Students & Educators —Menu
- Educational Resources
- Educators & Faculty
- Standards & Guidelines
- Periodic Table
- Adventures in Chemistry
- Landmarks Directory
- Frontiers of Knowledge
- Medical Miracles
- Industrial Advances
- Consumer Products
- Cradles of Chemistry
- Nomination Process
- Science Outreach
- Publications
- ACS Student Communities
- LEADS Conference
- ChemMatters
- You are here:
- American Chemical Society
- Students & Educators
- Explore Chemistry
- Chemical Landmarks
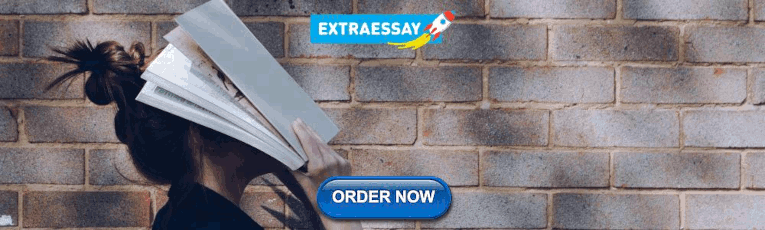
Deciphering the Genetic Code
National historic chemical landmark.
Dedicated November 12, 2009, at the National Institutes of Health in Bethesda, Maryland.
Commemorative Booklet (PDF)
DNA consists of a code language comprising four letters which make up what are known as codons, or words, each three letters long. Interpreting the language of the genetic code was the work of Marshall Nirenberg and his colleagues at the National Institutes of Health. Their careful work, conducted in the 1960s, paved the way for interpreting the sequences of the entire human genome.
Modern Genetics: A Monk and a Double Helix
Marshall nirenberg’s early career, experiments with synthetic rna, breaking the genetic code: the “poly-u” experiment, the nobel prize and reactions, further reading, landmark designation and acknowledgments, cite this page.
Modern genetics begins with an obscure Augustinian monk studying the inheritance of various traits in pea plants. Gregor Mendel’s laws of inheritance revealed the probabilities of dominant and recessive traits being passed from generation to generation. Mendel’s research received little recognition in his lifetime. The significance of Mendel’s laws was recognized only in the early 20th century.
With that rediscovery came interest in how genetic information is transmitted. Oswald Avery, a bacteriologist at New York’s Rockefeller Institute, demonstrated that deoxyribonucleic acid, DNA, produced inheritable changes. This discovery was not well received: How could DNA, a substance containing only four different nucleotide building blocks, store genetic information? Others discovered that DNA varies from species to species. Then, in 1953, James Watson and Francis Crick at Cambridge University electrified the scientific world with their model of DNA, the double helix. Watson and Crick recognized that the double strand might allow replication.
How could DNA, a double helix made up of only four different nucleotides, determine the composition of enzymes (proteins), long peptide chains composed of twenty different amino acids? The race to discover the genetic code which translates DNA’s information into proteins was underway. To stimulate the chase, George Gamow, a theoretical physicist, organized the twenty-member “RNA Tie Club”: members wore ties with the symbol for one of the 20 amino acids. The members shared ideas on how DNA transmitted information.
The scientist who won the race was not a member of the “club.”
Back to top
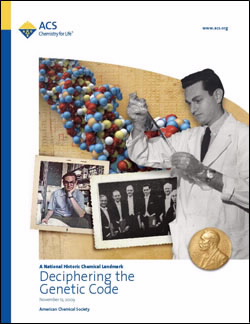
I thought if I’m going to work this hard, I might just as well have fun and by fun I mean I wanted to explore an important problem and I wanted to discover things.” — Interview with Marshall Nirenberg, July 15, 2009.
Marshall Nirenberg earned a Ph.D. in biological chemistry from the University of Michigan with a dissertation on the mechanism of sugar uptake in tumor cells. He continued that research as a postdoctoral fellow at the National Institutes of Health. In 1959, he joined the staff of NIH as a research biochemist.
Nirenberg gave some thought to what he wanted to study as an independent investigator. “At that time,” he said in 2009, “the mechanism of protein synthesis was very incompletely known and messenger RNA had not been discovered.”*
Nirenberg’s initial goal was to determine whether DNA or RNA (ribonucleic acid), copied from DNA, was the template for protein synthesis. But Nirenberg had no formal training in molecular genetics, and he knew “that this was an incredibly risky project, because when you take your first position, you want to hit the deck running and show you are a productive scientist.” With no experience in the field, with no staff at the outset, and in a race against the best scientists, Nirenberg knew he “could fail easily.”
*This and subsequent quotations, unless the text indicates differently, are from an interview by Judah Ginsberg and Marshall Nirenberg, conducted in his laboratory on the campus of NIH on July 15, 2009.
Nirenberg and Heinrich Matthaei, a postdoctoral fellow from Germany, began their experiments by studying the long linear molecule DNA and RNA. In DNA, the nucleotides are adenine (A), guanine (G), cytosine (C) and thymine (T); in RNA, uracil (U), replaces thymine.
They chose a cell-free environment, created when cell walls are broken down, releasing the cell’s contents. The remaining cytoplasm can still synthesize protein when RNA is added, allowing the researchers to design experiments to determine how RNA works free of the complicated biological processes that could shroud molecular activity.
Nirenberg and Matthaei selected E. coli bacteria cells as their source of cytoplasm. They added the E. coli extract to 20 test tubes, each containing a mixture of all 20 amino acids. In each test tube one amino acid was radioactively tagged, a different one in each test tube. The reaction could be followed by monitoring radioactivity: incorporation of a “hot” amino acid would form a “hot” protein.
3:00 in the morning, May 27, 1961, a Saturday: Matthaei adds synthetic RNA made of only uracil units to each of the 20 test tubes, finding unusual activity in one of the tubes, containing phenylalanine. The spectacular result demonstrates that a chain of uracil units in the “hot” tube instructed the addition of the “hot” amino acid.
Nirenberg and Matthaei understood what had happened: Synthetic RNA made of a chain of multiple units of uracil instructed a chain of amino acids to add phenylalanine. The uracil chain (poly-U) served as a messenger directing protein synthesis. Although the question of how many units of U were required was yet unanswered, the experiment proved that messenger RNA transcribes genetic information from DNA, directing the assembly of amino acids into complex proteins. The key to breaking the genetic code—molecular biology’s Rosetta Stone—had been discovered.
Nirenberg presented his successful poly-U experiment at an international biochemistry congress held in Moscow in August, a few months later. He was acutely aware of his outsider status: “I didn’t know the people in molecular biology… I didn’t know anybody in protein synthesis… I was working on my own.” That may explain why only 35 people attended his talk and why the audience “was absolutely dead.”
But in one of those serendipitous events that change everything, Nirenberg had met Watson the day before and told the co-discoverer of the double helix about his results. Watson was skeptical about Nirenberg’s claims, but he convinced a colleague to attend the paper; when the colleague reported that Nirenberg’s findings were real, Watson told Crick who arranged for Nirenberg to present his paper again, this time in a major symposium on nucleic acids at the same congress. “The reaction was incredible,” Nirenberg remembered. “It was a standing ovation… I didn’t know it then, but for the next five years I became like a scientific rock star.”

After Nirenberg and Matthaei “cracked” the first “word” of the genetic code, scientists raced to translate the unique code words for each amino acid in hopes of someday reading the entire genetic code of living organisms. Nirenberg assembled a team of about twenty researchers and technicians.
Using the poly-U experiment as a model, Nirenberg and his colleagues identified nucleotide combinations for the incorporation of other amino acids. The researchers found that the coding units for amino acids contain three nucleotides (a triplet). Combining four nucleotides in three-letter codes yielded 64 possible combinations (4 x 4 x 4), sufficient to describe 20 amino acids.
They discovered the codes for other amino acids: for example, AAA for lysine and CCC for proline. Replacing one unit of a triplet code with another nucleotide yielded a different amino acid, for one example, synthetic RNA containing one unit of guanine and two of uracil (code word: GUU) caused incorporation of valine.
In 1964 Nirenberg and Philip Leder, a postdoctoral fellow at NIH, discovered a way to determine the sequence of the letters in each triplet word for amino acids. By 1966 Nirenberg had deciphered the 64 RNA three-letter code words (codons) for all 20 amino acids. The language of DNA was now understood and the code could be expressed in a chart.
In 1968 Nirenberg won the Nobel Prize in Physiology or Medicine for his seminal work on the genetic code. He shared the award with Har Gobind Khorana (University of Wisconsin), who mastered the synthesis of nucleic acids, and Robert Holley (Cornell University), who discovered the chemical structure of transfer-RNA. Collectively, the three were recognized “for their interpretation of the genetic code and its function in protein synthesis."
Nirenberg describes the ceremonies surrounding the Nobel as “a week of parties.” Not quite all parties, however, since the rules of the Nobel require recipients to write a review article. This proved a challenge for Nirenberg, who had turned his research attention to neurobiology. ”I found it very difficult,” he later admitted, “to break off from neurobiology and go back to nucleic acids.”
As a Nobel Laureate, Nirenberg received many university offers that included higher salary, more laboratory space, and larger staff. He turned them all down, preferring to spend the rest of his career at NIH. “The reason I stayed,” he says, “was because the thing I had least of was time. I figured that if I went to a university I would use a third of my time to write grants… I thought I could use that time more productively by doing experiments.”
In 1961 The New York Times , echoing President Kennedy, reported that Nirenberg’s research showed that biology “has reached a new frontier.” One journalist suggested the biggest news story of the year was not Russian cosmonaut Yuri Gagarin orbiting the earth but the cracking of the genetic code.
Deciphering the genetic code raised ethical concerns about the potential for genetic engineering. Nirenberg addressed these concerns in a famous editorial in Science in August 1967, noting “that man may be able to program his own cells” before “he has sufficient wisdom to use this knowledge for the benefit of mankind… [D]ecisions concerning the application of this knowledge must be made by society, and only an informed society can make such decisions wisely.” When asked several decades later if society has acted “wisely” regarding genetic engineering, Nirenberg answered, “Absolutely!”
- Marshall W. Nirenberg Biographical (NobelPrize.org)
- The Marshall W. Nirenberg Papers (The National Institutes of Health)
Landmark Designation
The American Chemical Society designated the deciphering of the genetic code by Marshall Nirenberg as a National Historic Chemical Landmark at the National Institutes of Health in Bethesda, Maryland, on November 12, 2009. The plaque commemorating the research reads:
In this building, Marshall Nirenberg and Heinrich Matthaei discovered the key to breaking the genetic code when they conducted an experiment using a synthetic RNA chain of multiple units of uracil to instruct a chain of amino acids to add phenylalanine. The uracil (poly-U) served as a messenger directing protein synthesis. This experiment demonstrated that messenger RNA transcribes genetic information from DNA, regulating the assembly of amino acids into complex proteins. Nirenberg would go on to decipher the code by demonstrating the correspondence of various trinucleotides to individual amino acids. He was a co-winner of the Nobel Prize in 1968.
Acknowledgments
Adapted for the internet from “Deciphering the Genetic Code,” produced by the National Historic Chemical Landmarks program of the American Chemical Society in 2009.
American Chemical Society National Historic Chemical Landmarks. Deciphering the Genetic Code. http://www.acs.org/content/acs/en/education/whatischemistry/landmarks/geneticcode.html (accessed Month Day, Year).
Back to National Historic Chemical Landmarks Main Page .
Learn more: About the Landmarks Program .
Take action: Nominate a Landmark and Contact the NHCL Coordinator .

Accept & Close The ACS takes your privacy seriously as it relates to cookies. We use cookies to remember users, better understand ways to serve them, improve our value proposition, and optimize their experience. Learn more about managing your cookies at Cookies Policy .
1155 Sixteenth Street, NW, Washington, DC 20036, USA | service@acs.org | 1-800-333-9511 (US and Canada) | 614-447-3776 (outside North America)
- Terms of Use
- Accessibility
Copyright © 2024 American Chemical Society

An official website of the United States government
The .gov means it’s official. Federal government websites often end in .gov or .mil. Before sharing sensitive information, make sure you’re on a federal government site.
The site is secure. The https:// ensures that you are connecting to the official website and that any information you provide is encrypted and transmitted securely.
- Publications
- Account settings
Preview improvements coming to the PMC website in October 2024. Learn More or Try it out now .
- Advanced Search
- Journal List
- Life (Basel)

Efforts and Challenges in Engineering the Genetic Code
This year marks the 48th anniversary of Francis Crick’s seminal work on the origin of the genetic code, in which he first proposed the “frozen accident” hypothesis to describe evolutionary selection against changes to the genetic code that cause devastating global proteome modification. However, numerous efforts have demonstrated the viability of both natural and artificial genetic code variations. Recent advances in genetic engineering allow the creation of synthetic organisms that incorporate noncanonical, or even unnatural, amino acids into the proteome. Currently, successful genetic code engineering is mainly achieved by creating orthogonal aminoacyl-tRNA/synthetase pairs to repurpose stop and rare codons or to induce quadruplet codons. In this review, we summarize the current progress in genetic code engineering and discuss the challenges, current understanding, and future perspectives regarding genetic code modification.
1. Introduction
In 1968, Francis Crick first proposed the frozen accident theory of the genetic code [ 1 ]. The 20 canonical amino acids were once believed to be immutable elements of the code. The genetic code appears to be universal, from simple unicellular organisms to complex vertebrates. Yet in contrast to studies of the natural selection of lifeforms wherein the gradual evolution of species can be observed in a myriad of taxa, relatively few examples of natural genetic code variations (e.g., selenocysteine [ 2 ], pyrrolysine [ 3 , 4 ], and stop codon read through [ 5 , 6 ]) have been observed. Different explanations have been proposed to address these variations, such as the codon capture hypothesis [ 7 ], the ambiguous intermediate hypothesis [ 8 ], and the genome streamlining hypothesis [ 9 ]. These hypotheses have been reviewed elsewhere [ 10 , 11 ]. Although some existing noncanonical amino acids (NCAAs) are known to be compatible with enzymatic aminoacylation [ 12 , 13 , 14 , 15 , 16 , 17 , 18 , 19 , 20 ], the 20 canonical amino acids in the standard genetic code have been stringently selected over the course of biological evolution. Organisms that require peptides with modified side chains will often resort to pre-translational or post-translational modifications to incorporate NCAAs [ 21 , 22 , 23 , 24 , 25 , 26 ]. Some organisms require alternative genetic codes to survive in harsh living conditions [ 27 ].
Genetic code engineering refers to the modification, or the directed evolution of cellular machineries, in order to incorporate NCAAs into the proteome of an organism. In general, NCAAs can be artificially incorporated in a site-specific or proteome-wide manner. In the former, scientists have attempted to artificially engineer organisms for compatibility with various NCAAs by employing orthogonal tRNA/aminoacyl-tRNA synthetase pairs [ 28 , 29 , 30 , 31 , 32 ]. In the latter, an organism is forced to take up specific NCAAs, followed by isolating mutants in media containing NCAAs [ 33 , 34 , 35 ]. Currently, researchers are recording cellular responses and genetic changes in engineered organisms to understand the mechanisms behind the use of alternative genetic codes. Such efforts enable an understanding of the evolutionary course of the genetic code and provide a foundation for the derivation of additional alternative codes, a particularly important feature in the era of synthetic biology given the increased focus on engineering synthetic organisms with modified genetic codes [ 36 , 37 ]. Engineered genetic code holds tremendous potential in the field of protein engineering and xenobiology, which was extensively reviewed by Budisa et al. in 2017 [ 38 ].
In this review, we first give a brief introduction of current studies on both site-specific and proteome-wide incorporation of NCAAs. Next, we will focus on the challenges of engineering organisms to use modified genetic codes and their implications, such as inhibitory effects caused by NCAAs. Finally, we will discuss current trends in this research area.
2. Genetic Code Engineering
2.1. incorporation of ncaas into specific sites.
Currently, three major approaches are used to engineer the genetic code in a site-specific manner: (1) amber codon suppression; (2) rare sense codon reassignment; and (3) quadruplet codon. Figure 1 provides a schematic illustration of each method. Because organisms such as E. coli BL21 rarely use the amber stop codon (UAG) (only 275 of 4160 stop codons in BL21 are amber codons), which minimizes disturbances to existing protein termination signals, this codon has been preferably selected for NCAA encoding [ 30 , 39 , 40 ]. To enhance the efficiency of amber codon recognition by the orthogonal tRNA CUA , Release Factor 1 [ 41 , 42 ] is usually mutated or knocked out [ 43 ], thus enabling orthogonal tRNA CUA to recognize and increase its competitive binding to the amber codon [ 30 ] ( Figure 1 a). The role of different artificial tRNA/tRNA synthetase pairs, as well as their structural relationship with different NCAAs, were extensively reviewed by Anaëlle et al. [ 44 ].

An overview of approaches to incorporate NCAAs into specific sites. ( a ) The wild-type release factor is mutated or knocked out, allowing the newly introduced tRNA CUA to read through the stop codon, followed by NCAA incorporation with assistance from the compatible aminoacyl-tRNA synthetase. ( b ) The tRNA and corresponding tRNA synthetase for a rare sense codon are genetically engineered to confer the ability to encode NCAA. ( c ) A single-base is inserted after the canonical codon (e.g. “CUC” for Leu). The newly introduced quadruplet tRNA (e.g., tRNA AGAG ) can encode NCAA by targeting the quadruplet codon “CUCU.”
Rare sense codon assignment [ 45 , 46 , 47 ], which is based on a similar principle, repurposes rare sense codons, particularly rare codons including AGG [ 45 , 46 ] and AUA [ 47 ], using newly designed tRNA/aminoacyl-tRNA synthetase pairs. In this method, the introduction of a NCAA during protein synthesis requires either competition between a genetically modified tRNA and the corresponding wild-type tRNA [ 45 ] or the inhibition of wild-type tRNA via the deletion of its tRNA synthetase [ 47 ] ( Figure 1 b).
To circumvent the limitations of reprogramming existing codons, some researchers have explored NCAA encoding via expansion of the genetic code using quadruplet codons [ 48 , 49 , 50 , 51 , 52 , 53 ]. In brief, a single-base (e.g., “U”) is inserted after a canonical triplet codon (e.g., a “CUC” triplet codon) to form a frameshift mutation at the specific position ( Figure 1 c). The additional base also creates a new quadruplet codon (e.g., “CUCU”) at this position, which can be recognized by an engineered quadruplet tRNA (e.g., tRNA AGAG ). Early versions of the quadruplet in vivo coding system were initially tested in E. coli [ 48 , 51 , 52 ], followed by Xenopus oocytes [ 49 ] and mammalian cells [ 50 , 53 ]. It is also worth mentioning that noncanonical RNA translations, such as the use of tetra- and penta-codon, were observed in mitochondria; however, the 4th and 5th nucleotides were found to be silent during translation [ 54 ]. More mechanistic studies would be required to establish their roles in genetic code engineering.
Although the site-specific incorporation approach is arguably the most widely used to produce artificial proteins with NCAAs, some challenges can limit the stability of the engineered code. The efficiency of an engineered tRNA/aminoacyl-tRNA synthetase pair must be high enough to minimize the generation of truncated proteins [ 55 ]. Methods such as orthogonal ribosome use can lead to a threefold improvement in the efficiency of unnatural amino acid incorporation [ 55 ]. Endogenous tRNA/aminoacyl-tRNA synthetase pairs can also be engineered to incorporate unnatural amino acids. For example, by changing the phenylalanyl-tRNA synthetase amino acid recognition site, phenylalanine analogs such as p-Cl-phenylalanine or p-Br-phenylalanine can be successfully charged to tRNA Phe [ 14 , 56 ]. Advances in genome editing techniques, such as multiplex automated genomic engineering [ 31 ] and CRISPR/Cas [ 51 ], may further increase the efficiency and accuracy of NCAA incorporation in specific sites of the proteome.
2.2. Proteome-Wide Incorporation of NCAAs
Proteome-wide incorporation offers an alternative approach toward unnatural amino acid incorporation. In the most common approach, amino acid uptake is artificially controlled by feeding auxotrophs with NCAAs [ 33 , 34 , 35 ] ( Figure 2 a). Attempts to control NCAA synthesis have involved supplying organisms with NCAA precursors [ 57 , 58 , 59 , 60 , 61 , 62 ] ( Figure 2 b), which is also known as metabolic engineering [ 63 , 64 ]. In one example, the precursor l -β-thieno [3,2-b]pyrrolyl ([3,2]Trp) was fed to a tryptophan (Trp)-auxotrophic E. coli capable of synthesizing [3,2]Tpa (a Trp analog) to generate mutants that could propagate on l -β-(thieno [3,2-b]pyrrolyl)alanine ([3,2]Tpa) [ 57 ] ( Figure 2 b). Although directly feeding auxotrophs with NCAAs is a simpler approach, metabolic engineering could reduce the unwanted effects of impure commercial NCAAs [ 57 ].

An overview of proteome-wide approaches to incorporate NCAAs. ( a ) The NCAA enters a cell via membrane transporters or diffusion across the membrane. ( b ) The NCAA precursor similarly enters a cell in which it will be used to synthesize NCAAs. Following several generations of propagation with either the NCAA or its precursor, cells that can stably utilize the NCAA are selected.
Regardless of approach, the incorporation of NCAAs in the proteome may negatively affect the growth of an organism. The inherent toxicities of many unnatural amino acids could suppress propagation of the wild-type strain and select mutants that respond favorably to the NCAA, ultimately causing rejection of the expanded genetic code [ 34 , 35 ]. In the following section, we will focus on the challenges in genetic code modification.
3. Challenges of Genetic Code Engineering
3.1. inhibitory effects of engineered genetic codes.
The growth inhibitory effects caused by NCAAs, which have been demonstrated in different species including bacteria [ 65 , 66 , 67 ], yeasts [ 68 ], insects [ 69 , 70 ], and mammals [ 71 ], comprise one major challenge encountered during genetic code modification. The inhibitory effects of NCAAs are mainly attributable to two aspects. First, minor structural and chemical differences between NCAAs and their canonical counterparts can drastically affect enzymatic activities [ 72 , 73 , 74 , 75 ]. Second, these structural and chemical differences may also negatively affect protein synthesis, as some NCAAs cannot be efficiently charged to tRNAs by aminoacyl-tRNA synthetases [ 15 , 76 ]. A better understanding of the key genes and cellular responses associated with these modified genetic codes is of paramount importance to alleviating these inhibitory effects.
3.2. Discovering the Key Genes Controlling the Genetic Code
The growth inhibitory potentials of NCAAs create negative selective pressure, while the organism adapts to the modified genetic code. One effective strategy for overcoming this evolutionary barrier comprises an increase in the mutation rate via mutagenesis with the expectation of generating beneficial mutations that would favor the NCAA. Wong and colleagues isolated mutants from a Trp auxotroph ( Bacillus subtilis str. QB928) via sequential mutagenesis in an early attempt to modify the genetic code. The resultant HR23 strain could propagate indefinitely on 4-fluoro-tryptophan (4FTrp) but became inviable on canonical Trp [ 34 , 35 ]. As Trp is encoded by a single codon (UGG), the research by Wong and colleagues provided the first evidence of codon membership malleability under external selection pressure. Subsequently, Yu et al. traced mutations in intermediate mutants, as well as the HR23 strain [ 77 ]. A nonsense mutation in the Trp operon RNA-binding attenuation protein (TRAP), which controls transcriptional attenuation of the Trp operon [ 78 , 79 ] and translational repression of Trp transporters [ 80 , 81 , 82 , 83 ], was shared by all mutants. This lack of TRAP would increase 4-FTrp uptake to compensate for the relatively low charge rate of 4-FTrp to tRNA Trp [ 15 ].
In a separate attempt, Bacher et al. isolated E. coli mutants that could propagate in medium wherein 4-FTrp comprised ~99% of available Trp. However, the mutant strains could not grow indefinitely under these conditions and required minimal canonical Trp [ 33 ]. E. coli mutants were found to harbor several mutations affecting genes such as aroP , which encodes an aromatic amino acid transporter [ 84 ], and tyrR , which encodes the associated regulator [ 85 ]. Mutated aroP and tyrR might cooperatively increase 4-FTrp uptake, similar to the effect of TRAP knockout in B. subtilis. Taken together, these findings suggest that an efficient NCAA uptake system is essential to accommodation of the modified genetic codes.
RNA polymerase might also play a key role in controlling the genetic code. The above-mentioned B. subtilis mutant HR23 was found to harbor a nonsynonymous mutation in the RNA polymerase subunit gene ( rpoB ) that was absent from all other intermediate strains that could still propagate on Trp, suggesting a potential role for this mutation in switching membership of the UGG codon from Trp to 4-FTrp [ 77 ]. In an independent study of amber codon-directed 3-iodotyrosine (3-iodoTyr) incorporation in E. coli , a rpoB mutation was found to confer rifampicin resistance via amber suppression at Gln513 [ 86 ], and the same research group also engineered a bacteriophage, T7, that could incorporate 3-iodoTyr at amber codons [ 29 ]. In that study, Hammerling et al. observed high mutation frequencies in genes encoding RNA polymerase and the lysis timing regulator type II holin. The authors suggested that these two genes played important roles in the evolution of the expanded genetic code [ 29 ]. These studies have shed light on the previously unexplored roles of key genes in genetic code identity.
3.3. Lack of Transcriptomic and Proteomic Studies Related to Engineered Genetic Codes
In addition to mutations, gene and protein expression profiles might also reveal key factors needed to fine-tune the use of modified genetic codes. Technologies such as RNA-seq and mass spectrometry can be used to investigate the cellular responses of organisms in high resolution. RNA-seq was used to compare the cellular responses between mutant (grown on 4-FTrp) and wild-type strains of the above-mentioned B. subtilis HR23 mutant (unpublished data). Here, a gene ontology analysis of the gene expression profiles of these strains demonstrated enrichment of genes related to reactive oxygen species responses and branched-chain amino acid biosynthetic processes among upregulated genes, and enrichment of genes related to siderophore biosynthetic processes among downregulated genes (unpublished data). Unsurprisingly, stress response genes were modulated in response to the new genetic code, and the downregulation of siderophore biosynthetic process related genes was consistent with a previous observation of the reduced growth rate of HR23 cells grown on 4-FTrp [ 77 ] because iron homeostasis is closely related to bacterial growth [ 87 ]. This unique set of data was the first to demonstrate the adaptation of an organism to a new genetic code at the transcriptomic level.
Methanosarcina acetivorans is a methanogenic archaea strain that uses the alternative genetic codon UAG to encode pyrrolysine (Pyl) [ 88 ]. O’Donoghue et al. attempted to reduce the genetic code of this strain by deleting tRNA Pyl , thus blocking the incorporation of Pyl in the proteome. A comparison of the proteomes of mutant and wild-type M. acetivorans strains revealed that most upregulated peptides were related to methanogenesis, protein synthesis, and the stress response [ 89 ], suggesting that, in this organism, various stress response genes must be fine-tuned before a reduced genetic code can be used.
Very few transcriptomic and proteomic studies of organisms with modified genetic codes have been conducted, and we have only glimpsed the potential factors involved in adaptation to modified genetic codes. Additional genes that contribute to this adaptation might remain to be discovered. In the future, studies of gene and protein expression in organisms with modified genetic codes will be necessary.
3.4. Environmental Factors Affecting Adaptation to Engineered Genetic Codes
Environmental factors, such as the growth medium and selection method, are important when optimizing the use of a modified genetic code. The amino acid source is the first and most obvious factor, as an organism can either take up NCAAs directly from the environment or synthesize them using environmentally available molecules. If the source of NCAAs is from the environment, mutations in amino acid transporters are often needed to facilitate NCAA uptake [ 33 , 77 ].
Positive selection pressure is also needed to maintain stability of the modified genetic code. In a previous study, incorporation of the methionine analog azidohomoalanine (Aha) into the coat protein of a human adenovirus and the subsequent addition of a folate group to Aha facilitated adenoviral infection in mouse hosts [ 90 ]. In other words, adenovirus strains that can use modified Aha have a selective survival advantage over other strains. In a more recent study of different E. coli strains, the site-specific incorporation of two tyrosine analogs in β-lactamase was selected, and enzymatic function was found to depend on the presence of these analogs [ 91 ]. As described above regarding adenovirus, E. coli mutants that could utilize NCAAs enjoyed a selective advantage under growth mediums containing certain classes of antibiotics [ 91 ]. In one interesting example, even the carbon source may affect the selection of genetic codes by the Pyl-utilizing bacteria Acetohalobium arabaticum [ 92 ]. A. arabaticum used the standard genetic code when grown on pyruvate, but gained the ability to use an expanded genetic code that included Pyl in the presence of the alternative carbon source trimethylamine [ 92 ].
4. Future Directions
Current efforts in genetic code engineering have reshaped our ideas regarding genetic code evolution and have paved the way for expanding the genetic alphabet. Based on these studies, we have outlined the key steps by which an organism accommodates a modified genetic code ( Figure 3 ). During adaptation, mutations in amino acid transporters and/or their key regulators allow more efficient NCAA uptake, possibly by increasing the number of amino acid transporters [ 33 , 77 ]. Mutations in the key genes might also favor the use of a modified genetic code [ 29 , 33 , 77 , 86 ]. Additionally, environmental positive selection forces contribute to stability of the modified genetic code [ 77 , 91 , 92 ]. Currently, the genomic changes in organisms with modified genetic codes have been well explored [ 29 , 33 , 77 , 86 ] relative to transcriptomic (unpublished data) and proteomic [ 89 ] changes. Future trends in elucidation of the biological mechanisms underlying genetic code modifications include the integration of genomic, transcriptomic, and proteomic data and the refining of functional study targets.

Key steps in the accommodation of a modified genetic code.
From the viewpoint of synthetic biology and xenobiology, genetic code engineering increases the repertoire of building blocks available for protein engineering, thus enabling the development of novel proteins that would be impossible with canonical amino acids [ 38 , 93 ]. Xenobiology is an emerging field that involves synthesizing xenonucleic acids other than the canonical nucleic acids with adenine (A), thymine (T), cytosine (C), and guanine (G) as bases, with alternative pairing rules for protein engineering [ 94 ]. It has been demonstrated experimentally that two such xenonucleic acids can be integrated into the current DNA backbone [ 95 , 96 , 97 ], and more have been tested for their potentials as novel building blocks of DNA [ 98 ]. With the addition of xenonucleic acids, the number of encoded amino acids is likely to be increased to far beyond 20 [ 94 ].
High-throughput genome editing technologies, such as MAGE [ 36 ] and the emerging CRISPR/Cas technology [ 99 ], allow an organism’s genetic code to be directly rewritten [ 37 , 100 ] and facilitate the creation of synthetic life [ 101 ]. Although the first synthetic minimal bacterial genome still uses the standard genetic code [ 101 ], it is now possible to synthesize genomes based on alternative genetic codes. A full exploration of the possibilities enabled by genetic code engineering requires an understanding of the key molecular biological and biochemical mechanisms underlying the modifications. Gradual efforts to address this main question may improve our understanding of the process of genetic code evolution and lay a better foundation for future synthetic biology research.
Acknowledgments
This work is partially supported by a CUHK Direct Grant 4053041, the Lo Kwee-Seong Biomedical Research Fund, Lee Hysan Foundation, and the Partner State Key Laboratory of Agrobiotechnology, The Chinese University of Hong Kong to T.F.C.
Author Contributions
X.L., A.C.S.Y., and T.F.C. wrote the paper.
Conflicts of Interest
The authors declare no conflicts of interest.
Evolution of the Genetic Code
- First Online: 10 May 2024
Cite this chapter
- Marcello Barbieri 7
Part of the book series: Biosemiotics ((BSEM,volume 29))
120 Accesses
The genetic code is the set of rules that all living cells use for the synthesis of proteins, and to this purpose they employ three different types of RNAs: the messenger-RNAs, the transfer-RNAs and the ribosomal-RNAs. The messenger-RNAs carry genetic information in the form of sequences of codons, where the codons are groups of three nucleotides. The transfer-RNAs transport amino acids to the place of protein synthesis and contain anticodons that recognize the codons of the messenger-RNAs. The ribosomal-RNAs are the molecules that actually stick amino acids together in the order provided by the messenger-RNAs.
This is a preview of subscription content, log in via an institution to check access.
Access this chapter
Subscribe and save.
- Get 10 units per month
- Download Article/Chapter or eBook
- 1 Unit = 1 Article or 1 Chapter
- Cancel anytime
- Available as PDF
- Read on any device
- Instant download
- Own it forever
- Available as EPUB and PDF
- Durable hardcover edition
- Dispatched in 3 to 5 business days
- Free shipping worldwide - see info
Tax calculation will be finalised at checkout
Purchases are for personal use only
Institutional subscriptions
Ashby WR (1962) Principles of the self-organizing system. In: Von Foerster H, Zopf GW Jr (eds) Principles of self-organization: transactions of the University of Illinois Symposium. Pergamon Press, London, pp 255–278
Google Scholar
Barbieri M (2019) Evolution of the genetic code. The ambiguity-reduction theory. Biosystems 185:1–7. https://doi.org/10.1016/j.biosystems.2019.104024
Article CAS Google Scholar
Bollenbach T, Kalin Vetsigian K, Kishony R (2007) Evolution and multilevel optimization of the genetic code. Genome Res 17:401–404
Article CAS PubMed Google Scholar
Burks AW (1970) Essays on cellular automata. University of Illinois Press, Urbana
Cech TR (1983) RNA splicing: three themes with variations. Cell 34:713–716
Cech TR (1986) RNA as an enzyme. Sci Am 255:64–75
Crick FHC (1966) Codon–anticodon pairing: the wobble hypothesis. J Mol Biol 19:548–555
Di Giulio M (2008) An extension of the coevolution theory of the origin of the genetic code. Biol Direct 3:1–37
Article Google Scholar
Drummond DA, Wilke CO (2008) Mistranslation-induced protein misfolding as a dominant constraint on coding-sequence evolution. Cell 134:341–352
Article CAS PubMed PubMed Central Google Scholar
Fox GE (2010) Origin and evolution of the ribosome. Cold Spring Harb Perspect Biol 2:a003483
Article PubMed PubMed Central Google Scholar
Freeland SJ, Hurst LD (1998) The genetic code is one in a million. J Mol Evol 47:238–248
Gilbert W (1986) The RNA world. Nature 319:618
Gilis D, Massar S, Cerf NJ, Rooman M (2001) Optimality of the genetic code with respect to protein stability and amino-acid frequencies. Genome Biol 2:41–49
Guerrier-Takada C, Altman S (1984) Catalytic activity of an RNA molecule prepared by transcription in vitro. Science 223:285–286
Guerrier-Takada C, Gardiner K, Marsh T, Pace N, Altman S (1983) The RNA moiety of ribonuclease P is the catalytic subunit of the enzyme. Cell 35:849–857
Haig D, Hurst LD (1991) A quantitative measure of error minimization in the genetic code. J Mol Evol 33:412–417
Harold FM (2014) In search of cell history. The evolution of life’s building blocks. The University of Chicago Press, Chicago/London
Book Google Scholar
Higgs PG, Pudritz RE (2007) From protoplanetary disks to prebiotic amino acids and the origin of the genetic code. In: Pudritz RE, Higgs PG, Stone J (eds) Planetary systems and the origins of life, Cambridge series in astrobiology, vol 3. Cambridge University Press
Itzkovitz S, Alon U (2007) The genetic code is nearly optimal for allowing additional information within protein-coding sequences. Genome Res 17:405–412
Koonin EV, Martin W (2005) On the origin of genomes and cells within inorganic compartments. Trends Genet 21:647–654
Kurland CG (1970) Ribosome structure and function emergent. Science 169:1171–1177
Mulkidjanian AY, Makarova KS, Galperin MY, Koonin EV (2007) Inventing the dynamo machine: the evolution of the F-type and V-type ATPases. Nat Rev Microbiol 5:892–899
Ninio J (1982) Molecular approaches to evolution. Pitman books, London
Nitta I, Kamada Y, Noda H, Ueda T, Watanabe K (1998) Reconstitution of peptide bond formation. Science 281:666–669
Novozhilov AS, Wolf YI, Koonin EV (2007) Evolution of the genetic code: partial optimization of a random code for robustness to translation error in a rugged fitness landscape. Biol Direct 2(24)
Osawa S (1995) Evolution of the genetic code. Oxford University Press, Oxford/New York
Petrov AS et al (2015) History of the ribosome and the origin of translation. Proc Natl Acad Sci USA 112:15396–15401. https://doi.org/10.1073/pnas.159761112
Rodin SN, Ohno S (1995) Two types of aminoacyl-tRNA synthetases could be originally encoded by complementary strands of the same nucleic acid. Orig Life Evol Biosph 25(6):565–589
Vetsigian K, Woese C, Goldenfeld N (2006) Collective evolution and the genetic code. Proc Natl Acad Sci USA 103(28):10696–10701
Woese CR (1965) Order in the genetic code. Proc Natl Acad Sci USA 54:71–75
Woese CR (1987) Bacterial evolution. Microbiol Rev 51:221–271
Woese CR (2000) Interpreting the universal phylogenetic tree. Proc Natl Acad Sci USA 97:8392–8396
Woese CR (2002) On the evolution of cells. Proc Natl Acad Sci USA 99:8742–8747
Woese CR, Dugre DH, Saxinger WC, Dugre SA (1966) The molecular basis for the genetic code. Proc Natl Acad Sci USA 55:966–974
Wolf YI, Koonin EV (2007) On the origin of the translation system and the genetic code in the RNA world by means of natural selection, exaptation, and subfunctionalization. Biol Direct 2:14
Wong JTF (1981) Coevolution of genetic code and amino acid biosynthesis. TIBS 6:33–36
CAS Google Scholar
Wong JT, Bronskill PM (1979) Inadequacy of prebiotic synthesis as origin of proteinous amino acids. J Mol Evol 13:115–125
Download references
Author information
Authors and affiliations.
Dipto. di Morfologia ed Embriologia, University of Ferrara, Ferrara, Italy
Marcello Barbieri
You can also search for this author in PubMed Google Scholar
Rights and permissions
Reprints and permissions
Copyright information
© 2024 The Author(s), under exclusive license to Springer Nature Switzerland AG
About this chapter
Barbieri, M. (2024). Evolution of the Genetic Code. In: Codes and Evolution. Biosemiotics, vol 29. Springer, Cham. https://doi.org/10.1007/978-3-031-58484-8_7
Download citation
DOI : https://doi.org/10.1007/978-3-031-58484-8_7
Published : 10 May 2024
Publisher Name : Springer, Cham
Print ISBN : 978-3-031-58483-1
Online ISBN : 978-3-031-58484-8
eBook Packages : Biomedical and Life Sciences Biomedical and Life Sciences (R0)
Share this chapter
Anyone you share the following link with will be able to read this content:
Sorry, a shareable link is not currently available for this article.
Provided by the Springer Nature SharedIt content-sharing initiative
- Publish with us
Policies and ethics
- Find a journal
- Track your research

Deciphering the Genetic Code: A 50 Year Anniversary
By Christie Moffatt

Fifty years ago, on January 18, 1965, Dr. Marshall W. Nirenberg (1927–2010) completed his first summary of the genetic code —one of the most significant documents in the history of twentieth-century science—a painstaking, handwritten chart of the discovery of how sequences of DNA, known as “triplets,” direct the assembly of amino acids into the structural and functional proteins essential to life. The chart is included in the Marshall W. Nirenberg Papers , held at the National Library of Medicine. Preserving this genetic code chart has been an important and exciting challenge for NLM conservators, as they study the best way to store this important document written on multiple sheets of paper in pencil, India ink, and multiple ball-point pen inks, and held together with adhesive tape.
The data provided in this summary was compiled beginning in 1961 after Dr. Nirenberg presented, in a now-famous speech given to a small group of speech about 30 scientists at the International Congress of Biochemistry in Moscow, the results of his “ poly-U experiments ” conducted with post-doctoral researcher J. Heinrich Matthaei. Francis Crick, who was in attendance at the Moscow meeting, had heard that Nirenberg and Matthaei had found a clue that might unravel one of the central mysteries of molecular genetics. Crick arranged to have the young scientist deliver his paper again, this time to the assembled body of about a thousand people. Many scientists–especially the 1959 Nobel Laureate Severo Ochoa–were eager to take it to the next level. Thus began a research race with some of the world’s most famous (and well-funded) molecular geneticists. Over the course of the next five years, Nirenberg worked steadily with a team of about twenty postdoctoral researchers and laboratory technicians, including Norma Heaton, who remained a member of Nirenberg’s team at the National Heart, Blood, and Lung Institute for forty years. Dr. DeWitt “Hans” Stetten, former director of the National Institute of General Medical Sciences, called this period of time “the finest hour of the NIH.”
In 2006, Dr. Nirenberg provided a description of this first summary chart:
“The DNA that we inherit from our parents contains the information that is needed to make the more than 100,000 kinds of proteins that are the molecular machinery of the body. DNA consists of four kinds of [nucleotides represented by the] letters, T, C, A and G, in long sequences. DNA is transcribed to RNA that also consists of long sequences of four letters, U, C, A, and G. The sequence of letters in RNA determines the sequence of amino acids in proteins. There are 64 kinds of 3 letter words or triplets in RNA. When we deciphered the genetic code, we determined the sequence of letters in each RNA triplet that corresponds to each kind of amino acid in protein. All forms of life on this planet use the same genetic code, although small differences in the code have been found in some organisms.
This is the first summary of the genetic code that I made on Jan. 18, 1965, when we had deciphered more than half of the code. The columns on the left and right of the chart are the 64 RNA triplets. The row across the top, ALA, ARG, ASP, etc., are the 20 amino acids. The numbers in the chart correspond to the amount of radioactive aminoacyl-tRNA that bound to a triplet on ribosomes. The second column on the right-hand side contains the tentative amino acid-triplet assignments that we made at that time. Some of the tentative assignments were changed when we obtained more data, and all of the assignments that we published were correct.”

Throughout 1965, there would be several versions of the chart created to incorporate new findings from the many experiments conducted during this intense period of discovery. A second and last summary of the code was prepared at Dr. Nirenberg’s request by his technician, Norma Heaton, in late 1965 or early 1966, when almost all of the 64 codons had been deciphered. In an oral history interview from 2010 Norma Heaton remembers:

“I do not even know if the term spreadsheet had been invented then. It got to the point where we knew we needed to have some sort of means of seeing it all in one big picture. So I taped data paper together and drew the columns and rows with a pen and ruler…
One of my memories…is that I can remember being in the room, and Mert Bernfield and Edward M. Scolnick—Ed went on to be vice president of Merck.…They were both kind of, I don’t want to say loud, but they were not quiet, soft-spoken people. One of my memories is, they would hang over the [experiment], and they would have the tape and they would say, “Which one is this? Which one is this?” Then you would hear this shout, like, “Oh, we discovered a new one.””
Dr. Nirenberg would go on to win the Nobel Prize in Physiology or Medicine in 1968 for this work, sharing the award with Robert W. Holley of Cornell University and Har Gobind Khorana of the University of Wisconsin at Madison “for their interpretation of the genetic code and its function in protein synthesis.” He began donating his papers to NLM in 1999, and since then nearly 500 selections from the Marshall Nirenberg Papers have been digitized and made available on NLM’s Profiles in Science . Visitors can access archival materials related to the code, including pages from Dr. Nirenberg’s laboratory notebooks , photographs of Dr. Nirenberg in his laboratory , correspondence with other scientists working on the code, such as Francis Crick , and the full 2010 oral history interview with Norma Heaton, in which she recalls the technical challenges of the research, and more broadly, what it was like to work in Dr. Nirenberg’s laboratory during this time.

On March 17, 2015 at 1:00, NLM will host a special two-hour program, “A Tribute to Marshall Nirenberg.” The program marks the 50th anniversary of Nirenberg’s Genetic Code Charts—groundbreaking scientific documents now held in NLM’s historical collections. Marshall Nirenberg’s Nobel Prize medal and certificate, newly given to NLM through the generous donation of his wife, Dr. Myrna Weissman, will be on display. Dr. Frank Portugal, author of The Least Likely Man: Marshall Nirenberg and the Discovery of the Genetic Code (MIT Press), and Dr. David Serlin, historian and curator of NLM’s Profiles in Science site on the Marshall Nirenberg Papers , will speak at the event. For more information and directions please visit our 2015 lectures page . Subsequent NIH events will be announced soon.
This article is part of a series that commemorates the 50th anniversary of the Genetic Code Charts. Stay tuned throughout the year to learn more about Marshall Nirenberg and these groundbreaking documents.

Circulate this:
- Click to share on Twitter (Opens in new window)
- Click to share on Facebook (Opens in new window)
- Click to share on Pinterest (Opens in new window)
- Click to share on Tumblr (Opens in new window)
- Click to share on Reddit (Opens in new window)
- Click to share on LinkedIn (Opens in new window)
- Click to email a link to a friend (Opens in new window)
- Click to print (Opens in new window)
- Click to share on WhatsApp (Opens in new window)
- Click to share on Pocket (Opens in new window)
- Click to share on Telegram (Opens in new window)
- Pingback: Revealing Data: Explorations of Data in Collections – Circulating Now from NLM
- Pingback: An Airtight Case: Custom Fabrication for a Medical History Treasure – Circulating Now from NLM
- Pingback: Molecular Biology Behind the Blackboard – Circulating Now from NLM
- Pingback: The History of Genomics Program at 10 Years – Circulating Now from NLM
- Pingback: Revealing Data: Visualizations in Historical Collections – Circulating Now from the NLM Historical Collections
- Pingback: Maxine Singer—A Life in Research and Advocacy – Circulating Now from the NLM Historical Collections
Leave a Reply Cancel reply
This site uses Akismet to reduce spam. Learn how your comment data is processed .
Discover more from Circulating Now from the NLM Historical Collections
Subscribe now to keep reading and get access to the full archive.
Type your email…
Continue reading
Genetic Code - Science topic

- Recruit researchers
- Join for free
- Login Email Tip: Most researchers use their institutional email address as their ResearchGate login Password Forgot password? Keep me logged in Log in or Continue with Google Welcome back! Please log in. Email · Hint Tip: Most researchers use their institutional email address as their ResearchGate login Password Forgot password? Keep me logged in Log in or Continue with Google No account? Sign up

An official website of the United States government
The .gov means it’s official. Federal government websites often end in .gov or .mil. Before sharing sensitive information, make sure you’re on a federal government site.
The site is secure. The https:// ensures that you are connecting to the official website and that any information you provide is encrypted and transmitted securely.
- Publications
- Account settings
- My Bibliography
- Collections
- Citation manager
Save citation to file
Email citation, add to collections.
- Create a new collection
- Add to an existing collection
Add to My Bibliography
Your saved search, create a file for external citation management software, your rss feed.
- Search in PubMed
- Search in NLM Catalog
- Add to Search
The genetic code
- PMID: 13882204
- DOI: 10.1038/scientificamerican1062-66
PubMed Disclaimer
Similar articles
- Overlapping genetic codes. WALL R. WALL R. Nature. 1962 Mar 31;193:1268-70. doi: 10.1038/1931268a0. Nature. 1962. PMID: 14004641 No abstract available.
- On the genetic code. CRICK FH. CRICK FH. Science. 1963 Feb 8;139(3554):461-4. doi: 10.1126/science.139.3554.461. Science. 1963. PMID: 14023853 No abstract available.
- [Some moments in the history of the nucleic acids. III. The history of the genetic code]. Eşanu V. Eşanu V. Virologie. 1989 Jul-Sep;40(3):201-19. Virologie. 1989. PMID: 2688297 French. No abstract available.
- [The nature of the genotype and heredity]. Rautian AS. Rautian AS. Zh Obshch Biol. 1993 Mar-Apr;54(2):131-48. Zh Obshch Biol. 1993. PMID: 8493814 Review. Russian.
- Mathematical fundamentals for the noise immunity of the genetic code. Fimmel E, Strüngmann L. Fimmel E, et al. Biosystems. 2018 Feb;164:186-198. doi: 10.1016/j.biosystems.2017.09.007. Epub 2017 Sep 14. Biosystems. 2018. PMID: 28918301 Review.
- THE LEUKEMIAS. PETRAKIS NL. PETRAKIS NL. Calif Med. 1964 Jul;101(1):33-41. Calif Med. 1964. PMID: 14179529 Free PMC article. Review. No abstract available.
- Search in MeSH
Related information
- Cited in Books
- Citation Manager
NCBI Literature Resources
MeSH PMC Bookshelf Disclaimer
The PubMed wordmark and PubMed logo are registered trademarks of the U.S. Department of Health and Human Services (HHS). Unauthorized use of these marks is strictly prohibited.
- Frontiers in Applied Mathematics and Statistics
- Mathematical Biology
- Research Topics
Mathematical and Computational Approaches to Study The Genetic Code
Total Downloads
Total Views and Downloads
About this Research Topic
The study of the mathematical structure of the genetic code, after an uproar in the 1990’s mostly inspired by group theory, extensively used in particle physics, is right now somewhat stagnating. Other mathematical techniques, such as number theory, graph theory, information theory, quantum groups, ...
Keywords : Genetic Code, Amino Acids, RNA, DNA, Symmetries, Patterns
Important Note : All contributions to this Research Topic must be within the scope of the section and journal to which they are submitted, as defined in their mission statements. Frontiers reserves the right to guide an out-of-scope manuscript to a more suitable section or journal at any stage of peer review.
Topic Editors
Topic coordinators, recent articles, submission deadlines.
Submission closed.
Participating Journals
Total views.
- Demographics
No records found
total views article views downloads topic views
Top countries
Top referring sites, about frontiers research topics.
With their unique mixes of varied contributions from Original Research to Review Articles, Research Topics unify the most influential researchers, the latest key findings and historical advances in a hot research area! Find out more on how to host your own Frontiers Research Topic or contribute to one as an author.
The McDonnell Genome Institute
Why it’s so hard to crack the genome regulatory code
A new massively parallel binding assay finds binding rules but they’re not always obeyed, august 6, 2024.
One of the important discoveries of 21st century biology is that only a small portion of our genome encodes genes, while much more of it encodes regulatory information that controls when and where genes are expressed. Unfortunately, unlike the genetic code that describes how genes are encoded (which was cracked in the 1960’s), the code for regulatory DNA has been much harder to work out. That’s a problem, because genetic variants in regulatory DNA have important effects on human health.
A paper published in Science in October of 2022 shows how these regulatory variants can affect our risk for disease, and why the regulatory code is so hard to figure out. With an impressively thorough set of experiments, the paper’s authors show how a single-nucleotide variant in a regulatory DNA sequence leads to a six-fold increase in the risk for a particular type of low-grade glioma, a slow-growing tumor that eventually develops into an aggressive form of brain cancer. The variant disrupts a binding site for a regulatory protein called OCT2, and thereby increases the expression of the Myc gene, which in turn has an impact on tumor growth.
It’s a nice piece of work, but if you ask genome scientists why disrupting this particular binding site changes Myc expression, they couldn’t tell you. The OCT2 binding site is the short sequence TCTGCAAT, and here is the problem: there are millions of these short OCT2 binding sequence motifs in the genome, and yet only a small fraction (1% or fewer) are actually bound by OCT2. What makes the OCT2-bound sites different from the vast excess of identical but unbound sites? The problem isn’t limited to OCT2, but holds for basically all of the regulatory proteins that function by recognizing short DNA sequences. To answer this question is what it means to solve the regulatory code.
In the latest issue of Molecular Cell , a paper (free preprint here ) introduces a clever new technology to get at the question of regulatory protein binding sites. The technology, called ChIP-ISO, is a variation on a common theme in functional genomics: rather than just test natural genomic sequences, it is much more effective to develop assays that can perturb sequences at scale. Such functional perturbation data is great for training models and for identifying functional differences among sequences, such as bound versus unbound transcription factor binding motifs.
ChIP-ISO, developed by Lu Bai’s lab at Penn State, is a way to test regulatory protein binding to thousands of binding site perturbations in one experiment. It involves (1) synthesizing a library of perturbed DNA sequences, (2) integrating that library into a population of cells at one location in the genome, and (3) measuring protein binding to those sequences via immunoprecipitation and sequencing (ChIP-seq).
With ChIP-ISO, the Bai lab analyzed binding of the protein FOXA1 to a regulatory DNA sequence containing three FOXA1 binding motifs (orange bars in the figure below). In the same sequence are a handful of other binding motifs for different regulatory proteins. Looking at a sequence like this, we have no good models of the regulatory code that tell us ahead of time which of these binding motifs is important. So what do the data say?

Transcription factor binding sites in the CCND1 enhancer analyzed by Xu, et al.
ChIP-ISO showed that FOXA1 binding site 3 has the biggest effect when deleted, and that deleting binding sites for two other factors, CEPB (green) and AP-1 (purple) also affect FOXA1 binding. In other words, FOXA1, CEPB, and AP-1 bind DNA cooperatively when their binding motifs are close together. But if cooperative binding is the rule, why isn’t there cooperative binding at FOXA1 sites 1 and 2, which are close to other binding motifs (light gray bars)? Nobody knows. Maybe the proteins that bind these sites aren’t expressed, or maybe those proteins don’t bind cooperatively with FOXA1. And what about thousands of other sites in the genome where FOXA1 binds, but AP-1 and CEPB do not? Or the millions of unbound FOXA1 binding motifs?

FOXA1 binding motif from HOCOMOCO
We don’t have good models that answer these questions, just a set of loose rules that seem to apply in some cases and not others. Our standard models for regulatory protein binding specificities are short motifs like the one above, and we have very little idea how they combine to create functional regulatory DNA elements. And if we can’t say why a regulatory DNA element works, we certainly can’t easily say why this genetic variant breaks it and not that one.
But with scalable methods like ChIP-ISO, we have a chance figuring this out, or at least building deep learning models that figure it out. Right now ChIP-ISO is limited to thousands of perturbations in an experiment, but if we scale up to millions, the models might figure it out.
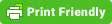
Information
- Author Services
Initiatives
You are accessing a machine-readable page. In order to be human-readable, please install an RSS reader.
All articles published by MDPI are made immediately available worldwide under an open access license. No special permission is required to reuse all or part of the article published by MDPI, including figures and tables. For articles published under an open access Creative Common CC BY license, any part of the article may be reused without permission provided that the original article is clearly cited. For more information, please refer to https://www.mdpi.com/openaccess .
Feature papers represent the most advanced research with significant potential for high impact in the field. A Feature Paper should be a substantial original Article that involves several techniques or approaches, provides an outlook for future research directions and describes possible research applications.
Feature papers are submitted upon individual invitation or recommendation by the scientific editors and must receive positive feedback from the reviewers.
Editor’s Choice articles are based on recommendations by the scientific editors of MDPI journals from around the world. Editors select a small number of articles recently published in the journal that they believe will be particularly interesting to readers, or important in the respective research area. The aim is to provide a snapshot of some of the most exciting work published in the various research areas of the journal.
Original Submission Date Received: .
- Active Journals
- Find a Journal
- Proceedings Series
- For Authors
- For Reviewers
- For Editors
- For Librarians
- For Publishers
- For Societies
- For Conference Organizers
- Open Access Policy
- Institutional Open Access Program
- Special Issues Guidelines
- Editorial Process
- Research and Publication Ethics
- Article Processing Charges
- Testimonials
- Preprints.org
- SciProfiles
- Encyclopedia
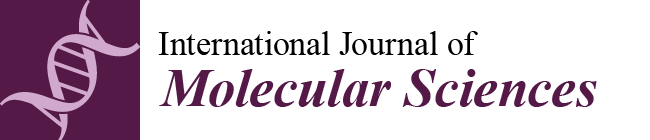
Article Menu
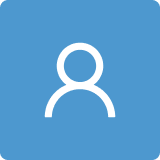
- Subscribe SciFeed
- Recommended Articles
- Google Scholar
- on Google Scholar
- Table of Contents
Find support for a specific problem in the support section of our website.
Please let us know what you think of our products and services.
Visit our dedicated information section to learn more about MDPI.
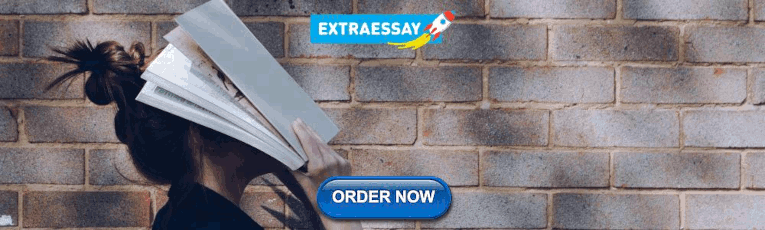
JSmol Viewer
Unveiling the secrets of oil palm genetics: a look into omics research, 1. introduction, 2. oil palm genomics, 2.1. oil palm genome sequencing, 2.2. research on molecular markers for important traits in oil palm.
Molecular Markers | Important Traits | References |
---|---|---|
SNP, SSR | oil content | [ ] |
SSR | hull thickness | [ ] |
SNP | stem height | [ ] |
SSR | drought-tolerant | [ ] |
SSR; | drought-tolerant | [ ] |
SNP | drought-tolerant | [ ] |
SSR | illegitimate progenies | [ ] |
SSR | identification of QTLs in oil palm | [ ] |
SSR | cold tolerance | [ ] |
SSR | identification of QTLs associated with callogenesis and embryogenesis in oil palm | [ ] |
EST-SSR | fruit exocarp colour | [ ] |
RFLP | genotypic discrimination | [ ] |
SNP | fatty acid content | [ ] |
SNP | height of oil palm | [ ] |
Intron polymorphisms (IP) | oil palm fatty acid composition | [ ] |
SSR | genetic diversity | [ , , ] |
SSR | sex ratio | [ ] |
SNP | vitamin E biosynthesis | [ ] |
SNP-CAPS | fruit traits | [ ] |
2.3. Gene Resource Mining and Function Analysis of Important Traits in Oil Palm
Gene Family | Function | Reference |
---|---|---|
Abiotic stress | ||
CBF | Increased tolerance to low temperatures | [ ] |
ABA | Enhance resistance to drought | [ ] |
bZIP | Highly expressed under low temperature, salinity, and drought stress conditions | [ ] |
AP2/ERF | Increased tolerance to salt, low temperatures, and drought | [ ] |
HRE2(ERF-VII) | HRE2 responds to flood-induced hypoxia | [ ] |
MYB | Increased tolerance to stresses such as salinity, cold, and drought | [ ] |
WRKY | Involved in abiotic stress responses such as drought, salinity, and heat | [ ] |
EgSPCH(bHLH) | EgSPCH produces more pores in response to salt stress | [ ] |
ARF | EgARF responds to abiotic stresses (cold, drought, and salt stress) | [ ] |
CAT | EgCAT enhances tolerance to low temperature, drought, and salt stress | [ ] |
Biotic stress | ||
CNL R gene | Expression in the early stages of oil palm defence mechanisms | [ ] |
AGLU1 RCH10 | Increasing oil palm resistance towards G. boninense | [ ] |
LCC | EgLCC24 gene detects oil palm seedlings resistant to G. boninense | [ ] |
Fatty acids | ||
MADS-box | Fruit development and oil accumulation | [ ] |
MAD | Mediating medium-chain fatty acid (MCFA) anabolism in oil palm mesocarp | [ ] |
GDSL | Enhanced lipid content | [ ] |
ACBP | Lipid accumulation | [ ] |
Others | ||
CAD | Lignin biosynthesis | [ ] |
3. Oil Palm Transcriptomics
Trait for Transcriptome Analysis | Transcription Factor | Summary | Reference |
---|---|---|---|
Somatic embryogenesis | FTIP, FRIGIDA-LIKE, and NF-YA, | The reproductive time of plants may be related to somatic embryogenesis potential | [ ] |
Drought | MYBs, HOXs, NF-Ys | A potential miRNA target gene for oil palm under water deficit conditions | [ ] |
Pi-deficiency | PHL7, NIP6–1 and 14–3-3 | Involved in the lack of regulation and domestication of oil palm Pi | [ ] |
Waterlogging | LBD41, HRA1, HRE2 | Respond to waterlogging | [ ] |
High somatic embryogenesis rate | LE, DDX28, HD-ZIP, NPF | The physiological state related to somatic embryogenesis potential was revealed | [ ] |
Bud Rot Disease | Clon34 and Clon57 | Control pathogen | [ ] |
Defoliation stress | / | Elucidating the mechanism of oil palm sex determination. | [ ] |
Bagworm stress | P450, GST and CCE AChE, nAChR, ACCase, GABA, RyR | Insecticide targets for detoxification and modification potential | [ ] |
Aluminium stress | DREB1F, NAC | Induces the expression of internal detoxification enzymes | [ ] |
Water deprivation | NF-YA3, HOX32, GRF1 | It plays a certain role in the response of oil palm seedlings to drought and salt stress. | [ ] |
Salt stress | MYC, G-Box, ABRE, TATA-box | Using identified genes to try to mitigate salt stress | [ ] |
G. boninense infection | target gene #5, #13, #16, #6 and #15, #1, #9, #12, and #14 | It may be related to early infection reaction to G. boninense | [ ] |
4. Oil Palm Proteomics
5. oil palm metabolomics, 6. conclusions and outlook, author contributions, conflicts of interest.
- Damayant, S.; Andry, S.; Khairurrijal; Kartasasmita, R.E. Isolation of β-carotene from Palm ( Elaeis guineensis Jacq.) Oil Using Transesterification-adsorption-desorption Method and its Characterization. J. Appl. Sci. 2014 , 14 , 2615–2621. [ Google Scholar ] [ CrossRef ]
- Babu, B.K.; Mathur, R.K.; Anitha, P.; Ravichandran, G.; Bhagya, H.P. Phenomics, genomics of oil palm ( Elaeis guineensis Jacq.): Way forward for making sustainable and high yielding quality oil palm. Physiol. Mol. Biol. Plants 2021 , 27 , 587–604. [ Google Scholar ] [ CrossRef ] [ PubMed ]
- Singh, R.; Ong-Abdullah, M.; Low, E.-T.L.; Manaf, M.A.A.; Rosli, R.; Nookiah, R.; Ooi, L.C.-L.; Ooi, S.E.; Chan, K.-L.; Halim, M.A.; et al. Oil palm genome sequence reveals divergence of interfertile species in Old and New worlds. Nature 2013 , 500 , 335–339. [ Google Scholar ] [ CrossRef ] [ PubMed ]
- Sundram, K.; Sambanthamurthi, R.; Tan, Y.A. Palm fruit chemistry and nutrition. Asia Pac. J. Clin. Nutr. 2003 , 12 , 355–362. [ Google Scholar ]
- Mba, O.I.; Dumont, M.-J.; Ngadi, M. Palm Oil: Processing, Characterization and Utilization in the Food Industry—A Review. Food Biosci. 2015 , 10 , 26–41. [ Google Scholar ] [ CrossRef ]
- Nainggolan, M.; Sinaga, A.G. Characteristics of fatty acid composition and minor constituents of red palm olein and palm kernel oil combination. J. Adv. Pharm. Technol. Res. 2021 , 12 , 22–26. [ Google Scholar ] [ CrossRef ]
- Barcelos, E.; Rios Sde, A.; Cunha, R.N.; Lopes, R.; Motoike, S.Y.; Babiychuk, E.; Skirycz, A.; Kushnir, S. Oil palm natural diversity and the potential for yield improvement. Front. Plant Sci. 2015 , 6 , 190. [ Google Scholar ] [ CrossRef ]
- Rival, A.; Beule, T.; Barre, P.; Hamon, S.; Duval, Y.; Noirot, M. Comparative flow cytometric estimation of nuclear DNA content in oil palm ( Elaeis guineensis Jacq) tissue cultures and seed-derived plants. Plant Cell Rep. 1997 , 16 , 884–887. [ Google Scholar ] [ CrossRef ] [ PubMed ]
- Pellicer, J.; Leitch, I.J. The Plant DNA C-values database (release 7.1): An updated online repository of plant genome size data for comparative studies. New Phytol. 2020 , 226 , 301–305. [ Google Scholar ] [ CrossRef ]
- Jin, J.; Lee, M.; Bai, B.; Sun, Y.; Qu, J.; Rahmadsyah; Alfiko, Y.; Lim, C.H.; Suwanto, A.; Sugiharti, M.; et al. Draft genome sequence of an elite Dura palm and whole-genome patterns of DNA variation in oil palm. DNA Res. 2016 , 23 , 527–533. [ Google Scholar ] [ CrossRef ]
- Utomo, C.; Tanjung, Z.A.; Aditama, R.; Buana, R.F.N.; Pratomo, A.D.M.; Tryono, R.; Liwang, T. Draft Genome Sequence of the Phytopathogenic Fungus Ganoderma boninense , the Causal Agent of Basal Stem Rot Disease on Oil Palm. Am. Soc. Microbiol. 2018 , 6 . [ Google Scholar ] [ CrossRef ] [ PubMed ]
- Ong, A.-L.; Teh, C.-K.; Mayes, S.; Massawe, F.; Appleton, D.R.; Kulaveerasingam, H. An Improved Oil Palm Genome Assembly as a Valuable Resource for Crop Improvement and Comparative Genomics in the Arecoideae Subfamily. Plants 2020 , 9 , 1476. [ Google Scholar ] [ CrossRef ] [ PubMed ]
- Mohd Sanusi, N.S.N.; Rosli, R.; Chan, K.-L.; Halim, M.A.A.; Ting, N.-C.; Singh, R.; Low, E.-T.L. Integrated consensus genetic map and genomic scaffold re-ordering of oil palm ( Elaeis guineensis ) genome. Comput. Biol. Chem. 2023 , 102 , 107801. [ Google Scholar ] [ CrossRef ] [ PubMed ]
- Sanusi, N.S.N.M.; Rosli, R.; Halim, M.A.A.; Chan, K.-L.; Nagappan, J.; Azizi, N.; Amiruddin, N.; Tatarinova, T.V.; Low, E.-T.L. PalmXplore: Oil palm gene database. Database 2018 , 2018 , bay095. [ Google Scholar ] [ CrossRef ] [ PubMed ]
- Uthaipaisanwong, P.; Chanprasert, J.; Shearman, J.R.; Sangsrakru, D.; Yoocha, T.; Jomchai, N.; Jantasuriyarat, C.; Tragoonrung, S.; Tangphatsornruang, S. Characterization of the chloroplast genome sequence of oil palm ( Elaeis guineensis Jacq.). Gene 2012 , 500 , 172–180. [ Google Scholar ] [ CrossRef ] [ PubMed ]
- Jaligot, E.; Rival, A.; Beulé, T.; Dussert, S.; Verdeil, J.L. Somaclonal variation in oil palm ( Elaeis guineensis Jacq.): The DNA methylation hypothesis. Plant Cell Rep. 2000 , 19 , 684–690. [ Google Scholar ] [ CrossRef ]
- Low, E.T.; Rosli, R.; Jayanthi, N.; Mohd-Amin, A.H.; Azizi, N.; Chan, K.L.; Maqbool, N.J.; Maclean, P.; Brauning, R.; McCulloch, A.; et al. Analyses of hypomethylated oil palm gene space. PLoS ONE 2014 , 9 , e86728. [ Google Scholar ] [ CrossRef ] [ PubMed ]
- Ong-Abdullah, M.; Ordway, J.M.; Jiang, N.; Ooi, S.-E.; Kok, S.-Y.; Sarpan, N.; Azimi, N.; Hashim, A.T.; Ishak, Z.; Rosli, S.K.; et al. Loss of Karma transposon methylation underlies the mantled somaclonal variant of oil palm. Nature 2015 , 525 , 533–537. [ Google Scholar ] [ CrossRef ] [ PubMed ]
- Sarpan, N.; Taranenko, E.; Ooi, S.-E.; Low, E.-T.L.; Espinoza, A.; Tatarinova, T.V.; Ong-Abdullah, M. DNA methylation changes in clonally propagated oil palm. Plant Cell Rep. 2020 , 39 , 1219–1233. [ Google Scholar ] [ CrossRef ]
- Ooi, S.-E.; Choo, C.-N.; Sarpan, N.; Wong, C.-K.; Wong, W.-C. Karma - EgDEF1 methylation in Elaeis guineensis clonal mother palms that produced high mantling rates in the second clonal generation. Vitr. Cell. Dev. Biol. Plant 2024 , 60 , 176–182. [ Google Scholar ] [ CrossRef ]
- Billotte, N.; Marseillac, N.; Risterucci, A.M.; Adon, B.; Brottier, P.; Baurens, F.C.; Singh, R.; Herrán, A.; Asmady, H.; Billot, C.; et al. Microsatellite-based high density linkage map in oil palm ( Elaeis guineensis Jacq.). Theor. Appl. Genet. 2005 , 110 , 754–765. [ Google Scholar ] [ CrossRef ] [ PubMed ]
- Jaligot, E.; Beulé, T.; Rival, A. Methylation-sensitive RFLPs: Characterisation of two oil palm markers showing somaclonal variation-associated polymorphism. Theor. Appl. Genet. 2002 , 104 , 1263–1269. [ Google Scholar ] [ CrossRef ] [ PubMed ]
- Singh, R.; Cheah, S.C. Analysis of the inheritance of AFLP markers in an interspecific cross of oil palm using the pseudo-testcross strategy. J. Oil Palm Res. 1999 , 11 , 64–73. [ Google Scholar ]
- Mayes, S.; Jack, P.L.; Corley, R.H.; Marshall, D.F. Construction of a RFLP genetic linkage map for oil palm ( Elaeis guineensis Jacq.). Genome 1997 , 40 , 116–122. [ Google Scholar ] [ CrossRef ] [ PubMed ]
- Mohan, M.; Nair, S.; Bhagwat, A.; Krishna, T.G.; Yano, M.; Bhatia, C.R.; Sasaki, T. Genome mapping, molecular markers and marker-assisted selection in crop plants. Mol. Breed. 1997 , 3 , 87–103. [ Google Scholar ] [ CrossRef ]
- Ho, C.-L.; Kwan, Y.-Y.; Choi, M.-C.; Tee, S.-S.; Ng, W.-H.; Lim, K.-A.; Lee, Y.-P.; Ooi, S.-E.; Lee, W.-W.; Tee, J.-M.; et al. Analysis and functional annotation of expressed sequence tags (ESTs) from multiple tissues of oil palm ( Elaeis guineensis Jacq.). BMC Genom. 2007 , 8 , 381. [ Google Scholar ] [ CrossRef ]
- Zhao, H.; Zhang, T.; Meng, X.; Song, J.; Zhang, C.; Gao, P. Genetic Mapping and QTL Analysis of Fruit Traits in Melon ( Cucumis melo L.). Curr. Issues Mol. Biol. 2023 , 45 , 3419–3433. [ Google Scholar ] [ CrossRef ]
- Cardona, C.C.C.; Coronado, Y.M.; Conronado, A.C.M.; Ochoa, I.J.B. Genetic diversity in oil palm ( Elaeis guineensis Jacq) using RAM (Random Amplified Microsatellites). Bragantia 2018 , 77 , 546–556. [ Google Scholar ] [ CrossRef ]
- Sunilkumar, K.; Murugesan, P.; Mathur, R.K.; Rajesh, M.K. Genetic diversity in oil palm ( Elaeis guineensis and Elaeis oleifera ) germplasm as revealed by microsatellite (SSR) markers. Indian J. Agric. Sci. 2020 , 90 , 741–745. [ Google Scholar ] [ CrossRef ]
- Suraninpong, P.; Nuanlaong, S. Comparative transcriptome profiling and molecular marker development for oil palm fruit color. Sci. Rep. 2022 , 12 , 15507. [ Google Scholar ] [ CrossRef ]
- Mohd Shaha, F.R.; Liew, P.L.; Qamaruz Zaman, F.; Nulit, R.; Barin, J.; Rolland, J.; Yong, H.Y.; Boon, S.H. Genotyping by sequencing for the construction of oil palm ( Elaeis guineensis Jacq.) genetic linkage map and mapping of yield related quantitative trait loci. PeerJ 2024 , 12 , e16570. [ Google Scholar ] [ CrossRef ] [ PubMed ]
- Kwong, Q.B.; Teh, C.K.; Ong, A.L.; Heng, H.Y.; Lee, H.L.; Mohamed, M.; Low, J.Z.-B.; Apparow, S.; Chew, F.T.; Mayes, S.; et al. Development and Validation of a High-Density SNP Genotyping Array for African Oil Palm. Mol. Plant 2016 , 9 , 1132–1141. [ Google Scholar ] [ CrossRef ] [ PubMed ]
- Babu, B.K.; Mathur, R.K.; Ravichandran, G.; Anita, P.; Venu, M.V.B. Genome wide association study (GWAS) and identification of candidate genes for yield and oil yield related traits in oil palm ( Eleaeis guineensis ) using SNPs by genotyping-based sequencing. Genomics 2020 , 112 , 1011–1020. [ Google Scholar ] [ CrossRef ] [ PubMed ]
- Wibowo, C.S.; Apriyanto, A.; Ernawan, R.; Neing, D.; Susilo, R.; Cordell, H.J.; Gatehouse, A.M.R.; Edwards, M.G. Genetic variants associated with leaf spot disease resistance in oil palm ( Elaeis guineensis ): A genome-wide association study. Plant Pathol. 2023 , 72 , 1626–1636. [ Google Scholar ] [ CrossRef ]
- Yaakub, Z.; Kamaruddin, K.; Singh, R.; Mustafa, S.; Marjuni, M.; Ting, N.C.; Amiruddin, M.D.; Leslie, L.E.; Cheng-Li, O.L.; Sritharan, K.; et al. An integrated linkage map of interspecific backcross 2 (BC(2)) populations reveals QTLs associated with fatty acid composition and vegetative parameters influencing compactness in oil palm. BMC Plant Biol. 2020 , 20 , 356. [ Google Scholar ] [ CrossRef ] [ PubMed ]
- Zhou, L.; Yarra, R.; Zhao, Z.; Jin, L.; Cao, H. Development of SSR markers based on transcriptome data and association mapping analysis for fruit shell thickness associated traits in oil palm ( Elaeis guineensis Jacq.). 3 Biotech 2020 , 10 , 280. [ Google Scholar ] [ CrossRef ] [ PubMed ]
- Lee, M.; Xia, J.H.; Zou, Z.; Ye, J.; Rahmadsyah; Alfiko, Y.; Jin, J.; Lieando, J.V.; Purnamasari, M.I.; Lim, C.H.; et al. A consensus linkage map of oil palm and a major QTL for stem height. Sci. Rep. 2015 , 5 , 8232. [ Google Scholar ] [ CrossRef ] [ PubMed ]
- Nuanlaong, S.; Wuthisuthimethavee, S.; Azzeme, A.M.; Suraninpong, P. Optimized Method for the Identification of Candidate Genes and Molecular Maker Development Related to Drought Tolerance in Oil Palm ( Elaeis guineensis Jacq.). Plants 2022 , 11 , 2317. [ Google Scholar ] [ CrossRef ]
- Suraninpong, P.; Thongkhao, K.; Azzeme, A.M.; Suksa-Ard, P. Monitoring Drought Tolerance in Oil Palm: Choline Monooxygenase as a Novel Molecular Marker. Plants 2023 , 12 , 3089. [ Google Scholar ] [ CrossRef ]
- Zolkafli, S.H.; Ithnin, M.; Chan, K.-L.; Zainol Abidin, M.I.; Ismail, I.; Ting, N.C.; Ooi, L.C.-L.; Singh, R. Optimal set of microsatellite markers required to detect illegitimate progenies in selected oil palm ( Elaeis guineensis Jacq.) breeding crosses. Breed. Sci. 2021 , 71 , 253–260. [ Google Scholar ] [ CrossRef ]
- Bhagya, H.P.; Kalyana Babu, B.; Gangadharappa, P.M.; Naika, M.B.N.; Satish, D.; Mathur, R.K. Identification of QTLs in oil palm ( Elaeis guineensis Jacq.) using SSR markers through association mapping. J. Genet. 2020 , 99 , 19. [ Google Scholar ] [ CrossRef ] [ PubMed ]
- Zulfugarov, I.S.; Tovuu, A.; Eu, Y.J.; Dogsom, B.; Poudyal, R.S.; Nath, K.; Hall, M.; Banerjee, M.; Yoon, U.C.; Moon, Y.H.; et al. Production of superoxide from Photosystem II in a rice ( Oryza sativa L.) mutant lacking PsbS. BMC Plant Biol. 2014 , 14 , 242. [ Google Scholar ] [ CrossRef ] [ PubMed ]
- Ting, N.C.; Jansen, J.; Nagappan, J.; Ishak, Z.; Chin, C.W.; Tan, S.G.; Cheah, S.C.; Singh, R. Identification of QTLs associated with callogenesis and embryogenesis in oil palm using genetic linkage maps improved with SSR markers. PLoS ONE 2013 , 8 , e53076. [ Google Scholar ] [ CrossRef ] [ PubMed ]
- Jack, P.L.; Dimitrijevic, T.A.F.; Mayes, S. Assessment of nuclear, mitochondrial and chloroplast RFLP markers in oil palm ( Elaeis guineensis Jacq.). Theor. Appl. Genet. 1995 , 90 , 643–649. [ Google Scholar ] [ CrossRef ]
- Xia, W.; Luo, T.; Dou, Y.; Zhang, W.; Mason, A.S.; Huang, D.; Huang, X.; Tang, W.; Wang, J.; Zhang, C.; et al. Identification and Validation of Candidate Genes Involved in Fatty Acid Content in Oil Palm by Genome-Wide Association Analysis. Front. Plant Sci. 2019 , 10 , 1263. [ Google Scholar ] [ CrossRef ]
- Somyong, S.; Phetchawang, P.; Bihi, A.K.; Sonthirod, C.; Kongkachana, W.; Sangsrakru, D.; Jomchai, N.; Pootakham, W.; Tangphatsornruang, S. A SNP variation in an expansin ( EgExp4 ) gene affects height in oil palm. PeerJ 2022 , 10 , e13046. [ Google Scholar ] [ CrossRef ]
- Li, J.; Yang, Y.; Sun, X.; Liu, R.; Xia, W.; Shi, P.; Zhou, L.; Wang, Y.; Wu, Y.; Lei, X.; et al. Development of Intron Polymorphism Markers and Their Association with Fatty Acid Component Variation in Oil Palm. Front. Plant Sci. 2022 , 13 , 885418. [ Google Scholar ] [ CrossRef ]
- Myint, K.A.; Yaakub, Z.; Rafii, M.Y.; Oladosu, Y.; Samad, M.Y.A.; Ramlee, S.I.; Mustaffa, S.; Arolu, F.; Abdullah, N.; Marjuni, M.; et al. Genetic Diversity Assessment of MPOB-Senegal Oil Palm Germplasm Using Microsatellite Markers. BioMed Res. Int. 2021 , 2021 , 6620645. [ Google Scholar ] [ CrossRef ] [ PubMed ]
- Zaki, N.M.; Singh, R.; Rosli, R.; Ismail, I. Elaeis oleifera Genomic-SSR Markers: Exploitation in Oil Palm Germplasm Diversity and Cross-Amplification in Arecaceae. Int. J. Mol. Sci. 2012 , 13 , 4069–4088. [ Google Scholar ] [ CrossRef ]
- Ting, N.C.; Zaki, N.M.; Rosli, R.; Low, E.T.; Ithnin, M.; Cheah, S.C.; Tan, S.G.; Singh, R. SSR mining in oil palm EST database: Application in oil palm germplasm diversity studies. J. Genet. 2010 , 89 , 135–145. [ Google Scholar ] [ CrossRef ]
- Somyong, S.; Poopear, S.; Sunner, S.K.; Wanlayaporn, K.; Jomchai, N.; Yoocha, T.; Ukoskit, K.; Tangphatsornruang, S.; Tragoonrung, S. ACC oxidase and miRNA 159a, and their involvement in fresh fruit bunch yield (FFB) via sex ratio determination in oil palm. Mol. Genet. Genom. 2016 , 291 , 1243–1257. [ Google Scholar ] [ CrossRef ] [ PubMed ]
- Dou, Y.; Xia, W.; Mason, A.S.; Huang, D.; Sun, X.; Fan, H.; Xiao, Y. Developing functional markers for vitamin E biosynthesis in oil palm. PLoS ONE 2021 , 16 , e0259684. [ Google Scholar ] [ CrossRef ]
- Venu, M.V.B.; Babu, B.K. Identification of fruit forms using CAPS marker among the oil palm indigenous germplasm. J. Oilseeds Res. 2023 , 37 . [ Google Scholar ] [ CrossRef ]
- Ithnin, M.; Xu, Y.; Marjuni, M.; Serdari, N.M.; Amiruddin, M.D.; Low, E.-T.L.; Tan, Y.-C.; Yap, S.-J.; Ooi, L.C.L.; Nookiah, R.; et al. Multiple locus genome-wide association studies for important economic traits of oil palm. Tree Genet. Genomes 2017 , 13 , 103. [ Google Scholar ] [ CrossRef ]
- Ithnin, M.; Vu, W.T.; Shin, M.-G.; Suryawanshi, V.; Sherbina, K.; Zolkafli, S.H.; Serdari, N.M.; Amiruddin, M.D.; Abdullah, N.; Mustaffa, S.; et al. Genomic diversity and genome-wide association analysis related to yield and fatty acid composition of wild American oil palm. Plant Sci. 2021 , 304 , 110731. [ Google Scholar ] [ CrossRef ]
- Osorio-Guarín, J.A.; Garzón-Martínez, G.A.; Delgadillo-Duran, P.; Bastidas, S.; Moreno, L.P.; Enciso-Rodríguez, F.E.; Cornejo, O.E.; Barrero, L.S. Genome-wide association study (GWAS) for morphological and yield-related traits in an oil palm hybrid ( Elaeis oleifera × Elaeis guineensis) population. BMC Plant Biol. 2019 , 19 , 533. [ Google Scholar ] [ CrossRef ] [ PubMed ]
- Babu, B.K.; Mathur, R.K.; Ravichandran, G.; Venu, M.V.B. Genome-wide association study (GWAS) for stem height increment in oil palm ( Elaeis guineensis ) germplasm using SNP markers. Tree Genet. Genomes 2019 , 15 , 40. [ Google Scholar ] [ CrossRef ]
- Bhagya, H.P.; Kalyana Babu, B.; Mathur, R.K.; Ramajayam, D.; Ravichandran, G.; Anitha, P. Oil palm ( Elaeis guineensis Jacq.) germplasm genome-wide association analysis for the oil yield traits utilizing microsatellite markers. Ind. Crops Prod. 2024 , 218 , 118934. [ Google Scholar ] [ CrossRef ]
- Teh, C.-K.; Ong, A.-L.; Kwong, Q.-B.; Apparow, S.; Chew, F.-T.; Mayes, S.; Mohamed, M.; Appleton, D.; Kulaveerasingam, H. Genome-wide association study identifies three key loci for high mesocarp oil content in perennial crop oil palm. Sci. Rep. 2016 , 6 , 19075. [ Google Scholar ] [ CrossRef ]
- Babu, B.K.; Mathur, R.K.; Ravichandran, G.; Anitha, P.; Venu, M.V.B. Genome-wide association study for leaf area, rachis length and total dry weight in oil palm ( Eleaeis guineensis ) using genotyping by sequencing. PLoS ONE 2019 , 14 , e0220626. [ Google Scholar ] [ CrossRef ]
- Babu, K.; Mathur, R.K.; Venu, M.V.; Shil, S.; Ravichandran, G.; Anita, P.; Bhagya, H.P. Genome-wide association study (GWAS) of major QTLs for bunch and oil yield related traits in Elaeis guineensis L. Plant Sci. 2021 , 305 , 110810. [ Google Scholar ] [ CrossRef ]
- Teh, C.-K.; Muaz, S.D.; Tangaya, P.; Fong, P.-Y.; Ong, A.-L.; Mayes, S.; Chew, F.-T.; Kulaveerasingam, H.; Appleton, D. Characterizing haploinsufficiency of SHELL gene to improve fruit form prediction in introgressive hybrids of oil palm. Sci. Rep. 2017 , 7 , 3118. [ Google Scholar ] [ CrossRef ] [ PubMed ]
- Singh, R.; Low, E.-T.L.; Ooi, L.C.-L.; Ong-Abdullah, M.; Nookiah, R.; Ting, N.-C.; Marjuni, M.; Chan, P.-L.; Ithnin, M.; Manaf, M.A.A.; et al. The oil palm VIRESCENS gene controls fruit colour and encodes a R2R3-MYB . Nat. Commun. 2014 , 5 , 4106. [ Google Scholar ] [ CrossRef ] [ PubMed ]
- Chan, K.-L.; Tatarinova, T.V.; Rosli, R.; Amiruddin, N.; Azizi, N.; Halim, M.A.A.; Sanusi, N.S.N.M.; Jayanthi, N.; Ponomarenko, P.; Triska, M.; et al. Evidence-based gene models for structural and functional annotations of the oil palm genome. Biol. Direct 2017 , 12 , 21. [ Google Scholar ] [ CrossRef ]
- Zhang, Y.; Bai, B.; Lee, M.; Alfiko, Y.; Suwanto, A.; Yue, G.H. Cloning and characterization of EgGDSL , a gene associated with oil content in oil palm. Sci. Rep. 2018 , 8 , 11406. [ Google Scholar ] [ CrossRef ]
- Jin, Y.; Yuan, Y.; Gao, L.; Sun, R.; Chen, L.; Li, D.; Zheng, Y. Characterization and Functional Analysis of a Type 2 Diacylglycerol Acyltransferase ( DGAT2 ) Gene from Oil Palm ( Elaeis guineensis Jacq.) Mesocarp in Saccharomyces cerevisiae and Transgenic Arabidopsis thaliana . Front. Plant Sci. 2017 , 8 , 1791. [ Google Scholar ] [ CrossRef ] [ PubMed ]
- Rosli, R.; Chan, P.-L.; Chan, K.-L.; Amiruddin, N.; Low, E.-T.L.; Singh, R.; Harwood, J.L.; Murphy, D.J. In silico characterization and expression profiling of the diacylglycerol acyltransferase gene family (DGAT1, DGAT2, DGAT3 and WS/DGAT) from oil palm, Elaeis guineensis . Plant Sci. 2018 , 275 , 84–96. [ Google Scholar ] [ CrossRef ] [ PubMed ]
- Li, S.Y.; Zhang, Q.; Jin, Y.H.; Zou, J.X.; Zheng, Y.S.; Li, D.D. A MADS-box gene, EgMADS21 , negatively regulates EgDGAT2 expression and decreases polyunsaturated fatty acid accumulation in oil palm ( Elaeis guineensis Jacq.). Plant Cell Rep. 2020 , 39 , 1505–1516. [ Google Scholar ] [ CrossRef ]
- Chan, P.L.; Rose, R.J.; Abdul Murad, A.M.; Zainal, Z.; Ong, P.W.; Ooi, L.C.; Low, E.L.; Ishak, Z.; Yahya, S.; Song, Y.; et al. Early nodulin 93 protein gene: Essential for induction of somatic embryogenesis in oil palm. Plant Cell Rep. 2020 , 39 , 1395–1413. [ Google Scholar ] [ CrossRef ]
- Nizan, I.E.F.; Kamaruddin, K.; Ong, P.W.; Ramli, Z.; Singh, R.; Rose, R.J.; Chan, P.L. Overexpression of Oil Palm Early Nodulin 93 Protein Gene ( EgENOD93 ) Enhances In Vitro Shoot Regeneration in Arabidopsis thaliana. Mol. Biotechnol. 2022 , 64 , 743–757. [ Google Scholar ] [ CrossRef ]
- Tregear, J.W.; Richaud, F.; Collin, M.; Esbelin, J.; Parrinello, H.; Cochard, B.; Nodichao, L.; Morcillo, F.; Adam, H.; Jouannic, S. Micro-RNA-Regulated SQUAMOSA-PROMOTER BINDING PROTEIN-LIKE ( SPL ) Gene Expression and Cytokinin Accumulation Distinguish Early-Developing Male and Female Inflorescences in Oil Palm ( Elaeis guineensis ). Plants 2022 , 11 , 685. [ Google Scholar ] [ CrossRef ] [ PubMed ]
- Zhou, L.; Yarra, R. Genome-Wide Analysis of SPL /miR156 Module and Its Expression Analysis in Vegetative and Reproductive Organs of Oil Palm ( Elaeis guineensis ). Int. J. Mol. Sci. 2023 , 24 , 13658. [ Google Scholar ] [ CrossRef ] [ PubMed ]
- Li, J.; Yang, Y.; Iqbal, A.; Qadri, R.; Shi, P.; Wang, Y.; Wu, Y.; Fan, H.; Wu, G. Correlation analysis of cold-related gene expression with physiological and biochemical indicators under cold stress in oil palm. PLoS ONE 2019 , 14 , e0225768. [ Google Scholar ] [ CrossRef ]
- Wang, L.; Lee, M.; Ye, B.; Yue, G.H. Genes, pathways and networks responding to drought stress in oil palm roots. Sci. Rep. 2020 , 10 , 21303. [ Google Scholar ] [ CrossRef ]
- Zhou, L.; Yarra, R. Genome-wide identification and expression analysis of bZIP transcription factors in oil palm ( Elaeis guineensis Jacq.) under abiotic stress. Protoplasma 2022 , 259 , 469–483. [ Google Scholar ] [ CrossRef ]
- Zhou, L.; Yarra, R. Genome-Wide Identification and Characterization of AP2/ERF Transcription Factor Family Genes in Oil Palm under Abiotic Stress Conditions. Int. J. Mol. Sci. 2021 , 22 , 2821. [ Google Scholar ] [ CrossRef ] [ PubMed ]
- Lim, H.; Kobayashi, M.J.; Marsoem, S.N.; Irawati, D.; Kosugi, A.; Kondo, T.; Tani, N. Transcriptomic responses of oil palm ( Elaeis guineensis ) stem to waterlogging at plantation in relation to precipitation seasonality. Front. Plant Sci. 2023 , 14 , 1213496. [ Google Scholar ] [ CrossRef ]
- Zhou, L.; Yarra, R.; Yang, Y.; Liu, Y.; Yang, M.; Cao, H. The oil palm R2R3-MYB subfamily genes EgMYB111 and EgMYB157 improve multiple abiotic stress tolerance in transgenic Arabidopsis plants. Plant Cell Rep. 2022 , 41 , 377–393. [ Google Scholar ] [ CrossRef ]
- Lee, F.C.; Yeap, W.C.; Appleton, D.R.; Ho, C.-L.; Kulaveerasingam, H. Identification of drought responsive Elaeis guineensis WRKY transcription factors with sensitivity to other abiotic stresses and hormone treatments. BMC Genom. 2022 , 23 , 164. [ Google Scholar ] [ CrossRef ]
- Song, Z.; Wang, L.; Lai, C.; Lee, M.; Yang, Z.; Yue, G. EgSPEECHLESS Responses to Salt Stress by Regulating Stomatal Development in Oil Palm. Int. J. Mol. Sci. 2022 , 23 , 4659. [ Google Scholar ] [ CrossRef ]
- Jin, L.; Yarra, R.; Zhou, L.; Cao, H. The auxin response factor (ARF) gene family in Oil palm ( Elaeis guineensis Jacq.): Genome-wide identification and their expression profiling under abiotic stresses. Protoplasma 2022 , 259 , 47–60. [ Google Scholar ] [ CrossRef ] [ PubMed ]
- Zhou, L.; John Martin, J.J.; Li, R.; Zeng, X.; Wu, Q.; Li, Q.; Fu, D.; Li, X.; Liu, X.; Ye, J.; et al. Catalase (CAT) Gene Family in Oil Palm ( Elaeis guineensis Jacq.): Genome-Wide Identification, Analysis, and Expression Profile in Response to Abiotic Stress. Int. J. Mol. Sci. 2024 , 25 , 1480. [ Google Scholar ] [ CrossRef ] [ PubMed ]
- Rosli, R.; Amiruddin, N.; Ab Halim, M.A.; Chan, P.-L.; Chan, K.-L.; Azizi, N.; Morris, P.E.; Leslie Low, E.-T.; Ong-Abdullah, M.; Sambanthamurthi, R.; et al. Comparative genomic and transcriptomic analysis of selected fatty acid biosynthesis genes and CNL disease resistance genes in oil palm. PLoS ONE 2018 , 13 , e0194792. [ Google Scholar ] [ CrossRef ] [ PubMed ]
- Hanin, A.N.; Parveez, G.K.A.; Rasid, O.A.; Masani, M.Y.A. Biolistic-mediated oil palm transformation with alfalfa glucanase ( AGLU1 ) and rice chitinase ( RCH10 ) genes for increasing oil palm resistance towards Ganoderma boninense . Ind. Crops Prod. 2020 , 144 , 112008. [ Google Scholar ] [ CrossRef ]
- Faizah, R.; Putranto, R.A.; Raharti, V.R.; Supena, N.; Sukma, D.; Budiani, A.; Wening, S.; Sudarsono, S. Defense response changes in roots of oil palm ( Elaeis guineensis Jacq.) seedlings after internal symptoms of Ganoderma boninense Pat. infection. BMC Plant Biol. 2022 , 22 , 139. [ Google Scholar ] [ CrossRef ] [ PubMed ]
- Cao, J.; Wang, W.; Xu, X.; Li, S.-Y.; Zheng, Y.; Li, D.-D. Identification and Analysis of MADS-Box Genes Expressed in the Mesocarp of Oil Palm Fruit ( Elaeis guineensis Jacq.). Biochem. Genet. 2023 , 61 , 2382–2400. [ Google Scholar ] [ CrossRef ] [ PubMed ]
- Wang, Y.; Yan, J.; Yang, M.; Zou, J.; Zheng, Y.; Li, D. EgMADS3 directly regulates EgLPAAT to mediate medium-chain fatty acids (MCFA) anabolism in the mesocarp of oil palm. Plant Cell Rep. 2024 , 43 , 107. [ Google Scholar ] [ CrossRef ]
- Amiruddin, N.; Chan, P.-L.; Azizi, N.; Morris, P.E.; Chan, K.-L.; Ong, P.W.; Rosli, R.; Masura, S.S.; Murphy, D.J.; Sambanthamurthi, R.; et al. Characterization of Oil Palm Acyl-CoA-Binding Proteins and Correlation of Their Gene Expression with Oil Synthesis. Plant Cell Physiol. 2019 , 61 , 735–747. [ Google Scholar ] [ CrossRef ]
- Yusuf, C.Y.L.; Nabilah, N.S.; Taufik, N.; Seman, I.A.; Abdullah, M.P. Genome-wide analysis of the CAD gene family reveals two bona fide CAD genes in oil palm. 3 Biotech 2022 , 12 , 149. [ Google Scholar ] [ CrossRef ]
- Manzoni, C.; Kia, D.A.; Vandrovcova, J.; Hardy, J.; Wood, N.W.; Lewis, P.A.; Ferrari, R. Genome, transcriptome and proteome: The rise of omics data and their integration in biomedical sciences. Brief. Bioinform. 2016 , 19 , 286–302. [ Google Scholar ] [ CrossRef ]
- Yang, Z.; Liu, Z.; Xu, H.; Li, Y.; Huang, S.; Cao, G.; Shi, M.; Zhu, J.; Zhou, J.; Li, R.; et al. ArecaceaeMDB: A comprehensive multi-omics database for Arecaceae breeding and functional genomics studies. Plant Biotechnol. J. 2023 , 21 , 11–13. [ Google Scholar ] [ CrossRef ] [ PubMed ]
- Xiao, Y.; Xia, W.; Mason, A.S.; Cao, Z.; Fan, H.; Zhang, B.; Zhang, J.; Ma, Z.; Peng, M.; Huang, D. Genetic control of fatty acid composition in coconut ( Cocos nucifera ), African oil palm ( Elaeis guineensis ), and date palm ( Phoenix dactylifera ). Planta 2019 , 249 , 333–350. [ Google Scholar ] [ CrossRef ] [ PubMed ]
- Zhang, A.; Jin, L.; Yarra, R.; Cao, H.; Chen, P.; John Martin, J.J. Transcriptome analysis reveals key developmental and metabolic regulatory aspects of oil palm ( Elaeis guineensis Jacq.) during zygotic embryo development. BMC Plant Biol. 2022 , 22 , 112. [ Google Scholar ] [ CrossRef ] [ PubMed ]
- Apriyanto, A.; Compart, J.; Fettke, J. Transcriptomic analysis of mesocarp tissue during fruit development of the oil palm revealed specific isozymes related to starch metabolism that control oil yield. Front. Plant Sci. 2023 , 14 , 1220237. [ Google Scholar ] [ CrossRef ] [ PubMed ]
- Chu, K.L.; Koley, S.; Jenkins, L.M.; Bailey, S.R.; Kambhampati, S.; Foley, K.; Arp, J.J.; Morley, S.A.; Czymmek, K.J.; Bates, P.D.; et al. Metabolic flux analysis of the non-transitory starch tradeoff for lipid production in mature tobacco leaves. Metab. Eng. 2022 , 69 , 231–248. [ Google Scholar ] [ CrossRef ]
- Apriyanto, A.; Ernawan, R.; Putra, M.K.A.; Susilo, R.; Ajambang, W.; Pancoro, A.; Wibowo, C.S. Gene expression profiles of oil palm leaves from different oil yields and genetic population: Transcriptomic dataset. Data Brief 2023 , 48 , 109043. [ Google Scholar ] [ CrossRef ] [ PubMed ]
- Yang, M.; Yarra, R.; Zhang, R.; Zhou, L.; Jin, L.; Martin, J.J.J.; Cao, H. Transcriptome analysis of oil palm pistil during pollination and fertilization to unravel the role of phytohormone biosynthesis and signaling genes. Funct. Integr. Genom. 2022 , 22 , 261–278. [ Google Scholar ] [ CrossRef ]
- Zheng, Y.; Chen, C.; Liang, Y.; Sun, R.; Gao, L.; Liu, T.; Li, D. Genome-wide association analysis of the lipid and fatty acid metabolism regulatory network in the mesocarp of oil palm ( Elaeis guineensis Jacq.) based on small noncoding RNA sequencing. Tree Physiol. 2018 , 39 , 356–371. [ Google Scholar ] [ CrossRef ]
- Xia, W.; Dou, Y.; Liu, R.; Gong, S.; Huang, D.; Fan, H.; Xiao, Y. Genome-wide discovery and characterization of long noncoding RNAs in African oil palm ( Elaeis guineensis Jacq.). PeerJ 2020 , 8 , e9585. [ Google Scholar ] [ CrossRef ]
- Ooi, S.-E.; Feshah, I.; Nuraziyan, A.; Sarpan, N.; Ata, N.; Lim, C.-C.; Choo, C.-N.; Wong, W.-C.; Wong, F.-H.; Wong, C.-K.; et al. Leaf transcriptomic signatures for somatic embryogenesis potential of Elaeis guineensis . Plant Cell Rep. 2021 , 40 , 1141–1154. [ Google Scholar ] [ CrossRef ]
- Salgado, F.F.; da Silva, T.L.C.; Vieira, L.R.; Silva, V.N.B.; Leão, A.P.; Costa, M.; Togawa, R.C.; de Sousa, C.A.F.; Grynberg, P.; Souza, M.T., Jr. The early response of oil palm ( Elaeis guineensis Jacq.) plants to water deprivation: Expression analysis of miRNAs and their putative target genes, and similarities with the response to salinity stress. Front. Plant Sci. 2022 , 13 , 970113. [ Google Scholar ] [ CrossRef ] [ PubMed ]
- Kong, S.-L.; Abdullah, S.N.A.; Ho, C.-L.; Musa, M.H.b.; Yeap, W.-C. Comparative transcriptome analysis reveals novel insights into transcriptional responses to phosphorus starvation in oil palm ( Elaeis guineensis ) root. BMC Genom. Data 2021 , 22 , 6. [ Google Scholar ] [ CrossRef ] [ PubMed ]
- Sahara, A.; Roberdi, R.; Wiendi, N.M.A.; Liwang, T. Transcriptome profiling of high and low somatic embryogenesis rate of oil palm ( Elaeis guineensis Jacq. var. Tenera). Front. Plant Sci. 2023 , 14 , 1142868. [ Google Scholar ] [ CrossRef ] [ PubMed ]
- García-Gaona, M.; Botero-Rozo, D.; Araque, L.; Romero, H.M. The Dynamic Interaction between Oil Palm and Phytophthora palmivora in Bud Rot Disease: Insights from Transcriptomic Analysis and Network Modelling. J. Fungi 2024 , 10 , 164. [ Google Scholar ] [ CrossRef ] [ PubMed ]
- Apriyanto, A.; Ajambang, W. Transcriptomic dataset for early inflorescence stages of oil palm in response to defoliation stress. Data Brief 2022 , 41 , 107914. [ Google Scholar ] [ CrossRef ] [ PubMed ]
- Zainuddin, N.; Maidin, M.S.T.; Kamarudin, N.; Napiah, N.R.A.M.A.; Keni, M.F.; Masri, M.M.M. De novo transcriptome analysis of bagworm Metisa plana from highly infested oil palm estate in Perak revealed detoxification genes and potential insecticide targets. J. Asia Pac. Entomol. 2023 , 26 , 102039. [ Google Scholar ] [ CrossRef ]
- Mejia-Alvarado, F.S.; Botero-Rozo, D.; Araque, L.; Bayona, C.; Herrera-Corzo, M.; Montoya, C.; Ayala-Díaz, I.; Romero, H.M. Molecular network of the oil palm root response to aluminum stress. BMC Plant Biol. 2023 , 23 , 346. [ Google Scholar ] [ CrossRef ] [ PubMed ]
- Salgado, F.F.; Vieira, L.R.; Silva, V.N.B.; Leão, A.P.; Grynberg, P.; do Carmo Costa, M.M.; Togawa, R.C.; de Sousa, C.A.F.; Júnior, M.T.S. Expression analysis of miRNAs and their putative target genes confirm a preponderant role of transcription factors in the early response of oil palm plants to salinity stress. BMC Plant Biol. 2021 , 21 , 518. [ Google Scholar ] [ CrossRef ]
- Ferreira, T.M.M.; Ferreira Filho, J.A.; Leão, A.P.; de Sousa, C.A.F.; Souza, M.T., Jr. Structural and functional analysis of stress-inducible genes and their promoters selected from young oil palm ( Elaeis guineensis ) under salt stress. BMC Genom. 2022 , 23 , 735. [ Google Scholar ] [ CrossRef ]
- Faizah, R.; Putranto, R.A.; Wening, S.; Sukma, D.; Raharti, V.R.; Budiani, A.; Sudarsono, S.J.I.C.S.E.; Science, E. Differential expression of root specific genes of oil palm seedlings at early stage of Ganoderma boninense infection. IOP Conf. Ser. Earth Environ. Sci. 2020 , 418 , 012044. [ Google Scholar ] [ CrossRef ]
- Saand, M.A.; Li, J.; Wu, Y.; Zhou, L.; Cao, H.; Yang, Y. Integrative Omics Analysis of Three Oil Palm Varieties Reveals (Tanzania × Ekona) TE as a Cold-Resistant Variety in Response to Low-Temperature Stress. Int. J. Mol. Sci 2022 , 23 , 14926. [ Google Scholar ] [ CrossRef ] [ PubMed ]
- Silva Rde, C.; Carmo, L.S.; Luis, Z.G.; Silva, L.P.; Scherwinski-Pereira, J.E.; Mehta, A. Proteomic identification of differentially expressed proteins during the acquisition of somatic embryogenesis in oil palm ( Elaeis guineensis Jacq.). J. Proteom. 2014 , 104 , 112–127. [ Google Scholar ] [ CrossRef ] [ PubMed ]
- Tan, H.S.; Liddell, S.; Ong Abdullah, M.; Wong, W.C.; Chin, C.F. Differential proteomic analysis of embryogenic lines in oil palm ( Elaeis guineensis Jacq). J. Proteom. 2016 , 143 , 334–345. [ Google Scholar ] [ CrossRef ]
- Ribeiro, D.G.; de Almeida, R.F.; Fontes, W.; de Souza Castro, M.; de Sousa, M.V.; Ricart, C.A.O.; da Cunha, R.N.V.; Lopes, R.; Scherwinski-Pereira, J.E.; Mehta, A. Stress and cell cycle regulation during somatic embryogenesis plays a key role in oil palm callus development. J. Proteom. 2019 , 192 , 137–146. [ Google Scholar ] [ CrossRef ] [ PubMed ]
- Aroonluk, S.; Roytrakul, S.; Jantasuriyarat, C. Identification and Characterization of Phosphoproteins in Somatic Embryogenesis Acquisition during Oil Palm Tissue Culture. Plants 2020 , 9 , 36. [ Google Scholar ] [ CrossRef ] [ PubMed ]
- Balotf, S.; Wilson, R.; Tegg, R.S.; Nichols, D.S.; Wilson, C.R. Shotgun Proteomics as a Powerful Tool for the Study of the Proteomes of Plants, Their Pathogens, and Plant-Pathogen Interactions. Proteomes 2022 , 10 , 5. [ Google Scholar ] [ CrossRef ]
- Loei, H.; Lim, J.; Tan, M.; Lim, T.K.; Lin, Q.S.; Chew, F.T.; Kulaveerasingam, H.; Chung, M.C. Proteomic analysis of the oil palm fruit mesocarp reveals elevated oxidative phosphorylation activity is critical for increased storage oil production. J. Proteome Res. 2013 , 12 , 5096–5109. [ Google Scholar ] [ CrossRef ]
- Kok, S.-Y.; Namasivayam, P.; Ee, G.C.-L.; Ong-Abdullah, M. Comparative proteomic analysis of oil palm ( Elaeis guineensis Jacq.) during early fruit development. J. Proteom. 2021 , 232 , 104052. [ Google Scholar ] [ CrossRef ]
- Hassan, H.; Amiruddin, M.D.; Weckwerth, W.; Ramli, U.S. Deciphering key proteins of oil palm ( Elaeis guineensis Jacq.) fruit mesocarp development by proteomics and chemometrics. Electrophoresis 2019 , 40 , 254–265. [ Google Scholar ] [ CrossRef ]
- Rodrigues-Neto, J.C.; Correia, M.V.; Souto, A.L.; Ribeiro, J.A.d.A.; Vieira, L.R.; Souza, M.T.; Rodrigues, C.M.; Abdelnur, P.V. Metabolic fingerprinting analysis of oil palm reveals a set of differentially expressed metabolites in fatal yellowing symptomatic and non-symptomatic plants. Metabolomics 2018 , 14 , 142. [ Google Scholar ] [ CrossRef ]
- Neto, J.C.R.; Vieira, L.R.; de Aquino Ribeiro, J.A.; de Sousa, C.A.F.; Júnior, M.T.S.; Abdelnur, P.V. Metabolic effect of drought stress on the leaves of young oil palm ( Elaeis guineensis) plants using UHPLC–MS and multivariate analysis. Sci. Rep. 2021 , 11 , 18271. [ Google Scholar ] [ CrossRef ] [ PubMed ]
- Muhammad, I.I.; Abdullah, S.N.A.; Saud, H.M.; Shaharuddin, N.A.; Isa, N.M. The Dynamic Responses of Oil Palm Leaf and Root Metabolome to Phosphorus Deficiency. Metabolites 2021 , 11 , 217. [ Google Scholar ] [ CrossRef ] [ PubMed ]
- Santiago, K.A.A.; Wong, W.C.; Goh, Y.K.; Tey, S.H.; Ting, A.S.Y. Pathogenicity of monokaryotic and dikaryotic mycelia of Ganoderma boninense revealed via LC–MS-based metabolomics. Sci. Rep. 2024 , 14 , 5330. [ Google Scholar ] [ CrossRef ] [ PubMed ]
- Zakaria, L. Basal Stem Rot of Oil Palm: The Pathogen, Disease Incidence, and Control Methods. Plant Dis. 2023 , 107 , 603–615. [ Google Scholar ] [ CrossRef ] [ PubMed ]
- Neoh, B.K.; Teh, H.F.; Ng, T.L.; Tiong, S.H.; Thang, Y.M.; Ersad, M.A.; Mohamed, M.; Chew, F.T.; Kulaveerasingam, H.; Appleton, D.R. Profiling of metabolites in oil palm mesocarp at different stages of oil biosynthesis. J. Agric. Food Chem. 2013 , 61 , 1920–1927. [ Google Scholar ] [ CrossRef ]
- Rozali, N.L.; Tahir, N.I.; Hassan, H.; Othman, A.; Ramli, U.S. Identification of amines, amino and organic acids in oil palm ( Elaeis guineensis Jacq.) spear leaf using GC- and LC/Q-TOF MS metabolomics platforms. Biocatal. Agric. Biotechnol. 2021 , 37 , 102165. [ Google Scholar ] [ CrossRef ]
Click here to enlarge figure
Traits | Number of Accessions | Chromosomes | Number of QTLs | Number of Candidate Genes | Reference |
---|---|---|---|---|---|
compact fruit bunch | 422 | 4, 8, 11 | 4 | / | [ ] |
oil content | 196 | / | 33 | 55 | [ ] |
fatty acid composition; nutrient content | 210 | 2, 13 | 6 | 3 | [ ] |
oil content | 471 | 3, 5, 10, 13, 15 | / | / | [ ] |
disease resistance | 96 | 6, 7, 9 | 5 | / | [ ] |
leaf area size; high yield; | 115 | / | / | 5 | [ ] |
trunk height | 132 | 5, 6 | / | / | [ ] |
stem height | 96 | 1, 2, 4, 14, 16 | 7 | / | [ ] |
high oil production | 310 | 1, 4, 7, 10, 11, 12, 15 | 43 | / | [ ] |
The statements, opinions and data contained in all publications are solely those of the individual author(s) and contributor(s) and not of MDPI and/or the editor(s). MDPI and/or the editor(s) disclaim responsibility for any injury to people or property resulting from any ideas, methods, instructions or products referred to in the content. |
Share and Cite
Xu, W.; John Martin, J.J.; Li, X.; Liu, X.; Zhang, R.; Hou, M.; Cao, H.; Cheng, S. Unveiling the Secrets of Oil Palm Genetics: A Look into Omics Research. Int. J. Mol. Sci. 2024 , 25 , 8625. https://doi.org/10.3390/ijms25168625
Xu W, John Martin JJ, Li X, Liu X, Zhang R, Hou M, Cao H, Cheng S. Unveiling the Secrets of Oil Palm Genetics: A Look into Omics Research. International Journal of Molecular Sciences . 2024; 25(16):8625. https://doi.org/10.3390/ijms25168625
Xu, Wen, Jerome Jeyakumar John Martin, Xinyu Li, Xiaoyu Liu, Ruimin Zhang, Mingming Hou, Hongxing Cao, and Shuanghong Cheng. 2024. "Unveiling the Secrets of Oil Palm Genetics: A Look into Omics Research" International Journal of Molecular Sciences 25, no. 16: 8625. https://doi.org/10.3390/ijms25168625
Article Metrics
Article access statistics, further information, mdpi initiatives, follow mdpi.
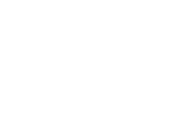
Subscribe to receive issue release notifications and newsletters from MDPI journals
Grab your spot at the free arXiv Accessibility Forum
Help | Advanced Search
Computer Science > Computation and Language
Title: rag foundry: a framework for enhancing llms for retrieval augmented generation.
Abstract: Implementing Retrieval-Augmented Generation (RAG) systems is inherently complex, requiring deep understanding of data, use cases, and intricate design decisions. Additionally, evaluating these systems presents significant challenges, necessitating assessment of both retrieval accuracy and generative quality through a multi-faceted approach. We introduce RAG Foundry, an open-source framework for augmenting large language models for RAG use cases. RAG Foundry integrates data creation, training, inference and evaluation into a single workflow, facilitating the creation of data-augmented datasets for training and evaluating large language models in RAG settings. This integration enables rapid prototyping and experimentation with various RAG techniques, allowing users to easily generate datasets and train RAG models using internal or specialized knowledge sources. We demonstrate the framework effectiveness by augmenting and fine-tuning Llama-3 and Phi-3 models with diverse RAG configurations, showcasing consistent improvements across three knowledge-intensive datasets. Code is released as open-source in this https URL .
Comments: | 10 pages |
Subjects: | Computation and Language (cs.CL); Artificial Intelligence (cs.AI); Information Retrieval (cs.IR); Machine Learning (cs.LG) |
Cite as: | [cs.CL] |
(or [cs.CL] for this version) | |
Focus to learn more arXiv-issued DOI via DataCite |
Submission history
Access paper:.
- Other Formats

References & Citations
- Google Scholar
- Semantic Scholar
BibTeX formatted citation

Bibliographic and Citation Tools
Code, data and media associated with this article, recommenders and search tools.
- Institution
arXivLabs: experimental projects with community collaborators
arXivLabs is a framework that allows collaborators to develop and share new arXiv features directly on our website.
Both individuals and organizations that work with arXivLabs have embraced and accepted our values of openness, community, excellence, and user data privacy. arXiv is committed to these values and only works with partners that adhere to them.
Have an idea for a project that will add value for arXiv's community? Learn more about arXivLabs .
Thank you for visiting nature.com. You are using a browser version with limited support for CSS. To obtain the best experience, we recommend you use a more up to date browser (or turn off compatibility mode in Internet Explorer). In the meantime, to ensure continued support, we are displaying the site without styles and JavaScript.
- View all journals
- Explore content
- About the journal
- Publish with us
- Sign up for alerts
- Open access
- Published: 07 August 2024
Highest ocean heat in four centuries places Great Barrier Reef in danger
- Benjamin J. Henley ORCID: orcid.org/0000-0003-3940-1963 1 , 2 , 3 ,
- Helen V. McGregor ORCID: orcid.org/0000-0002-4031-2282 1 , 2 ,
- Andrew D. King ORCID: orcid.org/0000-0001-9006-5745 4 , 5 ,
- Ove Hoegh-Guldberg ORCID: orcid.org/0000-0001-7510-6713 6 ,
- Ariella K. Arzey 1 , 2 ,
- David J. Karoly 4 ,
- Janice M. Lough 7 ,
- Thomas M. DeCarlo ORCID: orcid.org/0000-0003-3269-1320 8 , 9 &
- Braddock K. Linsley ORCID: orcid.org/0000-0003-2085-0662 10
Nature volume 632 , pages 320–326 ( 2024 ) Cite this article
2950 Accesses
1 Citations
1131 Altmetric
Metrics details
- Climate change
- Environmental impact
- Palaeoclimate
Mass coral bleaching on the Great Barrier Reef (GBR) in Australia between 2016 and 2024 was driven by high sea surface temperatures (SST) 1 . The likelihood of temperature-induced bleaching is a key determinant for the future threat status of the GBR 2 , but the long-term context of recent temperatures in the region is unclear. Here we show that the January–March Coral Sea heat extremes in 2024, 2017 and 2020 (in order of descending mean SST anomalies) were the warmest in 400 years, exceeding the 95th-percentile uncertainty limit of our reconstructed pre-1900 maximum. The 2016, 2004 and 2022 events were the next warmest, exceeding the 90th-percentile limit. Climate model analysis confirms that human influence on the climate system is responsible for the rapid warming in recent decades. This attribution, together with the recent ocean temperature extremes, post-1900 warming trend and observed mass coral bleaching, shows that the existential threat to the GBR ecosystem from anthropogenic climate change is now realized. Without urgent intervention, the iconic GBR is at risk of experiencing temperatures conducive to near-annual coral bleaching 3 , with negative consequences for biodiversity and ecosystems services. A continuation on the current trajectory would further threaten the ecological function 4 and outstanding universal value 5 of one of Earth’s greatest natural wonders.
Similar content being viewed by others
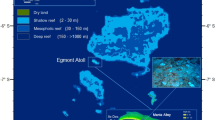
Mesophotic coral bleaching associated with changes in thermocline depth

Atypical weather patterns cause coral bleaching on the Great Barrier Reef, Australia during the 2021–2022 La Niña
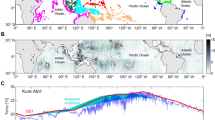
Internal tides can provide thermal refugia that will buffer some coral reefs from future global warming
Like many coral reefs globally, the World Heritage-listed GBR in Australia is under threat 4 , 6 . Mass coral bleaching, declining calcification rates 5 , 7 , outbreaks of crown-of-thorns starfish ( Acanthaster spp.) 8 , severe tropical cyclones 9 and overfishing 10 have placed compounding detrimental pressures on the reef ecosystem. Coral bleaching typically occurs when heat stress triggers the breakdown of the symbiosis between corals and their symbiotic dinoflagellates 11 . Although coral bleaching can occur locally as a result of low salinity, cold waters or pollution, regional and global mass bleaching events, in which the majority of corals in one or more regions bleach at once, are strongly associated with increasing SST linked to global warming 2 .
The first modern observations of mass coral bleaching on the GBR occurred in the 1980s, but these events were less widespread and generally less severe 3 than the bleaching events in the twenty-first century 4 . Stress bands in coral skeletal cores have provided potential evidence for pre-1980s bleaching in the GBR and Coral Sea, such as during the 1877–78 El Niño 12 . However, stress bands are evident in relatively few cores before 1980 (ref. 12 ), suggesting that severe mass bleaching did not occur in the 1800s and most of the 1900s.
As the oceans have warmed, however, mass coral bleaching events have become increasingly lethal to corals 4 . Coral bleaching on the GBR 1 in 1998 coincided with a strong eastern-Pacific El Niño, and in 2002 with a weak El Niño. El Niño events can induce lower cloud cover and increased solar irradiance over the GBR 13 , increasing the risk of thermal stress and mass bleaching events 14 . In 2004, water temperatures were anomalously warm, and although bleaching occurred in the Coral Sea 15 , it was not widespread in the GBR, probably because there was reduced upwelling and an associated reduced influence of nutrients on symbiotic dinoflagellate expulsion 16 .
However, in the nine January–March periods from 2016 to 2024 (inclusive) there were five mass coral bleaching events on the GBR. Each was associated with high SSTs and affected large sections of the reef. GBR mass bleaching occurred in both 2016 and 2017, influenced by the presence of an El Niño event in 2016, and led to the death of at least 50% of shallow-water (depths of 5–10 m) reef-building corals 4 . Major bleaching events occurred again in quick succession in 2020 and 2022, with the accumulated heat stress for large sections of the GBR reaching levels conducive to widespread bleaching but lower levels of coral mortality 1 . The bleaching event in 2022 occurred, unusually, during a La Niña event, which is typically associated with cooler summer SSTs, higher than average rainfall and higher cloud cover on the GBR 1 . At the time of writing, researchers are assessing the impacts of the 2024 mass bleaching event.
The frequency of recent mass coral bleaching and mortality on the GBR is cause for concern. In 2021, the World Heritage Committee of the United Nations Educational, Scientific and Cultural Organization (UNESCO) drafted 17 a decision to inscribe the GBR on the List of World Heritage in Danger, stating that the reef is “facing ascertained danger”, citing recent mass coral bleaching events and insufficient progress by the State Party (Australia) in countering climate change, improving water quality and land management issues. The committee’s adopted decisions 18 have not included inscription of the ‘in danger’ status, but the draft inscription highlights the seriousness of the recent mass coral bleaching events. Authorities in Australia 5 have noted that climate change and coral bleaching have deteriorated the integrity of the outstanding universal value of the GBR, a defining feature of its World Heritage status.
Although rapidly rising SSTs are attributed to human activities with virtual certainty 19 , understanding the multi-century SST history of the GBR is critical to understanding the influence of SST on mass coral bleaching and mortality in recent decades. Putting aside a problematic attempt to do this 20 , which was discredited 21 , 22 , knowledge of the long-term context for GBR SSTs comes primarily from two multi-century reconstructions based on the geochemistry of coral cores collected from the inner shelf 23 and outer shelf 24 (Flinders Reef) in the central GBR. These reconstructions showed that SSTs in the early 2000s were not unusually high relative to levels in the past three centuries, with five-year mean SSTs (and salinities) estimated to be higher in the 1700s than in the 1900s. However, these records were limited by their relatively coarse five-year sampling resolution and their most recent data point being from the early 2000s. After these studies were published, SSTs in the GBR have continued to rise. Updated analysis of coral data from Flinders Reef provides valuable improved temporal resolution 25 , but interpretations of these records remain limited spatially.
Here, we investigate the recent high SST events in the GBR region in the context of the past four centuries. We combine a network of 22 coral Sr/Ca and δ 18 O palaeothermometer series (Supplementary Tables 1 and 2 ) located in and near to the Coral Sea region to infer spatial mean SST anomalies (SSTAs) for January–March, the months when maximum SST and thermal bleaching are most likely to occur in the Coral Sea 16 , 26 , each year from 1618 to 1995 ( Methods and Supplementary Information ). Anthropogenic climate change began and proceeded entirely within the multi-century lives of some of these massive coral colonies, offering a continuous multi-century record covering the industrial era. We use this 1618–1995 reconstruction and the available 1900–2024 instrumental data to contextualize the modern trend and rank four centuries of January–March SSTAs with greater precision than was previously possible. We then assess the degree of human influence on ocean temperatures in the region using climate model simulations run both with and without anthropogenic forcing.
The instrumental period (1900–present)
Mass coral bleaching on the GBR in 2016, 2017, 2020, 2022 and 2024 during January–March coincided with widespread warm SSTAs in the surrounding seas 1 , including the Coral Sea (Fig. 1a–e , using ERSSTv5 data 27 ). The Coral Sea and GBR have experienced a strong warming trend since 1900 (Fig. 1f ). January–March SSTAs averaged over the GBR are strongly correlated ( ρ = 0.84, P ≪ 0.01) with those in the broader Coral Sea (Fig. 1f ), including when the long-term warming trend is removed from both time series ( ρ = 0.69, P < 0.01; Supplementary Fig. 4 ). Based on the strength of this correlation, we associate high January–March area-averaged Coral Sea SSTAs with increased thermal bleaching risk in the GBR.
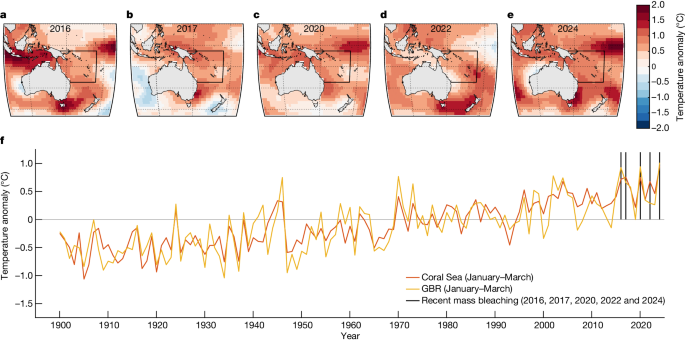
a – e , SSTAs (using ERSSTv5 data) for January–March in the Australasian region relative to the 1961–90 average for the five recent GBR mass coral bleaching years: 2016, 2017, 2020, 2022 and 2024. The black box shows the Coral Sea region (4° S–26° S, 142° E–174° E). f , Coral Sea and GBR mean SSTAs for 1900–2024 in January–March relative to the 1961–90 average. The black vertical lines indicate the five recent GBR mass coral bleaching years.
Record temperatures were set in 2016 and 2017 in the Coral Sea, and in 2020 they peaked fractionally below the record high of 2017. The January–March of 2022 was another warm event, the fifth warmest on record at the time. Recent data (ERSSTv5) indicate that 2024 set a new record by a margin of more than 0.19 °C above the previous record for the region. The January–March mean SSTs averaged over the five mass bleaching years during the period 2016–2024 are 0.77 °C higher than the 1961–90 January–March averages in both the Coral Sea and the GBR. The multidecadal warming trend, extreme years and association between GBR and Coral Sea SSTs are similar for the HadISST 28 gridded SST dataset, with some notable differences in the 1900–40 period (Supplementary Fig. 3 ). Furthermore, analysis of modern temperature-sensitive Sr/Ca series from GBR corals for 1900–2017 provides coherent independent evidence of statistically significant multi-decadal warming trends in January–March SSTs in the central and southern GBR (Supplementary Information section 4.2 ).
A multi-century context (1618–present)
Reconstructing Coral Sea January–March SSTs from 1618 to 1995 extends the century-long instrumental record back in time by an additional three centuries (Fig. 2a and Methods ). The reconstruction (calibrated to ERSSTv5) shows that multi-decadal SST variability was a persistent feature in the past. At the centennial timescale, there is relative stability before 1900, with the exception that cooler temperatures prevailed in the 1600s. Warming during the industrial era has been evident since the early 1900s (Fig. 2a ). There is a warming trend for January–March of 0.09 °C per decade for 1900–2024 and 0.12 °C per decade for 1960–2024 (Fig. 1f ) using ERSSTv5 data. Calibrating our reconstruction to HadISST1.1 yields similar results, with some differences in the degree of pre-1900 variability at both multi-decadal and centennial timescales (Supplementary Information section 5.2.6 ).
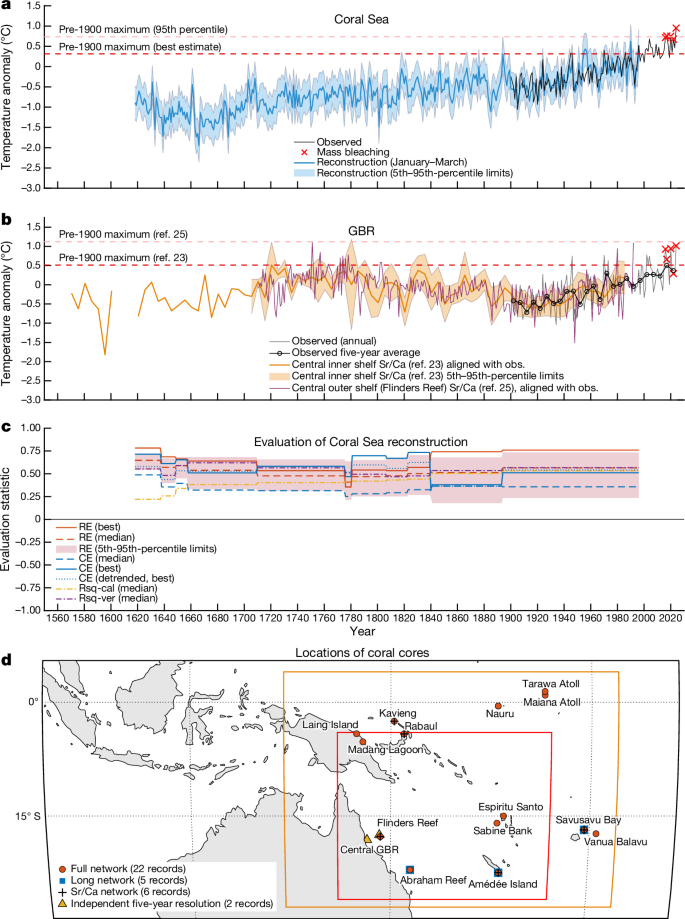
a , Reconstructed and observed mean January–March SSTAs in the Coral Sea for 1618–2024 relative to 1961–90. Dark blue, highest skill (maximum coefficient of efficiency) reconstruction with the full proxy network; light blue, 5th–95th-percentile reconstruction uncertainty; black, observed (ERSSTv5) data. Red crosses indicate the five recent mass bleaching events. Dashed lines indicate the best estimate (highest skill, red) and 95th-percentile (pink) uncertainty bound for the maximum pre-1900 January–March SSTA. b , Central GBR SSTA for the inner shelf 23 in thick orange and outer shelf 25 (Flinders Reef) in thin orange lines; these series are aligned here (see Methods ) with modern observations of mean GBR SSTAs for January–March relative to 1961–90. Observed data are shown at annual (grey line) and five-year (black line with open circles, plotted at the centre of each five-year period and temporally aligned with the five-year coral series 23 ) resolution. Dashed lines indicate best-estimate pre-1900 January–March maxima for refs. 23 (red) and 25 (pink). Orange shading indicates 5th–95th-percentile uncertainty bounds. Red crosses indicate the five recent mass bleaching events. c , Evaluation metrics for the Coral Sea reconstruction (Supplementary Information section 3.1 ); RE, reduction of error; CE, coefficient of efficiency; Rsq-cal, R-squared in the calibration period; Rsq-ver, R-squared in the verification (evaluation) period. d , Coral data locations relative to source data region (orange box) and Coral Sea region (red box). Coral proxy metadata are given in Supplementary Tables 1 and 2 .
Our best-estimate (highest skill; Methods ) annual-resolution Coral Sea reconstruction (Fig. 2a ), using the full coral network calibrated to the ERSSTv5 instrumental data, indicates that the January–March mean SSTAs in 2016, 2017, 2020, 2022 and 2024 were, respectively, 1.50 °C, 1.54 °C, 1.53 °C, 1.46 °C and 1.73 °C above the 1618–1899 (hereafter ‘pre-1900’) reconstructed average. Using the same best-estimate reconstruction, Coral Sea January–March SSTs during these GBR mass bleaching years were five of the six warmest years the region has experienced in the past 400 years (Fig. 2a ).
By comparing the recent warm events to the reconstruction’s uncertainty range ( Methods ), we quantify, using likelihood terminology consistent with recent reports from the Intergovernmental Panel on Climate Change 19 , that the recent heat extremes in 2017, 2020 and 2024 are ‘extremely likely’ (>95th percentile; Fig. 2a ) to be higher than any January–March in the period 1618–1899. Furthermore, the heat extremes in 2016 and 2022 are (at least) ‘very likely’ (>90th percentile) to be above the pre-1900 maximum. We perform a series of tests that verify that our findings are not simply an artefact of the nature of the coral network itself (Supplementary Information section 5.2 ). In a network perturbation test, we generate 22 subsets of the reconstruction by adding proxy records incrementally in order from the highest to the lowest correlation with the target (Supplementary Information section 5.2.5 ). We confirm that 2017, 2020 and 2024 were ‘extremely likely’ (>95th percentile) to have been warmer than any year pre-1900 (using ERSSTv5 data) for all of these proxy subsets. Furthermore, in 20 of the 22 subsets, 2016 was also ‘extremely likely’ (>95th percentile), rather than ‘very likely’, to be warmer (2022 was ‘extremely likely’ in 14 of the 22 subsets). All our additional tests, including a reconstruction with only Sr/Ca coral data (thereby omitting the possibility of any non-temperature signal in δ 18 O coral on the reconstruction), achieve high reconstruction skill and confirm the extraordinary nature of recent extreme temperatures in the multi-century context (Supplementary Information section 5.2 ). Analyses using HadISST1.1 generally show lower correlations with the coral data and reconstructions with slightly warmer regional SSTs before 1900, along with more-muted centennial and multi-decadal variability in the pre-instrumental period. Nevertheless, the HadISST1.1-calibrated reconstructions show that the recent thermal extremes are well above the best estimate (highest skill) of the pre-1900 maximum of reconstructed January–March SSTAs (Supplementary Fig. 42 ). Furthermore, lower SSTAs (in the HadISST1.1 data) relative to the previous three centuries (as in our reconstructions calibrated to HadISST1.1), coupled with the recently observed mass coral bleaching events, could indicate that long-lived corals have a greater sensitivity to warming than is currently recognized.
Reconstructed regional GBR SSTAs based on a five-year-resolution, multi-century coral δ 18 O record from the central inshore GBR 23 (Fig. 2b ) show similarly strong warming since 1900 but more multi-decadal-to-centennial variability than the Coral Sea reconstruction. Recent five-year mean January–March GBR SSTAs narrowly exceed the best estimate of the maximum pre-1900 five-year mean since the early 1600s (Fig. 2b ). The averages for the five-year periods centred on 2018 and 2022 exceed the pre-1900 maximum by 0.11 °C and 0.06 °C, respectively. Results are similar using the five-year-resolution Flinders Reef (central outer shelf) 24 record (Supplementary Fig. 24 ), although its interpretation is limited by the lack of uncertainty estimates available for that record. Our Coral Sea reconstruction incorporates an updated (annual resolution) record from Flinders Reef 25 , which indicates similar centennial trends (thin orange line in Fig. 2b ) and shows that the recent high January–March SSTA events have approached the estimated local pre-1900 maximum SSTA. Although contiguous multi-century cores from within the GBR are limited in their spatial extent, twentieth-century warming is evident in these records.
The extraordinary nature of the recent Coral Sea January–March SSTs in the context of the past 400 years is further illustrated by comparing the ranked temperature anomalies (Fig. 3 ) for the combined reconstructed and instrumental period from 1618–2024, incorporating reconstruction uncertainty ( Methods ). The mass coral bleaching years of 2016, 2017, 2020, 2022 and 2024, and the heat event of 2004, stand out as the warmest events across the whole 407-year record. The warmest three years (2024, 2017 and 2020) exceed the upper uncertainty bound (95th percentile) of the warmest reconstructed January–March in the pre-1900 period (pink (upper) dashed line in Fig. 3 ); 2016, 2004 and 2022 exceed the 90th percentile bound (red (lower) dashed line in Fig. 3 ). The warming trend is clear in the association between the ascending rank of the temperature anomalies and the year (shown as the colour of the filled circles in Fig. 3 ). Despite high interannual variability, 78 of the warmest 100 January–March periods between 1618 and 2024 occurred after 1900, and the 23 warmest all occur after 1900. The warmest 20 January–March periods all occur after 1950, coinciding with accelerated global warming.
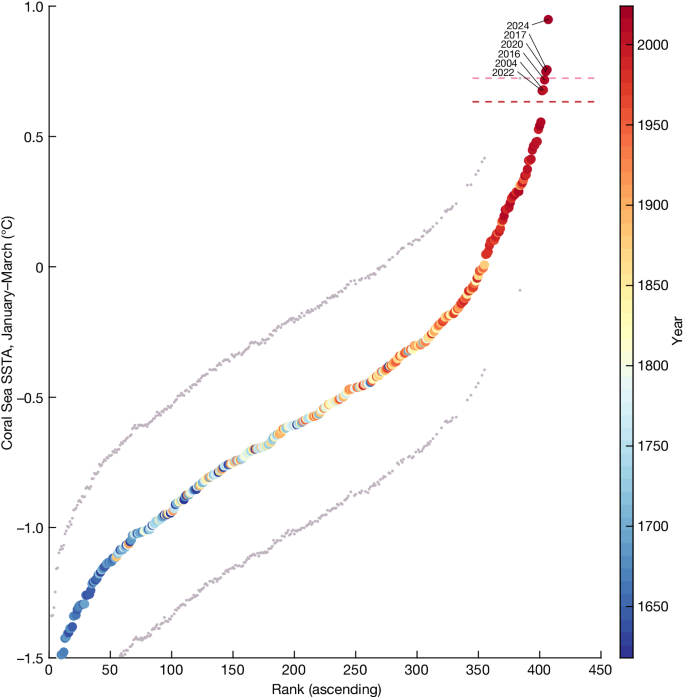
Ranked January–March SSTAs for 1618–2024 relative to 1961–90 (coloured circles) from the best-estimate (highest skill, full coral network) reconstruction (1618–1899) and instrumental (ERSSTv5) data (1900–2024). The year is indicated by the colour of the filled circles. The 5th–95th-percentile uncertainty bounds of the pre-1900 reconstructed SSTAs are shown by small grey dots. The year labels indicate the warmest six years on record, five of which were mass coral bleaching years on the GBR. The pink (upper) dashed line indicates the 95th-percentile uncertainty bound of the maximum pre-1900 reconstructed SSTA; the red (lower) dashed line indicates the 90th-percentile limit.
Assessing anthropogenic influence
Using climate model simulations from the most recent (sixth) phase of the Coupled Model Intercomparison Project 29 (CMIP6), we assess the human influence on January–March SSTAs in the Coral Sea. The model simulations are from two experiments in the Detection and Attribution Model Intercomparison Project (DAMIP) 30 . The first set of simulations represents historical climate conditions, including both the natural and human influences on the climate system over the 1850–2014 period (‘historical’; red in Fig. 4 ). The second experiment is a counterfactual climate that spans the same period and uses the same models but includes only natural influences on the climate, omitting all human influences (‘historical-natural’; blue in Fig. 4 ). The historical experiment includes anthropogenic emissions of greenhouse gases and aerosols, stratospheric ozone changes and anthropogenic land-use changes; the historical-natural experiment does not. Variations in natural climate forcings, such as from volcanic eruptions and solar variability, are incorporated in both experiments. We include models that have a transient climate response (the global mean surface-temperature anomaly at the time of a doubling of atmospheric CO 2 concentration) in the range 1.4–2.2 °C, which is deemed ‘likely’ by the science community 31 ( Methods and Supplementary Information ).
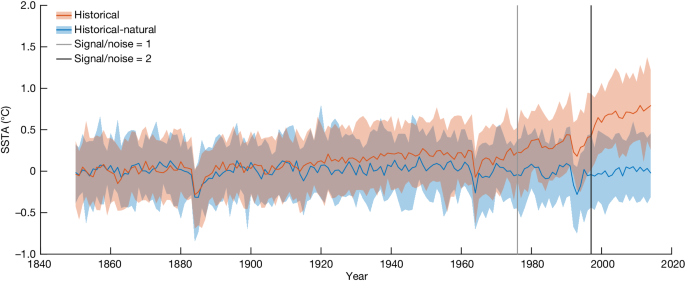
Climate-model simulations of Coral Sea January–March SSTAs relative to the 1850–1900 average for the period 1850–2014, for models within the ‘likely’ range for their transient climate response 31 . The blue line (median) and light blue shading (5th–95th-percentile limits) are from the ‘historical-natural’ climate model simulations (no anthropogenic climate forcing); the red line and light red shading are from the ‘historical’ simulations (anthropogenic influences on the climate included) using the same set of climate models. The climate-model-derived time of emergence of anthropogenic climate change, shown by the grey and black vertical lines (1976 and 1997), is when the ratio of the climate change signal to the standard deviation of noise/variability 32 across model ensemble members first rises above 1 and 2, respectively. All models are represented equally in the model ensemble.
It is only with the incorporation of anthropogenic influences on the climate that the model simulations capture the modern-era warming of the Coral Sea January–March SSTA (Fig. 4 ). The median of the historical simulations has statistically significant warming trends of 0.05 °C, 0.10 °C and 0.15 °C per decade for the periods from 1900, 1950 and 1970 to 2014, respectively; the equivalent historical-natural trends are smaller in magnitude than ±0.01 °C per decade. To further explore the centennial-scale trends, we use a bootstrap ensemble ( Methods ) of the two sets of 165-year simulations from 1850–2014. We found that 100% of the historical bootstrap ensemble has statistically significant positive trends ( Methods ) for 1900–2014, but this value is 0% for the historical-natural ensemble. The observed (ERSSTv5) mean SSTA for 2016–2024 of 0.60 °C relative to 1961–90 is warmer than any nine-year sequence in the 7,095 simulated years in the historical-natural experiments from models with transient climate responses in the ‘likely’ range 31 .
We also use the simulations to estimate the time of emergence of the anthropogenic influence on January–March Coral Sea SSTAs above the natural background variability. The anthropogenic warming signal 32 increases from near zero in 1900 to around 0.5 standard deviations of the variability (‘noise’) in 1960. The climate change signal-to-noise ratio then increases rapidly from 1960 to 2014, exceeding 1.0 in 1976, 2.0 in 1997 and around 2.8 by 2014, the end of these simulations (Fig. 4 , Methods and Supplementary Fig. 50 ). Anthropogenic impacts on the climate are virtually certain to be the primary driver of this long-term warming in the Coral Sea.
Previously, our knowledge of the SST history of the GBR and the Coral Sea region has been highly dependent on instrumental observations, with the exception of the five-year-resolution multi-century coral Sr/Ca and U/Ca SST reconstructions from the two point locations in the central GBR 23 , 24 , an update at one of these locations 25 , seasonal resolution ‘floating’ (in time) chronologies from the GBR in the Holocene 33 , 34 and point SST estimates further back in time 35 . Thus, the context of recent warming trends in the Coral Sea and GBR and their relation to natural variability on decadal to centennial timescales is largely unknown without reconstructions such as the one we developed here.
Our coral proxy network is located mostly beyond the GBR, in the Coral Sea, and some series are located outside the Coral Sea region (Fig. 2d ). The selection of the Coral Sea as a study region allowed for a larger sample of contributing coral proxy data than exists for the GBR. However, coral bleaching on the GBR can be influenced by factors other than large-scale SST, including local oceanic and atmospheric dynamics that can modulate the occurrence and severity of thermal bleaching and mortality events 13 . Nonetheless, warming of seasonal SSTs over the larger Coral Sea region is likely to prime the background state and increase the likelihood of smaller spatio-temporal-scale heat anomalies. Furthermore, where we use only the five-year resolution series directly from the GBR to reconstruct GBR SSTAs, we draw similar conclusions about the long-term trajectory of SSTAs as for our full coral network (Fig. 2b and Supplementary Fig. 24 ). Furthermore, short modern coral series from within the GBR, analysed in this study, document a multi-decadal warming signal that is coherent with instrumental data (Supplementary Figs. 29 and 30 ). Nonetheless, additional high-resolution, multi-century, temperature-sensitive coral geochemical series from within the GBR would help unravel the local and remote ocean–atmosphere contributions to past bleaching events and reduce uncertainties.
The focus on the larger Coral Sea study region also takes advantage of the global modelling efforts of CMIP6. The large number of ensemble members available for CMIP6 means that greater climate model diversity, and therefore greater certainty in our attribution analysis, is possible compared with most single model analyses. There is also a methodological benefit in having high replication of the same experiments run with multiple climate models. However, coarse-resolution global-scale models do not accurately simulate smaller-scale processes, such as inshore currents and mesoscale eddies in the Coral Sea or the Gulf of Carpentaria, which probably affect local surface temperatures and variations in nutrient upwelling in the GBR 36 , 37 . Upwelling on the GBR is linked to the strength of the East Australian Current 16 , the southward branch of the South Pacific subtropical gyre. The CMIP-scale models we use do capture these gyre dynamics. The models show that the East Australian Current is expected to increase in strength as the climate continues to warm through this century 38 , and this may lead to more nutrient inputs that can exacerbate coral sensitivity to rising heat stress 39 , 40 . As well as focusing our model analysis on the larger Coral Sea region, we use a three-month time step. In doing so, we minimize the impact of model spatio-temporal resolution on our inferences about the role of anthropogenic greenhouse-gas emissions on the SST conditions that give rise to GBR mass bleaching.
Remaining uncertainties
We present analyses and interpretations that are as robust as possible given currently available data and methods. However, several sources of remaining uncertainty mean that future reconstructions of past Coral Sea and GBR SSTs could differ from those presented here. Although bias corrections are applied to observational SST datasets such as ERSST and HadISST, these datasets probably retain biases, especially for the period during and before 1945 (ref. 41 ), and these may not be fully accounted for in the uncertainty estimates 42 . Because our reconstructions are calibrated directly to these datasets, future observational-bias corrections are likely to improve proxy-based reconstructions.
Reconstructions of SST that use coral δ 18 O records may be susceptible to the influence of changes in the coral δ 18 O–SST relationship on time periods longer than the instrumental training period, along with non-SST changes in the δ 18 O of seawater, which can covary with salinity. As such, new coral records of temperature-sensitive trace-element ratios such as Sr/Ca, Li/Mg or U/Ca may prove influential in future efforts to distinguish between changes in past temperature and hydroclimate. Owing to the limited availability of multi-century coral data from within the GBR itself, the reconstructed low-frequency variability of GBR SSTs in recent centuries is likely to change as more temperature proxy data become available. It is also likely that new sub-annual resolution records would aid in removing potential signal damping or bias from our use of some annual-resolution records to reconstruct seasonal SSTAs.
Ecological consequences
With global warming of 0.8–1.1 °C above pre-industrial levels 19 there has been a marked increase in mass coral bleaching globally 43 . Even limiting global warming to the Paris Agreement’s ambitious 1.5 °C level would be likely to lead to the loss of 70–90% of corals that are on reefs today 44 . If all current international mitigation commitments are implemented, global mean surface temperature is still estimated to increase in the coming decades, with estimates varying between 1.9 °C (ref. 45 ) and 3.2 °C (ref. 46 ) above pre-industrial levels by the end of this century. Global warming above 2 °C would have disastrous consequences for coral ecosystems 19 , 44 and the hundreds of millions of people who currently depend on them.
Coral reefs of the future, if they can persist, are likely to have a different community structure to those in the recent past, probably one with much less diversity in coral species 4 . This is because mass bleaching events have a differential impact on different coral species. For example, fast-growing branching and tabulate corals are affected more than slower-growing massive species because they have different thermal tolerance 4 . The simplification of reef structures will have adverse impacts on the many thousands of species that rely on the complex three-dimensional structure of reefs 4 . Therefore, even with an ambitious long-term international mitigation goal, the ecological function 4 of the GBR is likely to deteriorate further 5 before it stabilizes.
Coral adaptation and acclimatization may be the only realistic prospect for the conservation of some parts of the GBR this century. However, although adaptation opportunities may be plausible to some extent 47 , they are no panacea because evolutionary changes to fundamental variables such as temperature take decades, if not centuries, to occur, especially in long-lived species such as reef-building corals 48 . There is currently no clear evidence of the real-time evolution of thermally tolerant corals 48 . Most rapid changes depend on a history of exposure to key genetic types and extremes, and there are limitations to genetic adaptation that prevent species-level adaptation to environments outside of their ecological and evolutionary history 19 . Model projections also indicate that rates of coral adaptation are too slow to keep pace with global warming 49 . In a rapidly warming world, the temperature conditions that give rise to mass coral bleaching events are likely to soon become commonplace. So, although we may see some resilience of coral to future marine heat events through acclimatization, thermal refugia are likely to be overwhelmed 50 . Global warming of more than 1.5 °C above pre-industrial levels will probably be catastrophic for coral reefs 44 .
Our new multi-century reconstruction illustrates the exceptional nature of ocean surface warming in the Coral Sea today and the resulting existential risk for the reef-building corals that are the backbone of the GBR. The reconstruction shows that SSTs were relatively cool and stable for hundreds of years, and that recent January–March ocean surface heat in the Coral Sea is unprecedented in at least the past 400 years. The coral colonies and reefs that have lived through the past several centuries, and that yielded the valuable Sr/Ca and δ 18 O data on which our reconstruction is based, are themselves under serious threat. Our analysis of climate-model simulations confirms that human influence is the driver of recent January–March Coral Sea surface warming. Together, the evidence presented in our study indicates that the GBR is in danger. Given this, it is conceivable that UNESCO may in the future reconsider its determination that the iconic GBR is not in danger. In the absence of rapid, coordinated and ambitious global action to combat climate change, we will likely be witness to the demise of one of Earth’s great natural wonders.
Instrumental observations
The Coral Sea and GBR area-averaged monthly SSTAs relative to 1961–90 for January–March are obtained from version 5 of the Extended Reconstructed Sea Surface Temperature dataset (ERSSTv5) 27 . We compare our results using ERSSTv5 with those generated using the Hadley Centre Sea Ice and Sea Surface Temperature dataset (HadISST1.1) 28 . We use only post-1900 instrumental SST observations here. Although gridded datasets have some coverage before 1900, ship-derived temperature data in the region for that period are too sparse to be reliable for calibrating our reconstruction (Supplementary Information section 1.2 ). The regional mean for the GBR is computed using the seven grid-cell locations used by the Australian Bureau of Meteorology (Supplementary Information section 1.1 ). We define the Coral Sea region as the ocean areas inside 4° S–26° S, 142° E–174° E.
Coral-derived temperature proxy data
We use a network of 22 published and publicly available sub-annual and annual resolution temperature-sensitive coral geochemical series (proxies; Fig. 2d , Supplementary Tables 1 and 2 , and Supplementary Fig. 5a–v ) from the western tropical Pacific in our source data region (4° N–27° S, 134° E–184° E) that cover at least the period from 1900 to 1995. Of these 22 series, 16 are δ 18 O, which are in per mil (‰) notation relative to Vienna PeeDee Belemnite (VPDB) 51 ; the remaining six are Sr/Ca series. The coral data are used as predictors in the reconstruction of January–March mean SSTAs in the Coral Sea region. We apply the inverse Rosenblatt transformation 52 , 53 to the coral data to ensure that our reconstruction predictors are normally distributed. Sub-annually resolved series are converted to the annual time step by averaging across the November–April window. This maximizes the detection of the summer peak values, allowing for some inaccuracy in sub-annual dating and the timing of coral skeleton deposition 54 , 55 . A small fraction (less than 0.8%) of missing data is infilled using the regularized expectation maximization (RegEM) algorithm 56 (Supplementary Information section 2.3 ), after which the proxy series are standardized such that each has a mean of zero and a standard deviation of one over their common 1900–1995 period.
Reconstruction method
To produce our Coral Sea reconstruction, we use nested principal component regression 57 (PCR), in which the principal components of the network of 22 coral proxies are used as regressors against the target-region January–March SSTA relative to the 1961–90 average. We perform the reconstructions separately for each nest of proxies, where a nest is a set of proxies that cover the same time period. The longest nest dates back to 1618, when at least two series are available. The nests allow for the use of all coral proxies over the full time period of their coverage. The 96-year portion of the instrumental period (1900–1995) that overlaps with the reconstruction period is used for calibration and evaluation (or equivalently, verification) against observations. We reconstruct regional SSTAs from the principal components of the coral network of δ 18 O and Sr/Ca data, rather than their local SST calibrations, to minimize the number of computational steps and to aid in representing the full reconstruction uncertainty.
Principal component analysis (PCA) is used to reduce the dimensionality of the proxy matrix, as follows. Let P ( t , r ) denote the palaeoclimate-data matrix during the time period t = 1,..., n at an annual time step for proxy series r = 1,..., p . PCA is undertaken on this matrix during the calibration period, P cal . We obtain the principal component coefficients matrix P coeff ( r , e ) for principal components e = 1,..., n PC and principal component scores P score ( t , e ), which are representations of the input matrix P cal in the principal component space. P score is truncated to include n PC,use principal components to form \({P}_{{\rm{score}}}^{{\prime} }\) such that the variance of the proxy network explained by the n PC,use principal components is greater than \({\sigma }_{{\rm{expl}}}^{2}\) (which we set to 95%). Reconstruction tests in which \({\sigma }_{{\rm{expl}}}^{2}\) is varied from 70% to 95% show that our results are not strongly sensitive to this choice, and tests based on lag-one autoregressive noise for \({\sigma }_{{\rm{expl}}}^{2}\) from 50% to 99% further support this choice (Supplementary Information section 3.2 ). These principal components are used as predictors against which the Coral Sea January–March instrumental SSTAs are regressed. We regress the standardized SSTA target data during the calibration period, I cal , against the retained principal components of the predictor data, \({P}_{{\rm{score}}}^{{\prime} }\) :
Thus, we obtain n PC,use estimates of the regression coefficients γ e with gaussian error term ε t ~ N (0, \({\sigma }_{N}^{2}\) ). The principal components are extended back into the pre-instrumental period by multiplying the entire proxy matrix P ( t , p ) with the truncated principal component coefficient matrix \({P}_{{\rm{coeff}}}^{{\prime} }\) ( t , e ) to obtain \({Q}_{{\rm{coeff}}}^{{\prime} }\) :
The reconstruction proceeds with the fitted regression coefficients γ e and extended coefficient matrix \({Q}_{{\rm{coeff}}}^{{\prime} }\) to obtain a reconstruction time series R m ( t ) for a given nest of proxy series
The standardized reconstruction R m ( t ) is then calibrated to the instrumental data such that the standard deviation and mean of the reconstruction and target during the calibration interval are equal. As well as obtaining reconstructions for each nest of available proxies, we compute stitched reconstructions S c ( t ) for each calibration period c , which include at each time step the reconstructed data for the proxy nest with maximum coefficient of efficiency 58 , 59 (Supplementary Information section 3.1 ). This procedure is performed for contiguous calibration intervals between 60 and 80 years duration between 1900 and 1995, with interval width and location increments of two years, reserving the remaining data in the overlapping period for independent evaluation, and for all proxy nests. The reconstruction error is modelled with a lag-one autoregressive process fitted to the residuals. We evaluate the capacity of our reconstruction method to achieve spurious skill from overfitting by performing a test in which we replace the coral data with synthetic noise (Supplementary Information section 3.2i ). We find that reconstructions based on synthetic noise achieve extremely low or zero skill and as more noise principal components are included in the regression, the evaluation metrics indicate declining skill. Our reconstruction and evaluation methods therefore guard against the potential for spurious skill.
Pseudo-proxy reconstructions
Our reconstruction method is further evaluated by using a pseudo-proxy modelling approach based on the Community Earth System Model (CESM) Last Millennium Experiment (LME) 60 , for which there are 13 full-forcing ensemble members covering the period 850–2005. We use the pseudo-proxy reconstructions to evaluate our reconstruction method and coral network in a fully coupled climate-model environment. We form pseudo-proxies by extracting from each LME ensemble member the SST and sea surface salinity (SSS) from the 1.5° × 1.5° grid cell located nearest to our coral data. We then apply proxy system models in the form of linear regression models, basing δ 18 O on both SST and SSS, and Sr/Ca on SST only (Supplementary Information section 3.3 ). We set the spatial and temporal availability of the pseudo-coral network to match that of the coral network. We then apply our PCR reconstruction and evaluation procedure to the pseudo-proxy network, taking advantage of the availability of the modelled Coral Sea SSTA data across the multi-century period of 1618–2005, which allows for the evaluation of the pseudo-proxy reconstruction over this entire time period. We first test our method using a ‘perfect proxy’ approach (with no proxy measurement error) before superimposing synthetic noise on the pseudo-proxy time series, evaluating our methodology at two separate levels of measurement error, quantified by signal-to-noise ratios of 1.0 and 4.0. The evaluation metrics for these tests indicate that our coral network and reconstruction method obtain skilful reconstructions of Coral Sea SSTAs in the climate-model environment (Supplementary Figs. 17b , 18 , 20b , 21 , 22b and 23 ).
Comparison with independent coral datasets
We use two multi-century five-year-resolution coral series from the central GBR 23 , 24 (Fig. 2b and Supplementary Fig. 24 ) and a network of sub-annual and annual resolution modern coral series (dated from 1900 onwards but not covering the full 1900–1995 period) from 44 sites in the GBR (Supplementary Information section 4.2 ) for independent evaluation of coral-derived evidence for warming in the region. We estimate five-year GBR SSTAs (Fig. 2b ) by aligning the post-1900 mean and variance of the proxy and instrumental (ERSSTv5) data.
Reconstruction sensitivity to non-SST influences
Of the 22 available coral series, 16 are records of δ 18 O, a widely used measure of the ratio of the stable isotopes 18 O and 16 O. In the tropical Pacific Ocean, δ 18 O is significantly correlated with SST 61 , 62 , 63 , 64 . Coral δ 18 O is also sensitive to the δ 18 O of seawater 65 , which can reflect advection of different water masses and/or changes in freshwater input, such as from riverine sources or precipitation, which in turn co-vary with SSS. Thus, it is generally considered that the main non-SST contributions to coral δ 18 O are processes that co-vary with SSS 62 , 66 . Our methodology minimizes the influence of non-temperature impacts on the reconstruction by exploiting the contrast in spatial heterogeneity between SST and SSS in January–March (Supplementary Information section 5.1 ). SSS is spatially inhomogeneous in the tropical Pacific 66 , 67 , leading to low coherence in SSS signals across our coral network. By contrast, the strong and coherent SST signal across our coral network locations and the Coral Sea region leads to principal components that are strongly representative of SST variations. This produces a skilful reconstruction of SST, as determined by evaluation against independent observations, and low correlations with SSS across the Coral Sea region (Supplementary Fig. 31 ).
Although the likelihood of non-SST influences on our SST reconstruction is low, we nonetheless test the sensitivity of our reconstruction and its associated interpretations to the possibility of these influences on the coral data. The tests compute the correlations between our best-estimate SSTA reconstruction (highest coefficient of efficiency) and observations of SSS, along with a series of additional reconstructions based on subsets of our coral network. The correlations between our highest coefficient of efficiency January–March Coral Sea SSTA reconstruction and January–March SSS are mapped for the Coral Sea and its neighbouring domain using three instrumental SSS datasets (Supplementary Fig. 31 ). Correlations are not statistically significant over most of the domain. Noting differing spatial correlation patterns between the instrumental SSS datasets 68 , which also cover different time periods (Supplementary Information section 5.1 ), we undertake six sensitivity tests using subsets of the coral network (Supplementary Information section 5.2 ). We use the following combinations of coral series: (1) the full network of 22 δ 18 O and Sr/Ca series (Figs. 2a and 3 ); (2) a subset of the six available Sr/Ca series (Supplementary Figs. 32 – 33 ), to test how the reconstruction is influenced by the inclusion of coral δ 18 O records; (3) a fixed nest subset of the five longest coral series, extending back to at least 1700 (Supplementary Figs. 34 – 35 ), to test for the potential influence of combining series of differing lengths (from our splicing of portions of the best reconstructions from each nest); (4) a subset of the ten coral series that are most strongly correlated with the target (Supplementary Figs. 36 and 37 ), to test how our reconstruction is influenced by the inclusion of coral series that are less strongly correlated with our target; (5) a subset of coral series that excludes the six records that are reported to potentially include biological mediation or non-climatic effects, or have low correlation with the target (Supplementary Figs. 38 and 39 ), to test their influence on the reconstruction; and (6) a network perturbation test comprising 22 separate subsets of proxies, in which proxy records are added incrementally in order of highest to lowest correlation with the target, starting with a single coral series and increasing the number of included proxies to all 22 series in our network (Supplementary Information section 5.2.5 ), to systematically quantify the influence of gradually including more coral datasets on our reconstruction and its interpretations.
The evaluation metrics (Fig. 2c and Supplementary Figs. 32b , 34b , 36b and 38b ) indicate a skilful reconstruction back to 1618 for the reconstructions based on the Full, Sr/Ca only, Long, Best-10 and OmitBioMed networks. These reconstructions explain 82.7%, 80.6%, 77.6%, 79.8% and 80.4% (R-squared values) of the variance in January–March SSTAs, respectively, in the independent evaluation periods (using ERSSTv5b). All coral subsets in the network perturbation test produce skilful reconstructions (Supplementary Fig. 40 ). The highest-skill reconstructions for all subsets in the network perturbation test align with our key interpretations (Supplementary Figs. 41 and 42 ). Together, our sensitivity tests show that the coral network, observational data and reconstruction methodology are a sound basis for reconstructing Coral Sea January–March SSTAs in past centuries and contextualizing recent high-SST events ( Supplementary Information ).
Climate-model attribution ensembles and experiments
The multi-model attribution analysis used here is based on simulations from CMIP6. We analyse simulations from the historical experiment (including natural and anthropogenic influences for 1850–2014) and the historical-natural experiment (natural-only forcings for 1850–2014). We select climate models for which monthly surface temperature is available in at least three historical and historical-natural simulations (Supplementary Table 5 ). All model simulations are interpolated to a common regular 1.5° × 1.5° latitude–longitude grid. January–March SSTAs relative to 1961–90 are calculated for each simulation. The full historical all-forcings ensemble is composed of 14 models with 268 simulations for 1850–2014. The natural-only ensemble is composed of the same 14 models with 95 individual simulations. A subset of climate models in the CMIP6 ensemble are considered by the science community to be ‘too hot’, simulating warming in response to increased atmospheric carbon dioxide concentrations that is larger than that supported by independent evidence 31 . We omit these models from our analysis by including only models with a transient climate response in the ‘likely’ range 31 of 1.4–2.2 °C. Our results are not strongly sensitive to this selection (Supplementary Information section 6.3 ). The ten remaining models yield a total of 25,410 years from 154 historical ensemble members and 7,095 years from 43 historical-natural ensemble members. We weight the models equally in our analysis using bootstrap sampling. We report linear trends based on simple linear regression models fitted with ordinary least squares. The statistical significance of linear trends is assessed using the Spearman’s rank correlation test 69 .
Time of emergence of the anthropogenic impact
We assess the anthropogenic influence on SSTAs in the Coral Sea region by starting with the assumption that any anthropogenic influence on SSTAs in the Coral Sea is indistinguishable from natural variability at the commencement of the model experiments. We measure the impact of anthropogenic influence on the climate in the region using a signal-to-noise approach 32 , 70 . We calculate the anthropogenic ‘signal’ as the mean of the difference between the smoothed (using a 41-year Lowess filter) modelled historical Coral Sea SSTA and the mean smoothed modelled historical-natural SSTA. Our ‘noise’ is the standard deviation of the difference between the modelled historical SSTA and its smoothed time series (Supplementary Information section 6 ).
Methods additionally rely on Supplementary Information and refs. 71 , 72 , 73 , 74 , 75 , 76 , 77 , 78 , 79 , 80 , 81 , 82 , 83 , 84 , 85 , 86 , 87 , 88 , 89 , 90 , 91 , 92 , 93 , 94 , 95 , 96 , 97 , 98 , 99 , 100 , 101 , 102 , 103 , 104 .
Data availability
The ERSSTv5 instrumental SST data are available from the US National Oceanic and Atmospheric Administration at https://psl.noaa.gov/data/gridded/data.noaa.ersst.v5.html . The HadISST1.1 data are available from the UK Met Office at https://www.metoffice.gov.uk/hadobs/hadisst/ . The original coral palaeoclimate data are available at the links provided in Supplementary Table 2 . Land areas for maps are obtained from the Mapping Toolbox v.23.2 in Matlab v.2023b and the Global Self-consistent, Hierarchical, High-resolution Geography (GSHHS) Database at https://www.soest.hawaii.edu/pwessel/gshhg/ through the m_map toolbox by R. Pawlowicz, available at https://www.eoas.ubc.ca/%7Erich/map.html . Prepared data from the coral geochemical series, reconstructions and climate models that support the findings of this study are available at: https://doi.org/10.24433/CO.4883292.v1 .
Code availability
The code that supports the findings of this study is available and can be run at : https://doi.org/10.24433/CO.4883292.v1 .
Australian Institute of Marine Science. Long-Term Monitoring Program. https://www.aims.gov.au/research-topics/monitoring-and-discovery/monitoring-great-barrier-reef/long-term-monitoring-program (2024).
Hughes, T. P. et al. Global warming and recurrent mass bleaching of corals. Nature 543 , 373–377 (2017).
Article ADS CAS PubMed Google Scholar
Hoegh-Guldberg, O. Climate change, coral bleaching and the future of the world’s coral reefs. Mar. Freshw. Res. 50 , 839–866 (1999).
Google Scholar
Hughes, T. P. et al. Global warming transforms coral reef assemblages. Nature 556 , 492–496 (2018).
Great Barrier Reef Marine Park Authority. Great Barrier Reef Outlook Report 2019 (Great Barrier Reef Marine Park Authority, 2019).
Hughes, T. P. et al. Coral reefs in the Anthropocene. Nature 546 , 82–90 (2017).
Davis, K. L., Colefax, A. P., Tucker, J. P., Kelaher, B. P. & Santos, I. R. Global coral reef ecosystems exhibit declining calcification and increasing primary productivity. Commun. Earth Environ. 2 , 105 (2021).
Article ADS Google Scholar
Westcott, D. A. et al. Relative efficacy of three approaches to mitigate Crown-of-Thorns Starfish outbreaks on Australia’s Great Barrier Reef. Sci. Rep. 10 , 12594 (2020).
Article ADS CAS PubMed PubMed Central Google Scholar
Mellin, C. et al. Spatial resilience of the Great Barrier Reef under cumulative disturbance impacts. Glob. Chang. Biol. 25 , 2431–2445 (2019).
Article ADS PubMed Google Scholar
Jackson, J. B. C. et al. Historical overfishing and the recent collapse of coastal ecosystems. Science 293 , 629–637 (2001).
Article CAS PubMed Google Scholar
Hoegh-Guldberg, O. & Smith, G. J. The effect of sudden changes in temperature, light and salinity on the population density and export of zooxanthellae from the reef corals Stylophora pistillata Esper and Seriatopora hystrix Dana. J. Exp. Mar. Biol. Ecol. 129 , 279–303 (1989).
Article Google Scholar
DeCarlo, T. M. et al. Acclimatization of massive reef-building corals to consecutive heatwaves. Proc. Biol. Sci. 286 , 20190235 (2019).
PubMed PubMed Central Google Scholar
McGowan, H. & Theobald, A. ENSO weather and coral bleaching on the Great Barrier Reef, Australia. Geophys. Res. Lett. 44 , 10,601–10,607 (2017).
Zhao, W., Huang, Y., Siems, S. & Manton, M. The role of clouds in coral bleaching events over the Great Barrier Reef. Geophys. Res. Lett. 48 , e2021GL093936 (2021).
Oxley, W. G., Emslie, M., Muir, P. & Thompson, A. Marine Surveys Undertaken in the Lihou Reef National Nature Reserve (Australian Institute of Marine Science, 2004).
DeCarlo, T. M. & Harrison, H. B. An enigmatic decoupling between heat stress and coral bleaching on the Great Barrier Reef. PeerJ 7 , e7473 (2019).
Article PubMed PubMed Central Google Scholar
UNESCO World Heritage Committee. Extended 44th Session of the World Heritage Committee, Fuzhou (China) 16–31 July 2021 . Draft decision 44 COM 7B.90. https://whc.unesco.org/document/188005 (UNESCO, 2021).
UNESCO World Heritage Committee. Extended 45th Session of the World Heritage Committee, Riyadh (Saudi Arabia) 10–25 September 2023 . Decision 45 COM 7B.13. https://whc.unesco.org/document/199654 (UNESCO, 2023).
IPCC. Summary for Policymakers. In Climate Change 2021: The Physical Science Basis. Contribution of Working Group I to the Sixth Assessment Report of the Intergovernmental Panel on Climate Change (eds Masson-Delmotte, V. et al.) (Cambridge Univ. Press, 2021).
Kamenos, N. A. & Hennige, S. J. Reconstructing four centuries of temperature-induced coral bleaching on the Great Barrier Reef. Front. Mar. Sci. 5 , 283 (2018).
Hoegh-Guldberg, O. et al. Commentary: reconstructing four centuries of temperature-induced coral bleaching on the Great Barrier Reef. Front. Mar. Sci. 6 , 86 (2019).
DeCarlo, T. M. Commentary: reconstructing four centuries of temperature-induced coral bleaching on the Great Barrier Reef. Front. Mar. Sci. 7 , 30 (2020).
Hendy, E. J. et al. Abrupt decrease in tropical Pacific sea surface salinity at end of Little Ice Age. Science 295 , 1511–1514 (2002).
Calvo, E. et al. Interdecadal climate variability in the Coral Sea since 1708 A.D. Palaeogeogr. Palaeoclimatol. Palaeoecol. 248 , 190–201 (2007).
Zinke, J. et al. North Flinders Reef (Coral Sea, Australia) Porites sp. corals as a candidate global boundary stratotype section and point for the Anthropocene series. Anthropocene Rev. 10 , 201–224 (2023).
Spady, B. L. et al. Global Coral Bleaching Database (NCEI Accession 0228498) (NOAA National Centers for Environmental Information, 2022); https://www.ncei.noaa.gov/archive/accession/0228498 .
Huang, B. et al. Extended reconstructed sea surface temperature, Version 5 (ERSSTv5): Upgrades, validations, and intercomparisons. J. Clim. 30 , 8179–8205 (2017).
Rayner, N. A. et al. Global analyses of sea surface temperature, sea ice, and night marine air temperature since the late nineteenth century. J. Geophys. Res. Atmos. 108 , 4407 (2003).
Eyring, V. et al. Overview of the Coupled Model Intercomparison Project Phase 6 (CMIP6) experimental design and organization. Geosci. Model Dev. 9 , 1937–1958 (2016).
Gillett, N. P. et al. The Detection and Attribution Model Intercomparison Project (DAMIP v1.0) contribution to CMIP6. Geosci. Model Dev. 9 , 3685–3697 (2016).
Hausfather, Z., Marvel, K., Schmidt, G. A., Nielsen-Gammon, J. W. & Zelinka, M. Climate simulations: recognize the ‘hot model’ problem. Nature 605 , 26–29 (2022).
Hawkins, E. et al. Observed emergence of the climate change signal: from the familiar to the unknown. Geophys. Res. Lett. 47 , e2019GL086259 (2020).
Gagan, M. K. et al. Temperature and surface-ocean water balance of the mid-Holocene tropical western Pacific. Science 279 , 1014–1018 (1998).
Arzey, A. K. et al. Coral skeletal proxy records database for the Great Barrier Reef, Australia. Preprint at Earth Syst. Sci. Data https://doi.org/10.5194/essd-2024-159 (2024).
Brenner, L. D. et al. Coral record of Younger Dryas Chronozone warmth on the Great Barrier Reef. Paleoceanogr. Paleoclimatol. 35 , e2020PA003962 (2020).
Furnas, M. J. & Mitchell, A. W. Nutrient inputs into the central Great Barrier Reef (Australia) from subsurface intrusions of Coral Sea waters: a two-dimensional displacement model. Cont. Shelf Res. 16 , 1127–1148 (1996).
Wolanski, E., Andutta, F., Deleersnijder, E., Li, Y. & Thomas, C. J. The Gulf of Carpentaria heated Torres Strait and the Northern Great Barrier Reef during the 2016 mass coral bleaching event. Estuar. Coast. Shelf Sci. 194 , 172–181 (2017).
Oliver, E. C. J. & Holbrook, N. J. Extending our understanding of South Pacific gyre ‘spin-up’: modeling the East Australian Current in a future climate. J. Geophys. Res. Oceans 119 , 2788–2805 (2014).
DeCarlo, T. M. et al. Nutrient-supplying ocean currents modulate coral bleaching susceptibility. Sci. Adv. 6 , eabc5493 (2020).
Article ADS PubMed PubMed Central Google Scholar
Wiedenmann, J. et al. Nutrient enrichment can increase the susceptibility of reef corals to bleaching. Nat. Clim. Chang. 3 , 160–164 (2013).
Article ADS CAS Google Scholar
Chan, D. & Huybers, P. Correcting observational biases in sea surface temperature observations removes anomalous warmth during World War II. J. Clim. 34 , 4585–4602 (2021).
Chan, D., Kent, E. C., Berry, D. I. & Huybers, P. Correcting datasets leads to more homogeneous early-twentieth-century sea surface warming. Nature 571 , 393–397 (2019).
Hughes, T. P. et al. Spatial and temporal patterns of mass bleaching of corals in the Anthropocene. Science 359 , 80–83 (2018).
Hoegh-Guldberg, O. et al. Chapter 3: Impacts of 1.5°C global warming on natural and human systems. In Global Warming of 1.5°C (eds Masson-Delmotte, V. et al.) (IPCC, 2018).
Meinshausen, M. et al. Realization of Paris Agreement pledges may limit warming just below 2 °C. Nature 604 , 304–309 (2022).
Matthews, H. D. & Wynes, S. Current global efforts are insufficient to limit warming to 1.5 °C. Science 376 , 1404–1409 (2022).
Coles, S. L. et al. Evidence of acclimatization or adaptation in Hawaiian corals to higher ocean temperatures. PeerJ 6 , e5347 (2018).
Hughes, T. P., Baird, A. H., Morrison, T. H. & Torda, G. Principles for coral reef restoration in the anthropocene. One Earth 6 , 656–665 (2023).
Logan, C. A., Dunne, J. P., Ryan, J. S., Baskett, M. L. & Donner, S. D. Quantifying global potential for coral evolutionary response to climate change. Nat. Clim. Chang. 11 , 537–542 (2021).
Dixon, A. M., Forster, P. M., Heron, S. F., Stoner, A. M. K. & Beger, M. Future loss of local-scale thermal refugia in coral reef ecosystems. PLOS Clim. 1 , e0000004 (2022).
Coplen, T. B. Discontinuance of SMOW and PDB. Nature 375 , 285 (1995).
van Albada, S. J. & Robinson, P. A. Transformation of arbitrary distributions to the normal distribution with application to EEG test-retest reliability. J. Neurosci. Methods 161 , 205–211 (2007).
Article PubMed Google Scholar
Emile-Geay, J. & Tingley, M. Inferring climate variability from nonlinear proxies: application to palaeo-ENSO studies. Clim. Past 12 , 31–50 (2016).
Barnes, D. J., Taylor, R. B. & Lough, J. M. On the inclusion of trace materials into massive coral skeletons. Part II: distortions in skeletal records of annual climate cycles due to growth processes. J. Exp. Mar. Biol. Ecol. 194 , 251–275 (1995).
Article CAS Google Scholar
Gagan, M. K., Dunbar, G. B. & Suzuki, A. The effect of skeletal mass accumulation in Porites on coral Sr/Ca and δ 18 O paleothermometry. Paleoceanogr. Paleoclimatol. 27 , PA1203 (2012).
ADS Google Scholar
Schneider, T. Analysis of incomplete climate data: estimation of mean values and covariance matrices and imputation of missing values. J. Clim. 14 , 853–871 (2001).
PAGES 2k Consortium. Consistent multidecadal variability in global temperature reconstructions and simulations over the Common Era. Nat. Geosci. 12 , 643–649 (2019).
Cook, E. R., Briffa, K. R. & Jones, P. D. Spatial regression methods in dendroclimatology: a review and comparison of two techniques. Int. J. Climatol. 14 , 379–402 (1994).
Nash, J. E. & Sutcliffe, J. V. River flow forecasting through conceptual models part I − a discussion of principles. J. Hydrol. 10 , 282–290 (1970).
Otto-Bliesner, B. L. et al. Climate variability and change since 850 CE: an ensemble approach with the Community Earth System Model. Bull. Am. Meteorol. Soc. 97 , 735–754 (2016).
Evans, M. N., Kaplan, A. & Cane, M. A. Optimal sites for coral-based reconstruction of global sea surface temperature. Paleoceanogr. Paleoclimatol. 13 , 502–516 (1998).
Russon, T., Tudhope, A. W., Hegerl, G. C., Collins, M. & Tindall, J. Inter-annual tropical Pacific climate variability in an isotope-enabled CGCM: Implications for interpreting coral stable oxygen isotope records of ENSO. Clim. Past 9 , 1543–1557 (2013).
PAGES Hydro2k Consortium. Comparing proxy and model estimates of hydroclimate variability and change over the Common Era. Clim. Past 13 , 1851–1900 (2017).
Freund, M. B. et al. Higher frequency of Central Pacific El Niño events in recent decades relative to past centuries. Nat. Geosci. 12 , 450–455 (2019).
Gagan, M. K. et al. New views of tropical paleoclimates from corals. Quat. Sci. Rev. 19 , 45–64 (2000).
Thompson, D. M., Ault, T. R., Evans, M. N., Cole, J. E. & Emile-Geay, J. Comparison of observed and simulated tropical climate trends using a forward model of coral δ 18 O. Geophys. Res. Lett. 38 , L14706 (2011).
LeGrande, A. N. & Schmidt, G. A. Global gridded data set of the oxygen isotopic composition in seawater. Geophys. Res. Lett. 33 , L12604 (2006).
Reed, E. V., Thompson, D. M. & Anchukaitis, K. J. Coral-based sea surface salinity reconstructions and the role of observational uncertainties in inferred variability and trends. Paleoceanogr. Paleoclimatol. 37 , e2021PA004371 (2022).
Khaliq, M. N., Ouarda, T. B. M. J., Gachon, P., Sushama, L. & St-Hilaire, A. Identification of hydrological trends in the presence of serial and cross correlations: A review of selected methods and their application to annual flow regimes of Canadian rivers. J. Hydrol. 368 , 117–130 (2009).
Mahlstein, I., Hegerl, G. & Solomon, S. Emerging local warming signals in observational data. Geophys. Res. Lett. 39 , L21711 (2012).
Freeman, E. et al. ICOADS Release 3.0: a major update to the historical marine climate record. Int. J. Climatol. 37 , 2211–2232 (2017).
Huang, B. et al. Uncertainty estimates for sea surface temperature and land surface air temperature in NOAAGlobalTemp version 5. J. Clim. 33 , 1351–1379 (2020).
Druffel, E. R. M. & Griffin, S. Variability of surface ocean radiocarbon and stable isotopes in the southwestern Pacific. J. Geophys. Res. 104 , 23607–23613 (1999).
DeLong, K. L., Quinn, T. M., Taylor, F. W., Lin, K. & Shen, C.-C. Sea surface temperature variability in the southwest tropical Pacific since AD 1649. Nat. Clim. Change 2 , 799–804 (2012).
Quinn, T. et al. A multicentury stable isotope record from a New Caledonia coral: Interannual and decadal SST variability in the southwest Pacific since 1657. Paleoceanography 13 , 412–426 (1998).
Quinn, T. M., Crowley, T. J. & Taylor, F. W. New stable isotope results from a 173-year coral from Espiritu Santo, Vanuatu. Geophys. Res. Lett. 23 , 3413–3416 (1996).
Alibert, C. & Kinsley, L. A 170-year Sr/Ca and Ba/Ca coral record from the western Pacific warm pool: 1. What can we learn from an unusual coral record? J. Geophys. Res. Oceans 113 , C04008 (2008).
Tudhope, A. W. et al. Variability in the El Niño-Southern Oscillation through a glacial-interglacial cycle. Science 291 , 1511–1517 (2001).
Urban, F. E., Cole, J. E. & Overpeck, J. T. Influence of mean climate change on climate variability from a 155-year tropical Pacific coral record. Nature 407 , 989–993 (2000).
Guilderson, T. P. & Schrag, D. P. Reliability of coral isotope records from the western Pacific warm pool: A comparison using age-optimized records. Paleoceanography 14 , 457–464 (1999).
Quinn, T. M., Taylor, F. W. & Crowley, T. J. Coral-based climate variability in the Western Pacific Warm Pool since 1867. J. Geophys. Res. 111 , C11006 (2006).
Gorman, M. K. et al. A coral-based reconstruction of sea surface salinity at Sabine Bank, Vanuatu from 1842 to 2007 CE. Paleoceanography 27 , PA3226 (2012).
Bagnato, S., Linsley, B. K., Howe, S. S., Wellington, G. M. & Salinger, J. Coral oxygen isotope records of interdecadal climate variations in the South Pacific Convergence Zone region. Geochem. Geophys. Geosyst. 6 , Q06001 (2005).
Linsley, B. K. et al. Tracking the extent of the South Pacific Convergence Zone since the early 1600s. Geochem. Geophys. Geosyst. 7 , Q05003 (2006).
Cole, J. E., Fairbanks, R. G. & Shen, G. T. Recent variability in the Southern Oscillation: Isotopic results from a Tarawa Atoll coral. Science 260 , 1790–1793 (1993).
Dassié, E. P. et al. A Fiji multi-coral δ 18 O composite approach to obtaining a more accurate reconstruction of the last two-centuries of the ocean-climate variability in the South Pacific Convergence Zone region. Paleoceanography 29 , 1196–1213 (2014).
Carton, J. A., Chepurin, G. A. & Chen, L. SODA3: A new ocean climate reanalysis. J. Clim. 31 , 6967–6983 (2018).
Zuo, H., Balmaseda, M. A., Tietsche, S., Mogensen, K. & Mayer, M. The ECMWF operational ensemble reanalysis-analysis system for ocean and sea ice: A description of the system and assessment. Ocean Sci. 15 , 779–808 (2019).
Cheng, L. et al. Improved estimates of changes in upper ocean salinity and the hydrological cycle. J. Clim. 33 , 10357–10381 (2020).
Thompson, D. M. et al. Identifying hydro‐sensitive coral δ18O records for improved high‐resolution temperature and salinity reconstructions. Geophys. Res. Lett. 49 , e2021GL096153 (2022).
Wu, Y., Fallon, S. J., Cantin, N. E. & Lough, J. M. Assessing multiproxy approaches (Sr/Ca, U/Ca, Li/Mg, and B/Mg) to reconstruct sea surface temperature from coral skeletons throughout the Great Barrier Reef. Sci. Total Environ. 786 , 147393 (2021).
Sadler, J., Webb, G. E., Leonard, N. D., Nothdurft, L. D. & Clark, T. R. Reef core insights into mid-Holocene water temperatures of the southern Great Barrier Reef. Paleoceanography 31 , 1395–1408 (2016).
Roche, R. C. et al. Mid-Holocene sea surface conditions and riverine influence on the inshore Great Barrier Reef. Holocene 24 , 885–897 (2014).
Reed, E. V., Cole, J. E., Lough, J. M., Thompson, D. & Cantin, N. E. Linking climate variability and growth in coral skeletal records from the Great Barrier Reef. Coral Reefs 38 , 29–43 (2019).
Razak, T. B. et al. Use of skeletal Sr/Ca ratios to determine growth patterns in a branching coral Isopora palifera. Mar. Biol. 164 , 96 (2017).
Marshall, J. F. Decadal-scale, High Resolution Records of Sea Surface Temperature in the Eastern Indian and South Western Pacific Oceans from Proxy Records of the Strontium/calcium Ratio of Massive Porites Corals PhD thesis, Australian National Univ. (2000).
Marshall, J. F. & McCulloch, M. T. An assessment of the Sr/Ca ratio in shallow water hermatypic corals as a proxy for sea surface temperature. Geochim. Cosmochim. Acta 66 , 3263–3280 (2002).
Gagan, M. K. et al. Coral oxygen isotope evidence for recent groundwater fluxes to the Australian Great Barrier Reef. Geophys. Res. Lett. 29 , 43-1–43-4 (2002).
D’Olivo, J. P., Sinclair, D. J., Rankenburg, K. & McCulloch, M. T. A universal multi-trace element calibration for reconstructing sea surface temperatures from long-lived Porites corals: Removing ‘vital-effects’. Geochim. Cosmochim. Acta 239 , 109–135 (2018).
Fallon, S. J., McCulloch, M. T. & Alibert, C. Examining water temperature proxies in Porites corals from the Great Barrier Reef: a cross-shelf comparison. Coral Reefs 22 , 389–404 (2003).
Brenner, L. D., Linsley, B. K. & Potts, D. C. A modern Sr/Ca-δ 18 O-sea surface temperature calibration for Isopora corals on the Great Barrier Reef. Paleoceanography 32 , 182–194 (2017).
Alibert, C. et al. Source of trace element variability in Great Barrier Reef corals affected by the Burdekin flood plumes. Geochim. Cosmochim. Acta 67 , 231–246 (2003).
Murty, S. A. et al. Spatial and temporal robustness of Sr/Ca-SST calibrations in Red Sea corals: Evidence for influence of mean annual temperature on calibration slopes. Paleoceanogr. Paleoclimatol. 33 , 443–456 (2018).
Sayani, H. R., Cobb, K. M., DeLong, K., Hitt, N. T. & Druffel, E. R. M. Intercolony δ 18 O and Sr/Ca variability among Porites spp. corals at Palmyra Atoll: Toward more robust coral-based estimates of climate. Geochem. Geophys. Geosyst. 20 , 5270–5284 (2019).
Otto, F. E. L. Geert Jan van Oldenborgh 1961–2021. Nat. Clim. Chang. 11 , 1017 (2021).
Download references
Acknowledgements
We acknowledge the originators of the coral data cited in Supplementary Tables 1 and 2 ; S. E. Perkins-Kirkpatrick and the deceased G. J. van Oldenborgh 105 for contributions to an earlier version of this manuscript; E. P. Dassié and J. Zinke for discussions and data; R. Neukom for advice on an earlier version of the reconstruction code; and B. Trewin and K. Braganza for advice about the Bureau of Meteorology GBR SST time series. B.J.H. and H.V.M. acknowledge support from an Australian Research Council (ARC) SRIEAS grant, Securing Antarctica’s Environmental Future (SR200100005), and ARC Discovery Project DP200100206. A.D.K. acknowledges support from an ARC DECRA (DE180100638) and the Australian government’s National Environmental Science Program. B.J.H. and A.D.K. acknowledge an affiliation with the ARC Centre of Excellence for Climate Extremes (CE170100023). H.V.M. acknowledges support from an ARC Future Fellowship (FT140100286). A.K.A. acknowledges support from an Australian government research training program scholarship and an AINSE postgraduate research award. Funding was provided to B.K.L. by the Vetlesen Foundation through a gift to the Lamont-Doherty Earth Observatory. Grants to B.K.L. enabled the generation of coral oxygen isotope and Sr/Ca data from Fiji that were used in our reconstruction (US National Science Foundation OCE-0318296 and ATM-9901649 and US National Oceanic and Atmospheric Administration NA96GP0406). We acknowledge the support of the NCI facility in Australia and the World Climate Research Programme’s working group on coupled modelling, which is responsible for CMIP. We thank the climate-modelling groups for producing and making available their model output. For CMIP, the US Department of Energy’s Program for Climate Model Diagnosis and Intercomparison provided coordinating support and led the development of software infrastructure in partnership with the Global Organisation for Earth System Science Portals.
Author information
Authors and affiliations.
Environmental Futures, School of Earth, Atmospheric and Life Sciences, University of Wollongong, Wollongong, New South Wales, Australia
Benjamin J. Henley, Helen V. McGregor & Ariella K. Arzey
Securing Antarctica’s Environmental Future, University of Wollongong, Wollongong, New South Wales, Australia
School of Agriculture, Food and Ecosystem Sciences, University of Melbourne, Parkville, Victoria, Australia
Benjamin J. Henley
School of Geography, Earth and Atmospheric Sciences, University of Melbourne, Parkville, Victoria, Australia
Andrew D. King & David J. Karoly
ARC Centre of Excellence for Climate Extremes, University of Melbourne, Parkville, Victoria, Australia
Andrew D. King
School of the Environment, The University of Queensland, Brisbane, Queensland, Australia
Ove Hoegh-Guldberg
Australian Institute of Marine Science, Townsville, Queensland, Australia
Janice M. Lough
ARC Centre of Excellence for Coral Reef Studies and School of Earth Sciences, University of Western Australia, Crawley, Western Australia, Australia
Thomas M. DeCarlo
Department of Earth and Environmental Sciences, Tulane University, New Orleans, LA, USA
Lamont-Doherty Earth Observatory of Columbia University, Palisades, NY, USA
Braddock K. Linsley
You can also search for this author in PubMed Google Scholar
Contributions
B.J.H., H.V.M. and A.D.K. conceived the study and developed the methodology. B.J.H. did most of the analysis. A.K.A. contributed analysis of modern coral data (Supplementary Information section 4.2 ). T.M.D. contributed analysis of instrumental data coverage (Supplementary Information section 1.2 ). B.K.L. contributed sub-annual coral data. B.J.H. and H.V.M. led the preparation of the manuscript, with contributions from A.D.K., O.H.-G., A.K.A., D.J.K., J.M.L., T.M.D. and B.K.L. Generative artificial intelligence was not used in any aspect of this study or manuscript.
Corresponding author
Correspondence to Benjamin J. Henley .
Ethics declarations
Competing interests.
The authors declare no competing interests.
Peer review
Peer review information.
Nature thanks Simon Michel, Miriam Pfeiffer, Claudia Tebaldi and the other, anonymous, reviewer(s) for their contribution to the peer review of this work.
Additional information
Publisher’s note Springer Nature remains neutral with regard to jurisdictional claims in published maps and institutional affiliations.
Supplementary information
Supplementary information ., rights and permissions.
Open Access This article is licensed under a Creative Commons Attribution 4.0 International License, which permits use, sharing, adaptation, distribution and reproduction in any medium or format, as long as you give appropriate credit to the original author(s) and the source, provide a link to the Creative Commons licence, and indicate if changes were made. The images or other third party material in this article are included in the article’s Creative Commons licence, unless indicated otherwise in a credit line to the material. If material is not included in the article’s Creative Commons licence and your intended use is not permitted by statutory regulation or exceeds the permitted use, you will need to obtain permission directly from the copyright holder. To view a copy of this licence, visit http://creativecommons.org/licenses/by/4.0/ .
Reprints and permissions
About this article
Cite this article.
Henley, B.J., McGregor, H.V., King, A.D. et al. Highest ocean heat in four centuries places Great Barrier Reef in danger. Nature 632 , 320–326 (2024). https://doi.org/10.1038/s41586-024-07672-x
Download citation
Received : 02 November 2022
Accepted : 04 June 2024
Published : 07 August 2024
Issue Date : 08 August 2024
DOI : https://doi.org/10.1038/s41586-024-07672-x
Share this article
Anyone you share the following link with will be able to read this content:
Sorry, a shareable link is not currently available for this article.
Provided by the Springer Nature SharedIt content-sharing initiative
This article is cited by
Great barrier reefâs temperature soars to 400-year high.
- Jeff Tollefson
Nature (2024)
By submitting a comment you agree to abide by our Terms and Community Guidelines . If you find something abusive or that does not comply with our terms or guidelines please flag it as inappropriate.
Quick links
- Explore articles by subject
- Guide to authors
- Editorial policies
Sign up for the Nature Briefing: Anthropocene newsletter — what matters in anthropocene research, free to your inbox weekly.

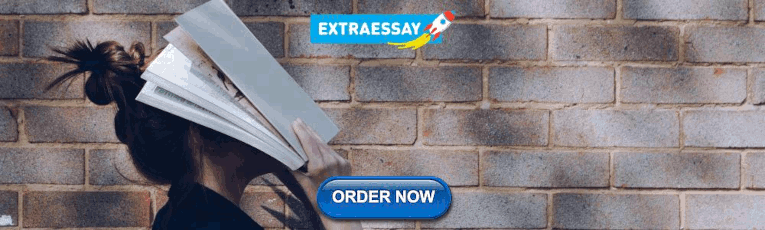
IMAGES
COMMENTS
The genetic code is nearly universal, and the arrangement of the codons in the standard codon table is highly non-random. ... The writing of this review coincides with the 40 th anniversary of Crick's seminal paper on the evolution of the genetic code that synthesized the preceding research in this area and presciently outlined the principal ...
Genomic research has evolved from seeking to understand the fundamentals of the human genetic code to examining the ways in which this code varies among people, and then applying this knowledge to ...
The paper appeared in Nature at the end of December 1961, signed by Crick, Brenner, Barnett and Watts-Tobin. As the title of the article indicated, unlike the work by Nirenberg, Ochoa and others, the results from the Cambridge group were solely focused on the general nature of the genetic code. They said nothing about its specific nature ...
The standard extant genetic code includes a number of minor organismal differences, particularly in eukaryotic organelles as well as in parasitic and symbiotic prokaryotes with small genomes. ... This is currently an active field of research, and new advances are continuously being made. ... This is a hot topic of investigation as ∼100 papers ...
Landmark Designation. The American Chemical Society designated the deciphering of the genetic code by Marshall Nirenberg as a National Historic Chemical Landmark at the National Institutes of Health in Bethesda, Maryland, on November 12, 2009. The plaque commemorating the research reads:
However, a new wave of research is building on genetic code expansion to drive strategies for incorporating multiple distinct ncAAs into proteins and the wholesale reprogramming of the genetic ...
Nirenberg took a novel yet simple approach to cracking the problem. Published papers had predicted it would take 20-30 years to crack the code using genetic methods. Nirenberg's insight was to use biochemistry instead. "It was incredibly simple," says C. Thomas Caskey, now at Baylor College of Medicine in Houston, Texas, who began ...
The elucidation of the genetic code was one of the greatest discoveries of the 20th century. The genetic code is an algorithm that connects 64 RNA triplets to 20 amino acids, and functions as the Rosetta stone of molecular biology. Figure 1. Sir Francis Crick, La Jolla 1982, Photograph by Norman Seeff.
2.1. Incorporation of NCAAs into Specific Sites. Currently, three major approaches are used to engineer the genetic code in a site-specific manner: (1) amber codon suppression; (2) rare sense codon reassignment; and (3) quadruplet codon. Figure 1 provides a schematic illustration of each method.
In 2021, Chin's team hacked the genetic code to synthesize short polymers, and even an artificial circular structure called a macrocycle, in E. coli 9. Now, Chin hopes to push this technology ...
Strategies developed for genetic code expansion in eukaryotic cells have been extended to whole animals and plants 5, and recently genetic code expansion was reported in the mouse 20,21, with ...
The genetic code is the set of rules that all living cells use for the synthesis of proteins, and to this purpose they employ three different types of RNAs: the messenger-RNAs, the transfer-RNAs and the ribosomal-RNAs. The messenger-RNAs carry genetic information in the form of sequences of codons, where the codons are groups of three nucleotides.
The genetic code of Escherichia coli was engineered to abolish the use of two sense codons (UCA and UCG) and a nonsense codon (UAG). This freed these codons up for incorporating up to three different noncanonical amino acids (ncAAs) at the same time when these codons appear in messenger RNA. There is a high degree of redundancy within the ...
By Christie Moffatt Fifty years ago, on January 18, 1965, Dr. Marshall W. Nirenberg (1927-2010) completed his first summary of the genetic code—one of the most significant documents in the history of twentieth-century science—a painstaking, handwritten chart of the discovery of how sequences of DNA, known as "triplets," direct the assembly of amino acids into…
Special Issue on Code Biology in BioSystems The evolution of the genetic code: impasses and challenges Ádám Kun1,2,3 & Ádám Radványi4 1 Parmenides Center for the Conceptual Foundations of Science, Munich/Pullach, Germany. 2 MTA-ELTE Theoretical Biology and Evolutionary Ecology Research Group, Budapest, Hungary. 3 Evolutionary Systems Research Group, Centre for Ecological Research, Tihany ...
I suspect their conclusions had a great impact on many scientists engaged in research in which the general nature of the genetic code was of concern. The 1961 paper by Crick et al. is an outstanding example of the use of thought and logic to solve basic biological problems. In my opinion, it is a superb paper to assign to students in courses ...
The Journal publishes papers by leading scientists from all over the world that report on advances in the understanding of proteins in the broadest sense. ... Expansion of the genetic code of a living organism requires adding new components to the translational machinery which have exquisite bioorthogonality to the endogenous components (do not ...
Explore the latest full-text research PDFs, articles, conference papers, preprints and more on GENETIC CODE. Find methods information, sources, references or conduct a literature review on GENETIC ...
The universal triple-nucleotide genetic code, allowing DNA-encoded mRNA to be translated into the amino acid sequences of proteins using transfer RNAs (tRNAs) and many accessory and modification factors, is essentially common to all living organisms on Earth (1 - 3).Thousands of studies have focused on various aspects of the genetic code, revealing aspects of the basis for its structure and ...
Abstract This article reconsiders the theoretical role of the genetic code. By drawing on published and unpublished sources from the 1950s, I analyse how the code metaphor was actually employed by the scientists who first promoted its use. The analysis shows that the term 'code' picked out mechanism sketches, consisting of more or less detailed descriptions of ordinary molecular components ...
View a PDF of the paper titled The "Wow! signal" of the terrestrial genetic code, by Vladimir I. shCherbak and Maxim A. Makukov. It has been repeatedly proposed to expand the scope for SETI, and one of the suggested alternatives to radio is the biological media. Genomic DNA is already used on Earth to store non-biological information.
The genetic code. The genetic code. The genetic code Sci Am. 1962 Oct;207:66-74. doi: 10.1038/scientificamerican1062-66. Author F H CRICK. PMID: 13882204 DOI: 10.1038/scientificamerican1062-66 No abstract available. MeSH terms Genetic Code* Genetics* ...
The aim of this Research Topic is to bring together researchers from different disciplines, astrophysics, biochemistry, biophysics, mathematics, , molecular modeling and simulation etc. in a collection dedicated to the mathematical study of the genetic code and everything related to it. The great challenge in the study of the genetic code ...
Cracking the code of life: new AI model learns DNA's hidden language. ScienceDaily . Retrieved August 9, 2024 from www.sciencedaily.com / releases / 2024 / 08 / 240805134159.htm
That's a problem, because genetic variants in regulatory DNA have important effects on human health. A paper published in Science in October of 2022 shows how these regulatory variants can affect our risk for disease, and why the regulatory code is so hard to figure out. With an impressively thorough set of experiments, the paper's authors ...
Research in La Jolla, California. ... to create phage-resistant bacteria in a paper ... code to create polymers. In 2021, Chin's team hacked the genetic code to synthesize short polymers, and ...
Research paper Genetic algorithm-based optimization of combined supercritical CO 2 power and flash-tank enhanced transcritical CO 2 refrigeration cycle for shipboard waste heat recuperation Author links open overlay panel Fairooz Nanzeeba , Tajwar A. Baigh , Afrida Kabir , Yasin Khan , M. Monjurul Ehsan
Oil palm is a versatile oil crop with numerous applications. Significant progress has been made in applying histological techniques in oil palm research in recent years. Whole genome sequencing of oil palm has been carried out to explain the function and structure of the order genome, facilitating the development of molecular markers and the construction of genetic maps, which are crucial for ...
Implementing Retrieval-Augmented Generation (RAG) systems is inherently complex, requiring deep understanding of data, use cases, and intricate design decisions. Additionally, evaluating these systems presents significant challenges, necessitating assessment of both retrieval accuracy and generative quality through a multi-faceted approach. We introduce RAG Foundry, an open-source framework ...
Most rapid changes depend on a history of exposure to key genetic types ... The code that supports the findings of ... B.J.H. and H.V.M. acknowledge support from an Australian Research Council ...