantages of experimental research show that it is a useful system to use, but it must be tightly controlled in order to be beneficial. It produces results that can be replicated, but it can also be easily influenced by internal or external influences that may alter the outcomes being achieved. By taking these key points into account, it will become possible to see if this research process is appropriate for your next product, theory, or idea.
How to Write Limitations of the Study (with examples)
This blog emphasizes the importance of recognizing and effectively writing about limitations in research. It discusses the types of limitations, their significance, and provides guidelines for writing about them, highlighting their role in advancing scholarly research.
Updated on August 24, 2023

No matter how well thought out, every research endeavor encounters challenges. There is simply no way to predict all possible variances throughout the process.
These uncharted boundaries and abrupt constraints are known as limitations in research . Identifying and acknowledging limitations is crucial for conducting rigorous studies. Limitations provide context and shed light on gaps in the prevailing inquiry and literature.
This article explores the importance of recognizing limitations and discusses how to write them effectively. By interpreting limitations in research and considering prevalent examples, we aim to reframe the perception from shameful mistakes to respectable revelations.
What are limitations in research?
In the clearest terms, research limitations are the practical or theoretical shortcomings of a study that are often outside of the researcher’s control . While these weaknesses limit the generalizability of a study’s conclusions, they also present a foundation for future research.
Sometimes limitations arise from tangible circumstances like time and funding constraints, or equipment and participant availability. Other times the rationale is more obscure and buried within the research design. Common types of limitations and their ramifications include:
- Theoretical: limits the scope, depth, or applicability of a study.
- Methodological: limits the quality, quantity, or diversity of the data.
- Empirical: limits the representativeness, validity, or reliability of the data.
- Analytical: limits the accuracy, completeness, or significance of the findings.
- Ethical: limits the access, consent, or confidentiality of the data.
Regardless of how, when, or why they arise, limitations are a natural part of the research process and should never be ignored . Like all other aspects, they are vital in their own purpose.
Why is identifying limitations important?
Whether to seek acceptance or avoid struggle, humans often instinctively hide flaws and mistakes. Merging this thought process into research by attempting to hide limitations, however, is a bad idea. It has the potential to negate the validity of outcomes and damage the reputation of scholars.
By identifying and addressing limitations throughout a project, researchers strengthen their arguments and curtail the chance of peer censure based on overlooked mistakes. Pointing out these flaws shows an understanding of variable limits and a scrupulous research process.
Showing awareness of and taking responsibility for a project’s boundaries and challenges validates the integrity and transparency of a researcher. It further demonstrates the researchers understand the applicable literature and have thoroughly evaluated their chosen research methods.
Presenting limitations also benefits the readers by providing context for research findings. It guides them to interpret the project’s conclusions only within the scope of very specific conditions. By allowing for an appropriate generalization of the findings that is accurately confined by research boundaries and is not too broad, limitations boost a study’s credibility .
Limitations are true assets to the research process. They highlight opportunities for future research. When researchers identify the limitations of their particular approach to a study question, they enable precise transferability and improve chances for reproducibility.
Simply stating a project’s limitations is not adequate for spurring further research, though. To spark the interest of other researchers, these acknowledgements must come with thorough explanations regarding how the limitations affected the current study and how they can potentially be overcome with amended methods.
How to write limitations
Typically, the information about a study’s limitations is situated either at the beginning of the discussion section to provide context for readers or at the conclusion of the discussion section to acknowledge the need for further research. However, it varies depending upon the target journal or publication guidelines.
Don’t hide your limitations
It is also important to not bury a limitation in the body of the paper unless it has a unique connection to a topic in that section. If so, it needs to be reiterated with the other limitations or at the conclusion of the discussion section. Wherever it is included in the manuscript, ensure that the limitations section is prominently positioned and clearly introduced.
While maintaining transparency by disclosing limitations means taking a comprehensive approach, it is not necessary to discuss everything that could have potentially gone wrong during the research study. If there is no commitment to investigation in the introduction, it is unnecessary to consider the issue a limitation to the research. Wholly consider the term ‘limitations’ and ask, “Did it significantly change or limit the possible outcomes?” Then, qualify the occurrence as either a limitation to include in the current manuscript or as an idea to note for other projects.
Writing limitations
Once the limitations are concretely identified and it is decided where they will be included in the paper, researchers are ready for the writing task. Including only what is pertinent, keeping explanations detailed but concise, and employing the following guidelines is key for crafting valuable limitations:
1) Identify and describe the limitations : Clearly introduce the limitation by classifying its form and specifying its origin. For example:
- An unintentional bias encountered during data collection
- An intentional use of unplanned post-hoc data analysis
2) Explain the implications : Describe how the limitation potentially influences the study’s findings and how the validity and generalizability are subsequently impacted. Provide examples and evidence to support claims of the limitations’ effects without making excuses or exaggerating their impact. Overall, be transparent and objective in presenting the limitations, without undermining the significance of the research.
3) Provide alternative approaches for future studies : Offer specific suggestions for potential improvements or avenues for further investigation. Demonstrate a proactive approach by encouraging future research that addresses the identified gaps and, therefore, expands the knowledge base.
Whether presenting limitations as an individual section within the manuscript or as a subtopic in the discussion area, authors should use clear headings and straightforward language to facilitate readability. There is no need to complicate limitations with jargon, computations, or complex datasets.
Examples of common limitations
Limitations are generally grouped into two categories , methodology and research process .
Methodology limitations
Methodology may include limitations due to:
- Sample size
- Lack of available or reliable data
- Lack of prior research studies on the topic
- Measure used to collect the data
- Self-reported data

The researcher is addressing how the large sample size requires a reassessment of the measures used to collect and analyze the data.
Research process limitations
Limitations during the research process may arise from:
- Access to information
- Longitudinal effects
- Cultural and other biases
- Language fluency
- Time constraints

The author is pointing out that the model’s estimates are based on potentially biased observational studies.
Final thoughts
Successfully proving theories and touting great achievements are only two very narrow goals of scholarly research. The true passion and greatest efforts of researchers comes more in the form of confronting assumptions and exploring the obscure.
In many ways, recognizing and sharing the limitations of a research study both allows for and encourages this type of discovery that continuously pushes research forward. By using limitations to provide a transparent account of the project's boundaries and to contextualize the findings, researchers pave the way for even more robust and impactful research in the future.
Charla Viera, MS
See our "Privacy Policy"
Ensure your structure and ideas are consistent and clearly communicated
Pair your Premium Editing with our add-on service Presubmission Review for an overall assessment of your manuscript.
17 Advantages and Disadvantages of Experimental Research Method in Psychology
There are numerous research methods used to determine if theories, ideas, or even products have validity in a market or community. One of the most common options utilized today is experimental research. Its popularity is due to the fact that it becomes possible to take complete control over a single variable while conducting the research efforts. This process makes it possible to manipulate the other variables involved to determine the validity of an idea or the value of what is being proposed.
Outcomes through experimental research come through a process of administration and monitoring. This structure makes it possible for researchers to determine the genuine impact of what is under observation. It is a process which creates outcomes with a high degree of accuracy in almost any field.
The conclusion can then offer a final value potential to consider, making it possible to know if a continued pursuit of the information is profitable in some way.
The pros and cons of experimental research show that this process is highly efficient, creating data points for evaluation with speed and regularity. It is also an option that can be manipulated easily when researchers want their work to draw specific conclusions.
List of the Pros of Experimental Research
1. Experimental research offers the highest levels of control. The procedures involved with experimental research make it possible to isolate specific variables within virtually any topic. This advantage makes it possible to determine if outcomes are viable. Variables are controllable on their own or in combination with others to determine what can happen when each scenario is brought to a conclusion. It is a benefit which applies to ideas, theories, and products, offering a significant advantage when accurate results or metrics are necessary for progress.
2. Experimental research is useful in every industry and subject. Since experimental research offers higher levels of control than other methods which are available, it offers results which provide higher levels of relevance and specificity. The outcomes that are possible come with superior consistency as well. It is useful in a variety of situations which can help everyone involved to see the value of their work before they must implement a series of events.
3. Experimental research replicates natural settings with significant speed benefits. This form of research makes it possible to replicate specific environmental settings within the controls of a laboratory setting. This structure makes it possible for the experiments to replicate variables that would require a significant time investment otherwise. It is a process which gives the researchers involved an opportunity to seize significant control over the extraneous variables which may occur, creating limits on the unpredictability of elements that are unknown or unexpected when driving toward results.
4. Experimental research offers results which can occur repetitively. The reason that experimental research is such an effective tool is that it produces a specific set of results from documented steps that anyone can follow. Researchers can duplicate the variables used during the work, then control the variables in the same way to create an exact outcome that duplicates the first one. This process makes it possible to validate scientific discoveries, understand the effectiveness of a program, or provide evidence that products address consumer pain points in beneficial ways.
5. Experimental research offers conclusions which are specific. Thanks to the high levels of control which are available through experimental research, the results which occur through this process are usually relevant and specific. Researchers an determine failure, success, or some other specific outcome because of the data points which become available from their work. That is why it is easier to take an idea of any type to the next level with the information that becomes available through this process. There is always a need to bring an outcome to its natural conclusion during variable manipulation to collect the desired data.
6. Experimental research works with other methods too. You can use experimental research with other methods to ensure that the data received from this process is as accurate as possible. The results that researchers obtain must be able to stand on their own for verification to have findings which are valid. This combination of factors makes it possible to become ultra-specific with the information being received through these studies while offering new ideas to other research formats simultaneously.
7. Experimental research allows for the determination of cause-and-effect. Because researchers can manipulate variables when performing experimental research, it becomes possible to look for the different cause-and-effect relationships which may exist when pursuing a new thought. This process allows the parties involved to dig deeply into the possibilities which are present, demonstrating whatever specific benefits are possible when outcomes are reached. It is a structure which seeks to understand the specific details of each situation as a way to create results.
List of the Cons of Experimental Research
1. Experimental research suffers from the potential of human errors. Experimental research requires those involved to maintain specific levels of variable control to create meaningful results. This process comes with a high risk of experiencing an error at some stage of the process when compared to other options that may be available. When this issue goes unnoticed as the results become transferable, the data it creates will reflect a misunderstanding of the issue under observation. It is a disadvantage which could eliminate the value of any information that develops from this process.
2. Experimental research is a time-consuming process to endure. Experimental research must isolate each possible variable when a subject matter is being studied. Then it must conduct testing on each element under consideration until a resolution becomes possible, which then requires data collection to occur. This process must continue to repeat itself for any findings to be valid from the effort. Then combinations of variables must go through evaluation in the same manner. It is a field of research that sometimes costs more than the potential benefits or profits that are achievable when a favorable outcome is eventually reached.
3. Experimental research creates unrealistic situations that still receive validity. The controls which are necessary when performing experimental research increase the risks of the data becoming inaccurate or corrupted over time. It will still seem authentic to the researchers involved because they may not see that a variable is an unrealistic situation. The variables can skew in a specific direction if the information shifts in a certain direction through the efforts of the researchers involved. The research environment can also be extremely different than real-life circumstances, which can invalidate the value of the findings.
4. Experimental research struggles to measure human responses. People experience stress in uncountable ways during the average day. Personal drama, political arguments, and workplace deadlines can influence the data that researchers collect when measuring human response tendencies. What happens inside of a controlled situation is not always what happens in real-life scenarios. That is why this method is not the correct choice to use in group or individual settings where a human response requires measurement.
5. Experimental research does not always create an objective view. Objective research is necessary for it to provide effective results. When researchers have permission to manipulate variables in whatever way they choose, then the process increases the risk of a personal bias, unconscious or otherwise, influencing the results which are eventually obtained. People can shift their focus because they become uncomfortable, are aroused by the event, or want to manipulate the results for their personal agenda. Data samples are therefore only a reflection of that one group instead of offering data across an entire demographic.
6. Experimental research can experience influences from real-time events. The issue with human error in experimental research often involves the researchers conducting the work, but it can also impact the people being studied as well. Numerous outside variables can impact responses or outcomes without the knowledge of researchers. External triggers, such as the environment, political stress, or physical attraction can alter a person’s regular perspective without it being apparent. Internal triggers, such as claustrophobia or social interactions, can alter responses as well. It is challenging to know if the data collected through this process offers an element of honesty.
7. Experimental research cannot always control all of the variables. Although experimental research attempts to control every variable or combination that is possible, laboratory settings cannot reach this limitation in every circumstance. If data must be collected in a natural setting, then the risk of inaccurate information rises. Some research efforts place an emphasis on one set of variables over another because of a perceived level of importance. That is why it becomes virtually impossible in some situations to apply obtained results to the overall population. Groups are not always comparable, even if this process provides for more significant transferability than other methods of research.
8. Experimental research does not always seek to find explanations. The goal of experimental research is to answer questions that people may have when evaluating specific data points. There is no concern given to the reason why specific outcomes are achievable through this system. When you are working in a world of black-and-white where something works or it does not, there are many shades of gray in-between these two colors where additional information is waiting to be discovered. This method ignores that information, settling for whatever answers are found along the extremes instead.
9. Experimental research does not make exceptions for ethical or moral violations. One of the most significant disadvantages of experimental research is that it does not take the ethical or moral violations that some variables may create out of the situation. Some variables cannot be manipulated in ways that are safe for people, the environment, or even the society as a whole. When researchers encounter this situation, they must either transfer their data points to another method, continue on to produce incomplete results, fabricate results, or set their personal convictions aside to work on the variable anyway.
10. Experimental research may offer results which apply to only one situation. Although one of the advantages of experimental research is that it allows for duplication by others to obtain the same results, this is not always the case in every situation. There are results that this method can find which may only apply to that specific situation. If this process is used to determine highly detailed data points which require unique circumstances to obtain, then future researchers may find that result replication is challenging to obtain.
These experimental research pros and cons offer a useful system that can help determine the validity of an idea in any industry. The only way to achieve this advantage is to place tight controls over the process, and then reduce any potential for bias within the system to appear. This makes it possible to determine if a new idea of any type offers current or future value.

8 Advantages and Disadvantages of Experimental Research
Experimental research has become an important part of human life. Babies conduct their own rudimentary experiments (such as putting objects in their mouth) to learn about the world around them, while older children and teens conduct experiments at school to learn more science. Ancient scientists used experimental research to prove their hypotheses correct; Galileo Galilei and Antoine Lavoisier, for instance, did various experiments to uncover key concepts in physics and chemistry, respectively. The same goes for modern experts, who utilize this scientific method to see if new drugs are effective, discover treatments for illnesses, and create new electronic gadgets (among others).
Experimental research clearly has its advantages, but is it really a perfect way to verify and validate scientific concepts? Many people point out that it has several disadvantages and might even be harmful to subjects in some cases. To learn more about these, let’s take a look into the pros and cons of this type of procedure.
List of Advantages of Experimental Research
1. It gives researchers a high level of control. When people conduct experimental research, they can manipulate the variables so they can create a setting that lets them observe the phenomena they want. They can remove or control other factors that may affect the overall results, which means they can narrow their focus and concentrate solely on two or three variables.
In the pharmaceutical industry, for example, scientists conduct studies in which they give a new kind drug to a group of subjects and a placebo drug to another group. They then give the same kind of food to the subjects and even house them in the same area to ensure that they won’t be exposed to other factors that may affect how the drugs work. At the end of the study, the researchers analyze the results to see how the new drug affects the subjects and identify its side effects and adverse results.
2. It allows researchers to utilize many variations. As mentioned above, researchers have almost full control when they conduct experimental research studies. This lets them manipulate variables and use as many (or as few) variations as they want to create an environment where they can test their hypotheses — without destroying the validity of the research design. In the example above, the researchers can opt to add a third group of subjects (in addition to the new drug group and the placebo group), who would be given a well-known and widely available drug that has been used by many people for years. This way, they can compare how the new drug performs compared to the placebo drug as well as the widely used drug.
3. It can lead to excellent results. The very nature of experimental research allows researchers to easily understand the relationships between the variables, the subjects, and the environment and identify the causes and effects in whatever phenomena they’re studying. Experimental studies can also be easily replicated, which means the researchers themselves or other scientists can repeat their studies to confirm the results or test other variables.
4. It can be used in different fields. Experimental research is usually utilized in the medical and pharmaceutical industries to assess the effects of various treatments and drugs. It’s also used in other fields like chemistry, biology, physics, engineering, electronics, agriculture, social science, and even economics.
List of Disadvantages of Experimental Research
1. It can lead to artificial situations. In many scenarios, experimental researchers manipulate variables in an attempt to replicate real-world scenarios to understand the function of drugs, gadgets, treatments, and other new discoveries. This works most of the time, but there are cases when researchers over-manipulate their variables and end up creating an artificial environment that’s vastly different from the real world. The researchers can also skewer the study to fit whatever outcome they want (intentionally or unintentionally) and compromise the results of the research.
2. It can take a lot of time and money. Experimental research can be costly and time-consuming, especially if the researchers have to conduct numerous studies to test each variable. If the studies are supported by the government, they would consume millions or even billions of taxpayers’ dollars, which could otherwise have been spent on other community projects such as education, housing, and healthcare. If the studies are privately funded, they can be a huge burden on the companies involved who, in turn, would pass on the costs to the customers. As a result, consumers have to spend a large amount if they want to avail of these new treatments, gadgets, and other innovations.
3. It can be affected by errors. Just like any kind of research, experimental research isn’t always perfect. There might be blunders in the research design or in the methodology as well as random mistakes that can’t be controlled or predicted, which can seriously affect the outcome of the study and require the researchers to start all over again.
There might also be human errors; for instance, the researchers may allow their personal biases to affect the study. If they’re conducting a double-blind study (in which both the researchers and the subjects don’t know which the control group is), the researchers might be made aware of which subjects belong to the control group, destroying the validity of the research. The subjects may also make mistakes. There have been cases (particularly in social experiments) in which the subjects give answers that they think the researchers want to hear instead of truthfully saying what’s on their mind.
4. It might not be feasible in some situations. There are times when the variables simply can’t be manipulated or when the researchers need an impossibly large amount of money to conduct the study. There are also cases when the study would impede on the subjects’ human rights and/or would give rise to ethical issues. In these scenarios, it’s better to choose another kind of research design (such as review, meta-analysis, descriptive, or correlational research) instead of insisting on using the experimental research method.
Experimental research has become an important part of the history of the world and has led to numerous discoveries that have made people’s lives better, longer, and more comfortable. However, it can’t be denied that it also has its disadvantages, so it’s up to scientists and researchers to find a balance between the benefits it provides and the drawbacks it presents.
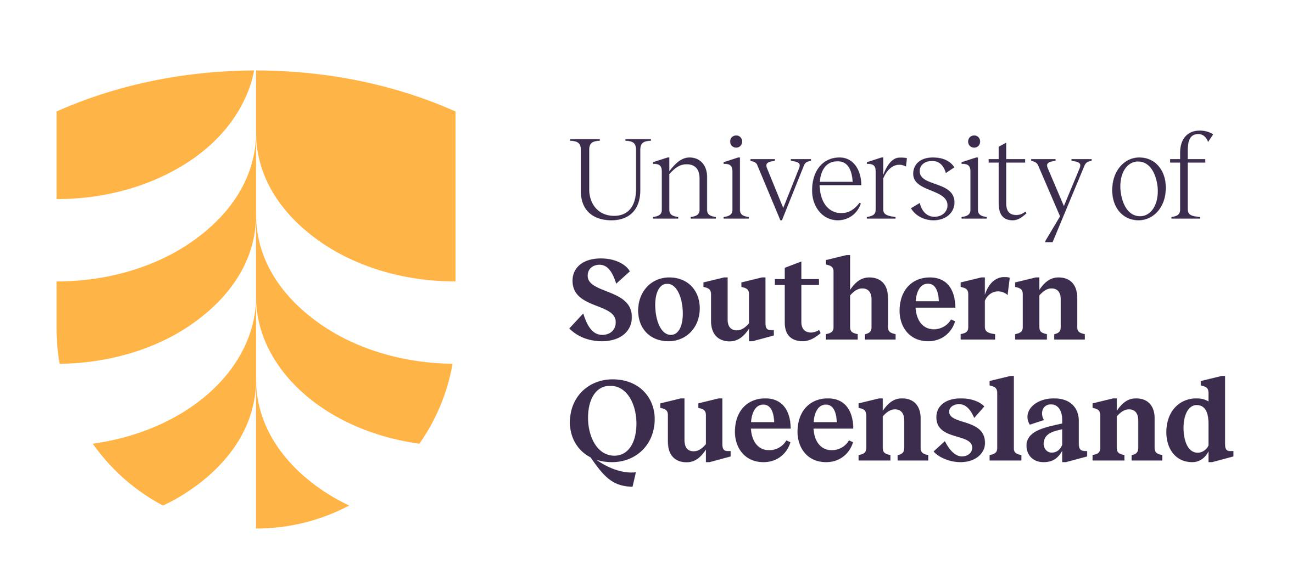
Want to create or adapt books like this? Learn more about how Pressbooks supports open publishing practices.
10 Experimental research
Experimental research—often considered to be the ‘gold standard’ in research designs—is one of the most rigorous of all research designs. In this design, one or more independent variables are manipulated by the researcher (as treatments), subjects are randomly assigned to different treatment levels (random assignment), and the results of the treatments on outcomes (dependent variables) are observed. The unique strength of experimental research is its internal validity (causality) due to its ability to link cause and effect through treatment manipulation, while controlling for the spurious effect of extraneous variable.
Experimental research is best suited for explanatory research—rather than for descriptive or exploratory research—where the goal of the study is to examine cause-effect relationships. It also works well for research that involves a relatively limited and well-defined set of independent variables that can either be manipulated or controlled. Experimental research can be conducted in laboratory or field settings. Laboratory experiments , conducted in laboratory (artificial) settings, tend to be high in internal validity, but this comes at the cost of low external validity (generalisability), because the artificial (laboratory) setting in which the study is conducted may not reflect the real world. Field experiments are conducted in field settings such as in a real organisation, and are high in both internal and external validity. But such experiments are relatively rare, because of the difficulties associated with manipulating treatments and controlling for extraneous effects in a field setting.
Experimental research can be grouped into two broad categories: true experimental designs and quasi-experimental designs. Both designs require treatment manipulation, but while true experiments also require random assignment, quasi-experiments do not. Sometimes, we also refer to non-experimental research, which is not really a research design, but an all-inclusive term that includes all types of research that do not employ treatment manipulation or random assignment, such as survey research, observational research, and correlational studies.
Basic concepts
Treatment and control groups. In experimental research, some subjects are administered one or more experimental stimulus called a treatment (the treatment group ) while other subjects are not given such a stimulus (the control group ). The treatment may be considered successful if subjects in the treatment group rate more favourably on outcome variables than control group subjects. Multiple levels of experimental stimulus may be administered, in which case, there may be more than one treatment group. For example, in order to test the effects of a new drug intended to treat a certain medical condition like dementia, if a sample of dementia patients is randomly divided into three groups, with the first group receiving a high dosage of the drug, the second group receiving a low dosage, and the third group receiving a placebo such as a sugar pill (control group), then the first two groups are experimental groups and the third group is a control group. After administering the drug for a period of time, if the condition of the experimental group subjects improved significantly more than the control group subjects, we can say that the drug is effective. We can also compare the conditions of the high and low dosage experimental groups to determine if the high dose is more effective than the low dose.
Treatment manipulation. Treatments are the unique feature of experimental research that sets this design apart from all other research methods. Treatment manipulation helps control for the ‘cause’ in cause-effect relationships. Naturally, the validity of experimental research depends on how well the treatment was manipulated. Treatment manipulation must be checked using pretests and pilot tests prior to the experimental study. Any measurements conducted before the treatment is administered are called pretest measures , while those conducted after the treatment are posttest measures .
Random selection and assignment. Random selection is the process of randomly drawing a sample from a population or a sampling frame. This approach is typically employed in survey research, and ensures that each unit in the population has a positive chance of being selected into the sample. Random assignment, however, is a process of randomly assigning subjects to experimental or control groups. This is a standard practice in true experimental research to ensure that treatment groups are similar (equivalent) to each other and to the control group prior to treatment administration. Random selection is related to sampling, and is therefore more closely related to the external validity (generalisability) of findings. However, random assignment is related to design, and is therefore most related to internal validity. It is possible to have both random selection and random assignment in well-designed experimental research, but quasi-experimental research involves neither random selection nor random assignment.
Threats to internal validity. Although experimental designs are considered more rigorous than other research methods in terms of the internal validity of their inferences (by virtue of their ability to control causes through treatment manipulation), they are not immune to internal validity threats. Some of these threats to internal validity are described below, within the context of a study of the impact of a special remedial math tutoring program for improving the math abilities of high school students.
History threat is the possibility that the observed effects (dependent variables) are caused by extraneous or historical events rather than by the experimental treatment. For instance, students’ post-remedial math score improvement may have been caused by their preparation for a math exam at their school, rather than the remedial math program.
Maturation threat refers to the possibility that observed effects are caused by natural maturation of subjects (e.g., a general improvement in their intellectual ability to understand complex concepts) rather than the experimental treatment.
Testing threat is a threat in pre-post designs where subjects’ posttest responses are conditioned by their pretest responses. For instance, if students remember their answers from the pretest evaluation, they may tend to repeat them in the posttest exam.
Not conducting a pretest can help avoid this threat.
Instrumentation threat , which also occurs in pre-post designs, refers to the possibility that the difference between pretest and posttest scores is not due to the remedial math program, but due to changes in the administered test, such as the posttest having a higher or lower degree of difficulty than the pretest.
Mortality threat refers to the possibility that subjects may be dropping out of the study at differential rates between the treatment and control groups due to a systematic reason, such that the dropouts were mostly students who scored low on the pretest. If the low-performing students drop out, the results of the posttest will be artificially inflated by the preponderance of high-performing students.
Regression threat —also called a regression to the mean—refers to the statistical tendency of a group’s overall performance to regress toward the mean during a posttest rather than in the anticipated direction. For instance, if subjects scored high on a pretest, they will have a tendency to score lower on the posttest (closer to the mean) because their high scores (away from the mean) during the pretest were possibly a statistical aberration. This problem tends to be more prevalent in non-random samples and when the two measures are imperfectly correlated.
Two-group experimental designs

Pretest-posttest control group design . In this design, subjects are randomly assigned to treatment and control groups, subjected to an initial (pretest) measurement of the dependent variables of interest, the treatment group is administered a treatment (representing the independent variable of interest), and the dependent variables measured again (posttest). The notation of this design is shown in Figure 10.1.

Statistical analysis of this design involves a simple analysis of variance (ANOVA) between the treatment and control groups. The pretest-posttest design handles several threats to internal validity, such as maturation, testing, and regression, since these threats can be expected to influence both treatment and control groups in a similar (random) manner. The selection threat is controlled via random assignment. However, additional threats to internal validity may exist. For instance, mortality can be a problem if there are differential dropout rates between the two groups, and the pretest measurement may bias the posttest measurement—especially if the pretest introduces unusual topics or content.
Posttest -only control group design . This design is a simpler version of the pretest-posttest design where pretest measurements are omitted. The design notation is shown in Figure 10.2.

The treatment effect is measured simply as the difference in the posttest scores between the two groups:
![Rendered by QuickLaTeX.com \[E = (O_{1} - O_{2})\,.\]](https://usq.pressbooks.pub/app/uploads/quicklatex/quicklatex.com-90f2ce47275e7b35def5c5b52b7d7d45_l3.png)
The appropriate statistical analysis of this design is also a two-group analysis of variance (ANOVA). The simplicity of this design makes it more attractive than the pretest-posttest design in terms of internal validity. This design controls for maturation, testing, regression, selection, and pretest-posttest interaction, though the mortality threat may continue to exist.

Because the pretest measure is not a measurement of the dependent variable, but rather a covariate, the treatment effect is measured as the difference in the posttest scores between the treatment and control groups as:
Due to the presence of covariates, the right statistical analysis of this design is a two-group analysis of covariance (ANCOVA). This design has all the advantages of posttest-only design, but with internal validity due to the controlling of covariates. Covariance designs can also be extended to pretest-posttest control group design.
Factorial designs
Two-group designs are inadequate if your research requires manipulation of two or more independent variables (treatments). In such cases, you would need four or higher-group designs. Such designs, quite popular in experimental research, are commonly called factorial designs. Each independent variable in this design is called a factor , and each subdivision of a factor is called a level . Factorial designs enable the researcher to examine not only the individual effect of each treatment on the dependent variables (called main effects), but also their joint effect (called interaction effects).

In a factorial design, a main effect is said to exist if the dependent variable shows a significant difference between multiple levels of one factor, at all levels of other factors. No change in the dependent variable across factor levels is the null case (baseline), from which main effects are evaluated. In the above example, you may see a main effect of instructional type, instructional time, or both on learning outcomes. An interaction effect exists when the effect of differences in one factor depends upon the level of a second factor. In our example, if the effect of instructional type on learning outcomes is greater for three hours/week of instructional time than for one and a half hours/week, then we can say that there is an interaction effect between instructional type and instructional time on learning outcomes. Note that the presence of interaction effects dominate and make main effects irrelevant, and it is not meaningful to interpret main effects if interaction effects are significant.
Hybrid experimental designs
Hybrid designs are those that are formed by combining features of more established designs. Three such hybrid designs are randomised bocks design, Solomon four-group design, and switched replications design.
Randomised block design. This is a variation of the posttest-only or pretest-posttest control group design where the subject population can be grouped into relatively homogeneous subgroups (called blocks ) within which the experiment is replicated. For instance, if you want to replicate the same posttest-only design among university students and full-time working professionals (two homogeneous blocks), subjects in both blocks are randomly split between the treatment group (receiving the same treatment) and the control group (see Figure 10.5). The purpose of this design is to reduce the ‘noise’ or variance in data that may be attributable to differences between the blocks so that the actual effect of interest can be detected more accurately.
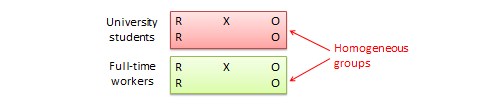
Solomon four-group design . In this design, the sample is divided into two treatment groups and two control groups. One treatment group and one control group receive the pretest, and the other two groups do not. This design represents a combination of posttest-only and pretest-posttest control group design, and is intended to test for the potential biasing effect of pretest measurement on posttest measures that tends to occur in pretest-posttest designs, but not in posttest-only designs. The design notation is shown in Figure 10.6.
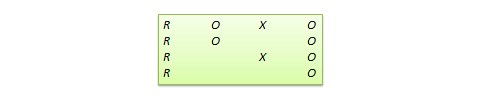
Switched replication design . This is a two-group design implemented in two phases with three waves of measurement. The treatment group in the first phase serves as the control group in the second phase, and the control group in the first phase becomes the treatment group in the second phase, as illustrated in Figure 10.7. In other words, the original design is repeated or replicated temporally with treatment/control roles switched between the two groups. By the end of the study, all participants will have received the treatment either during the first or the second phase. This design is most feasible in organisational contexts where organisational programs (e.g., employee training) are implemented in a phased manner or are repeated at regular intervals.

Quasi-experimental designs
Quasi-experimental designs are almost identical to true experimental designs, but lacking one key ingredient: random assignment. For instance, one entire class section or one organisation is used as the treatment group, while another section of the same class or a different organisation in the same industry is used as the control group. This lack of random assignment potentially results in groups that are non-equivalent, such as one group possessing greater mastery of certain content than the other group, say by virtue of having a better teacher in a previous semester, which introduces the possibility of selection bias . Quasi-experimental designs are therefore inferior to true experimental designs in interval validity due to the presence of a variety of selection related threats such as selection-maturation threat (the treatment and control groups maturing at different rates), selection-history threat (the treatment and control groups being differentially impacted by extraneous or historical events), selection-regression threat (the treatment and control groups regressing toward the mean between pretest and posttest at different rates), selection-instrumentation threat (the treatment and control groups responding differently to the measurement), selection-testing (the treatment and control groups responding differently to the pretest), and selection-mortality (the treatment and control groups demonstrating differential dropout rates). Given these selection threats, it is generally preferable to avoid quasi-experimental designs to the greatest extent possible.

In addition, there are quite a few unique non-equivalent designs without corresponding true experimental design cousins. Some of the more useful of these designs are discussed next.
Regression discontinuity (RD) design . This is a non-equivalent pretest-posttest design where subjects are assigned to the treatment or control group based on a cut-off score on a preprogram measure. For instance, patients who are severely ill may be assigned to a treatment group to test the efficacy of a new drug or treatment protocol and those who are mildly ill are assigned to the control group. In another example, students who are lagging behind on standardised test scores may be selected for a remedial curriculum program intended to improve their performance, while those who score high on such tests are not selected from the remedial program.

Because of the use of a cut-off score, it is possible that the observed results may be a function of the cut-off score rather than the treatment, which introduces a new threat to internal validity. However, using the cut-off score also ensures that limited or costly resources are distributed to people who need them the most, rather than randomly across a population, while simultaneously allowing a quasi-experimental treatment. The control group scores in the RD design do not serve as a benchmark for comparing treatment group scores, given the systematic non-equivalence between the two groups. Rather, if there is no discontinuity between pretest and posttest scores in the control group, but such a discontinuity persists in the treatment group, then this discontinuity is viewed as evidence of the treatment effect.
Proxy pretest design . This design, shown in Figure 10.11, looks very similar to the standard NEGD (pretest-posttest) design, with one critical difference: the pretest score is collected after the treatment is administered. A typical application of this design is when a researcher is brought in to test the efficacy of a program (e.g., an educational program) after the program has already started and pretest data is not available. Under such circumstances, the best option for the researcher is often to use a different prerecorded measure, such as students’ grade point average before the start of the program, as a proxy for pretest data. A variation of the proxy pretest design is to use subjects’ posttest recollection of pretest data, which may be subject to recall bias, but nevertheless may provide a measure of perceived gain or change in the dependent variable.

Separate pretest-posttest samples design . This design is useful if it is not possible to collect pretest and posttest data from the same subjects for some reason. As shown in Figure 10.12, there are four groups in this design, but two groups come from a single non-equivalent group, while the other two groups come from a different non-equivalent group. For instance, say you want to test customer satisfaction with a new online service that is implemented in one city but not in another. In this case, customers in the first city serve as the treatment group and those in the second city constitute the control group. If it is not possible to obtain pretest and posttest measures from the same customers, you can measure customer satisfaction at one point in time, implement the new service program, and measure customer satisfaction (with a different set of customers) after the program is implemented. Customer satisfaction is also measured in the control group at the same times as in the treatment group, but without the new program implementation. The design is not particularly strong, because you cannot examine the changes in any specific customer’s satisfaction score before and after the implementation, but you can only examine average customer satisfaction scores. Despite the lower internal validity, this design may still be a useful way of collecting quasi-experimental data when pretest and posttest data is not available from the same subjects.
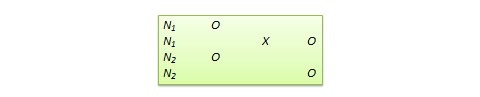
An interesting variation of the NEDV design is a pattern-matching NEDV design , which employs multiple outcome variables and a theory that explains how much each variable will be affected by the treatment. The researcher can then examine if the theoretical prediction is matched in actual observations. This pattern-matching technique—based on the degree of correspondence between theoretical and observed patterns—is a powerful way of alleviating internal validity concerns in the original NEDV design.

Perils of experimental research
Experimental research is one of the most difficult of research designs, and should not be taken lightly. This type of research is often best with a multitude of methodological problems. First, though experimental research requires theories for framing hypotheses for testing, much of current experimental research is atheoretical. Without theories, the hypotheses being tested tend to be ad hoc, possibly illogical, and meaningless. Second, many of the measurement instruments used in experimental research are not tested for reliability and validity, and are incomparable across studies. Consequently, results generated using such instruments are also incomparable. Third, often experimental research uses inappropriate research designs, such as irrelevant dependent variables, no interaction effects, no experimental controls, and non-equivalent stimulus across treatment groups. Findings from such studies tend to lack internal validity and are highly suspect. Fourth, the treatments (tasks) used in experimental research may be diverse, incomparable, and inconsistent across studies, and sometimes inappropriate for the subject population. For instance, undergraduate student subjects are often asked to pretend that they are marketing managers and asked to perform a complex budget allocation task in which they have no experience or expertise. The use of such inappropriate tasks, introduces new threats to internal validity (i.e., subject’s performance may be an artefact of the content or difficulty of the task setting), generates findings that are non-interpretable and meaningless, and makes integration of findings across studies impossible.
The design of proper experimental treatments is a very important task in experimental design, because the treatment is the raison d’etre of the experimental method, and must never be rushed or neglected. To design an adequate and appropriate task, researchers should use prevalidated tasks if available, conduct treatment manipulation checks to check for the adequacy of such tasks (by debriefing subjects after performing the assigned task), conduct pilot tests (repeatedly, if necessary), and if in doubt, use tasks that are simple and familiar for the respondent sample rather than tasks that are complex or unfamiliar.
In summary, this chapter introduced key concepts in the experimental design research method and introduced a variety of true experimental and quasi-experimental designs. Although these designs vary widely in internal validity, designs with less internal validity should not be overlooked and may sometimes be useful under specific circumstances and empirical contingencies.
Social Science Research: Principles, Methods and Practices (Revised edition) Copyright © 2019 by Anol Bhattacherjee is licensed under a Creative Commons Attribution-NonCommercial-ShareAlike 4.0 International License , except where otherwise noted.
Share This Book

Research Limitations 101 📖
A Plain-Language Explainer (With Practical Examples)
By: Derek Jansen (MBA) | Expert Reviewer: Dr. Eunice Rautenbach | May 2024
Research limitations are one of those things that students tend to avoid digging into, and understandably so. No one likes to critique their own study and point out weaknesses. Nevertheless, being able to understand the limitations of your study – and, just as importantly, the implications thereof – a is a critically important skill.
In this post, we’ll unpack some of the most common research limitations you’re likely to encounter, so that you can approach your project with confidence.
Overview: Research Limitations 101
- What are research limitations ?
- Access – based limitations
- Temporal & financial limitations
- Sample & sampling limitations
- Design limitations
- Researcher limitations
- Key takeaways
What (exactly) are “research limitations”?
At the simplest level, research limitations (also referred to as “the limitations of the study”) are the constraints and challenges that will invariably influence your ability to conduct your study and draw reliable conclusions .
Research limitations are inevitable. Absolutely no study is perfect and limitations are an inherent part of any research design. These limitations can stem from a variety of sources , including access to data, methodological choices, and the more mundane constraints of budget and time. So, there’s no use trying to escape them – what matters is that you can recognise them.
Acknowledging and understanding these limitations is crucial, not just for the integrity of your research, but also for your development as a scholar. That probably sounds a bit rich, but realistically, having a strong understanding of the limitations of any given study helps you handle the inevitable obstacles professionally and transparently, which in turn builds trust with your audience and academic peers.
Simply put, recognising and discussing the limitations of your study demonstrates that you know what you’re doing , and that you’ve considered the results of your project within the context of these limitations. In other words, discussing the limitations is a sign of credibility and strength – not weakness. Contrary to the common misconception, highlighting your limitations (or rather, your study’s limitations) will earn you (rather than cost you) marks.
So, with that foundation laid, let’s have a look at some of the most common research limitations you’re likely to encounter – and how to go about managing them as effectively as possible.
Need a helping hand?
Limitation #1: Access To Information
One of the first hurdles you might encounter is limited access to necessary information. For example, you may have trouble getting access to specific literature or niche data sets. This situation can manifest due to several reasons, including paywalls, copyright and licensing issues or language barriers.
To minimise situations like these, it’s useful to try to leverage your university’s resource pool to the greatest extent possible. In practical terms, this means engaging with your university’s librarian and/or potentially utilising interlibrary loans to get access to restricted resources. If this sounds foreign to you, have a chat with your librarian 🙃
In emerging fields or highly specific study areas, you might find that there’s very little existing research (i.e., literature) on your topic. This scenario, while challenging, also offers a unique opportunity to contribute significantly to your field , as it indicates that there’s a significant research gap .
All of that said, be sure to conduct an exhaustive search using a variety of keywords and Boolean operators before assuming that there’s a lack of literature. Also, remember to snowball your literature base . In other words, scan the reference lists of the handful of papers that are directly relevant and then scan those references for more sources. You can also consider using tools like Litmaps and Connected Papers (see video below).
Limitation #2: Time & Money
Almost every researcher will face time and budget constraints at some point. Naturally, these limitations can affect the depth and breadth of your research – but they don’t need to be a death sentence.
Effective planning is crucial to managing both the temporal and financial aspects of your study. In practical terms, utilising tools like Gantt charts can help you visualise and plan your research timeline realistically, thereby reducing the risk of any nasty surprises. Always take a conservative stance when it comes to timelines, especially if you’re new to academic research. As a rule of thumb, things will generally take twice as long as you expect – so, prepare for the worst-case scenario.
If budget is a concern, you might want to consider exploring small research grants or adjusting the scope of your study so that it fits within a realistic budget. Trimming back might sound unattractive, but keep in mind that a smaller, well-planned study can often be more impactful than a larger, poorly planned project.
If you find yourself in a position where you’ve already run out of cash, don’t panic. There’s usually a pivot opportunity hidden somewhere within your project. Engage with your research advisor or faculty to explore potential solutions – don’t make any major changes without first consulting your institution.
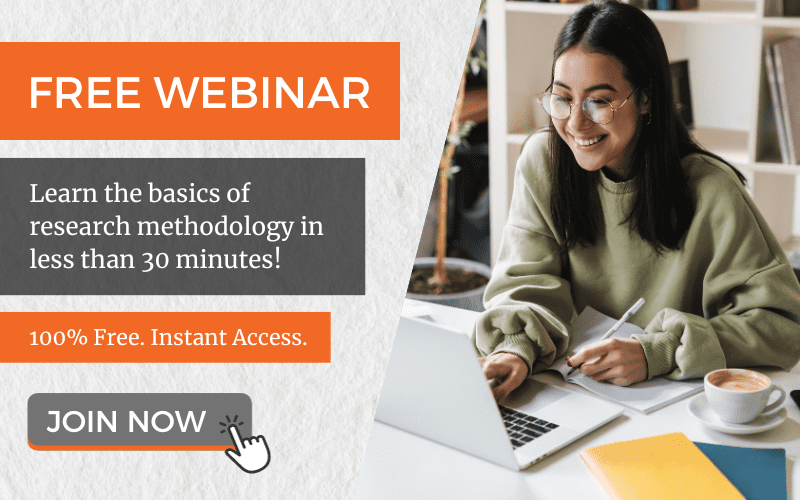
Limitation #3: Sample Size & Composition
As we’ve discussed before , the size and representativeness of your sample are crucial , especially in quantitative research where the robustness of your conclusions often depends on these factors. All too often though, students run into issues achieving a sufficient sample size and composition.
To ensure adequacy in terms of your sample size, it’s important to plan for potential dropouts by oversampling from the outset . In other words, if you aim for a final sample size of 100 participants, aim to recruit 120-140 to account for unexpected challenges. If you still find yourself short on participants, consider whether you could complement your dataset with secondary data or data from an adjacent sample – for example, participants from another city or country. That said, be sure to engage with your research advisor before making any changes to your approach.
A related issue that you may run into is sample composition. In other words, you may have trouble securing a random sample that’s representative of your population of interest. In cases like this, you might again want to look at ways to complement your dataset with other sources, but if that’s not possible, it’s not the end of the world. As with all limitations, you’ll just need to recognise this limitation in your final write-up and be sure to interpret your results accordingly. In other words, don’t claim generalisability of your results if your sample isn’t random.
Limitation #4: Methodological Limitations
As we alluded earlier, every methodological choice comes with its own set of limitations . For example, you can’t claim causality if you’re using a descriptive or correlational research design. Similarly, as we saw in the previous example, you can’t claim generalisability if you’re using a non-random sampling approach.
Making good methodological choices is all about understanding (and accepting) the inherent trade-offs . In the vast majority of cases, you won’t be able to adopt the “perfect” methodology – and that’s okay. What’s important is that you select a methodology that aligns with your research aims and research questions , as well as the practical constraints at play (e.g., time, money, equipment access, etc.). Just as importantly, you must recognise and articulate the limitations of your chosen methods, and justify why they were the most suitable, given your specific context.
Limitation #5: Researcher (In)experience
A discussion about research limitations would not be complete without mentioning the researcher (that’s you!). Whether we like to admit it or not, researcher inexperience and personal biases can subtly (and sometimes not so subtly) influence the interpretation and presentation of data within a study. This is especially true when it comes to dissertations and theses , as these are most commonly undertaken by first-time (or relatively fresh) researchers.
When it comes to dealing with this specific limitation, it’s important to remember the adage “ We don’t know what we don’t know ”. In other words, recognise and embrace your (relative) ignorance and subjectivity – and interpret your study’s results within that context . Simply put, don’t be overly confident in drawing conclusions from your study – especially when they contradict existing literature.
Cultivating a culture of reflexivity within your research practices can help reduce subjectivity and keep you a bit more “rooted” in the data. In practical terms, this simply means making an effort to become aware of how your perspectives and experiences may have shaped the research process and outcomes.
As with any new endeavour in life, it’s useful to garner as many outsider perspectives as possible. Of course, your university-assigned research advisor will play a large role in this respect, but it’s also a good idea to seek out feedback and critique from other academics. To this end, you might consider approaching other faculty at your institution, joining an online group, or even working with a private coach .
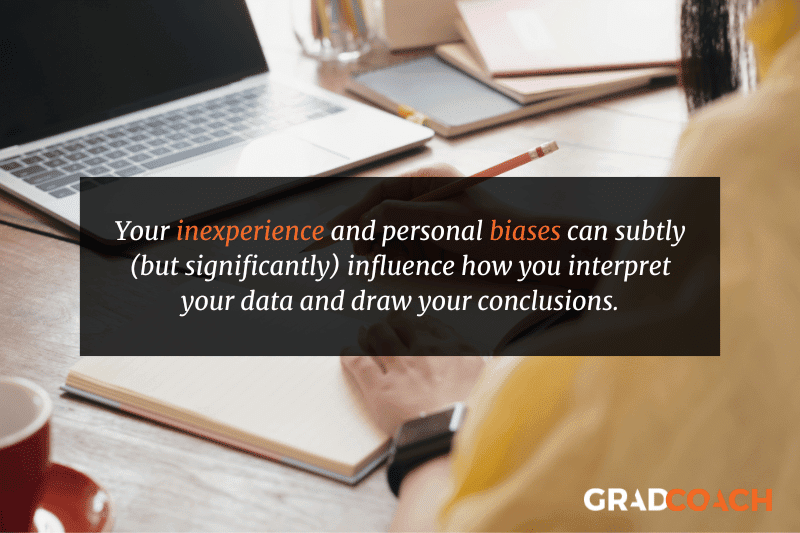
Key Takeaways
Understanding and effectively navigating research limitations is key to conducting credible and reliable academic work. By acknowledging and addressing these limitations upfront, you not only enhance the integrity of your research, but also demonstrate your academic maturity and professionalism.
Whether you’re working on a dissertation, thesis or any other type of formal academic research, remember the five most common research limitations and interpret your data while keeping them in mind.
- Access to Information (literature and data)
- Time and money
- Sample size and composition
- Research design and methodology
- Researcher (in)experience and bias
If you need a hand identifying and mitigating the limitations within your study, check out our 1:1 private coaching service .
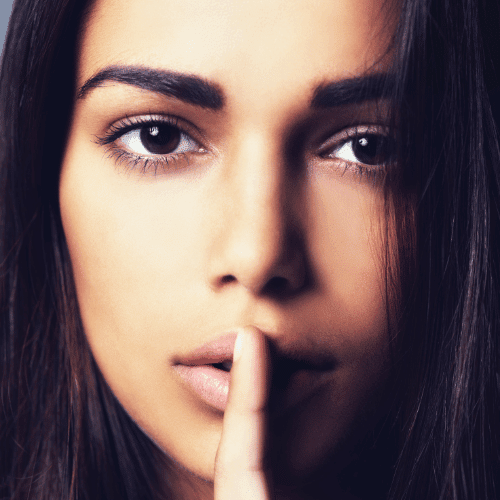
Psst… there’s more!
This post is an extract from our bestselling short course, Methodology Bootcamp . If you want to work smart, you don't want to miss this .
Submit a Comment Cancel reply
Your email address will not be published. Required fields are marked *
Save my name, email, and website in this browser for the next time I comment.
- Print Friendly
Experimental and Quasi-Experimental Research
Guide Title: Experimental and Quasi-Experimental Research Guide ID: 64
You approach a stainless-steel wall, separated vertically along its middle where two halves meet. After looking to the left, you see two buttons on the wall to the right. You press the top button and it lights up. A soft tone sounds and the two halves of the wall slide apart to reveal a small room. You step into the room. Looking to the left, then to the right, you see a panel of more buttons. You know that you seek a room marked with the numbers 1-0-1-2, so you press the button marked "10." The halves slide shut and enclose you within the cubicle, which jolts upward. Soon, the soft tone sounds again. The door opens again. On the far wall, a sign silently proclaims, "10th floor."
You have engaged in a series of experiments. A ride in an elevator may not seem like an experiment, but it, and each step taken towards its ultimate outcome, are common examples of a search for a causal relationship-which is what experimentation is all about.
You started with the hypothesis that this is in fact an elevator. You proved that you were correct. You then hypothesized that the button to summon the elevator was on the left, which was incorrect, so then you hypothesized it was on the right, and you were correct. You hypothesized that pressing the button marked with the up arrow would not only bring an elevator to you, but that it would be an elevator heading in the up direction. You were right.
As this guide explains, the deliberate process of testing hypotheses and reaching conclusions is an extension of commonplace testing of cause and effect relationships.
Basic Concepts of Experimental and Quasi-Experimental Research
Discovering causal relationships is the key to experimental research. In abstract terms, this means the relationship between a certain action, X, which alone creates the effect Y. For example, turning the volume knob on your stereo clockwise causes the sound to get louder. In addition, you could observe that turning the knob clockwise alone, and nothing else, caused the sound level to increase. You could further conclude that a causal relationship exists between turning the knob clockwise and an increase in volume; not simply because one caused the other, but because you are certain that nothing else caused the effect.
Independent and Dependent Variables
Beyond discovering causal relationships, experimental research further seeks out how much cause will produce how much effect; in technical terms, how the independent variable will affect the dependent variable. You know that turning the knob clockwise will produce a louder noise, but by varying how much you turn it, you see how much sound is produced. On the other hand, you might find that although you turn the knob a great deal, sound doesn't increase dramatically. Or, you might find that turning the knob just a little adds more sound than expected. The amount that you turned the knob is the independent variable, the variable that the researcher controls, and the amount of sound that resulted from turning it is the dependent variable, the change that is caused by the independent variable.
Experimental research also looks into the effects of removing something. For example, if you remove a loud noise from the room, will the person next to you be able to hear you? Or how much noise needs to be removed before that person can hear you?
Treatment and Hypothesis
The term treatment refers to either removing or adding a stimulus in order to measure an effect (such as turning the knob a little or a lot, or reducing the noise level a little or a lot). Experimental researchers want to know how varying levels of treatment will affect what they are studying. As such, researchers often have an idea, or hypothesis, about what effect will occur when they cause something. Few experiments are performed where there is no idea of what will happen. From past experiences in life or from the knowledge we possess in our specific field of study, we know how some actions cause other reactions. Experiments confirm or reconfirm this fact.
Experimentation becomes more complex when the causal relationships they seek aren't as clear as in the stereo knob-turning examples. Questions like "Will olestra cause cancer?" or "Will this new fertilizer help this plant grow better?" present more to consider. For example, any number of things could affect the growth rate of a plant-the temperature, how much water or sun it receives, or how much carbon dioxide is in the air. These variables can affect an experiment's results. An experimenter who wants to show that adding a certain fertilizer will help a plant grow better must ensure that it is the fertilizer, and nothing else, affecting the growth patterns of the plant. To do this, as many of these variables as possible must be controlled.
Matching and Randomization
In the example used in this guide (you'll find the example below), we discuss an experiment that focuses on three groups of plants -- one that is treated with a fertilizer named MegaGro, another group treated with a fertilizer named Plant!, and yet another that is not treated with fetilizer (this latter group serves as a "control" group). In this example, even though the designers of the experiment have tried to remove all extraneous variables, results may appear merely coincidental. Since the goal of the experiment is to prove a causal relationship in which a single variable is responsible for the effect produced, the experiment would produce stronger proof if the results were replicated in larger treatment and control groups.
Selecting groups entails assigning subjects in the groups of an experiment in such a way that treatment and control groups are comparable in all respects except the application of the treatment. Groups can be created in two ways: matching and randomization. In the MegaGro experiment discussed below, the plants might be matched according to characteristics such as age, weight and whether they are blooming. This involves distributing these plants so that each plant in one group exactly matches characteristics of plants in the other groups. Matching may be problematic, though, because it "can promote a false sense of security by leading [the experimenter] to believe that [the] experimental and control groups were really equated at the outset, when in fact they were not equated on a host of variables" (Jones, 291). In other words, you may have flowers for your MegaGro experiment that you matched and distributed among groups, but other variables are unaccounted for. It would be difficult to have equal groupings.
Randomization, then, is preferred to matching. This method is based on the statistical principle of normal distribution. Theoretically, any arbitrarily selected group of adequate size will reflect normal distribution. Differences between groups will average out and become more comparable. The principle of normal distribution states that in a population most individuals will fall within the middle range of values for a given characteristic, with increasingly fewer toward either extreme (graphically represented as the ubiquitous "bell curve").
Differences between Quasi-Experimental and Experimental Research
Thus far, we have explained that for experimental research we need:
- a hypothesis for a causal relationship;
- a control group and a treatment group;
- to eliminate confounding variables that might mess up the experiment and prevent displaying the causal relationship; and
- to have larger groups with a carefully sorted constituency; preferably randomized, in order to keep accidental differences from fouling things up.
But what if we don't have all of those? Do we still have an experiment? Not a true experiment in the strictest scientific sense of the term, but we can have a quasi-experiment, an attempt to uncover a causal relationship, even though the researcher cannot control all the factors that might affect the outcome.
A quasi-experimenter treats a given situation as an experiment even though it is not wholly by design. The independent variable may not be manipulated by the researcher, treatment and control groups may not be randomized or matched, or there may be no control group. The researcher is limited in what he or she can say conclusively.
The significant element of both experiments and quasi-experiments is the measure of the dependent variable, which it allows for comparison. Some data is quite straightforward, but other measures, such as level of self-confidence in writing ability, increase in creativity or in reading comprehension are inescapably subjective. In such cases, quasi-experimentation often involves a number of strategies to compare subjectivity, such as rating data, testing, surveying, and content analysis.
Rating essentially is developing a rating scale to evaluate data. In testing, experimenters and quasi-experimenters use ANOVA (Analysis of Variance) and ANCOVA (Analysis of Co-Variance) tests to measure differences between control and experimental groups, as well as different correlations between groups.
Since we're mentioning the subject of statistics, note that experimental or quasi-experimental research cannot state beyond a shadow of a doubt that a single cause will always produce any one effect. They can do no more than show a probability that one thing causes another. The probability that a result is the due to random chance is an important measure of statistical analysis and in experimental research.
Example: Causality
Let's say you want to determine that your new fertilizer, MegaGro, will increase the growth rate of plants. You begin by getting a plant to go with your fertilizer. Since the experiment is concerned with proving that MegaGro works, you need another plant, using no fertilizer at all on it, to compare how much change your fertilized plant displays. This is what is known as a control group.
Set up with a control group, which will receive no treatment, and an experimental group, which will get MegaGro, you must then address those variables that could invalidate your experiment. This can be an extensive and exhaustive process. You must ensure that you use the same plant; that both groups are put in the same kind of soil; that they receive equal amounts of water and sun; that they receive the same amount of exposure to carbon-dioxide-exhaling researchers, and so on. In short, any other variable that might affect the growth of those plants, other than the fertilizer, must be the same for both plants. Otherwise, you can't prove absolutely that MegaGro is the only explanation for the increased growth of one of those plants.
Such an experiment can be done on more than two groups. You may not only want to show that MegaGro is an effective fertilizer, but that it is better than its competitor brand of fertilizer, Plant! All you need to do, then, is have one experimental group receiving MegaGro, one receiving Plant! and the other (the control group) receiving no fertilizer. Those are the only variables that can be different between the three groups; all other variables must be the same for the experiment to be valid.
Controlling variables allows the researcher to identify conditions that may affect the experiment's outcome. This may lead to alternative explanations that the researcher is willing to entertain in order to isolate only variables judged significant. In the MegaGro experiment, you may be concerned with how fertile the soil is, but not with the plants'; relative position in the window, as you don't think that the amount of shade they get will affect their growth rate. But what if it did? You would have to go about eliminating variables in order to determine which is the key factor. What if one receives more shade than the other and the MegaGro plant, which received more shade, died? This might prompt you to formulate a plausible alternative explanation, which is a way of accounting for a result that differs from what you expected. You would then want to redo the study with equal amounts of sunlight.
Methods: Five Steps
Experimental research can be roughly divided into five phases:
Identifying a research problem
The process starts by clearly identifying the problem you want to study and considering what possible methods will affect a solution. Then you choose the method you want to test, and formulate a hypothesis to predict the outcome of the test.
For example, you may want to improve student essays, but you don't believe that teacher feedback is enough. You hypothesize that some possible methods for writing improvement include peer workshopping, or reading more example essays. Favoring the former, your experiment would try to determine if peer workshopping improves writing in high school seniors. You state your hypothesis: peer workshopping prior to turning in a final draft will improve the quality of the student's essay.
Planning an experimental research study
The next step is to devise an experiment to test your hypothesis. In doing so, you must consider several factors. For example, how generalizable do you want your end results to be? Do you want to generalize about the entire population of high school seniors everywhere, or just the particular population of seniors at your specific school? This will determine how simple or complex the experiment will be. The amount of time funding you have will also determine the size of your experiment.
Continuing the example from step one, you may want a small study at one school involving three teachers, each teaching two sections of the same course. The treatment in this experiment is peer workshopping. Each of the three teachers will assign the same essay assignment to both classes; the treatment group will participate in peer workshopping, while the control group will receive only teacher comments on their drafts.
Conducting the experiment
At the start of an experiment, the control and treatment groups must be selected. Whereas the "hard" sciences have the luxury of attempting to create truly equal groups, educators often find themselves forced to conduct their experiments based on self-selected groups, rather than on randomization. As was highlighted in the Basic Concepts section, this makes the study a quasi-experiment, since the researchers cannot control all of the variables.
For the peer workshopping experiment, let's say that it involves six classes and three teachers with a sample of students randomly selected from all the classes. Each teacher will have a class for a control group and a class for a treatment group. The essay assignment is given and the teachers are briefed not to change any of their teaching methods other than the use of peer workshopping. You may see here that this is an effort to control a possible variable: teaching style variance.
Analyzing the data
The fourth step is to collect and analyze the data. This is not solely a step where you collect the papers, read them, and say your methods were a success. You must show how successful. You must devise a scale by which you will evaluate the data you receive, therefore you must decide what indicators will be, and will not be, important.
Continuing our example, the teachers' grades are first recorded, then the essays are evaluated for a change in sentence complexity, syntactical and grammatical errors, and overall length. Any statistical analysis is done at this time if you choose to do any. Notice here that the researcher has made judgments on what signals improved writing. It is not simply a matter of improved teacher grades, but a matter of what the researcher believes constitutes improved use of the language.
Writing the paper/presentation describing the findings
Once you have completed the experiment, you will want to share findings by publishing academic paper (or presentations). These papers usually have the following format, but it is not necessary to follow it strictly. Sections can be combined or not included, depending on the structure of the experiment, and the journal to which you submit your paper.
- Abstract : Summarize the project: its aims, participants, basic methodology, results, and a brief interpretation.
- Introduction : Set the context of the experiment.
- Review of Literature : Provide a review of the literature in the specific area of study to show what work has been done. Should lead directly to the author's purpose for the study.
- Statement of Purpose : Present the problem to be studied.
- Participants : Describe in detail participants involved in the study; e.g., how many, etc. Provide as much information as possible.
- Materials and Procedures : Clearly describe materials and procedures. Provide enough information so that the experiment can be replicated, but not so much information that it becomes unreadable. Include how participants were chosen, the tasks assigned them, how they were conducted, how data were evaluated, etc.
- Results : Present the data in an organized fashion. If it is quantifiable, it is analyzed through statistical means. Avoid interpretation at this time.
- Discussion : After presenting the results, interpret what has happened in the experiment. Base the discussion only on the data collected and as objective an interpretation as possible. Hypothesizing is possible here.
- Limitations : Discuss factors that affect the results. Here, you can speculate how much generalization, or more likely, transferability, is possible based on results. This section is important for quasi-experimentation, since a quasi-experiment cannot control all of the variables that might affect the outcome of a study. You would discuss what variables you could not control.
- Conclusion : Synthesize all of the above sections.
- References : Document works cited in the correct format for the field.
Experimental and Quasi-Experimental Research: Issues and Commentary
Several issues are addressed in this section, including the use of experimental and quasi-experimental research in educational settings, the relevance of the methods to English studies, and ethical concerns regarding the methods.
Using Experimental and Quasi-Experimental Research in Educational Settings
Charting causal relationships in human settings.
Any time a human population is involved, prediction of casual relationships becomes cloudy and, some say, impossible. Many reasons exist for this; for example,
- researchers in classrooms add a disturbing presence, causing students to act abnormally, consciously or unconsciously;
- subjects try to please the researcher, just because of an apparent interest in them (known as the Hawthorne Effect); or, perhaps
- the teacher as researcher is restricted by bias and time pressures.
But such confounding variables don't stop researchers from trying to identify causal relationships in education. Educators naturally experiment anyway, comparing groups, assessing the attributes of each, and making predictions based on an evaluation of alternatives. They look to research to support their intuitive practices, experimenting whenever they try to decide which instruction method will best encourage student improvement.
Combining Theory, Research, and Practice
The goal of educational research lies in combining theory, research, and practice. Educational researchers attempt to establish models of teaching practice, learning styles, curriculum development, and countless other educational issues. The aim is to "try to improve our understanding of education and to strive to find ways to have understanding contribute to the improvement of practice," one writer asserts (Floden 1996, p. 197).
In quasi-experimentation, researchers try to develop models by involving teachers as researchers, employing observational research techniques. Although results of this kind of research are context-dependent and difficult to generalize, they can act as a starting point for further study. The "educational researcher . . . provides guidelines and interpretive material intended to liberate the teacher's intelligence so that whatever artistry in teaching the teacher can achieve will be employed" (Eisner 1992, p. 8).
Bias and Rigor
Critics contend that the educational researcher is inherently biased, sample selection is arbitrary, and replication is impossible. The key to combating such criticism has to do with rigor. Rigor is established through close, proper attention to randomizing groups, time spent on a study, and questioning techniques. This allows more effective application of standards of quantitative research to qualitative research.
Often, teachers cannot wait to for piles of experimentation data to be analyzed before using the teaching methods (Lauer and Asher 1988). They ultimately must assess whether the results of a study in a distant classroom are applicable in their own classrooms. And they must continuously test the effectiveness of their methods by using experimental and qualitative research simultaneously. In addition to statistics (quantitative), researchers may perform case studies or observational research (qualitative) in conjunction with, or prior to, experimentation.
Relevance to English Studies
Situations in english studies that might encourage use of experimental methods.
Whenever a researcher would like to see if a causal relationship exists between groups, experimental and quasi-experimental research can be a viable research tool. Researchers in English Studies might use experimentation when they believe a relationship exists between two variables, and they want to show that these two variables have a significant correlation (or causal relationship).
A benefit of experimentation is the ability to control variables, such as the amount of treatment, when it is given, to whom and so forth. Controlling variables allows researchers to gain insight into the relationships they believe exist. For example, a researcher has an idea that writing under pseudonyms encourages student participation in newsgroups. Researchers can control which students write under pseudonyms and which do not, then measure the outcomes. Researchers can then analyze results and determine if this particular variable alone causes increased participation.
Transferability-Applying Results
Experimentation and quasi-experimentation allow for generating transferable results and accepting those results as being dependent upon experimental rigor. It is an effective alternative to generalizability, which is difficult to rely upon in educational research. English scholars, reading results of experiments with a critical eye, ultimately decide if results will be implemented and how. They may even extend that existing research by replicating experiments in the interest of generating new results and benefiting from multiple perspectives. These results will strengthen the study or discredit findings.
Concerns English Scholars Express about Experiments
Researchers should carefully consider if a particular method is feasible in humanities studies, and whether it will yield the desired information. Some researchers recommend addressing pertinent issues combining several research methods, such as survey, interview, ethnography, case study, content analysis, and experimentation (Lauer and Asher, 1988).
Advantages and Disadvantages of Experimental Research: Discussion
In educational research, experimentation is a way to gain insight into methods of instruction. Although teaching is context specific, results can provide a starting point for further study. Often, a teacher/researcher will have a "gut" feeling about an issue which can be explored through experimentation and looking at causal relationships. Through research intuition can shape practice .
A preconception exists that information obtained through scientific method is free of human inconsistencies. But, since scientific method is a matter of human construction, it is subject to human error . The researcher's personal bias may intrude upon the experiment , as well. For example, certain preconceptions may dictate the course of the research and affect the behavior of the subjects. The issue may be compounded when, although many researchers are aware of the affect that their personal bias exerts on their own research, they are pressured to produce research that is accepted in their field of study as "legitimate" experimental research.
The researcher does bring bias to experimentation, but bias does not limit an ability to be reflective . An ethical researcher thinks critically about results and reports those results after careful reflection. Concerns over bias can be leveled against any research method.
Often, the sample may not be representative of a population, because the researcher does not have an opportunity to ensure a representative sample. For example, subjects could be limited to one location, limited in number, studied under constrained conditions and for too short a time.
Despite such inconsistencies in educational research, the researcher has control over the variables , increasing the possibility of more precisely determining individual effects of each variable. Also, determining interaction between variables is more possible.
Even so, artificial results may result . It can be argued that variables are manipulated so the experiment measures what researchers want to examine; therefore, the results are merely contrived products and have no bearing in material reality. Artificial results are difficult to apply in practical situations, making generalizing from the results of a controlled study questionable. Experimental research essentially first decontextualizes a single question from a "real world" scenario, studies it under controlled conditions, and then tries to recontextualize the results back on the "real world" scenario. Results may be difficult to replicate .
Perhaps, groups in an experiment may not be comparable . Quasi-experimentation in educational research is widespread because not only are many researchers also teachers, but many subjects are also students. With the classroom as laboratory, it is difficult to implement randomizing or matching strategies. Often, students self-select into certain sections of a course on the basis of their own agendas and scheduling needs. Thus when, as often happens, one class is treated and the other used for a control, the groups may not actually be comparable. As one might imagine, people who register for a class which meets three times a week at eleven o'clock in the morning (young, no full-time job, night people) differ significantly from those who register for one on Monday evenings from seven to ten p.m. (older, full-time job, possibly more highly motivated). Each situation presents different variables and your group might be completely different from that in the study. Long-term studies are expensive and hard to reproduce. And although often the same hypotheses are tested by different researchers, various factors complicate attempts to compare or synthesize them. It is nearly impossible to be as rigorous as the natural sciences model dictates.
Even when randomization of students is possible, problems arise. First, depending on the class size and the number of classes, the sample may be too small for the extraneous variables to cancel out. Second, the study population is not strictly a sample, because the population of students registered for a given class at a particular university is obviously not representative of the population of all students at large. For example, students at a suburban private liberal-arts college are typically young, white, and upper-middle class. In contrast, students at an urban community college tend to be older, poorer, and members of a racial minority. The differences can be construed as confounding variables: the first group may have fewer demands on its time, have less self-discipline, and benefit from superior secondary education. The second may have more demands, including a job and/or children, have more self-discipline, but an inferior secondary education. Selecting a population of subjects which is representative of the average of all post-secondary students is also a flawed solution, because the outcome of a treatment involving this group is not necessarily transferable to either the students at a community college or the students at the private college, nor are they universally generalizable.
When a human population is involved, experimental research becomes concerned if behavior can be predicted or studied with validity. Human response can be difficult to measure . Human behavior is dependent on individual responses. Rationalizing behavior through experimentation does not account for the process of thought, making outcomes of that process fallible (Eisenberg, 1996).
Nevertheless, we perform experiments daily anyway . When we brush our teeth every morning, we are experimenting to see if this behavior will result in fewer cavities. We are relying on previous experimentation and we are transferring the experimentation to our daily lives.
Moreover, experimentation can be combined with other research methods to ensure rigor . Other qualitative methods such as case study, ethnography, observational research and interviews can function as preconditions for experimentation or conducted simultaneously to add validity to a study.
We have few alternatives to experimentation. Mere anecdotal research , for example is unscientific, unreplicatable, and easily manipulated. Should we rely on Ed walking into a faculty meeting and telling the story of Sally? Sally screamed, "I love writing!" ten times before she wrote her essay and produced a quality paper. Therefore, all the other faculty members should hear this anecdote and know that all other students should employ this similar technique.
On final disadvantage: frequently, political pressure drives experimentation and forces unreliable results. Specific funding and support may drive the outcomes of experimentation and cause the results to be skewed. The reader of these results may not be aware of these biases and should approach experimentation with a critical eye.
Advantages and Disadvantages of Experimental Research: Quick Reference List
Experimental and quasi-experimental research can be summarized in terms of their advantages and disadvantages. This section combines and elaborates upon many points mentioned previously in this guide.
|
|
gain insight into methods of instruction | subject to human error |
intuitive practice shaped by research | personal bias of researcher may intrude |
teachers have bias but can be reflective | sample may not be representative |
researcher can have control over variables | can produce artificial results |
humans perform experiments anyway | results may only apply to one situation and may be difficult to replicate |
can be combined with other research methods for rigor | groups may not be comparable |
use to determine what is best for population | human response can be difficult to measure |
provides for greater transferability than anecdotal research | political pressure may skew results |
Ethical Concerns
Experimental research may be manipulated on both ends of the spectrum: by researcher and by reader. Researchers who report on experimental research, faced with naive readers of experimental research, encounter ethical concerns. While they are creating an experiment, certain objectives and intended uses of the results might drive and skew it. Looking for specific results, they may ask questions and look at data that support only desired conclusions. Conflicting research findings are ignored as a result. Similarly, researchers, seeking support for a particular plan, look only at findings which support that goal, dismissing conflicting research.
Editors and journals do not publish only trouble-free material. As readers of experiments members of the press might report selected and isolated parts of a study to the public, essentially transferring that data to the general population which may not have been intended by the researcher. Take, for example, oat bran. A few years ago, the press reported how oat bran reduces high blood pressure by reducing cholesterol. But that bit of information was taken out of context. The actual study found that when people ate more oat bran, they reduced their intake of saturated fats high in cholesterol. People started eating oat bran muffins by the ton, assuming a causal relationship when in actuality a number of confounding variables might influence the causal link.
Ultimately, ethical use and reportage of experimentation should be addressed by researchers, reporters and readers alike.
Reporters of experimental research often seek to recognize their audience's level of knowledge and try not to mislead readers. And readers must rely on the author's skill and integrity to point out errors and limitations. The relationship between researcher and reader may not sound like a problem, but after spending months or years on a project to produce no significant results, it may be tempting to manipulate the data to show significant results in order to jockey for grants and tenure.
Meanwhile, the reader may uncritically accept results that receive validity by being published in a journal. However, research that lacks credibility often is not published; consequentially, researchers who fail to publish run the risk of being denied grants, promotions, jobs, and tenure. While few researchers are anything but earnest in their attempts to conduct well-designed experiments and present the results in good faith, rhetorical considerations often dictate a certain minimization of methodological flaws.
Concerns arise if researchers do not report all, or otherwise alter, results. This phenomenon is counterbalanced, however, in that professionals are also rewarded for publishing critiques of others' work. Because the author of an experimental study is in essence making an argument for the existence of a causal relationship, he or she must be concerned not only with its integrity, but also with its presentation. Achieving persuasiveness in any kind of writing involves several elements: choosing a topic of interest, providing convincing evidence for one's argument, using tone and voice to project credibility, and organizing the material in a way that meets expectations for a logical sequence. Of course, what is regarded as pertinent, accepted as evidence, required for credibility, and understood as logical varies according to context. If the experimental researcher hopes to make an impact on the community of professionals in their field, she must attend to the standards and orthodoxy's of that audience.
Related Links
Contrasts: Traditional and computer-supported writing classrooms. This Web presents a discussion of the Transitions Study, a year-long exploration of teachers and students in computer-supported and traditional writing classrooms. Includes description of study, rationale for conducting the study, results and implications of the study.
http://kairos.technorhetoric.net/2.2/features/reflections/page1.htm
Annotated Bibliography
A cozy world of trivial pursuits? (1996, June 28) The Times Educational Supplement . 4174, pp. 14-15.
A critique discounting the current methods Great Britain employs to fund and disseminate educational research. The belief is that research is performed for fellow researchers not the teaching public and implications for day to day practice are never addressed.
Anderson, J. A. (1979, Nov. 10-13). Research as argument: the experimental form. Paper presented at the annual meeting of the Speech Communication Association, San Antonio, TX.
In this paper, the scientist who uses the experimental form does so in order to explain that which is verified through prediction.
Anderson, Linda M. (1979). Classroom-based experimental studies of teaching effectiveness in elementary schools . (Technical Report UTR&D-R- 4102). Austin: Research and Development Center for Teacher Education, University of Texas.
Three recent large-scale experimental studies have built on a database established through several correlational studies of teaching effectiveness in elementary school.
Asher, J. W. (1976). Educational research and evaluation methods . Boston: Little, Brown.
Abstract unavailable by press time.
Babbie, Earl R. (1979). The Practice of Social Research . Belmont, CA: Wadsworth.
A textbook containing discussions of several research methodologies used in social science research.
Bangert-Drowns, R.L. (1993). The word processor as instructional tool: a meta-analysis of word processing in writing instruction. Review of Educational Research, 63 (1), 69-93.
Beach, R. (1993). The effects of between-draft teacher evaluation versus student self-evaluation on high school students' revising of rough drafts. Research in the Teaching of English, 13 , 111-119.
The question of whether teacher evaluation or guided self-evaluation of rough drafts results in increased revision was addressed in Beach's study. Differences in the effects of teacher evaluations, guided self-evaluation (using prepared guidelines,) and no evaluation of rough drafts were examined. The final drafts of students (10th, 11th, and 12th graders) were compared with their rough drafts and rated by judges according to degree of change.
Beishuizen, J. & Moonen, J. (1992). Research in technology enriched schools: a case for cooperation between teachers and researchers . (ERIC Technical Report ED351006).
This paper describes the research strategies employed in the Dutch Technology Enriched Schools project to encourage extensive and intensive use of computers in a small number of secondary schools, and to study the effects of computer use on the classroom, the curriculum, and school administration and management.
Borg, W. P. (1989). Educational Research: an Introduction . (5th ed.). New York: Longman.
An overview of educational research methodology, including literature review and discussion of approaches to research, experimental design, statistical analysis, ethics, and rhetorical presentation of research findings.
Campbell, D. T., & Stanley, J. C. (1963). Experimental and quasi-experimental designs for research . Boston: Houghton Mifflin.
A classic overview of research designs.
Campbell, D.T. (1988). Methodology and epistemology for social science: selected papers . ed. E. S. Overman. Chicago: University of Chicago Press.
This is an overview of Campbell's 40-year career and his work. It covers in seven parts measurement, experimental design, applied social experimentation, interpretive social science, epistemology and sociology of science. Includes an extensive bibliography.
Caporaso, J. A., & Roos, Jr., L. L. (Eds.). Quasi-experimental approaches: Testing theory and evaluating policy. Evanston, WA: Northwestern University Press.
A collection of articles concerned with explicating the underlying assumptions of quasi-experimentation and relating these to true experimentation. With an emphasis on design. Includes a glossary of terms.
Collier, R. Writing and the word processor: How wary of the gift-giver should we be? Unpublished manuscript.
Unpublished typescript. Charts the developments to date in computers and composition and speculates about the future within the framework of Willie Sypher's model of the evolution of creative discovery.
Cook, T.D. & Campbell, D.T. (1979). Quasi-experimentation: design and analysis issues for field settings . Boston: Houghton Mifflin Co.
The authors write that this book "presents some quasi-experimental designs and design features that can be used in many social research settings. The designs serve to probe causal hypotheses about a wide variety of substantive issues in both basic and applied research."
Cutler, A. (1970). An experimental method for semantic field study. Linguistic Communication, 2 , N. pag.
This paper emphasizes the need for empirical research and objective discovery procedures in semantics, and illustrates a method by which these goals may be obtained.
Daniels, L. B. (1996, Summer). Eisenberg's Heisenberg: The indeterminancies of rationality. Curriculum Inquiry, 26 , 181-92.
Places Eisenberg's theories in relation to the death of foundationalism by showing that he distorts rational studies into a form of relativism. He looks at Eisenberg's ideas on indeterminacy, methods and evidence, what he is against and what we should think of what he says.
Danziger, K. (1990). Constructing the subject: Historical origins of psychological research. Cambridge: Cambridge University Press.
Danzinger stresses the importance of being aware of the framework in which research operates and of the essentially social nature of scientific activity.
Diener, E., et al. (1972, December). Leakage of experimental information to potential future subjects by debriefed subjects. Journal of Experimental Research in Personality , 264-67.
Research regarding research: an investigation of the effects on the outcome of an experiment in which information about the experiment had been leaked to subjects. The study concludes that such leakage is not a significant problem.
Dudley-Marling, C., & Rhodes, L. K. (1989). Reflecting on a close encounter with experimental research. Canadian Journal of English Language Arts. 12 , 24-28.
Researchers, Dudley-Marling and Rhodes, address some problems they met in their experimental approach to a study of reading comprehension. This article discusses the limitations of experimental research, and presents an alternative to experimental or quantitative research.
Edgington, E. S. (1985). Random assignment and experimental research. Educational Administration Quarterly, 21 , N. pag.
Edgington explores ways on which random assignment can be a part of field studies. The author discusses both non-experimental and experimental research and the need for using random assignment.
Eisenberg, J. (1996, Summer). Response to critiques by R. Floden, J. Zeuli, and L. Daniels. Curriculum Inquiry, 26 , 199-201.
A response to critiques of his argument that rational educational research methods are at best suspect and at worst futile. He believes indeterminacy controls this method and worries that chaotic research is failing students.
Eisner, E. (1992, July). Are all causal claims positivistic? A reply to Francis Schrag. Educational Researcher, 21 (5), 8-9.
Eisner responds to Schrag who claimed that critics like Eisner cannot escape a positivistic paradigm whatever attempts they make to do so. Eisner argues that Schrag essentially misses the point for trying to argue for the paradigm solely on the basis of cause and effect without including the rest of positivistic philosophy. This weakens his argument against multiple modal methods, which Eisner argues provides opportunities to apply the appropriate research design where it is most applicable.
Floden, R.E. (1996, Summer). Educational research: limited, but worthwhile and maybe a bargain. (response to J.A. Eisenberg). Curriculum Inquiry, 26 , 193-7.
Responds to John Eisenberg critique of educational research by asserting the connection between improvement of practice and research results. He places high value of teacher discrepancy and knowledge that research informs practice.
Fortune, J. C., & Hutson, B. A. (1994, March/April). Selecting models for measuring change when true experimental conditions do not exist. Journal of Educational Research, 197-206.
This article reviews methods for minimizing the effects of nonideal experimental conditions by optimally organizing models for the measurement of change.
Fox, R. F. (1980). Treatment of writing apprehension and tts effects on composition. Research in the Teaching of English, 14 , 39-49.
The main purpose of Fox's study was to investigate the effects of two methods of teaching writing on writing apprehension among entry level composition students, A conventional teaching procedure was used with a control group, while a workshop method was employed with the treatment group.
Gadamer, H-G. (1976). Philosophical hermeneutics . (D. E. Linge, Trans.). Berkeley, CA: University of California Press.
A collection of essays with the common themes of the mediation of experience through language, the impossibility of objectivity, and the importance of context in interpretation.
Gaise, S. J. (1981). Experimental vs. non-experimental research on classroom second language learning. Bilingual Education Paper Series, 5 , N. pag.
Aims on classroom-centered research on second language learning and teaching are considered and contrasted with the experimental approach.
Giordano, G. (1983). Commentary: Is experimental research snowing us? Journal of Reading, 27 , 5-7.
Do educational research findings actually benefit teachers and students? Giordano states his opinion that research may be helpful to teaching, but is not essential and often is unnecessary.
Goldenson, D. R. (1978, March). An alternative view about the role of the secondary school in political socialization: A field-experimental study of theory and research in social education. Theory and Research in Social Education , 44-72.
This study concludes that when political discussion among experimental groups of secondary school students is led by a teacher, the degree to which the students' views were impacted is proportional to the credibility of the teacher.
Grossman, J., and J. P. Tierney. (1993, October). The fallibility of comparison groups. Evaluation Review , 556-71.
Grossman and Tierney present evidence to suggest that comparison groups are not the same as nontreatment groups.
Harnisch, D. L. (1992). Human judgment and the logic of evidence: A critical examination of research methods in special education transition literature. In D. L. Harnisch et al. (Eds.), Selected readings in transition.
This chapter describes several common types of research studies in special education transition literature and the threats to their validity.
Hawisher, G. E. (1989). Research and recommendations for computers and composition. In G. Hawisher and C. Selfe. (Eds.), Critical Perspectives on Computers and Composition Instruction . (pp. 44-69). New York: Teacher's College Press.
An overview of research in computers and composition to date. Includes a synthesis grid of experimental research.
Hillocks, G. Jr. (1982). The interaction of instruction, teacher comment, and revision in teaching the composing process. Research in the Teaching of English, 16 , 261-278.
Hillock conducted a study using three treatments: observational or data collecting activities prior to writing, use of revisions or absence of same, and either brief or lengthy teacher comments to identify effective methods of teaching composition to seventh and eighth graders.
Jenkinson, J. C. (1989). Research design in the experimental study of intellectual disability. International Journal of Disability, Development, and Education, 69-84.
This article catalogues the difficulties of conducting experimental research where the subjects are intellectually disables and suggests alternative research strategies.
Jones, R. A. (1985). Research Methods in the Social and Behavioral Sciences. Sunderland, MA: Sinauer Associates, Inc..
A textbook designed to provide an overview of research strategies in the social sciences, including survey, content analysis, ethnographic approaches, and experimentation. The author emphasizes the importance of applying strategies appropriately and in variety.
Kamil, M. L., Langer, J. A., & Shanahan, T. (1985). Understanding research in reading and writing . Newton, Massachusetts: Allyn and Bacon.
Examines a wide variety of problems in reading and writing, with a broad range of techniques, from different perspectives.
Kennedy, J. L. (1985). An Introduction to the Design and Analysis of Experiments in Behavioral Research . Lanham, MD: University Press of America.
An introductory textbook of psychological and educational research.
Keppel, G. (1991). Design and analysis: a researcher's handbook . Englewood Cliffs, NJ: Prentice Hall.
This updates Keppel's earlier book subtitled "a student's handbook." Focuses on extensive information about analytical research and gives a basic picture of research in psychology. Covers a range of statistical topics. Includes a subject and name index, as well as a glossary.
Knowles, G., Elija, R., & Broadwater, K. (1996, Spring/Summer). Teacher research: enhancing the preparation of teachers? Teaching Education, 8 , 123-31.
Researchers looked at one teacher candidate who participated in a class which designed their own research project correlating to a question they would like answered in the teaching world. The goal of the study was to see if preservice teachers developed reflective practice by researching appropriate classroom contexts.
Lace, J., & De Corte, E. (1986, April 16-20). Research on media in western Europe: A myth of sisyphus? Paper presented at the annual meeting of the American Educational Research Association. San Francisco.
Identifies main trends in media research in western Europe, with emphasis on three successive stages since 1960: tools technology, systems technology, and reflective technology.
Latta, A. (1996, Spring/Summer). Teacher as researcher: selected resources. Teaching Education, 8 , 155-60.
An annotated bibliography on educational research including milestones of thought, practical applications, successful outcomes, seminal works, and immediate practical applications.
Lauer. J.M. & Asher, J. W. (1988). Composition research: Empirical designs . New York: Oxford University Press.
Approaching experimentation from a humanist's perspective to it, authors focus on eight major research designs: Case studies, ethnographies, sampling and surveys, quantitative descriptive studies, measurement, true experiments, quasi-experiments, meta-analyses, and program evaluations. It takes on the challenge of bridging language of social science with that of the humanist. Includes name and subject indexes, as well as a glossary and a glossary of symbols.
Mishler, E. G. (1979). Meaning in context: Is there any other kind? Harvard Educational Review, 49 , 1-19.
Contextual importance has been largely ignored by traditional research approaches in social/behavioral sciences and in their application to the education field. Developmental and social psychologists have increasingly noted the inadequacies of this approach. Drawing examples for phenomenology, sociolinguistics, and ethnomethodology, the author proposes alternative approaches for studying meaning in context.
Mitroff, I., & Bonoma, T. V. (1978, May). Psychological assumptions, experimentations, and real world problems: A critique and an alternate approach to evaluation. Evaluation Quarterly , 235-60.
The authors advance the notion of dialectic as a means to clarify and examine the underlying assumptions of experimental research methodology, both in highly controlled situations and in social evaluation.
Muller, E. W. (1985). Application of experimental and quasi-experimental research designs to educational software evaluation. Educational Technology, 25 , 27-31.
Muller proposes a set of guidelines for the use of experimental and quasi-experimental methods of research in evaluating educational software. By obtaining empirical evidence of student performance, it is possible to evaluate if programs are making the desired learning effect.
Murray, S., et al. (1979, April 8-12). Technical issues as threats to internal validity of experimental and quasi-experimental designs . San Francisco: University of California.
The article reviews three evaluation models and analyzes the flaws common to them. Remedies are suggested.
Muter, P., & Maurutto, P. (1991). Reading and skimming from computer screens and books: The paperless office revisited? Behavior and Information Technology, 10 (4), 257-66.
The researchers test for reading and skimming effectiveness, defined as accuracy combined with speed, for written text compared to text on a computer monitor. They conclude that, given optimal on-line conditions, both are equally effective.
O'Donnell, A., Et al. (1992). The impact of cooperative writing. In J. R. Hayes, et al. (Eds.). Reading empirical research studies: The rhetoric of research . (pp. 371-84). Hillsdale, NJ: Lawrence Erlbaum Associates.
A model of experimental design. The authors investigate the efficacy of cooperative writing strategies, as well as the transferability of skills learned to other, individual writing situations.
Palmer, D. (1988). Looking at philosophy . Mountain View, CA: Mayfield Publishing.
An introductory text with incisive but understandable discussions of the major movements and thinkers in philosophy from the Pre-Socratics through Sartre. With illustrations by the author. Includes a glossary.
Phelps-Gunn, T., & Phelps-Terasaki, D. (1982). Written language instruction: Theory and remediation . London: Aspen Systems Corporation.
The lack of research in written expression is addressed and an application on the Total Writing Process Model is presented.
Poetter, T. (1996, Spring/Summer). From resistance to excitement: becoming qualitative researchers and reflective practitioners. Teaching Education , 8109-19.
An education professor reveals his own problematic research when he attempted to institute a educational research component to a teacher preparation program. He encountered dissent from students and cooperating professionals and ultimately was rewarded with excitement towards research and a recognized correlation to practice.
Purves, A. C. (1992). Reflections on research and assessment in written composition. Research in the Teaching of English, 26 .
Three issues concerning research and assessment is writing are discussed: 1) School writing is a matter of products not process, 2) school writing is an ill-defined domain, 3) the quality of school writing is what observers report they see. Purves discusses these issues while looking at data collected in a ten-year study of achievement in written composition in fourteen countries.
Rathus, S. A. (1987). Psychology . (3rd ed.). Poughkeepsie, NY: Holt, Rinehart, and Winston.
An introductory psychology textbook. Includes overviews of the major movements in psychology, discussions of prominent examples of experimental research, and a basic explanation of relevant physiological factors. With chapter summaries.
Reiser, R. A. (1982). Improving the research skills of instructional designers. Educational Technology, 22 , 19-21.
In his paper, Reiser starts by stating the importance of research in advancing the field of education, and points out that graduate students in instructional design lack the proper skills to conduct research. The paper then goes on to outline the practicum in the Instructional Systems Program at Florida State University which includes: 1) Planning and conducting an experimental research study; 2) writing the manuscript describing the study; 3) giving an oral presentation in which they describe their research findings.
Report on education research . (Journal). Washington, DC: Capitol Publication, Education News Services Division.
This is an independent bi-weekly newsletter on research in education and learning. It has been publishing since Sept. 1969.
Rossell, C. H. (1986). Why is bilingual education research so bad?: Critique of the Walsh and Carballo study of Massachusetts bilingual education programs . Boston: Center for Applied Social Science, Boston University. (ERIC Working Paper 86-5).
The Walsh and Carballo evaluation of the effectiveness of transitional bilingual education programs in five Massachusetts communities has five flaws and the five flaws are discussed in detail.
Rubin, D. L., & Greene, K. (1992). Gender-typical style in written language. Research in the Teaching of English, 26.
This study was designed to find out whether the writing styles of men and women differ. Rubin and Green discuss the pre-suppositions that women are better writers than men.
Sawin, E. (1992). Reaction: Experimental research in the context of other methods. School of Education Review, 4 , 18-21.
Sawin responds to Gage's article on methodologies and issues in educational research. He agrees with most of the article but suggests the concept of scientific should not be regarded in absolute terms and recommends more emphasis on scientific method. He also questions the value of experiments over other types of research.
Schoonmaker, W. E. (1984). Improving classroom instruction: A model for experimental research. The Technology Teacher, 44, 24-25.
The model outlined in this article tries to bridge the gap between classroom practice and laboratory research, using what Schoonmaker calls active research. Research is conducted in the classroom with the students and is used to determine which two methods of classroom instruction chosen by the teacher is more effective.
Schrag, F. (1992). In defense of positivist research paradigms. Educational Researcher, 21, (5), 5-8.
The controversial defense of the use of positivistic research methods to evaluate educational strategies; the author takes on Eisner, Erickson, and Popkewitz.
Smith, J. (1997). The stories educational researchers tell about themselves. Educational Researcher, 33 (3), 4-11.
Recapitulates main features of an on-going debate between advocates for using vocabularies of traditional language arts and whole language in educational research. An "impasse" exists were advocates "do not share a theoretical disposition concerning both language instruction and the nature of research," Smith writes (p. 6). He includes a very comprehensive history of the debate of traditional research methodology and qualitative methods and vocabularies. Definitely worth a read by graduates.
Smith, N. L. (1980). The feasibility and desirability of experimental methods in evaluation. Evaluation and Program Planning: An International Journal , 251-55.
Smith identifies the conditions under which experimental research is most desirable. Includes a review of current thinking and controversies.
Stewart, N. R., & Johnson, R. G. (1986, March 16-20). An evaluation of experimental methodology in counseling and counselor education research. Paper presented at the annual meeting of the American Educational Research Association, San Francisco.
The purpose of this study was to evaluate the quality of experimental research in counseling and counselor education published from 1976 through 1984.
Spector, P. E. (1990). Research Designs. Newbury Park, California: Sage Publications.
In this book, Spector introduces the basic principles of experimental and nonexperimental design in the social sciences.
Tait, P. E. (1984). Do-it-yourself evaluation of experimental research. Journal of Visual Impairment and Blindness, 78 , 356-363 .
Tait's goal is to provide the reader who is unfamiliar with experimental research or statistics with the basic skills necessary for the evaluation of research studies.
Walsh, S. M. (1990). The current conflict between case study and experimental research: A breakthrough study derives benefits from both . (ERIC Document Number ED339721).
This paper describes a study that was not experimentally designed, but its major findings were generalizable to the overall population of writers in college freshman composition classes. The study was not a case study, but it provided insights into the attitudes and feelings of small clusters of student writers.
Waters, G. R. (1976). Experimental designs in communication research. Journal of Business Communication, 14 .
The paper presents a series of discussions on the general elements of experimental design and the scientific process and relates these elements to the field of communication.
Welch, W. W. (March 1969). The selection of a national random sample of teachers for experimental curriculum evaluation. Scholastic Science and Math , 210-216.
Members of the evaluation section of Harvard project physics describe what is said to be the first attempt to select a national random sample of teachers, and list 6 steps to do so. Cost and comparison with a volunteer group are also discussed.
Winer, B.J. (1971). Statistical principles in experimental design , (2nd ed.). New York: McGraw-Hill.
Combines theory and application discussions to give readers a better understanding of the logic behind statistical aspects of experimental design. Introduces the broad topic of design, then goes into considerable detail. Not for light reading. Bring your aspirin if you like statistics. Bring morphine is you're a humanist.
Winn, B. (1986, January 16-21). Emerging trends in educational technology research. Paper presented at the Annual Convention of the Association for Educational Communication Technology.
This examination of the topic of research in educational technology addresses four major areas: (1) why research is conducted in this area and the characteristics of that research; (2) the types of research questions that should or should not be addressed; (3) the most appropriate methodologies for finding answers to research questions; and (4) the characteristics of a research report that make it good and ultimately suitable for publication.
Citation Information
Luann Barnes, Jennifer Hauser, Luana Heikes, Anthony J. Hernandez, Paul Tim Richard, Katherine Ross, Guo Hua Yang, and Mike Palmquist. (1994-2024). Experimental and Quasi-Experimental Research. The WAC Clearinghouse. Colorado State University. Available at https://wac.colostate.edu/repository/writing/guides/.
Copyright Information
Copyright © 1994-2024 Colorado State University and/or this site's authors, developers, and contributors . Some material displayed on this site is used with permission.
- USC Libraries
- Research Guides
Organizing Your Social Sciences Research Paper
- Limitations of the Study
- Purpose of Guide
- Design Flaws to Avoid
- Independent and Dependent Variables
- Glossary of Research Terms
- Reading Research Effectively
- Narrowing a Topic Idea
- Broadening a Topic Idea
- Extending the Timeliness of a Topic Idea
- Academic Writing Style
- Applying Critical Thinking
- Choosing a Title
- Making an Outline
- Paragraph Development
- Research Process Video Series
- Executive Summary
- The C.A.R.S. Model
- Background Information
- The Research Problem/Question
- Theoretical Framework
- Citation Tracking
- Content Alert Services
- Evaluating Sources
- Primary Sources
- Secondary Sources
- Tiertiary Sources
- Scholarly vs. Popular Publications
- Qualitative Methods
- Quantitative Methods
- Insiderness
- Using Non-Textual Elements
- Common Grammar Mistakes
- Writing Concisely
- Avoiding Plagiarism
- Footnotes or Endnotes?
- Further Readings
- Generative AI and Writing
- USC Libraries Tutorials and Other Guides
- Bibliography
The limitations of the study are those characteristics of design or methodology that impacted or influenced the interpretation of the findings from your research. Study limitations are the constraints placed on the ability to generalize from the results, to further describe applications to practice, and/or related to the utility of findings that are the result of the ways in which you initially chose to design the study or the method used to establish internal and external validity or the result of unanticipated challenges that emerged during the study.
Price, James H. and Judy Murnan. “Research Limitations and the Necessity of Reporting Them.” American Journal of Health Education 35 (2004): 66-67; Theofanidis, Dimitrios and Antigoni Fountouki. "Limitations and Delimitations in the Research Process." Perioperative Nursing 7 (September-December 2018): 155-163. .
Importance of...
Always acknowledge a study's limitations. It is far better that you identify and acknowledge your study’s limitations than to have them pointed out by your professor and have your grade lowered because you appeared to have ignored them or didn't realize they existed.
Keep in mind that acknowledgment of a study's limitations is an opportunity to make suggestions for further research. If you do connect your study's limitations to suggestions for further research, be sure to explain the ways in which these unanswered questions may become more focused because of your study.
Acknowledgment of a study's limitations also provides you with opportunities to demonstrate that you have thought critically about the research problem, understood the relevant literature published about it, and correctly assessed the methods chosen for studying the problem. A key objective of the research process is not only discovering new knowledge but also to confront assumptions and explore what we don't know.
Claiming limitations is a subjective process because you must evaluate the impact of those limitations . Don't just list key weaknesses and the magnitude of a study's limitations. To do so diminishes the validity of your research because it leaves the reader wondering whether, or in what ways, limitation(s) in your study may have impacted the results and conclusions. Limitations require a critical, overall appraisal and interpretation of their impact. You should answer the question: do these problems with errors, methods, validity, etc. eventually matter and, if so, to what extent?
Price, James H. and Judy Murnan. “Research Limitations and the Necessity of Reporting Them.” American Journal of Health Education 35 (2004): 66-67; Structure: How to Structure the Research Limitations Section of Your Dissertation. Dissertations and Theses: An Online Textbook. Laerd.com.
Descriptions of Possible Limitations
All studies have limitations . However, it is important that you restrict your discussion to limitations related to the research problem under investigation. For example, if a meta-analysis of existing literature is not a stated purpose of your research, it should not be discussed as a limitation. Do not apologize for not addressing issues that you did not promise to investigate in the introduction of your paper.
Here are examples of limitations related to methodology and the research process you may need to describe and discuss how they possibly impacted your results. Note that descriptions of limitations should be stated in the past tense because they were discovered after you completed your research.
Possible Methodological Limitations
- Sample size -- the number of the units of analysis you use in your study is dictated by the type of research problem you are investigating. Note that, if your sample size is too small, it will be difficult to find significant relationships from the data, as statistical tests normally require a larger sample size to ensure a representative distribution of the population and to be considered representative of groups of people to whom results will be generalized or transferred. Note that sample size is generally less relevant in qualitative research if explained in the context of the research problem.
- Lack of available and/or reliable data -- a lack of data or of reliable data will likely require you to limit the scope of your analysis, the size of your sample, or it can be a significant obstacle in finding a trend and a meaningful relationship. You need to not only describe these limitations but provide cogent reasons why you believe data is missing or is unreliable. However, don’t just throw up your hands in frustration; use this as an opportunity to describe a need for future research based on designing a different method for gathering data.
- Lack of prior research studies on the topic -- citing prior research studies forms the basis of your literature review and helps lay a foundation for understanding the research problem you are investigating. Depending on the currency or scope of your research topic, there may be little, if any, prior research on your topic. Before assuming this to be true, though, consult with a librarian! In cases when a librarian has confirmed that there is little or no prior research, you may be required to develop an entirely new research typology [for example, using an exploratory rather than an explanatory research design ]. Note again that discovering a limitation can serve as an important opportunity to identify new gaps in the literature and to describe the need for further research.
- Measure used to collect the data -- sometimes it is the case that, after completing your interpretation of the findings, you discover that the way in which you gathered data inhibited your ability to conduct a thorough analysis of the results. For example, you regret not including a specific question in a survey that, in retrospect, could have helped address a particular issue that emerged later in the study. Acknowledge the deficiency by stating a need for future researchers to revise the specific method for gathering data.
- Self-reported data -- whether you are relying on pre-existing data or you are conducting a qualitative research study and gathering the data yourself, self-reported data is limited by the fact that it rarely can be independently verified. In other words, you have to the accuracy of what people say, whether in interviews, focus groups, or on questionnaires, at face value. However, self-reported data can contain several potential sources of bias that you should be alert to and note as limitations. These biases become apparent if they are incongruent with data from other sources. These are: (1) selective memory [remembering or not remembering experiences or events that occurred at some point in the past]; (2) telescoping [recalling events that occurred at one time as if they occurred at another time]; (3) attribution [the act of attributing positive events and outcomes to one's own agency, but attributing negative events and outcomes to external forces]; and, (4) exaggeration [the act of representing outcomes or embellishing events as more significant than is actually suggested from other data].
Possible Limitations of the Researcher
- Access -- if your study depends on having access to people, organizations, data, or documents and, for whatever reason, access is denied or limited in some way, the reasons for this needs to be described. Also, include an explanation why being denied or limited access did not prevent you from following through on your study.
- Longitudinal effects -- unlike your professor, who can literally devote years [even a lifetime] to studying a single topic, the time available to investigate a research problem and to measure change or stability over time is constrained by the due date of your assignment. Be sure to choose a research problem that does not require an excessive amount of time to complete the literature review, apply the methodology, and gather and interpret the results. If you're unsure whether you can complete your research within the confines of the assignment's due date, talk to your professor.
- Cultural and other type of bias -- we all have biases, whether we are conscience of them or not. Bias is when a person, place, event, or thing is viewed or shown in a consistently inaccurate way. Bias is usually negative, though one can have a positive bias as well, especially if that bias reflects your reliance on research that only support your hypothesis. When proof-reading your paper, be especially critical in reviewing how you have stated a problem, selected the data to be studied, what may have been omitted, the manner in which you have ordered events, people, or places, how you have chosen to represent a person, place, or thing, to name a phenomenon, or to use possible words with a positive or negative connotation. NOTE : If you detect bias in prior research, it must be acknowledged and you should explain what measures were taken to avoid perpetuating that bias. For example, if a previous study only used boys to examine how music education supports effective math skills, describe how your research expands the study to include girls.
- Fluency in a language -- if your research focuses , for example, on measuring the perceived value of after-school tutoring among Mexican-American ESL [English as a Second Language] students and you are not fluent in Spanish, you are limited in being able to read and interpret Spanish language research studies on the topic or to speak with these students in their primary language. This deficiency should be acknowledged.
Aguinis, Hermam and Jeffrey R. Edwards. “Methodological Wishes for the Next Decade and How to Make Wishes Come True.” Journal of Management Studies 51 (January 2014): 143-174; Brutus, Stéphane et al. "Self-Reported Limitations and Future Directions in Scholarly Reports: Analysis and Recommendations." Journal of Management 39 (January 2013): 48-75; Senunyeme, Emmanuel K. Business Research Methods. Powerpoint Presentation. Regent University of Science and Technology; ter Riet, Gerben et al. “All That Glitters Isn't Gold: A Survey on Acknowledgment of Limitations in Biomedical Studies.” PLOS One 8 (November 2013): 1-6.
Structure and Writing Style
Information about the limitations of your study are generally placed either at the beginning of the discussion section of your paper so the reader knows and understands the limitations before reading the rest of your analysis of the findings, or, the limitations are outlined at the conclusion of the discussion section as an acknowledgement of the need for further study. Statements about a study's limitations should not be buried in the body [middle] of the discussion section unless a limitation is specific to something covered in that part of the paper. If this is the case, though, the limitation should be reiterated at the conclusion of the section.
If you determine that your study is seriously flawed due to important limitations , such as, an inability to acquire critical data, consider reframing it as an exploratory study intended to lay the groundwork for a more complete research study in the future. Be sure, though, to specifically explain the ways that these flaws can be successfully overcome in a new study.
But, do not use this as an excuse for not developing a thorough research paper! Review the tab in this guide for developing a research topic . If serious limitations exist, it generally indicates a likelihood that your research problem is too narrowly defined or that the issue or event under study is too recent and, thus, very little research has been written about it. If serious limitations do emerge, consult with your professor about possible ways to overcome them or how to revise your study.
When discussing the limitations of your research, be sure to:
- Describe each limitation in detailed but concise terms;
- Explain why each limitation exists;
- Provide the reasons why each limitation could not be overcome using the method(s) chosen to acquire or gather the data [cite to other studies that had similar problems when possible];
- Assess the impact of each limitation in relation to the overall findings and conclusions of your study; and,
- If appropriate, describe how these limitations could point to the need for further research.
Remember that the method you chose may be the source of a significant limitation that has emerged during your interpretation of the results [for example, you didn't interview a group of people that you later wish you had]. If this is the case, don't panic. Acknowledge it, and explain how applying a different or more robust methodology might address the research problem more effectively in a future study. A underlying goal of scholarly research is not only to show what works, but to demonstrate what doesn't work or what needs further clarification.
Aguinis, Hermam and Jeffrey R. Edwards. “Methodological Wishes for the Next Decade and How to Make Wishes Come True.” Journal of Management Studies 51 (January 2014): 143-174; Brutus, Stéphane et al. "Self-Reported Limitations and Future Directions in Scholarly Reports: Analysis and Recommendations." Journal of Management 39 (January 2013): 48-75; Ioannidis, John P.A. "Limitations are not Properly Acknowledged in the Scientific Literature." Journal of Clinical Epidemiology 60 (2007): 324-329; Pasek, Josh. Writing the Empirical Social Science Research Paper: A Guide for the Perplexed. January 24, 2012. Academia.edu; Structure: How to Structure the Research Limitations Section of Your Dissertation. Dissertations and Theses: An Online Textbook. Laerd.com; What Is an Academic Paper? Institute for Writing Rhetoric. Dartmouth College; Writing the Experimental Report: Methods, Results, and Discussion. The Writing Lab and The OWL. Purdue University.
Writing Tip
Don't Inflate the Importance of Your Findings!
After all the hard work and long hours devoted to writing your research paper, it is easy to get carried away with attributing unwarranted importance to what you’ve done. We all want our academic work to be viewed as excellent and worthy of a good grade, but it is important that you understand and openly acknowledge the limitations of your study. Inflating the importance of your study's findings could be perceived by your readers as an attempt hide its flaws or encourage a biased interpretation of the results. A small measure of humility goes a long way!
Another Writing Tip
Negative Results are Not a Limitation!
Negative evidence refers to findings that unexpectedly challenge rather than support your hypothesis. If you didn't get the results you anticipated, it may mean your hypothesis was incorrect and needs to be reformulated. Or, perhaps you have stumbled onto something unexpected that warrants further study. Moreover, the absence of an effect may be very telling in many situations, particularly in experimental research designs. In any case, your results may very well be of importance to others even though they did not support your hypothesis. Do not fall into the trap of thinking that results contrary to what you expected is a limitation to your study. If you carried out the research well, they are simply your results and only require additional interpretation.
Lewis, George H. and Jonathan F. Lewis. “The Dog in the Night-Time: Negative Evidence in Social Research.” The British Journal of Sociology 31 (December 1980): 544-558.
Yet Another Writing Tip
Sample Size Limitations in Qualitative Research
Sample sizes are typically smaller in qualitative research because, as the study goes on, acquiring more data does not necessarily lead to more information. This is because one occurrence of a piece of data, or a code, is all that is necessary to ensure that it becomes part of the analysis framework. However, it remains true that sample sizes that are too small cannot adequately support claims of having achieved valid conclusions and sample sizes that are too large do not permit the deep, naturalistic, and inductive analysis that defines qualitative inquiry. Determining adequate sample size in qualitative research is ultimately a matter of judgment and experience in evaluating the quality of the information collected against the uses to which it will be applied and the particular research method and purposeful sampling strategy employed. If the sample size is found to be a limitation, it may reflect your judgment about the methodological technique chosen [e.g., single life history study versus focus group interviews] rather than the number of respondents used.
Boddy, Clive Roland. "Sample Size for Qualitative Research." Qualitative Market Research: An International Journal 19 (2016): 426-432; Huberman, A. Michael and Matthew B. Miles. "Data Management and Analysis Methods." In Handbook of Qualitative Research . Norman K. Denzin and Yvonna S. Lincoln, eds. (Thousand Oaks, CA: Sage, 1994), pp. 428-444; Blaikie, Norman. "Confounding Issues Related to Determining Sample Size in Qualitative Research." International Journal of Social Research Methodology 21 (2018): 635-641; Oppong, Steward Harrison. "The Problem of Sampling in qualitative Research." Asian Journal of Management Sciences and Education 2 (2013): 202-210.
- << Previous: 8. The Discussion
- Next: 9. The Conclusion >>
- Last Updated: Aug 13, 2024 12:57 PM
- URL: https://libguides.usc.edu/writingguide

Experimental Research Design — 6 mistakes you should never make!
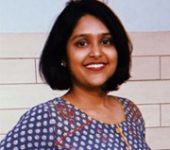
Since school days’ students perform scientific experiments that provide results that define and prove the laws and theorems in science. These experiments are laid on a strong foundation of experimental research designs.
An experimental research design helps researchers execute their research objectives with more clarity and transparency.
In this article, we will not only discuss the key aspects of experimental research designs but also the issues to avoid and problems to resolve while designing your research study.
Table of Contents
What Is Experimental Research Design?
Experimental research design is a framework of protocols and procedures created to conduct experimental research with a scientific approach using two sets of variables. Herein, the first set of variables acts as a constant, used to measure the differences of the second set. The best example of experimental research methods is quantitative research .
Experimental research helps a researcher gather the necessary data for making better research decisions and determining the facts of a research study.
When Can a Researcher Conduct Experimental Research?
A researcher can conduct experimental research in the following situations —
- When time is an important factor in establishing a relationship between the cause and effect.
- When there is an invariable or never-changing behavior between the cause and effect.
- Finally, when the researcher wishes to understand the importance of the cause and effect.
Importance of Experimental Research Design
To publish significant results, choosing a quality research design forms the foundation to build the research study. Moreover, effective research design helps establish quality decision-making procedures, structures the research to lead to easier data analysis, and addresses the main research question. Therefore, it is essential to cater undivided attention and time to create an experimental research design before beginning the practical experiment.
By creating a research design, a researcher is also giving oneself time to organize the research, set up relevant boundaries for the study, and increase the reliability of the results. Through all these efforts, one could also avoid inconclusive results. If any part of the research design is flawed, it will reflect on the quality of the results derived.
Types of Experimental Research Designs
Based on the methods used to collect data in experimental studies, the experimental research designs are of three primary types:
1. Pre-experimental Research Design
A research study could conduct pre-experimental research design when a group or many groups are under observation after implementing factors of cause and effect of the research. The pre-experimental design will help researchers understand whether further investigation is necessary for the groups under observation.
Pre-experimental research is of three types —
- One-shot Case Study Research Design
- One-group Pretest-posttest Research Design
- Static-group Comparison
2. True Experimental Research Design
A true experimental research design relies on statistical analysis to prove or disprove a researcher’s hypothesis. It is one of the most accurate forms of research because it provides specific scientific evidence. Furthermore, out of all the types of experimental designs, only a true experimental design can establish a cause-effect relationship within a group. However, in a true experiment, a researcher must satisfy these three factors —
- There is a control group that is not subjected to changes and an experimental group that will experience the changed variables
- A variable that can be manipulated by the researcher
- Random distribution of the variables
This type of experimental research is commonly observed in the physical sciences.
3. Quasi-experimental Research Design
The word “Quasi” means similarity. A quasi-experimental design is similar to a true experimental design. However, the difference between the two is the assignment of the control group. In this research design, an independent variable is manipulated, but the participants of a group are not randomly assigned. This type of research design is used in field settings where random assignment is either irrelevant or not required.
The classification of the research subjects, conditions, or groups determines the type of research design to be used.
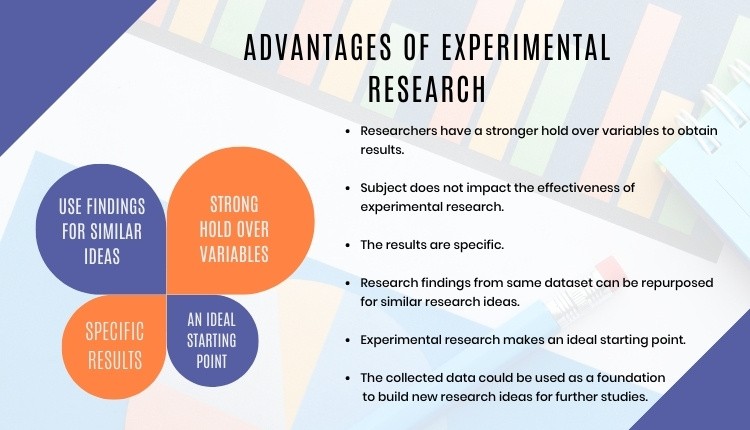
Advantages of Experimental Research
Experimental research allows you to test your idea in a controlled environment before taking the research to clinical trials. Moreover, it provides the best method to test your theory because of the following advantages:
- Researchers have firm control over variables to obtain results.
- The subject does not impact the effectiveness of experimental research. Anyone can implement it for research purposes.
- The results are specific.
- Post results analysis, research findings from the same dataset can be repurposed for similar research ideas.
- Researchers can identify the cause and effect of the hypothesis and further analyze this relationship to determine in-depth ideas.
- Experimental research makes an ideal starting point. The collected data could be used as a foundation to build new research ideas for further studies.
6 Mistakes to Avoid While Designing Your Research
There is no order to this list, and any one of these issues can seriously compromise the quality of your research. You could refer to the list as a checklist of what to avoid while designing your research.
1. Invalid Theoretical Framework
Usually, researchers miss out on checking if their hypothesis is logical to be tested. If your research design does not have basic assumptions or postulates, then it is fundamentally flawed and you need to rework on your research framework.
2. Inadequate Literature Study
Without a comprehensive research literature review , it is difficult to identify and fill the knowledge and information gaps. Furthermore, you need to clearly state how your research will contribute to the research field, either by adding value to the pertinent literature or challenging previous findings and assumptions.
3. Insufficient or Incorrect Statistical Analysis
Statistical results are one of the most trusted scientific evidence. The ultimate goal of a research experiment is to gain valid and sustainable evidence. Therefore, incorrect statistical analysis could affect the quality of any quantitative research.
4. Undefined Research Problem
This is one of the most basic aspects of research design. The research problem statement must be clear and to do that, you must set the framework for the development of research questions that address the core problems.
5. Research Limitations
Every study has some type of limitations . You should anticipate and incorporate those limitations into your conclusion, as well as the basic research design. Include a statement in your manuscript about any perceived limitations, and how you considered them while designing your experiment and drawing the conclusion.
6. Ethical Implications
The most important yet less talked about topic is the ethical issue. Your research design must include ways to minimize any risk for your participants and also address the research problem or question at hand. If you cannot manage the ethical norms along with your research study, your research objectives and validity could be questioned.
Experimental Research Design Example
In an experimental design, a researcher gathers plant samples and then randomly assigns half the samples to photosynthesize in sunlight and the other half to be kept in a dark box without sunlight, while controlling all the other variables (nutrients, water, soil, etc.)
By comparing their outcomes in biochemical tests, the researcher can confirm that the changes in the plants were due to the sunlight and not the other variables.
Experimental research is often the final form of a study conducted in the research process which is considered to provide conclusive and specific results. But it is not meant for every research. It involves a lot of resources, time, and money and is not easy to conduct, unless a foundation of research is built. Yet it is widely used in research institutes and commercial industries, for its most conclusive results in the scientific approach.
Have you worked on research designs? How was your experience creating an experimental design? What difficulties did you face? Do write to us or comment below and share your insights on experimental research designs!
Frequently Asked Questions
Randomization is important in an experimental research because it ensures unbiased results of the experiment. It also measures the cause-effect relationship on a particular group of interest.
Experimental research design lay the foundation of a research and structures the research to establish quality decision making process.
There are 3 types of experimental research designs. These are pre-experimental research design, true experimental research design, and quasi experimental research design.
The difference between an experimental and a quasi-experimental design are: 1. The assignment of the control group in quasi experimental research is non-random, unlike true experimental design, which is randomly assigned. 2. Experimental research group always has a control group; on the other hand, it may not be always present in quasi experimental research.
Experimental research establishes a cause-effect relationship by testing a theory or hypothesis using experimental groups or control variables. In contrast, descriptive research describes a study or a topic by defining the variables under it and answering the questions related to the same.

good and valuable
Very very good
Good presentation.
Rate this article Cancel Reply
Your email address will not be published.
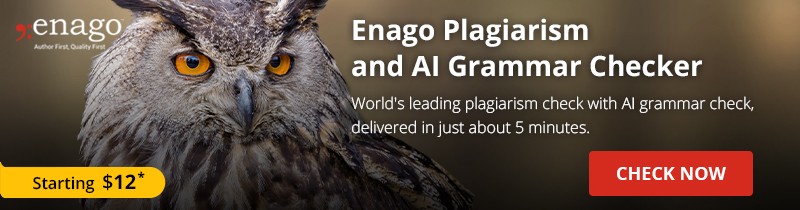
Enago Academy's Most Popular Articles
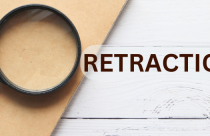
- Publishing Research
10 Tips to Prevent Research Papers From Being Retracted
Research paper retractions represent a critical event in the scientific community. When a published article…
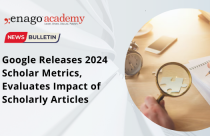
- Industry News
Google Releases 2024 Scholar Metrics, Evaluates Impact of Scholarly Articles
Google has released its 2024 Scholar Metrics, assessing scholarly articles from 2019 to 2023. This…
![limitations in experimental research What is Academic Integrity and How to Uphold it [FREE CHECKLIST]](https://www.enago.com/academy/wp-content/uploads/2024/05/FeatureImages-59-210x136.png)
Ensuring Academic Integrity and Transparency in Academic Research: A comprehensive checklist for researchers
Academic integrity is the foundation upon which the credibility and value of scientific findings are…
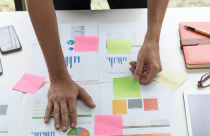
- Reporting Research
How to Optimize Your Research Process: A step-by-step guide
For researchers across disciplines, the path to uncovering novel findings and insights is often filled…
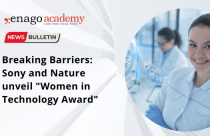
- Trending Now
Breaking Barriers: Sony and Nature unveil “Women in Technology Award”
Sony Group Corporation and the prestigious scientific journal Nature have collaborated to launch the inaugural…
Choosing the Right Analytical Approach: Thematic analysis vs. content analysis for…
Comparing Cross Sectional and Longitudinal Studies: 5 steps for choosing the right…

Sign-up to read more
Subscribe for free to get unrestricted access to all our resources on research writing and academic publishing including:
- 2000+ blog articles
- 50+ Webinars
- 10+ Expert podcasts
- 50+ Infographics
- 10+ Checklists
- Research Guides
We hate spam too. We promise to protect your privacy and never spam you.
- AI in Academia
- Promoting Research
- Career Corner
- Diversity and Inclusion
- Infographics
- Expert Video Library
- Other Resources
- Enago Learn
- Upcoming & On-Demand Webinars
- Peer-Review Week 2023
- Open Access Week 2023
- Conference Videos
- Enago Report
- Journal Finder
- Enago Plagiarism & AI Grammar Check
- Editing Services
- Publication Support Services
- Research Impact
- Translation Services
- Publication solutions
- AI-Based Solutions
- Thought Leadership
- Call for Articles
- Call for Speakers
- Author Training
- Edit Profile
I am looking for Editing/ Proofreading services for my manuscript Tentative date of next journal submission:
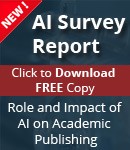
In your opinion, what is the most effective way to improve integrity in the peer review process?
- Privacy Policy

Home » Limitations in Research – Types, Examples and Writing Guide
Limitations in Research – Types, Examples and Writing Guide
Table of Contents

Limitations in Research
Limitations in research refer to the factors that may affect the results, conclusions , and generalizability of a study. These limitations can arise from various sources, such as the design of the study, the sampling methods used, the measurement tools employed, and the limitations of the data analysis techniques.
Types of Limitations in Research
Types of Limitations in Research are as follows:
Sample Size Limitations
This refers to the size of the group of people or subjects that are being studied. If the sample size is too small, then the results may not be representative of the population being studied. This can lead to a lack of generalizability of the results.
Time Limitations
Time limitations can be a constraint on the research process . This could mean that the study is unable to be conducted for a long enough period of time to observe the long-term effects of an intervention, or to collect enough data to draw accurate conclusions.
Selection Bias
This refers to a type of bias that can occur when the selection of participants in a study is not random. This can lead to a biased sample that is not representative of the population being studied.
Confounding Variables
Confounding variables are factors that can influence the outcome of a study, but are not being measured or controlled for. These can lead to inaccurate conclusions or a lack of clarity in the results.
Measurement Error
This refers to inaccuracies in the measurement of variables, such as using a faulty instrument or scale. This can lead to inaccurate results or a lack of validity in the study.
Ethical Limitations
Ethical limitations refer to the ethical constraints placed on research studies. For example, certain studies may not be allowed to be conducted due to ethical concerns, such as studies that involve harm to participants.
Examples of Limitations in Research
Some Examples of Limitations in Research are as follows:
Research Title: “The Effectiveness of Machine Learning Algorithms in Predicting Customer Behavior”
Limitations:
- The study only considered a limited number of machine learning algorithms and did not explore the effectiveness of other algorithms.
- The study used a specific dataset, which may not be representative of all customer behaviors or demographics.
- The study did not consider the potential ethical implications of using machine learning algorithms in predicting customer behavior.
Research Title: “The Impact of Online Learning on Student Performance in Computer Science Courses”
- The study was conducted during the COVID-19 pandemic, which may have affected the results due to the unique circumstances of remote learning.
- The study only included students from a single university, which may limit the generalizability of the findings to other institutions.
- The study did not consider the impact of individual differences, such as prior knowledge or motivation, on student performance in online learning environments.
Research Title: “The Effect of Gamification on User Engagement in Mobile Health Applications”
- The study only tested a specific gamification strategy and did not explore the effectiveness of other gamification techniques.
- The study relied on self-reported measures of user engagement, which may be subject to social desirability bias or measurement errors.
- The study only included a specific demographic group (e.g., young adults) and may not be generalizable to other populations with different preferences or needs.
How to Write Limitations in Research
When writing about the limitations of a research study, it is important to be honest and clear about the potential weaknesses of your work. Here are some tips for writing about limitations in research:
- Identify the limitations: Start by identifying the potential limitations of your research. These may include sample size, selection bias, measurement error, or other issues that could affect the validity and reliability of your findings.
- Be honest and objective: When describing the limitations of your research, be honest and objective. Do not try to minimize or downplay the limitations, but also do not exaggerate them. Be clear and concise in your description of the limitations.
- Provide context: It is important to provide context for the limitations of your research. For example, if your sample size was small, explain why this was the case and how it may have affected your results. Providing context can help readers understand the limitations in a broader context.
- Discuss implications : Discuss the implications of the limitations for your research findings. For example, if there was a selection bias in your sample, explain how this may have affected the generalizability of your findings. This can help readers understand the limitations in terms of their impact on the overall validity of your research.
- Provide suggestions for future research : Finally, provide suggestions for future research that can address the limitations of your study. This can help readers understand how your research fits into the broader field and can provide a roadmap for future studies.
Purpose of Limitations in Research
There are several purposes of limitations in research. Here are some of the most important ones:
- To acknowledge the boundaries of the study : Limitations help to define the scope of the research project and set realistic expectations for the findings. They can help to clarify what the study is not intended to address.
- To identify potential sources of bias: Limitations can help researchers identify potential sources of bias in their research design, data collection, or analysis. This can help to improve the validity and reliability of the findings.
- To provide opportunities for future research: Limitations can highlight areas for future research and suggest avenues for further exploration. This can help to advance knowledge in a particular field.
- To demonstrate transparency and accountability: By acknowledging the limitations of their research, researchers can demonstrate transparency and accountability to their readers, peers, and funders. This can help to build trust and credibility in the research community.
- To encourage critical thinking: Limitations can encourage readers to critically evaluate the study’s findings and consider alternative explanations or interpretations. This can help to promote a more nuanced and sophisticated understanding of the topic under investigation.
When to Write Limitations in Research
Limitations should be included in research when they help to provide a more complete understanding of the study’s results and implications. A limitation is any factor that could potentially impact the accuracy, reliability, or generalizability of the study’s findings.
It is important to identify and discuss limitations in research because doing so helps to ensure that the results are interpreted appropriately and that any conclusions drawn are supported by the available evidence. Limitations can also suggest areas for future research, highlight potential biases or confounding factors that may have affected the results, and provide context for the study’s findings.
Generally, limitations should be discussed in the conclusion section of a research paper or thesis, although they may also be mentioned in other sections, such as the introduction or methods. The specific limitations that are discussed will depend on the nature of the study, the research question being investigated, and the data that was collected.
Examples of limitations that might be discussed in research include sample size limitations, data collection methods, the validity and reliability of measures used, and potential biases or confounding factors that could have affected the results. It is important to note that limitations should not be used as a justification for poor research design or methodology, but rather as a way to enhance the understanding and interpretation of the study’s findings.
Importance of Limitations in Research
Here are some reasons why limitations are important in research:
- Enhances the credibility of research: Limitations highlight the potential weaknesses and threats to validity, which helps readers to understand the scope and boundaries of the study. This improves the credibility of research by acknowledging its limitations and providing a clear picture of what can and cannot be concluded from the study.
- Facilitates replication: By highlighting the limitations, researchers can provide detailed information about the study’s methodology, data collection, and analysis. This information helps other researchers to replicate the study and test the validity of the findings, which enhances the reliability of research.
- Guides future research : Limitations provide insights into areas for future research by identifying gaps or areas that require further investigation. This can help researchers to design more comprehensive and effective studies that build on existing knowledge.
- Provides a balanced view: Limitations help to provide a balanced view of the research by highlighting both strengths and weaknesses. This ensures that readers have a clear understanding of the study’s limitations and can make informed decisions about the generalizability and applicability of the findings.
Advantages of Limitations in Research
Here are some potential advantages of limitations in research:
- Focus : Limitations can help researchers focus their study on a specific area or population, which can make the research more relevant and useful.
- Realism : Limitations can make a study more realistic by reflecting the practical constraints and challenges of conducting research in the real world.
- Innovation : Limitations can spur researchers to be more innovative and creative in their research design and methodology, as they search for ways to work around the limitations.
- Rigor : Limitations can actually increase the rigor and credibility of a study, as researchers are forced to carefully consider the potential sources of bias and error, and address them to the best of their abilities.
- Generalizability : Limitations can actually improve the generalizability of a study by ensuring that it is not overly focused on a specific sample or situation, and that the results can be applied more broadly.
About the author
Muhammad Hassan
Researcher, Academic Writer, Web developer
You may also like

Research Methods – Types, Examples and Guide

Significance of the Study – Examples and Writing...

Critical Analysis – Types, Examples and Writing...

Conceptual Framework – Types, Methodology and...

Assignment – Types, Examples and Writing Guide

Literature Review – Types Writing Guide and...
7 Advantages and Disadvantages of Experimental Research
There are multiple ways to test and do research on new ideas, products, or theories. One of these ways is by experimental research. This is when the researcher has complete control over one set of the variable, and manipulates the others. A good example of this is pharmaceutical research. They will administer the new drug to one group of subjects, and not to the other, while monitoring them both. This way, they can tell the true effects of the drug by comparing them to people who are not taking it. With this type of research design, only one variable can be tested, which may make it more time consuming and open to error. However, if done properly, it is known as one of the most efficient and accurate ways to reach a conclusion. There are other things that go into the decision of whether or not to use experimental research, some bad and some good, let’s take a look at both of these.
The Advantages of Experimental Research
1. A High Level Of Control With experimental research groups, the people conducting the research have a very high level of control over their variables. By isolating and determining what they are looking for, they have a great advantage in finding accurate results.
2. Can Span Across Nearly All Fields Of Research Another great benefit of this type of research design is that it can be used in many different types of situations. Just like pharmaceutical companies can utilize it, so can teachers who want to test a new method of teaching. It is a basic, but efficient type of research.
3. Clear Cut Conclusions Since there is such a high level of control, and only one specific variable is being tested at a time, the results are much more relevant than some other forms of research. You can clearly see the success, failure, of effects when analyzing the data collected.
4. Many Variations Can Be Utilized There is a very wide variety of this type of research. Each can provide different benefits, depending on what is being explored. The investigator has the ability to tailor make the experiment for their own unique situation, while still remaining in the validity of the experimental research design.
The Disadvantages of Experimental Research
1. Largely Subject To Human Errors Just like anything, errors can occur. This is especially true when it comes to research and experiments. Any form of error, whether a systematic (error with the experiment) or random error (uncontrolled or unpredictable), or human errors such as revealing who the control group is, they can all completely destroy the validity of the experiment.
2. Can Create Artificial Situations By having such deep control over the variables being tested, it is very possible that the data can be skewed or corrupted to fit whatever outcome the researcher needs. This is especially true if it is being done for a business or market study.
3. Can Take An Extensive Amount of Time To Do Full Research With experimental testing individual experiments have to be done in order to fully research each variable. This can cause the testing to take a very long amount of time and use a large amount of resources and finances. These costs could transfer onto the company, which could inflate costs for consumers.
Important Facts About Experimental Research
- Experimental Research is most used in medical ways, with animals.
- Every single new medicine or drug is testing using this research design.
- There are countless variations of experimental research, including: probability, sequential, snowball, and quota.
You Might Also Like
Recent Posts
- Only Child Characteristics
- Does Music Affect Your Mood
- Negative Motivation
- Positive Motivation
- External and Internal Locus of Control
- How To Leave An Emotionally Abusive Relationship
- The Ability To Move Things With Your Mind
- How To Tell Is Someone Is Lying About Cheating
- Interpersonal Attraction Definition
- Napoleon Compex Symptoms
Experimental Method In Psychology
Saul McLeod, PhD
Editor-in-Chief for Simply Psychology
BSc (Hons) Psychology, MRes, PhD, University of Manchester
Saul McLeod, PhD., is a qualified psychology teacher with over 18 years of experience in further and higher education. He has been published in peer-reviewed journals, including the Journal of Clinical Psychology.
Learn about our Editorial Process
Olivia Guy-Evans, MSc
Associate Editor for Simply Psychology
BSc (Hons) Psychology, MSc Psychology of Education
Olivia Guy-Evans is a writer and associate editor for Simply Psychology. She has previously worked in healthcare and educational sectors.
On This Page:
The experimental method involves the manipulation of variables to establish cause-and-effect relationships. The key features are controlled methods and the random allocation of participants into controlled and experimental groups .
What is an Experiment?
An experiment is an investigation in which a hypothesis is scientifically tested. An independent variable (the cause) is manipulated in an experiment, and the dependent variable (the effect) is measured; any extraneous variables are controlled.
An advantage is that experiments should be objective. The researcher’s views and opinions should not affect a study’s results. This is good as it makes the data more valid and less biased.
There are three types of experiments you need to know:
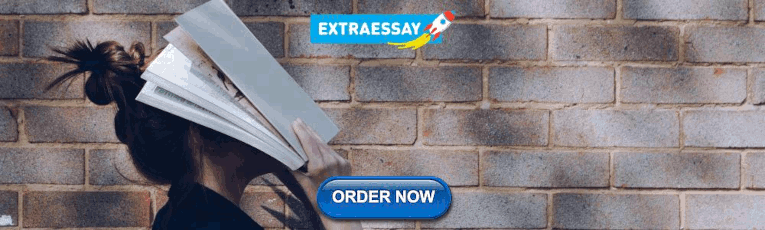
1. Lab Experiment
A laboratory experiment in psychology is a research method in which the experimenter manipulates one or more independent variables and measures the effects on the dependent variable under controlled conditions.
A laboratory experiment is conducted under highly controlled conditions (not necessarily a laboratory) where accurate measurements are possible.
The researcher uses a standardized procedure to determine where the experiment will take place, at what time, with which participants, and in what circumstances.
Participants are randomly allocated to each independent variable group.
Examples are Milgram’s experiment on obedience and Loftus and Palmer’s car crash study .
- Strength : It is easier to replicate (i.e., copy) a laboratory experiment. This is because a standardized procedure is used.
- Strength : They allow for precise control of extraneous and independent variables. This allows a cause-and-effect relationship to be established.
- Limitation : The artificiality of the setting may produce unnatural behavior that does not reflect real life, i.e., low ecological validity. This means it would not be possible to generalize the findings to a real-life setting.
- Limitation : Demand characteristics or experimenter effects may bias the results and become confounding variables .
2. Field Experiment
A field experiment is a research method in psychology that takes place in a natural, real-world setting. It is similar to a laboratory experiment in that the experimenter manipulates one or more independent variables and measures the effects on the dependent variable.
However, in a field experiment, the participants are unaware they are being studied, and the experimenter has less control over the extraneous variables .
Field experiments are often used to study social phenomena, such as altruism, obedience, and persuasion. They are also used to test the effectiveness of interventions in real-world settings, such as educational programs and public health campaigns.
An example is Holfing’s hospital study on obedience .
- Strength : behavior in a field experiment is more likely to reflect real life because of its natural setting, i.e., higher ecological validity than a lab experiment.
- Strength : Demand characteristics are less likely to affect the results, as participants may not know they are being studied. This occurs when the study is covert.
- Limitation : There is less control over extraneous variables that might bias the results. This makes it difficult for another researcher to replicate the study in exactly the same way.
3. Natural Experiment
A natural experiment in psychology is a research method in which the experimenter observes the effects of a naturally occurring event or situation on the dependent variable without manipulating any variables.
Natural experiments are conducted in the day (i.e., real life) environment of the participants, but here, the experimenter has no control over the independent variable as it occurs naturally in real life.
Natural experiments are often used to study psychological phenomena that would be difficult or unethical to study in a laboratory setting, such as the effects of natural disasters, policy changes, or social movements.
For example, Hodges and Tizard’s attachment research (1989) compared the long-term development of children who have been adopted, fostered, or returned to their mothers with a control group of children who had spent all their lives in their biological families.
Here is a fictional example of a natural experiment in psychology:
Researchers might compare academic achievement rates among students born before and after a major policy change that increased funding for education.
In this case, the independent variable is the timing of the policy change, and the dependent variable is academic achievement. The researchers would not be able to manipulate the independent variable, but they could observe its effects on the dependent variable.
- Strength : behavior in a natural experiment is more likely to reflect real life because of its natural setting, i.e., very high ecological validity.
- Strength : Demand characteristics are less likely to affect the results, as participants may not know they are being studied.
- Strength : It can be used in situations in which it would be ethically unacceptable to manipulate the independent variable, e.g., researching stress .
- Limitation : They may be more expensive and time-consuming than lab experiments.
- Limitation : There is no control over extraneous variables that might bias the results. This makes it difficult for another researcher to replicate the study in exactly the same way.
Key Terminology
Ecological validity.
The degree to which an investigation represents real-life experiences.
Experimenter effects
These are the ways that the experimenter can accidentally influence the participant through their appearance or behavior.
Demand characteristics
The clues in an experiment lead the participants to think they know what the researcher is looking for (e.g., the experimenter’s body language).
Independent variable (IV)
The variable the experimenter manipulates (i.e., changes) is assumed to have a direct effect on the dependent variable.
Dependent variable (DV)
Variable the experimenter measures. This is the outcome (i.e., the result) of a study.
Extraneous variables (EV)
All variables which are not independent variables but could affect the results (DV) of the experiment. EVs should be controlled where possible.
Confounding variables
Variable(s) that have affected the results (DV), apart from the IV. A confounding variable could be an extraneous variable that has not been controlled.
Random Allocation
Randomly allocating participants to independent variable conditions means that all participants should have an equal chance of participating in each condition.
The principle of random allocation is to avoid bias in how the experiment is carried out and limit the effects of participant variables.
Order effects
Changes in participants’ performance due to their repeating the same or similar test more than once. Examples of order effects include:
(i) practice effect: an improvement in performance on a task due to repetition, for example, because of familiarity with the task;
(ii) fatigue effect: a decrease in performance of a task due to repetition, for example, because of boredom or tiredness.


21 Research Limitations Examples
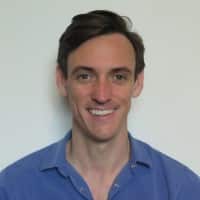
Chris Drew (PhD)
Dr. Chris Drew is the founder of the Helpful Professor. He holds a PhD in education and has published over 20 articles in scholarly journals. He is the former editor of the Journal of Learning Development in Higher Education. [Image Descriptor: Photo of Chris]
Learn about our Editorial Process
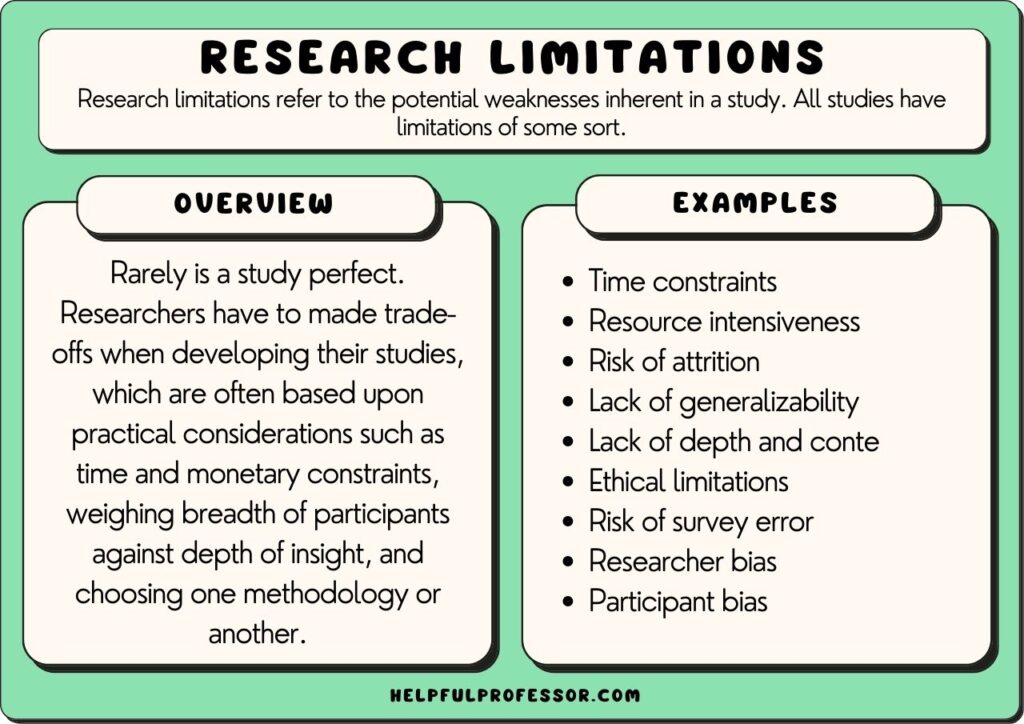
Research limitations refer to the potential weaknesses inherent in a study. All studies have limitations of some sort, meaning declaring limitations doesn’t necessarily need to be a bad thing, so long as your declaration of limitations is well thought-out and explained.
Rarely is a study perfect. Researchers have to make trade-offs when developing their studies, which are often based upon practical considerations such as time and monetary constraints, weighing the breadth of participants against the depth of insight, and choosing one methodology or another.
In research, studies can have limitations such as limited scope, researcher subjectivity, and lack of available research tools.
Acknowledging the limitations of your study should be seen as a strength. It demonstrates your willingness for transparency, humility, and submission to the scientific method and can bolster the integrity of the study. It can also inform future research direction.
Typically, scholars will explore the limitations of their study in either their methodology section, their conclusion section, or both.
Research Limitations Examples
Qualitative and quantitative research offer different perspectives and methods in exploring phenomena, each with its own strengths and limitations. So, I’ve split the limitations examples sections into qualitative and quantitative below.
Qualitative Research Limitations
Qualitative research seeks to understand phenomena in-depth and in context. It focuses on the ‘why’ and ‘how’ questions.
It’s often used to explore new or complex issues, and it provides rich, detailed insights into participants’ experiences, behaviors, and attitudes. However, these strengths also create certain limitations, as explained below.
1. Subjectivity
Qualitative research often requires the researcher to interpret subjective data. One researcher may examine a text and identify different themes or concepts as more dominant than others.
Close qualitative readings of texts are necessarily subjective – and while this may be a limitation, qualitative researchers argue this is the best way to deeply understand everything in context.
Suggested Solution and Response: To minimize subjectivity bias, you could consider cross-checking your own readings of themes and data against other scholars’ readings and interpretations. This may involve giving the raw data to a supervisor or colleague and asking them to code the data separately, then coming together to compare and contrast results.
2. Researcher Bias
The concept of researcher bias is related to, but slightly different from, subjectivity.
Researcher bias refers to the perspectives and opinions you bring with you when doing your research.
For example, a researcher who is explicitly of a certain philosophical or political persuasion may bring that persuasion to bear when interpreting data.
In many scholarly traditions, we will attempt to minimize researcher bias through the utilization of clear procedures that are set out in advance or through the use of statistical analysis tools.
However, in other traditions, such as in postmodern feminist research , declaration of bias is expected, and acknowledgment of bias is seen as a positive because, in those traditions, it is believed that bias cannot be eliminated from research, so instead, it is a matter of integrity to present it upfront.
Suggested Solution and Response: Acknowledge the potential for researcher bias and, depending on your theoretical framework , accept this, or identify procedures you have taken to seek a closer approximation to objectivity in your coding and analysis.
3. Generalizability
If you’re struggling to find a limitation to discuss in your own qualitative research study, then this one is for you: all qualitative research, of all persuasions and perspectives, cannot be generalized.
This is a core feature that sets qualitative data and quantitative data apart.
The point of qualitative data is to select case studies and similarly small corpora and dig deep through in-depth analysis and thick description of data.
Often, this will also mean that you have a non-randomized sample size.
While this is a positive – you’re going to get some really deep, contextualized, interesting insights – it also means that the findings may not be generalizable to a larger population that may not be representative of the small group of people in your study.
Suggested Solution and Response: Suggest future studies that take a quantitative approach to the question.
4. The Hawthorne Effect
The Hawthorne effect refers to the phenomenon where research participants change their ‘observed behavior’ when they’re aware that they are being observed.
This effect was first identified by Elton Mayo who conducted studies of the effects of various factors ton workers’ productivity. He noticed that no matter what he did – turning up the lights, turning down the lights, etc. – there was an increase in worker outputs compared to prior to the study taking place.
Mayo realized that the mere act of observing the workers made them work harder – his observation was what was changing behavior.
So, if you’re looking for a potential limitation to name for your observational research study , highlight the possible impact of the Hawthorne effect (and how you could reduce your footprint or visibility in order to decrease its likelihood).
Suggested Solution and Response: Highlight ways you have attempted to reduce your footprint while in the field, and guarantee anonymity to your research participants.
5. Replicability
Quantitative research has a great benefit in that the studies are replicable – a researcher can get a similar sample size, duplicate the variables, and re-test a study. But you can’t do that in qualitative research.
Qualitative research relies heavily on context – a specific case study or specific variables that make a certain instance worthy of analysis. As a result, it’s often difficult to re-enter the same setting with the same variables and repeat the study.
Furthermore, the individual researcher’s interpretation is more influential in qualitative research, meaning even if a new researcher enters an environment and makes observations, their observations may be different because subjectivity comes into play much more. This doesn’t make the research bad necessarily (great insights can be made in qualitative research), but it certainly does demonstrate a weakness of qualitative research.
6. Limited Scope
“Limited scope” is perhaps one of the most common limitations listed by researchers – and while this is often a catch-all way of saying, “well, I’m not studying that in this study”, it’s also a valid point.
No study can explore everything related to a topic. At some point, we have to make decisions about what’s included in the study and what is excluded from the study.
So, you could say that a limitation of your study is that it doesn’t look at an extra variable or concept that’s certainly worthy of study but will have to be explored in your next project because this project has a clearly and narrowly defined goal.
Suggested Solution and Response: Be clear about what’s in and out of the study when writing your research question.
7. Time Constraints
This is also a catch-all claim you can make about your research project: that you would have included more people in the study, looked at more variables, and so on. But you’ve got to submit this thing by the end of next semester! You’ve got time constraints.
And time constraints are a recognized reality in all research.
But this means you’ll need to explain how time has limited your decisions. As with “limited scope”, this may mean that you had to study a smaller group of subjects, limit the amount of time you spent in the field, and so forth.
Suggested Solution and Response: Suggest future studies that will build on your current work, possibly as a PhD project.
8. Resource Intensiveness
Qualitative research can be expensive due to the cost of transcription, the involvement of trained researchers, and potential travel for interviews or observations.
So, resource intensiveness is similar to the time constraints concept. If you don’t have the funds, you have to make decisions about which tools to use, which statistical software to employ, and how many research assistants you can dedicate to the study.
Suggested Solution and Response: Suggest future studies that will gain more funding on the back of this ‘ exploratory study ‘.
9. Coding Difficulties
Data analysis in qualitative research often involves coding, which can be subjective and complex, especially when dealing with ambiguous or contradicting data.
After naming this as a limitation in your research, it’s important to explain how you’ve attempted to address this. Some ways to ‘limit the limitation’ include:
- Triangulation: Have 2 other researchers code the data as well and cross-check your results with theirs to identify outliers that may need to be re-examined, debated with the other researchers, or removed altogether.
- Procedure: Use a clear coding procedure to demonstrate reliability in your coding process. I personally use the thematic network analysis method outlined in this academic article by Attride-Stirling (2001).
Suggested Solution and Response: Triangulate your coding findings with colleagues, and follow a thematic network analysis procedure.
10. Risk of Non-Responsiveness
There is always a risk in research that research participants will be unwilling or uncomfortable sharing their genuine thoughts and feelings in the study.
This is particularly true when you’re conducting research on sensitive topics, politicized topics, or topics where the participant is expressing vulnerability .
This is similar to the Hawthorne effect (aka participant bias), where participants change their behaviors in your presence; but it goes a step further, where participants actively hide their true thoughts and feelings from you.
Suggested Solution and Response: One way to manage this is to try to include a wider group of people with the expectation that there will be non-responsiveness from some participants.
11. Risk of Attrition
Attrition refers to the process of losing research participants throughout the study.
This occurs most commonly in longitudinal studies , where a researcher must return to conduct their analysis over spaced periods of time, often over a period of years.
Things happen to people over time – they move overseas, their life experiences change, they get sick, change their minds, and even die. The more time that passes, the greater the risk of attrition.
Suggested Solution and Response: One way to manage this is to try to include a wider group of people with the expectation that there will be attrition over time.
12. Difficulty in Maintaining Confidentiality and Anonymity
Given the detailed nature of qualitative data , ensuring participant anonymity can be challenging.
If you have a sensitive topic in a specific case study, even anonymizing research participants sometimes isn’t enough. People might be able to induce who you’re talking about.
Sometimes, this will mean you have to exclude some interesting data that you collected from your final report. Confidentiality and anonymity come before your findings in research ethics – and this is a necessary limiting factor.
Suggested Solution and Response: Highlight the efforts you have taken to anonymize data, and accept that confidentiality and accountability place extremely important constraints on academic research.
13. Difficulty in Finding Research Participants
A study that looks at a very specific phenomenon or even a specific set of cases within a phenomenon means that the pool of potential research participants can be very low.
Compile on top of this the fact that many people you approach may choose not to participate, and you could end up with a very small corpus of subjects to explore. This may limit your ability to make complete findings, even in a quantitative sense.
You may need to therefore limit your research question and objectives to something more realistic.
Suggested Solution and Response: Highlight that this is going to limit the study’s generalizability significantly.
14. Ethical Limitations
Ethical limitations refer to the things you cannot do based on ethical concerns identified either by yourself or your institution’s ethics review board.
This might include threats to the physical or psychological well-being of your research subjects, the potential of releasing data that could harm a person’s reputation, and so on.
Furthermore, even if your study follows all expected standards of ethics, you still, as an ethical researcher, need to allow a research participant to pull out at any point in time, after which you cannot use their data, which demonstrates an overlap between ethical constraints and participant attrition.
Suggested Solution and Response: Highlight that these ethical limitations are inevitable but important to sustain the integrity of the research.
For more on Qualitative Research, Explore my Qualitative Research Guide
Quantitative Research Limitations
Quantitative research focuses on quantifiable data and statistical, mathematical, or computational techniques. It’s often used to test hypotheses, assess relationships and causality, and generalize findings across larger populations.
Quantitative research is widely respected for its ability to provide reliable, measurable, and generalizable data (if done well!). Its structured methodology has strengths over qualitative research, such as the fact it allows for replication of the study, which underpins the validity of the research.
However, this approach is not without it limitations, explained below.
1. Over-Simplification
Quantitative research is powerful because it allows you to measure and analyze data in a systematic and standardized way. However, one of its limitations is that it can sometimes simplify complex phenomena or situations.
In other words, it might miss the subtleties or nuances of the research subject.
For example, if you’re studying why people choose a particular diet, a quantitative study might identify factors like age, income, or health status. But it might miss other aspects, such as cultural influences or personal beliefs, that can also significantly impact dietary choices.
When writing about this limitation, you can say that your quantitative approach, while providing precise measurements and comparisons, may not capture the full complexity of your subjects of study.
Suggested Solution and Response: Suggest a follow-up case study using the same research participants in order to gain additional context and depth.
2. Lack of Context
Another potential issue with quantitative research is that it often focuses on numbers and statistics at the expense of context or qualitative information.
Let’s say you’re studying the effect of classroom size on student performance. You might find that students in smaller classes generally perform better. However, this doesn’t take into account other variables, like teaching style , student motivation, or family support.
When describing this limitation, you might say, “Although our research provides important insights into the relationship between class size and student performance, it does not incorporate the impact of other potentially influential variables. Future research could benefit from a mixed-methods approach that combines quantitative analysis with qualitative insights.”
3. Applicability to Real-World Settings
Oftentimes, experimental research takes place in controlled environments to limit the influence of outside factors.
This control is great for isolation and understanding the specific phenomenon but can limit the applicability or “external validity” of the research to real-world settings.
For example, if you conduct a lab experiment to see how sleep deprivation impacts cognitive performance, the sterile, controlled lab environment might not reflect real-world conditions where people are dealing with multiple stressors.
Therefore, when explaining the limitations of your quantitative study in your methodology section, you could state:
“While our findings provide valuable information about [topic], the controlled conditions of the experiment may not accurately represent real-world scenarios where extraneous variables will exist. As such, the direct applicability of our results to broader contexts may be limited.”
Suggested Solution and Response: Suggest future studies that will engage in real-world observational research, such as ethnographic research.
4. Limited Flexibility
Once a quantitative study is underway, it can be challenging to make changes to it. This is because, unlike in grounded research, you’re putting in place your study in advance, and you can’t make changes part-way through.
Your study design, data collection methods, and analysis techniques need to be decided upon before you start collecting data.
For example, if you are conducting a survey on the impact of social media on teenage mental health, and halfway through, you realize that you should have included a question about their screen time, it’s generally too late to add it.
When discussing this limitation, you could write something like, “The structured nature of our quantitative approach allows for consistent data collection and analysis but also limits our flexibility to adapt and modify the research process in response to emerging insights and ideas.”
Suggested Solution and Response: Suggest future studies that will use mixed-methods or qualitative research methods to gain additional depth of insight.
5. Risk of Survey Error
Surveys are a common tool in quantitative research, but they carry risks of error.
There can be measurement errors (if a question is misunderstood), coverage errors (if some groups aren’t adequately represented), non-response errors (if certain people don’t respond), and sampling errors (if your sample isn’t representative of the population).
For instance, if you’re surveying college students about their study habits , but only daytime students respond because you conduct the survey during the day, your results will be skewed.
In discussing this limitation, you might say, “Despite our best efforts to develop a comprehensive survey, there remains a risk of survey error, including measurement, coverage, non-response, and sampling errors. These could potentially impact the reliability and generalizability of our findings.”
Suggested Solution and Response: Suggest future studies that will use other survey tools to compare and contrast results.
6. Limited Ability to Probe Answers
With quantitative research, you typically can’t ask follow-up questions or delve deeper into participants’ responses like you could in a qualitative interview.
For instance, imagine you are surveying 500 students about study habits in a questionnaire. A respondent might indicate that they study for two hours each night. You might want to follow up by asking them to elaborate on what those study sessions involve or how effective they feel their habits are.
However, quantitative research generally disallows this in the way a qualitative semi-structured interview could.
When discussing this limitation, you might write, “Given the structured nature of our survey, our ability to probe deeper into individual responses is limited. This means we may not fully understand the context or reasoning behind the responses, potentially limiting the depth of our findings.”
Suggested Solution and Response: Suggest future studies that engage in mixed-method or qualitative methodologies to address the issue from another angle.
7. Reliance on Instruments for Data Collection
In quantitative research, the collection of data heavily relies on instruments like questionnaires, surveys, or machines.
The limitation here is that the data you get is only as good as the instrument you’re using. If the instrument isn’t designed or calibrated well, your data can be flawed.
For instance, if you’re using a questionnaire to study customer satisfaction and the questions are vague, confusing, or biased, the responses may not accurately reflect the customers’ true feelings.
When discussing this limitation, you could say, “Our study depends on the use of questionnaires for data collection. Although we have put significant effort into designing and testing the instrument, it’s possible that inaccuracies or misunderstandings could potentially affect the validity of the data collected.”
Suggested Solution and Response: Suggest future studies that will use different instruments but examine the same variables to triangulate results.
8. Time and Resource Constraints (Specific to Quantitative Research)
Quantitative research can be time-consuming and resource-intensive, especially when dealing with large samples.
It often involves systematic sampling, rigorous design, and sometimes complex statistical analysis.
If resources and time are limited, it can restrict the scale of your research, the techniques you can employ, or the extent of your data analysis.
For example, you may want to conduct a nationwide survey on public opinion about a certain policy. However, due to limited resources, you might only be able to survey people in one city.
When writing about this limitation, you could say, “Given the scope of our research and the resources available, we are limited to conducting our survey within one city, which may not fully represent the nationwide public opinion. Hence, the generalizability of the results may be limited.”
Suggested Solution and Response: Suggest future studies that will have more funding or longer timeframes.
How to Discuss Your Research Limitations
1. in your research proposal and methodology section.
In the research proposal, which will become the methodology section of your dissertation, I would recommend taking the four following steps, in order:
- Be Explicit about your Scope – If you limit the scope of your study in your research question, aims, and objectives, then you can set yourself up well later in the methodology to say that certain questions are “outside the scope of the study.” For example, you may identify the fact that the study doesn’t address a certain variable, but you can follow up by stating that the research question is specifically focused on the variable that you are examining, so this limitation would need to be looked at in future studies.
- Acknowledge the Limitation – Acknowledging the limitations of your study demonstrates reflexivity and humility and can make your research more reliable and valid. It also pre-empts questions the people grading your paper may have, so instead of them down-grading you for your limitations; they will congratulate you on explaining the limitations and how you have addressed them!
- Explain your Decisions – You may have chosen your approach (despite its limitations) for a very specific reason. This might be because your approach remains, on balance, the best one to answer your research question. Or, it might be because of time and monetary constraints that are outside of your control.
- Highlight the Strengths of your Approach – Conclude your limitations section by strongly demonstrating that, despite limitations, you’ve worked hard to minimize the effects of the limitations and that you have chosen your specific approach and methodology because it’s also got some terrific strengths. Name the strengths.
Overall, you’ll want to acknowledge your own limitations but also explain that the limitations don’t detract from the value of your study as it stands.
2. In the Conclusion Section or Chapter
In the conclusion of your study, it is generally expected that you return to a discussion of the study’s limitations. Here, I recommend the following steps:
- Acknowledge issues faced – After completing your study, you will be increasingly aware of issues you may have faced that, if you re-did the study, you may have addressed earlier in order to avoid those issues. Acknowledge these issues as limitations, and frame them as recommendations for subsequent studies.
- Suggest further research – Scholarly research aims to fill gaps in the current literature and knowledge. Having established your expertise through your study, suggest lines of inquiry for future researchers. You could state that your study had certain limitations, and “future studies” can address those limitations.
- Suggest a mixed methods approach – Qualitative and quantitative research each have pros and cons. So, note those ‘cons’ of your approach, then say the next study should approach the topic using the opposite methodology or could approach it using a mixed-methods approach that could achieve the benefits of quantitative studies with the nuanced insights of associated qualitative insights as part of an in-study case-study.
Overall, be clear about both your limitations and how those limitations can inform future studies.
In sum, each type of research method has its own strengths and limitations. Qualitative research excels in exploring depth, context, and complexity, while quantitative research excels in examining breadth, generalizability, and quantifiable measures. Despite their individual limitations, each method contributes unique and valuable insights, and researchers often use them together to provide a more comprehensive understanding of the phenomenon being studied.
Attride-Stirling, J. (2001). Thematic networks: an analytic tool for qualitative research. Qualitative research , 1 (3), 385-405. ( Source )
Atkinson, P., Delamont, S., Cernat, A., Sakshaug, J., & Williams, R. A. (2021). SAGE research methods foundations . London: Sage Publications.
Clark, T., Foster, L., Bryman, A., & Sloan, L. (2021). Bryman’s social research methods . Oxford: Oxford University Press.
Köhler, T., Smith, A., & Bhakoo, V. (2022). Templates in qualitative research methods: Origins, limitations, and new directions. Organizational Research Methods , 25 (2), 183-210. ( Source )
Lenger, A. (2019). The rejection of qualitative research methods in economics. Journal of Economic Issues , 53 (4), 946-965. ( Source )
Taherdoost, H. (2022). What are different research approaches? Comprehensive review of qualitative, quantitative, and mixed method research, their applications, types, and limitations. Journal of Management Science & Engineering Research , 5 (1), 53-63. ( Source )
Walliman, N. (2021). Research methods: The basics . New York: Routledge.
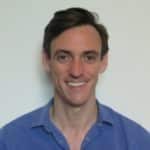
- Chris Drew (PhD) https://helpfulprofessor.com/author/chris-drew-phd-2/ 25 Number Games for Kids (Free and Easy)
- Chris Drew (PhD) https://helpfulprofessor.com/author/chris-drew-phd-2/ 25 Word Games for Kids (Free and Easy)
- Chris Drew (PhD) https://helpfulprofessor.com/author/chris-drew-phd-2/ 25 Outdoor Games for Kids
- Chris Drew (PhD) https://helpfulprofessor.com/author/chris-drew-phd-2/ 50 Incentives to Give to Students
Leave a Comment Cancel Reply
Your email address will not be published. Required fields are marked *
- Experimental Vs Non-Experimental Research: 15 Key Differences

There is a general misconception around research that once the research is non-experimental, then it is non-scientific, making it more important to understand what experimental and experimental research entails. Experimental research is the most common type of research, which a lot of people refer to as scientific research.
Non experimental research, on the other hand, is easily used to classify research that is not experimental. It clearly differs from experimental research, and as such has different use cases.
In this article, we will be explaining these differences in detail so as to ensure proper identification during the research process.
What is Experimental Research?
Experimental research is the type of research that uses a scientific approach towards manipulating one or more control variables of the research subject(s) and measuring the effect of this manipulation on the subject. It is known for the fact that it allows the manipulation of control variables.
This research method is widely used in various physical and social science fields, even though it may be quite difficult to execute. Within the information field, they are much more common in information systems research than in library and information management research.
Experimental research is usually undertaken when the goal of the research is to trace cause-and-effect relationships between defined variables. However, the type of experimental research chosen has a significant influence on the results of the experiment.
Therefore bringing us to the different types of experimental research. There are 3 main types of experimental research, namely; pre-experimental, quasi-experimental, and true experimental research.
Pre-experimental Research
Pre-experimental research is the simplest form of research, and is carried out by observing a group or groups of dependent variables after the treatment of an independent variable which is presumed to cause change on the group(s). It is further divided into three types.
- One-shot case study research
- One-group pretest-posttest research
- Static-group comparison
Quasi-experimental Research
The Quasi type of experimental research is similar to true experimental research, but uses carefully selected rather than randomized subjects. The following are examples of quasi-experimental research:
- Time series
- No equivalent control group design
- Counterbalanced design.
True Experimental Research
True experimental research is the most accurate type, and may simply be called experimental research. It manipulates a control group towards a group of randomly selected subjects and records the effect of this manipulation.
True experimental research can be further classified into the following groups:
- The posttest-only control group
- The pretest-posttest control group
- Solomon four-group
Pros of True Experimental Research
- Researchers can have control over variables.
- It can be combined with other research methods.
- The research process is usually well structured.
- It provides specific conclusions.
- The results of experimental research can be easily duplicated.
Cons of True Experimental Research
- It is highly prone to human error.
- Exerting control over extraneous variables may lead to the personal bias of the researcher.
- It is time-consuming.
- It is expensive.
- Manipulating control variables may have ethical implications.
- It produces artificial results.
What is Non-Experimental Research?
Non-experimental research is the type of research that does not involve the manipulation of control or independent variable. In non-experimental research, researchers measure variables as they naturally occur without any further manipulation.
This type of research is used when the researcher has no specific research question about a causal relationship between 2 different variables, and manipulation of the independent variable is impossible. They are also used when:
- subjects cannot be randomly assigned to conditions.
- the research subject is about a causal relationship but the independent variable cannot be manipulated.
- the research is broad and exploratory
- the research pertains to a non-causal relationship between variables.
- limited information can be accessed about the research subject.
There are 3 main types of non-experimental research , namely; cross-sectional research, correlation research, and observational research.
Cross-sectional Research
Cross-sectional research involves the comparison of two or more pre-existing groups of people under the same criteria. This approach is classified as non-experimental because the groups are not randomly selected and the independent variable is not manipulated.
For example, an academic institution may want to reward its first-class students with a scholarship for their academic excellence. Therefore, each faculty places students in the eligible and ineligible group according to their class of degree.
In this case, the student’s class of degree cannot be manipulated to qualify him or her for a scholarship because it is an unethical thing to do. Therefore, the placement is cross-sectional.
Correlational Research
Correlational type of research compares the statistical relationship between two variables .Correlational research is classified as non-experimental because it does not manipulate the independent variables.
For example, a researcher may wish to investigate the relationship between the class of family students come from and their grades in school. A questionnaire may be given to students to know the average income of their family, then compare it with CGPAs.
The researcher will discover whether these two factors are positively correlated, negatively corrected, or have zero correlation at the end of the research.
Observational Research
Observational research focuses on observing the behavior of a research subject in a natural or laboratory setting. It is classified as non-experimental because it does not involve the manipulation of independent variables.
A good example of observational research is an investigation of the crowd effect or psychology in a particular group of people. Imagine a situation where there are 2 ATMs at a place, and only one of the ATMs is filled with a queue, while the other is abandoned.
The crowd effect infers that the majority of newcomers will also abandon the other ATM.
You will notice that each of these non-experimental research is descriptive in nature. It then suffices to say that descriptive research is an example of non-experimental research.
Pros of Observational Research
- The research process is very close to a real-life situation.
- It does not allow for the manipulation of variables due to ethical reasons.
- Human characteristics are not subject to experimental manipulation.
Cons of Observational Research
- The groups may be dissimilar and nonhomogeneous because they are not randomly selected, affecting the authenticity and generalizability of the study results.
- The results obtained cannot be absolutely clear and error-free.
What Are The Differences Between Experimental and Non-Experimental Research?
- Definitions
Experimental research is the type of research that uses a scientific approach towards manipulating one or more control variables and measuring their defect on the dependent variables, while non-experimental research is the type of research that does not involve the manipulation of control variables.
The main distinction in these 2 types of research is their attitude towards the manipulation of control variables. Experimental allows for the manipulation of control variables while non-experimental research doesn’t.
Examples of experimental research are laboratory experiments that involve mixing different chemical elements together to see the effect of one element on the other while non-experimental research examples are investigations into the characteristics of different chemical elements.
Consider a researcher carrying out a laboratory test to determine the effect of adding Nitrogen gas to Hydrogen gas. It may be discovered that using the Haber process, one can create Nitrogen gas.
Non-experimental research may further be carried out on Ammonia, to determine its characteristics, behaviour, and nature.
There are 3 types of experimental research, namely; experimental research, quasi-experimental research, and true experimental research. Although also 3 in number, non-experimental research can be classified into cross-sectional research, correlational research, and observational research.
The different types of experimental research are further divided into different parts, while non-experimental research types are not further divided. Clearly, these divisions are not the same in experimental and non-experimental research.
- Characteristics
Experimental research is usually quantitative, controlled, and multivariable. Non-experimental research can be both quantitative and qualitative , has an uncontrolled variable, and also a cross-sectional research problem.
The characteristics of experimental research are the direct opposite of that of non-experimental research. The most distinct characteristic element is the ability to control or manipulate independent variables in experimental research and not in non-experimental research.
In experimental research, a level of control is usually exerted on extraneous variables, therefore tampering with the natural research setting. Experimental research settings are usually more natural with no tampering with the extraneous variables.
- Data Collection/Tools
The data used during experimental research is collected through observational study, simulations, and surveys while non-experimental data is collected through observations, surveys, and case studies. The main distinction between these data collection tools is case studies and simulations.
Even at that, similar tools are used differently. For example, an observational study may be used during a laboratory experiment that tests how the effect of a control variable manifests over a period of time in experimental research.
However, when used in non-experimental research, data is collected based on the researcher’s discretion and not through a clear scientific reaction. In this case, we see a difference in the level of objectivity.
The goal of experimental research is to measure the causes and effects of variables present in research, while non-experimental research provides very little to no information about causal agents.
Experimental research answers the question of why something is happening. This is quite different in non-experimental research, as they are more descriptive in nature with the end goal being to describe what .
Experimental research is mostly used to make scientific innovations and find major solutions to problems while non-experimental research is used to define subject characteristics, measure data trends, compare situations and validate existing conditions.
For example, if experimental research results in an innovative discovery or solution, non-experimental research will be conducted to validate this discovery. This research is done for a period of time in order to properly study the subject of research.
Experimental research process is usually well structured and as such produces results with very little to no errors, while non-experimental research helps to create real-life related experiments. There are a lot more advantages of experimental and non-experimental research , with the absence of each of these advantages in the other leaving it at a disadvantage.
For example, the lack of a random selection process in non-experimental research leads to the inability to arrive at a generalizable result. Similarly, the ability to manipulate control variables in experimental research may lead to the personal bias of the researcher.
- Disadvantage
Experimental research is highly prone to human error while the major disadvantage of non-experimental research is that the results obtained cannot be absolutely clear and error-free. In the long run, the error obtained due to human error may affect the results of the experimental research.
Some other disadvantages of experimental research include the following; extraneous variables cannot always be controlled, human responses can be difficult to measure, and participants may also cause bias.
In experimental research, researchers can control and manipulate control variables, while in non-experimental research, researchers cannot manipulate these variables. This cannot be done due to ethical reasons.
For example, when promoting employees due to how well they did in their annual performance review, it will be unethical to manipulate the results of the performance review (independent variable). That way, we can get impartial results of those who deserve a promotion and those who don’t.
Experimental researchers may also decide to eliminate extraneous variables so as to have enough control over the research process. Once again, this is something that cannot be done in non-experimental research because it relates more to real-life situations.
Experimental research is carried out in an unnatural setting because most of the factors that influence the setting are controlled while the non-experimental research setting remains natural and uncontrolled. One of the things usually tampered with during research is extraneous variables.
In a bid to get a perfect and well-structured research process and results, researchers sometimes eliminate extraneous variables. Although sometimes seen as insignificant, the elimination of these variables may affect the research results.
Consider the optimization problem whose aim is to minimize the cost of production of a car, with the constraints being the number of workers and the number of hours they spend working per day.
In this problem, extraneous variables like machine failure rates or accidents are eliminated. In the long run, these things may occur and may invalidate the result.
- Cause-Effect Relationship
The relationship between cause and effect is established in experimental research while it cannot be established in non-experimental research. Rather than establish a cause-effect relationship, non-experimental research focuses on providing descriptive results.
Although it acknowledges the causal variable and its effect on the dependent variables, it does not measure how or the extent to which these dependent variables change. It, however, observes these changes, compares the changes in 2 variables, and describes them.
Experimental research does not compare variables while non-experimental research does. It compares 2 variables and describes the relationship between them.
The relationship between these variables can be positively correlated, negatively correlated or not correlated at all. For example, consider a case whereby the subject of research is a drum, and the control or independent variable is the drumstick.
Experimental research will measure the effect of hitting the drumstick on the drum, where the result of this research will be sound. That is, when you hit a drumstick on a drum, it makes a sound.
Non-experimental research, on the other hand, will investigate the correlation between how hard the drum is hit and the loudness of the sound that comes out. That is, if the sound will be higher with a harder bang, lower with a harder bang, or will remain the same no matter how hard we hit the drum.
- Quantitativeness
Experimental research is a quantitative research method while non-experimental research can be both quantitative and qualitative depending on the time and the situation where it is been used. An example of a non-experimental quantitative research method is correlational research .
Researchers use it to correlate two or more variables using mathematical analysis methods. The original patterns, relationships, and trends between variables are observed, then the impact of one of these variables on the other is recorded along with how it changes the relationship between the two variables.
Observational research is an example of non-experimental research, which is classified as a qualitative research method.
- Cross-section
Experimental research is usually single-sectional while non-experimental research is cross-sectional. That is, when evaluating the research subjects in experimental research, each group is evaluated as an entity.
For example, let us consider a medical research process investigating the prevalence of breast cancer in a certain community. In this community, we will find people of different ages, ethnicities, and social backgrounds.
If a significant amount of women from a particular age are found to be more prone to have the disease, the researcher can conduct further studies to understand the reason behind it. A further study into this will be experimental and the subject won’t be a cross-sectional group.
A lot of researchers consider the distinction between experimental and non-experimental research to be an extremely important one. This is partly due to the fact that experimental research can accommodate the manipulation of independent variables, which is something non-experimental research can not.
Therefore, as a researcher who is interested in using any one of experimental and non-experimental research, it is important to understand the distinction between these two. This helps in deciding which method is better for carrying out particular research.
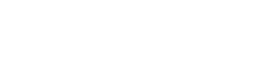
Connect to Formplus, Get Started Now - It's Free!
- examples of experimental research
- non experimental research
- busayo.longe
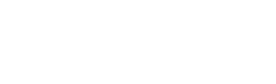
You may also like:
What is Experimenter Bias? Definition, Types & Mitigation
In this article, we will look into the concept of experimental bias and how it can be identified in your research

Simpson’s Paradox & How to Avoid it in Experimental Research
In this article, we are going to look at Simpson’s Paradox from its historical point and later, we’ll consider its effect in...
Experimental Research Designs: Types, Examples & Methods
Ultimate guide to experimental research. It’s definition, types, characteristics, uses, examples and methodolgy
Response vs Explanatory Variables: Definition & Examples
In this article, we’ll be comparing the two types of variables, what they both mean and see some of their real-life applications in research
Formplus - For Seamless Data Collection
Collect data the right way with a versatile data collection tool. try formplus and transform your work productivity today..
Advantages and Limitations of Experiments for Researching Participatory Enterprise Modeling and Recommendations for Their Implementation
- Conference paper
- First Online: 17 November 2022
- Cite this conference paper
- Anne Gutschmidt ORCID: orcid.org/0000-0001-8038-4435 8
Part of the book series: Lecture Notes in Business Information Processing ((LNBIP,volume 456))
Included in the following conference series:
- IFIP Working Conference on The Practice of Enterprise Modeling
517 Accesses
Participatory enterprise modeling (PEM) means that stakeholders become directly involved in the process of creating enterprise models. Based on their different perspectives, they discuss and exchange knowledge and ideas in joint meetings and, with the support of modeling experts, they collaboratively create the models. Although there is a lot of empirical and theoretical work on group work and collaboration that we can build on, there are still many aspects of PEM that we should research. The participatory approach is claimed to lead to higher model quality and commitment, empirical evidence is, however, still scarce. Moreover, there are many factors that might influence productivity and the outcome of participatory modeling projects, such as facilitation methods or the tools used for modeling. In this paper, I will discuss the special value, but also methodical challenges and limitations of experimental studies on PEM compared to surveys and case studies. I will give methodical recommendations on how to design and implement experiments on PEM and discuss how they can eventually add to case studies carried out in companies.
This is a preview of subscription content, log in via an institution to check access.
Access this chapter
Subscribe and save.
- Get 10 units per month
- Download Article/Chapter or eBook
- 1 Unit = 1 Article or 1 Chapter
- Cancel anytime
- Available as PDF
- Read on any device
- Instant download
- Own it forever
- Available as EPUB and PDF
- Compact, lightweight edition
- Dispatched in 3 to 5 business days
- Free shipping worldwide - see info
Tax calculation will be finalised at checkout
Purchases are for personal use only
Institutional subscriptions
Similar content being viewed by others
A Study on the Impact of the Level of Participation in Enterprise Modeling
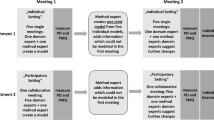
Participatory modeling from a stakeholder perspective: On the influence of collaboration and revisions on psychological ownership and perceived model quality
Researching Participatory Modeling Sessions: An Experimental Study on the Influence of Evaluation Potential and the Opportunity to Draw Oneself
Barjis, J.: CPI modeling: collaborative, participative, interactive modeling. In: Proceedings of the 2011 Winter Simulation Conference (WSC), pp. 3094–3103 (2011). https://doi.org/10.1109/WSC.2011.6148009
Bowling, A., Ebrahim, S.: Quantitative social science: the survey. In: Handbook of Health Research Methods: Investigation, Measurement and Analysis, pp. 190–214 (2005)
Google Scholar
Deith, M.C., et al.: Lessons learned for collaborative approaches to management when faced with diverse stakeholder groups in a rebuilding fishery. Mar. Policy 130 , 104555 (2021). https://doi.org/10.1016/j.marpol.2021.104555 . https://www.sciencedirect.com/science/article/pii/S0308597X21001664
Döring, N., Bortz, J.: Forschungsmethoden und Evaluation in den Sozial- und Humanwissenschaften, 5th edn. Springer, Heidelberg (2016). https://doi.org/10.1007/978-3-642-41089-5
Book Google Scholar
Fellmann, M., Sandkuhl, K., Gutschmidt, A., Poppe, M.: Structuring participatory enterprise modelling sessions. In: Grabis, J., Bork, D. (eds.) PoEM 2020. LNBIP, vol. 400, pp. 58–72. Springer, Cham (2020). https://doi.org/10.1007/978-3-030-63479-7_5
Chapter Google Scholar
Field, A.: Discovering Statistics Using IBM SPSS Statistics. Sage, Thousand Oaks (2013)
Fischer, M., Rosenthal, K., Strecker, S.: Experimentation in conceptual modeling research: a systematic review. In: AMCIS (2019)
Gjersvik, R., Krogstie, J., Folstad, A.: Participatory development of enterprise process models. In: Information Modeling Methods and Methodologies, pp. 195–215 (2005). https://doi.org/10.4018/978-1-59140-375-3.ch010
Goodrick, D.: Comparative Case Studies. SAGE Publications Limited, Thousand Oaks (2020)
Gutschmidt, A.: Empirical insights into the appraisal of tool support for participative enterprise modeling. In: EMISA Forum, vol. 38, no. 1. De Gruyter (2018)
Gutschmidt, A.: On the influence of tools on collaboration in participative enterprise modeling—an experimental comparison between whiteboard and multi-touch table. In: Andersson, B., Johansson, B., Barry, C., Lang, M., Linger, H., Schneider, C. (eds.) Advances in Information Systems Development. LNISO, vol. 34, pp. 151–168. Springer, Cham (2019). https://doi.org/10.1007/978-3-030-22993-1_9
Gutschmidt, A.: An exploratory comparison of tools for remote collaborative and participatory enterprise modeling. In: ECIS 2021 Research-in-Progress Papers (2021)
Gutschmidt, A., Lantow, B., Hellmanzik, B., Ramforth, B., Wiese, M., Martins, E.: Participatory modeling from a stakeholder perspective: on the influence of collaboration and revisions on psychological ownership and perceived model quality. Softw. Syst. Model. 1–17 (2022). https://doi.org/10.1007/s10270-022-01036-7
Gutschmidt, A., Sauer, V., Schönwälder, M., Szilagyi, T.: Researching participatory modeling sessions: an experimental study on the influence of evaluation potential and the opportunity to draw oneself. In: Pańkowska, M., Sandkuhl, K. (eds.) BIR 2019. LNBIP, vol. 365, pp. 44–58. Springer, Cham (2019). https://doi.org/10.1007/978-3-030-31143-8_4
Koç, H., Sandkuhl, K., Stirna, J.: Design thinking and enterprise modeling: an investigation of eight enterprise architecture management projects. In: Augusto, A., Gill, A., Nurcan, S., Reinhartz-Berger, I., Schmidt, R., Zdravkovic, J. (eds.) BPMDS/EMMSAD -2021. LNBIP, vol. 421, pp. 228–242. Springer, Cham (2021). https://doi.org/10.1007/978-3-030-79186-5_15
Krogstie, J.: Model-Based Development and Evolution of Information Systems: A Quality Approach. Springer, London (2012). https://doi.org/10.1007/978-1-4471-2936-3
Luebbe, A., Weske, M.: Tangible media in process modeling – a controlled experiment. In: Mouratidis, H., Rolland, C. (eds.) CAiSE 2011. LNCS, vol. 6741, pp. 283–298. Springer, Heidelberg (2011). https://doi.org/10.1007/978-3-642-21640-4_22
Nolte, A., Herrmann, T.: Facilitating participation of stakeholders during process analysis and design. In: De Angeli, A., Bannon, L., Marti, P., Bordin, S. (eds.) COOP 2016: Proceedings of the 12th International Conference on the Design of Cooperative Systems, 23-27 May 2016, Trento, Italy, pp. 225–241. Springer, Cham (2016). https://doi.org/10.1007/978-3-319-33464-6_14
Pierce, J.L., Jussila, I.: Collective psychological ownership within the work and organizational context: construct introduction and elaboration. J. Organ. Behav. 31 (6), 810–834 (2010)
Article Google Scholar
Rittgen, P.: Collaborative modeling of business processes: a comparative case study. In: Proceedings of the 2009 ACM Symposium on Applied Computing, SAC 2009, pp. 225–230. Association for Computing Machinery, New York (2009). https://doi.org/10.1145/1529282.1529333
Runeson, P., Höst, M.: Guidelines for conducting and reporting case study research in software engineering. Empir. Softw. Eng. 14 (2), 131–164 (2009). https://doi.org/10.1007/s10664-008-9102-8
Sandkuhl, K., Seigerroth, U.: Participative or conventional enterprise modelling? Multiple-case analysis on decision criteria. In: Rowe, F., et al. (eds.) 28th European Conference on Information Systems - Liberty, Equality, and Fraternity in a Digitizing World, ECIS 2020, Marrakech, Morocco, 15–17 June 2020 (2020)
Sandkuhl, K., Stirna, J., Persson, A., Wißotzki, M.: Enterprise Modeling: Tackling Business Challenges with the 4EM Method. The Enterprise Engineering Series, Springer, Heidelberg (2014). https://doi.org/10.1007/978-3-662-43725-4
Stirna, J., Persson, A.: Enterprise Modeling - Facilitating the Process and the People. Springer, Cham (2018). https://doi.org/10.1007/978-3-319-94857-7
Stirna, J., Persson, A., Sandkuhl, K.: Participative enterprise modeling: experiences and recommendations. In: Krogstie, J., Opdahl, A., Sindre, G. (eds.) CAiSE 2007. LNCS, vol. 4495, pp. 546–560. Springer, Heidelberg (2007). https://doi.org/10.1007/978-3-540-72988-4_38
Van Dyne, L., Pierce, J.L.: Psychological ownership and feelings of possession: three field studies predicting employee attitudes and organizational citizenship behavior. J. Organ. Behav. Int. J. Ind. Occup. Organ. Psychol. Behav. 25 (4), 439–459 (2004)
Veisi, H., Jackson-Smith, D., Arrueta, L.: Alignment of stakeholder and scientist understandings and expectations in a participatory modeling project. Environ. Sci. Policy 134 , 57–66 (2022)
Vernadat, F.B.: Enterprise modelling and integration. In: Kosanke, K., Jochem, R., Nell, J.G., Bas, A.O. (eds.) Enterprise Inter- and Intra-Organizational Integration. ITIFIP, vol. 108, pp. 25–33. Springer, Boston, MA (2003). https://doi.org/10.1007/978-0-387-35621-1_4
Wohlin, C., Runeson, P., Höst, M., Ohlsson, M.C., Regnell, B., Wesslén, A.: Experimentation in Software Engineering. Springer, Heidelberg (2012). https://doi.org/10.1007/978-3-642-29044-2
Book MATH Google Scholar
Yin, R.K.: Case Study Research: Design and Methods, vol. 5. Sage, Thousand Oaks (2009)
Download references
Author information
Authors and affiliations.
University of Rostock, 18059, Rostock, Germany
Anne Gutschmidt
You can also search for this author in PubMed Google Scholar
Corresponding author
Correspondence to Anne Gutschmidt .
Editor information
Editors and affiliations.
Middlesex University, London, UK
Balbir S. Barn
Rostock University, Rostock, Germany
Kurt Sandkuhl
Rights and permissions
Reprints and permissions
Copyright information
© 2022 IFIP International Federation for Information Processing
About this paper
Cite this paper.
Gutschmidt, A. (2022). Advantages and Limitations of Experiments for Researching Participatory Enterprise Modeling and Recommendations for Their Implementation. In: Barn, B.S., Sandkuhl, K. (eds) The Practice of Enterprise Modeling. PoEM 2022. Lecture Notes in Business Information Processing, vol 456. Springer, Cham. https://doi.org/10.1007/978-3-031-21488-2_14
Download citation
DOI : https://doi.org/10.1007/978-3-031-21488-2_14
Published : 17 November 2022
Publisher Name : Springer, Cham
Print ISBN : 978-3-031-21487-5
Online ISBN : 978-3-031-21488-2
eBook Packages : Computer Science Computer Science (R0)
Share this paper
Anyone you share the following link with will be able to read this content:
Sorry, a shareable link is not currently available for this article.
Provided by the Springer Nature SharedIt content-sharing initiative
- Publish with us
Policies and ethics
Societies and partnerships
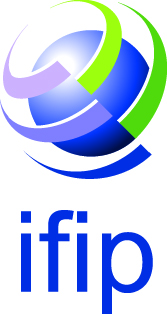
- Find a journal
- Track your research

The library building will be open from 9:00am-3:00pm on Friday, March 29th. Our services will be available online 7:45am-4:30pm for your convenience.
- University of Wisconsin-Superior
- Jim Dan Hill Library
- Help Guides
- TRIO McNair Undergraduate Research Guide
- Limitations of the Study
TRIO McNair Undergraduate Research Guide: Limitations of the Study
- Purpose of Guide
- Design Flaws to Avoid
- Glossary of Research Terms
- Ethics of Research
- Narrowing a Topic Idea
- Broadening a Topic Idea
- Expanding the Timeliness of a Topic Idea
- Writing a Research Proposal
- Academic Writing Style
- Choosing a Title
- Making an Outline
- Paragraph Development
- The Abstract
- Background Information
- The Research Problem/The Question
- Theoretical Framework
- Citation Mining
- Content Alert Services
- Evaluating Sources
- Primary Sources
- Secondary Sources
- Tertiary Sources
- Scholarly v. Popular Sources
- Qualitative Methods
- Quantitative Methods
- Using Non-Textual Elements
- Avoiding Plagiarism
- Footnotes or Endnotes?
- Further Readings
- Annotated Bibliography
- Preparing Your Poster
- Dealing with Nervousness
- Using Visual Aids
- Peer Review Process
- Informed Consent
- Writing Field Notes
The limitations of the study are those characteristics of design or methodology that impacted or influenced the application or interpretation of the results of your study. They are the constraints on generalizability and utility of findings that are the result of the ways in which you chose to design the study and/or the method used to establish internal and external validity.
Importance of...
Always acknowledge a study's limitations. It is far better for you to identify and acknowledge your study’s limitations than to have them pointed out by your professor and be graded down because you appear to have ignored them.
Keep in mind that acknowledgement of a study's limitations is an opportunity to make suggestions for further research . If you do connect your study's limitations to suggestions for further research, be sure to explain the ways in which these unanswered questions may become more focused because of your study.
Acknowledgement of a study's limitations also provides you with an opportunity to demonstrate to your professor that you have thought critically about the research problem, understood the relevant literature published about it, and correctly assessed the methods chosen for studying the problem. A key objective of the research process is not only discovering new knowledge but also to confront assumptions and explore what we don't know.
Claiming limitations is a subjective process because you must evaluate the impact of those limitations. Don't just list key weaknesses and the magnitude of a study's limitations. To do so diminishes the validity of your research because it leaves the reader wondering whether, or in what ways, limitation(s) in your study may have impacted the findings and conclusions. Limitations require a critical, overall appraisal and interpretation of their impact. You should answer the question: do these problems with errors, methods, validity, etc. eventually matter and, if so, to what extent?
Structure: How to Structure the Research Limitations Section of Your Dissertation . Dissertations and Theses: An Online Textbook. Laerd.com.
Descriptions of Possible Limitations
All studies have limitations. However, it is important that you restrict your discussion to limitations related to the research problem under investigation. For example, if a meta-analysis of existing literature is not a stated purpose of your research, it should not be discussed as a limitation. Do not apologize for not addressing issues that you did not promise to investigate in your paper.
Here are examples of limitations you may need to describe and to discuss how they possibly impacted your findings. Descriptions of limitations should be stated in the past tense.
Possible Methodological Limitations
Sample size -- the number of the units of analysis you use in your study is dictated by the type of research problem you are investigating. Note that, if your sample size is too small, it will be difficult to find significant relationships from the data, as statistical tests normally require a larger sample size to ensure a representative distribution of the population and to be considered representative of groups of people to whom results will be generalized or transferred.
Lack of available and/or reliable data -- a lack of data or of reliable data will likely require you to limit the scope of your analysis, the size of your sample, or it can be a significant obstacle in finding a trend and a meaningful relationship. You need to not only describe these limitations but to offer reasons why you believe data is missing or is unreliable. However, don’t just throw up your hands in frustration; use this as an opportunity to describe the need for future research.
Lack of prior research studies on the topic -- citing prior research studies forms the basis of your literature review and helps lay a foundation for understanding the research problem you are investigating. Depending on the currency or scope of your research topic, there may be little, if any, prior research on your topic. Before assuming this to be true, consult with a librarian! In cases when a librarian has confirmed that there is a lack of prior research, you may be required to develop an entirely new research typology [for example, using an exploratory rather than an explanatory research design]. Note that this limitation can serve as an important opportunity to describe the need for further research.
Measure used to collect the data -- sometimes it is the case that, after completing your interpretation of the findings, you discover that the way in which you gathered data inhibited your ability to conduct a thorough analysis of the results. For example, you regret not including a specific question in a survey that, in retrospect, could have helped address a particular issue that emerged later in the study. Acknowledge the deficiency by stating a need in future research to revise the specific method for gathering data.
Self-reported data -- whether you are relying on pre-existing self-reported data or you are conducting a qualitative research study and gathering the data yourself, self-reported data is limited by the fact that it rarely can be independently verified. In other words, you must take what people say, whether in interviews, focus groups, or on questionnaires, at face value. However, self-reported data contain several potential sources of bias that should be noted as limitations: (1) selective memory (remembering or not remembering experiences or events that occurred at some point in the past); (2) telescoping [recalling events that occurred at one time as if they occurred at another time]; (3) attribution [the act of attributing positive events and outcomes to one's own agency but attributing negative events and outcomes to external forces]; and, (4) exaggeration [the act of representing outcomes or embellishing events as more significant than is actually suggested from other data].
Possible Limitations of the Researcher
Access -- if your study depends on having access to people, organizations, or documents and, for whatever reason, access is denied or otherwise limited, the reasons for this need to be described.
Longitudinal effects -- unlike your professor, who can devote years [even a lifetime] to studying a single research problem, the time available to investigate a research problem and to measure change or stability within a sample is constrained by the due date of your assignment. Be sure to choose a topic that does not require an excessive amount of time to complete the literature review, apply the methodology, and gather and interpret the results. If you're unsure, talk to your professor.
Cultural and other types of bias -- we all have biases, whether we are conscience of them or not. Bias is when a person, place, or thing is viewed or shown in a consistently inaccurate way. It is usually negative, though one can have a positive bias as well. When proof-reading your paper, be especially critical in reviewing how you have stated a problem, selected the data to be studied, what may have been omitted, the way you have ordered events, people, or places and how you have chosen to represent a person, place, or thing, to name a phenomenon, or to use possible words with a positive or negative connotation. Note that if you detect bias in prior research, it must be acknowledged, and you should explain what measures were taken to avoid perpetuating bias.
Fluency in a language -- if your research focuses on measuring the perceived value of after-school tutoring among Mexican American ESL [English as a Second Language] students, for example, and you are not fluent in Spanish, you are limited in being able to read and interpret Spanish language research studies on the topic. This deficiency should be acknowledged.
Brutus, Stéphane et al. Self-Reported Limitations and Future Directions in Scholarly Reports: Analysis and Recommendations. Journal of Management 39 (January 2013): 48-75; Senunyeme, Emmanuel K. Business Research Methods . Powerpoint Presentation. Regent University of Science and Technology.
Structure and Writing Style
Information about the limitations of your study is generally placed either at the beginning of the discussion section of your paper so the reader knows and understands the limitations before reading the rest of your analysis of the findings, or the limitations are outlined at the conclusion of the discussion section as an acknowledgement of the need for further study. Statements about a study's limitations should not be buried in the body [middle] of the discussion section unless a limitation is specific to something covered in that part of the paper. If this is the case, though, the limitation should be reiterated at the conclusion of the section.
If you determine that your study is seriously flawed due to important limitations, such as an inability to acquire critical data, consider reframing it as a pilot study intended to lay the groundwork for a more complete research study in the future. Be sure, though, to specifically explain the ways that these flaws can be successfully overcome in later studies.
But do not use this as an excuse for not developing a thorough research paper! Review the tab in this guide for developing a research topic. If serious limitations exist, it generally indicates a likelihood that your research problem is too narrowly defined or that the issue or event under study is too recent and, thus, very little research has been written about it. If serious limitations do emerge, consult with your professor about possible ways to overcome them or how to reframe your study.
When discussing the limitations of your research, be sure to:
Describe each limitation in detailed but concise terms;
Explain why each limitation exists;
Provide the reasons why each limitation could not be overcome using the method(s) chosen to gather the data [cite to other studies that had similar problems when possible];
Assess the impact of each limitation in relation to the overall findings and conclusions of your study; and,
If appropriate, describe how these limitations could point to the need for further research.
Remember that the method you chose may be the source of a significant limitation that has emerged during your interpretation of the results [for example, you didn't ask a particular question in a survey that you later wish you had]. If this is the case, don't panic. Acknowledge it and explain how applying a different or more robust methodology might address the research problem more effectively in any future study. An underlying goal of scholarly research is not only to prove what works, but to demonstrate what doesn't work or what needs further clarification.
Brutus, Stéphane et al. Self-Reported Limitations and Future Directions in Scholarly Reports: Analysis and Recommendations. Journal of Management 39 (January 2013): 48-75; Ioannidis, John P.A. Limitations are not Properly Acknowledged in the Scientific Literature. Journal of Clinical Epidemiology 60 (2007): 324-329; Pasek, Josh. Writing the Empirical Social Science Research Paper: A Guide for the Perplexed . January 24, 2012. Academia.edu; Structure: How to Structure the Research Limitations Section of Your Dissertation . Dissertations and Theses: An Online Textbook. Laerd.com; What Is an Academic Paper? Institute for Writing Rhetoric. Dartmouth College; Writing the Experimental Report: Methods, Results, and Discussion. The Writing Lab and The OWL. Purdue University.
Writing Tip
Don't Inflate the Importance of Your Findings! After all the hard work and long hours devoted to writing your research paper, it is easy to get carried away with attributing unwarranted importance to what you’ve done. We all want our academic work to be viewed as excellent and worthy of a good grade, but it is important that you understand and openly acknowledge the limitations of your study. Inflating the importance of your study's findings in an attempt to hide its flaws is a big turn off to your readers. A measure of humility goes a long way!
Another Writing Tip
Negative Results are Not a Limitation!
Negative evidence refers to findings that unexpectedly challenge rather than support your hypothesis. If you didn't get the results you anticipated, it may mean your hypothesis was incorrect and needs to be reformulated, or perhaps you have stumbled onto something unexpected that warrants further study. Moreover, the absence of an effect may be very telling in many situations, particularly in experimental research designs. In any case, your results may be of importance to others even though they did not support your hypothesis. Do not fall into the trap of thinking that results contrary to what you expected is a limitation to your study. If you carried out the research well, they are simply your results and only require additional interpretation.
Yet Another Writing Tip
A Note about Sample Size Limitations in Qualitative Research
Sample sizes are typically smaller in qualitative research because, as the study goes on, acquiring more data does not necessarily lead to more information. This is because one occurrence of a piece of data, or a code, is all that is necessary to ensure that it becomes part of the analysis framework. However, it remains true that sample sizes that are too small cannot adequately support claims of having achieved valid conclusions and sample sizes that are too large do not permit the deep, naturalistic, and inductive analysis that defines qualitative inquiry. Determining adequate sample size in qualitative research is ultimately a matter of judgment and experience in evaluating the quality of the information collected against the uses to which it will be applied, and the particular research method and purposeful sampling strategy employed. If the sample size is found to be a limitation, it may reflect your judgement about the methodological technique chosen [e.g., single life history study versus focus group interviews] rather than the number of respondents used.
Huberman, A. Michael and Matthew B. Miles. Data Management and Analysis Methods. In Handbook of Qualitative Research. Norman K. Denzin and Yvonna S. Lincoln, eds. (Thousand Oaks, CA: Sage, 1994), pp. 428-444.
- << Previous: The Discussion
- Next: The Conclusion >>
Educational resources and simple solutions for your research journey
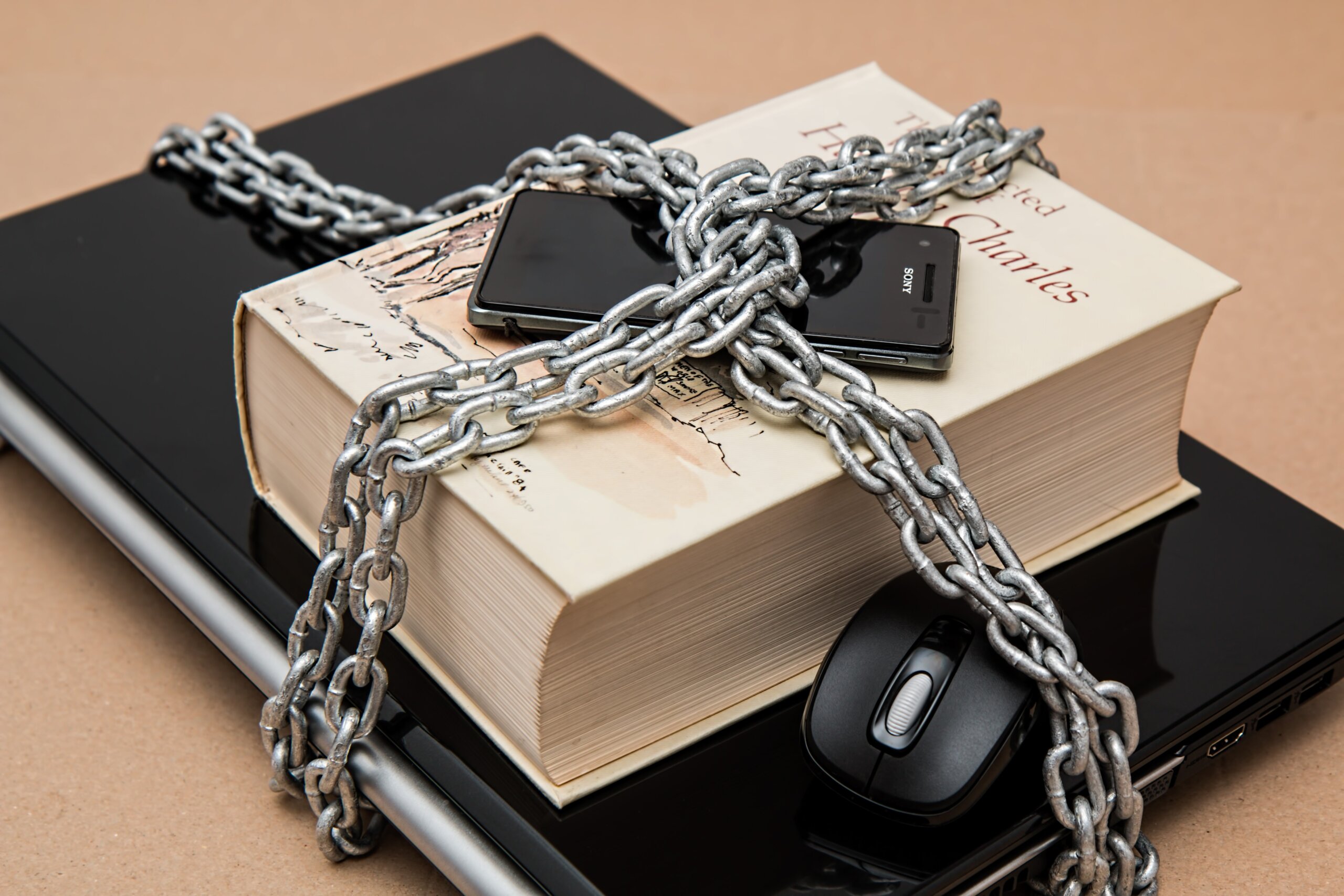
How to Present the Limitations of a Study in Research?
The limitations of the study convey to the reader how and under which conditions your study results will be evaluated. Scientific research involves investigating research topics, both known and unknown, which inherently includes an element of risk. The risk could arise due to human errors, barriers to data gathering, limited availability of resources, and researcher bias. Researchers are encouraged to discuss the limitations of their research to enhance the process of research, as well as to allow readers to gain an understanding of the study’s framework and value.
Limitations of the research are the constraints placed on the ability to generalize from the results and to further describe applications to practice. It is related to the utility value of the findings based on how you initially chose to design the study, the method used to establish internal and external validity, or the result of unanticipated challenges that emerged during the study. Knowing about these limitations and their impact can explain how the limitations of your study can affect the conclusions and thoughts drawn from your research. 1
Table of Contents
What are the limitations of a study
Researchers are probably cautious to acknowledge what the limitations of the research can be for fear of undermining the validity of the research findings. No research can be faultless or cover all possible conditions. These limitations of your research appear probably due to constraints on methodology or research design and influence the interpretation of your research’s ultimate findings. 2 These are limitations on the generalization and usability of findings that emerge from the design of the research and/or the method employed to ensure validity internally and externally. But such limitations of the study can impact the whole study or research paper. However, most researchers prefer not to discuss the different types of limitations in research for fear of decreasing the value of their paper amongst the reviewers or readers.
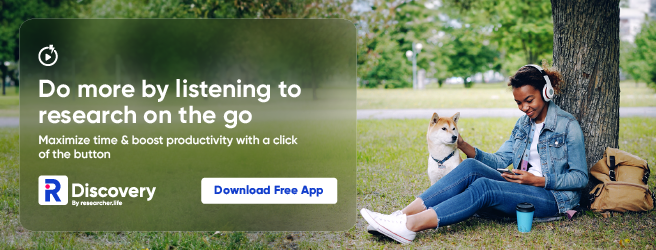
Importance of limitations of a study
Writing the limitations of the research papers is often assumed to require lots of effort. However, identifying the limitations of the study can help structure the research better. Therefore, do not underestimate the importance of research study limitations. 3
- Opportunity to make suggestions for further research. Suggestions for future research and avenues for further exploration can be developed based on the limitations of the study.
- Opportunity to demonstrate critical thinking. A key objective of the research process is to discover new knowledge while questioning existing assumptions and exploring what is new in the particular field. Describing the limitation of the research shows that you have critically thought about the research problem, reviewed relevant literature, and correctly assessed the methods chosen for studying the problem.
- Demonstrate Subjective learning process. Writing limitations of the research helps to critically evaluate the impact of the said limitations, assess the strength of the research, and consider alternative explanations or interpretations. Subjective evaluation contributes to a more complex and comprehensive knowledge of the issue under study.
Why should I include limitations of research in my paper
All studies have limitations to some extent. Including limitations of the study in your paper demonstrates the researchers’ comprehensive and holistic understanding of the research process and topic. The major advantages are the following:
- Understand the study conditions and challenges encountered . It establishes a complete and potentially logical depiction of the research. The boundaries of the study can be established, and realistic expectations for the findings can be set. They can also help to clarify what the study is not intended to address.
- Improve the quality and validity of the research findings. Mentioning limitations of the research creates opportunities for the original author and other researchers to undertake future studies to improve the research outcomes.
- Transparency and accountability. Including limitations of the research helps maintain mutual integrity and promote further progress in similar studies.
- Identify potential bias sources. Identifying the limitations of the study can help researchers identify potential sources of bias in their research design, data collection, or analysis. This can help to improve the validity and reliability of the findings.
Where do I need to add the limitations of the study in my paper
The limitations of your research can be stated at the beginning of the discussion section, which allows the reader to comprehend the limitations of the study prior to reading the rest of your findings or at the end of the discussion section as an acknowledgment of the need for further research.
Types of limitations in research
There are different types of limitations in research that researchers may encounter. These are listed below:
- Research Design Limitations : Restrictions on your research or available procedures may affect the research outputs. If the research goals and objectives are too broad, explain how they should be narrowed down to enhance the focus of your study. If there was a selection bias in your sample, explain how this may affect the generalizability of your findings. This can help readers understand the limitations of the study in terms of their impact on the overall validity of your research.
- Impact Limitations : Your study might be limited by a strong regional-, national-, or species-based impact or population- or experimental-specific impact. These inherent limitations on impact affect the extendibility and generalizability of the findings.
- Data or statistical limitations : Data or statistical limitations in research are extremely common in experimental (such as medicine, physics, and chemistry) or field-based (such as ecology and qualitative clinical research) studies. Sometimes, it is either extremely difficult to acquire sufficient data or gain access to the data. These limitations of the research might also be the result of your study’s design and might result in an incomplete conclusion to your research.
Limitations of study examples
All possible limitations of the study cannot be included in the discussion section of the research paper or dissertation. It will vary greatly depending on the type and nature of the study. These include types of research limitations that are related to methodology and the research process and that of the researcher as well that you need to describe and discuss how they possibly impacted your results.
Common methodological limitations of the study
Limitations of research due to methodological problems are addressed by identifying the potential problem and suggesting ways in which this should have been addressed. Some potential methodological limitations of the study are as follows. 1
- Sample size: The sample size 4 is dictated by the type of research problem investigated. If the sample size is too small, finding a significant relationship from the data will be difficult, as statistical tests require a large sample size to ensure a representative population distribution and generalize the study findings.
- Lack of available/reliable data: A lack of available/reliable data will limit the scope of your analysis and the size of your sample or present obstacles in finding a trend or meaningful relationship. So, when writing about the limitations of the study, give convincing reasons why you feel data is absent or untrustworthy and highlight the necessity for a future study focused on developing a new data-gathering strategy.
- Lack of prior research studies: Citing prior research studies is required to help understand the research problem being investigated. If there is little or no prior research, an exploratory rather than an explanatory research design will be required. Also, discovering the limitations of the study presents an opportunity to identify gaps in the literature and describe the need for additional study.
- Measure used to collect the data: Sometimes, the data gathered will be insufficient to conduct a thorough analysis of the results. A limitation of the study example, for instance, is identifying in retrospect that a specific question could have helped address a particular issue that emerged during data analysis. You can acknowledge the limitation of the research by stating the need to revise the specific method for gathering data in the future.
- Self-reported data: Self-reported data cannot be independently verified and can contain several potential bias sources, such as selective memory, attribution, and exaggeration. These biases become apparent if they are incongruent with data from other sources.
General limitations of researchers
Limitations related to the researcher can also influence the study outcomes. These should be addressed, and related remedies should be proposed.
- Limited access to data : If your study requires access to people, organizations, data, or documents whose access is denied or limited, the reasons need to be described. An additional explanation stating why this limitation of research did not prevent you from following through on your study is also needed.
- Time constraints : Researchers might also face challenges in meeting research deadlines due to a lack of timely participant availability or funds, among others. The impacts of time constraints must be acknowledged by mentioning the need for a future study addressing this research problem.
- Conflicts due to biased views and personal issues : Differences in culture or personal views can contribute to researcher bias, as they focus only on the results and data that support their main arguments. To avoid this, pay attention to the problem statement and data gathering.
Steps for structuring the limitations section
Limitations are an inherent part of any research study. Issues may vary, ranging from sampling and literature review to methodology and bias. However, there is a structure for identifying these elements, discussing them, and offering insight or alternatives on how the limitations of the study can be mitigated. This enhances the process of the research and helps readers gain a comprehensive understanding of a study’s conditions.
- Identify the research constraints : Identify those limitations having the greatest impact on the quality of the research findings and your ability to effectively answer your research questions and/or hypotheses. These include sample size, selection bias, measurement error, or other issues affecting the validity and reliability of your research.
- Describe their impact on your research : Reflect on the nature of the identified limitations and justify the choices made during the research to identify the impact of the study’s limitations on the research outcomes. Explanations can be offered if needed, but without being defensive or exaggerating them. Provide context for the limitations of your research to understand them in a broader context. Any specific limitations due to real-world considerations need to be pointed out critically rather than justifying them as done by some other author group or groups.
- Mention the opportunity for future investigations : Suggest ways to overcome the limitations of the present study through future research. This can help readers understand how the research fits into the broader context and offer a roadmap for future studies.
Frequently Asked Questions
- Should I mention all the limitations of my study in the research report?
Restrict limitations to what is pertinent to the research question under investigation. The specific limitations you include will depend on the nature of the study, the research question investigated, and the data collected.
- Can the limitations of a study affect its credibility?
Stating the limitations of the research is considered favorable by editors and peer reviewers. Connecting your study’s limitations with future possible research can help increase the focus of unanswered questions in this area. In addition, admitting limitations openly and validating that they do not affect the main findings of the study increases the credibility of your study. However, if you determine that your study is seriously flawed, explain ways to successfully overcome such flaws in a future study. For example, if your study fails to acquire critical data, consider reframing the research question as an exploratory study to lay the groundwork for more complete research in the future.
- How can I mitigate the limitations of my study?
Strategies to minimize limitations of the research should focus on convincing reviewers and readers that the limitations do not affect the conclusions of the study by showing that the methods are appropriate and that the logic is sound. Here are some steps to follow to achieve this:
- Use data that are valid.
- Use methods that are appropriate and sound logic to draw inferences.
- Use adequate statistical methods for drawing inferences from the data that studies with similar limitations have been published before.
Admit limitations openly and, at the same time, show how they do not affect the main conclusions of the study.
- Can the limitations of a study impact its publication chances?
Limitations in your research can arise owing to restrictions in methodology or research design. Although this could impact your chances of publishing your research paper, it is critical to explain your study’s limitations to your intended audience. For example, it can explain how your study constraints may impact the results and views generated from your investigation. It also shows that you have researched the flaws of your study and have a thorough understanding of the subject.
- How can limitations in research be used for future studies?
The limitations of a study give you an opportunity to offer suggestions for further research. Your study’s limitations, including problems experienced during the study and the additional study perspectives developed, are a great opportunity to take on a new challenge and help advance knowledge in a particular field.
References:
- Brutus, S., Aguinis, H., & Wassmer, U. (2013). Self-reported limitations and future directions in scholarly reports: Analysis and recommendations. Journal of Management , 39 (1), 48-75.
- Ioannidis, J. P. (2007). Limitations are not properly acknowledged in the scientific literature. Journal of Clinical Epidemiology , 60 (4), 324-329.
- Price, J. H., & Murnan, J. (2004). Research limitations and the necessity of reporting them. American Journal of Health Education , 35 (2), 66.
- Boddy, C. R. (2016). Sample size for qualitative research. Qualitative Market Research: An International Journal , 19 (4), 426-432.
R Discovery is a literature search and research reading platform that accelerates your research discovery journey by keeping you updated on the latest, most relevant scholarly content. With 250M+ research articles sourced from trusted aggregators like CrossRef, Unpaywall, PubMed, PubMed Central, Open Alex and top publishing houses like Springer Nature, JAMA, IOP, Taylor & Francis, NEJM, BMJ, Karger, SAGE, Emerald Publishing and more, R Discovery puts a world of research at your fingertips.
Try R Discovery Prime FREE for 1 week or upgrade at just US$72 a year to access premium features that let you listen to research on the go, read in your language, collaborate with peers, auto sync with reference managers, and much more. Choose a simpler, smarter way to find and read research – Download the app and start your free 7-day trial today !
Related Posts

What are Experimental Groups in Research

What is IMRaD Format in Research?

8 Main Advantages and Disadvantages of Experimental Research
Commonly used in sciences such as sociology, psychology, physics, chemistry, biology and medicine, experimental research is a collection of research designs which make use of manipulation and controlled testing in order to understand casual processes. To determine the effect on a dependent variable, one or more variables need to be manipulated.
Experimental research is used where:
- time priority in a causal relationship.
- consistency in a causal relationship.
- magnitude of the correlation is great.
In the strictest sense, experimental research is called a true experiment. This is where a researcher manipulates one variable and controls or randomizers the rest of the variables. The study involves a control group where the subjects are randomly assigned between groups. A researcher only tests one effect at a time. The variables that need to be test and measured should be known beforehand as well.
Another way experimental research can be defined is as a quasi experiment. It’s where scientists are actively influencing something in order to observe the consequences.
The aim of experimental research is to predict phenomenons. In most cases, an experiment is constructed so that some kinds of causation can be explained. Experimental research is helpful for society as it helps improve everyday life.
When a researcher decides on a topic of interest, they try to define the research problem, which really helps as it makes the research area narrower thus they are able to study it more appropriately. Once the research problem is defined, a researcher formulates a research hypothesis which is then tested against the null hypothesis.
In experimental research, sampling groups play a huge part and should therefore be chosen correctly, especially of there is more than one condition involved in the experiment. One of the sample groups usually serves as the control group while the others are used for the experimental conditions. Determination of sampling groups is done through a variety of ways, and these include:
- probability sampling
- non-probability sampling
- simple random sampling
- convenience sampling
- stratified sampling
- systematic sampling
- cluster sampling
- sequential sampling
- disproportional sampling
- judgmental sampling
- snowball sampling
- quota sampling
Being able to reduce sampling errors is important when researchers want to get valid results from their experiments. As such, researchers often make adjustments to the sample size to lessen the chances of random errors.
All this said, what are the popular examples of experimental research?
Stanley Milgram Experiment – Conducted to determine whether people obey orders, even if its clearly dangerous. It was created to explain why many people were slaughtered by Nazis during World War II. The killings were done after certain orders were made. In fact, war criminals were deemed just following orders and therefore not responsible for their actions.
Law of Segregation – based on the Mendel Pea Plant Experiment and was performed in the 19th century. Gregory Mendel was an Austrian monk who was studying at the University of Vienna. He didn’t know anything about the process behind inherited behavior, but found rules about how characteristics are passed down through generations. Mendel was able to generate testable rather than observational data.
Ben Franklin Kite Experiment – it is believed that Benjamin Franklin discovered electricity by flying his kite into a storm cloud therefore receiving an electric shock. This isn’t necessarily true but the kite experiment was a major contribution to physics as it increased our knowledge on natural phenomena.
But just like any other type of research, there are certain sides who are in support of this method and others who are on the opposing side. Here’s why that’s the case:
List of Advantages of Experimental Research
1. Control over variables This kind of research looks into controlling independent variables so that extraneous and unwanted variables are removed.
2. Determination of cause and effect relationship is easy Because of its experimental design, this kind of research looks manipulates variables so that a cause and effect relationship can be easily determined.
3. Provides better results When performing experimental research, there are specific control set ups as well as strict conditions to adhere to. With these two in place, better results can be achieved. With this kind of research, the experiments can be repeated and the results checked again. Getting better results also gives a researcher a boost of confidence.
Other advantages of experimental research include getting insights into instruction methods, performing experiments and combining methods for rigidity, determining the best for the people and providing great transferability.
List of Disadvantages of Experimental Research
1. Can’t always do experiments Several issues such as ethical or practical reasons can hinder an experiment from ever getting started. For one, not every variable that can be manipulated should be.
2. Creates artificial situations Experimental research also means controlling irrelevant variables on certain occasions. As such, this creates a situation that is somewhat artificial.
3. Subject to human error Researchers are human too and they can commit mistakes. However, whether the error was made by machine or man, one thing remains certain: it will affect the results of a study.
Other issues cited as disadvantages include personal biases, unreliable samples, results that can only be applied in one situation and the difficulty in measuring the human experience.
Also cited as a disadvantage, is that the results of the research can’t be generalized into real-life situation. In addition, experimental research takes a lot of time and can be really expensive.
4. Participants can be influenced by environment Those who participate in trials may be influenced by the environment around them. As such, they might give answers not based on how they truly feel but on what they think the researcher wants to hear. Rather than thinking through what they feel and think about a subject, a participant may just go along with what they believe the researcher is trying to achieve.
5. Manipulation of variables isn’t seen as completely objective Experimental research mainly involves the manipulation of variables, a practice that isn’t seen as being objective. As mentioned earlier, researchers are actively trying to influence variable so that they can observe the consequences.
Thank you for visiting nature.com. You are using a browser version with limited support for CSS. To obtain the best experience, we recommend you use a more up to date browser (or turn off compatibility mode in Internet Explorer). In the meantime, to ensure continued support, we are displaying the site without styles and JavaScript.
- View all journals
- Explore content
- About the journal
- Publish with us
- Sign up for alerts
- Open access
- Published: 25 July 2024
Experimental demonstration of magnetic tunnel junction-based computational random-access memory
- Yang Lv 1 ,
- Brandon R. Zink 1 ,
- Robert P. Bloom 1 ,
- Hüsrev Cılasun 1 ,
- Pravin Khanal 2 ,
- Salonik Resch 1 ,
- Zamshed Chowdhury 1 ,
- Ali Habiboglu 2 ,
- Weigang Wang 2 ,
- Sachin S. Sapatnekar 1 ,
- Ulya Karpuzcu 1 &
- Jian-Ping Wang 1
npj Unconventional Computing volume 1 , Article number: 3 ( 2024 ) Cite this article
5324 Accesses
247 Altmetric
Metrics details
- Computational science
- Electrical and electronic engineering
- Electronic and spintronic devices
- Magnetic devices
The conventional computing paradigm struggles to fulfill the rapidly growing demands from emerging applications, especially those for machine intelligence because much of the power and energy is consumed by constant data transfers between logic and memory modules. A new paradigm, called “computational random-access memory (CRAM),” has emerged to address this fundamental limitation. CRAM performs logic operations directly using the memory cells themselves, without having the data ever leave the memory. The energy and performance benefits of CRAM for both conventional and emerging applications have been well established by prior numerical studies. However, there is a lack of experimental demonstration and study of CRAM to evaluate its computational accuracy, which is a realistic and application-critical metric for its technological feasibility and competitiveness. In this work, a CRAM array based on magnetic tunnel junctions (MTJs) is experimentally demonstrated. First, basic memory operations, as well as 2-, 3-, and 5-input logic operations, are studied. Then, a 1-bit full adder with two different designs is demonstrated. Based on the experimental results, a suite of models has been developed to characterize the accuracy of CRAM computation. Scalar addition, multiplication, and matrix multiplication, which are essential building blocks for many conventional and machine intelligence applications, are evaluated and show promising accuracy performance. With the confirmation of MTJ-based CRAM’s accuracy, there is a strong case that this technology will have a significant impact on power- and energy-demanding applications of machine intelligence.
Similar content being viewed by others
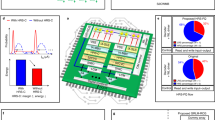
A CMOS-integrated compute-in-memory macro based on resistive random-access memory for AI edge devices
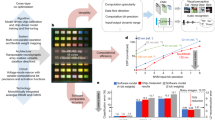
A compute-in-memory chip based on resistive random-access memory
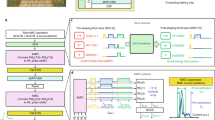
A four-megabit compute-in-memory macro with eight-bit precision based on CMOS and resistive random-access memory for AI edge devices
Introduction.
Recent advances in machine intelligence 1 , 2 for tasks such as recommender systems 3 , speech recognition 4 , natural language processing 5 , and computer vision 6 , have been placing growing demands on our computing systems, especially for implementations with artificial neural networks. A variety of platforms are used, from general-purpose CPUs and GPUs 7 , 8 , to FPGAs 9 , to custom-designed accelerators and processors 10 , 11 , 12 , 13 , to mixed- or fully- analog circuits 14 , 15 , 16 , 17 , 18 , 19 , 20 . Most are based on the Von Neumann architecture, with separate logic and memory systems. As shown in Fig. 1a , the inherent segregation of logic and memory requires large amounts of data to be transferred between these modules. In data-intensive scenarios, this transfer becomes a major bottleneck in terms of performance, energy consumption, and cost 21 , 22 , 23 . For example, the data movement consumes about 200 times the energy used for computation when reading three 64-bit source operands from and writing one 64-bit destination operand to an off-chip main memory 21 . This bottleneck has long been studied. Research aiming at connecting logic and memory more closely has led to new computation paradigms.
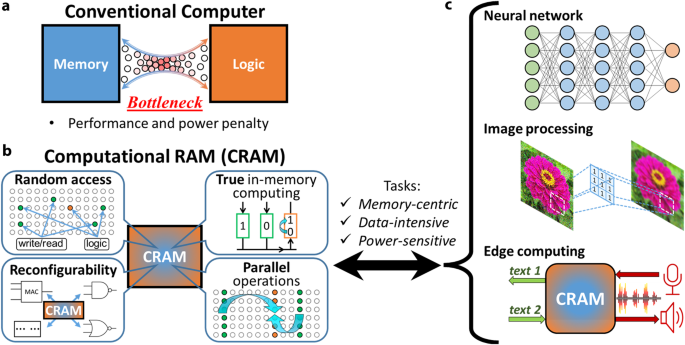
a , b Compared to a conventional computer architecture ( a ), which suffers from the memory-logic transfer bottleneck, CRAM ( b ) offers significant power and performance improvements. Its unique architecture allows for computation in memory, as well as, random access, reconfigurability, and parallel operation capability. c The CRAM could excel in data-intensive, memory-centric, or power-sensitive applications, such as neural networks, image processing, or edge computing ( c ).
Promising paradigms include “near-memory” and “in-memory” computing. Near-memory processing brings logic physically closer to memory by employing 3D-stacked architectures 24 , 25 , 26 , 27 , 28 , 29 . In-memory computing scatters clusters of logic throughout or around the memory banks on a single chip 14 , 15 , 16 , 17 , 18 , 19 , 20 , 30 , 31 , 32 , 33 , 34 , 35 . Yet another approach is to build systems where the memory itself can perform computation. This has been dubbed “true” in-memory computing 36 , 37 , 38 , 39 , 40 , 41 , 42 . The computational random-access memory (CRAM) 38 , 40 is one of the true in-memory computing paradigms. Logic is performed natively by the memory cells; the data for logic operations never has to leave the memory (Fig. 1b ). Additionally, CRAM operates in a fully digital fashion, unlike most other reported in-memory computing schemes 14 , 15 , 16 , 17 , 18 , 19 , 20 , which are partially or mostly analog. CRAM promises superior energy efficiency and processing performance for machine intelligence applications. It has unique additional features, such as random-access of data and operands, massive parallel computing capabilities, and reconfigurability of operations 38 , 40 . Also note that although the transistor-less (crossbar) architecture employed by most of the previous true-in-memory computing paradigms 36 , 37 , 39 , 42 allows for higher density, the maximum allowable size of the memory array is often severely limited due to the sneak path issues. CRAM includes transistors in each of its cells for better-controlled electrical accessibility and, therefore, a larger array size.
The CRAM was initially proposed based on the MTJ device 38 , an emerging memory device that relies on spin electronics 43 . Such “spintronic” devices, along with other non-volatile emerging memory devices, usually referred to as “X” for logic applications, have been intensively investigated over the past several decades for emerging memory and computing applications as “beyond-CMOS” and/or “CMOS + X” technologies. They offer vastly improved speed, energy efficiency, area, and cost. An additional feature that is exploited by CRAM is their non-volatility 44 . The MTJ device is the most mature of spintronic devices for embedded memory applications, based on endurance 45 , energy efficiency 46 , and speed 47 . We note that CRAM can be implemented based not only on spintronics devices but also on other non-volatile emerging memory devices.
In its simplest form, an MTJ consists of a thin tunneling barrier layer sandwiched between two ferromagnetic (FM) layers. When a voltage is applied between the two layers, electrons tunnel through the barrier, resulting in a charge current. The resistance of the MTJ is a function of the magnetic state of the two FM layers, due to the tunneling magnetoresistance (TMR) effect 48 , 49 , 50 . An MTJ can be engineered to be magnetically bi-stable. Accordingly, it can store information based on its magnetic state. This information can be retrieved by reading the resistance of the device. The MTJ can be electrically switched from one state to the other with a current due to the spin-transfer torque (STT) effect 51 , 52 . In this way, an MTJ can be used as an electrically operated memory device with both read and write functionality. A type of random-access memory, the STT-MRAM 53 , 54 , 55 , 56 has been developed commercially, utilizing MTJs as memory cells. A typical STT-MRAM consists of an array of bit cells, each containing one transistor and one MTJ. These are referred to as 1 transistor 1 MTJ (1T1M) cells.
A typical CRAM cell design, as shown in Fig. 2a , is a modification of the 1T1M STT-MRAM architecture 57 . The MTJ, one of the transistors, word line (WL), bit select line (BSL), and memory bit line (MBL) resemble the 1T1M cell architecture of STT-MRAM, which allows the CRAM to perform memory operations. In order to enable logic operations, a second transistor, as well as a logic line (LL) and a logic bit line (LBL), are added to each memory cell. During a logic operation, corresponding transistors and lines are manipulated so that several MTJs in a row are temporarily connected to a shared LL 40 . While the LL is left floating, voltage pulses are applied to the lines connecting to input MTJs, with that of the output MTJ being grounded. The logic operation is based on a working principle called voltage-controlled logic (VCL) 58 , 59 , which utilizes the thresholding effect that occurs when switching an MTJ and the TMR effect of MTJ. As shown in Fig. 2b , when a voltage is applied across the input MTJs, the different resistance values result in different current levels. The current flows through the output MTJ, which may or may not switch its state, depending on the states of the input MTJs. In this way, basic bitwise logic operations, such as AND, OR, NAND, NOR, and MAJ, can be realized. A unique feature of VCL is that the logic operation itself does not require the data in the input MTJs to be read-out through sense amplifiers at the edge of the array. Rather, it is used locally within the set of MTJs involved in the computation. This is fundamentally why the CRAM computation represents true-in-memory computing: the computation does not require data to travel out of the memory array. It is always processed locally by nearby cells. We note that this concept would also work with other two-terminal stateful passive memory devices, such as memristors. Accordingly, a CRAM could be implemented with such devices. A CRAM could also be implemented with three-terminal stateful devices, such as spin-orbit torque (SOT) devices. This could result in greater energy efficiency and reliability 60 . Although devices with progressive or accumulative switching behavior, such as spintronic domain wall devices 61 , 62 , can be adopted as well, CRAM would otherwise work best if adopting bi-stable memory devices with strong threshold switching behavior. As an oversimplified speculation, the performance comparison between CRAMs implemented by various emerging memory devices is expected to roughly follow the comparison between these for memory applications, since CRAM utilizes memory devices in similar manners as in-memory applications. For example, a CRAM implemented based on MTJs should be expected to offer high endurance and high speed. Also, generally, a CRAM logic operation should consume energy comparable to the energy consumption of a memory write operation, for the same emerging memory device operating at the same speed. However, a careful case-by-case analysis is necessary for CRAMs implemented by each emerging memory device technology. Also, note that we do not show a specific circuit design of CRAM peripherals because CRAM does not require significant circuit design change in sense amplifiers or peripherals compared to 1T1M STT-MRAM. And these in the STT-MRAM are already common and mature. Lastly, the true in-memory computing characteristic of CRAM is limited to within a continuous CRAM array: any computation that requires access to data across separate CRAM arrays will require additional data access and movement. The size of an array is ultimately limited by parasitic effects of interconnects 63 . However, these limitations are true for all other in-memory computing paradigms. CRAM is not at any disadvantage in this scenario.
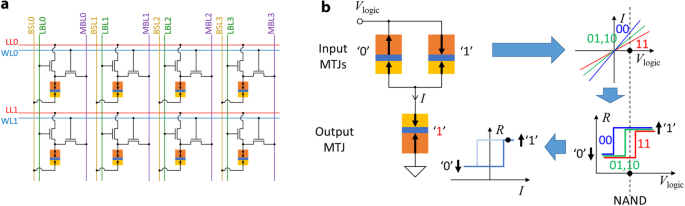
a CRAM adopts the so-called 2 transistor 1 MTJ (2T1M) cell architecture. On top of the 1T1M cell architecture of STT-MRAM, an additional transistor, as well as the added logic line (LL) and logic bit line (LBL), allow the CRAM to perform logic operations. During a CRAM logic operation, the transistors and lines are manipulated to form an equivalent circuit, as shown in b . Although CRAM can be built based on various emerging memory devices, we use MTJs and MTJ-based CRAM as an example for illustration purposes. b The working principle of CRAM logic operation, the VCL, utilizes the thresholding effect that occurs when switching an MTJ and the TMR effect of the MTJ. With an appropriate V logic amplitude, the voltage is translated into different currents flowing through the output MTJ by the TMR effect of the input MTJs. Whether the output MTJ switches or not is dependent on the state of the input MTJs.
On top of the potential performance benefits that the emerging memory devices bring, at circuit and architecture level, CRAM fundamentally provides several benefits (Fig. 1b ): (1) the elimination of the costly performance and energy penalties associated with transferring data between logic and memory; (2) random access of data for the inputs and outputs to operations; (3) the reconfigurability of operations, as any of the logic operations, AND, OR, NAND, NOR, and MAJ can be programmed; and (4) the performance gain of massive parallelism, as identical operations can be performed in parallel in each row of the CRAM array when data is allocated properly. Based on analysis and benchmarking, CRAM has the potential to deliver significant gains in performance and power efficiency, particularly for data-intensive, memory-centric, or power-sensitive applications, such as bioinformatics 40 , 64 , 65 , image 66 and signal 67 processing, neural networks 66 , 68 , and edge computing 69 (Fig. 1c ). For example, a CRAM-based machine-learning inference accelerator was estimated to achieve an improvement on the order of 1000× over a state-of-art solution, in terms of the energy-delay product 70 . Another example shows that CRAM (at the 10 nm technology node) consumes 0.47 µJ and 434 ns of energy and time, respectively, to perform an MNIST handwritten digit classifier task. It is 2500× and 1700× less in energy and time, respectively, compared to a near-memory processing system at the 16 nm technology node 66 . And yet, to date, there have been no experimental studies of CRAM.
In this work, we present the first experimental demonstration of a CRAM array. Although based on a small 1 × 7 array, it successfully shows complete CRAM array operations. We illustrate computation with a 1-bit full adder. This work provides a proof-of-concept as well as a platform with which to study key aspects of the technology experimentally. We provide detailed projections and guidelines for future CRAM design and development. Specifically, based on the experimental results, models and calculations of CRAM logic operations are developed and verified. The results connect the CRAM gate-level accuracy or error rate to MTJ TMR ratio, logic operation pulse width, and other parameters. Then we evaluate the accuracy of a multi-bit adder, a multiplier, and a matrix multiplication unit, which are essential building blocks for many conventional and machine intelligence applications, including artificial neural networks.
Experiments
Figure 3 shows the experimental setup, consisting of both hardware and software. The hardware is built with a so-called ‘circuit-around-die’ approach 71 : semiconductor circuitry is built with commercially available components around the MTJ dies. This approach offers a more rapid development cycle and flexibility needed for exploratory experimental studies on CRAM arrays and potential new MTJ technologies, while the major foundries lack the specific process design kit available for making a CRAM array fully integrated with CMOS. The hardware is a 1 × 7 CRAM array, with the design of cells taken from the 2T1M CRAM cells 38 , 40 , modified for simplified memory access. Software on a PC controls the operation. It communicates with the hardware with basic commands: ‘open/close transistors’; ‘apply voltage pulses’ to perform write and logic operations; and ‘read cell resistance’. The software collects real-time measurements of the data associated with CRAM operations for analysis and visualization. All aspects of the 1 × 7 CRAM array are functional: memory write, memory read, and logic operations (more details in Methods section, and Supplementary Note S 1 ).
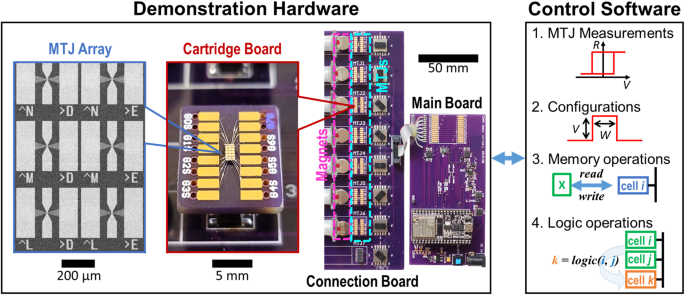
The setup consists of custom-built hardware and a suite of control software. It demonstrates a fully functioning 1 × 7 CRAM array. The hardware consists of a main board hosting all necessary electronics except for the MTJ devices; a connection board on which passive switches, connectors, and magnetic bias field mechanisms are hosted; and multiple cartridge boards that each have an MTJ array mounted and multiple MTJ devices that are wire bonded. The gray-scale scanning electron microscopy image shows the MTJ array used. The color optical photographs show the cartridge board and the entire hardware setup. The software is responsible for real-time measurements of the MTJs; configuration and execution of CRAM operations: memory write, memory read, and logic; and data collection. It is run on a PC, which communicates wirelessly with the main board.
MTJs with perpendicular interfacial anisotropy are used in the CRAM. They exhibit low resistance-area (RA) product and high TMR ratio—approximately 100%—when sized at 100 nm in diameter (more details in Supplementary Note S 2 ).
Device properties and CRAM memory operations
The experiments begin with measuring the resistance (R)–voltage (V) properties of each MTJ device and of each die. In order to compensate for device-to-device variations, the bias magnetic fields for each MTJ are adjusted so that the R–V properties are as close to each other as possible (more details in Supplementary Note S 2 ). As the processes of making CRAM arrays mature, bias magnetic fields are expected to be no longer needed and all CRAM cells will be able to be operated with uniform parameters and under uniform conditions. The resistance threshold for the MTJs logic states is also determined in this stage.
Then the seven MTJ cells are tested for memory operations with various write pulse amplitudes and widths. Based on the observed write error rates for memory write operations, appropriate pulse amplitudes and widths are configured, achieving reliable memory write operations with an average write error rate of less than 1.5 × 10 −4 (more details in Supplementary Note S 3 ). We designate logic ‘0’ and ‘1’ to the parallel (P) low resistance state and anti-parallel (AP) high resistance state of MTJ, respectively.
CRAM logic operations
Two-input logic operations are studied. The output cell is first initialized by writing ‘0’ to it. Then two input cells are connected to the output cell through the LL by turning on the corresponding transistors. Voltage pulses of amplitude of V logic , V logic , and 0, are simultaneously applied to the two input cells and the output cell, respectively. This is the same as grounding the output cell while applying a voltage pulse of V logic to the two input cells. Then, depending on the input cells’ states, the output cell will have a certain probability of being switched from ‘0’ to ‘1’. Such a cycle of operations is repeated n times, and the statistical mean of the output logic state, < D out >, is obtained. The entire process is repeated for different V logic values and input states. The basis for logic operations in the CRAM is the state-dependent resistance of the input cells. These shift and displace the output cell’s switching probability transfer curve. As a result, the output cell switches state based on specific input states, thereby implementing a logic function such as AND, OR, NAND, NOR, or MAJ. A specific initial state of the output cell and V logic value corresponds to one of these logic gates 66 . The time duration or pulse width of the voltage pulse applied during a logic operation is expected to contribute to most of the time required to complete a logic operation. In the following, we use the term logic speed to generally refer to the speed of a logic operation. Logic speed is approximately inversely proportional to the time duration of the voltage pulse used during a logic operation.
The experimental results are shown in Fig. 4 a, b . Generally, for a given input state, < D out > increases with increasing V logic . The < D out > response curves are input state-dependent. The four input states can be divided into three groups:
The ‘00’ input state yields the lowest resistance at the two input cells, so the output cell switches from ‘0’ to ‘1’ first (with the lowest V logic ).
The ‘11’ input state yields the highest resistance at the two input cells, so the output cell switches from ‘0’ to ‘1’ last (with the highest V logic ).
The ‘01’ and ‘10’ input states both yield resistance that falls in between that of ‘00’ and ‘11’so that the output cell’s response curve falls in between that of ‘00’ and ‘11’.
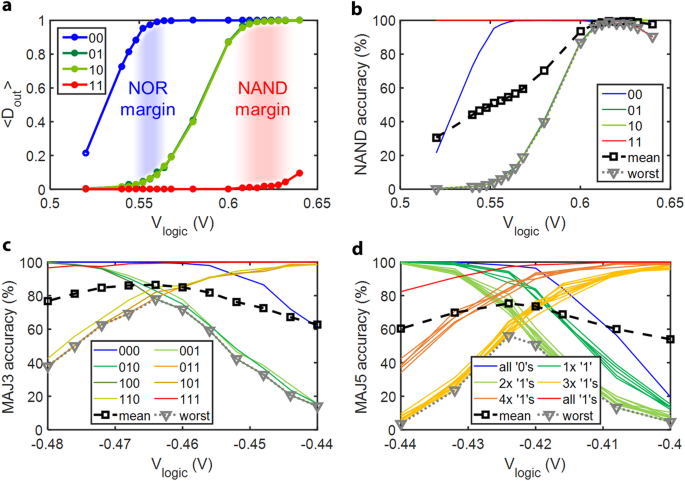
a Output logic average, D out , vs. logic voltage, V logic . In a 2-input logic operation, two input cells and one output MTJ cell are involved. The output cell’s terminal is grounded, while the common line is left floating. A logic operation voltage pulse is applied to the two input cells’ terminals for a fixed duration (pulse width) of 1 ms. Before each logic operation, input data is written to the input cells. After each logic operation, the output cell’s state is read. Each curve corresponds to a specific input state. Each data point represents the statistical average of the output cell’s logic state, < D out >, sampled by 1000 repeats ( n = 1000) of the operations. The separation between the < D out > curves indicates the margins for NOR or NAND operation, highlighted in blue and red, respectively. b Accuracy of 2-input NAND operation vs. logic voltage, V logic . The results in a can be converted into a more straightforward metric, accuracy, for the NAND truth table. The curve labeled ‘mean’ and ‘worst’ indicates the average and the worst-case accuracy across all input states, respectively. So, for NAND operation, the optimal logic voltage is indicated in such a plot where the mean or worst accuracy is maximized. c , d Accuracy of MAJ3 ( c ) and MAJ5 ( d ) logic operations vs. logic voltage, V logic . Each curve corresponds to an input state or a group of input states. And each data point represents the statistical average of the output MTJ logic state sampled by n = 1000 and n = 250, for c and d , respectively.
Figure 4a shows the experiment results. The two regions highlighted in blue and red that fall in between the three groups of response curves are suitable for NOR and NAND operations, respectively. For example, in the red region, the ‘11’ input has a high probability of yielding a ‘0’ output, while the other three input states have a high probability of yielding a ‘1’ output. This matches the expected truth table for a NAND logic gate. Therefore, if V logic is chosen carefully—within the red region for the CRAM 2-input logic operation—the operation performed is highly likely to be NAND.
The experimental results of < D out > can be converted into a straightforward format representing the accuracy for a specified logic function. This translation can be computed by simply subtracting < D out > from 1 for those input states where a ‘0’ output is expected in the truth table of the logic function. Figure 4b shows the NAND accuracy of the same 2-input CRAM logic operation. The ‘mean’ and ‘worst’ plots are based on the average value and minimum value of the accuracy, respectively, across all input state combinations at a fixed value for V logic . Based on the experimental results, if V logic = 0.624 or 0.616 V, the CRAM delivers a NAND operation with a best mean and a worst-case accuracy of about 99.4% and 99.0%, respectively. From a circuit perspective, both increasing the effective TMR ratio of input cells and/or making the output cell’s response curve steeper would increase the vertical separation of these input state-dependent curves, resulting in higher accuracy. For example, a higher effective TMR ratio of input cells results in a larger contrast of current in the output cell between different input states. Therefore, there is more ‘horizontal’ room to separate the < D out > curves associated with different input states so that for the inputs with which the output is expected to be ‘0’ or ‘1’, the < D out > of the output cell is closer to the expected value (‘0’ or ‘1’). Also note that for a logic operation, the ‘accuracy’ and ‘error rate’ are essentially two quantities describing the same thing: how true the logic operation is, statistically. By definition, the sum of accuracy and error rate is always 1. The higher or closer to 1 the accuracy is, the better. The lower or closer to 0 the error rate is, the better. Lastly, to facilitate better visualization of how the resistance changes of different input cell states are translated into voltage differences on the output cell resulting in it being switched or unswitched, we list the equivalent resistance of the two input cells combined in parallel and the resulting voltage on the output cell as follows: With V logic = 0.620 V, the equivalent resistance of input cells and the resulting voltage on the output cell are 0.4133 V and 1120 Ω, 0.3753 V, and 1461 Ω, and 0.3248 V and 2037 Ω, for input states ‘00’, ‘01’ or ‘10’, and ‘11’, respectively. Note that these values are estimated by the experiment-based modeling, which is introduced in the later part of this paper.
With more input cells, we also studied 3-input and 5-input majority logic operations. Figure 4c shows the accuracy of a 3-input MAJ3 logic operation. At V logic = −0.464 V, both the optimal mean and the worst-case accuracy are observed to be 86.5% and 78.0%, respectively. Similarly, for a 5-input MAJ5 logic operation, shown in Fig. 4d , both the optimal mean and the worst-case accuracy are observed to be 75% and 56%, respectively. As expected, comparing 2-input, 3-input, and 5-input logic operations, the accuracy decreases with an increasing number of inputs (more discussions and explanations in Supplementary Note S 4 ).
CRAM full adder
Having demonstrated fundamental elements of CRAM—memory write operations, memory read operations, and logic operations—we turn to more complex operations. We demonstrate a 1-bit full adder. This device takes two 1-bit operands, A and B, as well as a 1-bit carry-in, C, as inputs, and outputs a 1-bit sum, S, and a 1-bit carry-out, C out . A variety of implementations exist. We investigate two common designs: (1) one that uses a combination of majority and inversion logic gates, which we will refer to as a ‘MAJ + NOT’ design; and (2) one that uses only NAND gates, which we will refer to as an ‘all-NAND’ design. Figures 5 a and 5b illustrate these designs. Supplementary Note S 5 provides more details.
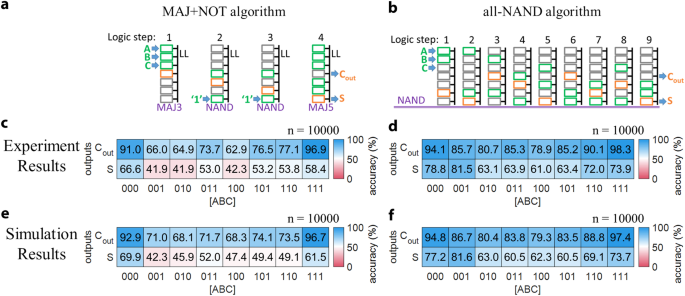
a , b Illustrations of the ‘MAJ + NOT’ and ‘all-NAND’ 1-bit full adder designs. Green and orange letter symbols indicate input and output data for the full adder, respectively. From left to right, numbered by ‘logic step,’ each drawing shows the intended input (green rectangle) and output (orange rectangle) cells involved in the logic operation. The text in purple under each drawing indicates the intended function of the logic operation (MAJ3, NAND, or MAJ5). c – f Experimental ( c , d ) and simulation ( e , f ) results of the output accuracy of 1-bit full adder operations by CRAM with the MAJ + NOT ( c , e ) and all-NAND ( d , f ) designs. The CRAM adder’s outputs, S and C out , are assessed against the expected values, i.e., their truth table, for all input states of A, B, and C. The accuracy of each result for each input state is shown by the numerical value in black font, as well as, represented by the color of the box with red (or blue) indicating wrong (or correct), or accuracy of 0% (100%). The accuracy is calculated based on the statistical average of outputs obtained by repeating the full adder execution n times, for n = 10,000. The experimental results for the MAJ + NOT ( c ) and all-NAND ( d ) designs are obtained by repeatedly executing the operation for all input states and observing the output states. The simulation results for the MAJ + NOT ( e ) and all-NAND ( f ) designs are obtained with probabilistic modeling, using Monte Carlo methods. The accuracy of individual logic operations is set to what was observed experimentally.
Figure 5c, f shows the experimental and simulation results for the MAJ + NOT and the all-NAND designs, respectively. Each plot is a colormap that lists the accuracy of the output bits S and C out , with each input state coded as [ABC]. The blue (red) indicates good/desired (bad/undesired) accuracy. In the boxes of colormap, results in saturated blue are the most desirable. The numerical values of accuracy are also labeled accordingly. From the experimental results for the MAJ + NOT design full adder shown in Fig. 5c , we make two observations:
The accuracy of C out is generally higher than that of S. This is because C out is directly produced by the first MAJ3 operation from inputs A, B, and C, while S is produced after additional logic operations. We also note that since C out is produced earlier than S, it is less impacted by error propagation and accumulation during each step; and the MAJ5 involved in producing S is inherently less accurate than the MAJ3.
Both C out and S have higher accuracy when the input [ABC] = 000 or 111 than in the other cases. This is expected since the input states of all ‘0’s and all ‘1’s yield higher accuracy than those with mixed numbers of ‘0’s and ‘1’s for both MAJ3 and MAJ5.
The experimental results for the all-NAND design are shown in Fig. 5d . The same observations regarding accuracy vs. inputs as the MAJ + NOT design apply. However, it is clear that the accuracy of the all-NAND full adder, at 78.5%, is higher than that of the MAJ + NOT full adder, at 63.8%. This is likely due to the fact that 2-input NAND operations are inherently more accurate than MAJ3 and MAJ5 operations. This offsets the impact of the additional steps required in the all-NAND design. We note that the accuracy of all computation blocks will improve as the underlying MTJ technology evolves. Accordingly, the relative accuracy of the all-NAND versus the MAJ + NOT designs may change 66 .
Modeling and analysis of CRAM logic accuracy
To understand the origin of errors, how they accumulate, and how they propagate, we performed numerical simulations of the full adder designs. These are based on probabilistic models of logic operations, implemented using Monte Carlo methods. Figure 5 e, f shows the simulation results for the MAJ + NOT and all-NAND designs, respectively. In these simulations, the accuracy of individual logic operations was set to match what was experimentally observed. The simulation results for the overall designs of the full adders correspond well to what was observed experimentally for these, confirming the validity of the proposed probabilistic models (more details in the Methods section and Supplementary Note S 6 ).
We note that beyond the inherent inaccuracy of logic operations, other factors such as device drift and device-to-device variation in MTJ devices will contribute to error in a CRAM. Specifically, drifts in temperature, external magnetic field, MTJ anisotropy, and MTJ resistance can lead to drift of the response curve, < D out >. Most likely, any such drift will result in a reduction (increase) of accuracy (error rate). More discussion regarding device-to-device variation is provided in Supplementary Note S 7 .
On the other hand, the accuracy of logic operations will significantly benefit from improvements in TMR ratio as MTJ technology evolves. To project the future accuracy of CRAM operations, we employ various types of physical modeling informed by existing experimental results (more details are provided in the Methods section and Supplementary Note S 8 ).
Three sets of assumptions on the accuracies (or error rates) of NAND logic operations underlie the following studies.
The ‘experimental’ assumptions are based on the best accuracy experimentally observed among the 9 NAND steps involved with the all-NAND 1-bit full adder. These are adjusted linearly to ensure that the error for inputs ‘01’ and ‘10’ equals that for input ‘11’. In reality, as supported by the experimental results shown in Fig. 4a , such a condition can be reached by properly tuning the V logic . Therefore, assuming the gate-level error rate is already optimized by tuning the V logic , then the per-input state NAND accuracies can be further simplified so that an error rate, δ (0 ≤ δ ≤ 1), can be used to characterize the error, accuracy, and probabilistic truth table of NAND operations in a CRAM. The NAND accuracy is [1, 1–δ, 1–δ, 1–δ], and the NAND probabilistic truth table is [1, 1– δ , 1– δ , δ ], both being a function of δ. Through the above-mentioned modeling and calculations, the ‘experimental’ assumptions yield δ = 0.0076, which corresponds to a TMR ratio of approximately 109%, based on experiments.
Two additional sets of assumptions, labeled as ‘production’ and ‘improved’, assume MTJ TMR ratios of 200% and 300%, respectively. These two assumptions yield δ = 2.1 × 10 −4 , and δ = 7.6 × 10 −6 , respectively, based on modeling and calculations. The ‘production’ assumptions represent the current industry-level TMR ratios developed for STT-MRAM technologies. The ‘improved’ assumptions present reasonable expectations for near-future MTJ developments.
CRAM NAND error rates vs. TMR ratio with various logic voltage pulse widths are shown in Fig. 6a . Higher TMR ratios and faster logic speed—so shorter V logic pulse widths—lead to smaller error rates. Further details can be found in Supplementary Note S 8 and in Supplementary Figure S 5 . Also included is an analysis of error rates vs. effective TMR ratio, which is independent of the specific TMR modeling. Note that, for all subsequent results, we will use the NAND error rate at the assumed TMR ratios, with pulse widths of 1 ms. This is more conservative but is consistent with the pulse widths used in the experimental results reported above.
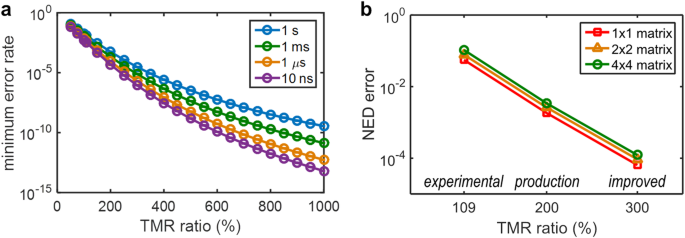
a NAND gate minimum error rate vs. MTJ TMR ratio with various V logic pulse widths. For a given TMR ratio, the error rate is a function of V logic . So, the ‘minimum error rate’ represents the minimum error rate achievable with an appropriate V logic value. All subsequent studies use the error rates observed with 1 ms pulse widths (to be consistent with the earlier experimental studies) at assumed TMR ratios. b The NED error of a 4-bit dot-product matrix multiplier vs. TMR ratio. TMR ratios of 109%, 200%, and 300% are adopted for the ‘experimental,’ ‘production,’ and ‘improved’ assumptions, respectively. The size of the input matrix is indicated in the legend of the plot.
Analysis of CRAM multi-bit adder, multiplier, and matrix multiplier
With these defined sets of assumptions, we provide projections of CRAM accuracy at a larger scale for meaningful applications. First, we evaluate ripple-carry adders and array multipliers 72 operating on scalar operands, with up to 6 bits. To evaluate the results, we adopt the normalized error distance (NED) metric 73 to represent the error of these primitives, as it has been shown to be more suitable for arithmetic primitives in the presence of computational error. We will refer to the error for a given primitive as ‘NED error’. We also define a complementary metric of ‘NED accuracy’ as the NED subtracted from 1 and then multiplied by 100%, to facilitate a more intuitive visualization of the error values. While the ‘experimental’ assumptions with a TMR ratio of 109% yield good overall accuracy for adders and multipliers, as the TMR ratio increases, the ‘production’ assumption with a TMR ratio of 200%, and the ‘improved’ assumption with a TMR ratio of 300%, yield significantly better or higher accuracies. Specifically, a 4-bit adder produces NED error of 2.8 × 10 −2 , 8.6 × 10 −4 , and 3.3 × 10 −5 , or NED accuracy of 97.2%, 99.914%, and 99.9967%, for the ‘experimental’, ‘production’, and ‘improved’ assumptions, respectively. A 4-bit multiplier produces NED error of 5.5 × 10 −2 , 1.8 × 10 −3 , and 6.6 × 10 −5 , or NED accuracy of 94.5%, 99.82%, and 99.9934%, for the three sets of assumptions, respectively. It is expected that when comparing the adder to the multiplier, since the latter is more complex and involves more gates, its accuracy is generally lower than that of the adder. Similarly, as the bit width of the adder or multiplier increases, their accuracy decreases. Further details and results with bit width up to 6-bit are provided in the Methods section and in Supplementary Note S 9 .
Then, using these primitives, we evaluate dot-product operations, which form the basis of matrix multiplication. They are heavily employed in many applications in both conventional domains and machine intelligence. Dot products consist of element-wise multiplication of two unsigned integer vectors, followed by addition. We perform additions with binary trees to maintain smaller circuit depth. Figure 6b shows the NED error of a 4-bit 4 × 4 dot-product matrix multiplier with respect to various TMR ratio assumptions. Like the adders and multipliers, as the TMR ratio increases, the NED error decreases, or the NED accuracy improves. Specifically, a 4-bit 4 × 4 dot-product matrix multiplier produces an NED error of 0.11, 3.4 × 10 −3 , and 1.2 × 10 −4 , or NED accuracy of 89%, 99.66%, and 99.988%, for the ‘experimental’, ‘production’, and ‘improved’ assumptions, respectively. Also, when comparing different input sizes (e.g., 1 × 1 to 4 × 4), as expected, the NED error is larger for larger input sizes due to the increased number of gates involved. Further details and results with bit width up to 5-bit are provided in the Methods section and in Supplementary Note S 9 .
Discussions
To summarize the experimental work, an MTJ-based 1 × 7 CRAM array hardware was experimentally demonstrated and systematically evaluated. The basic memory write and read operations of CRAM were achieved with high reliability. The study on CRAM logic operations began with 2-input logic operations. It was found that a 2-input NAND operation could be performed with accuracy as high as 99.4%. As the number of input cells was increased, for example, for 3-input MAJ3 and 5-input MAJ5 operations, the accuracy decreased to 86.5% and 75%, respectively. The decrease was attributed to having too many levels corresponding to the input states crowding a limited operating margin. Next, two versions of a 1-bit full adder were experimentally demonstrated using the 1 × 7 CRAM array: an all-NAND version and a MAJ + NOT version. The all-NAND design achieved an accuracy of 78.5%, while the seemingly simpler MAJ + NOT, which involves 3- and 5-input MAJ operations, only achieved an accuracy of 63.8%. Note that although each type of logic operation achieves optimal accuracy performance with a specific voltage value, the value is expected to only need to be static or constant. Therefore, only a finite number of power rails is needed to accommodate the logic operations of the CRAM array. Also, if the multiple logic pulse duration is allowed by a peripheral design, it is possible to operate the CRAM array with a single set of power rails for both memory write and logic operations.
A probabilistic model was proposed that accounts for the origin of errors, their propagation, and their accumulation during a multi-step CRAM operation. The model was shown to be effective when matched with the experimental results for the 1-bit full adder. The working principles of this model were adopted for the rest of the studies.
A suite of MTJ device circuit models was fitted to the existing experimental data and used to project CRAM NAND gate-level accuracy in the form of error rates. The gate-level error rates were shown to be 7.6 × 10 −6 , with reasonable expectations of TMR ratio improvement as MTJ technology develops. Other device properties, such as the switching probability transfer curve, could also significantly affect the CRAM gate-level error rate. This calls for improvements or new discoveries of the physical mechanisms for memory read-out and memory write. Error is an inherent property of any physical hardware, including CMOS logic components, which are commonly perceived as deterministic. As the development of CRAM proceeds, the gate-level error rate of CRAM will be further reduced over time. For now, while the error rate of CRAM is still higher compared to that of CMOS logic circuits, CRAM is naturally more suitable for applications that require less precision but can still benefit from the true-in-memory computing features and advantages of CRAM, instead of those that require high precision and determinism. Additionally, logic operations with many inputs, such as majority, may be desirable in certain scenarios. And yet, these were shown to have lower accuracy than 2-input operations. So, a tradeoff might exist.
Lastly, building on the experimental demonstration and evaluation of the 1-bit full adder designs, simulation and analysis were performed for larger functional circuits: scalar addition and multiplication up to 6 bits and matrix multiplication up to 5 bits with input size up to 4 × 4. These are essential building blocks for many conventional and machine intelligence applications. The parameters for the simulations were experimentally measured values as well as reasonable projections for future MTJ technology. The results show promising accuracy performance of CRAM at a functional building block level. Furthermore, as device technologies progress, improved performance or new switching mechanisms could further reduce the gate-level error rate of CRAM. Error correction techniques could also be employed to suppress CRAM gate errors.
In summary, this work serves as the first step in experimentally demonstrating the viability, feasibility, and realistic properties of MTJ-based CRAM hardware. Through modeling and simulation, it also lays out the foundation for a coherent view of CRAM, from the device physics level up to the application level. Prior work had established the potential of CRAM through numerical simulation only. Accordingly, there had been considerable interest in the unique features, speed, power, and energy benefits of the technology. This study puts the earlier work on a firm experimental footing, providing application-critical metrics of gate-level accuracy or error rate and linking it to the application accuracy. It paves the way for future work on large-scale applications, in conventional domains as well as new ones emerging in machine intelligence. It also indicates the possibility of making competitive large-scale CMOS-integrated CRAM hardware.
MTJ fabrication and preparation
The MTJ thin film stacks were grown by magnetron sputtering in a 12-source deposition system with a base pressure of 5 × 10 −9 Torr. The MgO barrier was fabricated by RF sputtering, while all the metallic layers were fabricated by DC sputtering. The stack structure is Si/SiO 2 /Ta(3)/Ru(6)/Ta(4)/Mo(1.2)/Co 20 Fe 60 B 20 (1)/MgO(0.9)/Co 20 Fe 60 B 20 (1.4)/Mo(1.9)/Ta(5)/Ru(7), where numbers in brackets indicate the thickness of the layer in nm. The stack was then annealed at 300 °C for 20 minutes in a rapid thermal annealing system under an Ar atmosphere (more information on the MTJ stack fabrication can be found in refs. 74 , 75 ).
The MTJ stacks were fabricated using three rounds of lithography similar to those described in ref. 76 . First, the bottom contacts were defined using photolithography followed by Ar+ ion milling etching. Then, the MTJ pillars were patterned into 120-nm circular nano-pillars via E-beam lithography and etched through Ar+ ion milling. After etching, SiO 2 was deposited via plasma-enhanced chemical vapor deposition (PECVD) to protect the nano-pillars. Finally, the top contacts were defined using photolithography, and the metallic electrodes of Ti (10 nm)/Au (100 nm) were deposited using electron beam evaporation.
The die of the MTJ array was diced into smaller pieces, with each piece containing about 10 MTJ devices. Each of the small pieces was mounted on a cartridge board, and up to 8 MTJ devices were wire-bonded to the electrodes of the cartridge board. Seven cartridge boards were inserted into the connection board, providing MTJs to the CRAM. The MTJ in each CRAM cell is selected among up to 8 MTJs on the corresponding cartridge board. In total, seven MTJs are selected from up to 56 MTJs. This method allows the user to find a collection of seven MTJs with minimum device-device variations.
CRAM experiment
An individual bias magnetic field was implemented for each of the seven MTJs on the connection board by positioning a permanent magnet at a certain distance from the MTJ devices. The bias magnetic field was used to compensate for intrinsic magnetic exchange bias and stray fields in the MTJ devices, thereby restoring the balance between the P and AP states. Additionally, slight rotation of bias field in the device plane was used to effectively adjust the switching voltage of each MTJ. More details can be found in Supplementary Note S 2 .
The connection board with seven MTJs was connected to the main board. On the main board, necessary active and passive electronic components were populated on the custom-designed PCB. The CRAM demo hardware circuit implemented a 1 × 7 CRAM array with a modified architecture to emphasize logic operations while compromising on memory operations bandwidth for simplicity. It was modified from the full-fledged 2T1M 40 architecture. It was equivalent to a 2T1M CRAM in logic mode, but it only had serial access to all cells for memory read and write operations (more details in Supplementary Note S 1 ). The hardware was powered by a battery and communicated with the controller PC wirelessly via Bluetooth®. In this way, the entire hardware was electrically isolated from the environment so that the risk of ESD to these sensitive MTJs was minimized.
The experiment control software running on a PC was implemented using National Instruments’ LabVIEW™. It was responsible for real-time measurements and control of the experiments, as well as necessary visualizations. Certain results were further analyzed post-experiment.
CRAM modeling and simulations
The simulation studies of accuracy as well as error origination, accumulation, and propagation began with a simple probabilistic model of each NAND logic operation. A probabilistic truth table was used to describe the expected statistical average of the output logical state. Then, the 1-bit full adder designs and operations were simulated using the Monte Carlo method with assumed probabilistic truth tables for each of the logic steps (see Supplementary Note S 6 ).
The experiment-based physics modeling and calculations for obtaining the projected CRAM logic operation accuracies began with an MTJ resistance-voltage model 77 , which was fit to the experimental data of TMR vs. bias voltage. The coefficients of this model were scaled accordingly to model projected TMR ratios higher than those observed experimentally. Then, a thermal activation model 78 , 79 of MTJ switching probability was fit to experimental data and was used to calculate the switching probability of the output MTJ cell under various bias voltages. Finally, the average of the output state, < D out >, could be calculated under various V logic , and the optimal NAND accuracies could be obtained in a manner similar to that discussed with Fig. 4 (more details in Supplementary Note S 8 ).
Further simulation studies of a ripple-carry adder, a systolic multiplier, and the dot-product operation of a matrix multiplication for various numbers of bits as well as matrix sizes were carried out using the same methods. More details can be found in Supplementary Note S 9 .
Data availability
The authors declare that the data supporting the findings of this study are available within the paper and its supplementary information files.
Lecun, Y., Bengio, Y. & Hinton, G. Deep learning. Nature 521 , 436–444 (2015).
Article ADS CAS PubMed Google Scholar
Jordan, M. I. & Mitchell, T. M. Machine learning: trends, perspectives, and prospects. Science 349 , 255–260 (2015).
Article ADS MathSciNet CAS PubMed Google Scholar
Adomavicius, G. & Tuzhilin, A. Toward the next generation of recommender systems: a survey of the state-of-the-art and possible extensions. IEEE Trans. Knowl. Data Eng. 17 , 734–749 (2005).
Article Google Scholar
Hinton, G. et al. Deep neural networks for acoustic modeling in speech recognition: the shared views of four research groups. IEEE Signal Process. Mag. 29 , 82–97 (2012).
Article ADS Google Scholar
Collobert, R. et al. Natural language processing (almost) from scratch. J. Mach. Learn. Res. 12 , 2493–2537 (2011).
Google Scholar
Krizhevsky, A., Sutskever, I. & Hinton, G. E. ImageNet classification with deep convolutional neural networks. Commun. ACM 60 , 84–90 (2017).
Oh, K. S. & Jung, K. GPU implementation of neural networks. Pattern Recognit. 37 , 1311–1314 (2004).
Strigl, D., Kofler, K. & Podlipnig, S. Performance and scalability of GPU-based convolutional neural networks. In 2010 18th Euromicro Conference on Parallel, Distributed and Network-based Processing 317–324 (IEEE, 2010).
Nurvitadhi, E. et al. Accelerating binarized neural networks: comparison of FPGA, CPU, GPU, and ASIC. In 2016 International Conference on Field-Programmable Technology (FPT) 77–84 (IEEE, 2017).
Sawada, J. et al. TrueNorth ecosystem for brain-inspired computing: scalable systems, software, and applications. In SC ’16: Proceedings of the International Conference for High Performance Computing, Networking, Storage and Analysis 130–141 (IEEE, 2016).
Jouppi, N. P. et al. In-datacenter performance analysis of a tensor processing unit. In Proceedings of the 44th Annual International Symposium on Computer Architecture 1–12 (ACM, 2017).
Chen, Y. H., Krishna, T., Emer, J. S. & Sze, V. Eyeriss: an energy-efficient reconfigurable accelerator for deep convolutional neural networks. IEEE J. Solid-State Circuits 52 , 127–138 (2017).
Yin, S. et al. A high energy efficient reconfigurable hybrid neural network processor for deep learning applications. IEEE J. Solid-State Circuits 53 , 968–982 (2018).
Borghetti, J. et al. Memristive switches enable stateful logic operations via material implication. Nature 464 , 873–876 (2010).
Chi, P. et al. PRIME: a novel processing-in-memory architecture for neural network computation in ReRAM-based main memory. In 2016 ACM/IEEE 43rd Annual International Symposium on Computer Architecture (ISCA) 27–39 (ACM, 2016).
Shafiee, A. et al. ISAAC: a convolutional neural network accelerator with in-situ analog arithmetic in crossbars. In 2016 ACM/IEEE 43rd Annual International Symposium on Computer Architecture (ISCA) , 14–26 (2016).
Hu, M. et al. Dot-product engine for neuromorphic computing: programming 1T1M crossbar to accelerate matrix-vector multiplication. In 2016 53nd ACM/EDAC/IEEE Design Automation Conference (DAC) 1–6 (IEEE, 2016).
Seshadri, V. et al. Ambit: in-memory accelerator for bulk bitwise operations using commodity DRAM technology. In 2017 50th Annual IEEE/ACM International Symposium on Microarchitecture (MICRO) 273–287 (IEEE, 2017).
Yao, P. et al. Fully hardware-implemented memristor convolutional neural network. Nature 577 , 641–646 (2020).
Jung, S. et al. A crossbar array of magnetoresistive memory devices for in-memory computing. Nature 601 , 211–216 (2022).
Keckler, S. W., Dally, W. J., Khailany, B., Garland, M. & Glasco, D. GPUs and the future of parallel computing. IEEE Micro 31 , 7–17 (2011).
Bergman, K. et al. ExaScale Computing Study: Technology Challenges in Achieving Exascale Systems . www.cse.nd.edu/Reports/2008/TR-2008-13.pdf (2008).
Horowitz, M. Computing’s energy problem (and what we can do about it). In 2014 IEEE International Solid-State Circuits Conference Digest of Technical Papers (ISSCC) 10–14 (IEEE, 2014).
Kim, D., Kung, J., Chai, S., Yalamanchili, S. & Mukhopadhyay, S. Neurocube: a programmable digital neuromorphic architecture with high-density 3D memory. In 2016 ACM/IEEE 43rd Annual International Symposium on Computer Architecture (ISCA) , 380–392 (2016).
Huang, J. et al. Active-routing: compute on the way for near-data processing. In 2019 IEEE International Symposium on High Performance Computer Architecture (HPCA) 674–686 (IEEE, 2019).
Nair, R. et al. Active memory cube: a processing-in-memory architecture for exascale systems. IBM J. Res. Dev. 59 , 17:1–17:14 (2015).
Pawlowski, J. T. Hybrid memory cube (HMC). In 2011 IEEE Hot Chips 23 Symposium (HCS) 1–24 (IEEE, 2011).
Gao, M., Ayers, G. & Kozyrakis, C. Practical near-data processing for in-memory analytics frameworks. In 2015 International Conference on Parallel Architecture and Compilation (PACT) 113–124 (IEEE, 2015).
Gao, M., Pu, J., Yang, X., Horowitz, M. & Kozyrakis, C. TETRIS: scalable and efficient neural network acceleration with 3D memory. SIGARCH Comput. Arch. News 45 , 751–764 (2017).
Aga, S. et al. Compute caches. In 2017 IEEE International Symposium on High Performance Computer Architecture (HPCA) 481–492 (IEEE, 2017).
Zidan, M. A., Strachan, J. P. & Lu, W. D. The future of electronics based on memristive systems. Nat. Electron. 1 , 22–29 (2018).
Jeon, K., Ryu, J. J., Jeong, D. S. & Kim, G. H. Dot-product operation in crossbar array using a self-rectifying resistive device. Adv. Mater. Interfaces 9 , 2200392 (2022).
Article CAS Google Scholar
Matsunaga, S. et al. Fabrication of a nonvolatile full adder based on logic-in-memory architecture using magnetic tunnel junctions. Appl. Phys. Express 1 , 091301 (2008).
Hanyu, T. et al. Standby-power-free integrated circuits using MTJ-based VLSI computing. Proc. IEEE 104 , 1844–1863 (2016).
Li, S. et al. Pinatubo: a processing-in-memory architecture for bulk bitwise operations in emerging non-volatile memories. In 2016 53rd ACM/EDAC/IEEE Design Automation Conference (DAC) 1–6 (IEEE, 2016).
Kvatinsky, S. et al. Memristor-based material implication (IMPLY) logic: design principles and methodologies. IEEE Trans. Very Large Scale Integr. Syst. 22 , 2054–2066 (2014).
Kvatinsky, S. et al. MAGIC—memristor-aided logic. IEEE Trans. Circuits Syst. II Express Briefs 61 , 895–899 (2014).
Wang, J.-P. & Harms, J. D. General structure for computational random access memory (CRAM). US patent 14/259,568 (2015).
Gupta, S., Imani, M. & Rosing, T. FELIX: fast and energy-efficient logic in memory. In 2018 IEEE/ACM International Conference on Computer-Aided Design (ICCAD) 1–7 (IEEE, 2018).
Chowdhury, Z. et al. Efficient in-memory processing using spintronics. IEEE Comput. Archit. Lett. 17 , 42–46 (2018).
Gao, F., Tziantzioulis, G. & Wentzlaff, D. ComputeDRAM: in-memory compute using off-the-shelf DRAMs. In 2019 52nd Annual IEEE/ACM International Symposium on Microarchitecture (MICRO) 100–113 (IEEE, 2019).
Truong, M. S. Q. et al. RACER: Bit-pipelined processing using resistive memory. In MICRO-54: 54th Annual IEEE/ACM International Symposium on Microarchitecture 100–116 (ACM, 2021).
Žutić, I., Fabian, J. & Das Sarma, S. Spintronics: fundamentals and applications. Rev. Mod. Phys. 76 , 323–410 (2004).
Nikonov, D. E. & Young, I. A. Benchmarking of beyond-CMOS exploratory devices for logic integrated circuits. IEEE J. Explor. Solid-State Comput. Devices Circuits 1 , 3–11 (2015).
Lee, T. Y. et al. World-most energy-efficient MRAM technology for non-volatile RAM applications. In 2022 International Electron Devices Meeting (IEDM) 10.7.1–10.7.4 (IEEE, 2022).
Jan, G. et al. Demonstration of ultra-low voltage and ultra low power STT-MRAM designed for compatibility with 0x node embedded LLC applications. In 2018 IEEE Symposium on VLSI Technology 65–66 (IEEE, 2018).
Zhao, H. et al. Sub-200 ps spin transfer torque switching in in-plane magnetic tunnel junctions with interface perpendicular anisotropy. J. Phys. D. Appl. Phys. 45 , 025001 (2012).
Julliere, M. Tunneling between ferromagnetic films. Phys. Lett. A 54 , 225–226 (1975).
Parkin, S. S. P. et al. Giant tunnelling magnetoresistance at room temperature with MgO (100) tunnel barriers. Nat. Mater. 3 , 862–867 (2004).
Yuasa, S., Nagahama, T., Fukushima, A., Suzuki, Y. & Ando, K. Giant room-temperature magnetoresistance in single-crystal Fe/MgO/Fe magnetic tunnel junctions. Nat. Mater. 3 , 868–871 (2004).
Berger, L. Emission of spin waves by a magnetic mulitlayer traversed by a current. Phys. Rev. B 54 , 9353–9358 (1996).
Article ADS CAS Google Scholar
Slonczewski, J. C. Current-driven excitation of magnetic multilayers. J. Magn. Magn. Mater. 159 , L1–L7 (1996).
Wei, L. et al. A 7Mb STT-MRAM in 22FFL FinFET technology with 4ns read sensing time at 0.9V using write-verify-write scheme and offset-cancellation sensing technique. In 2019 IEEE International Solid- State Circuits Conference - (ISSCC) 214–216 (IEEE, 2019).
Gallagher, W. J. et al. 22nm STT-MRAM for reflow and automotive uses with high yield, reliability, and magnetic immunity and with performance and shielding options. In 2019 International Electron Devices Meeting (IEDM) 2.7.1-2.7.4 (IEEE, 2019).
Chih, Y. Der et al. A 22nm 32Mb embedded STT-MRAM with 10ns read speed, 1M cycle write endurance, 10 years retention at 150 °C and high immunity to magnetic field interference. In 2020 IEEE International Solid- State Circuits Conference - (ISSCC) 222–224 (IEEE, 2020).
Edelstein, D. et al. A 14 nm embedded STT-MRAM CMOS technology. In 2020 International Electron Devices Meeting (IEDM) 11.5.1-11.5.4 (IEEE, 2020).
Chun, K. C. et al. A scaling roadmap and performance evaluation of in-plane and perpendicular MTJ based STT-MRAMs for high-density cache memory. IEEE J. Solid-State Circuits 48 , 598–610 (2013).
Lilja, D. J. et al. Systems and methods for direct communication between magnetic tunnel junctions. US patent 13/475,544 (2014).
Lyle, A. et al. Direct communication between magnetic tunnel junctions for nonvolatile logic fan-out architecture. Appl. Phys. Lett. 97 , 152504 (2010).
Zabihi, M. et al. Using spin-Hall MTJs to build an energy-efficient in-memory computation platform. In 20th International Symposium on Quality Electronic Design (ISQED) 52–57 (IEEE, 2019).
Currivan-Incorvia, J. A. et al. Logic circuit prototypes for three-terminal magnetic tunnel junctions with mobile domain walls. Nat. Commun. 7 , 1–7 (2016).
Alamdar, M. et al. Domain wall-magnetic tunnel junction spin-orbit torque devices and circuits for in-memory computing. Appl. Phys. Lett. 118 , 112401 (2021).
Zabihi, M. et al. Analyzing the effects of interconnect parasitics in the STT CRAM in-memory computational platform. IEEE J. Explor. Solid-State Comput. Devices Circuits 6 , 71–79 (2020).
Chowdhury, Z. I. et al. A DNA read alignment accelerator based on computational RAM. IEEE J. Explor. Solid-State Comput. Devices Circuits 6 , 80–88 (2020).
Chowdhury, Z. I. et al. CRAM-Seq: accelerating RNA-Seq abundance quantification using computational RAM. IEEE Trans. Emerg. Top. Comput. 10 , 2055–2071 (2022).
Zabihi, M. et al. In-memory processing on the spintronic CRAM: from hardware design to application mapping. IEEE Trans. Comput. 68 , 1159–1173 (2019).
Article MathSciNet Google Scholar
Cilasun, H. et al. CRAFFT: High resolution FFT accelerator in spintronic computational RAM. In 2020 57th ACM/IEEE Design Automation Conference (DAC) 1–6 (IEEE, 2020).
Resch, S. et al. PIMBALL: Binary neural networks in spintronic memory. ACM Trans. Archit. Code Optim. 16 , 41 (2019).
Chowdhury, Z. I. et al. CAMeleon: reconfigurable B(T)CAM in computational RAM. In Proceedings of the 2021 on Great Lakes Symposium on VLSI 57–63 (ACM, 2021).
Resch, S. et al. MOUSE: inference in non-volatile memory for energy harvesting applications. In 2020 53rd Annual IEEE/ACM International Symposium on Microarchitecture (MICRO) 400–414 (IEEE, 2020).
Lv, Y., Bloom, R. P. & Wang, J.-P. Experimental demonstration of probabilistic spin logic by magnetic tunnel junctions. IEEE Magn. Lett. 10 , 1–5 (2019).
Subathradevi, S. & Vennila, C. Systolic array multiplier for augmenting data center networks communication link. Cluster Comput. 22 , 13773–13783 (2019).
Liang, J., Han, J. & Lombardi, F. New metrics for the reliability of approximate and probabilistic adders. IEEE Trans. Comput. 62 , 1760–1771 (2013).
Almasi, H. et al. Perpendicular magnetic tunnel junction with W seed and capping layers. J. Appl. Phys. 121 , 153902 (2017).
Xu, M. et al. Voltage-controlled antiferromagnetism in magnetic tunnel junctions. Phys. Rev. Lett. 124 , 187701 (2020).
Lyu, D. et al. Sub-ns switching and cryogenic-temperature performance of mo-based perpendicular magnetic tunnel junctions. IEEE Electron Device Lett. 43 , 1215–1218 (2022).
Kim, J. et al. A technology-agnostic MTJ SPICE model with user-defined dimensions for STT-MRAM scalability studies. In 2015 IEEE Custom Integrated Circuits Conference (CICC) 1–4 (IEEE, 2015).
Diao, Z. et al. Spin-transfer torque switching in magnetic tunnel junctions and spin-transfer torque random access memory. J. Phys. Condens. Matter 19 , 165209 (2007).
Heindl, R., Rippard, W. H., Russek, S. E., Pufall, M. R. & Kos, A. B. Validity of the thermal activation model for spin-transfer torque switching in magnetic tunnel junctions. J. Appl. Phys. 109 , 073910 (2011).
Download references
Acknowledgements
This work was supported in part by the Defense Advanced Research Projects Agency (DARPA) via No. HR001117S0056-FP-042 “Advanced MTJs for computation in and near random-access memory” and by the National Institute of Standards and Technology. This work was supported in part by NSF SPX grant no. 1725420 and NSF ASCENT grant no. 2230963. The work at the University of Arizona is supported in part by NSF grant no. 2230124. The authors also thank Cisco Inc. for the support. Portions of this work were conducted in the Minnesota Nano Center, which was supported by the National Science Foundation through the National Nanotechnology Coordinated Infrastructure (NNCI) under Award No. ECCS-2025124. The authors acknowledge the Minnesota Supercomputing Institute (MSI, URL: http://www.msi.umn.edu ) at the University of Minnesota for providing resources that contributed to the research results reported within this paper. The authors thank Prof. Marc Riedel and Prof. John Sartori from the Department of Electrical and Computer Engineering at the University of Minnesota for proofreading the manuscript. Yang Lv, Brandon Zink, and Hüsrev Cılasun were CISCO Fellows.
Author information
Authors and affiliations.
Department of Electrical and Computer Engineering, University of Minnesota, Minneapolis, MN, 55455, USA
Yang Lv, Brandon R. Zink, Robert P. Bloom, Hüsrev Cılasun, Salonik Resch, Zamshed Chowdhury, Sachin S. Sapatnekar, Ulya Karpuzcu & Jian-Ping Wang
Department of Physics, University of Arizona, Tucson, Arizona, 85721, USA
Pravin Khanal, Ali Habiboglu & Weigang Wang
You can also search for this author in PubMed Google Scholar
Contributions
J.-P.W. conceived the CRAM research and coordinated the entire project. Y.L. and J.-P.W. designed the experiments. Y.L. and R.P.B. designed and developed the demonstration hardware and software. P.K., A.H., and W.W. grew part of the perpendicular MTJ stacks. B.R.Z. fabricated the MTJ nanodevices. Y.L. conducted the CRAM demonstration experiments and analyzed the results. Y.L. studied the probabilistic model of CRAM operations and conducted simulations of a 1-bit full adder. Y.L., B.R.Z., and R.P.B. developed the device physics modeling of CRAM logic operations and gate-level error rates and conducted related calculations. H.C., S.R., Z.C., and U.K. carried out the simulation studies of the multi-bit adder, multiplier, and matrix multiplication. S.S. participated in discussions of modeling and simulation. All authors reviewed and discussed the results. Y.L. and J.-P.W. wrote the draft manuscript. All authors contributed to the completion of the manuscript.
Corresponding author
Correspondence to Jian-Ping Wang .
Ethics declarations
Competing interests.
The authors declare no competing interests.
Additional information
Publisher’s note Springer Nature remains neutral with regard to jurisdictional claims in published maps and institutional affiliations.
Supplementary information
Supplementary information, rights and permissions.
Open Access This article is licensed under a Creative Commons Attribution 4.0 International License, which permits use, sharing, adaptation, distribution and reproduction in any medium or format, as long as you give appropriate credit to the original author(s) and the source, provide a link to the Creative Commons licence, and indicate if changes were made. The images or other third party material in this article are included in the article’s Creative Commons licence, unless indicated otherwise in a credit line to the material. If material is not included in the article’s Creative Commons licence and your intended use is not permitted by statutory regulation or exceeds the permitted use, you will need to obtain permission directly from the copyright holder. To view a copy of this licence, visit http://creativecommons.org/licenses/by/4.0/ .
Reprints and permissions
About this article
Cite this article.
Lv, Y., Zink, B.R., Bloom, R.P. et al. Experimental demonstration of magnetic tunnel junction-based computational random-access memory. npj Unconv. Comput. 1 , 3 (2024). https://doi.org/10.1038/s44335-024-00003-3
Download citation
Received : 29 January 2024
Accepted : 29 May 2024
Published : 25 July 2024
DOI : https://doi.org/10.1038/s44335-024-00003-3
Share this article
Anyone you share the following link with will be able to read this content:
Sorry, a shareable link is not currently available for this article.
Provided by the Springer Nature SharedIt content-sharing initiative
Quick links
- Explore articles by subject
- Guide to authors
- Editorial policies
Sign up for the Nature Briefing: AI and Robotics newsletter — what matters in AI and robotics research, free to your inbox weekly.


Multi-strategies embedded framework for neoantigen vaccine maturation
- Find this author on Google Scholar
- Find this author on PubMed
- Search for this author on this site
- ORCID record for Ruhong Zhou
- For correspondence: [email protected]
- Info/History
- Supplementary material
- Preview PDF
Effective cancer immunotherapy hinges on the precise recognition of neoantigens, presented as binary complexes with major histocompatibility complex (MHC) molecules, by T cell receptors (TCR). The development of immunogenic peptide predictors and generators plays a central role in personalizing immunotherapies while reducing experimental costs. However, the current methods often fall short in leveraging structural data efficiently and providing comprehensive guidance for neoantigen selection. To address these limitations, we introduce NEOM, a novel neoantigen maturation framework encompassing five distinct modules: policy, structure, evaluation, selection and filter. This framework is designed to enhance precision, interpretability, customizability and cost-effectiveness in neoantigen screening. We evaluated NEOM using a set of random synthetic peptides, followed by available clinically-derived peptides. NEOM achieved higher performance on generated peptide quality compared to other baseline models. Using established predictors for filtering revealed a substantial number of peptides with immunogenic potential. Subsequently, a more rigorous binding affinity evaluation using free energy perturbation methods identified 6 out of 38 candidates showing superior binding characteristics. MHC tetramer peptide exchange assays and flow cytometry experiments further validate five of them. These results demonstrate that NEOM not only excels in identifying diverse peptides with enhanced binding stability and affinity for MHC molecules but also augments their immunogenic potential, showcasing its utility in advancing personalized immunotherapies.
Competing Interest Statement
The authors have declared no competing interest.
View the discussion thread.
Supplementary Material
Thank you for your interest in spreading the word about bioRxiv.
NOTE: Your email address is requested solely to identify you as the sender of this article.

Citation Manager Formats
- EndNote (tagged)
- EndNote 8 (xml)
- RefWorks Tagged
- Ref Manager
- Tweet Widget
- Facebook Like
- Google Plus One
Subject Area
- Bioinformatics
- Animal Behavior and Cognition (5524)
- Biochemistry (12565)
- Bioengineering (9426)
- Bioinformatics (30804)
- Biophysics (15840)
- Cancer Biology (12913)
- Cell Biology (18520)
- Clinical Trials (138)
- Developmental Biology (9996)
- Ecology (14967)
- Epidemiology (2067)
- Evolutionary Biology (19148)
- Genetics (12729)
- Genomics (17528)
- Immunology (12674)
- Microbiology (29713)
- Molecular Biology (12365)
- Neuroscience (64695)
- Paleontology (479)
- Pathology (2000)
- Pharmacology and Toxicology (3451)
- Physiology (5324)
- Plant Biology (11089)
- Scientific Communication and Education (1728)
- Synthetic Biology (3063)
- Systems Biology (7682)
- Zoology (1728)
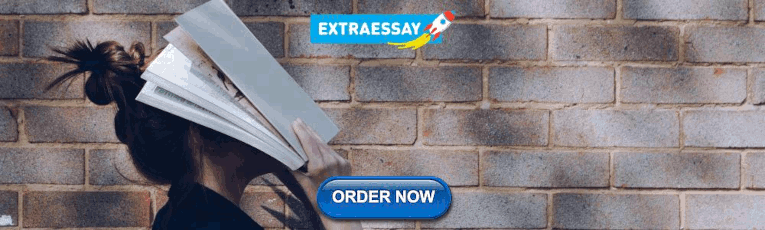
IMAGES
COMMENTS
6. Experimental research allows cause and effect to be determined. The manipulation of variables allows for researchers to be able to look at various cause-and-effect relationships that a product, theory, or idea can produce. It is a process which allows researchers to dig deeper into what is possible, showing how the various variable ...
Common types of limitations and their ramifications include: Theoretical: limits the scope, depth, or applicability of a study. Methodological: limits the quality, quantity, or diversity of the data. Empirical: limits the representativeness, validity, or reliability of the data. Analytical: limits the accuracy, completeness, or significance of ...
Experimental research serves as a fundamental scientific method aimed at unraveling. cause-and-effect relationships between variables across various disciplines. This. paper delineates the key ...
7. Experimental research cannot always control all of the variables. Although experimental research attempts to control every variable or combination that is possible, laboratory settings cannot reach this limitation in every circumstance. If data must be collected in a natural setting, then the risk of inaccurate information rises.
List of Disadvantages of Experimental Research. 1. It can lead to artificial situations. In many scenarios, experimental researchers manipulate variables in an attempt to replicate real-world scenarios to understand the function of drugs, gadgets, treatments, and other new discoveries. This works most of the time, but there are cases when ...
10 Experimental research. 10. Experimental research. Experimental research—often considered to be the 'gold standard' in research designs—is one of the most rigorous of all research designs. In this design, one or more independent variables are manipulated by the researcher (as treatments), subjects are randomly assigned to different ...
The limitations of experimental research must also be noted. The first and foremost limitation is that it can only be used when the conditions are appropriate for manipulating the variables. Sometimes, certain practical and ethical issues may prevent us from adopting this method.
Research limitations are one of those things that students tend to avoid digging into, and understandably so. No one likes to critique their own study and point out weaknesses. Nevertheless, being able to understand the limitations of your study - and, just as importantly, the implications thereof - a is a critically important skill. In this post, we'll unpack some of the most common ...
Researchers, Dudley-Marling and Rhodes, address some problems they met in their experimental approach to a study of reading comprehension. This article discusses the limitations of experimental research, and presents an alternative to experimental or quantitative research. Edgington, E. S. (1985). Random assignment and experimental research.
external validity. Experimental research can control many of the threats to the validity of an experiment. It is the responsibility of the researcher to control for threats to internal and external validity. 1.1 True Experimental Designs The first type of experimental research is referred to as true experimental designs. For a research design ...
When discussing the limitations of your research, be sure to: Describe each limitation in detailed but concise terms; ... Moreover, the absence of an effect may be very telling in many situations, particularly in experimental research designs. In any case, your results may very well be of importance to others even though they did not support ...
The findings suggest that experimental research is subject to a number of methodological limitations that may jeopardise internal and external validity of the research results and, consequently, limit their applicability for practice. Nurses are therefore encouraged to carefully consider the virtues of experimental designs, in their quest for ...
Experimental research design is a framework of protocols and procedures created to conduct experimental research with a scientific approach using two sets of variables. Herein, the first set of variables acts as a constant, used to measure the differences of the second set. ... Research Limitations. Every study has some type of limitations. You ...
Identify the limitations: Start by identifying the potential limitations of your research. These may include sample size, selection bias, measurement error, or other issues that could affect the validity and reliability of your findings. Be honest and objective: When describing the limitations of your research, be honest and objective.
The Advantages of Experimental Research. 1. A High Level Of Control With experimental research groups, the people conducting the research have a very high level of control over their variables. By isolating and determining what they are looking for, they have a great advantage in finding accurate results. 2.
There are three types of experiments you need to know: 1. Lab Experiment. A laboratory experiment in psychology is a research method in which the experimenter manipulates one or more independent variables and measures the effects on the dependent variable under controlled conditions. A laboratory experiment is conducted under highly controlled ...
In research, studies can have limitations such as limited scope, researcher subjectivity, and lack of available research tools. Acknowledging the limitations of your study should be seen as a strength. It demonstrates your willingness for transparency, humility, and submission to the scientific method and can bolster the integrity of the study.
There are 3 main types of experimental research, namely; pre-experimental, quasi-experimental, and true experimental research. Pre-experimental Research Pre-experimental research is the simplest form of research, and is carried out by observing a group or groups of dependent variables after the treatment of an independent variable which is ...
Experimental research on PEM seems generally hard to find. That is why all of the above-presented PEM studies are own studies. To underline this, in 2018, Fischer et al. presented a systematic review of experimental studies on conceptual modeling which takes a more general perspective than just considering PEM. The review shows that even in the ...
When discussing the limitations of your research, be sure to: Describe each limitation in detailed but concise terms; ... Moreover, the absence of an effect may be very telling in many situations, particularly in experimental research designs. In any case, your results may be of importance to others even though they did not support your ...
Writing the limitations of the research papers is often assumed to require lots of effort. However, identifying the limitations of the study can help structure the research better. Therefore, do not underestimate the importance of research study limitations. 3. Opportunity to make suggestions for further research.
Our set of six recommendations for online experiments differs significantly from the "Ten Commandments" of experimental research (Lonati et al., 2018), ... To summarize, despite the limitations of online panels, online experiments are expected to be popular in travel and hospitality settings in future (Kim, Kim et al., 2023). We hope that ...
List of Advantages of Experimental Research. 1. Control over variables. This kind of research looks into controlling independent variables so that extraneous and unwanted variables are removed. 2. Determination of cause and effect relationship is easy.
AI tools are used in many fields, including learning English for high school pupils. This study aims to synthesize the benefits and limitations of using AI in learning English and the current ...
Experimental plan for field testing, including protocols for participant engagement, instrumentation, monitoring, and data collection (Metzger et al. Citation 2019). Conduct study: Acquire and install test windows, refine test protocols with feedback from local research partners, and complete sensor installation, monitoring, and data collection.
The conventional computing paradigm struggles to fulfill the rapidly growing demands from emerging applications, especially those for machine intelligence because much of the power and energy is ...
Effective cancer immunotherapy hinges on the precise recognition of neoantigens, presented as binary complexes with major histocompatibility complex (MHC) molecules, by T cell receptors (TCR). The development of immunogenic peptide predictors and generators plays a central role in personalizing immunotherapies while reducing experimental costs. However, the current methods often fall short in ...