Academia.edu no longer supports Internet Explorer.
To browse Academia.edu and the wider internet faster and more securely, please take a few seconds to upgrade your browser .
Enter the email address you signed up with and we'll email you a reset link.
- We're Hiring!
- Help Center
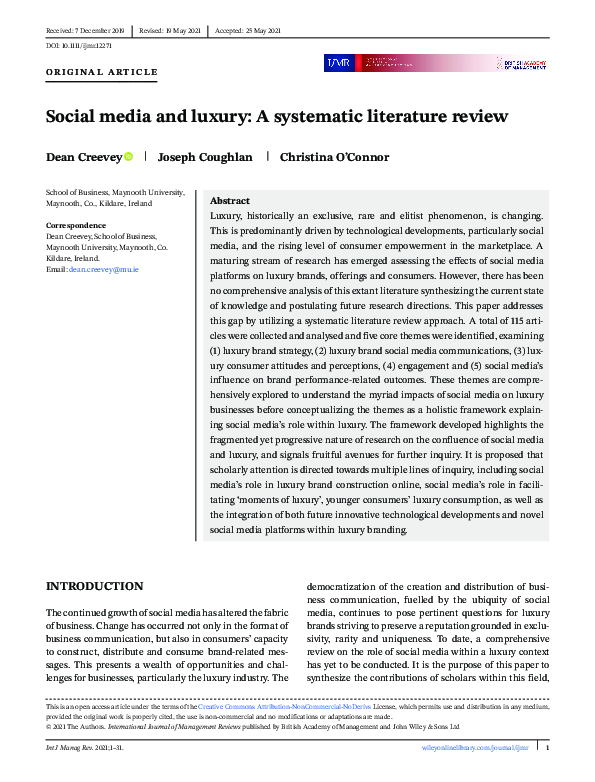
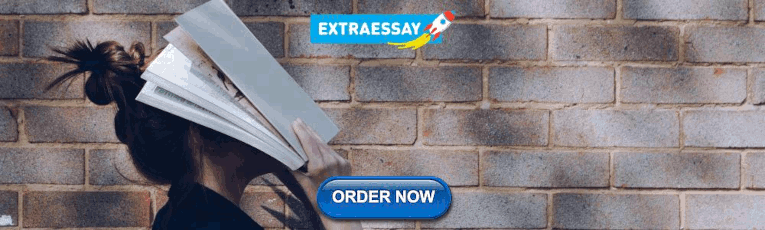
Social media and luxury: A systematic literature review

2021, International Journal of Management Reviews
Related Papers
Delphine Veissiere
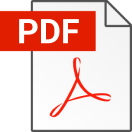
International Journal of Marketing Studies
cesare amatulli
People and companies worldwide are by now involved and attached to web 2.0 technologies and in particular to social media platforms. In this context, these digital technologies and social media have modified and improved the way to communicate and collaborate between companies and customers in all sectors through by implementing effective interaction mechanisms. However, the approach to social media and digital technologies in sectors such as luxury has been slower. Therefore, the main aim of this study is firstly to systematically examine and review the current studies conducted on the related area of social media and luxury marketing. Secondly, in addition to providing an overview of the main themes and trends covered by the relevant literature, this review has focused on the consumption of luxury and the dual dimension that it can take in a social media context. Third, a systematic review of the literature on academic research on social media marketing in luxury brands was cond...
Journal of Theoretical and Applied Electronic Commerce Research
Amadea Agapie
As consumers spend more time on social media, brands can take advantage of this opportunity to better serve and communicate with their followers. Still, given the characteristics of luxury, luxury brands may be reluctant to use social media. To determine the extent to which Millennials and Generation Z consumers perceive compatibility between social media marketing and luxury brands, a purposive sampling technique was employed. We collected data from 303 Romanian visitors of the Facebook page entitled Do You Like Luxury? This page, with postings from luxury producers, was specially created and sponsored in order to raise interest in completing the questionnaires. For data analysis, we used statistical tests, including confirmatory factorial analysis, regression, and independent-samples t-test. Our research shows that, for Millennials and Generation Z, luxury brands are compatible with social media apps as marketing channels. Even in the case of inadequacy, managers can still use the...
Sophie Visvikis-siest
Creative Commons Attribution Non-Commercial License
Doç. Dr. Merve Çelik Varol
The main purpose of this search, to examine the effects of social media on luxury consumption. Online survey form created for the purpose, between the dates of 1-6 June 2016 social media has been applied to the 208 user. The Program SPSS 22.0 statistics of the survey data is realized through the evaluation of the achieved. Impact of social media on luxury consumption in determining the level of the participants, the average and standard deviation statistics were used, descriptive characteristics of the participants to examine the effects of social media on luxury consumption in terms of the kruskal-Wallis H and Mann-Whitney u tests were used. As a result of the research , the impact of social media on a low level of participants, the effects of luxury consumption on social media sites increased with the increment of income level and it was concluded that used. The effects of social media on luxury consumption, individuals ' gender, age, educational status, and the social media sites they use most in the research that does not vary according to other results that can be reached. SOSYAL MEDYANIN LÜKS TÜKETİM ÜZERİNDEKİ ETKİLERİ ÖZ Bu araştırmanın temel amacı, sosyal medyanın lüks tüketim üzerindeki etkilerini incelemektir. Amaç doğrultusunda oluşturulan çevrimiçi anket formu, 1-6 Haziran 2016 tarihleri arasında 208 sosyal medya kullanıcısına uygulanmıştır. Anketlerden ulaşılan verilerin değerlendirilmesi bilgisayar ortamında SPSS 22.0 istatistik programı aracılığıyla gerçekleştirilmiştir. Katılımcıların lüks tüketimleri üzerinde sosyal medyanın etki düzeyinin belirlenmesinde ortalama ve standart sapma istatistiklerinden, katılımcıların tanımlayıcı özellikleri açısından sosyal medyanın lüks tüketim üzerindeki etkilerini incelemek için Kruskal Wallis H ve Mann Whitney U testlerinden faydalanılmıştır. Araştırma sonucunda, sosyal medyanın katılımcılar üzerindeki etkisinin düşük düzeyde olduğu, sosyal medyanın lüks tüketim üzerindeki etkilerinin gelir düzeyi ve sosyal medya sitelerinde kullanılan süre artışıyla birlikte arttığı sonucuna varılmıştır. Sosyal medyanın lüks tüketim üzerindeki etkilerinin, bireylerin cinsiyetlerine, yaşlarına, eğitim durumları ve en fazla kullandıkları sosyal medya sitelerine göre farklılık göstermediği araştırmada ulaşılan diğer sonuçlardır.
Gilberto Sarfati
Jianqiong Xu
The advances in communication media have changed the way people search or share information. Social media are the two-way forms of communication that provide new opportunities to users to interact with each other on platforms to share ideas. This study analyses customer expectations and interactions through integrating social media and luxury fashion brands. Also, by identifying the advantages that can be obtained by businesses from social media, this paper develops a structural model that contributes to address the gap and current knowledge of consumer buying behaviour in luxury product purchases under the effect of social media. The research also suggests that it is important to understand and interact with customer expectations via the combination of social media and traditional marketing media to develop effective marketing communications. The paper concludes with some strategies that practitioners and researchers can adopt to develop effective marketing communication strategies...
Sekandar Riaz
jyoti peswani
Sandrina Teixeira
The luxury sector is currently one of the most exciting market segments, standing out because of its ability to keep growing during economic crisis. In fact, high end consumers are a particularly attractive segment. They are willing to pay more for exclusive products and unique experiences, they value the symbolic meanings carried out by luxury products and brands, and they demand superior performance from luxury brand suppliers. But they are not the only ones choosing and buying luxury brands, as luxury is being increasingly adopted by the middle class, namely as a signal of success (Wiedmann & Hennigs, 2013)...
RELATED PAPERS
Nature Genetics
Raju Kucherlapati
Lokender Kumar
Infection and Immunity
Luis Antonio Corchete
Scott Wells
Bryan Medina
Cécile Sakai
Journal of Microwaves, Optoelectronics and Electromagnetic Applications
ivan casella
Acta Universitatis Cibiniensis. Series E: Food Technology
Charles Chukwu
PLOS Neglected Tropical Diseases
Michael Kosoy
Endocrinology
Gloria Perez
David Torres
2020 25th International Conference on Pattern Recognition (ICPR)
Ita Muzdalifah
Acta Dermato Venereologica
Yogesh Yadav
The Lancet. Infectious diseases
Mary Ramsay
SSRN Electronic Journal
Marie Claire Villeval
OncoImmunology
Filippo Fraggetta
Ameghiniana
Matías Armella
fbd.aydin.edu.tr
Ahmet tokgözlü
Zbigniew Truchlewski
Seventh International Conference on Document Analysis and Recognition, 2003. Proceedings.
Jin Hyung Kim
Ayşegül ILGAZ
ARAM 34: 1&2 (2022), 271-292
Annette Landes-Nagar
Julie Spielberger
See More Documents Like This
RELATED TOPICS
- We're Hiring!
- Help Center
- Find new research papers in:
- Health Sciences
- Earth Sciences
- Cognitive Science
- Mathematics
- Computer Science
- Academia ©2024
To read this content please select one of the options below:
Please note you do not have access to teaching notes, social media marketing in luxury brands: a systematic literature review and implications for management research.
Management Research Review
ISSN : 2040-8269
Article publication date: 3 April 2018
Issue publication date: 5 June 2018
Digital technologies and social media have improved the connectivity and collaboration between firms and customers in all sectors. However, in the luxury sector, the approach to social media and digital technologies has been slower than in other industries. The purpose of this paper is to review the academic literature on social media marketing in luxury brands to highlight the current state of the art, the addressed key research themes and the implications for management research and practice.
Design/methodology/approach
A systematic literature review of academic research on social media marketing has been conducted to gather, examine and synthetize studies related to luxury brands. By following a review protocol based on both automatic and manual search on the Scopus database, all relevant studies on luxury brands were identified and analyzed.
A critical conceptualization of social media marketing in luxury brands has been provided and the emerging key research themes have been categorized into four main areas.
Originality/value
Academic literature about social media marketing activities in luxury firms is very limited and existing studies focus only on certain aspects, contexts or single cases. In contrast, the value of this study, for both academics and practitioners, lies in providing, for the first time, a comprehensive and critical systematization of social media marketing academic literature in the field of luxury brands.
- Systematic review
- Co-creation
- Competitive advantage
- Social media marketing
- Digital luxury
- Luxury brand
Arrigo, E. (2018), "Social media marketing in luxury brands: A systematic literature review and implications for management research", Management Research Review , Vol. 41 No. 6, pp. 657-679. https://doi.org/10.1108/MRR-04-2017-0134
Emerald Publishing Limited
Copyright © 2018, Emerald Publishing Limited
Related articles
We’re listening — tell us what you think, something didn’t work….
Report bugs here
All feedback is valuable
Please share your general feedback
Join us on our journey
Platform update page.
Visit emeraldpublishing.com/platformupdate to discover the latest news and updates
Questions & More Information
Answers to the most commonly asked questions here
Conceptualising and measuring social media engagement: A systematic literature review
- Review Article
- Open access
- Published: 11 August 2021
- Volume 2021 , pages 267–292, ( 2021 )
Cite this article
You have full access to this open access article
- Mariapina Trunfio 1 &
- Simona Rossi ORCID: orcid.org/0000-0003-4384-0002 1
26k Accesses
30 Citations
Explore all metrics
The spread of social media platforms enhanced academic and professional debate on social media engagement that attempted to better understand its theoretical foundations and measurements. This paper aims to systematically contribute to this academic debate by analysing, discussing, and synthesising social media engagement literature in the perspective of social media metrics. Adopting a systematic literature review, the research provides an overarching picture of what has already been investigated and the existing gaps that need further research. The paper confirms the polysemic and multidimensional nature of social media engagement. It identifies the behavioural dimension as the most used proxy for users' level of engagement suggesting the COBRA model as a conceptual tool to classify and interpret the construct. Four categories of metrics emerged: quantitative metrics, normalised indexes, set of indexes, qualitative metrics. It also offers insights and guidance to practitioners on modelling and managing social media engagement.
Similar content being viewed by others
A Model of Social Media Engagement: User Profiles, Gratifications, and Experiences
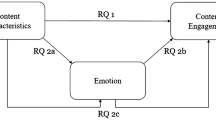
Impact of content characteristics and emotion on behavioral engagement in social media: literature review and research agenda
Melanie Schreiner, Thomas Fischer & Rene Riedl
Social Media as Online Information Grounds: A Preliminary Conceptual Framework
Avoid common mistakes on your manuscript.
1 Introduction
Over the last decade, customer engagement has received increasing attention in academic and professional debate (Hollebeek, 2019 ; Kumar et al., 2019 ; Marketing Science Institute, 2020 ; Peltier et al., 2020 ; Rather et al., 2019 ; Rossmann et al., 2016 ). It can be considered a “consumer’s positively brand-related cognitive, emotional and behavioural activity during, or related to, focal consumer/brand interactions” (Hollebeek, 2014 , p.149). Engaged customers display greater brand loyalty and satisfaction (Bowden, 2009 ; Jaakkola & Alexander, 2014 ) and are more likely to contribute to new product development (Haumann et al., 2015 ), service innovation (Kumar et al., 2010 ), and viral marketing activity spread by word of mouth (Wu et al., 2018 ). Customer engagement can also be linked with important brand performance indicators, including sales growth, feedback, and referrals (Van Doorn et al., 2010 ).
Acknowledging the potential of ICTs, scholars and practitioners are experimenting with new ways to capitalise on customer engagement and adapt to the new challenges of digital platforms (Barger et al., 2016 ; Peltier et al., 2020 ). Social media platforms reshaped the dyadic interaction between customers and organisations, creating spaces for digital sharing and engagement. By enabling users to comment, review, create, and share content across online networks, social media provide direct access to brands and allow co-creation processes. As such, the pervasive character of social media with its potential for engaging with customers and building relationships generated much interest in the concept of social media engagement (Barger et al., 2016 ; Hallock et al., 2019 ; Oviedo-García et al., 2014 ; Peltier et al., 2020 ; Schivinski et al., 2016 ). Engaging with customers in real-time and managing many incoming customers’ big data interested academic investigation and opened opportunities for marketers to enhance social media marketing success (Liu et al., 2019 ).
Understanding, monitoring, and measuring social media engagement are key aspects that interest scholars and practitioners who proposed diverse conceptualisations, several indicators and KPIs. With the spread of social media analytics, social networking platforms, digital service providers, marketers, and freelancers developed their metrics to measure engagement with brand-related social media contents and advertising campaigns. At the same time, scholars have pointed out various metrics and procedures that contribute to evaluating social media engagement in different fields (Mariani et al., 2018 ; Muñoz-Expósito et al., 2017 ; Trunfio & Della Lucia, 2019 ). Nevertheless, many of these studies offer a partial perspective of analysis that does not allow the phenomenon to be represented in diverse aspects (Oviedo-García et al., 2014 ). As a result, social media engagement remains an enigma wrapped in a riddle for many executives (McKinsey, 2012 ). How communities across an ever-growing variety of platforms, new forms of customer-brand interactions, different dimensions and cultural differences impact social media engagement measurement represents one of the main challenges (Peltier et al., 2020 ).
Although social media engagement represented a key topic in marketing research (Barger et al., 2016 ; Peltier et al., 2020 ), an overarching perspective of the existing knowledge can drive the investigation of the state of the field, including the study of the research streams, and the analysis of the measurement tools. This paper aims to systematically contribute to the academic debate by analysing, discussing, and synthesising social media engagement literature from the social media metrics perspective. A systematic literature review approach provides an overarching picture of what has already been investigated and the existing gaps that need further research. It contributes towards a systematic advancement of knowledge in the field and offers insights and guidance to practitioners on modelling and managing social media engagement (Tranfield et al., 2003 ).
The remainder of the paper is structured as follows. Section 2 presents the theoretical background of the study on customer engagement and social media engagement. Section 3 describes the methodology used for conducting the systematic literature review (Pickering & Byrne, 2014 ; Tranfield et al., 2003 ). Section 4 presents the bibliometric analysis results, including the year in which research began, the journals that publish most research, and the most relevant authors with publications on the topic. Then, Sect. 5 classifies these studies in terms of four macro-themes, conceptualisations, platforms, measurement, and behaviours and describes the key results available in the literature. Section 6 provides a critical discussion of the findings from the literature review and highlights its key contributions. Lastly, Sect. 7 concludes the study by highlighting its limitations and proposing directions for future research.
2 Theoretical background
2.1 customer engagement.
Although customer engagement research has increased theoretical and managerial relevance (Brodie et al., 2011 ; Hollebeek et al., 2016 , 2019 ; Kumar et al., 2019 ; Vivek et al., 2012 ), to date, there is still no consensus on its definition due to its multidimensional, multidisciplinary and polysemic nature.
Several customer engagement conceptualisations have been proposed in the literature, drawing on various theoretical backgrounds, particularly service-dominant logic, and relationship marketing. From a psychological perspective, one of the first definitions of customer engagement is the one of Bowden ( 2009 ) that conceptualises it as a psychological process that drives customer loyalty. Similarly, Brodie et al. ( 2011 ) define customer engagement as a psychological state that occurs by interactive, co-creative customer experiences with a focal object. Later, focusing on the behavioural aspects, it has been described as the intensity of an individual’s participation in an organisation’s offerings or organisational activities (Vivek et al., 2012 ). More recently, from a value-based perspective, customer engagement has been defined as the mechanics that customers use to add value to the firm (Kumar et al., 2019 ).
Although the perspectives may vary, common elements can be identified in various conceptualisations. Literature generally understands customer engagement as a highly experiential, subjective, and context-dependent construct (Brodie et al., 2011 ) based on customer-brand interactions (Hollebeek, 2018 ). Moreover, scholars agree on its multidimensional nature (Brodie et al., 2013 ; Hollebeek et al., 2016 ; So et al., 2016 ; Vivek et al., 2012 ) encompassing cognitive (customer focus and interest in a brand), emotional (feelings of inspiration or pride caused by a brand), and behavioural (customer effort and energy necessary for interaction with a brand) dimensions. Also, researchers have proposed that customer engagement affects different marketing constructs (Brodie et al., 2011 ; Van Doorn et al., 2010 ). For example, in Bowden’s research (2009), there is evidence to support that customer engagement is a predictor of loyalty. Brodie et al. ( 2011 ) explore its effects on customer satisfaction, empowerment, trust, and affective commitment towards the members of a community. Van Doorn et al. ( 2010 ) propose customer-based drivers, including attitudinal factors such as satisfaction, brand commitment and trust, as well as customer goals, resources, and value perceptions.
2.2 Social media engagement: The academic perspective
Social media engagement has also been investigated as brand-user interaction on social media platforms (Barger et al., 2016 ; De Vries & Carlson, 2014 ; Hallock et al., 2019 ; Oviedo-García et al., 2014 ; Peltier et al., 2020 ; Schivinski et al., 2016 ). However, while conceptual discussions appear to dominate the existing customer engagement literature, research results fragmented when moving to the online context. Scholars agree that social media engagement is a context-specific occurrence of customer engagement (Brodie et al., 2013 ) that reflects customers’ individual positive dispositions towards the community or a focal brand (Dessart, 2017 ). Social media engagement can emerge with respect to different objects: the community, representing other customers in the network, and the brand (Dessart, 2017 ). Furthermore, antecedents and consequences of social media engagement have been identified to understand why customers interact on social media and the possible outcomes (Barger et al., 2016 ), such as loyalty, satisfaction, trust, and commitment (Van Doorn et al., 2010 ).
In continuity with literature on customer engagement, also social media engagement can be traced back to affective, cognitive, and behavioural dimensions (Van Doorn et al., 2010 ). Most of the literature focuses on the behavioural dimension as it can be expressed through actions such as liking, commenting, sharing, and viewing contents from a brand (Barger et al., 2016 ; Muntinga et al., 2011 ; Oh et al., 2017 ; Oviedo-García et al., 2014 ; Peltier et al., 2020 ; Rietveld et al., 2020 ; Schivinski et al., 2016 ). It is worth pointing out that not all these actions determine the same level of engagement. Schivinski et al. ( 2016 ) in the COBRA (Consumer Online Brand Related Activities) Model differentiate between three levels of social media engagement: consumption, contribution, and creation. Consumption constitutes the minimum level of engagement and is the most common brand-related activity among customers (e.g., viewing brand-related audio, video, or pictures). Contribution denotes the response in peer-to-peer interactions related to brands (e.g., liking, sharing, commenting on brand-related contents). Creation is the most substantial level of the online brand-related activities that occur when customers spontaneously participate in customising the brand experiences (e.g., publishing brand-related content, uploading brand-related video, pictures, audio or writing brand-related articles). Starting from these social media actions, scholars attempted to measure social media engagement in several ways developing scales, indexes, and metrics (Harrigan et al., 2017 ; Oviedo-García et al., 2014 ; Schivinski et al., 2016 ; Trunfio & Della Lucia, 2019 ). Nevertheless, many of these studies offer a partial perspective of analysis that does not allow the phenomenon to be represented in its diverse aspects (Oviedo-García et al., 2014 ). Researchers have also examined emotional and cognitive dimensions (Dessart, 2017 ) as essential components of social media engagement that lead to positive brand outcomes (Loureiro et al., 2017 ).
2.3 Social media engagement: The practitioners’ perspective
In business practice, the concept of customer engagement appeared for the first time in 2006 when the Advertising Research Foundation (ARF), in conjunction with the American Association of Advertising Agencies and the Association of National Advertisers, defined it as a turning on a prospect to a brand idea enhanced by the surrounding context (ARF, 2006 ) . Later, several consulting firms tried to give their definition emphasising different aspects and perspectives. For example, in 2008, Forrester Consulting, an American market research company, defined customer engagement as a way to create ‘deep connections with customers that drive purchase decisions, interaction, and participation over time’ (Forrester Consulting, 2008 , p.4). Gallup Consulting identified four levels of customer engagement and defined it as an emotional connection between customers and companies (Gallup Consulting, 2009 ). Similarly, the famous American software provider Hubspot ( 2014 ) identified social media engagement as ‘ the ongoing interactions between company and customer, offered by the company, chosen by the customer’ (Hubspot, 2014 , p.1).
With the increasing spread of social networks and their exploitation as an important marketing tool, practitioners recognised a clear linkage between customer engagement and the metrics to assess digital strategy success. Over time, social networking platforms such as Facebook, LinkedIn, and YouTube, developed their metrics to measure engagement with brand-related social media contents and advertising campaigns (Table 1 ).
With the spread of social media analytics, platforms and digital service providers developed dashboards and analytical indicators to assess, measure and monitor the engagement generated by social media marketing activities (Table 2 ). At the same time, many bloggers, marketers, and freelancers have weighed in on the topic, enriching the debate with new contributions.
As a result, while scholars still have to agree upon a shared definition of social media engagement, marketers have recognised it as one of the most important online outcome companies need to deliver with social media and a key metric to assess social media strategy success . Despite the growing interest in business practice and its solid traditional theoretical roots, most of the existing literature on social media engagement offers only conceptual guidelines (Barger et al., 2016 ; Peltier et al., 2020 ). The measurement of engagement in social media and its financial impact remains an enigma wrapped in a riddle for many executives (McKinsey, 2012 ) and requires further investigations. Mainly, how new and emerging platforms, new forms of customer-brand interactions, different dimensions, and cultural differences impact social media engagement measurement remains an understudied phenomenon (Peltier et al., 2020 ).
3 Methodology
The literature review is one of the most appropriate research methods, which aims to map the relevant literature identifying the potential research gaps that need further research to contribute towards a systematic advancement of new knowledge in the field (Tranfield et al., 2003 ). This research is built upon the rigorous, transparent, and reproducible protocol of the systematic literature review as a scientific and transparent process that reduces the selection bias through an exhaustive literature search (Pencarelli & Mele, 2019 ; Pickering & Byrne, 2014 ; Tranfield et al., 2003 ). Building on recent studies (Inamdar et al., 2020 ; Linnenluecke et al., 2020 ; Phulwani et al., 2020 ), in addition to the systematic literature review, a bibliometric analysis (Li et al., 2017 ) was also performed to provide greater comprehensions into the field's current state and highlight the future research directions.
3.1 Database, keywords, inclusion, and exclusion criteria
To conduct a literature review, quality journals are considered the basis for selecting quality publications (Wallace & Wray, 2016 ). Therefore, the database Scopus, run by Elsevier Publishing, was considered to search for relevant literature, being the most significant abstract and citation source database used in recent reviews.
When conducting a literature review, a fundamental issue is determining the keywords that allow identifying the papers (Aveyard, 2007 ). To address it, the most frequently used keywords in peer-reviewed literature have been under investigation. As such, the following research chain was used: “Social media” “Engagement” AND “metric*”, searching under title, abstract, and keywords.
The systematic literature review protocol (Fig. 1 ) has been conducted on the 26 th of March 2020. The study considers an open starting time to trace back to the origin of social media engagement metrics research up to late March 2020. The initial search attempts identified 259 documents.
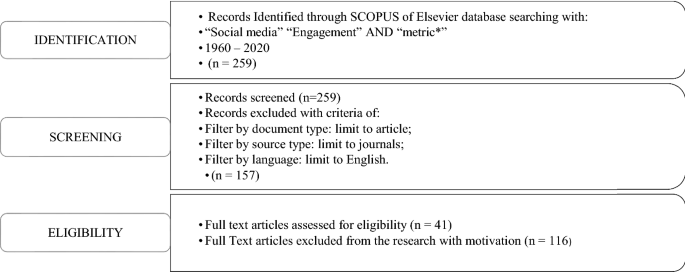
The systematic literature review protocol
After the articles’ identification, criteria for inclusion and exclusion were adopted. First, the 259 articles were screened, considering English-language articles published in peer-reviewed academic journals to safeguard the quality and effectiveness of the review. Due to variability in the peer-review process and their limited availability, book reviews, editorials, and papers from conference proceedings were excluded from this research. After the screening, a sample of 157 papers was obtained.
Afterwards, the full text of these papers was reviewed to assess eligible articles. As a result, 116 articles were excluded because their subject matter was not closely related to the topic of social media engagement metrics. In detail, papers were excluded when: 1) they mainly focused on social media engagement but superficially touched the metrics or 2) they mainly focused on metrics but superficially touched on social media engagement. In the end, 41 eligible articles were identified.
3.2 Analysis tools
The relevant data of the 41 documents in the final sample were saved and organised in a Microsoft Excel spreadsheet to include all the essential paper information such as paper title, authors’ names, and affiliations, abstract, keywords and references. Then, adopting the bibliometrics analysis method (Aria & Cuccurullo, 2017 ), the R-Tool ‘Biblioshiny for Bibliometrix’ was used to perform a comprehensive bibliometric analysis. Bibliometrix is a recent R-package that facilitates a more complete bibliometric analysis, employing specific tools for both bibliometric and scientometric quantitative research (Aria & Cuccurullo, 2017 ; Dervis, 2019 ; Jalal, 2019 ).
4 An overview of social media engagement metrics research.
The bibliometric analysis provided information on the 41 articles, allowing to highlight the significance of the topic.
4.1 Publication trend
The number of annual publications shows a rollercoaster trend (Fig. 2 ). Although the first relevant paper was published in 2013, only since 2016 publications begun to increase significantly with a slight decrease in 2018. This renders social media engagement metrics a relatively young research field.
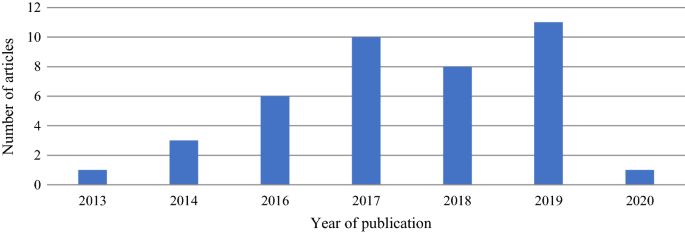
Timeline of the studies (January 2013- March 2020)
It is worth pointing out that the articles extraction was done in March 2020: this explains the low number of articles published in 2020.
4.2 Most relevant sources
When looking at the Journal sources overview, the analysis revealed 34 journals covering different fields, including marketing, management, economics, tourism and hospitality, engineering, communication, and technology. As shown in Fig. 3 , only four journals have more than two publications: Internet Research , Journal of Engineering and Applied Sciences , International Journal of Sports Marketing and Sponsorship. and Online Information Review .
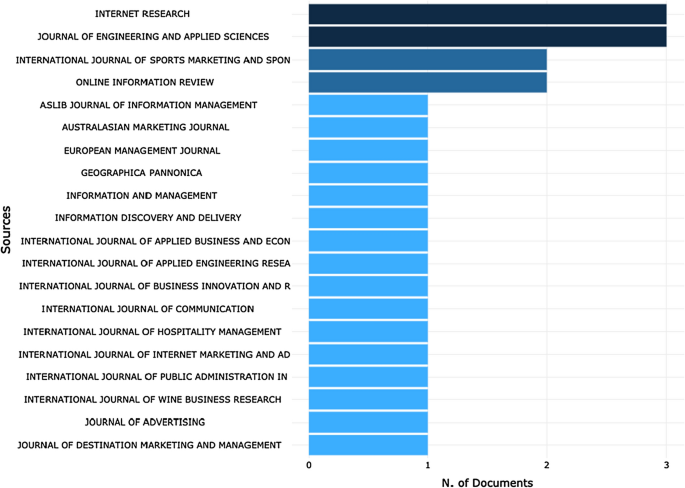
Most relevant sources
4.3 Seminal papers
Interesting findings emerged considering the most global cited documents that allow identifying the seminal articles in according to the timeliness, utility and quality, expressed by the scientific community (Okubo, 1997 ). The number of citations an article receives, and the studies cited in an article are two of the most popular bibliometric indicators used to determine the popularity of a publication.
Figure 4 shows the number of author citations for each article, identifying as seminal works: Malthouse’s (2013) paper ‘ Managing Customer Relationships in the Social Media Era: Introducing the Social CRM House’ with 278 global citations; Sabate’s (2014) paper ‘Factors influencing popularity of branded content in Facebook fan pages’ with 145 global citations; Mariani’s (2016) paper ‘ Facebook as a destination marketing tool: Evidence from Italian regional Destination Management Organizations ’ with 104 global citations; Oh’s (2017) paper ‘ Beyond likes and tweets: Consumer engagement behavior and movie box office in social media ’ with 54 global citations; Colicev’s (2018)’ Improving consumer mindset metrics and shareholder value through social media: The different roles of owned and earned media ’ with 39 global citations; Rossmann’s (2016) ‘ Drivers of user engagement in eWoM communication ’ with 35 global citations; Oviedo-Garcia’s (2014) ‘ Metric proposal for customer engagement in Facebook’ with 33 global citations .
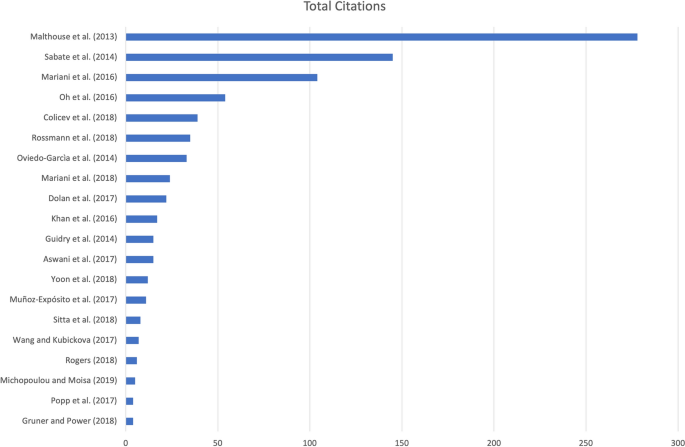
Most cited articles
The analysis of the papers reviewed revealed that the theme of social media engagement metrics turns out to be a hot topic and a newly emerging stream of research.
5 Social media engagement: areas of investigation
In recent years social media engagement has gained relevance in academic research, and many scholars have questioned its measurement, intensifying the academic debate with ever new contributions. Following previous studies, a comprehensive analysis allows framing the following categories of broad research subjects, used to conduct the subsequent systematic literature review (Fig. 5 ): (1) conceptualisation, (2) platforms, (3) measurement and (4) behaviours. All 41 articles were analysed according to the proposed scheme.
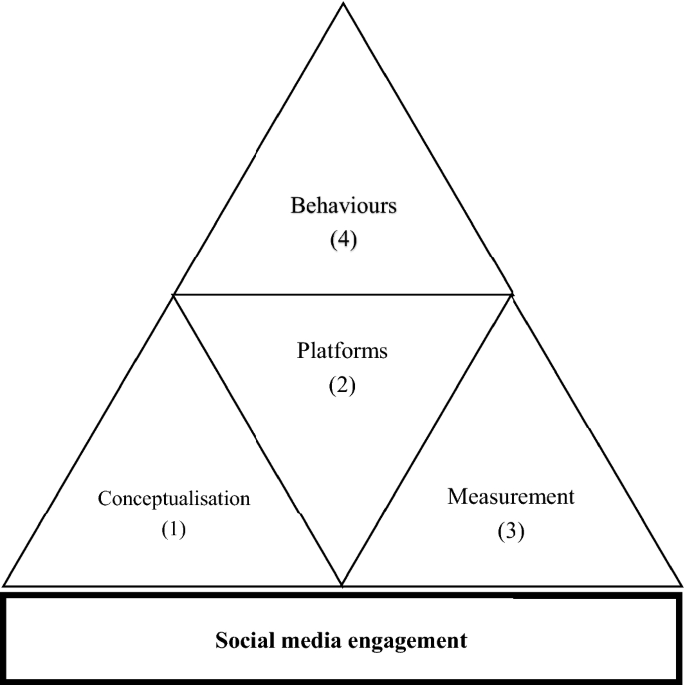
Areas of investigation
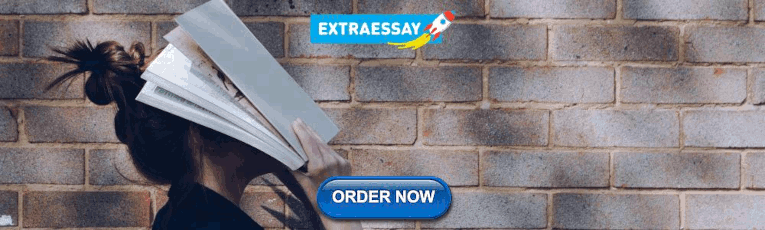
5.1 Investigating social media engagement
What emerges from the analysis of the 41 papers is that scholars used different approaches and methodologies to conceptualise and measure engagement in the digital context of social media.
As shown in Fig. 6 , most studies (66%) employ quantitative methodologies. For instance, Yoon et al. ( 2018 ) explored the relationship between digital engagement metrics and financial performance in terms of company revenue, confirming that customer engagement on a company’s Facebook fan page can influence revenue. Colicev et al. ( 2018 ) developed three social media metrics, including engagement, to study the effects of earned social media and owned social media on brand awareness, purchase intention, and customer satisfaction. In comparison, Wang and Kubickova ( 2017 ) examined factors affecting the engagement metrics of Facebook fan pages in the Northeast America hotel industry, factors such as time-of-day, day-of-week, age, gender and distance between the hotel and users’ origin of residence. They also analysed the impact of Facebook engagement on electronic word-of-mouth (eWOM), to better understand the importance of the engagement metrics within the hospitality context.
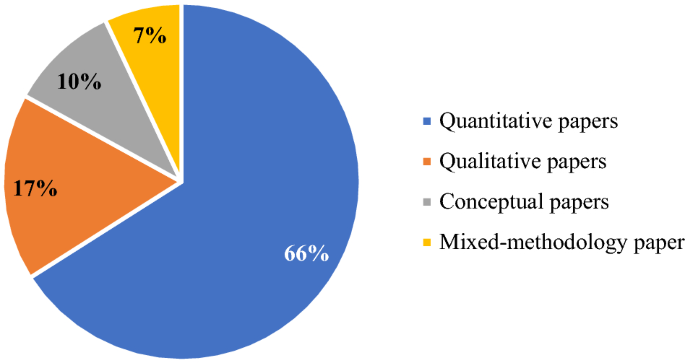
Classification of the 41 articles based on the methodology applied
From a qualitative point of view (17% of the papers), Hallock et al. ( 2019 ) used a case study approach to understand the firm perspective on social media engagement metrics, shedding light on how companies view engagement with social media as measurable metrics of customer interactions with the platform. Conversely, Michopoulou and Moisa ( 2019 ) used the same approach to investigate the use of social media marketing metrics and practices in the U.K. hotel industry.
Only a small part of the studies analysed (10% of the papers) explores social media engagement from a purely conceptual perspective. In this sense, Oviedo-Garcìa et al. ( 2014 ) and Muñoz-Expósito et al. ( 2017 ) directly identified social media engagement metrics for Facebook and Twitter, providing fascinating insights for scholars and practitioners.
Finally, among the papers analysed, only three studies (7% of the papers) use mixed methodologies to explore the phenomenon from qualitative and quantitative perspectives.
5.2 Defining social media engagement
Researchers identified 30 unique definitions of engagement applied to the social media context. Multiple definitions used several terms when defining engagement on social media. They were not singular and straightforward but were interspersed with various key terms and overlapping concepts, as presented in Table 3 .
The presence of synonymous terms directly addresses the lack of a standard definition and the challenges that this presents to researchers and practitioners in the field (Table 4 ).
As a relevant result, most authors focus on its behavioural manifestation (22% of the studies) resulting from motivational drivers when defining social media engagement. It is considered as the active behavioural efforts that both existing and potential customers exert toward online brand-related content (Yoon et al., 2018 ). It involves various activities that range from consuming content, participating in discussions, and interacting with other customers to digital buying (Oh et al., 2017 ; Yoon et al., 2018 ). Similarly, in addition to the behavioural manifestations, other scholars (12%) focus on the emotional connection expressed through the intensity of interactions and their implications, toward the offers and activities of a brand, product, or firm, regardless of whether it is initiated by the individual or by the firm (Muñoz-Expósito et al., 2017 ).
Shifting the observation lens from the customers to the firms, another group of scholars (10% of the studies) define social media engagement as the non-monetary return that derives from the online marketing strategies of brands (Khan, 2017 ; Medjani et al., 2019 ; Michopoulou & Moisa, 2019 ). In this case, engagement is viewed exclusively as a non-financial metric and as a measure of the performance of social media marketing activities.
Lastly, a small percentage of studies (10% of the studies) considers engagement as the number of people who acknowledge agreement or preference for content, who participate in creating, sharing and using content (Colicev et al., 2018 ; Li et al., 2019 ; Rahman et al., 2017 ).
5.3 Social Media Platforms
In a total of 41 articles reviewed, 85% of studies mention the platforms analysed, as shown in Table 5 . Facebook is the most popular platform analysed, followed by Twitter, YouTube, LinkedIn, and Instagram. These results were rather expected, given the fact that Facebook, with 2.6 billion monthly active users (Facebook, May 2020), is the most popular social media platform worldwide.
An interesting finding is that there are several articles (15% of the studies) which do not refer to a specific platform or that consider all the platforms together, when measuring social media engagement (e.g., Hallock et al., 2019 ; Medjani et al., 2019 ). This is interesting, given that each social network has different features that make the engagement measurement unique and not replicable.
5.4 Measuring social media engagement
The systematic literature review confirms that there is no theoretical certainty or solid consensus among scholars about measuring engagement on social media.
As can be seen from Table 6 , studies on social media engagement metrics can be grouped and classified into four macro-categories. The first group of studies, namely ‘quantitative metrics’, which is also the most numerous (66% of the studies), attempts to propose a simplistic assessment of the impact of social media engagement, based on the number of comments, likes, shares, followers etc. (Khan et al., 2019 ; Medjani et al., 2019 ; Yoon et al., 2018 ).
The second group of studies (17% of the studies), namely ‘normalised indexes’, provide a quantitative evaluation of the engagement a content generates in relation to the number of people to whom that content has been displayed. In this way, it is possible to obtain an average measure of the users’ engagement, dividing the total actions of interest by the total number of posts (Osokin, 2019 ; Zanini et al., 2019 ), the number of followers (Vlachvei & Kyparissi, 2017 ) or the number of people reached by a post (Muñoz-Expósito et al., 2017 ; Rossmann et al., 2016 ).
In a more complex and detailed way, studies from the third group (10% of the studies) identify social media engagement metrics developing ‘set of indexes’. For example, Li et al. ( 2019 ) use three social media metrics to measure engagement in the casual-dining restaurant setting: rates of conversation, amplification, and applause. In detail, conversation rate measures the number of comments or reviews in response to a post, amplification rate measures how much online content is shared, and applause rate measures the number of positive reactions on posts. Similarly, drawing from previous literature, Mariani et al. ( 2018 ) develop three social media metrics, namely generic engagement, brand engagement, and user engagement. Authors calculated these metrics by assessing different weights to different interaction actions, to emphasise the degree of users’ involvement implied by the underlying activities of respectively liking, sharing, or commenting.
Despite their great diffusion among academics and practitioners, some scholars (7% of the studies) argue that quantitative metrics are not enough to appreciate the real value of customer engagement on social media, and a qualitative approach is more suitable. For example, Abuljadail and Ha ( 2019 ) conducted an online survey of 576 Facebook users in Saudi Arabia to examine customer engagement on Facebook. Rogers ( 2018 ) critiques contemporary social media metrics considered ‘vanity metrics’ and repurpose alt metrics scores and other engagement measures for social research—namely dominant voice, concern, commitment, positioning, and alignment—to measure the ‘otherwise engaged’.
5.5 Social media engagement brand-related activities
When measuring social media engagement, scholars dealt with different social media actions that can be classified (Table 7 ) according to the three dimensions of the COBRA model (Consumer Online Brand Related Activities): consumption, contribution, or creation (Schivinski et al., 2016 ).
In a total of 41 articles reviewed, the most investigated dimension by researchers is contribution, i.e. when a customer comments, shares, likes a form of pre-existing brand content (e.g., Buffard et al., 2020 ; Khan et al., 2019 ). Its popularity among the studies may be due to its interactive nature of “liking” and “commenting”, which can be said to be the most common behaviour exhibited across social media platforms and often one of the most manageable interactions to obtain data. Additionally, studies that include creation in the measurement of social media engagement consider posting/publishing brand-related content, uploading brand-related video, pictures, audio or writing brand-related articles (e.g., Zanini et al., 2019 ). Among the sampled papers, the least investigated dimension of the COBRA model is consumption, considered by only seven studies (e.g., Colicev et al., 2018 ; Oh et al., 2017 ). It considers viewing brand-related audio, video, and pictures, following threads on online brand community forums or downloading branded widgets.
Dimensions have been investigated individually, for example, just considering the number of likes or comments (Khan et al., 2019 ; Yoon et al., 2018 ), or jointly using composite indicators, as in the case of Oviedo-Oviedo-García et al., 2014 ).
6 Discussion
This research presents fresh knowledge in the academic debate by providing an overarching picture of social media engagement, framing the phenomenon conceptually and offering a lens to interpret platforms and measuring tools. Conceptual and empirical studies tried to define, conceptualise, and measure social media engagement in diverse ways from different fields of research. They increased the gap between academia and managerial practice, where the topic of social media engagement metrics seems to be much more consolidated. The paper contributes to the academic debate on social media engagement, presenting continuity and discontinuity elements between different fields of enquiry. It also offers avenues for future research that both academics and marketers should explore. It also provides insights and guidance to practitioners on modelling and managing social media engagement.
6.1 Theoretical contribution
The article offers some theoretical contributions to this relatively young research field through the systematic literature review approach.
Firstly, the paper confirms the multidimensional and polysemic nature of engagement, even in the specific context of social media platforms, in continuity with the academic customer engagement research (Brodie et al., 2013 ; Hollebeek et al., 2016 ; So et al., 2016 ; Vivek et al., 2012 ). The concept of social media engagement can be traced back to three dimensions of analysis (Van Doorn, 2010 )—affective, cognitive, and behavioural—and some empirical studies measure it as such (Dessart, 2017 ; Vivek et al., 2014 ). However, the behavioural dimension is still the most used proxy to measure users’ level of engagement. Similarly, marketers and social media platforms have focused on behavioural interactions associated with likes, comments and sharing when reporting engagement metric (Peltier et al., 2020 ). What is worth pointing out is that emotional and cognitive dimensions are also essential components of social media engagement and should be adequately addressed by future research.
Secondly, strictly related to the first point, the paper suggests the COBRA model (Schivinski, 2016 ) as a conceptual tool to classify and interpret social media engagement from the behavioural perspective. Social media engagement can be manifested symbolically through actions (Barger et al., 2016 ; Oh et al., 2017 ; Van Doorn et al., 2010 ) that can be traced back to the three dimensions of consumption, contribution and creation (Schivinski et al., 2016 ). However, it is worth pointing out that not all these actions determine the same level of engagement. When measuring social media engagement, researchers should pay attention not only to ‘contribution’ but also to ‘consumption’ and ‘creation’, which are important indicators of the attention a post receives (Oviedo-Garcìa, 2014 ; Schivinski et al., 2016 ), giving them a different weight. It becomes even more important if considering that the same social networks provide different weights to users' actions. For example, in several countries, Instagram has tested removing the like feature on content posted by others, although users can still see the number of likes on their posts. YouTube has also decided to stop showing precise subscriber counts and Facebook is experimenting with hiding like counts, similar to Instagram.
Thirdly, the paper presents some of the key metrics used to evaluate social media engagement identifying quantitative metrics, normalised indexes, set of indexes and qualitative metrics. Although all indicators are based on the interaction between the user and the brand, as the literature suggests (Barger et al., 2016 ; Oviedo-Garcìa, 2014 ; Vivek et al., 2014 ), the paper argues that different metrics measure diverse aspects of social media engagement and should be used carefully by researchers. Despite the conceptual and qualitative research on the topic, even the most recent metrics offer measurements that do not allow engagement to be widely represented in its multidimensional and polysemic nature (Oviedo-García et al., 2014 ; Peltier et al., 2020 ). To get a deeper understanding of the construct, researchers should also consider some of the most recent advances in business practice. As an example, more and more practitioners have the chance to measure engagement by tracking the time spent on content and web pages to blend the different types of material, such as pictures, text, or even videos. Also, cursor movements, which are known to correlate with visual attention, and eye-tracking, can provide insights into the within-content engagement.
6.2 Managerial implications
Even if the topic of social media engagement seems to be more consolidated in business practice, this study also provides valuable implications for practitioners. Particularly, the findings shed light on the nature of social media engagement construct and on how metrics can be an extremely useful tool to evaluate, monitor, and interpret the effectiveness of social media strategies and campaigns.
This research offers a strategic-operational guide to the measurement of social media engagement, helping marketers understand what engagement is and choose the most effective and suitable KPIs to assess the performance and success of their marketing efforts. In this sense, marketers should accompany traditional metrics, such as likes, comments and shares, with new metrics capable of better capturing user behaviours.
Marketers also need to realise that engagement is a complex construct that goes beyond the simple behavioural dimension, encompassing cognitive and emotional traits. As a result, in some cases, the so-called “vanity metrics” could fail in fully representing all the aspects of social media engagement. In these cases, it should be accompanied by qualitative insights to analyse what users like to share or talk about and not merely look at likes, comments, and shares counts.
7 Limitations and future research
This research is not without limitations. First, the systematic literature review only includes English articles published in Journals. As social media engagement and engagement metrics are emerging research topics, conference proceedings and book chapters could also be included to deepen the understanding of the subject. Second, this research was conducted on the database Scopus of Elsevier for the keywords “social media engagement metrics”. Researchers could use a combination of different databases and keywords to search for new contributions and insights. Third, although the paper is based on a systematic literature review, this methodology reveals the subjectivity in the social sciences.
As this is a relatively young field of research, a further academic investigation is needed to overcome the limitations of the study and outline new scenarios and directions for future research. In addition, considering the growing importance of social media, there is value in broadening the analysis through additional studies. Future marketing research could use mixed approaches to integrate the three dimensions of social media engagement, linking qualitative and quantitative data. Advanced sentiment web mining techniques could be applied to allow researchers to analyse what users like to share or talk about and not merely look at likes, comments, and shares as the only metrics (Peltier et al., 2020 ).
Although Facebook and Twitter are the most used social network by brands, and the most significant part of the literature focuses on these two platforms, researchers should not forget that there are new and emerging social media in different countries (e.g., TikTok, Clubhouse). They already represent a hot topic for practitioners and are calling scholars to define new metrics to measure engagement. Additionally, as the use of social media increased during the COVID-19 pandemic, future research should take this into account to better understand social media engagement across different social media platforms.
Abuljadail, M., & Ha, L. (2019). Engagement and brand loyalty through social capital in social media. International Journal of Internet Marketing and Advertising, 13 (3), 197–217. https://doi.org/10.1504/IJIMA.2019.102557
Article Google Scholar
Advertising Research Foundation. (2006). Engagement: Definitions and Anatomy . ARF White Paper. https://thearf.org/ . Retrieved 5 May 2021
Aggrawal, N., & Arora, A. (2019). Behaviour of viewers: YouTube videos viewership analysis. International Journal of Business Innovation and Research, 20 (1), 106–128. https://doi.org/10.1504/IJBIR.2019.101692
Aria, M., & Cuccurullo, C. (2017). Bibliometrix: An R-tool for comprehensive science mapping analysis. Journal of Informetrics, 11 (4), 959–975. https://doi.org/10.1016/j.joi.2017.08.007
Aswani, R., Ghrera, S. P., Kar, A. K., & Chandra, S. (2017). Identifying buzz in social media: A hybrid approach using artificial bee colony and k-nearest neighbors for outlier detection. Social Network Analysis and Mining, 7 (1), 1–10. https://doi.org/10.1007/s13278-017-0461-2
Aveyard, H. (2007). Doing a Literature Review in Health and Social Care: A practical guide . Pennsylvania Plaza New York: McGrow Hill.
Google Scholar
Barger, V., Peltier, J. W., & Schultz, D. E. (2016). Social media and consumer engagement: A review and research agenda. Journal of Research in Interactive Marketing, 10 (4), 268–287. https://doi.org/10.1108/JRIM-06-2016-0065
Bowden, J. (2009). The process of customer engagement: A conceptual framework. Journal of Marketing Theory and Practice, 17 (1), 63–74.
Brodie, R. J., Hollebeek, L. D., Jurić, B., & Ilić, A. (2011). Customer engagement: Conceptual domain, fundamental propositions, and implications for research. Journal of Service Research, 14 (3), 252–271. https://doi.org/10.2753/MTP1069-6679170105
Brodie, R. J., Ilic, A., Juric, B., & Hollebeek, L. (2013). Consumer engagement in a virtual brand community: An exploratory analysis. Journal of Business Research, 66 (1), 105–114. https://doi.org/10.1016/j.jbusres.2011.07.029
Buffard, J., & Papasava, A. (2020). A quantitative study on the impact of emotion on social media engagement and conversion. Journal of Digital and Social Media Marketing, 7 (4), 355–375.
Colicev, A., Malshe, A., Pauwels, K., & O’Connor, P. (2018). Improving consumer mindset metrics and shareholder value through social media: The different roles of owned and earned media. Journal of Marketing, 82 (1), 37–56. https://doi.org/10.1509/jm.16.0055
De Vries, N. J., & Carlson, J. (2014). Examining the drivers and brand performance implications of customer engagement with brands in the social media environment. Journal of Brand Management, 21 (6), 495–515. https://doi.org/10.1057/bm.2014.18
Dervis, H. (2019). Bibliometric analysis using bibliometrix an R package. Journal of Scientometric Research, 8 (3), 156–160. https://doi.org/10.5530/jscires.8.3.32
Dessart, L. (2017). Social media engagement: A model of antecedents and relational outcomes. Journal of Marketing Management, 33 (5–6), 375–399. https://doi.org/10.1080/0267257X.2017.1302975
Dolan, R., Conduit, J., Fahy, J., & Goodman, S. (2017). Social media: Communication strategies, engagement and future research directions. International Journal of Wine Business Research, 29 (1), 2–19. https://doi.org/10.1108/IJWBR-04-2016-0013
Forrester Consulting. (2008). How engaged are your customers? . Forrester Consuting. http://docplayer.net/9663683-How-engaged-are-your-customers.html . Retrieved 5 May 2021
Gallup Consulting. (2009). Customer engagement: What’s your engagement ratio? . Gallup Consulting. https://strengthszone.com/wp-content/uploads/2016/01/Customer-Engagement-Ratio-Brochure.pdf . Retrieved 5 May 2021
Gruner, R. L., & Power, D. (2018). To integrate or not to integrate? Understanding B2B social media communications. Online Information Review, 42 (1), 73–92. https://doi.org/10.1108/OIR-04-2016-0116
Guidry, J. P. D., Waters, R. D., & Saxton, G. D. (2014). Moving social marketing beyond personal change to social change: Strategically using Twitter to mobilize supporters into vocal advocates. Journal of Social Marketing, 4 (3), 240–260. https://doi.org/10.1108/JSOCM-02-2014-0014
Hallock, W., Roggeveen, A. L., & Crittenden, V. (2019). Firm-level perspectives on social media engagement: An exploratory study. Qualitative Market Research, 22 (2), 217–226. https://doi.org/10.1108/QMR-01-2017-0025
Harrigan, P., Evers, U., Miles, M., & Daly, T. (2017). Customer engagement with tourism social media brands. Tourism Management, 59 , 597–609. https://doi.org/10.1016/j.tourman.2016.09.015
Haumann, T., Güntürkün, P., & Schons, L. M. (2015). Engaging customers in coproduction processes: How value-enhancing and intensity-reducing communication strategies mitigate the negative effects of coproduction intensity. Journal of Marketing, 79 (6), 17–33. https://doi.org/10.1509/jm.14.0357
Hollebeek, L. D. (2018). Individual-level cultural consumer engagement styles: Conceptualization, propositions and implications. International Marketing Review , 35 , 42–71.
Hollebeek, L. D. (2019). Developing business customer engagement through social media engagement-platforms: An integrative S-D logic/RBV-informed model. Industrial Marketing Management, 81 , 89–98. https://doi.org/10.1016/j.indmarman.2017.11.016
Hollebeek, L. D., Conduit, J., & Brodie, R. J. (2016). Strategic drivers, anticipated and unanticipated outcomes of customer engagement. Journal of Marketing Management, 32 (5–6), 393–398. https://doi.org/10.1080/0267257X.2016.1144360
Hollebeek, L. D., Glynn, M. S., & Brodie, R. J. (2014). Consumer brand engagement in social media: Conceptualization, scale development and validation. Journal of Interactive Marketing, 28 (2), 149–165. https://doi.org/10.1016/j.intmar.2013.12.002
Hollebeek, L. D., Srivastava, R. K., & Chen, T. (2019). S-D logic–informed customer engagement: Integrative framework, revised fundamental propositions, and application to CRM. Journal of the Academy of Marketing Science, 47 (1), 161–185. https://doi.org/10.1007/s11747-016-0494-5
Hubspot. (2014). CRM expert Paul Greenberg defines customer engagement . Hubspot. https://blog.hubspot.com/sales/paul-greenberg-defines-customer-engagement . Retrieved 5 May 2021
Inamdar, Z., Raut, R., Narwane, V. S., Gardas, B., Narkhede, B., & Sagnak, M. (2020). A systematic literature review with bibliometric analysis of big data analytics adoption from period 2014 to 2018. Journal of Enterprise Information Management, 34 (1), 101–139. https://doi.org/10.1108/JEIM-09-2019-0267
Jaakkola, E., & Alexander, M. (2014). The role of customer engagement behavior in value co-creation: A service system perspective. Journal of Service Research, 17 (3), 247–261. https://doi.org/10.1177/1094670514529187
Jalal, S. K. (2019). Co-authorship and co-occurrences analysis using bibliometrix r-package: A case study of india and bangladesh. Annals of Library and Information Studies, 66 (2), 57–64.
Kalinić, Č, & Vujičić, M. (2019). A subnational assessment of hotel social media metrics - The case of Serbia. Geographica Pannonica, 23 (2), 87–101.
Khan, G., Mohaisen, M., & Trier, M. (2019). The network ROI: Concept, metrics, and measurement of social media returns (a Facebook experiment). Internet Research, 30 (2), 631–652. https://doi.org/10.1108/INTR-07-2018-0346
Khan, I., Dongping, H., & Wahab, A. (2016). Does culture matter in effectiveness of social media marketing strategy? An investigation of brand fan pages. Aslib Journal of Information Management, 68 (6), 694–715. https://doi.org/10.1108/AJIM-03-2016-0035
Khan, M. L. (2017). Social media engagement: What motivates user participation and consumption on YouTube? Computers in Human Behavior, 66 , 236–247. https://doi.org/10.1016/j.chb.2016.09.024
Kumar, V., Aksoy, L., Donkers, B., Venkatesan, R., Wiesel, T., & Tillmanns, S. (2010). Undervalued or overvalued customers: Capturing total customer engagement value. Journal of Service Research, 13 (3), 297–310. https://doi.org/10.1177/1094670510375602
Kumar, V., Rajan, B., Gupta, S., & Dalla Pozza, I. (2019). Customer engagement in service. Journal of the Academy of Marketing Science, 47 (1), 138–160. https://doi.org/10.1007/s11747-017-0565-2
Le, T. D. (2018). Influence of WOM and content type on online engagement in consumption communities : The information flow from discussion forums to Facebook. Online Information Review, 42 (2), 161–175. https://doi.org/10.1108/OIR-09-2016-0246
Li, J., Kim, W. G., & Choi, H. M. (2019). Effectiveness of social media marketing on enhancing performance: Evidence from a casual-dining restaurant setting. Tourism Economics, 20 (10), 1–20. https://doi.org/10.1177/1354816619867807
Li, X., Wu, P., Shen, G. Q., Wang, X., & Teng, Y. (2017). Mapping the knowledge domains of building information modeling (BIM): A bibliometric approach. Automation in Construction, 84 , 195–206. https://doi.org/10.1016/j.autcon.2017.09.011
Linnenluecke, M. K., Marrone, M., & Singh, A. K. (2020). Conducting systematic literature reviews and bibliometric analyses. Australian Journal of Management, 45 (2), 175–194. https://doi.org/10.1177/0312896219877678
Liu, X., Shin, H., & Burns, A. C. (2019). Examining the impact of luxury brand’s social media marketing on customer engagement: Using big data analytics and natural language processing. Journal of Business Research . https://doi.org/10.1016/j.jbusres.2019.04.042
Loureiro, S. M. C., Gorgus, T., & Kaufmann, H. R. (2017). Antecedents and outcomes of online brand engagement: The role of brand love on enhancing electronic-word-of-mouth. Online Information Review, 41 (7), 985–1005. https://doi.org/10.1108/OIR-08-2016-0236
Malthouse, E. C., Haenlein, M., Skiera, B., Wege, E., & Zhang, M. (2013). Managing customer relationships in the social media era: Introducing the social CRM house. Journal of Interactive Marketing, 27 (4), 270–280. https://doi.org/10.1016/j.intmar.2013.09.008
Mariani, M. M., Di Felice, M., & Mura, M. (2016). Facebook as a destination marketing tool: Evidence from Italian regional destination management organizations. Tourism Management, 54 , 321–343. https://doi.org/10.1016/j.tourman.2015.12.008
Mariani, M. M., Mura, M., & Di Felice, M. (2018). The determinants of Facebook social engagement for national tourism organizations’ Facebook pages: A quantitative approach. Journal of Destination Marketing and Management, 8 , 312–325. https://doi.org/10.1016/j.jdmm.2017.06.003
Marketing Science Institute. (2020). Research priorities 2020–2022 . Marketing Science Institute. https://www.msi.org/wp-content/uploads/2020/06/MSI_RP20-22.pdf . Retrieved 5 May 2021
McCoy, C. G., Nelson, M. L., & Weigle, M. C. (2018). Mining the Web to approximate university rankings. Information Discovery and Delivery, 46 (3), 173–183. https://doi.org/10.1108/IDD-05-2018-0014
McKinsey. (2012). Demystifyng social media . McKinsey. https://www.mckinsey.com/business-functions/marketing-and-sales/our-insights/demystifying-social-media . Retrieved 5 May 2021
Medjani, F., Rutter, R., & Nadeau, J. (2019). Social media management, objectification and measurement in an emerging market. Business and Emerging Markets, 11 (3), 288–311. https://doi.org/10.1504/IJBEM.2019.102654
Michopoulou, E., & Moisa, D. G. (2019). Hotel social media metrics: The ROI dilemma. International Journal of Hospitality Management, 76 , 308–315. https://doi.org/10.1016/j.ijhm.2018.05.019
Muñoz-Expósito, M., Oviedo-García, M. Á., & Castellanos-Verdugo, M. (2017). How to measure engagement in Twitter: Advancing a metric. Internet Research, 27 (5), 1122–1148. https://doi.org/10.1108/IntR-06-2016-0170
Muntinga, D. G., Moorman, M., & Smit, E. G. (2011). Introducing COBRAs: Exploring motivations for brand-related social media use. International Journal of Advertising, 30 (1), 13–46. https://doi.org/10.2501/IJA-30-1-013-046
Oh, C., Roumani, Y., Nwankpa, J. K., & Hu, H. F. (2017). Beyond likes and tweets: Consumer engagement behavior and movie box office in social media. Information and Management, 54 (1), 25–37. https://doi.org/10.1016/j.im.2016.03.004
Okubo, Y. (1997). Bibliometric indicators and analysis of research systems: methods and examples . Paris: OECD Publishing.
Osokin, N. (2019). User engagement and gratifications of NSO supporters on Facebook: Evidence from European football. International Journal of Sports Marketing and Sponsorship, 20 (1), 61–80. https://doi.org/10.1108/IJSMS-11-2017-0115
Oviedo-García, M. Á., Muñoz-Expósito, M., Castellanos-Verdugo, M., & Sancho-Mejías, M. (2014). Metric proposal for customer engagement in Facebook. Journal of Research in Interactive Marketing, 8 (4), 327–344. https://doi.org/10.1108/JRIM-05-2014-0028
Peltier, J., Dahl, A. J., & VanderShee, B. A. (2020). Antecedent consumer factors, consequential branding outcomes and measures of online consumer engagement: Current research and future directions. Journal of Research in Interactive Marketing, 14 (2), 239–268. https://doi.org/10.1108/JRIM-01-2020-0010
Pencarelli, T., & Mele, G. (2019). A systematic literature review on social media metrics. Mercati & Competitività, 1 , 1–24.
Phulwani, P. R., Kumar, D., & Goyal, P. (2020). A Systematic Literature Review and Bibliometric Analysis of Recycling Behavior. Journal of Global Marketing, 33 (5), 354–376. https://doi.org/10.1080/08911762.2020.1765444
Pickering, C., & Byrne, J. (2014). The benefits of publishing systematic quantitative literature reviews for PhD candidates and other early-career researchers. Higher Education Research & Development, 33 (3), 534–548
Popp, N., McEvoy, C., & Watanabe, N. (2017). Do college athletics marketers convert social media growth into ticket sales? International Journal of Sports Marketing and Sponsorship, 18 (2), 212–227. https://doi.org/10.1108/IJSMS-05-2017-090
Rahman, Z., Suberamanian, K., Zanuddin, H., Moghavvemi, S., & Bin MdNasir, M. H. N. (2016). SNS metrics analysis “A study on fanpage interactive contents.” International Journal of Applied Business and Economic Research, 14 (2), 1405–1415.
Rahman, Z., Suberamanian, K., Zanuddin, H., Moghavvemi, S., & Nasir, M. H. N. M. (2017). Fanpage viral metrics analysis “study on frequently posted contents.” Journal of Engineering and Applied Sciences, 12 (16), 4039–4046.
Rather, R. A., Hollebeek, L. D., & Islam, J. U. (2019). Tourism-based customer engagement: The construct, antecedents, and consequences. The Service Industries Journal, 39 (7–8), 519–540. https://doi.org/10.1080/02642069.2019.1570154
Rietveld, R., Van Dolen, W., Mazloom, M., & Worring, M. (2020). What you feel, is what you like influence of message appeals on customer engagement on Instagram. Journal of Interactive Marketing, 49 , 20–53. https://doi.org/10.1016/j.intmar.2019.06.003
Rogers, R. (2018). Digital traces in context| Otherwise engaged: Social media from vanity metrics to critical analytics. International Journal of Communication, 12 (23), 450–472.
Rossmann, A., Ranjan, K. R., & Sugathan, P. (2016). Drivers of user engagement in eWoM communication. Journal of Services Marketing, 30 (5), 541–553. https://doi.org/10.1108/JSM-01-2015-0013
Sabate, F., Berbegal-Mirabent, J., Cañabate, A., & Lebherz, P. R. (2014). Factors influencing popularity of branded content in Facebook fan pages. European Management Journal, 32 (6), 1001–1011. https://doi.org/10.1016/j.emj.2014.05.001
Schivinski, B., Christodoulides, G., & Dabrowski, D. (2016). Measuring consumers’ engagement with brand-related social-media content: Development and validation of a scale that identifies levels of social-media engagement with brands. Journal of Advertising Research, 56 (1), 64–80. https://doi.org/10.2501/JAR-2016-004
Segijn, C. M., Maslowska, E., Araujo, T., & Viswanathan, V. (2019). Engaging with TV events on Twitter: The interrelations between TV consumption, engagement actors, and engagement content. Internet Research, 30 (2), 381–401. https://doi.org/10.1108/INTR-08-2018-0389
Sitta, D., Faulkner, M., & Stern, P. (2018). What can the brand manager expect from Facebook? Australasian Marketing Journal, 26 (1), 17–22. https://doi.org/10.1016/j.ausmj.2018.01.001
So, K. K. F., King, C., Sparks, B. A., & Wang, Y. (2016). Enhancing customer relationships with retail service brands: The role of customer engagement. Journal of Service Management, 27 (2), 170–193. https://doi.org/10.1108/JOSM-05-2015-0176
Tranfield, D., Denyer, D., & Smart, P. (2003). Towards a methodology for developing evidence-informed management knowledge by means of systematic review. British Journal of Management, 14 (3), 207–222. https://doi.org/10.1111/1467-8551.00375
Trunfio, M., & Della Lucia, M. (2019). Engaging destination stakeholders in the digital era: The best practice of italian regional DMOs. Journal of Hospitality and Tourism Research, 43 (3), 349–373. https://doi.org/10.1177/1096348018807293
Van Doorn, J., Lemon, K. N., Mittal, V., Nass, S., Pick, D., Pirner, P., & Verhoef, P. C. (2010). Customer engagement behavior: Theoretical foundations and research directions. Journal of Service Research, 13 (3), 253–266. https://doi.org/10.1177/1094670510375599
Vivek, S. D., Beatty, S. E., Dalela, V., & Morgan, R. M. (2014). A generalized multidimensional scale for measuring customer engagement. Journal of Marketing Theory and Practice , 22 (4), 401–420.
Vivek, S. D., Beatty, S. E., & Morgan, R. M. (2012). Customer engagement: Exploring customer relationships beyond purchase. Journal of Marketing Theory and Practice, 20 (2), 122–146. https://doi.org/10.2753/MTP1069-6679200201
Vlachvei, A., & Kyparissis, A. (2017). Museums on Facebook wall: A case staudy of Thessaloniki’s museums. Tourismos, 12 (3), 75–96.
Vrettos, K., & Gouscos, D. (2019). Evaluating the presence of Greek tourism-related public sector entities in online social networks. International Journal of Public Administration in the Digital Age, 6 (1), 15–40.
Wallace, M., & Wray, A. (2016). Critical reading and writing for postgraduates . Sage.
Wang, C., & Kubickova, M. (2017). The impact of engaged users on eWOM of hotel Facebook page. Journal of Hospitality and Tourism Technology, 8 (2), 190–204. https://doi.org/10.1108/JHTT-09-2016-0056
Wu, J., Fan, S., & Zhao, J. L. (2018). Community engagement and online word of mouth: An empirical investigation. Information & Management, 55 (2), 258–270. https://doi.org/10.1016/j.im.2017.07.002Get
Yoon, G., Li, C., Ji, Y., North, M., Hong, C., & Liu, J. (2018). Attracting comments: digital engagement metrics on facebook and financial performance. Journal of Advertising, 47 (1), 24–37. https://doi.org/10.1080/00913367.2017.1405753
Zanini, M. T., Carbone de Moraes, F., Lima, V., Migueles, C., Lourenco, C., & Reis Irigaray, H. A. (2019). Soccer and twitter: Virtual brand community engagement practices. Marketing Intelligence and Planning, 37 (7), 791–805. https://doi.org/10.1108/MIP-08-2018-0371
Download references
Open access funding provided by Università Parthenope di Napoli within the CRUI-CARE Agreement.
Author information
Authors and affiliations.
Department of Management and Quantitative Studies, University of Naples “Parthenope”, Naples, Italy
Mariapina Trunfio & Simona Rossi
You can also search for this author in PubMed Google Scholar
Corresponding author
Correspondence to Simona Rossi .
Additional information
Publisher's note.
Springer Nature remains neutral with regard to jurisdictional claims in published maps and institutional affiliations.
Rights and permissions
Open Access This article is licensed under a Creative Commons Attribution 4.0 International License, which permits use, sharing, adaptation, distribution and reproduction in any medium or format, as long as you give appropriate credit to the original author(s) and the source, provide a link to the Creative Commons licence, and indicate if changes were made. The images or other third party material in this article are included in the article's Creative Commons licence, unless indicated otherwise in a credit line to the material. If material is not included in the article's Creative Commons licence and your intended use is not permitted by statutory regulation or exceeds the permitted use, you will need to obtain permission directly from the copyright holder. To view a copy of this licence, visit http://creativecommons.org/licenses/by/4.0/ .
Reprints and permissions
About this article
Trunfio, M., Rossi, S. Conceptualising and measuring social media engagement: A systematic literature review. Ital. J. Mark. 2021 , 267–292 (2021). https://doi.org/10.1007/s43039-021-00035-8
Download citation
Received : 12 November 2020
Accepted : 29 July 2021
Published : 11 August 2021
Issue Date : September 2021
DOI : https://doi.org/10.1007/s43039-021-00035-8
Share this article
Anyone you share the following link with will be able to read this content:
Sorry, a shareable link is not currently available for this article.
Provided by the Springer Nature SharedIt content-sharing initiative
- Customer engagement
- Social media engagement
- Social media platforms
- Qualitative metrics
- Quantitative metrics
- Social media metrics
- COBRA model
- Find a journal
- Publish with us
- Track your research

An official website of the United States government
The .gov means it’s official. Federal government websites often end in .gov or .mil. Before sharing sensitive information, make sure you’re on a federal government site.
The site is secure. The https:// ensures that you are connecting to the official website and that any information you provide is encrypted and transmitted securely.
- Publications
- Account settings
Preview improvements coming to the PMC website in October 2024. Learn More or Try it out now .
- Advanced Search
- Journal List
- Springer Nature - PMC COVID-19 Collection

A systematic literature review of how and whether social media data can complement traditional survey data to study public opinion
Maud reveilhac.
1 Lausanne University (Switzerland), Faculty of Social and Political Sciences, Institute of Social Sciences, Life Course and Social Inequality Research Centre, Lausanne, Switzerland
Stephanie Steinmetz
Davide morselli.
2 Swiss Centre of Expertise in Life Course Research LIVES, Lausanne, Switzerland
Associated Data
Please refer to the appendix.
In this article, we review existing research on the complementarity of social media data and survey data for the study of public opinion. We start by situating our review in the extensive literature (N = 187) about the uses, challenges, and frameworks related to the use of social media for studying public opinion. Based on 187 relevant articles (141 empirical and 46 theoretical) - we identify within the 141 empircal ones six main research approaches concerning the complementarity of both data sources. Results show that the biggest share of the research has focused on how social media can be used to confirm survey findings, especially for election predictions. The main contribution of our review is to detail and classify other growing complementarity approaches, such as comparing both data sources on a given phenomenon, using survey measures as a proxy in social media research, enriching surveys with SMD, recruiting individuals on social media to conduct a second survey phase, and generating new insight on “old” or “under-investigated” topics or theories using SMD. We discuss the advantages and disadvantages associated with each of these approaches in relation to four main research purposes, namely the improvement of validity, sustainability, reliability, and interpretability. We conclude by discussing some limitations of our study and highlighting future paths for research.
Introduction
This paper provides a systematic literature review of how social media data (SMD) and traditional survey data have been used complementarily to study public opinion (PO) over the last decade. As social media users represent more than half of the world’s population (see [ 26 ]) and provide continuous reactions to daily socio-political events, it is not surprising that traditional survey research has been concerned about whether such data would make surveys obsolete or whether they could be used complementarily. Addressing these questions is particularly relevant in the area of PO. Social media plays a growing role in the formation of PO as user-generated content on these platforms is increasingly deployed as representations of PO (e.g. [ 27 , 56 ]). In addition, politicians increasingly consider social media, especially Twitter, to be a “barometer” of PO [ 44 ].
Despite the extensive literature about the benefits and challenges of using SMD to answer social and political questions, as well as about SMD as a possible replacement for traditional surveys, a comprehensive overview of the complementarity of both data sources remains limited. The aim of this paper is to fill this gap by providing a systematic literature review focusing on how SMD and survey data can complement each other to study PO. Inspired by the influential study of Japec et al. [ 45 ] which elaborated on the complementarity of survey data and “big data” (rather broadly defined), we want to concentrate, however, on one type of “big data”, namely SMD. There are two main reasons for this choice. First, SMD are a specific type of “non-survey” data which possess specific arrangements (or conventions) and paradata that are different from other types of administrative or “big data”, especially when it come to the assessment of PO. Second, whereas there is substantial research on augmenting survey data with administrative (e.g. electricity or water consumption) or other type of “web data” (e.g. Google searches or citation metrics) to improve estimates of PO or official statistics, we still lack an overarching picture of the (new) developments and approaches of complementing SMD and surveys with each other.
Our analysis is based on an extensive survey of the literature capturing a representative sample of the best published theoretical and empirical scientific papers on the topic (N = 187). We have restricted the analytical period to the last decade (2010–2020) as the discussion on complementarity is still a young field of study (e.g. [ 58 ]). On this basis, we have been able to identify six complementarity approaches which can be synthesised to four major purposes, namely predicting, substituting, comparing, and linking SMD and survey data.
In the next section, we situate our review within the existing literature by demonstrating how the scientific discussion surrounding the opportunities and challenges offered by SMD within survey research has evolved, especially by highlighting the complementary understanding of PO offered by both data sources. Then, we discuss more specifically which research approaches have emerged, and we classify them according to four main research purposes using both data sources complementarily. The analysis of the empirical studies aims to act as a guide for other researchers by identifying research gaps and highlighting the pros and cons of each approach. Furthermore, we underline areas for future improvements and point to technical and ethical considerations. We conclude by mentioning the main contributions and limitations of our review.
Background – The complementary understandings of PO
Surveys have long been the most predictive and accurate tools for collecting and measuring opinion. However, over the last decade, decreasing response rates have called into question the potential of using a random sample of individuals to represent an entire population (e.g. [ 37 , 49 ]), thus posing important concerns about the sustainability of survey research. Even by adapting to new modes, such as push-to-web, to increase response rates, it remains unclear whether surveys will maintain this dominant role as communication habits continue to change (e.g. [ 68 ]). Given the recent “survey crisis” (e.g. [ 13 , 22 ]), an increasingly rich source of PO data is commonly referred to as “big data”. These “new” data take the form of extraordinarily large and complex datasets. There are three attributes that are generally agreed upon to describe this type of data (e.g. [ 19 ]), namely volume, velocity, and variety. Social media are a sub-type of big data where people express their thoughts and opinions with the purpose of sharing them with others [ 18 ]. Due to their inherent properties, SMD have been seen as a promising complementary, and even alternative, source of data for exploring PO. However, researchers acknowledged early on that, almost universally, SMD are non-random, and thus discouraged using them as a means of making generalisable claims. This challenge is well highlighted by Schober et al. [ 68 ], who claim that, while the social media researcher seeks to achieve topic coverage, the survey researcher emphasises population coverage as a central endeavour.
An entire strand of research thus focussed on how surveys and social media differ in several aspects. Table Table1 1 attempts to classify the most prominent differences along which SMD and survey data are typically compared. We have identified several dimensions based on recurring criteria mentioned in the literature concerning the nature of and the relationship between both data sources. Often-cited criteria include the type of population and data signal, the unit of observation and analysis, and the available meta-data (for a thorough discussion of the differences see [ 18 , 68 ], and [ 77 ]).
Differences between SMD and survey data to study PO
To understand how to best use both data sources complementarily, it is also essential to reflect on how they construct PO differently. This is increasingly important, as what constitutes “the public” tends to be forged by the methods and data from which it is derived [ 56 ]. In survey research, PO is equivalent to the private opinion of a representative public, operationalised as a set of positions on a given topic. PO can thus be conceptualised as a reflection of a shared position among citizens on specific issues that are then amplified and reviewed by news media and political actors [ 42 ]. Survey measures of PO are constrained by the scope of the questionnaires, which usually provide little room for spontaneous expressions of opinion (except in open-ended survey questions). The diversity of opinions is thereby reduced into a set of discrete and aggregate data (e.g. [ 75 ]). Conversely, the reliance on social media for measuring PO expands the societal and collective components of opinions [ 59 ] by conceptualising it in Habermas’ [ 39 ] terms as a complex system of representations. In this respect, SMD are better suited to capturing the conversational and relational nature of PO formation [ 3 ]. Hence, where survey data weigh precision and standardisation, SMD excel in multidimensionality and polyphony. In addition to their focus on solicited private opinions, surveys are also less reactive to opinion changes than SMD. In theory, opinion changes could be assessed by frequent short opinion surveys (e.g. every two months). However, the advantage of SMD is that they can cover opinion change more rapidly (and on an ad hoc basis), thus reacting faster to events, which is almost impossible for surveys (e.g. it takes more time to set up probability-based surveys for the study of COVID compared to what can be done with SMD).
Despite the advantages offered by social media for measuring more social and timelier opinions, the reliance on SMD raises important questions for empirical research on (automated) measurements of opinions and on the choice of the indicators employed to model opinions. Indeed, constructing measures of PO based on SMD can be very time consuming and can involve a lot of pre-processing effort before the data can be translated into meaningful measures of expressed opinions. Furthermore, it sometimes remains quite difficult to know what is driving the evolution of ideas and concerns found in online conversations. Consequently, a current strand of research seeks to better understand the issues of representativeness of social media communities and the validity of measured opinion, especially opinions stemming from sentiment analysis. While there is a rising interest in applying SMD to understand opinion, and even to replace traditional surveys (e.g. [ 3 , 32 ]), SMD alone are of limited use for social scientific research as they usually provide incomplete and imprecise information. However, the issues associated with SMD are not necessarily fatal to the proposition that they can be used to generate social insights, especially in complementing survey data. An efficient strategy to enhance research lies, therefore, in the analysis of how both data sources can complement each other in ways that maximise their strengths.
In the next sections, we aim to show that there is a plethora of research practices in which both data sources complement each other for the study of PO. To date, however, there is still no consensus about the best way to use SMD for studying PO [ 58 ]. We are now at a point where we should reflect on what has been done so far, what lessons we can learn from it, and then specify suitable trends for social research. In this paper, we seek to fill this gap by reviewing research that uses both data sources complementarily for the purposes of measuring PO and by providing a critical evaluation of the identified research paths.
Method of analysis: Building a corpus of relevant articles
To build our corpus of scientific articles, we carried out several searches in bibliographic databases (focusing on Scopus and Google Scholar ) using the software PublishOrPerish [ 40 ]. We obtained an initial corpus of 3596 unique papers, which we reduced to papers that were relevant for the scope of our review. The initial corpus was deliberately based on a search-query that was broad enough to collect the relevant literature, while not missing important papers. We used the query “(social media OR twitter OR facebook OR instagram OR reddit) AND (survey OR surveys OR polls)” and specified that it should appear in the body of the text (using the keyword field) instead of appearing only in the title or abstract, which were found to be too restrictive to capture the literature of interest. The query was designed to restrict the focus of our review to SMD, thus ignoring other types of “big data” or “digital trace” data.
A first filter was applied to reduce the number of papers to journal articles, book chapters, and scientific reports (thus excluding books, theses, and conference papers) as we wanted to concentrate on high-valued scientific sources which have already been approved by the scientific community. In this respect, including conference papers would have drastically inflated the number of (duplicated) papers concerned with predictions and with replicating previous studies using alternative methods of analysis and algorithms. Among the remaining papers, we applied two eligibility criteria to disregard those that were not pertinent to the analysis as i) their focus was not on PO, ii) they were oriented towards a specific aspect of data treatment (e.g. estimating socio-demographics from texts or profile pictures) or an analytical strategy (e.g. elaborating algorithms). We also excluded articles mentioning survey findings without an explicit aim of supplementing, comparing, or combining those with SMD.
Results of the literature review on the uses of social media as a complement to surveys
Overall, the collection protocol left us with 187 papers - 141 of an empirical and 46 of a theoretical nature (these papers can be found in the Appendix). Most of these papers stem from political communication and computational social sciences journals. Although the sample of 187 papers may not cover the whole corpus of research on the subject, it is nonetheless sufficient to highlight the main research directions that have been endorsed on the topic of complementarity. Figure Figure1 1 provides an overview of the yearly repartition of the retrieved papers differentiating between those with a theoretical (N = 46) and an empirical (N = 141) focus. While the number of theoretical papers remains stable over the years, we can see a steady increase in empirical papers over time.

Number of empirical and theoretical articles according to our meta-review of the existing literature using surveys and SMD
Theoretical insights
Starting with the theoretical papers in our review (N = 46, see Table Table2 2 in the Appendix), survey and social media researchers have explored ways in which social media and survey data can yield congruent conclusions (e.g. [ 68 ]). One part of these articles (n = 14) tries to establish a framework regarding the predictive power of SMD as a potential substitute for surveys. This line of research stems principally from the fields of election and economy forecasting (for recent reviews see [ 15 , 66 ]).
List of theoretical papers focusing on the combination of survey and social media data (n = 46, continues next pages)
Another strand of theoretical articles (n = 14) focuses instead on the compliance of social media research with established reporting standards so as to guarantee transparency and replicability (e.g. [ 51 ]). Finding ways of integrating data obtained from different sources (n = 3) also constitutes a fertile path of research [ 46 ]. In this respect, Stier et al. [ 72 ] provide the most advanced guide on how to systematically link survey data with information from external data sources, including SMD, at different level of analysis. The authors demonstrate that integrating traditional survey data and digital trace data is of growing interest, notably because of the limited reliability of self-reported behavioural measures and declining response rates. Additionally, enriching survey data with SMD could also help to reduce unit non-response and to control for the unrepresentativeness of SMD, as they are limited to those respondents having social media profiles and consenting to the linkage. Finally, a smaller share of research (n = 5) focuses on developing a quality assessment framework for SMD which is similar to the Total Survey Error (TSE) [ 11 , 38 ]. The TSE framework has been extended to encompass SMD and their inherent quality challenges (see the studies by Sen et al. [ 70 ] on Twitter-based studies and Jungherr [ 47 ] for a measurement theory to account for the pitfalls of digital traces). In a similar vein, Hsieh and Murphy [ 43 ] analysed the potential benefits of evaluating estimates from surveys and SMD in common terms and arrived at a general error framework for Twitter opinion research. Olteanu et al. [ 61 ] went a step further by pointing to the errors and biases that could potentially affect studies based on digital behavioural data, outlining them in an idealised study framework. The paper by Sen et al. [ 70 ] provides the most advanced framework to date. It involves potential measurement and representation errors in a digital trace-based study lifecycle where they are classified according to their sources.
Other research (n = 5) tackles the ontology of SMD as compared to survey data. In these papers, prevalent discussions revolve around the conception of opinion as measured by both data sources, as well as debates related to the evolution of “new” research “paradigms” or “digital hermeneutics”. The remaining papers concentrate on behavioural research (n = 2), demographic research (n = 2), and small data analysis in political communication (n = 1).
Overall, the considered theoretical articles stress the importance of developing a framework that accounts for possible biases of SMD while remaining in, or mirroring, the TSE. Moreover, they also emphasize the need, in this debate, to focus on the complementarity rather than the replacing aspect, notably by developing clear and reliable linking strategies. These articles also encourage researchers to go beyond the dominant model for understanding PO from probability sample surveys to encompass other (“new”) expressions of opinions (e.g. Murphy et al. 2014) that can possibly supplement or even replace survey-based approaches.
Empirical insights
The empirical literature (N = 141) focuses on a rather narrow set of topics, such as elections, political issues, and approval ratings for the presidency (64%). Another important area of PO research using SMD complementarily with survey data is related to health (e.g. vaccination, drugs, etc.), equality issues, and climate or environment-related concerns. Most empirical studies in our review are based on Twitter data (73%), followed by Facebook (18%) and other social media (9%). This is related to the fact that not all social media platforms provide the same degree of data accessibility [ 8 ]. For instance, Facebook imposes severe limitations on the scope of retrievable data, whereas Twitter has less strong privacy settings, allowing researchers to get access to Twitter’s historical data.
Overall, we derived six major approaches on how survey data and SMD can complement each other namely i) predicting social and political outcomes using SMD (n = 48), ii) comparing both data sources on a given phenomenon (n = 26), iii) using survey measures as a proxy in social media research (n = 18), iv) enriching surveys with SMD (n = 9), v) recruiting individuals on social media to conduct a second survey phase (n = 8), and vi) generating new insight on “old” or “under-investigated” topics or theories using SMD (n = 32). These approaches can be synthesised in four, partly overlapping, ‘data complementing’ research purposes: i) validating survey findings with SMD, ii) improving the sustainability of the research by diversifying the views on a phenomenon, iii) improving the reliability of survey measures by specifying measurements, and iv) improving the interpretability of social or political issues. Figure Figure2 2 summarises the relationship between the six approaches and the four research purposes. Furthermore, it shows that each purpose leads to a typical way of using both data sources complementarily. For instance, improving reliability by specifying a research question involves data linkage strategies, while generating new insights involves a sequential use of social media and survey stages.

Complementary approaches using SMD and survey data for the study of PO
The analysis of our corpus suggests that the biggest part of research concentrates on whether SMD can potentially substitute survey data (n = 48, see Table Table3 3 in the Appendix). This has mostly been done by trying to replicate survey findings by using SMD for forecasting (see recent review by [ 66 ]). The aim to predict real-world outcomes with SMD in the realm of PO has essentially been applied to elections. Most of these papers directly refer to the much-cited study of O’Connor et al. [ 60 ] which purpose is to validate SMD against survey findings. While research in this area has tested a range of different methodologies, the results remain inconclusive, and only in some cases could elections be accurately predicted (e.g. [ 31 , 47 ]). Recent literature reviews on the use of SMD for running electoral predictions (e.g. [ 15 ]) classify studies according to the employed methods of prediction, such as volume, sentiment, or network approaches. These reviews show considerable variance in the accuracy of predictions, which, on average, lag behind the established survey measurements. A common problem of the aforementioned studies lies in the decision about which approach can most accurately yield predictions (but also which social media platforms are better suited, and how that varies in different geographical or temporal contexts). This inference problem is quite complex as various elements are involved in skewing the samples in social media debates. To date, the inconclusive state of the research has led to a research agenda aiming to respond to the plea from Gayo-Avello et al. [ 33 ] for a “model explaining the predictive power of social media” (p. 490). In this realm, for instance, the study of Pasek et al. (2019) assesses how patterns of approval among population subgroups compare to tweets about the president, while disentangling effects at the individual and group levels of analysis. On a more theoretical level, the study by Schober et al. [ 69 ] seeks to elaborate when and under what conditions SMD can be used to make valid inferences. However, the inconclusive state of the research may also be linked to the fact that predictions are often done based on the content created by users and overlook the characteristics of the creating users. For instance, SMD can be biased towards a particular group (see [ 5 , 24 ]). Moreover, interactions on social media platforms are not always the product of individuals, but also bots, organisations, political parties, etc. [ 80 ]. Based on the evaluation of the body of articles falling under the ‘substitution paradigm’, a path for future research could be to better account for the characteristics of social media users, insofar as these characteristics can be useful for assessing how individual tweets can be converted into meaningful measures of expressed opinion. To do so, future studies could survey social media users identified using relevant key terms (e.g. hashtags or mentions) to gauge the relationship between social media measures of their sentiment and survey measures of their attitudes.
List of publications combining social media and survey data for prediction purposes (n = 48, continues next pages)
The second dominant approach in our review is related to how surveys can be enriched with SMD (n = 9, see Table Table4 4 in the Appendix). Here, SMD are collected with the intention of improving the reliability of survey measures at the individual or aggregate level. Replication of survey-based opinions can be difficult, either because of improper interpretation of the findings or because insufficient information has been provided. Such issues undermine the credibility of survey research and make it difficult to evaluate the contributions of a given study. Research aiming to enrich surveys with SMD most often implies the adoption of a data-linking strategy. This can be done, for instance, either at the user level, public actor level, geographic level, or temporal level (see [ 72 ]). Enriching surveys with SMD can serve several goals. First, it can help to augment the explanatory potential of survey measures. For instance, De Sio & Weber [ 23 ] adopted an innovative research design to explain election outcomes based on party strategy on social media with respect to policy issue salience. They did this by linking representative mass surveys from six European countries with Twitter analysis of campaign activity. Second, enrichment of survey data with SMD can also help to test research hypotheses by relying on “true” behavioural measures (instead of self-reported survey measures). For instance, Karlsen and Enjolras [ 48 ] linked candidate survey data with Twitter data to study styles of social media campaigning. These differences in campaigning styles were then related to the extent to which candidates were successful on Twitter. Third, SMD also offer an opportunity to address issues of item non-response and calibration of novel measures. For instance, Shin [ 71 ] studied the extent to which social media users selectively consumed like-minded news stories by linking survey responses from Twitter users with their media following and exposure to news via their friends. The study further showed some differences between self-reports and digital measures, such as more pronounced patterns of selective exposure in the SMD. Finally, linking social survey and SMD further provides an opportunity to explore the relationship between attitudes and beliefs reported through surveys and content (and behaviours) generated online. For instance, Cardenal et al. [ 14 ] combined survey and Web-tracking data to analyse how Facebook-referred news consumption influenced social media users’ agendas. They found that selective exposure increased with amplified news consumption. The core problem in these studies lies in gaining consent to carry out the data linkage. This constitutes a complex procedure in which issues of anonymity, security, and disclosure all come to the fore. An additional problem is that social media measurements provide only one partial view of opinions. For instance, while researchers can measure how many times a given message has been liked, shared, or retweeted, it is much harder to account for (or measure) how often a given message has been seen or has attracted attention. Moreover, our corpus shows that research relying on linking strategies tends to remain at the individual and public actor levels of analysis, which requires requesting consent to use the linked data. This may, in turn, introduce consent or selection bias. To mitigate such difficulties, future studies should also explore the potentials of linking both data sources at higher levels of analysis, such as country or according to topicality level.
List of publications combining social media and survey data for enrichment purposes (n = 9)
A third purpose is to use surveys as a proxy in social media research. This approach therefore reverses the logic that SMD are always used as a complementary (side) element of the main survey-based analyses. In this kind of “survey proxy approach” (n = 18, see Table Table5 5 in the Appendix), SMD are used as the main source of analysis, while the survey data are used for contextualising or calibrating SMD. A first strand of research relies on SMD to complement traditional research approaches in political communication and citizens’ political engagement. For instance, the assessment of the importance of given public concerns in PO has been measured extensively with the “most important problem” survey item. Social media provide another way to measure this concern in an unintrusive way by (semi-)automatically classifying the content of social media texts, while also accounting for the extent to which different actors are responsive to these concerns. Following this logic, the study conducted by Eberl et al. [ 28 ] investigated the effects of sentiment and issue salience on emotionally labelled responses to posts written by political actors on Facebook. Another study, by Plescia et al. [ 64 ], analysed the responsiveness of populist parties to the issue salience amongst the public. They did this by relying on survey data to measure public salience and tweets to assess salience issue for parties. A second strand of studies aims at facilitating cross-national comparisons. For instance, a possible application consists in using survey data for classifying parties and voters along important dimensions (e.g. see [ 30 ]). Here, parties were placed on a left and right spectrum using the Chapel Hill Expert Survey [ 4 ]. Party score on the overall ideological stance was then used as an explanatory variable in subsequent analysis. Another example is the study by Park et al. [ 62 ] which investigated the consumption of popular YouTube videos in countries that differ in cultural values, language, gross domestic product, and Internet penetration rate. A possible issue encountered by these studies is linked to spurious effects between survey and social media measurements (e.g. misleading or unexplained correlations). Furthermore, these studies tend to remain poorly equipped to explain actual motives behind social media users’ expression of opinions or reactions. The “survey as proxy” approach requires a considerable dose of ingenuity and methodological innovation to mine social media for producing opinion estimates that can be merged with survey estimates. For instance, SMD corpora often deviate from a predefined (survey) coding scheme. Substantively, a future path of research should take advantage of the fact that a growing number of societal issues have become transnational, such as immigration, terrorism, women’s rights, and climate change. Such research could involve the combination of word embeddings and survey opinion measures at the country level.
List of publications using survey as proxy with social media data (n = 18, continues next page)
A fourth approach aims to compare SMD with survey responses that directly measure PO. Studies comparing SMD with survey data (n = 26, see Table Table6 6 in Appendix) essentially aim at improving sustainability of the research, which consists in the ability to gauge PO consistently over time. Sustainability thus implies that we should develop designs that include opportunities for “holistic merging” of the data that will generate more inclusive and fine-grained research insights. There are several reasons that comparing both data sources is meaningful for social research. Firstly, comparing SMD and survey data can be very useful in times of protests and collective actions, notably due to the difficulty of generating survey data to properly assess these disruptive changes (see critique of survey data by Lee [ 53 ]). The timing of an event might indeed not coincide with the timing of a survey, which is often done ex-post. For instance, Davis et al. [ 21 ] examined the extent to which tweets about the affordable care act (“Obamacare”) could be used to measure PO over time. Secondly, social media can be compared to surveys for research questions that require chronicity, on a weekly or daily basis, thus going beyond the few ongoing surveys that collect data monthly or yearly. For instance, Diaz et al. [ 25 ] demonstrated how social media activity functions like an “opt-in panel” where users repeatedly discuss the same topics. This allows us to study, longitudinally, quite rapid shifts in individual opinions and behaviours, thus complementing survey panels which are prohibitively expensive. Another example is the study by Loureiro & Alló [ 54 ], which aimed to complement surveys by providing up-to-date measurements about social concerns when debating mitigation and energy transition paths. Thirdly, survey questions are often designed to capture internal attitudes toward a specific object. However, the relevance of certain survey questions might vary over time and, in some cases, might no longer correspond to the issues discussed spontaneously online. For instance, at a geographical level, the study by Scarborough [ 67 ] compared gender equality attitudes found in survey data to sentiments emanating from tweets. Fourth, SMD can produce quicker and less expensive statistics for enabling informed policy and program decisions. However, this requires gaining knowledge of where any possible disparities in attitude distributions between SMD and survey data may lie. In this respect, the study by Amaya et al. [ 2 ] presented recent advancements. The authors compared attitude distributions between Reddit users and survey measures of political leaning, political interest, and policy issues. They showed that Reddit users tend to have more centrist and normally distributed scores than the survey data, skewing estimates toward the conservative end of the spectrum on all attitude measures. Another study, from Pasek et al. (2020), explained that SMD might be better conceived as providing insights about public attention rather than (“survey like”) attitudes or opinions. To do so, the authors compared tweets mentioning the presidential candidates and open-ended survey questions about the candidates to assess whether spikes surrounding political events correlate between both data sources. Results display some support for the correlation between social media attention and survey data, but they also show systematic differences that need to be better understood to assess when SMD can best generate insights about select topics. The research comparing both data sources tends to remain focused on volume analysis and tonality assessment. This type of research also tends to pay little attention to the domain-specificity of the SMD collected as well as to ways of mitigating replicability and consistency issues (e.g. [ 34 ]). For instance, the evolution of search queries around a given theme might lack precision and consistency over time. The connotation of hashtags can change or whole hashtags can even disappear. Better combining both data sources also requires elaborating more sophisticated measures of opinion and attitudes. One could think about pushing forward “stance detection” in complement to “sentiment detection”, but also about advancing “narrative analysis” in complement to “topic or frame detection”. These are avenues where computational social research would benefit from the expertise of applied computational linguistics.
List of publications combining social media and survey data for comparison purposes (n = 26, continues next pages)
A fifth approach implicates using SMD to generate new insights. This is especially useful when survey data are not available or when survey data are not recent enough (n = 32, see Table Table7 7 in Appendix). Here, the main purpose is to improve the interpretability of the research by adopting an “ethnographic” methodology. By avoiding rigid research design plans, SMD can remain responsive to, and pursue, new paths of discovery as they emerge. Based on the papers collected, we found typical reasons for relying on SMD to generate new insights, such as capturing emergent opinions, expanding the scope of survey measures, validating survey measures, proposing novel approaches to get a more nuanced or dynamic perspective on PO, and making causal analyses (see column “Reason to complement” in Table Table6 6 in Appendix). When used for capturing emergent opinions, SMD allow us to study the topical and normative climate around specific issues for which we have no theoretically grounded ideas yet. In this exploratory design, social media can provide survey researchers with a snapshot of important societal and political concerns worth surveying in future research. This is especially useful for emerging topics, such as nuclear power (e.g. [ 50 ]) or health-related policies [ 65 , 74 ]. On these emerging issues, SMD can be used in an exploratory or ethnographic perspective to generate initial and qualitative insights into under-studied research objects in order to develop quantitative survey measurements. SMD can also be useful for expanding the scope of survey measures on topics that are difficult to survey. For instance, Hatipoğlu et al. [ 41 ] used SMD to study international relationships with a case study on Turkish sentiments towards Syrian refugees using Twitter. Another study by Guan et al. (2020) relied on the social media platform Weibo to study Chinese views of the United States. SMD can also be useful for validating survey measures. For instance, the study by Dahlberg et al. [ 20 ] investigated the meanings of democracy in a cross-country perspective to better understand differences in the usage of the term “democracy” across languages and countries. The authors’ findings aimed to inform survey measurements about the different conceptualisations of democracy, notably by highlighting translations and language equivalence issues in survey items. Another reason is to propose novel approaches for achieving a more nuanced or dynamic perspective on PO. For instance, researchers can add new components and improve “old findings”, which are difficult to measure with survey data. In this view, the study by Barberá et al. [ 7 ] modelled policy issue responsiveness using Twitter data, thus going beyond the more static perspective on issue congruence offered by surveys. In another study, Clark et al. [ 16 ] investigated organisational legitimacy in a case study about public reactions on social media to the Supreme Court’s same-sex marriage cases. The authors argued that SMD can lessen some of the limitations of survey research in the field, notably by accessing not just policy positioning among individuals but also a variety of features of political discourse, such as opinion intensity and emotions like anger or happiness. SMD can also be used to make causal inferences in order to understand changes in opinion before and after an event, such as measuring the effect of a promulgated law on PO [ 1 ]. Here, SMD allow researchers to rely on spontaneous opinions expressed online rather than on retrospective survey questions, and this can help develop policy initiatives. For instance, Tavoschi et al. [ 73 ] used Twitter as a “sentinel system” to assess the orientation of PO in relation to vaccination. Despite the advantages of SMD in providing new research insights, these studies tend to lack a rigorous contextualisation of the findings derived from SMD. In this respect, a reliance on SMD would benefit from implementing sequential designs, where social media help to identify specific populations or sub-topics, which could then lead to a second quantitative survey phase. Whenever possible, SMD would further benefit from a comparison with longitudinal surveys to assess the extent to which both data sources reveal similar dynamics of change. Future studies could further exploit SMD’s ability to generate new insights for research in sensitive fields, such as war, racism, sexual orientation, and religious beliefs. These are often topics on which it remains difficult to collect survey data, notably because of the social desirability bias (e.g. [ 52 ]) and the like (e.g. extreme response style, moderacy bias, and acquiescence), but also because of the fear of being denounced or because the topic is controversial.
List of publications combining social media and survey data for generating new insights (n = 32, continues next pages)
The last approach using SMD and survey data complementarily focuses on using social media to recruit survey respondents. However, in comparison with the previous approach, the studies collected here usually analyse SMD and survey data in sequential phases. As we only consider papers that are in some way also related to PO and are not solely about recruitment of survey respondents and their socio-demographic characteristics, the number of studies we were able to analyse is much smaller (n = 8, see Table Table8 8 in the Appendix). Our review demonstrates that the papers essentially tackle the problem surveys have in recruiting specific politically involved sub-groups of the population. In particular, the research relies on social media to access representative samples of social media users, for instance, those who commented on their countries’ elections (see [ 9 , 12 ]) or who posted at least one election-related tweet [ 79 ]. Furthermore, in these studies, ethical concerns (e.g. privacy, tracking, etc.), but also the technical affordability of the social media platform used, are discussed. The latter issue is important, as each social media platform has particular arrangements which are likely to influence the group of individuals that can be reached. Overall, future studies could think about extending the recruitment approach to enhance our knowledge of reactions to systematic events, topics, or other repetitive features (such as supporting an issue or taking part in actions), while eliminating recall errors. Furthermore, relying on SMD can help researchers pre-test their hypotheses for future surveys by uncovering relevant underlying discursive patterns or by making smaller-scale qualitative observations.
List of publications using social media as a recruitment tool (n = 8)
Summary and concluding remarks
The aim of this article was to provide a review of published papers on the complementarity of SMD and survey data for PO research. We started this review by situating our work within theoretical advances concerning the complementarity of both data source. There has been extensive work underlying the opportunities and (quality) challenges of SMD for answering social research questions. However, research attention has only recently turned to SMD as a source of expression of PO and of its measurement. Consequently, there is a need for more research to uncover the ways in which SMD can be best used for fostering the understanding of PO.
The main contribution of our review is to provide a complete picture of the empirical research on the topic while calling attention to the pros and cons of each approach and possible future paths of advancements. Though this review might not be exhaustive, it has enabled us to show six major complementarity approaches which were identified as responding to four different research purposes. Below we highlight the main research paths for each approach. Using both data sources complementarily for prediction purposes was by far the most prominent approach and it remains a research area which raises many questions about the potential generalisability of the findings, namely in terms of the representativeness and validity of social media measurements of PO. We believe that the most important difficulty lies perhaps in the manner in which these studies deduce political opinions or attitudes from SMD. Survey researchers readily admit that opinions are more difficult to measure than behaviour because they involve what people think and not just how they act. Thereby, the choice to rely on sentiment analysis or merely on volume metrics (such as the number of retweets or mentions) seems unclear, at least for the near future.
Approaches concerned with improving sustainability have a significant potential for advancing social research, as they allow researchers to combine the richness of SMD content with established survey measures. When SMD are used in similar contexts to survey data, we believe that a critical view should prevail, informed by current social science best practices and expertise. For instance, whereas surveys draw a sample of carefully worded and standardised questions, social media can cover many topics as well as different facets of the same topic, which are not necessarily defined a priori on a theoretical basis. This research avenue is most likely to be fruitful for studies aiming to augment surveys by mapping discussions that are topical on social media, while allowing variations at country or regional levels of analysis to be discerned (e.g. Bennett et al. [ 10 ] on climate change opinions). Studies aiming to compare both data sources are certainly the most suitable to help improve our understanding about when and how both data sources can be validly combined. Survey methodologists can play a decisive role, notably by paying attention to the type of (open-ended) questions that can be more directly comparable with SMD. This direction can also inform the lack of consistent evidence for the first prediction approach.
Alternatively, studies aiming to improve reliability see research as mostly requiring control for the still severe limitations of using SMD appropriately in a PO context. In this respect, studies enriching survey data with SMD offer a solution to the fact that social media often lack relevant individual information, such as respondent’s attributes (e.g. sociodemographic characteristics or personality traits) or key outcome variables (e.g. voting, social, or political attitudes). Additionally, the “survey as proxy” approach enables researchers to calibrate SMD according to standardized survey measures at the actor (e.g. political candidates or parties) or context levels by reversing the data linking strategy. Future paths for both approaches implicate opening up the analysis to non-individual levels.
Studies aiming to improve the interpretability of survey research by generating new insights or by recruiting respondents on social media for a second survey phase, and that use both data sources complementarily, offer additional fertile ways to consider for new analyses that would not be possible using survey data alone. In this view, SMD do not aim to replace opinion surveys, but aim to provide a broader context for interpreting opinion, which will then serve to improve the quality of survey questions. This research avenue is most likely to be useful for knowing more about hard-to-reach populations (e.g. the LGBTQI* or disabled persons communities) or topics that are difficult to survey (e.g. violence and racism), especially when conducting iterative phases of analysis. It is also useful to get “opinion climates” about topics which have long been under survey scrutiny (e.g. emerging concerns related to feminism or social inclusion) in order to develop “updated” survey measurements.
Bringing together the opportunities offered by these different approaches shows that samples of social media users do not necessarily have to be representative of the general public to be used meaningfully as a complement to surveys. Most importantly, we believe that SMD should supplement, but not replace, traditional methods and data sources in the study of PO. By keeping up with current developments, we believe that remaining in the framework of survey research when using both data sources complementarily is paramount for identifying potential non-survey data sources, accessing them, and assessing their quality and usefulness for the study of PO. Like mixed-method approaches combining qualitative and quantitative data (e.g. [ 36 ]), the primary motive for complementing survey and SMD with one another is to allow researchers to mix datasets in a meaningful way for developing an overall interpretation.
Technical and ethical note
Regarding sustainability, it is important to consider that the patterns of social media consumption are influenced not only by user preferences, but also by technological changes and the availability of the platforms. For instance, social media companies may not survive and whole platforms could disappear, thus impeding data access. With changes in consumption patterns, PO may be difficult to measure consistently over time. From a more technical perspective, it is also important to assess the extent to which databases composed of social media texts collected by different means (e.g. different search queries or different platform algorithms) might raise consistency and replicability issues (e.g. [ 34 ]). As for reliability, several issues are worth considering. Even though SMD can provide complementary information to survey estimates though linkage, there are sometimes concerns about the veracity or honesty of the information collected. For instance, SMD may increase the potential for social stigmatisation, causing users to be more reluctant to share their true opinions [ 63 ]. However, the opposite may also be true: users could express more radical opinions to gain social approval (e.g. disinhibition effect). The identity of those who post can also raise veracity concerns [ 55 ], and it may be difficult to distinguish sarcastic content from texts that are straight-forwardly positive or negative (e.g. [ 35 ]). Another important issue is that we usually know how many people have liked a post, clicked on a link, or retweeted a message, but we rarely know how many people have seen the item and chosen not to take any action [ 77 ]. Furthermore, due to algorithms that favour selective exposure and homophily of opinion [ 6 , 17 ], it is important to assess the extent to which findings derived from online opinion generate more polarised opinions than the ones that would be obtained through the private setting of surveys.
When researchers aim to generate new insights, they should consider that each social media platform has particular arrangements. For instance, the orientation of the content (e.g. political, family-oriented, business-oriented) as well as the scope of the content (e.g. possible bias toward more visible events) can play a decisive role on what content is available and which user profiles are most likely to be active on the social media platform. Furthermore, the nature of the platform allows for different levels of engagement in debates (e.g. Twitter is mostly used for short text content, while YouTube and Instagram allow sharing and commenting on videos and pictures). Functional capabilities can not only influence the ways of recruiting respondents for a second survey phase (e.g. direct messages), but also the identifying of sub-groups of interest (e.g. differences between friend and follower networks, and the reciprocity of follower networks). In addition, social media platforms may give users control over the availability of the information (e.g. to suppress or filter unwanted comments), which will again impact what is available from whom and on what.
For each research purpose, we should also consider that there are important ethical factors that are likely to influence the possible paths of research relying on SMD. Each platform has its own rules which are subject to change at any time. For instance, anonymity settings also affect the content of SMD, with growing concerns about surveillance and the resulting loss of privacy [ 29 , 76 , 78 ], thus influencing what people are willing to post. There are also evolving rules about the banning of particular words and behaviours, as well as users, which may influence research findings (especially when conducting longitudinal research). SMD are private property of tech companies and can be arbitrarily erased or made inaccessible, compromising the replicability of research.
Our review has several limitations. First, it focuses on social media but do not include other data sources that are frequently compared to survey data to model PO (e.g. Google trends, mainstream media, or administrative data). We thus encourage future research to extend the proposed complementary framework to additional data sources. This would allow the building of knowledge about the most suitable ways of combining these data for answering specific research purposes. Furthermore, our review entails a conceptual aim with less focus on the variety of methods used to either collect, clean, analyse, and aggregate the data to generate statistics. Discussing the pros and cons of methodologies employed by these papers could constitute the object of another review.
Notwithstanding these limitations, our study is not only of interest for social and political scientists concerned by the declining response rates and restrictive budgeting for survey research [ 57 ]. As social media have been established as multifunctional tools, and many companies and researchers implement strategies based on social media to collect opinions, make predictions, study behaviours, conduct experiments, or recruit hard-to-reach populations, this review is also of interest for practitioners.
Extracting PO from social media text can foster social sciences by moving it forward as an applied field, thus bridging gaps between computational models and interpretative research. We see this collaboration as particularly important for developing more advanced and reliable measures of opinion from social media texts. This also constitutes an opportunity to challenge the opposition of the so-called data-driven and theory-driven approaches , a simplistic dichotomy which further consolidates the misconception that social research can be conducted by relying solely on text-based data. We encourage researchers to acknowledge the different conceptualisation of opinion when measured by SMD and surveys, and we advise them to adopt a mixed-method strategy where the complementarity of both data is paramount.
Code availability
Not applicable;
Authors contribution
All authors contributed to the design of the study. Maud Reveilhac planned the study, conducted the data analysis, and wrote the manuscript. All authors contributed to the review of the manuscript and approved the final version.
Open access funding provided by University of Lausanne.
Data availability
Declarations.
We have no conflict of interests to disclose;
Publisher’s note
Springer Nature remains neutral with regard to jurisdictional claims in published maps and institutional affiliations.
Contributor Information
Maud Reveilhac, Email: [email protected] .
Stephanie Steinmetz, Email: [email protected] .
Davide Morselli, Email: [email protected] .
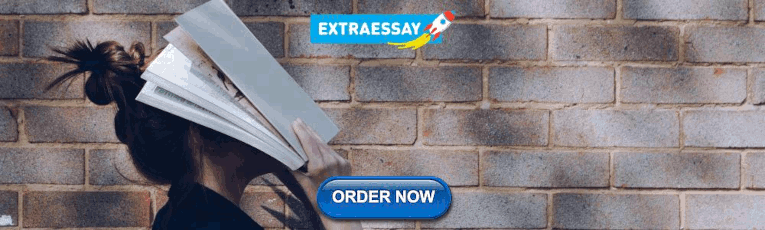
IMAGES
COMMENTS
This paper addresses this gap by utilizing a systematic literature review approach. A total of 115 articles were collected and analysed and five core themes were identified, examining (1) luxury brand strategy, (2) luxury brand social media communications, (3) luxury consumer attitudes and perceptions, (4) engagement and (5) social media's ...
Abstract. Luxury, historically an exclusive, rare and elitist phenomenon, is changing. This is predominantly driven by technological developments, particularly social media, and the rising level ...
Luxury, historically an exclusive, rare and elitist phenomenon, is changing. This is predominantly driven by technological developments, particularly social media, and the rising level of consumer empowerment in the marketplace. A maturing stream of research has emerged assessing the effects of social media platforms on luxury brands, offerings and consumers. However, there has been no ...
Socialmediaandluxury 101 Socialmediaandluxury Earlyluxurybrandresearchportraystheinternetasan unavoidable evolution, posing both threats and oppor-tunities for ...
Social media and luxury: A systematic literature review. Luxury, historically an exclusive, rare and elitist phenomenon, is changing. This is predominantly driven by technological developments, particularly social media, and the rising level of consumer empowerment in the marketplace. A maturing stream of research has emerged assessing the ...
To date, a comprehensive to construct, distribute and consume brand-related mes- review on the role of social media within a luxury context sages. This presents a wealth of opportunities and chal- has yet to be conducted. It is the purpose of this paper to lenges for businesses, particularly the luxury industry.
A systematic literature review of academic research on social media marketing has been conducted to gather, examine and synthetize studies related to luxury brands. By following a review protocol based on both automatic and manual search on the Scopus database, all relevant studies on luxury brands were identified and analyzed.
Store environment, online website experience, social media, and individualized brand communication all have symbolic or experiential significance. However, the onset of the pandemic and the subsequent lockdown restrictions proved to be a roadblock for luxury brands in providing offline brand experience to consumers towards the end of 2019.
Purpose Digital technologies and social media have improved the connectivity and collaboration between firms and customers in all sectors. However, in the luxury sector, the approach to social media and digital technologies has been slower than in other industries. The purpose of this paper is to review the academic literature on social media marketing in luxury brands to highlight the current ...
Third, a systematic review of the literature on academic research on social media marketing in luxury brands was conducted to collect, examine and synthesize the reactive studies. This review also provided important insights on social media marketing in luxury brands and key research topics were classified into three insights, on consumer ...
Request PDF | Social media marketing in luxury brands: A systematic literature review and implications for management research | Digital technologies and social media have improved the ...
2,599 followers. "Social media and luxury: A systematic literature review" by Dean Creevey, Joseph Coughlan, and Christina O'Connor provides a comprehensive analysis of the impact of social media ...
Third, a systematic review of the literature on academic research on social media marketing in luxury brands was conducted to collect, examine and synthesize the reactive studies.
Abstract. This article provides a systematic literature review of the hospitality, leisure, tourism, business, and management literature on luxury hospitality. We use a data-driven quantitative method (i.e., bibliometric review) to provide an integrated view of extant luxury hospitality research. The bibliographic coupling analysis allowed us ...
The luxury tourism industry immediately conjures up thoughts of exclusivity, with access to it confined to a small and elite group of travelers often located within their own social bubble. Our systematic literature review seeks to understand how tourism scholarship has addressed the issue of luxury travel based on social media pronouncements and the areas of concentration in which earlier ...
[13] Fisch C Block J. Six tips for your (systematic) literature review in business and management research. ... This study examines the potentials of social media marketing for luxury retailers. Social media marketing tactics of three luxury retail brands Barneys New York, Net-a-Porter.com, and Saks Fifth Avenue were examined across three major ...
This article focuses on studying the social media influence on an individual through systematic literature review ( Brereton et al., 2007) with respect to TCCM approach (Theory, Context, Characteristics and Methodology). Adopting a framework or lens in literature reviews help in bringing objectivity to the analysis.
This structured, systematic literature review explores who and in which ways can influence consumers on social media to engage with more sustainable fashion consumption practices. Based on an analysis of 92 research studies, the analysis findings indicate that most studies examined how brands can influence consumers via social media marketing ...
Adopting a systematic literature review, the research provides an overarching picture of what has already been investigated and the existing gaps that need further research. ... Examining the impact of luxury brand's social media marketing on customer engagement: Using big data analytics and natural language processing. Journal of Business ...
In this article, we review existing research on the complementarity of social media data and survey data for the study of public opinion. We start by situating our review in the extensive literature (N = 187) about the uses, challenges, and frameworks related to the use of social media for studying public opinion.
Based on a systematic review approach, the present study addresses recent trends and developments in green advertising on social media. We used PRISMA for the systematic review of eighty-one articles published between 2011 and 2022. Green advertising content, and greenwashing in particular have attracted significant research interest.
Our systematic literature review seeks to understand how tourism scholarship has addressed the issue of luxury travel based on social media pronouncements and the areas of concentration in which ...
2.3. Topic selection. The method Paul and Criado (Citation 2020) suggested for a systematic literature review was followed to analyze the existing literature on consumer ethnocentrism.Paul and Criado (Citation 2020) have posited that good reviews are impactful, and that happens for the reasons when the authors have not picked up a recurrent topic, do not have several other studies published ...
Methodology. In this research, we undertake a descriptive qualitative systematic literature review (SLR) to conduct a comprehensive analysis of the existing research on COVID-19 humor on social media and to specifically explore its themes and categories, coping mechanisms, effects on confidence in government institutions, and the utilization of specific linguistic devices.
Social commerce, the integration of social media into e-commerce, has resulted in significant transformations for both businesses and consumers. Several research have been conducted to understand social commerce, and numerous systematic literature reviews have produced insights from the current literature on social commerce.
This manuscript explores the profound impact of social media on society, with a focus on social behavior, politics, and cultural norms. Employing a systematic literature review, including diverse ...
Transformer technologies, like generative pre-trained transformers (GPTs) and bidirectional encoder representations from transformers (BERT) are increasingly utilized for understanding diverse social media content. Despite their popularity, there is a notable absence of a systematic literature review on their application in disaster analytics. This study investigates the utilization of ...