What Is Problem Solving? How Software Engineers Approach Complex Challenges
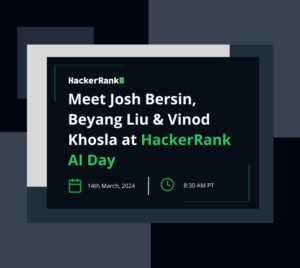
From debugging an existing system to designing an entirely new software application, a day in the life of a software engineer is filled with various challenges and complexities. The one skill that glues these disparate tasks together and makes them manageable? Problem solving .
Throughout this blog post, we’ll explore why problem-solving skills are so critical for software engineers, delve into the techniques they use to address complex challenges, and discuss how hiring managers can identify these skills during the hiring process.
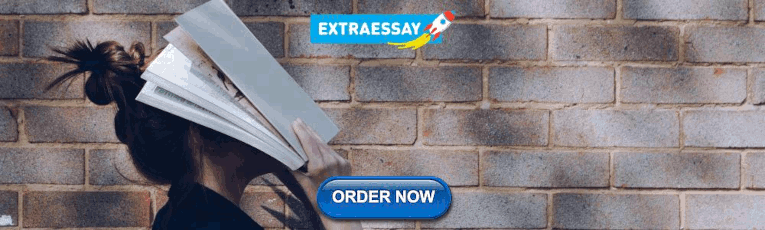
What Is Problem Solving?
But what exactly is problem solving in the context of software engineering? How does it work, and why is it so important?
Problem solving, in the simplest terms, is the process of identifying a problem, analyzing it, and finding the most effective solution to overcome it. For software engineers, this process is deeply embedded in their daily workflow. It could be something as simple as figuring out why a piece of code isn’t working as expected, or something as complex as designing the architecture for a new software system.
In a world where technology is evolving at a blistering pace, the complexity and volume of problems that software engineers face are also growing. As such, the ability to tackle these issues head-on and find innovative solutions is not only a handy skill — it’s a necessity.
The Importance of Problem-Solving Skills for Software Engineers
Problem-solving isn’t just another ability that software engineers pull out of their toolkits when they encounter a bug or a system failure. It’s a constant, ongoing process that’s intrinsic to every aspect of their work. Let’s break down why this skill is so critical.
Driving Development Forward
Without problem solving, software development would hit a standstill. Every new feature, every optimization, and every bug fix is a problem that needs solving. Whether it’s a performance issue that needs diagnosing or a user interface that needs improving, the capacity to tackle and solve these problems is what keeps the wheels of development turning.
It’s estimated that 60% of software development lifecycle costs are related to maintenance tasks, including debugging and problem solving. This highlights how pivotal this skill is to the everyday functioning and advancement of software systems.
Innovation and Optimization
The importance of problem solving isn’t confined to reactive scenarios; it also plays a major role in proactive, innovative initiatives . Software engineers often need to think outside the box to come up with creative solutions, whether it’s optimizing an algorithm to run faster or designing a new feature to meet customer needs. These are all forms of problem solving.
Consider the development of the modern smartphone. It wasn’t born out of a pre-existing issue but was a solution to a problem people didn’t realize they had — a device that combined communication, entertainment, and productivity into one handheld tool.
Increasing Efficiency and Productivity
Good problem-solving skills can save a lot of time and resources. Effective problem-solvers are adept at dissecting an issue to understand its root cause, thus reducing the time spent on trial and error. This efficiency means projects move faster, releases happen sooner, and businesses stay ahead of their competition.
Improving Software Quality
Problem solving also plays a significant role in enhancing the quality of the end product. By tackling the root causes of bugs and system failures, software engineers can deliver reliable, high-performing software. This is critical because, according to the Consortium for Information and Software Quality, poor quality software in the U.S. in 2022 cost at least $2.41 trillion in operational issues, wasted developer time, and other related problems.
Problem-Solving Techniques in Software Engineering
So how do software engineers go about tackling these complex challenges? Let’s explore some of the key problem-solving techniques, theories, and processes they commonly use.
Decomposition
Breaking down a problem into smaller, manageable parts is one of the first steps in the problem-solving process. It’s like dealing with a complicated puzzle. You don’t try to solve it all at once. Instead, you separate the pieces, group them based on similarities, and then start working on the smaller sets. This method allows software engineers to handle complex issues without being overwhelmed and makes it easier to identify where things might be going wrong.
Abstraction
In the realm of software engineering, abstraction means focusing on the necessary information only and ignoring irrelevant details. It is a way of simplifying complex systems to make them easier to understand and manage. For instance, a software engineer might ignore the details of how a database works to focus on the information it holds and how to retrieve or modify that information.
Algorithmic Thinking
At its core, software engineering is about creating algorithms — step-by-step procedures to solve a problem or accomplish a goal. Algorithmic thinking involves conceiving and expressing these procedures clearly and accurately and viewing every problem through an algorithmic lens. A well-designed algorithm not only solves the problem at hand but also does so efficiently, saving computational resources.
Parallel Thinking
Parallel thinking is a structured process where team members think in the same direction at the same time, allowing for more organized discussion and collaboration. It’s an approach popularized by Edward de Bono with the “ Six Thinking Hats ” technique, where each “hat” represents a different style of thinking.
In the context of software engineering, parallel thinking can be highly effective for problem solving. For instance, when dealing with a complex issue, the team can use the “White Hat” to focus solely on the data and facts about the problem, then the “Black Hat” to consider potential problems with a proposed solution, and so on. This structured approach can lead to more comprehensive analysis and more effective solutions, and it ensures that everyone’s perspectives are considered.
This is the process of identifying and fixing errors in code . Debugging involves carefully reviewing the code, reproducing and analyzing the error, and then making necessary modifications to rectify the problem. It’s a key part of maintaining and improving software quality.
Testing and Validation
Testing is an essential part of problem solving in software engineering. Engineers use a variety of tests to verify that their code works as expected and to uncover any potential issues. These range from unit tests that check individual components of the code to integration tests that ensure the pieces work well together. Validation, on the other hand, ensures that the solution not only works but also fulfills the intended requirements and objectives.
Explore verified tech roles & skills.
The definitive directory of tech roles, backed by machine learning and skills intelligence.
Explore all roles
Evaluating Problem-Solving Skills
We’ve examined the importance of problem-solving in the work of a software engineer and explored various techniques software engineers employ to approach complex challenges. Now, let’s delve into how hiring teams can identify and evaluate problem-solving skills during the hiring process.
Recognizing Problem-Solving Skills in Candidates
How can you tell if a candidate is a good problem solver? Look for these indicators:
- Previous Experience: A history of dealing with complex, challenging projects is often a good sign. Ask the candidate to discuss a difficult problem they faced in a previous role and how they solved it.
- Problem-Solving Questions: During interviews, pose hypothetical scenarios or present real problems your company has faced. Ask candidates to explain how they would tackle these issues. You’re not just looking for a correct solution but the thought process that led them there.
- Technical Tests: Coding challenges and other technical tests can provide insight into a candidate’s problem-solving abilities. Consider leveraging a platform for assessing these skills in a realistic, job-related context.
Assessing Problem-Solving Skills
Once you’ve identified potential problem solvers, here are a few ways you can assess their skills:
- Solution Effectiveness: Did the candidate solve the problem? How efficient and effective is their solution?
- Approach and Process: Go beyond whether or not they solved the problem and examine how they arrived at their solution. Did they break the problem down into manageable parts? Did they consider different perspectives and possibilities?
- Communication: A good problem solver can explain their thought process clearly. Can the candidate effectively communicate how they arrived at their solution and why they chose it?
- Adaptability: Problem-solving often involves a degree of trial and error. How does the candidate handle roadblocks? Do they adapt their approach based on new information or feedback?
Hiring managers play a crucial role in identifying and fostering problem-solving skills within their teams. By focusing on these abilities during the hiring process, companies can build teams that are more capable, innovative, and resilient.
Key Takeaways
As you can see, problem solving plays a pivotal role in software engineering. Far from being an occasional requirement, it is the lifeblood that drives development forward, catalyzes innovation, and delivers of quality software.
By leveraging problem-solving techniques, software engineers employ a powerful suite of strategies to overcome complex challenges. But mastering these techniques isn’t simple feat. It requires a learning mindset, regular practice, collaboration, reflective thinking, resilience, and a commitment to staying updated with industry trends.
For hiring managers and team leads, recognizing these skills and fostering a culture that values and nurtures problem solving is key. It’s this emphasis on problem solving that can differentiate an average team from a high-performing one and an ordinary product from an industry-leading one.
At the end of the day, software engineering is fundamentally about solving problems — problems that matter to businesses, to users, and to the wider society. And it’s the proficient problem solvers who stand at the forefront of this dynamic field, turning challenges into opportunities, and ideas into reality.
This article was written with the help of AI. Can you tell which parts?
Get started with HackerRank
Over 2,500 companies and 40% of developers worldwide use HackerRank to hire tech talent and sharpen their skills.
Recommended topics
- Hire Developers
- Problem Solving
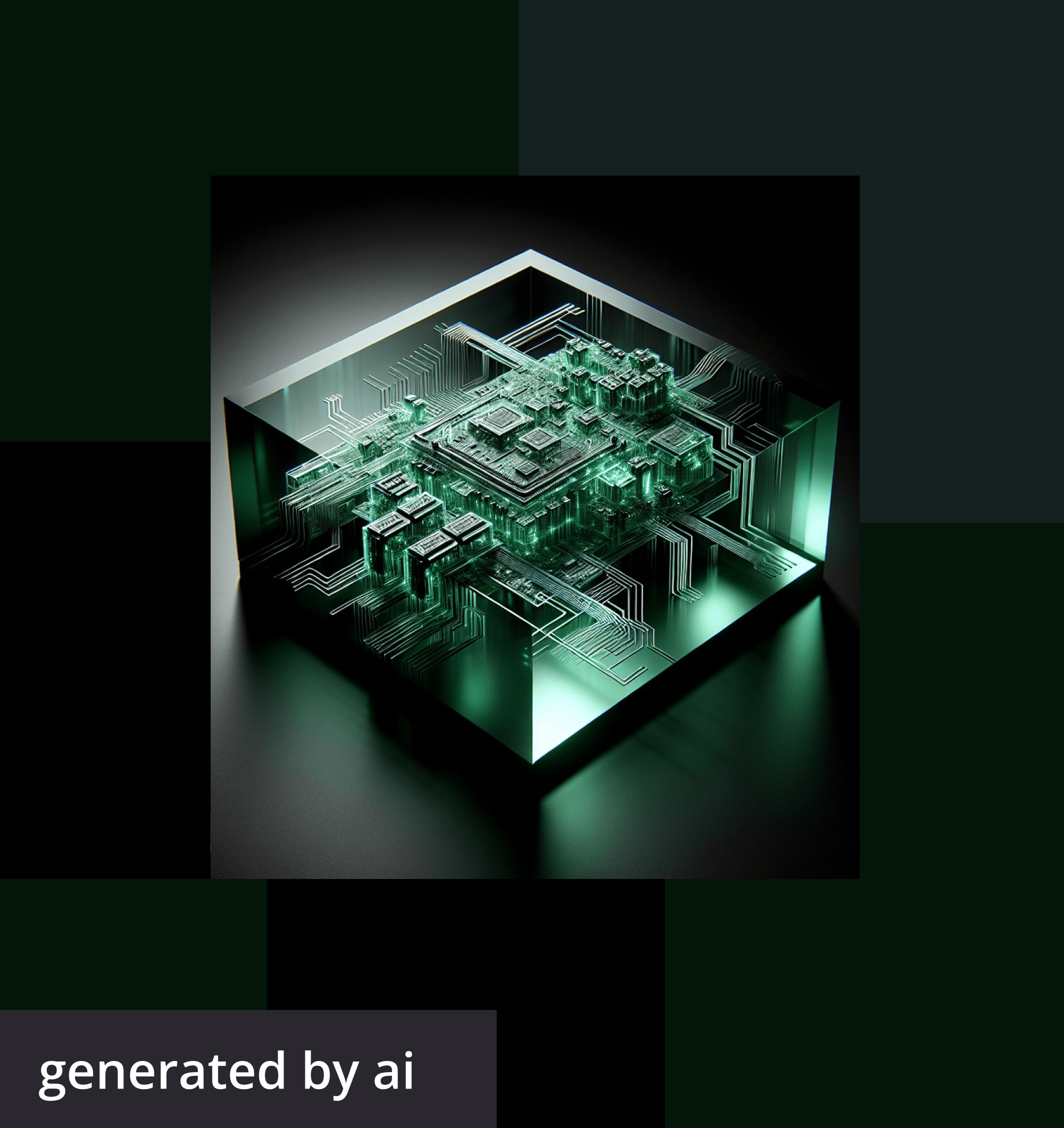
What Factors Actually Impact a Developer’s Decision to Accept an Offer?
- Admiral “Amazing Grace” Hopper
Exploring the Intricacies of NP-Completeness in Computer Science
Understanding p vs np problems in computer science: a primer for beginners, understanding key theoretical frameworks in computer science: a beginner’s guide.

Learn Computer Science with Python
CS is a journey, not a destination
- Foundations
Understanding Algorithms: The Key to Problem-Solving Mastery

The world of computer science is a fascinating realm, where intricate concepts and technologies continuously shape the way we interact with machines. Among the vast array of ideas and principles, few are as fundamental and essential as algorithms. These powerful tools serve as the building blocks of computation, enabling computers to solve problems, make decisions, and process vast amounts of data efficiently.
An algorithm can be thought of as a step-by-step procedure or a set of instructions designed to solve a specific problem or accomplish a particular task. It represents a systematic approach to finding solutions and provides a structured way to tackle complex computational challenges. Algorithms are at the heart of various applications, from simple calculations to sophisticated machine learning models and complex data analysis.
Understanding algorithms and their inner workings is crucial for anyone interested in computer science. They serve as the backbone of software development, powering the creation of innovative applications across numerous domains. By comprehending the concept of algorithms, aspiring computer science enthusiasts gain a powerful toolset to approach problem-solving and gain insight into the efficiency and performance of different computational methods.
In this article, we aim to provide a clear and accessible introduction to algorithms, focusing on their importance in problem-solving and exploring common types such as searching, sorting, and recursion. By delving into these topics, readers will gain a solid foundation in algorithmic thinking and discover the underlying principles that drive the functioning of modern computing systems. Whether you’re a beginner in the world of computer science or seeking to deepen your understanding, this article will equip you with the knowledge to navigate the fascinating world of algorithms.
What are Algorithms?
At its core, an algorithm is a systematic, step-by-step procedure or set of rules designed to solve a problem or perform a specific task. It provides clear instructions that, when followed meticulously, lead to the desired outcome.
Consider an algorithm to be akin to a recipe for your favorite dish. When you decide to cook, the recipe is your go-to guide. It lists out the ingredients you need, their exact quantities, and a detailed, step-by-step explanation of the process, from how to prepare the ingredients to how to mix them, and finally, the cooking process. It even provides an order for adding the ingredients and specific times for cooking to ensure the dish turns out perfect.
In the same vein, an algorithm, within the realm of computer science, provides an explicit series of instructions to accomplish a goal. This could be a simple goal like sorting a list of numbers in ascending order, a more complex task such as searching for a specific data point in a massive dataset, or even a highly complicated task like determining the shortest path between two points on a map (think Google Maps). No matter the complexity of the problem at hand, there’s always an algorithm working tirelessly behind the scenes to solve it.
Furthermore, algorithms aren’t limited to specific programming languages. They are universal and can be implemented in any language. This is why understanding the fundamental concept of algorithms can empower you to solve problems across various programming languages.
The Importance of Algorithms
Algorithms are indisputably the backbone of all computational operations. They’re a fundamental part of the digital world that we interact with daily. When you search for something on the web, an algorithm is tirelessly working behind the scenes to sift through millions, possibly billions, of web pages to bring you the most relevant results. When you use a GPS to find the fastest route to a location, an algorithm is computing all possible paths, factoring in variables like traffic and road conditions, to provide you the optimal route.
Consider the world of social media, where algorithms curate personalized feeds based on our previous interactions, or in streaming platforms where they recommend shows and movies based on our viewing habits. Every click, every like, every search, and every interaction is processed by algorithms to serve you a seamless digital experience.
In the realm of computer science and beyond, everything revolves around problem-solving, and algorithms are our most reliable problem-solving tools. They provide a structured approach to problem-solving, breaking down complex problems into manageable steps and ensuring that every eventuality is accounted for.
Moreover, an algorithm’s efficiency is not just a matter of preference but a necessity. Given that computers have finite resources — time, memory, and computational power — the algorithms we use need to be optimized to make the best possible use of these resources. Efficient algorithms are the ones that can perform tasks more quickly, using less memory, and provide solutions to complex problems that might be infeasible with less efficient alternatives.
In the context of massive datasets (the likes of which are common in our data-driven world), the difference between a poorly designed algorithm and an efficient one could be the difference between a solution that takes years to compute and one that takes mere seconds. Therefore, understanding, designing, and implementing efficient algorithms is a critical skill for any computer scientist or software engineer.
Hence, as a computer science beginner, you are starting a journey where algorithms will be your best allies — universal keys capable of unlocking solutions to a myriad of problems, big or small.
Common Types of Algorithms: Searching and Sorting
Two of the most ubiquitous types of algorithms that beginners often encounter are searching and sorting algorithms.
Searching algorithms are designed to retrieve specific information from a data structure, like an array or a database. A simple example is the linear search, which works by checking each element in the array until it finds the one it’s looking for. Although easy to understand, this method isn’t efficient for large datasets, which is where more complex algorithms like binary search come in.
Binary search, on the other hand, is like looking up a word in the dictionary. Instead of checking each word from beginning to end, you open the dictionary in the middle and see if the word you’re looking for should be on the left or right side, thereby reducing the search space by half with each step.
Sorting algorithms, meanwhile, are designed to arrange elements in a particular order. A simple sorting algorithm is bubble sort, which works by repeatedly swapping adjacent elements if they’re in the wrong order. Again, while straightforward, it’s not efficient for larger datasets. More advanced sorting algorithms, such as quicksort or mergesort, have been designed to sort large data collections more efficiently.
Diving Deeper: Graph and Dynamic Programming Algorithms
Building upon our understanding of searching and sorting algorithms, let’s delve into two other families of algorithms often encountered in computer science: graph algorithms and dynamic programming algorithms.
A graph is a mathematical structure that models the relationship between pairs of objects. Graphs consist of vertices (or nodes) and edges (where each edge connects a pair of vertices). Graphs are commonly used to represent real-world systems such as social networks, web pages, biological networks, and more.
Graph algorithms are designed to solve problems centered around these structures. Some common graph algorithms include:
Dynamic programming is a powerful method used in optimization problems, where the main problem is broken down into simpler, overlapping subproblems. The solutions to these subproblems are stored and reused to build up the solution to the main problem, saving computational effort.
Here are two common dynamic programming problems:
Understanding these algorithm families — searching, sorting, graph, and dynamic programming algorithms — not only equips you with powerful tools to solve a variety of complex problems but also serves as a springboard to dive deeper into the rich ocean of algorithms and computer science.
Recursion: A Powerful Technique
While searching and sorting represent specific problem domains, recursion is a broad technique used in a wide range of algorithms. Recursion involves breaking down a problem into smaller, more manageable parts, and a function calling itself to solve these smaller parts.
To visualize recursion, consider the task of calculating factorial of a number. The factorial of a number n (denoted as n! ) is the product of all positive integers less than or equal to n . For instance, the factorial of 5 ( 5! ) is 5 x 4 x 3 x 2 x 1 = 120 . A recursive algorithm for finding factorial of n would involve multiplying n by the factorial of n-1 . The function keeps calling itself with a smaller value of n each time until it reaches a point where n is equal to 1, at which point it starts returning values back up the chain.
Algorithms are truly the heart of computer science, transforming raw data into valuable information and insight. Understanding their functionality and purpose is key to progressing in your computer science journey. As you continue your exploration, remember that each algorithm you encounter, no matter how complex it may seem, is simply a step-by-step procedure to solve a problem.
We’ve just scratched the surface of the fascinating world of algorithms. With time, patience, and practice, you will learn to create your own algorithms and start solving problems with confidence and efficiency.
Related Articles

Three Elegant Algorithms Every Computer Science Beginner Should Know
Problem Solving
Solving problems is the core of computer science. Programmers must first understand how a human solves a problem, then understand how to translate this "algorithm" into something a computer can do, and finally how to "write" the specific syntax (required by a computer) to get the job done. It is sometimes the case that a machine will solve a problem in a completely different way than a human.
Computer Programmers are problem solvers. In order to solve a problem on a computer you must:
Know how to represent the information (data) describing the problem.
Determine the steps to transform the information from one representation into another.
Information Representation
A computer, at heart, is really dumb. It can only really know about a few things... numbers, characters, booleans, and lists (called arrays) of these items. (See Data Types). Everything else must be "approximated" by combinations of these data types.
A good programmer will "encode" all the "facts" necessary to represent a problem in variables (See Variables). Further, there are "good ways" and "bad ways" to encode information. Good ways allow the computer to easily "compute" new information.
An algorithm (see Algorithm) is a set of specific steps to solve a problem. Think of it this way: if you were to tell your 3 year old neice to play your favorite song on the piano (assuming the neice has never played a piano), you would have to tell her where the piano was, and how to sit on the bench, and how to open the cover, and which keys to press, and which order to press them in, etc, etc, etc.
The core of what good programmers do is being able to define the steps necessary to accomplish a goal. Unfortunately, a computer, only knows a very restricted and limited set of possible steps. For example a computer can add two numbers. But if you want to find the average of two numbers, this is beyond the basic capabilities of a computer. To find the average, you must:
- First: Add the two numbers and save this result in a variable
- Then: Divide this new number the number two, and save this result in a variable.
- Finally: provide this number to the rest of the program (or print it for the user).
We "compute" all the time. Computing is the act of solving problems (or coming up with a plan to solve problems) in an organized manner. We don't need computers to "compute". We can use our own brain.
Encapsulation and Abstraction and Complexity Hiding
Computer scientists like to use the fancy word "Encapsulation" to show how smart we are. This is just a term for things we do as humans every day. It is combined with another fancy term: "Abstraction".
Abstraction is the idea of "ignoring the details". For example, a forest is really a vastly complex ecosystem containing trees, animals, water paths, etc, etc, etc. But to a computer scientist (and to a normal person), its just "a forest".
For example, if your professor needs a cup of coffee, and asks you the single item: "Get me a cup of coffee", he has used both encapsulation and abstraction. The number of steps required to actually get the coffee are enumerable. Including, getting up, walking down the hall, getting in your car, driving to a coffee stand, paying for the coffee, etc, etc, etc. Further, the idea of what a cup of coffee is, is abstract. Do you bring a mug of coffee, or a Styrofoam cup? Is it caffeinated or not? Is it freshly brewed or from concentrate? Does it come from Africa or America?
All of this information is TOO MUCH and we would quickly be unable to funciton if we had to remember all of these details. Thus we "abstract away" the details and only remember the few important items.
This brings us to the idea of "Complexity Hiding". Complexity hiding is the idea that most of the times details don't matter. In a computer program, as simple an idea as drawing a square on the screen involves hundreds (if not thousands) of (low level) computer instructions. Again, a person couldn't possible create interesting programs if every time they wanted to do something, they had to re-write (correctly) every one of those instructions. By "ecapsulating" what is meant by "draw square" and "reusing" this operation over and over again, we make programming tractable.
Encapsulation
The idea behind encapsulation is to store the information necessary to a particular idea in a set of variables associated with a single "object". We then create functions to manipulate this object, regardless of what the actual data is. From that point on, we treat the idea from a "high level" rather than worry about all the parts (data) and actions (functions) necessary to represent the object in a computer.
Brute Force
Brute force is a technique for solving problems that relies on a computers speed (how fast it can repeat steps) to solve a problem. For example, if you wanted to know how many times the number 8 goes into the number 100, you could do the following:
Of course this is a silly way for a computer (or a human) to solve this problem. The real way we would do it is:
When in doubt, you can often use "brute force" to solve a problem, but it often saves time (at least computer time) to think about the problem and solve it in an elegant manner.
Browse Course Material
Course info.
- Prof. John Guttag
Departments
- Electrical Engineering and Computer Science
As Taught In
- Computer Science
Introduction to Computer Science and Programming
Lecture 3: problem solving.
- Download video
- Download transcript

You are leaving MIT OpenCourseWare
CS2104: Introduction to Problem Solving in Computer Science
This course introduces the student to a broad range of heuristics for solving problems in a range of settings. Emphasis on problem-solving techniques that aid programmers and computer scientists. Heuristics for solving problems ''in the small'' (classical math and word problems), generating potential solutions to ''real-life'' problems encountered in the profession, and problem solving in teams.
Having successfully completed this course, the student will be able to:
- Identify skills and personality traits of successful problem solving.
- Apply standard problem-solving heuristics to aid in problem solving.
- Apply problem-solving techniques to programming activities.
- Apply problem-solving techniques to school and personal interactions.
- Apply pairs and team problem-solving techniques.
- Generate potential solutions to problems with standard heuristics.
- Formulate and successfully communicate the solutions to problems.
Prerequisites: MATH 1205 or MATH 1225 or MATH 1526.
Taught By: Alexey Onufriev Dwight Barnette Layne Watson Margaret Ellis Cliff Shaffer William McQuain

3 Types of Computer Science Skills Every CS Professional Needs
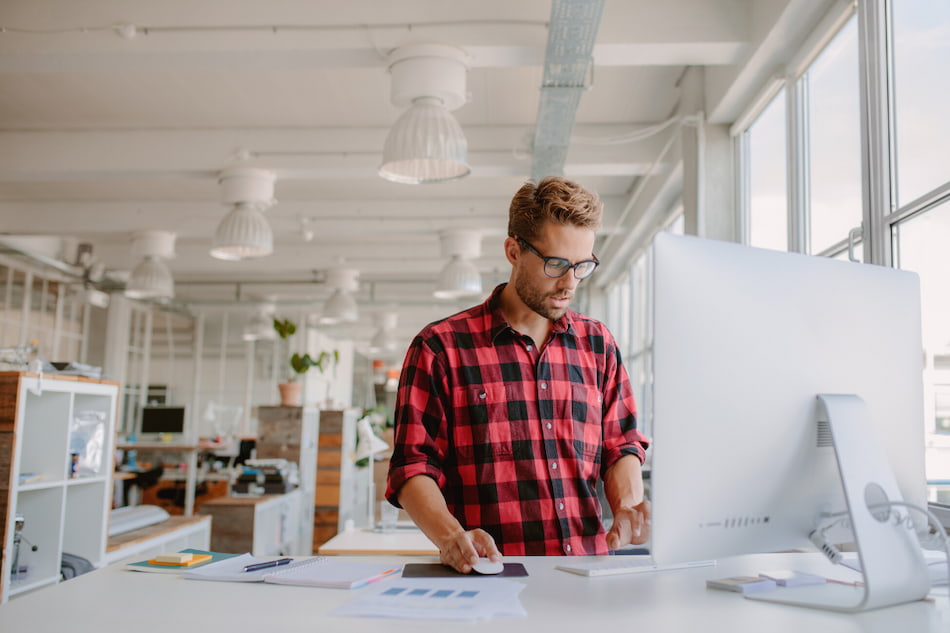
Industry Advice Computing and IT
Computer science is an increasingly popular field, and with good reason. Society has come to rely on the technology created by this industry to fulfill the functions of daily life, resulting in both exciting career opportunities and above-average salaries for individuals who choose to pursue a computer science career.
While the job outlook remains overwhelmingly positive , the evolution of the computer science field over the last few decades has created a demand for professionals with more than just basic coding skills. Now, professionals looking for success must have a strong combination of technical, interview, and soft skills unique to this specific sector.
Read on to explore what traits, skills, and subject matter fall into these three categories, and how a master’s degree from a university like Northeastern can help aspiring computer scientists hone them all.
Download Our Free Guide to Breaking into Computer Science
Whether you have a technical or non-technical background, here’s what you need to know.
DOWNLOAD NOW
Top Computer Science Skills to Learn
1. specific technical skills.
The most commonly acknowledged type of skills required among computer scientists are the practical abilities that allow them to develop software and digital tools. Some of the top skills in this category include:
- Programming: This incorporates deep knowledge of algorithms and data structures, and coding skills in languages like Java, C, Python, and JavaScript. Those hoping to improve their coding skills specifically should consider pursuing formal training in the form of a graduate degree or bootcamp .
- Software development: CS professionals should possess knowledge of software development and engineering principles, including the software development process from start to finish—writing programs using popular program languages, testing for usability, and ultimately implementing the programs.
- Mathematics : Alongside a comprehensive understanding of mathematical theory—which is equally as vital for aspiring CS professionals to obtain—knowledge of statistics, calculus, linear algebra, and other advanced mathematical techniques are mandatory for individuals in this field.
- Data Analysis: Basic data analysis skills are important for computer scientists, as data plays a key role in many advanced CS practices, including artificial intelligence (AI), predictive analysis , and more.
- Data Visualization: As crucial as it is to be able to analyze data effectively, it’s also essential that CS professionals can properly visualize data and results in a way that stakeholders can understand. This includes translating raw data into graphs, charts, and other visual tools to help communicate findings .
- Ethics: As technology continues to advance—especially with tools like artificial intelligence and machine learning—professionals in this field must develop an understanding of ethics and its impact on computer science.
The above list of skills includes only the baseline requirements for generic roles in the CS field. Those who wish to specialize in a certain niche area—such as AI , data science , cybersecurity , etc.—or plan to apply their computer science skills into a specific field will need to expand their knowledge in those areas, as well.
Honing Your Practical Skills With a Master’s Degree
One of the most efficient ways to gain these industry-specific skill sets is through the pursuit of a master’s degree in computer science from a top university like Northeastern. In a program like this, students participate in an array of courses designed to provide both the in-depth knowledge and hands-on abilities needed to thrive in this ever-changing industry.
“We really try to balance theory and practice [in our programs],” says Ian Gorton , director of the computer science master’s programs at Northeastern University—Seattle . “[We want] graduates to have a deep enough education theoretically so they can keep up with advances and changing technology, but also have the practical skills needed to get a job and thrive professionally.”
Northeastern’s MS in Computer Science curriculum is strategically designed to expose students to both computer science theory and practice. Courses like advanced software development, for example, cover advanced system design and solution-building, while simultaneously providing students the chance to practice explaining their solutions through “code walk-throughs.”
“[A code walk-through] is a common industry practice in which people stand up and explain their solution to an instructor…and then get questioned about it,” Gorton says. “This is obviously good interview practice, but also breeds a rigor in people that gets them thinking about how to solve problems.”
Learn More: Is a Master’s in Computer Science Worth the Investment?
Other courses focus on building software individually or as part of a small team to solve bigger problems. In these scenarios, students “work together to try to solve problems and build a solution,” Gorton says. Through this process, “they learn principles about how to split up a solution so that multiple people can work on it at the same time, and how they can plug the resulting components together and make it all work” the way they might as part of a future engineering team.
This type of hands-on learning also extends outside of the classroom in Northeastern’s programs. Through experiential learning opportunities, students get the chance to explore real-world problems for organizations that are a part of Northeastern’s expansive partner network.
“In our advanced software engineering course, we basically form a team of about 20 people, and we build a solution to a real problem…[for] an external stakeholder,” Gorton says. “It’s always a really tricky problem and requires teams to work across campuses to build a solution.”
Did You Know: Northeastern offers advanced computer science degrees at seven of our regional locations, including Arlington ; Boston ; Oakland ; Portland, Maine ; Seattle ; Silicon Valley ; and Vancouver .
Northeastern’s program also embraces the need for students in this field to have certain interdisciplinary skills that allow them to apply their technical knowledge to other industries. In fact, Northeastern’s Align MS in Computer Science program is specifically designed for students from a non-computer science background . In this program, students first learn the foundational CS skills they would have obtained in pursuing an undergraduate CS degree and then advance to the more complex topics covered in a master’s degree. These individuals graduate with advanced CS knowledge that complements their existing expertise in another sector and will allow them to change careers to computer science.
Students with or without a background in computer science also have the opportunity to declare a computer science specialization while enrolled in Northeastern’s master’s in CS programs. In doing so, they have the chance to learn an array of other relevant, practical skills that they can use in pursuit of specialized roles. For example, students who declare a specialization in AI might explore more advanced data science topics, as well as statistical modeling , natural language processing, machine learning, and more.
Gorton explains that, while specializations like these can be useful in setting professionals apart in competitive job markets, most will likely start their career in CS with a much broader role. For this reason, Northeastern strategically incorporates a balance between core skill sets and those explored in a specialization. “You have to have a good broad portfolio of skills and then have a specialization that you can kind of pull out of your pocket when you need to,” he says.
2. Relevant “Soft” Skills
Soft skills are considered those which are not tactical, technical, or tied directly to a specific career path. Emotional intelligence, leadership , and innovation are common examples of these kinds of traits, which are gained through hands-on experience and are valued across industries .
While technical skills were often thought of as the core of functioning workplaces in the past, businesses today now consider “soft skills” to be equally— if not more —relevant. Below, we explore the top “soft skills” that computer scientists should obtain.
- Critical Thinking: Being able to identify a problem, analyze the details of the situation, and then formulate an effective solution is an incredibly important aspect of computer science work.
- Attention to Detail: Effective computer scientists must be able to pay close attention to detail, as their work is often complex, demanding, and requires a keen eye.
- Creative Problem Solving: Solutions to many of the problems computer scientists are tasked with solving are not always obvious, and instead require these individuals to think outside of the box.
- Communication: Communication is a key soft skill in most industries , and computer science is no different. Professionals in this field must be able to communicate effectively with their teams , their bosses, and their stakeholders—including using data to tell stories and share insights.
- Listening : Tied to the need for proper communication skills is a need for computer scientists to be good listeners. Professionals should be able to listen to people’s problems and establish the necessary context from those conversations to solve them. This is especially important when CS specialists work with industries they’re not particularly familiar with.
- Collaboration: Despite common misconception, computer scientists very rarely work in isolation. In fact, more often than not, they are required to operate as part of a team, either working with other computer scientists to reach a solution or develop a product, or working with other members of a larger business team on a project. Either way, developing the skills needed to work as part of a larger group is essential to a computer scientist’s success.
Honing Your Soft Skills With a Master’s Degree
Taking the time to develop these soft skills can go a long way in setting aspiring computer scientists on a path toward success. Many of these abilities can be obtained through real-world experience or the pursuit of a Master of Science in Computer Science degree at a top university like Northeastern.
Many of the courses Gorton mentions have been designed to hone students’ practical skills in Northeastern’s CS programs also simultaneously provide opportunities for students to develop many of these integral skill sets. Courses that require students to stand up in front of their professors and peers and complete a code walk-through, for example, allow them to sharpen their communication skills. Similarly, courses in which students work as part of a team allow them to practice collaboration, listening, and leadership. By incorporating real-world problem solving into coursework early on—and providing countless opportunities for hands-on learning within functional workplaces—Northeastern also gives students the chance to practice critical thinking, creative problem solving, and more.
Graduate school provides a unique opportunity for aspiring computer scientists to acquire these vital skill sets alongside more technical ones, and develop themselves into well-rounded professionals.
3. Interview Skills
When pursuing a new role in any industry, applicants should work to develop their interview skills . This might include preparing for your interview by doing research on the company you’re interviewing with, prepping your answers to commonly asked questions , or even practicing maintaining eye-contact during a conversation.
In certain industries, however, there is more to an interview than a simple series of questions and answers. In the computer science field, for instance, an interview often includes an in-person presentation and a descriptive explanation of your work in which applicants answer in-depth questions about how they reached their solutions. An interview in this field may also require applicants to display certain examples of their live projects via a portfolio and to speak at length about the success of that work.
In order to prepare for this unique type of interview, Gorton identifies a few key skill sets that aspiring CS professionals should work to hone beforehand.
Presentation Skills
“There is a whole sort of ritual for a technical interview these days,” he says. “You have to not only be able to answer [an interviewer’s] questions, but you have to also know how to communicate your solution.”
In order to do this, an interviewee will be asked to write out a problem on a whiteboard and describe the thought process as they work to solve it. While the act of problem-solving should likely be second nature to those in this field, this interview task often feels more daunting to those without proper presentation skills. “You have to explain what you’re doing as you go along and engage the interviewer in a dialogue,” he says. “You don’t just want to turn around and start writing on the board for five minutes.”
To sharpen this skill, Gorton suggests practicing this process a few times through before each interview to ensure you are comfortable both with your actual mathematics work and your presentation abilities. “You’re probably not going to get it right the first time,” he says, emphasizing that practice makes perfect.
For this reason, Northeastern’s programs “spend a lot of time working with students on those sorts of technical problems and getting them not only to understand their solutions on a technical level, but [also] how to communicate them properly,” he says.
Portfolio Building
While computer scientists may not utilize a print or online portfolio to display their work the way professionals in other fields might, it is still important for them to know how to properly share their work in an interview if asked.
Gorton explains that prepared CS professionals will either link to live projects or show examples within code repositories online. “When we write solutions, we put them typically into something called GitHub , and you make your GitHub code or profile public so you can cite it on your resumé and show the projects you’ve contributed to.”
Setting You Up for Success: Having the ability to properly integrate coding examples into a resumé is an essential skill for computer scientists, but many computer scientists who are just starting out don’t have live examples of their work to share. To help build this portfolio of work early on, Northeastern’s master’s in computer science programs give students dozens of opportunities to practice their work hands-on through experiential learning opportunities, group projects, in-class work with real-world external stakeholders, and more.
Networking is a pivotal part of any interview process, no matter the industry. Today, 85 percent of all jobs are filled through this practice, and the computer science field is no different. The positive, professional connections aspiring CS professionals make through their time in graduate school or in the workforce have the ability to set them apart during interview processes—especially when it comes to applying to top tech companies like Google, Facebook, or Amazon.
For this reason, individuals need to take the time to learn how to properly make and maintain relationships with those in the industry. Northeastern’s graduate programs in computer science , for example, offer opportunities for students to attend university-hosted events, collaborate with industry professionals, and make real-world connections with those in their field through time spent working hands-on with leading organizations .
Pursuing a Master’s Degree from Northeastern
Whether you are looking to break into the computer science industry or are hoping to advance your career, a master’s degree from Northeastern University can help. Both the master’s in computer science and Align master’s in computer science programs are designed to help aspiring professionals obtain the technical, “soft,” and interview skills needed to thrive in this industry, all while under the guidance of leading industry professionals. Grow your network, build your resumé , and start your career off right with a master’s degree in computer science from Northeastern.
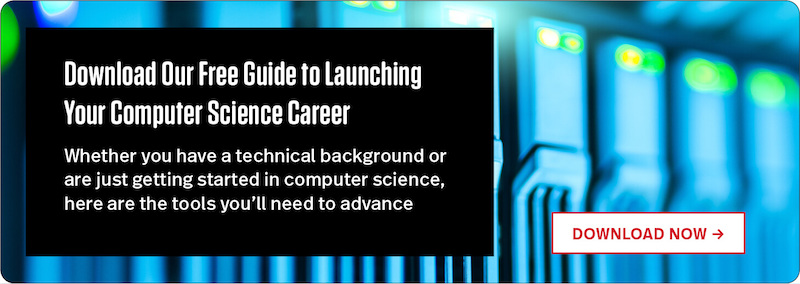
Subscribe below to receive future content from the Graduate Programs Blog.
About shayna joubert, related articles.
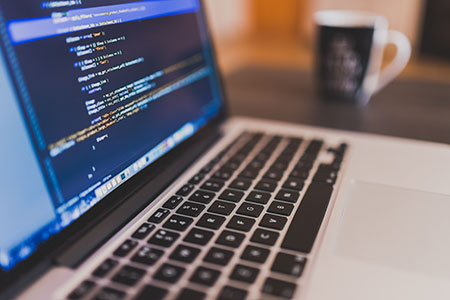
Why This Alum Decided to Earn His Master’s Degree in Computer Science
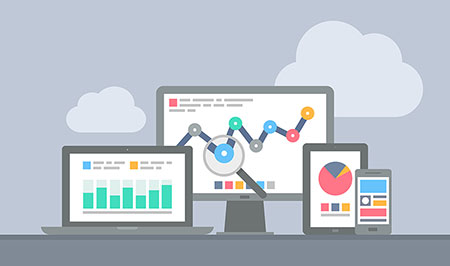
The Top 10 Highest-Paying Big Data Careers
Data scientist vs. data analyst: what’s the difference, did you know.
Computer science professionals see an average $30,000 salary increase after earning a master's degree. (Georgetown Center on Education)
Master of Science in Computer Science
Every company needs computer scientists. Launch your career today.
Most Popular:
Tips for taking online classes: 8 strategies for success, public health careers: what can you do with an mph, 7 international business careers that are in high demand, edd vs. phd in education: what’s the difference, 7 must-have skills for data analysts, in-demand biotechnology careers shaping our future, the benefits of online learning: 8 advantages of online degrees, how to write a statement of purpose for graduate school, the best of our graduate blog—right to your inbox.
Stay up to date on our latest posts and university events. Plus receive relevant career tips and grad school advice.
By providing us with your email, you agree to the terms of our Privacy Policy and Terms of Service.
Keep Reading:
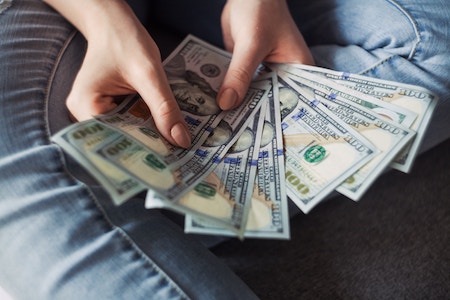
The 8 Highest-Paying Master’s Degrees in 2024
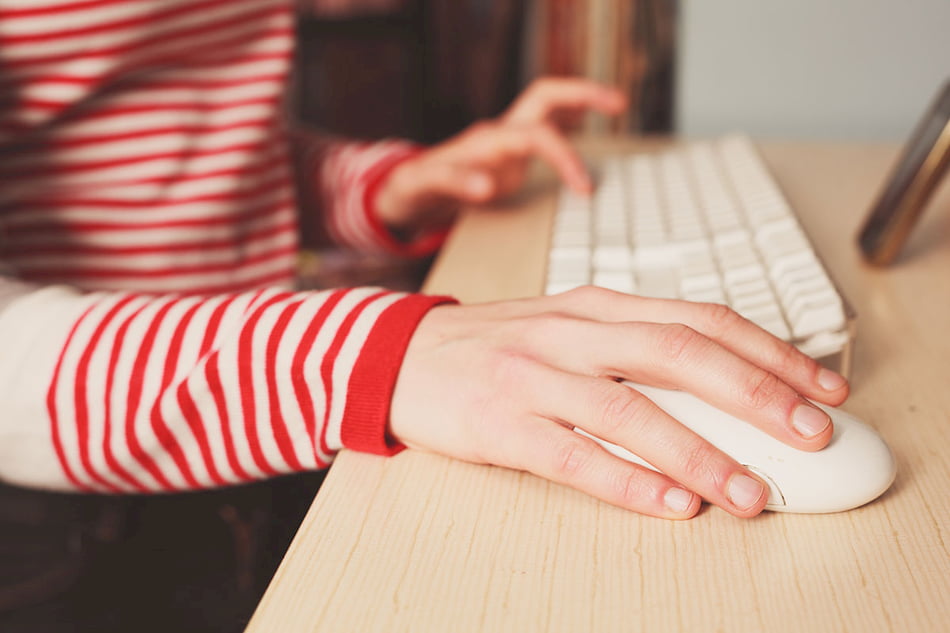
Graduate School Application Tips & Advice
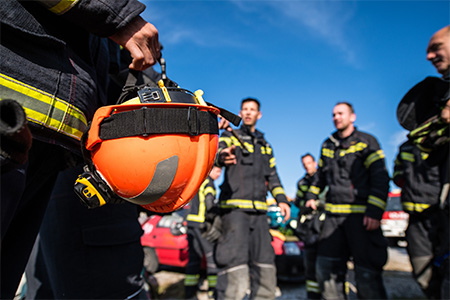
How To Get a Job in Emergency Management
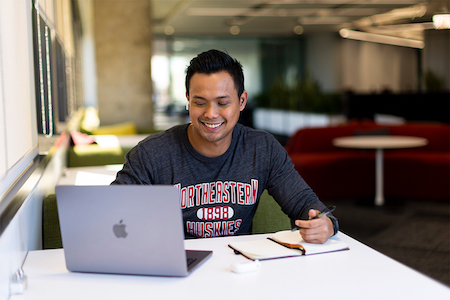
Join Us at Northeastern’s Virtual Graduate Open House | March 5–7, 2024
Oberlin College Computer Science
- Course Websites
- CS Lab Helper Schedule
Problem Solving Tips
- Course Catalog
- Major/Minor in CS
- Electives Schedule
- Intro Course Placement
- Academic Calendar
- Department Honors
- Bob Geitz (chair)
- Stephen Checkoway
- Roberto Hoyle
- Dylan McKay
- Sam Taggart
- Cynthia Taylor
- Blair Rossetti
- Molly Feldman
- 2013-Wearable-Electronics
- 2011 ACM Programming Contest
- 2013 Alexa's Tenure Celebration
- 2013 Pledge Ceremony
- 2012 Games Showcase
- 2011 CSMC Unix Night
- MCURCSM 2009
- 2009 Games Showcase
- OCCS 25th Anniversary
- 2010 Spring Social
- 2009 Spring Picnic
- 2009 Math/CS Ice Cream Social
- 2008 Spring Picnic
- 2010 Denison Programming Contest
- 2008 Math/CS Sundae Friday
- 2009 ACM Programming Contest
- 2009 Denison Programming Contest
- 2008 ACM Programming Contest
- 2008 Denison Programming Contest
- 2007 ACM Programming Contest
- 2006 ACM Programming Contest
- Oberlin College
One of the most important skills you learn in your computer science courses is how to problem solve. Although we cover some general problem solving paradigms in class, the best way to improve these skills is to get practice, practice, and more practice. Different people have different techniques that work best for them; below are some general tips that work for most people.
Please read these suggestions carefully.
Questions the Helpers May Ask You
When you ask a lab helper for their assistance, they will assume you have tried to solve the problem yourself. They will (reasonably) expect that you have tried out the steps outlined in this document; you should therefore be prepared to answer the following questions:
- Did you re-read the prelab and lab?
- Do you understand the problem?
- Have you tried solving some examples by hand?
- (For problems designing a solution) What have you tried? What topic from class does this most ressemble?
- If you can’t solve the problem whole-hog, what small case can you solve?
- (For syntax errors) What line of your code is causing the error? What do you think the compile error means, and what usually causes this kind of problem?
- (For logical errors) On what example does your program consistently break? Have you traced through the program? Which line of your program is not doing what it should?
Four Main Problem Solving Steps:
1. understand the problem..
Solving the right problem is the most important part of problem solving. Be sure, absolutely 100% positively sure, that you understand the problem before attempting a solution. This involves:
- Reading the prelab and lab very carefully (including all bold text, italicized text, and everything else);
- Reviewing class notes on related topics;
- Trying some small examples to make sure you understand what is being asked; if examples are given to you, make sure you understand them before continuing, as they are usually there to help clarify some common misconceptions; and
- Asking someone to help clarify anything that is still confusing.
2. Design a Solution.
Formulate an algorithm to solve your problem. This involves:
- Understanding what is being asked of you. See step 1.
- Draw out some examples. Use paper . How would you solve these small cases, by hand? Is there a method to what you are doing? Try to formalize the steps you are taking, and try to think about whether they would work more generally, in bigger cases. Then try some bigger cases and convince yourself.
- Reread the prelab . Did you already run some examples by hand? Did you have trouble with it then?
- Write down the stuff you know about the problem and the examples you’ve tried, so that you can more easily find patterns .
- Might a recent topic from class help? Usually at least some, if not most, of the lab will make use of recently covered material . Go over that topic, make sure you understand it, then try to make connections to lab.
- Split the problem into smaller (more manageable) chunks, and try to solve the simpler problems. Go as small as you need in order to find some solution. Once you have the smaller problem solved, worry about how to generalize it to a slightly larger problem.
- Just try something , anything, even if it is completely random and obviously wrong. When/if your attempt doesn’t work, it may still give you insight into what may work. It is not as crazy as it initially sounds!
- Use a friend, lab helper, puppet, etc. as a sounding board ; sometimes, just voicing your problem will lead you to the “aha!” moment you need.
- If you are still stuck, step away from the keyboard . Take a walk, go eat dinner or have a coffee. Sleep on it. Not literally. Taking a break is sometimes the most productive thing you can do, trust me.
- Finally, stay positive . Even when things don’t work, you can still gain a better understanding of the problem. Don’t give up, just go with the flow and see where it takes you. Struggling is part of the process!
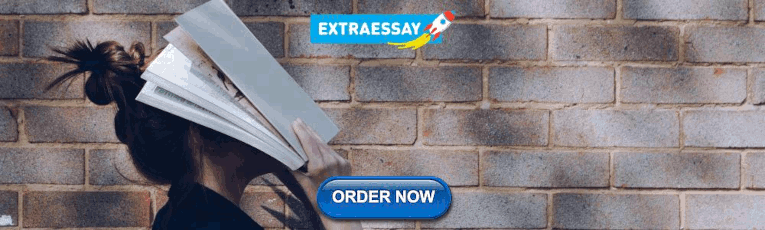
3. Implement your Solution.
Write the code to solve your problem. This involves
- Understanding the problem, and designing a solution on paper. See steps 1 and 2.
- Translating your design into actual code. Rather than doing this linearly, implement small chunks at a time. Break your code into subroutines, and make sure that each subroutine works before proceeding to the next. Compile and save often .
- If you run into syntax errors, determine which line of your code is causing the problem. You can do this by systematically commenting out blocks of code until you find the block that causes the problem.
- If you run into logical errors (as in, the program compiles but does not do what it is supposed to), find some examples on which your problem consistently fails. Trace through the program line by line, with one of these examples, to figure out exactly which line is not doing what you intend it to.
- If the output doesn’t match what you expect, use print statements to trace through what your program is doing, and compare that to what your program should be doing. Even better, if you know how to use a debugger (in eclipse, for example, use it!)
4. Check your Solution.
This step is often overlooked, but is absolutely crucial. Your program does not necessarily work because it works on the given test cases on the lab. You have to think critically about what you code. This involves
- Certainly check your program on all test cases given to you on the lab and prelab. The prelab often specifically contains hand-solved test cases precisely for this purpose!
- Thinking about the “ boundary cases ,” such as, when would this array go out of bounds? For what indices will this for loop start and end?
- Think: how would this program break ? Then, that failing: how would I convince my skeptical friend it can’t be broken?
Remember: problem solving is a creative process, which cannot be forced. Don’t get angry if you don’t see the answer right away, or you don’t see it as fast as your friend. You will have different strengths, and you can always improve. You will learn from your mistakes, so that’s always a plus!
Last updated July 3rd, 2012 by asharp
Recent Posts
- Congratulations Professor Stephen Checkoway; recipient of this prestigious award!
- Class of 2021 Celebration
- Undergraduate Research Symposium
- Grad School Information meeting
- Tech Resume Workshop Thursday April 1 4:45pm
- Information
- Jobs/Internships
- Uncategorized
- Association for Computing Machinery (ACM)
- National Center for Women & Information Technology (NCWIT)
- Computer Research Association (CRA)
OCCS Social Media
- Entries feed
- Comments feed
- WordPress.org
Oberlin College Computer Science Department -- 10 N. Professor St., Oberlin, OH 44074 -- Phone: (440) 775-8043
Copyright © 1985-2024 Oberlin College Computer Science. Design by Benjamin A. Kuperman . Powered by WordPress .
- IEEE CS Standards
- Career Center
- Subscribe to Newsletter
- IEEE Standards
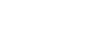
- For Industry Professionals
- For Students
- Launch a New Career
- Membership FAQ
- Membership FAQs
- Membership Grades
- Special Circumstances
- Discounts & Payments
- Distinguished Contributor Recognition
- Grant Programs
- Find a Local Chapter
- Find a Distinguished Visitor
- Find a Speaker on Early Career Topics
- Technical Communities
- Collabratec (Discussion Forum)
- Start a Chapter
- My Subscriptions
- My Referrals
- Computer Magazine
- ComputingEdge Magazine
- Let us help make your event a success. EXPLORE PLANNING SERVICES
- Events Calendar
- Calls for Papers
- Conference Proceedings
- Conference Highlights
- Top 2024 Conferences
- Conference Sponsorship Options
- Conference Planning Services
- Conference Organizer Resources
- Virtual Conference Guide
- Get a Quote
- CPS Dashboard
- CPS Author FAQ
- CPS Organizer FAQ
- Find the latest in advanced computing research. VISIT THE DIGITAL LIBRARY
- Open Access
- Tech News Blog
- Author Guidelines
- Reviewer Information
- Guest Editor Information
- Editor Information
- Editor-in-Chief Information
- Volunteer Opportunities
- Video Library
- Member Benefits
- Institutional Library Subscriptions
- Advertising and Sponsorship
- Code of Ethics
- Educational Webinars
- Online Education
- Certifications
- Industry Webinars & Whitepapers
- Research Reports
- Bodies of Knowledge
- CS for Industry Professionals
- Resource Library
- Newsletters
- Women in Computing
- Digital Library Access
- Organize a Conference
- Run a Publication
- Become a Distinguished Speaker
- Participate in Standards Activities
- Peer Review Content
- Author Resources
- Publish Open Access
- Society Leadership
- Boards & Committees
- Local Chapters
- Governance Resources
- Conference Publishing Services
- Chapter Resources
- About the Board of Governors
- Board of Governors Members
- Diversity & Inclusion
- Open Volunteer Opportunities
- Award Recipients
- Student Scholarships & Awards
- Nominate an Election Candidate
- Nominate a Colleague
- Corporate Partnerships
- Conference Sponsorships & Exhibits
- Advertising
- Recruitment
- Publications
- Education & Career
How Computer Engineering Helps You Think Creatively
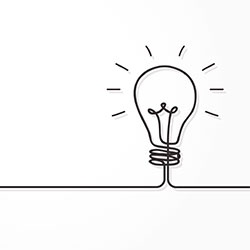
But in reality, learning the basics of computer science can help you think more critically and with more novel inspiration, ultimately helping you in other areas of your life.
Thinking of a career in computing? Our “Careers in Computing” blog will get you there
Apply Creative Problem Solving to Other Areas
Let’s start by explaining why the creative problem-solving skills you’ll learn in computer science can help you in everyday life:
- Novel solutions and new products. Being familiar with creating and polishing hardware and/or software can help you come up with ingenious solutions for your everyday life. You’re used to thinking about problems as solvable challenges, so you naturally come up with ways to address them. This applies to areas beyond computer science as well; for example, one former computer engineer used his creativity to engineer a pillow that reduces pressure on your face while sleeping .
- Lateral thinking and breaking patterns. Writing code and creating applications from scratch also incentivizes you to think laterally and break the patterns you’d otherwise fall into. Traditional lines of thinking just won’t work for some problems, so you’ll be forced to think in new, creative ways. That allows you to experiment with new approaches and keep trying until you find something that works.
- Seeing problems from other perspectives. As a computer engineer, you’ll be forced to see problems from other perspectives, whether you want to or not. That might mean reviewing code that someone else wrote, getting feedback from a client who has no familiarity with engineering, or imagining how an application might look to a user who’s never seen it before. In any case, you’ll quickly learn how to broaden your perspective, which means you’ll see problems in an entirely new light.
How Computer Engineering Improves Your Abilities
So how exactly does computer engineering improve your creative abilities in this way?
- Generating new ideas. You have to be creative if you’re going to generate new ideas . In some roles, you’ll be responsible for coming up with the ideas yourself—either designing your own apps for circulation, or making direct recommendations to your clients. In other scenarios, you’ll be responsible for coming up with novel ways to include a feature that might otherwise be impossible. In any case, you’ll be forced to come up with ideas constantly, which gets easier the more you practice it.
- Reviewing code. You’ll also be responsible for reviewing code—including code that you wrote and code that other people wrote. Reviewing your own code forces you to see it from an outsider’s perspective, and reviewing the code of others gives you insight into how they think. That diverse experience lends itself to imagining scenarios from different perspectives.
- Fixing bugs. Finding and fixing bugs is an important part of the job, and it’s one of the most creatively enlightening. To resolve the problem, you first have to understand why it’s happening. If you’ve written the code yourself, it’s easy to think the program will run flawlessly, so you’ll have to challenge yourself to start looking for the root cause of the problem. Sometimes, tinkering with the code will only result in more problems, which forces you to go back to the drawing board with a new angle of approach. It’s an ideal problem-solving exercise, and one you’ll have to undergo many times.
- Aesthetics and approachability. Finally, you’ll need to think about the aesthetics and approachability of what you’re creating. Your code might be perfectly polished on the backend, but if users have a hard time understanding the sequence of actions to follow to get a product to do what they want, you may need to rebuild it.
Latest career advice from our Career Round Table: With Demand for Data Scientists at an All-Time High, Top Experts Offer Sound Career Advice
Is Computer Science Worth Learning?
If you’re not already experienced in a field related to computer science, you might feel intimidated at the idea of getting involved in the subject. After all, people spend years, if not decades studying computer science to become professionals.
The good news is, you don’t need decades of experience to see the creative problem-solving benefits of the craft. Learning the basics of a programming language, or even familiarizing yourself with the type of logic necessary to code, can be beneficial to you in your daily life. Take a few hours and flesh out your skills; you’ll be glad you did.
Recommended by IEEE Computer Society
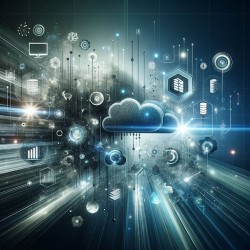
5 Ways to Save on Database Costs in AWS
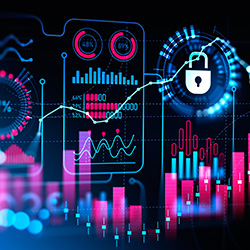
Redefining Trust: Cybersecurity Trends and Tactics for 2024
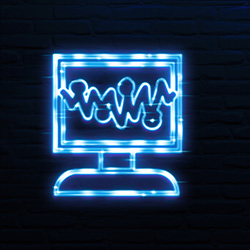
Why is Data Normalization Important?
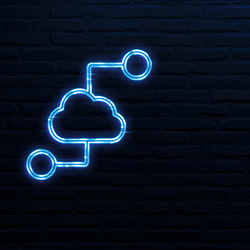
From Code Readability to Reusability, Here’s How Terraform Locals Enhance IaC Management
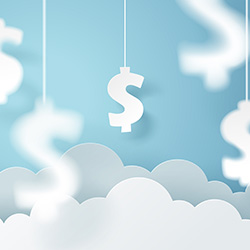
Cutting Cloud Costs: Key Strategies to Keep Budgets in Check
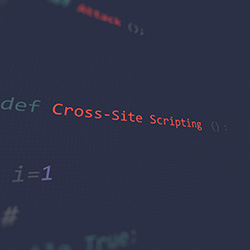
Understanding the Threat of XSS (Cross-Site Scripting)
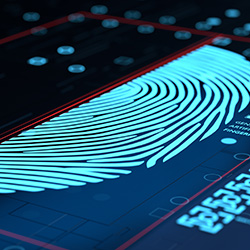
4 Legal Insights into Biometric Privacy Laws and Regulations
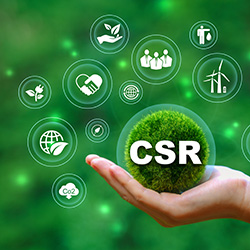
How to Use Corporate Social Responsibility to Enhance Communication and Relationships
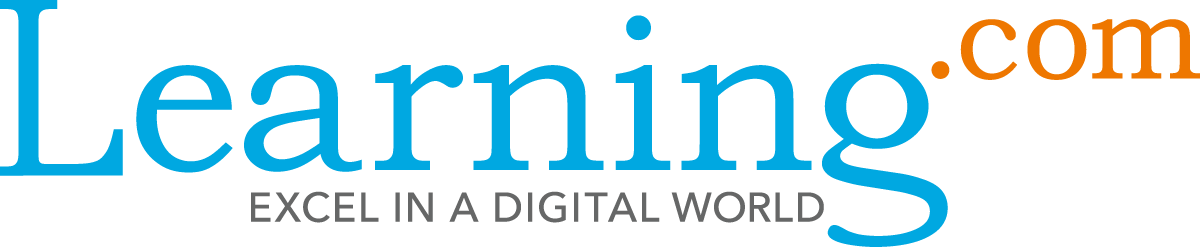
Computational Thinking: Its Purpose & Importance
by Lcom Team | Nov 14, 2023 | Blogs
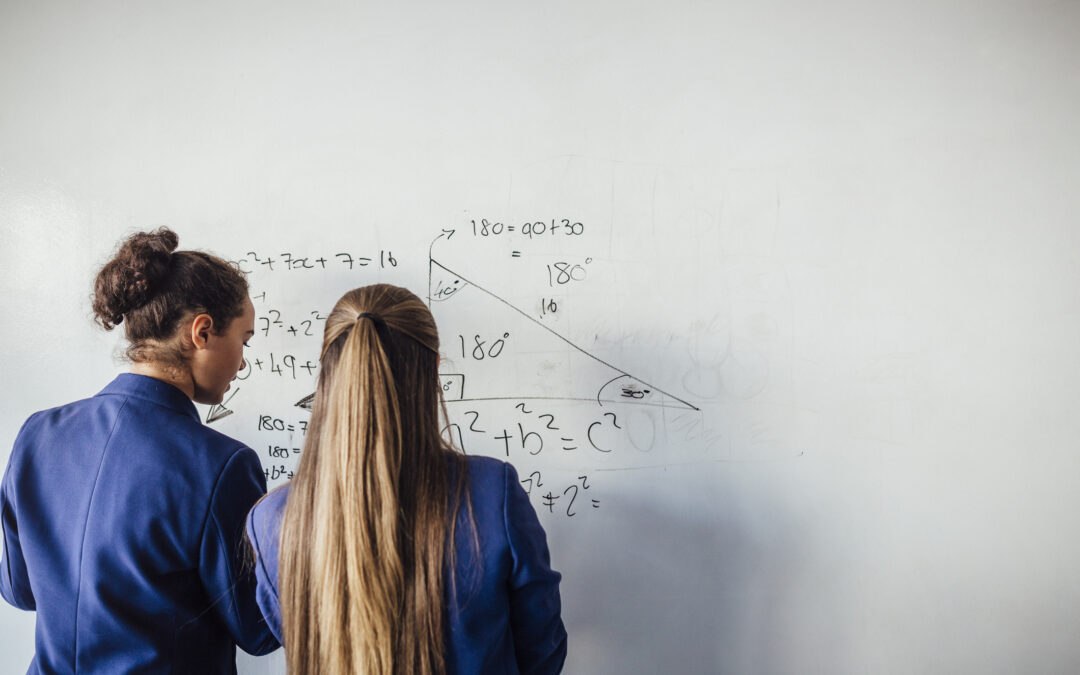
Share this article!
Computational thinking is more related to math and algorithms than it is to digital technology. It refers to “computing” a solution by breaking down a problem into its separate parts and discovering the effective steps that reliably and effectively resolve the problem. In math, this might look like the “ order of operations ,” which defines how to decompose and solve a linear math problem.
What is the Purpose of Computational Thinking?
The purpose of computational thinking is to be able to solve complex problems in a structured, effective and repeatable way. Computational thinking, while drawing on principles from computer science and mathematics, can be applied not only to mathematical or technology-related problems, but to real-world problems as well. Therefore, computational thinking provides an effective and repeatable process for solving complex issues regardless of whether they are technologically dependent.
How Does Computational Thinking Work?
In a previous article defining computational thinking , we discuss how computational thinking identifies a clear, defined step-by-step solution to a complex problem. But how, exactly, does one utilize computational thinking to define this solution?
Whether the problem to be solved is in a technological environment or is “offline,” (that is, not related to technology), computational thinking helps to approach, understand, analyze and resolve the problem in an effective and efficient manner. The process is as follows:
- Decomposition. First, the problem is decomposed into smaller, more manageable parts. This helps the problem-solver more effectively understand the problem while being able to eliminate those parts that are irrelevant.
- Pattern Recognition. In the next step, pattern recognition , the problem solver identifies patterns or connections between the different parts identified during decomposition—or even to other previously-solved problems. The purpose of this step in computational thinking is to further simplify the problem as well as to begin identifying areas of the problem that may be solved similarly.
- Abstraction. Decomposition and pattern recognition empower the problem solver to use abstraction to identify the most relevant information within the problem while eliminating that which is either repeated elsewhere or irrelevant. This simplifies an otherwise complex problem and creates a more efficient environment for the individual to identify how the different parts of the problem may be solved.
- Algorithmic Thinking. Algorithmic thinking is the process of defining a step-by-step solution to the problem. The key to an algorithmic solution is that it should be able to be replicated for a predictable and reliable outcome (in other words, for those familiar with billiards, “ slop shots ” don’t count). The benefit of having a replicable solution is that it is more certainly a reliable outcome if the result can be repeated. In addition, a well-defined replicable solution may be more effectively used in part or in whole to resolve other issues.
Why is Computational Thinking Important?
Computational thinking is an important future-ready skill for students and adults alike. This sophisticated process for problem-solving empowers the learner with more effective tools to solve complex problems as well as to produce more effective processes in the future.
- Problem solving. The most well-known benefit of computational thinking is the increased ability to solve complex problems. Just like how computational thinking provides effective steps to solve a complex problem, the process of computational thinking, itself, is a computational solution for solving complex problems.
- Automation and efficiency. Computational thinking is essential in the automation of tasks and processes, which means it’s critical for such applications as coding and automation. The applications of these are far-reaching, from science and engineering to marketing, sales, social sciences, big data and more.
- Data Analysis. In the age of big data , computational thinking is essential for processing and interpreting vast amounts of information. It helps in extracting meaningful insights and making data-driven decisions.
- Innovation. Computational thinking is a driver of innovation. At its core, computational thinking helps to solve complex problems, which is the same basis that inspires innovative solutions to these problems. Without the ability to problem-solve using computational thinking, it would be difficult to define and replicate innovative solutions to modern problems.
- Career opportunities. The ability for an individual to use computational thinking to problem-solve empowers the individual with a “soft skill” that is highly valued in most industries and leadership positions. From manufacturing to finance, technology to healthcare and beyond, these industries actively seek individuals who can solve complex problems and drive innovation.
Final Thoughts
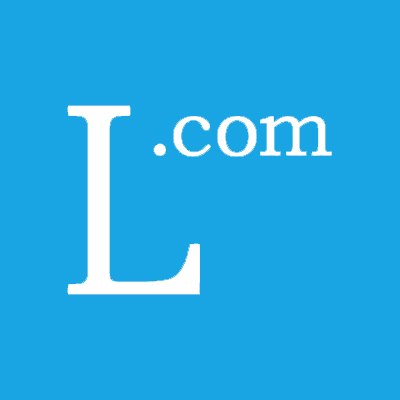
Learning.com Team
Staff Writers
Founded in 1999, Learning.com provides educators with solutions to prepare their students with critical digital skills. Our web-based curriculum for grades K-12 engages students as they learn keyboarding, online safety, applied productivity tools, computational thinking, coding and more.
Further Reading
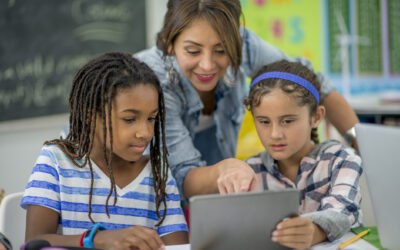
- Why Teaching Kids to Code Supports Community Development
by Lcom Team | Mar 26, 2024
Teaching kids to code undoubtedly prepares them for the future of work. But what does this mean for the communities that helped them become career...
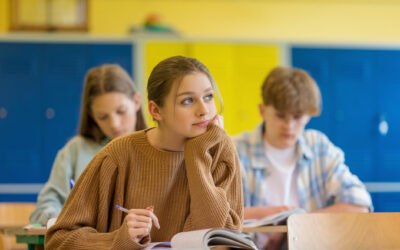
- Enhancing Digital Citizenship by Understanding Confirmation Bias
by Lcom Team | Mar 19, 2024
Teaching confirmation bias as part of the digital literacy curriculum in schools is essential in today's information-saturated world. Confirmation...
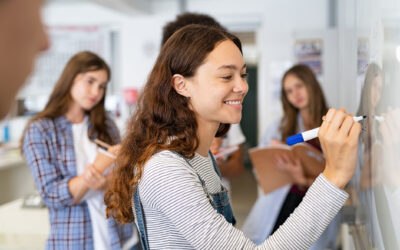
- Understanding Computational Thinking for More Effective Learning
by Lcom Team | Mar 12, 2024
The idea of bringing computational thinking into all areas of education is becoming increasingly important. Even though computational thinking has...
Quick Links
- Request More Info
- Cookie Settings
Recent news & Articles
- Digital Skills for North Carolina Students
- Teaching Computer Programming Principles Without a Computer
Why students need Computer Science to succeed
March 01, 2023.
By Microsoft Education Team
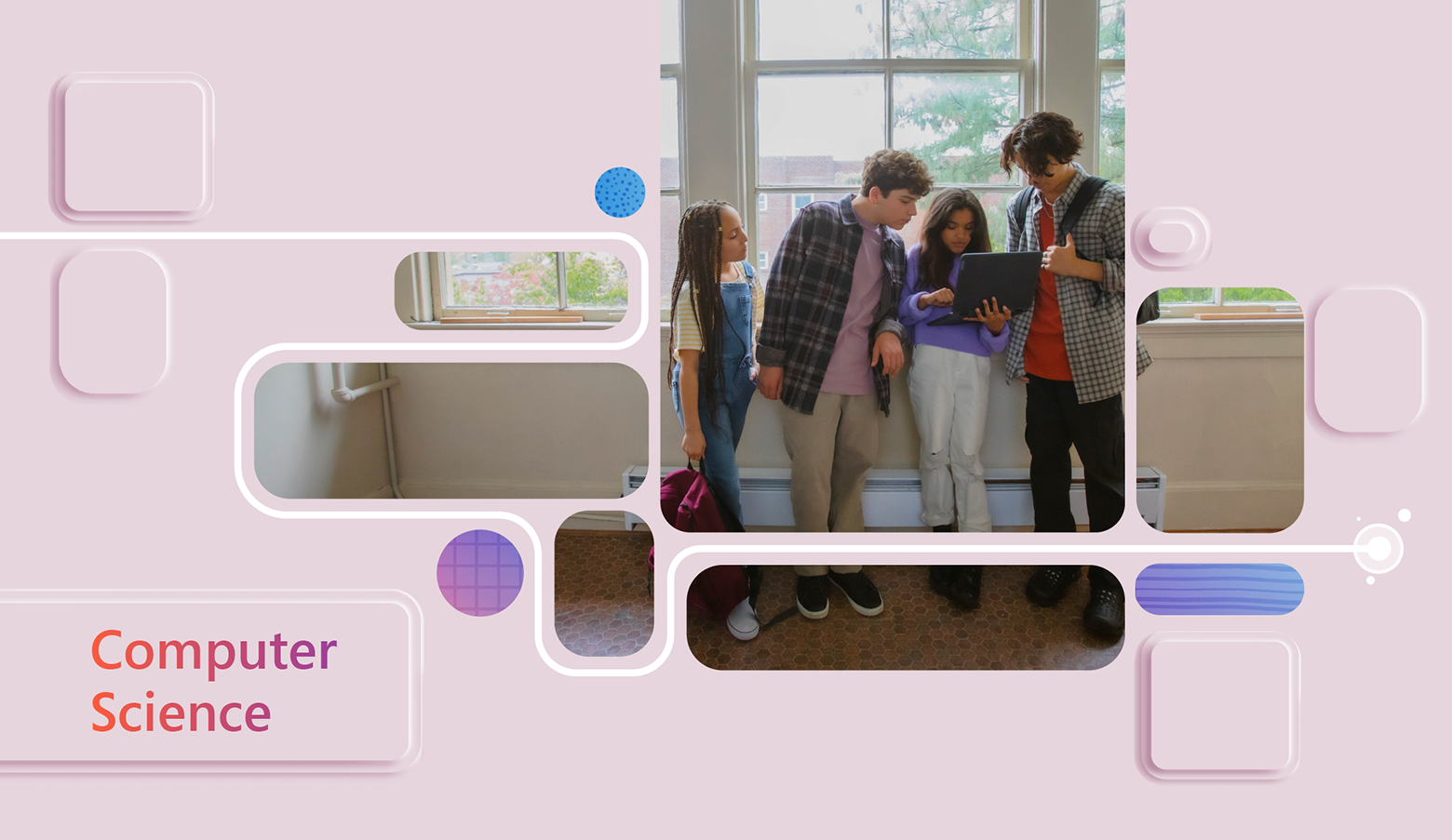
Share this article
As technology continues to evolve at an accelerated pace, transforming the way we live and work in the process, we find ourselves navigating the challenges of an always-changing digital landscape. Understanding the principles of computing is quickly becoming an essential skill. It provides people with a keen understanding of how technology impacts their lives, empowers them to become full participants in society, and unlocks a wide range of career opportunities. This is especially true for today’s students, who will rely on computing skills throughout their lives, making it necessary for them to have opportunities to learn Computer Science (CS).
A report by LinkedIn and Microsoft revealed that 149 million new digital jobs will be created by 2025 in fields such as software development, data analysis, cybersecurity, and AI. However, education cannot currently meet the growing demand for people with CS skills. As of October 2022, only 33% of technology jobs worldwide were filled by the adequately skilled. And by 2030, the global shortage of tech workers will represent an $8.5 trillion loss in annual revenue, according to research cited by the International Monetary Fund i .
“Around the world, technology is opening up opportunities for new ways to solve the challenges and needs of businesses and organizations, everything from technology-focused [industries] to agriculture, healthcare, financial services, transportation and so many more. They’re all struggling to find the talent they need to fill many of the jobs.” – Christina Thoresen, Director of Worldwide Education Industry Sales Strategy at Microsoft
A growing interest in CS curricula
Learning coding and software development, two key parts of CS, has been shown to improve students' creativity, critical thinking, math, and reasoning skills ii . CS skills like problem-solving iii and planning iv are transferable and can be applied across other subjects. A 2020 study examining the effects of CS courses on students’ academic careers in the United States showed that they have a significant impact on the likelihood of enrolling in college v . Moreover, CS can be useful for many courses and degrees including biology, chemistry, economics, engineering, geology, mathematics, materials science, medicine, physics, psychology, and sociology vi .
CS curricula that are relevant and engaging provide an additional benefit in that they attract traditionally marginalized groups and girls and empower those with lower access to technological resources to develop high value skills, and unlock new and exciting career opportunities. It is also worth noting that due to enduring talent shortages, CS-related fields consistently offer above-average pay and have the fastest-growing wages vii .
How Microsoft supports CS implementation
Microsoft has been helping educational institutions around the world develop rich CS curricula that empower all students with the skills they need to confidently transition from classroom to career. By creating content that is meaningful and engaging for all students, as well as helping promote equal access to CS in school, Microsoft is fulfilling its commitment to making learning more inclusive and equitable. One of the principal resources for this is Microsoft’s Computer Science Guide (MCSG) , a comprehensive CS framework that includes:
- An implementation plan
- Training for educators
- Lesson and project suggestions
- Practical guidance for coding activities
- Certification
An important part of building up students’ CS capabilities is to engage learners as early as possible, which encourages and supports creative expression and the development of computational thinking skills. However, CS curriculums at the national level often focus on ICT or simple coding exercises and offer little in terms of immersive, hands-on experiences that feel relevant, authentic, and inclusive. The MCSG was made to engage students of all ages through a learner-centric curriculum using constructivism, hands-on activities, problem-solving, and inquiry-based approaches that are often linked to real-world challenges viii .
CS curriculum design can also help address a well-documented gender divide ix by engaging all students as early as primary school using relevant and meaningful content. It can ensure that all students have access to CS courses based on their needs and abilities, regardless of socio-economic status, race, ethnicity, or special learning needs. Additionally, as students are likely to encounter changes in technology that are difficult to imagine over the course of their education, another key goal of the MCSG is to be future-proof by incorporating subjects that are likely to be highly relevant well into the future.
“Computer science skills are critical to succeed in today’s economy, but too many students – especially those from diverse backgrounds and experiences – are excluded from computer science. That’s why we’ve created a new resource guide which we hope will help teachers build inclusive computer science education programs.” -Naria Santa Lucia, General Manager of Digital Inclusion and Community Engagement for Microsoft Philanthropies
Georgia Ministry of Education develops national CS program
In 2022, the Ministry of Education and Science of Georgia launched a pilot program to test how the Microsoft CS Curriculum could be integrated into primary classes as part of a national campaign to introduce broader CS concepts and computational thinking to K-12 learning. The pilot project focused on two ICT teachers and was reviewed by volunteer educators from other cities. An advisory board was formed consisting of experts from the National Curriculum Department.
The process involved translating the Foundation Phase of the Microsoft CS Curriculum Toolkit into Georgian, as well as weekly meetings to discuss progress. In the end, the teachers designed two curriculums for the 2nd and 3rd grades, and the project team made a recommendation for a completely new framework concept that considered the existing National Curriculum context, the integration of the Microsoft CS Curriculum Framework, as well as additional concepts from the Computer Science Teachers Association.
Learn more about computer science with Microsoft
It is no longer possible to ignore the critical importance of CS skills to students whose lives are going to revolve around their ability to understand and engage with technology, both at work and in their day-to-day. At Microsoft Education, our goal is to empower every learner on the planet to achieve more. That is why we are working together with governments and education leaders around the world to implement CS in schools and ensure that students feel included, supported, and empowered to confidently follow their passions and achieve great success both in their careers and in life.
- Start building a CS curriculum using the Microsoft Computer Science Curriculum Toolkit .
- To inspire a STEM passion in K-12 learners and teach them how to code with purpose, use Minecraft’s Computer Science Progression .
- Find out how the Microsoft TEALS Program can help you create access to equitable, inclusive CS education and learn more about building inclusive economic growth .
- Enlist one of Microsoft's Global Training Partners to support your educators to incorporate CS into their curriculum and teaching practices.
i https://www.imf.org/en/Publications/fandd/issues/2019/03/global-competition-for-technology-workers-costa
ii https://codeorg.medium.com/cs-helps-students-outperform-in-school-college-and-workplace-66dd64a69536
iii Can Majoring in CS Improve General Problem-solving Skills?, ACM, Salehi et al., 2020
iv The effects of coding on children's planning and inhibition skills, Computers & Education, Arfé et al., 2020
v http://www.westcoastanalytics.com/uploads/6/9/6/7/69675515/longitudinal_study_-_combined_report_final_3_10_20__jgq_.pdf
vi https://www.hereford.ac.uk/explore-courses/courses/computer-science/
vii https://www.thebalancemoney.com/average-salary-information-for-us-workers-2060808
viii Kotsopoulos, D., Floyd, L., Khan, S., Namukasa, I.K., Somanath, S., Weber, J. & Yiu, C. (2017) A Pedagogical Framework for Computational Thinking. Digital Experiences in Mathematics Education vol. 3, pages 154–171(2017)
ix https://www.theguardian.com/careers/2021/jun/28/why-arent-more-girls-in-the-uk-choosing-to-study-computing-and-technology
Related stories
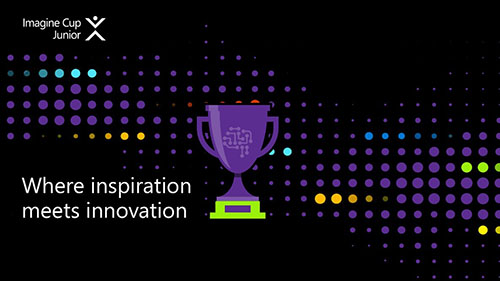
The fifth annual Imagine Cup Junior for students is now live!
Today we’re thrilled to launch the fifth annual Microsoft Imagine Cup Junior (ICJ) global student competition. Registration and content are now available for educators to help students learn about AI technology and how it can be used to solve some of the world’s biggest challenges.
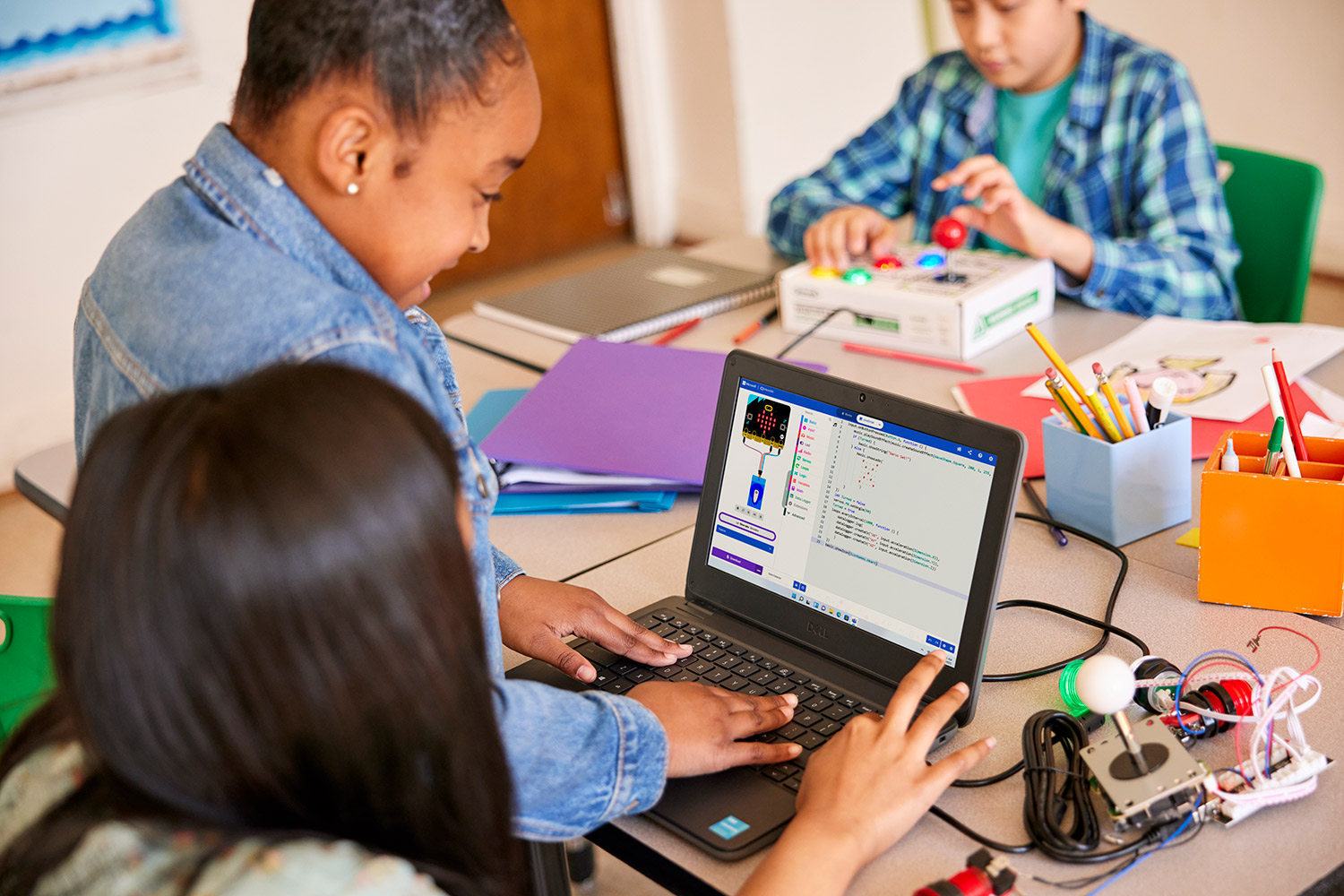
Gearing up for Computer Science Education Week
It’s the most wonderful time of the year: Computer Science Education Week! All year round, but especially December 4–10, 2023, we aim to inspire students to engage in computer science with opportunities to learn about AI, advocate for equity in digital careers, and become responsible coders.
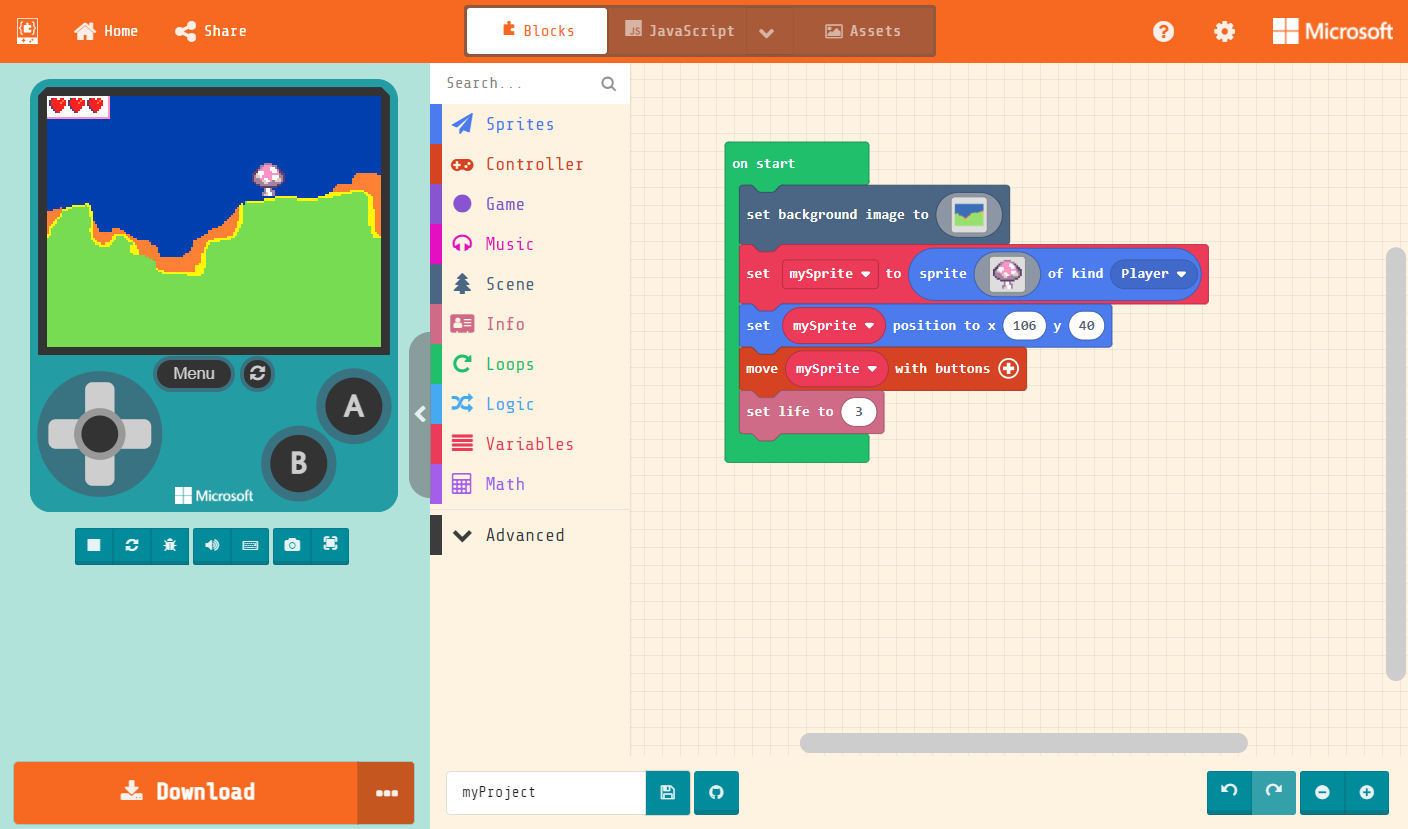
Announcing AP Computer Science Principles with Microsoft MakeCode
In partnership with the United States College Board organization, whose mission is to expand access to higher education for every student, Microsoft is proud to announce a free new curriculum resource for high school educators who teach the College Board’s Advanced Placement Computer Science Principles course.
- SCHOOL STORIES
- MICROSOFT EDUCATOR CENTER
- CONTACT SALES
December 22, 2023
The Most Important Unsolved Problem in Computer Science
Here’s a look at the $1-million math problem at the heart of computation
By Jack Murtagh
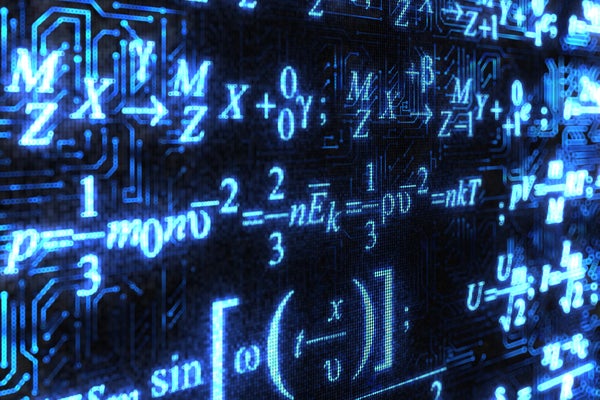
alengo/Getty Images
When the Clay Mathematics Institute put individual $1-million prize bounties on seven unsolved mathematical problems , they may have undervalued one entry—by a lot. If mathematicians were to resolve, in the right way, computer science's “P versus NP” question, the result could be worth worlds more than $1 million. They'd be cracking most online-security systems, revolutionizing science and even, in effect, solving the other six of the so-called Millennium Problems, all of which were chosen in the year 2000. It's hard to overstate the stakes surrounding the most important unsolved problem in computer science .
P versus NP concerns the apparent asymmetry between finding solutions to problems and verifying solutions to problems. For example, imagine you're planning a world tour to promote your new book. You pull up Priceline and start testing routes, but each one you try blows your total trip budget. Unfortunately, as the number of cities grows on your worldwide tour, the number of possible routes to check skyrockets exponentially, making it infeasible even for computers to exhaustively search through every case. But when you complain, your book agent writes back with a solution sequence of flights. You can easily verify whether their route stays in budget by simply checking that it hits every city and summing the fares to compare against the budget limit. Notice the asymmetry here: finding a solution is hard, but verifying a solution is easy.
The P versus NP question asks whether this asymmetry is real or an illusion. If you can efficiently verify a solution to a problem, does that mean you can also efficiently find a solution? It might seem obvious that finding a solution should be harder than verifying one. But researchers have been surprised before. Problems can look similarly difficult—but when you dig deeper you find shortcuts to some and hit brick walls on others. Perhaps a clever shortcut can circumvent searching through zillions of potential routes in the book tour problem. For example, if you instead wanted to find a sequence of flights between two specific remote airports while abiding by the budget, you might also throw up your hands at the immense number of possible routes to check. In fact, this problem contains enough structure that computer scientists have developed a fast procedure (or algorithm) for it that bypasses the need for an exhaustive search.
On supporting science journalism
If you're enjoying this article, consider supporting our award-winning journalism by subscribing . By purchasing a subscription you are helping to ensure the future of impactful stories about the discoveries and ideas shaping our world today.
The P versus NP question rears its head everywhere we look in the computational world well beyond the specifics of our travel scenario—so much so that it has come to symbolize a holy grail in our understanding of computation. Yet every attempt to resolve it only further exposes how monumentally difficult it is to prove one way or another.
In the subfield of theoretical computer science called complexity theory, researchers try to pin down how easily computers can solve various types of problems. P represents the class of problems they can solve efficiently, such as sorting a column of numbers in a spreadsheet or finding the shortest path between two addresses on a map. In contrast, NP represents the class of problems for which computers can verify solutions efficiently. Our book tour problem, which academics call the Traveling Salesperson Problem , lives in NP because we have an efficient procedure for verifying that the agent's solution worked.
Notice that NP actually contains P as a subset because solving a problem outright is one way to verify a solution to it. For example, how would you verify that 27 × 89 = 2,403? You would solve the multiplication problem yourself and check that your answer matches the claimed one. We typically depict the relation between P and NP with a simple Venn diagram:
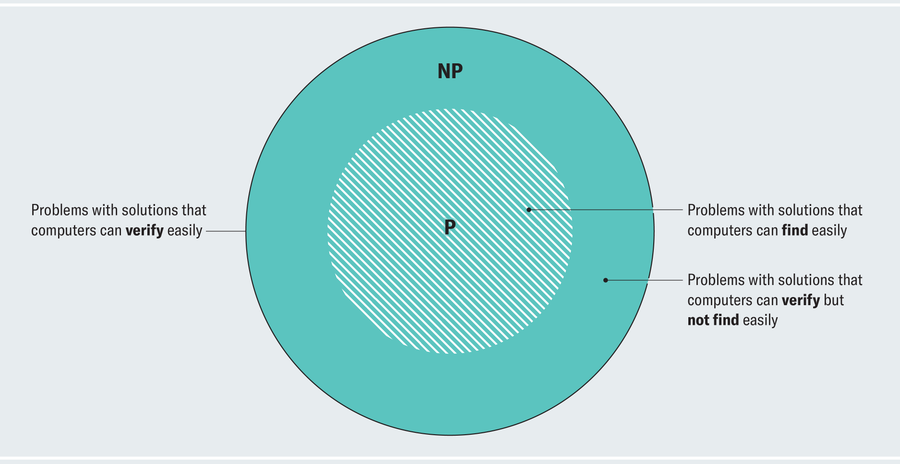
Credit: Amanda Montañez
The region inside of NP but not inside of P contains problems that can't be solved with any known efficient algorithm. (Theoretical computer scientists use a technical definition for “efficient” that can be debated, but it serves as a useful proxy for the colloquial concept.) But we don't know whether that's because such algorithms don't exist or we just haven't mustered the ingenuity to discover them. This representation provides another way to phrase the P versus NP question: Are these classes actually distinct? Or does the Venn diagram collapse into one circle? Can all NP problems be solved efficiently?
Here are some examples of problems in NP that are not currently known to be in P:
Given a social network, is there a group of a specified size in which all of the people in it are friends with one another?
Given a varied collection of boxes to be shipped, can all of them be fit into a specified number of trucks?
Given a sudoku (generalized to n × n puzzle grids), does it have a solution?
Given a map, can the countries be colored with only three colors such that no two neighboring countries are the same color?
Ask yourself how you would verify proposed solutions to some of the problems listed and then how you would find a solution. Note that approximating a solution or solving a small instance (most of us can solve a 9 × 9 sudoku ) doesn't suffice. To qualify as solving a problem, an algorithm needs to find an exact solution for all instances, including very large ones.
Each of the problems can be solved via brute-force search (for example, try every possible coloring of the map and see whether any of them work), but the number of cases to try grows exponentially with the size of the problem. This means that if we call the size of the problem n (for example, the number of countries on the map or the number of boxes to pack into trucks), then the number of cases to check looks something like 2 n . The world's fastest supercomputers have no hope against exponential growth. Even when n equals 300, a tiny input size by modern data standards, 2 300 exceeds the number of atoms in the observable universe. After hitting “go” on such an algorithm, your computer would display a spinning pinwheel that would outlive you and your descendants.
Thousands of other problems belong on our list. From cell biology to game theory, the P versus NP question reaches into far corners of science and industry. If P = NP (that is, our Venn diagram dissolves into a single circle, and we obtain fast algorithms for these seemingly hard problems), then the entire digital economy would become vulnerable to collapse. This is because much of the cryptography that secures such things as your credit card number and passwords works by shrouding private information behind computationally difficult problems that can become easy to solve only if you know the secret key. Online security as we know it rests on unproven mathematical assumptions that crumble if P = NP.
Amazingly, we can even cast mathematics itself as an NP problem because we can program computers to efficiently verify proofs. In fact, legendary mathematician Kurt Gödel first posed the P versus NP problem in a letter to his colleague John von Neumann in 1956. Gödel observed that P = NP “would have consequences of the greatest importance. Namely, it would obviously mean that ... the mental work of a mathematician concerning yes-or-no questions could be completely replaced by a machine.”
If you're a mathematician worried for your job, rest assured that most experts believe that P does not equal NP. Aside from the intuition that sometimes solutions should be harder to find than to verify, thousands of the hardest NP problems that are not known to be in P have sat unsolved across disparate fields, glowing with incentives of fame and fortune, and yet not one person has designed an efficient algorithm for a single one of them.
Of course, gut feeling and a lack of counterexamples don't constitute a proof. To prove that P is different from NP, you somehow have to rule out all potential algorithms for all of the hardest NP problems, a task that appears out of reach for current mathematical techniques. Indeed, the field has coped by proving so-called barrier theorems, which say that entire categories of tempting proof strategies to resolve P versus NP cannot succeed. Not only have we failed to find a proof, but we also have no clue what an eventual proof might look like.
Advertisement
Computational Thinking and Metacognition
- Original Paper
- Published: 05 February 2022
- Volume 66 , pages 405–411, ( 2022 )
Cite this article
- Aman Yadav ORCID: orcid.org/0000-0003-4247-2033 1 ,
- Ceren Ocak 1 &
- Amber Oliver 2
2584 Accesses
12 Citations
18 Altmetric
Explore all metrics
Since Wing Communications of the ACM , 49 , 33–35, ( 2006 ) popularized computational thinking (CT) as a skill for every student, it has gained significant traction as an approach to bring computer science tools and practices into K-12 classrooms. At the same time, teachers often see the relevance of CT as a tool to introduce problem solving and thinking strategies in the classroom. Despite the increasing use of CT in K-12, questions remain about its role in supporting teaching and learning of disciplinary ideas. While CT can be used to bring computer science to all students, we believe that it can serve a bigger purpose to explicitly teach metacognitive strategies, which play a significant role in academic outcomes. In the paper, we discuss the connections between CT and metacognition and how CT could support the development of metacognition in K-12 classrooms.
This is a preview of subscription content, log in via an institution to check access.
Access this article
Price includes VAT (Russian Federation)
Instant access to the full article PDF.
Rent this article via DeepDyve
Institutional subscriptions
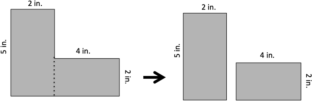
Similar content being viewed by others
Knowing the Mind
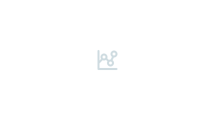
Metacognitive Theories Revisited
David Moshman
Computational Thinking, Grade 1 Students and the Binomial Theorem
George Gadanidis, Janette M. Hughes, … Bethany J. G. White
https://ct4edu.org/
Annevirta, T., & Vauras, M. (2006). Developmental changes of metacognitive skill in elementary school children. The Journal of Experimental Education, 74 (3), 195–226. https://doi.org/10.3200/JEXE.74.3.195-226
Article Google Scholar
Azeka, S., & Yadav, A. (2021). A computational thinking integration model for primary and secondary classrooms. In A. Yadav & U. D. Berthelsen (Eds.), Computational thinking in education: A pedagogical perspective (pp. 41–56). Routledge. https://doi.org/10.4324/978100310299
Chapter Google Scholar
Barr, D., Harrison, J., & Conery, L. (2011). Computational thinking: A digital age. Learning and Leading with Technology, 5191 (March/April), 20–23.
Google Scholar
Barr, V., & Stephenson, C. (2011). Bringing computational thinking to K-12: What is involved and what is the role of the computer science education community? ACM Inroads, 2 (1), 48–54. https://doi.org/10.1145/1929887.1929905
Berardi-Coletta, B., Buyer, L. S., Dominowski, R. L., & Rellinger, E. R. (1995). Metacognition and problem solving: A process-oriented approach. Journal of Experimental Psychology. Learning, Memory, and Cognition . https://doi.org/10.1037/0278-7393.21.1.205
Berland, M., & Wilensky, U. (2015). Comparing virtual and physical robotics environments for supporting complex systems and computational thinking. Journal of Science Education and Technology, 24 (5), 628–647. https://doi.org/10.1007/s10956-015-9552-x
Boulware-Gooden, R., Carreker, S., Thornhill, A., & Joshi, R. M. (2007). Instruction of metacognitive strategies enhances reading comprehension and vocabulary achievement of third-grade students. The Reading Teacher, 61 (1), 70–77. https://doi.org/10.1598/RT.61.1.7
Caeli, E. N., & Yadav, A. (2020). Unplugged approaches to computational thinking: A historical perspective. TechTrends, 64 (1), 29–36. https://doi.org/10.1007/s11528-019-00410-5
Clements, D. H. (1986). Logo and cognition: A theoretical foundation. Computers in Human Behavior, 2 (2), 95–110. https://doi.org/10.1016/0747-5632(86)90026-9
Cox, M. T. (2005). Metacognition in computation: A selected research review. Artificial Intelligence, 169 (2), 104–141. https://doi.org/10.1016/j.artint.2005.10.009
Csizmadia, A., Curzon, P., Dorling M., Humphreys, S., Ng, T., Selby, C., & Woollard, J. (2015). Computational thinking: A guide for teachers. (Available at: http://community.Computingatschool.org.uk/resources/2324 ). Unpublished report
Davidson, J., Deuser, R., & Sternberg, R. J. (1994). The role of metacognition in problem solving. In J. Metcalfe & A. P. Shimamura (Eds.), Metacognition: Knowing about knowing . MIT Press.
Denning, P. J. (2017). Remaining trouble spots with computational thinking. Communications of the ACM, 60 (6), 33–39. https://doi.org/10.1145/2998438
Denning, P. J., & Tedre, M. (2021). Computational thinking: A disciplinary perspective . Informatics in Education.
Desoete, A., Roeyers, H., & Buysse, A. (2001). Metacognition and mathematical problem solving in grade 3. Journal of Learning Disabilities, 34 (5), 435–447.
Flavell, J. H. (1979). Metacognition and cognitive monitoring: A new area of cognitive–developmental inquiry. The American Psychologist, 34 (10), 906. https://doi.org/10.1007/s40692-017-0090-9
Grover, S. (2018). A Tale of Two CTs (and a Revised Timeline for Computational Thinking) | blog@CACM | Communications of the ACM, (Part 2), 2–5. Retrieved from https://cacm.acm.org/blogs/blog-cacm/232488-a-tale-of-two-cts-and-a-revised-timeline-for-computational-thinking/fulltext
Hartman, H. J. (1998). Metacognition in teaching and learning: An introduction. Instructional Science , 1–3.
Haseski, H. I., Ilic, U., & Tugtekin, U. (2018). Defining a new 21st century skill-computational thinking: Concepts and trends. International Education Studies, 11 (4), 29–42.
Jacobse, A. E., & Harskamp, E. G. (2009). Student-controlled metacognitive training for solving word problems in primary school mathematics. Educational Research and Evaluation, 15 (5), 447–463. https://doi.org/10.1080/13803610903444519
Kuo, W. C., & Hsu, T. C. (2020). Learning computational thinking without a computer: How computational participation happens in a computational thinking board game. The Asia-Pacific Education Researcher, 29 (1), 67–83. https://doi.org/10.1007/s40299-019-00479-9
Livingston, J. A. (2003). Metacognition: An overview. Psychology, 13 , 259–266.
Martinez, M. E. (2006). What is metacognition? Phi Delta Kappan, 87 (9), 696–699.
Mayer, R. E. (1998). Cognitive, metacognitive, and motivational aspects of problem solving. Instructional Science, 26 (1), 49–63.
Papert, S. (1990). Mindstorms: Computers, children, and learning . Basic Books.
Resnick, M., Martin, F., Sargent, R., & Silverman, B. (1996). Programmable bricks: Toys to think with. IBM Systems Journal, 35 (3.4), 443–452. https://doi.org/10.1147/sj.353.0443
Rich, K. M., & Yadav, A. (2020). Applying levels of abstraction to mathematics word problems. TechTrends, 1-9 . https://doi.org/10.1007/s11528-020-00479-3
Rich, K. M., Yadav, A., & Zhu, M. (2019). Levels of abstraction in students’ mathematics strategies: What can applying computer science ideas about abstraction bring to elementary mathematics? Journal of Computers in Mathematics and Science Teaching, 38 (3), 267–298.
Rich, K. M., Yadav, A., & Larimore, R. A. (2020). Teacher implementation profiles for integrating computational thinking into elementary mathematics and science instruction. Education and Information Technologies, 25 (4) , 3161–3188.
Rivers, D. J., Vallance, M., & Nakamura, M. (2021). Metacognitive knowledge and the self as socially distanced online learner: A virtual reality assisted analysis of academic self-concept. Journal of Educational Technology Systems, 50 (1), 87–111. https://doi.org/10.1177/0047239521999779
Rodrigues, R. S., Andrade, W. L., & Campos, L. M. R. S. (2016). Can computational thinking help me? A quantitative study of its effects on education (pp. 1–8). IEEE Frontiers in Education Conference (FIE). https://doi.org/10.1109/FIE.2016.7757409
Book Google Scholar
Scherer, R., Siddiq, F., & Sánchez Viveros, B. (2019). The cognitive benefits of learning computer programming: A meta-analysis of transfer effects. Journal of Education & Psychology, 111 (5), 764. https://doi.org/10.1037/edu0000314
Schoenfeld, A. H. (1979). Explicit heuristic training as a variable in problem-solving performance. Journal for Research in Mathematics Education, 10 , 173–187.
Schoenfeld, A. H. (1985). Making sense of “out loud” problem solving protocols. The Journal of Mathematical Behavior , 4(2), 171-191
Schoenfeld, A. (1987). What's all the fuss about metacognition? In A. Schoenfeld (Ed.), Cognitive science and mathematics education (pp. 334–370). Erlbaum.
Schraw, G. (1998). Promoting general metacognitive awareness. Instructional Science, 26 (1), 113–125.
Selby, C. C., & Woollard, J. (2013). Computational thinking: The developing definition. In Paper presented at the 18th annual conference on innovation and Technology in Computer Science Education , Canterbury.
Shea, N. A., & Duncan, R. G. (2013). From theory to data: The process of refining learning progressions. The Journal of the Learning Sciences, 22 (1), 7–32. https://doi.org/10.1080/10508406.2012.691924
Shute, V. J., Sun, C., & Asbell-Clarke, J. (2017). Demystifying computational thinking. Educational Research Review, 22 , 142–158. https://doi.org/10.1016/j.edurev.2017.09.003
Sternberg, R. J. (1980). Sketch of a componential subtheory of human intelligence. The Behavioral and Brain Sciences, 3 , 573–584.
Sung, W., Ahn, J., & Black, J. B. (2017). Introducing computational thinking to young learners: Practicing computational perspectives through embodiment in mathematics education. Technology, Knowledge and Learning, 22 (3), 443–463. https://doi.org/10.1007/s10758-017-9328-x
Swanson, H. L. (1990). Influence of metacognitive knowledge and aptitude on problem solving. Journal of Education & Psychology, 82 (2), 306.
Tedre, M., & Denning, P. J. (2016). The long quest for computational thinking. In In proceedings of the 16th Koli calling conference on computing education research (pp. 120–129). Koli. https://doi.org/10.1145/2999541.2999542
Teong, S. K. (2003). The effect of metacognitive training on mathematical word-problem solving. Journal of Computer Assisted Learning, 19 (1), 46–55.
Veenman, M. V., Van Hout-Wolters, B. H., & Afflerbach, P. (2006). Metacognition and learning: Conceptual and methodological considerations. Metacognition and Learning, 1 (1), 3–14. https://doi.org/10.1007/s11409-006-6893-0
Veenman, M. V., Wilhelm, P., & Beishuizen, J. J. (2004). The relation between intellectual and metacognitive skills from a developmental perspective. Learning and Instruction, 14 (1), 89–109. https://doi.org/10.1016/j.learninstruc.2003.10.004
Vos, N., van der Meijden, H., & Denessen, E. (2011). Effects of constructing versus playing an educational game on student motivation and deep learning strategy use. Computers in Education, 56 , 127–137.
Weinert, F. E. (1987). Introduction and overview: Metacognition and motivation as determinants of effective learning and understanding. In Metacognition, motivation, and understanding (pp. 1–16). Erlbaum.
Wilson, J., & Clarke, D. (2004). Towards the modelling of mathematical metacognition. Mathematics Education Research Journal, 16 (2), 25–48.
Wing, J. M. (2006). Computational thinking. Communications of the ACM, 49 (3), 33–35.
Wing, J. (2011). Research notebook: Computational thinking – What and why? In The Link Magazine . Carnegie Mellon University.
Yadav, A., Hong, H., & Stephenson, C. (2016). Computational thinking for all: Pedagogical approaches to embedding 21st century problem solving in K-12 classrooms. TechTrends, 60 (6), 565–568. https://doi.org/10.1007/s11528-016-0087-7
Yadav, A., Rich, K., Schwarz, C., & Larimore, R. (in press). Developing elementary teachers’ competencies in integrating computational thinking ideas in classrooms: Using a toolkit as a scaffold. In C. Mouza, A. Leftwich, & A. Yadav (Eds.), Professional development for in-service teachers: Research and practices in computing education . Information Age.
Zamansky, M., Oliver, A., & Yadav, A. (2019). How an unplugged approach to computational thinking can move schools to computer science. EdSurge News. Retrieved 1 February, 2022, from https://www.edsurge.com/news/2019-12-19-how-an-unplugged-approach-to-computational-thinking-can-move-schools-to-computer-science
Download references
Author information
Authors and affiliations.
Michigan State University, East Lansing, MI, USA
Aman Yadav & Ceren Ocak
Robin Hood Learning + Technology Fund, New York City, NY, USA
Amber Oliver
You can also search for this author in PubMed Google Scholar
Corresponding author
Correspondence to Aman Yadav .
Additional information
Publisher’s note.
Springer Nature remains neutral with regard to jurisdictional claims in published maps and institutional affiliations.
Rights and permissions
Reprints and permissions
About this article
Yadav, A., Ocak, C. & Oliver, A. Computational Thinking and Metacognition. TechTrends 66 , 405–411 (2022). https://doi.org/10.1007/s11528-022-00695-z
Download citation
Accepted : 10 January 2022
Published : 05 February 2022
Issue Date : May 2022
DOI : https://doi.org/10.1007/s11528-022-00695-z
Share this article
Anyone you share the following link with will be able to read this content:
Sorry, a shareable link is not currently available for this article.
Provided by the Springer Nature SharedIt content-sharing initiative
- Computational thinking
- Metacognition
- Metacognitive strategies
- Problem-solving
- Find a journal
- Publish with us
- Track your research
- Skip to primary navigation
- Skip to main content
- Skip to footer
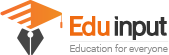
Eduinput- Online tutoring platform for Math, chemistry, Biology Physics
An online learning platform for Mcat, JEE, NEET and UPSC students
Problem Solving Techniques in Computer Science

Problem-solving is the process of identifying a problem and finding the best solution for it. Problem-solving is a technique that can be developed by following a well-organized approach. Every day we encounter many problems and solve them.
Every problem is different. Some problems are very difficult and are needed more attention to recognize the solution.
A problem may be solved by multiple methods. One solution may be faster, cheaper, and more reliable than others. It is important to choose a suitable worthy solution.
Different strategies, techniques, and tools are used to solve a problem. Computers are used as a tool to solve complex problems by developing computer programs.
Computer programs contain different instructions for computers. A programmer writes instructions and the computer executes these instructions to solve a problem. A person can be a good programmer if he has the skill of solving problems.
Table of Contents
Problem-Solving Techniques.
There are three different types of problem-solving techniques.
A set of instructions given to a computer to solve a problem is called a program.
A computer works according to the given instructions in the program. Computer programs are written in programming languages. A person who develops a program is called a programmer.
The programmer develops programs to instruct the computer on how to process data into information. The programmer uses programming languages or tools to write programs.
Advantages of Computer Program
Different advantages of computer programs are as follows:
- A computer program can solve many problems by giving instructions to the computer.
- A computer program can be used to perform a task again and again and fastly.
- A program can process a large amount of data easily.
- It can display the results in different styles.
- The processing of a program is more efficient and less time-consuming.
- Different types of programs are used in different fields to perform certain tasks.
Algorithms & Pseudo Code
An algorithm is a step-by-step procedure to solve a problem. The process of solving
problem becomes simpler and easier with help of algorithm. It is better to write an algorithm
before writing the actual computer program.
Properties of Algorithm
Following are some properties of an algorithm:
- The given problem should be broken down into simple and meaningful steps.
- The steps should be numbered sequentially.
- The steps should be descriptive and written in simple English.
Algorithms are written in a language that is similar to simple English called pseudocode. There is no standard to write pseudo code. It is used to specify program logic in an English-like manner that is independent of any particular programming language.
Pseudocode simplifies program development by separating it into two main parts.
Logic Design
In this part, the logic of the program is designed. We specify different steps required to solve the problem and the sequence of these steps.
In this part, the algorithm is converted into a program. The steps of the algorithm are
translated into instructions of any programming language.
The use of pseudo-code allows the programmer to focus on the planning of the program. After the planning is final, it can be written in any programming language.
The following algorithm inputs two numbers calculate the sum and then displays the result on the screen.
4. Total A+B
5. Display Total
The following algorithm inputs the radius from the user and calculates the area of a circle.
Hint: Area 3.14* radius* radius)
2. Input radius in r
3. area = 3.14* r* r
4. Print area
Advantages of Algorithm
There are many advantages of an algorithm
Reduce complexity
Writing algorithm and program separately simplifies the overall task by dividing it into two simpler tasks. While writing the algorithm, we can focus on solving the problem instead of concentrating on a particular language.
Increased Flexibility
An algorithm is written so that the code may be written in any language. Using an algorithm, the program could be written in Visual Basic, Java or C++, etc.
Ease of Understanding
It is not necessary to understand a particular programming language to understand an algorithm. It is written in an English-like manner.
A flowchart is a combination of two words flow and chart. A chart consists of different symbols to display information about any program. Flow indicates the direction processing that takes place in the program.
Flowchart is a graphical representation of an algorithm. It is a way of visually presenting the flow of data, operations performed on data, and the sequence of these operations.
Flowchart is similar to the layout plan of a building. A designer draws the layout plan of the building before constructing it. Similarly, a programmer prefers to design the flowchart before writing the computer program. Flowchart is designed according to the defined rule.
Uses of Logic Flowchart
Flowchart is used for the following reasons
- Flowchart is used to represent an algorithm in a simple graphical manner.
- Flowchart is used to show the steps of an algorithm easily.
- Flowchart is used to understand the flow of the program.
- Flowchart is used to improve the logic for solving a problem.
- Programs can be reviewed and debugged easily.
- Chapter-Getting Started with C
Related Articles
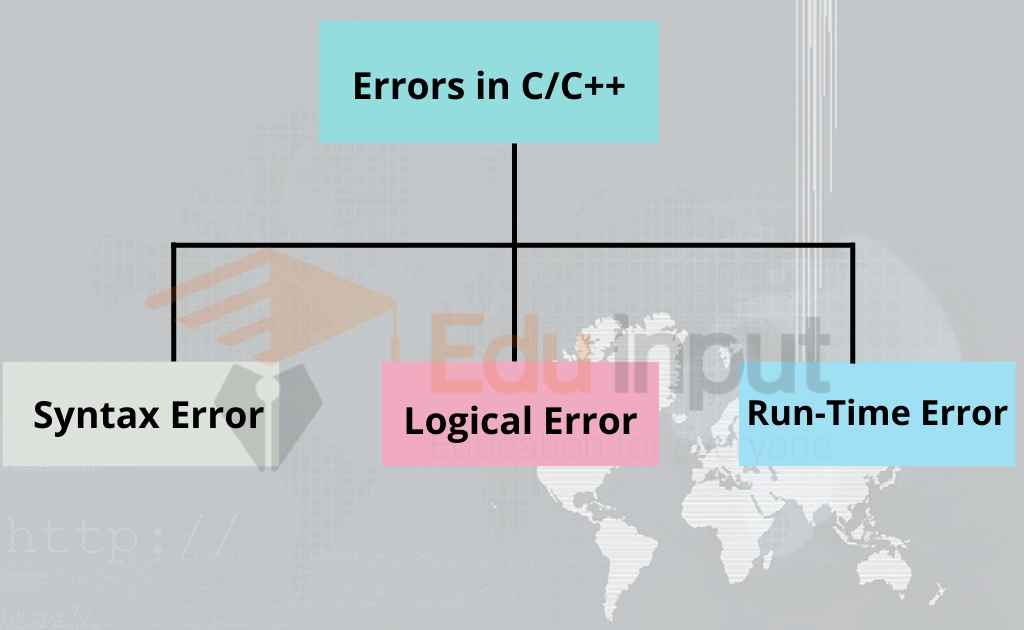
Debugging in Turbo C++ | Different types of Error in C/ C++
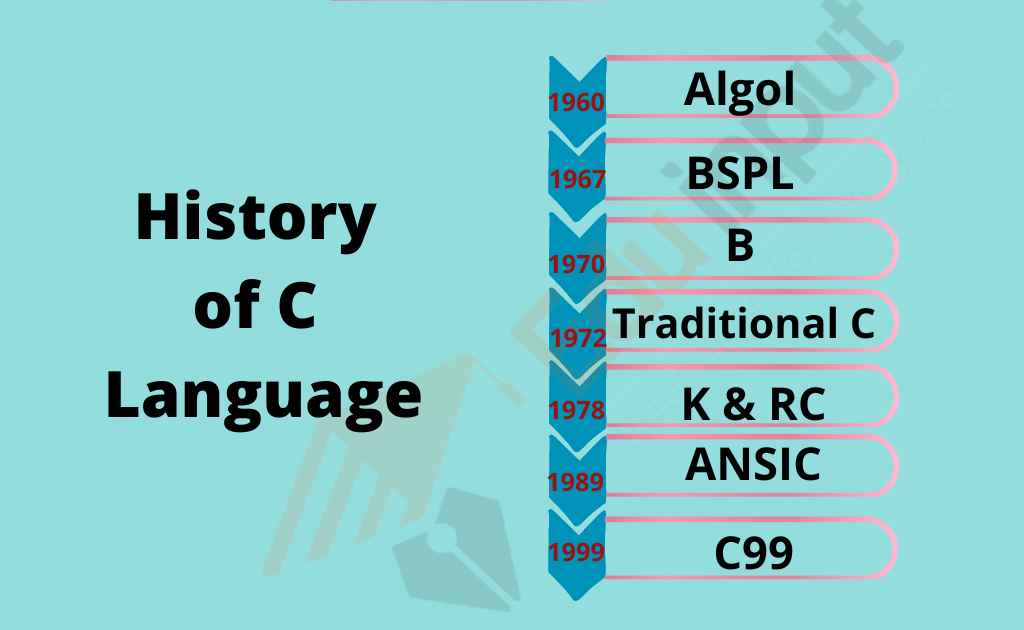
History of C Language – Advantages and Disadvantages of C Language
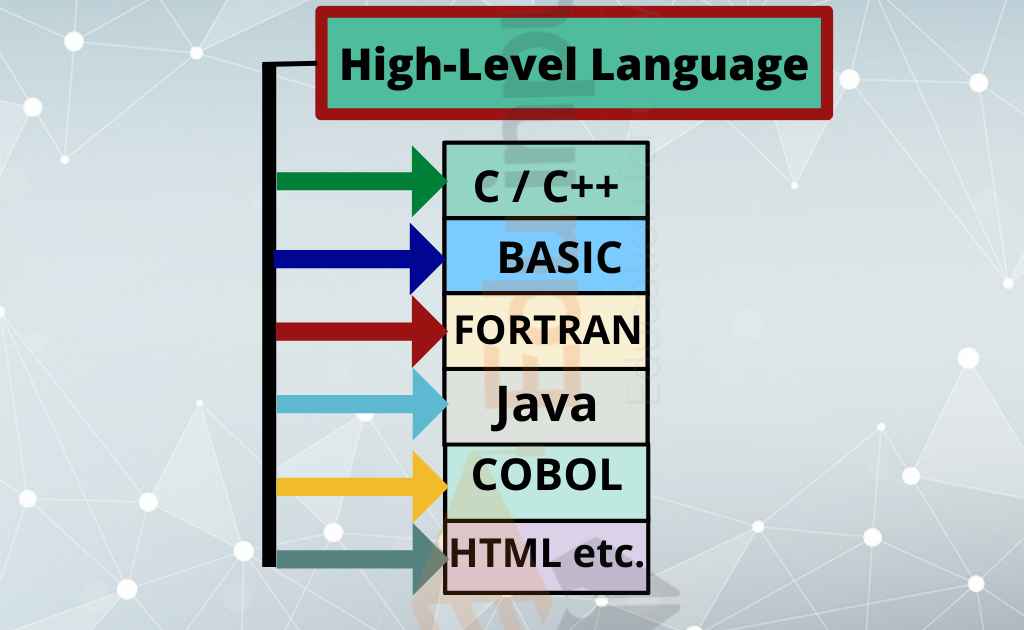
High-Level Programming Language-Popular High-level languages
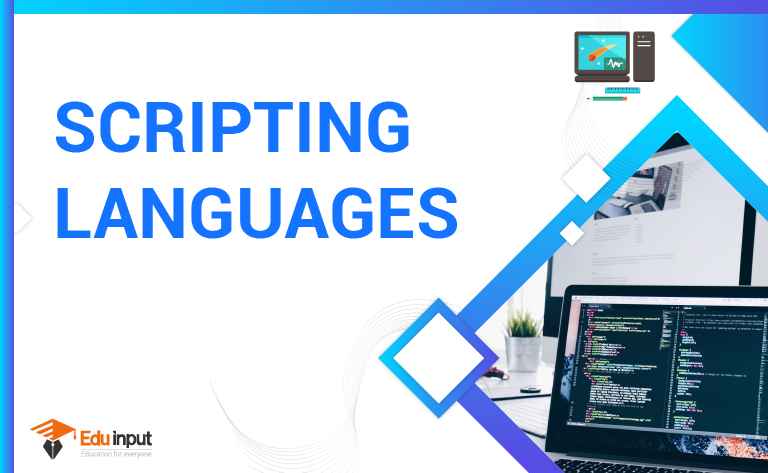
Scripting Languages-Different Types of Scripting Languages
Leave a reply cancel reply.
Your email address will not be published. Required fields are marked *
Save my name, email, and website in this browser for the next time I comment.
Get updates about new courses
NCERT solutions
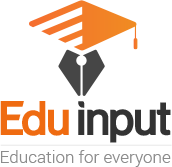
9th Class 10th Class 11 Class 12 Class
Join the groups below to get updates.
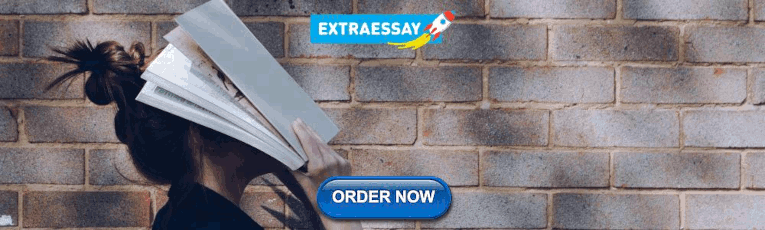
IMAGES
VIDEO
COMMENTS
Problem solving, in the simplest terms, is the process of identifying a problem, analyzing it, and finding the most effective solution to overcome it. For software engineers, this process is deeply embedded in their daily workflow. It could be something as simple as figuring out why a piece of code isn't working as expected, or something as ...
Understanding the Importance. In computer science, problem solving is a crucial skill that is at the core of the problem solving cycle. The problem solving cycle is a systematic approach to analyzing and solving problems, involving various stages such as problem identification, analysis, algorithm design, implementation, and evaluation.
Computer science learners often face difficulties in performing two of the main stages of a problem-solving process: problem analysis and solution construction. Therefore, it is important that computer science educators be aware of these difficulties and acquire appropriate pedagogical tools to guide and scaffold learners in learning these skills.
The world of computer science is a fascinating realm, where intricate concepts and technologies continuously shape the way we interact with machines. ... In this article, we aim to provide a clear and accessible introduction to algorithms, focusing on their importance in problem-solving and exploring common types such as searching, sorting, and ...
COMP1405/1005 - An Introduction to Computer Science and Problem Solving Fall 2011 - 4- There are also other types of programming languages such as functional programming languages and logic programming languages. According to the Tiobe index (i.e., a good site for ranking the popularity of programming languages), as of February 2011 the 10 most
In the high performers' problem-solving processes, in contrast, distinct fundamental ideas of computer science as described, for example, in Schubert and Schwill ( 2004) were visible. In the specific context, these were tree structures and recursion, which in combination with the key findings of the analysis phase were purposefully applied.
Solving problems is the core of computer science. Programmers must first understand how a human solves a problem, then understand how to translate this "algorithm" into something a computer can do, and finally how to "write" the specific syntax (required by a computer) to get the job done. It is sometimes the case that a machine will solve a ...
Please be advised that external sites may have terms and conditions, including license rights, that differ from ours. MIT OCW is not responsible for any content on third party sites, nor does a link suggest an endorsement of those sites and/or their content.
Emphasis on problem-solving techniques that aid programmers and computer scientists. Heuristics for solving problems ''in the small'' (classical math and word problems), generating potential solutions to ''real-life'' problems encountered in the profession, and problem solving in teams. Having successfully completed this course, the student ...
Computational thinking is a problem-solving process in which the last step is expressing the solution so that it can be executed on a computer. However, before we are able to write a program to implement an algorithm, we must understand what the computer is capable of doing -- in particular, how it executes instructions and how it uses data.
Below, we explore the top "soft skills" that computer scientists should obtain. Critical Thinking: Being able to identify a problem, analyze the details of the situation, and then formulate an effective solution is an incredibly important aspect of computer science work. Attention to Detail: Effective computer scientists must be able to pay ...
Introduction. In order for students to become "computational thinkers" they need experience solving a wide range of problems and the opportunity to experiment with a variety of solution strategies. This unit begins with an introduction to the problem solving process. Students are asked to solve new problems by planning a strategy, designing ...
ABSTRACT. Background: Recent developments in STEM and computer science education put a strong emphasis on twenty-first-century skills, such as solving authentic problems. These skills typically transcend single disciplines. Thus, problem-solving must be seen as a multidisciplinary challenge, and the corresponding practices and processes need to be described using an integrated framework.
One of the most important skills you learn in your computer science courses is how to problem solve. Although we cover some general problem solving paradigms in class, the best way to improve these skills is to get practice, practice, and more practice. ... Solving the right problem is the most important part of problem solving. Be sure ...
ing is transferable to problem-solving tasks across domains and discuss how the unique features of CS programming assignments could be generalized to other science and engineering courses to foster students' general problem-solving skills. KEYWORDS computational thinking, problem solving, simulations, computer science education, student ...
Apply Creative Problem Solving to Other Areas. Let's start by explaining why the creative problem-solving skills you'll learn in computer science can help you in everyday life: Novel solutions and new products. Being familiar with creating and polishing hardware and/or software can help you come up with ingenious solutions for your everyday ...
The purpose of computational thinking is to be able to solve complex problems in a structured, effective and repeatable way. Computational thinking, while drawing on principles from computer science and mathematics, can be applied not only to mathematical or technology-related problems, but to real-world problems as well.
The analysis suggests that the educational experiences of Computer Science (CS) students may better train them in problem-solving than the experiences of other majors. In this study, students from a variety of undergraduate majors and grade levels were given a 15-minute problem-solving task embedded in an interactive science simulation.
Computer science is the study of problems, problem-solving, and the solutions that come out of the problem-solving process. Given a problem, a computer scientist's goal is to develop an algorithm, a step-by-step list of instructions for solving any instance of the problem that might arise.Algorithms are finite processes that if followed will solve the problem.
A growing interest in CS curricula. Learning coding and software development, two key parts of CS, has been shown to improve students' creativity, critical thinking, math, and reasoning skills ii. CS skills like problem-solving iii and planning iv are transferable and can be applied across other subjects. A 2020 study examining the effects of ...
They'd be cracking most online-security systems, revolutionizing science and even, in effect, solving the other six of the so-called Millennium Problems, all of which were chosen in the year 2000 ...
From a CT perspective, this could also align with abstraction, which is an important CT practice and central to a problem-solving approach in computer science. Abstraction in this case involves focusing on the most relevant and essential details of the problem, such as using a subway map that ignores details of a city and just shows the train ...
Problem-solving is the process of identifying a problem and finding the best solution for it. Problem-solving is a technique that can be developed by ... Problem Solving Techniques in Computer Science. July 21, 2022. ... cheaper, and more reliable than others. It is important to choose a suitable worthy solution. Different strategies ...
However, computer science poses inherent complexity, demanding abstract thinking and diverse problem-solving methodologies. Computational thinking (CT) emerges as a promising approach to address these challenges, recognized as a vital skillset for fostering innovation in digital technology among students.