ORIGINAL RESEARCH article
Online consumer satisfaction during covid-19: perspective of a developing country.

- 1 Antai College of Economics & Management, Shanghai Jiao Tong University, Shanghai, China
- 2 School of Management, Zhejiang Shuren University, Hangzhou, China
- 3 Faculty of Management Sciences, Riphah International University, Faisalabad Campus, Punjab, Pakistan
- 4 Department of Professional Psychology, Bahria University, Islamabad, Pakistan
A conceptual model based on the antecedents and consequences of online consumer satisfaction has been proposed and empirically proved in this study. Data were collected during Smart Lockdown of COVID-19 from 800 respondents to observe the difference between perceived and actual, and direct and indirect e-stores. Confirmatory factor analysis was used to observe the validity of the data set. The structural equation modeling technique was used to test the hypotheses. The findings indicated that consumers feel more satisfied when they shop through direct e-store than indirect e-store, whereas their perception and actual experience are different. Implications have also been added to the study.
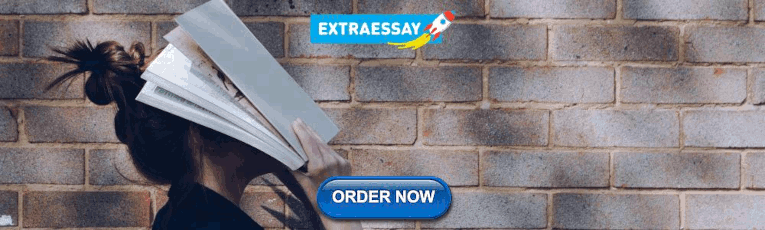
Introduction
Online shopping is the act of buying a product or service through any e-stores with the help of any website or app. Tarhini et al. (2021) stated that shopping through online channels is actively progressing due to the opportunity to save time and effort. Furthermore, online shopping varies from direct e-store and indirect e-store about their perception against the actual experience. Developing countries still face various conflicts and issues while promoting and utilizing e-commerce to the maximum compared with the developed countries ( Rossolov et al., 2021 ). In the developing countries, the difference between the perception and actual experience of the consumers varies when buying from indirect e-store compared to the direct e-store. On the contrary, as the world has been suffering from the COVID-19 pandemic, it has brought drastic changes globally in many sectors, business being one of them. De Vos (2020) stated that a large-scale lockdown was imposed worldwide to prevent the virus from spreading.
To survive, switching traditional shopping or trade toward digital was one factor that captured the attention across the globe on a larger scale. In April 2020, Walmart reported a 74% increase in online sales even though they faced a low customer walk-in at stores ( Nassauer, 2020 ; Redman, 2020 ). This upsurge of swift adoption of online channels has led this research to ask a few questions. First, what will be the difference between the perceived and the actual product purchased online? A recent study has documented that consumers bear actual risk after shopping through online channels ( Yang et al., 2020 ). Research reported that 30% of the products through online channels get returned and are not according to their perception ( Saleh, 2016 ). The same author also showed that the return and complaint rates are getting higher when consumers shop through an online channel.
Second, is there any difference between the perceived and the actual product purchase online from a direct e-store or an indirect e-store? Direct e-store means the online brand store, for example, Walmart, and indirect e-store means third-party stores such as Amazon, Alibaba, Jingdong (JD), and Daraz. The direct e-store strives hard to maintain a clear, potent perception in the mind of its buyer ( Grewal et al., 2009 ). Kumar and Kim (2014) stated that a brand strengthening its relationship with its consumer satisfies its needs through the actual product or services. In the literature ( Olotewo, 2017 ; Rossolov et al., 2021 ), it is stated that the shopping patterns of buyers from direct and indirect e-stores vary greatly, especially in the developing countries. In this way, when shopping through a direct e-store, consumers may easily recognize the difference in buying from a direct and indirect e-stores ( Mendez et al., 2008 ).
Third, a conceptual framework from a consumer perspective, antecedents and consequences of customer satisfaction, has been proposed and empirically proved. The literature ( Alharthey, 2020 ) discussed different risk types in online shopping. Three main types of risk, perceived uncertainty, perceived risk, and price, are addressed in this model. To the best of the knowledge of the authors, no such investigation directed specific circumstances, particularly in the developing countries. Therefore, it is necessary to look for the antecedents and consequences of customer satisfaction to promote online shopping in the developing countries. The degree of consumer satisfaction defines his/her experience and emotions about the product or service purchased through the online channel. Recent studies ( Guzel et al., 2020 ; Mamuaya and Pandowo, 2020 ) stated that the intention of the consumers to repurchase and their electronic-word-of-mouth (e-WOM) depends on their degree of satisfaction. In light of these heavy investments in online shopping, there is an exciting yet unexplored opportunity to comprehend better how the purchasing experiences of consumers through online channels influence their satisfaction level.
The study contributed to the current marketing literature in several ways. First, this study has highlighted that the perceived risk is very high when shopping through online channels, mainly the indirect e-stores. Therefore, the managers should develop strategies that reduce the perceived risk for the online consumer to shop more. Second, the study also disclosed that the perceived uncertainty in shopping through the online channel is high. While shopping online, the website design, graphics, and color scheme make the product more attractive than the actual one. Therefore, the managers must balance the visual appearance of the product on the website with the actual appearance of the product. This would increase the confidence and satisfaction of the consumer. Third, this study has also revealed that people are more satisfied while shopping from direct e-stores than indirect e-stores. Because the focal brands officially sponsor the direct e-stores, they pay more attention to their quality to retain consumers and maintain their brand reputation. Fourth, an indirect e-store works as a third party or a retailer who does not own the reputation of the product. This study exhibited the difference between the perception of the consumer being very high and the actual experience of using that product being quite different when shopping from the indirect channel. Last but not the least, this study is the first to report pre- and post-purchase consumer behavior and confirmed the perceived and the actual quality of a product bought from (i) direct e-store and (ii) indirect e-store.
Literature Review
Theoretical review.
Literature shows that when consumers get influenced to buy a particular product or service, some underlying roots are based on their behavior ( Wai et al., 2019 ). Appraisal theory significantly explains consumer behavior toward shopping and provides an opportunity to analyze the evaluation process (e.g., Roseman, 2013 ; Kähr et al., 2016 ; Moors et al., 2017 ; Ul Haq and Bonn, 2018 ). This research, aligned with the four dimensions of appraisal theory as the first stage, clearly defines the agency stage that either of the factors is responsible for customer satisfaction. The second stage explains that consumer's degree of satisfaction holds great importance and refers to novelty in the literature. The third stage of the model briefly explains the feelings and emotions of the consumers about the incident, aligning with the certainty phase. The last step explains whether the consumers have achieved their goal or are not aligned with the appetitive purpose.
Cognitive appraisal researchers stated that various emotions could be its root cause ( Scherer, 1997 ); it could be the reaction to any stimulus or unconscious response. On the contrary, four dimensions of appraisal theory are discussed in this research ( Ellsworth and Smith, 1988 ; Ma et al., 2013 ). Agency (considering themselves or objects are answerable for the result of the circumstance) ( Smith and Ellswoth, 1985 ; Durmaz et al., 2020 ); novelty (assessing the difference between the perception of an individual and his actual experience) ( Ma et al., 2013 ); certainty (analysis of the apparent probability of a specific outcome and its effect on the emotions of the buyer) ( Roseman, 1984 ), and appetitive goal (judging the degree to what extent the goal has been achieved) ( Hosany, 2012 ).
Hypotheses Development
Perceived risk and consumer satisfaction.
Perceived risk is the perception of shoppers having unpleasant results for buying any product or service ( Gozukara et al., 2014 ). Consumers who buy a specific product or service strongly impact their degree of risk perception toward buying ( Jain, 2021 ). Buyers who tend to indulge in buying through online channels face perceived risk characterized by their perception compared to the actual uncertainty involved in it ( Kim et al., 2008 ). Literature ( Ashoer and Said, 2016 ; Ishfaq et al., 2020 ) showed that as the risk of buying is getting higher, it influences the degree of consumers about information about their buying, either purchasing from the direct or indirect e-shop. Johnson et al. (2008) stated that consumer judgment that appears due to their experience strongly impacts their satisfaction level. Jin et al. (2016) said that as the ratio of risk perception of their consumer decreases, it enhances customer satisfaction. Thus, from the above arguments, it is hypothesized as follows:
H 1 : Perceived risk has a significant negative impact on consumer satisfaction—direct vs. indirect e-store; perceived vs. actual experience .
Perceived Uncertainty and Consumer Satisfaction
Uncertainty is defined as a time that occurs in the future that comprises the predictable situation due to the asymmetry nature of data ( Salancik and Pfeffer, 1978 ). Consumers may not expect the outcome of any type of exchange conducted as far as the retailer and product-oriented elements are concerned ( Pavlou et al., 2007 ). Therefore, uncertainty initiates that retailers may not be completely predictable; on the contrary, consumers tend to analyze and understand their actions about decision making ( Tzeng et al., 2021 ). Thus, the degree of uncertainty involved in buying through online channels influences that degree of customer satisfaction. In addition, when the performance of any particular product or service matches the degree of expectations, he gets satisfied and, hence, repeats his decision of buying ( Taylor and Baker, 1994 ). But if the product quality fails to meet the requirements, it negatively affects the degree of satisfaction ( Cai and Chi, 2018 ).
H 2 : Perceived uncertainty has a significant negative impact on consumer satisfaction—direct vs. indirect e-store; perceived vs. actual experience .
Price Value and Consumer Satisfaction
Oliver and DeSarbo (1988) suggested that the price value is the proportion of the result of the buyer to the input of the retailer. It is defined as an exchange of products/services based on their quality against a price that is to be paid ( Dodds et al., 1991 ). Consumers look for a higher value in return; consumers are willing to pay a higher price ( Pandey et al., 2020 ). Yet, it leads to higher dissatisfaction when they receive a lower degree of profitable products. Besides, the buyers associate such type of product/service they use as less favorable or not according to their needs and desires. Hence, the buyers regret their decision-making degree for choosing that particular product ( Zeelenberg and Pieters, 2007 ). Aslam et al. (2018) indicated that a product/service price influences the satisfaction of a buyer. Afzal et al. (2013) recommended that the price is among those factors that hold great significance for the degree of satisfaction of the consumer. If the price value of any product/service differs from consumer to consumer, consumers tend to switch brands. Hence, it is hypothesized that:
H3 : Price value has a significant positive impact on consumer satisfaction—direct vs. indirect e-store; perceived vs. actual experience .
Consumer Satisfaction With Consumer Delight, Consumer Regret, and Outrage
Satisfaction is defined as how a consumer is pleased with a particular brand or view about a product/service that matches requirements. It is an essential factor that triggers when the product or service performance exceeds the expectation and perception of the customers ( Woodside et al., 1989 ). The decision of the buyer significantly affects their satisfaction toward the product or service ( Park et al., 2010 ). If buyers are satisfied with the product/service they purchased online, this degree of satisfaction significantly affects their repurchase intention and WOM ( Butt et al., 2017 ). Tandon (2021) stated that a consumer satisfied with the product/service would get delighted. Consumer satisfaction, when exceeding the expectations, leads to consumer delight ( Mikulić et al., 2021 ). Mattila and Ro (2008) recommended that the buyer gets disappointed by anger, regret, and outrage. It also defines that negative emotions have a significant effect on the purchasing intention of the consumers. Oliver (1989) stated that unsatisfied buyers or products that do not fulfill the needs of the customers can create negative emotions. Sometimes, their feelings get stronger and result in sadness and outrage. Bechwati and Xia (2003) recommended that the satisfaction of the consumers influences their behavior to repurchase; outraged consumers due to dissatisfaction sometimes want to hurt the company. Besides deciding to purchase, consumers mostly regret their choices compared to other existing choices ( Rizal et al., 2018 ). Hechler and Kessler (2018) investigated that consumers who are outraged in nature actively want to hurt or harm the company or brand from which they got dissatisfied or hurt. Thus, it is proposed that:
H 4 : Consumer satisfaction has a significant negative impact on (a) consumer delight, (b) consumer regret, (c) consumer outrage—direct vs. indirect e-store; perceived vs. actual experience .
Consumer Delight and E-WOM
Oliver et al. (1997) recommended that a degree of delight in a buyer is termed as a positive emotion. Consumers purchase a product/service that raises their degree of expectation and gets them delighted ( Crotts and Magnini, 2011 ). Delighted buyers are involved in sharing their experiences with their friends and family and spreading positive WOM to others ( Parasuraman et al., 2020 ). Happy buyers generally share their opinions while posting positive feedback through social media platforms globally ( Zhang, 2017 ). A positive WOM of the buyer acts as a fundamental factor in spreading awareness about the product/service and strongly impacts other buyers regarding buying it ( Rahmadini and Halim, 2018 ). Thus, it is proposed that:
H5 : Consumer delight has a significant positive impact on E-WOM—direct vs. indirect e-store; perceived vs. actual experience .
Consumer Delight and Repurchase Intention
Delighted consumers tend toward brand loyalty; thus, they increase their buying intention of the service or product ( Ludwig et al., 2017 ; Ahmad et al., 2021 ). Customers can understand the objective of loyalty in purchasing a similar product or a new one from the same company. Delighted consumers tend to indulge in a higher degree of an emotional state that leads them to higher purchase intentions; it eliminates the switching of brands ( Parasuraman et al., 2020 ). Kim et al. (2015) stated that consumers delighted with a product or service of a brand become loyal to it, and the possibility of switching brands gets very low. Research ( Loureiro and Kastenholz, 2011 ; Tandon et al., 2020 ) shows that delighted consumers are more eager to purchase the same product again. Hence, it is proposed that:
H6 : Consumer delight has a significant positive impact on his repurchase intention—direct Vs. indirect e-store; Perceived Vs. actual experience
Consumer Regret and E-WOM
Regret is considered a negative emotion in reaction to an earlier experience or action ( Tsiros and Mittal, 2000 ; Kumar et al., 2020 ). Regret is when individuals frequently feel pity, disgrace, shame, or humiliation after acting in a particular manner and afterward try to amend their possible actions or decisions ( Westbrook and Oliver, 1991 ; Tsiros and Mittal, 2000 ). Regret is that specific negative emotion the buyers feel while making a bad decision that hurts them; their confidence level is badly affected. They blame themselves for choosing or creating a terrible decision ( Lee and Cotte, 2009 ). Li et al. (2010) suggested that buyers quickly start regretting and find their way to express their negative emotions. When they feel betrayed, they tend to spread negative WOM (NWOM) as a response to their anxiety or anger. Jalonen and Jussila (2016) suggested that buyers who get dissatisfied with their selections get involved in negative e-WOM about that particular brand/company. Earlier research says that buyers suffering from failure to buy any product/services tend to participate actively and play a role in spreading NWOM due to the degree of regret after making bad choices. Whelan and Dawar (2014) suggested that consumers sense that business has treated them unreasonably, and many consumers complain about their experience, resulting in e-WOM that may reduce consumer repurchase intention. Thus, it can be stated that:
H7 : Consumer regret has a significant negative impact on e-WOM—direct vs. indirect e-store; perceived vs. actual experience .
Consumer Regret and Repurchase Intention
Regret has a substantial influence on the intentions of the consumers to not entirely be measured by their degree of happiness ( Thibaut and Kelley, 2017 ). Results may not be evaluated by matching the structured degree of expectation but are also linked to alternatives reachable in the market. Therefore, such sort of evaluation and assessments will probably influence repurchase intention. For example, suppose the skipped reserve overtakes the picked alternative. In that case, the customer might change the replacement for the future purchase, regardless of whether the individual is profoundly happy with the picked option ( Liao et al., 2017 ). According to the researchers, there is a negative relationship between regret and consumer repurchase intention ( Liao et al., 2017 ; Durmaz et al., 2020 ). Furthermore, Unal and Aydin (2016) stated that perceived risk negatively impacts regret, influencing the repurchase intention of the consumers. Thus, it can be stated that:
H8 : Customer's regret has a significantly negative influence on his repurchase intention—direct vs. indirect e-store; perceived vs. actual experience .
Consumer Outrage and E-WOM
The disappointment of the consumers is a negative response to a product or a service ( Anderson and Sullivan, 1993 ). Outrage is the negative emotion a consumer experience when he purchases something totally against his requirements ( Lindenmeier et al., 2012 ). Besides, when the perception of the buyer is infringed, such behaviors occur. According to Torres et al. (2019) , enraged consumers get involved in communicating their outrage through e-WOM. Outraged consumers actively hurt the firm or brand from which they got hurt ( Hechler and Kessler, 2018 ). Consumers give e-WOM online reviews to decrease the negative emotions from the experiences of the consumer and re-establish a calm mental state to equilibrium ( Filieri et al., 2021 ). Thus, such consumers tend to give negative comments about the brand or product, which failed to match their expectations. NWOM has been characterized as negative reviews shared among people or a type of interpersonal communication among buyers concerning their experiences with a particular brand or service provider ( Balaji et al., 2016 ). Hence, it is hypothesized that:
H9 : Consumer outrage has a significant negative impact on e-WOM—direct vs. indirect e-store; perceived vs. actual experience .
Consumer Outrage and Repurchase Intentions
Repurchase intentions are characterized as the expressed trust of a buyer that they will or will not purchase a specific product and service again in the future ( Malhotra et al., 2006 ). Establishing relations with buyers should result in the repurchase. Negative disconfirmation ensues dissatisfaction or a higher level of outrage ( Escobar-Sierra et al., 2021 ). When a service/product fails and is not correctly addressed, the negative appraisal is overstated. Hence, “it may be more difficult to recover from feelings of victimization than to recover from irritation or annoyance” typically associated with dissatisfaction ( Schneider and Bowen, 1999 , p. 36). Therefore, consumers get outraged from buying such a product that fails to match their perception. When the experience of a consumer prompts a negative disconfirmation, the purchaser will also have a higher urging level through outrage. Therefore, consumers will probably have negative intentions to repurchase and do not want to indgule in making the same decision repeatedly ( Wang and Mattila, 2011 ; Tarofder et al., 2016 ). Therefore, it is proposed that:
H10 : Consumer outrage has a significant negative impact on repurchase intention—direct vs. indirect e-store; perceived vs. actual experience .
Methodology
This research explores the difference between the perception of the consumers and the actual online shopping experience through direct and indirect e-stores. It was an experimental design in which online shopping was studied in the developing countries. Data were collected from those individuals who shop from online channels; direct e-store and indirect e-store. Taking care of COVID-19 standard operating procedures, only 50 respondents were gathered two times, every time in a university auditorium after obtaining the permission from the administration. The total capacity of the auditorium was 500, as the lockdown restrictions were lifted after the first wave of the coronavirus.
Data Collection Tool
A questionnaire was used for the survey. The questionnaire was adapted in English to guarantee that the respondents quickly understood the questions used. A cross-sectional study technique was used for this research. A cross-sectional study helps in gathering the data immediately and collects data from a large sample size. The total number of distributed questionnaires was 1,250, out of which 800 were received in the usable form: 197 incomplete, 226 incorrect, and dubious responses, and 27 were eliminated. Thus, a 64% response rate was reported. Research showed that a 1:10 ratio is accepted ( Hair et al., 1998 ) as far as the data collection is concerned; for that instance, this study data fell in the acceptable range.
Indirect E-Store
Consumers who prefer to shop through online channels were gathered in an auditorium of an institute. Only those consumers were eligible for this experiment, who themselves buy through e-stores. A few products were brought from an indirect e-store, and later on, those products were shown to the respondents from the website of that indirect e-store. After showing products, we asked the respondents to fill the survey as per their perception of the product. Then we asked them to fill out another questionnaire to ascertain the difference between the perception and actual experience when purchasing from an indirect e-store. Once all the respondents completed the survey, we have shown them the actual products they have selected by seeing the website of the indirect e-store.
Direct E-Store
The second experiment was carried out on those consumers who shop from direct e-stores. For that purpose, a few popular reviewed clothing articles were purchased from the e-store. As in the case of an indirect e-store, respondents were also shown these articles from the websites of these direct e-stores. We then asked the respondents to fill the survey to confirm their perception of the products. Once all the respondents completed the survey, we showed them the actual product and asked them to fill out another questionnaire according to their actual purchasing experience from the direct e-store. The primary purpose of this experiment was to compare buying from direct e-store and indirect e-store.
Construct Instruments
The total number of items was 34, which were added in the earlier section of the questionnaire. These items were evaluated with the help of using a five-point Likert scale that falls from strongly disagree (1) to strongly agree (5). The items used in the study were empirically validated. Table 2 carries the details of the items of the questionnaire. The price value was evaluated using three items used by Venkatesh et al. (2012) . The perceived uncertainty was one of the independent variables that carry four items derived from Pavlou et al. (2007) . Perceived risk was the third independent variable used, held three items; thus, its scale was derived from Shim et al. (2001) . Wang (2011) validated consumer satisfaction carrying three items; consumer delight was measured by a 3-item scale proposed by Finn (2012) ; consumer regret was measured by the scale proposed by Wu and Wang (2017) . It carries a three-item scale. Consumer outrage was measured by Liu et al. (2015 ); it has six items. Repurchase intention was measured through a scale adapted from Zeithaml et al. (1996) , which carries four items. e-WOM was validated by the scale adapted from Goyette et al. (2010) ; it has five items.
Demographics of the Respondents
A total of 800 questionnaires were filled, and the respondents expressed their perception and actual experience from direct e-store and indirect e-store. Respondents belonged to different age groups from 18 to 50 years and above. There were 49% women and 51% men who took part in filling this survey. The income level of the respondents was grouped in different categories from “above 10,000 to above 50,000. The majority (56%) of the respondents were single, and 44% were married (Details can be viewed in Figure 1 ; Table 1 ). Data for both direct and indirect e-store was collected equally; 50% each to compare each category better.
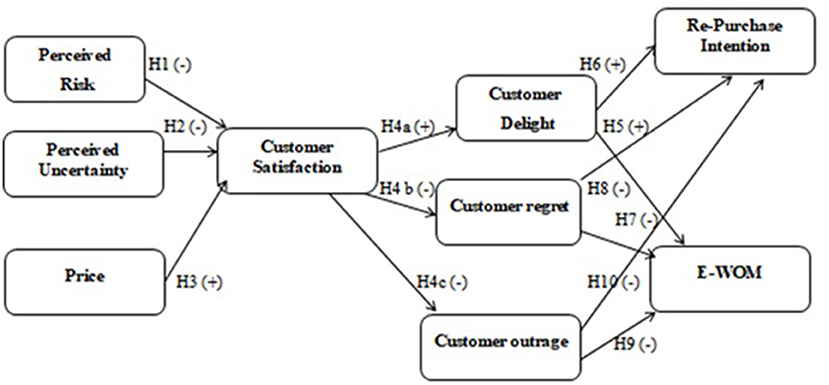
Figure 1 . Proposed conceptual framework.
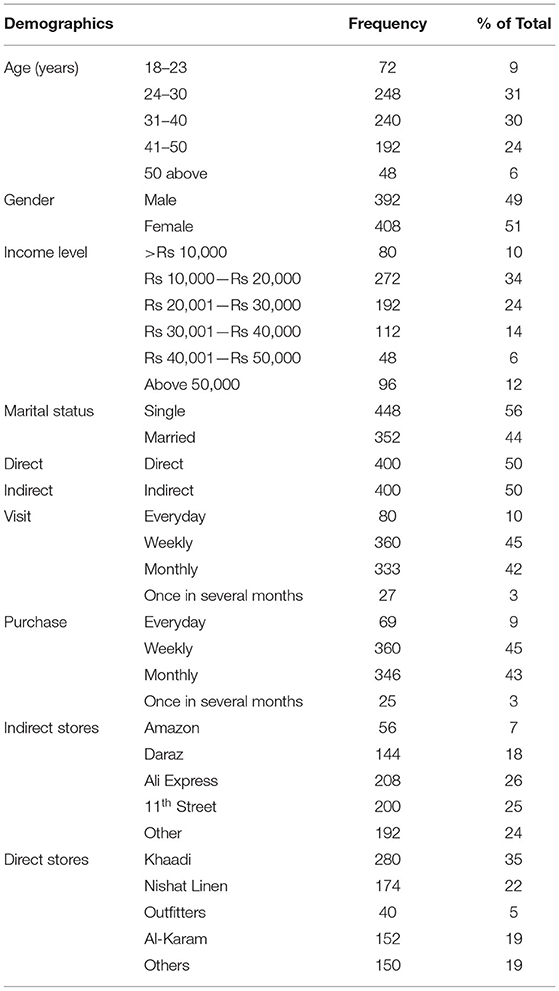
Table 1 . Demographics of the respondents.
Reliability and Validity
Reliability evaluates with the help of composite reliability (CR). All CR values fall into the range of 0.7–0.9, which is acceptable ( Hair et al., 2011 ). Convergent and discriminant validity has been observed through confirmatory factor analysis as recommended by some researchers ( Fornell and Larcker, 1981 ; Hair et al., 2010 ).
Convergent Validity
Convergent validity is evaluated with the help of two standards mentioned in the literature earlier, factor loading and average variance extracted (AVE), both the values should be >0.5 ( Yap and Khong, 2006 ). The values are mentioned in Table 2 .
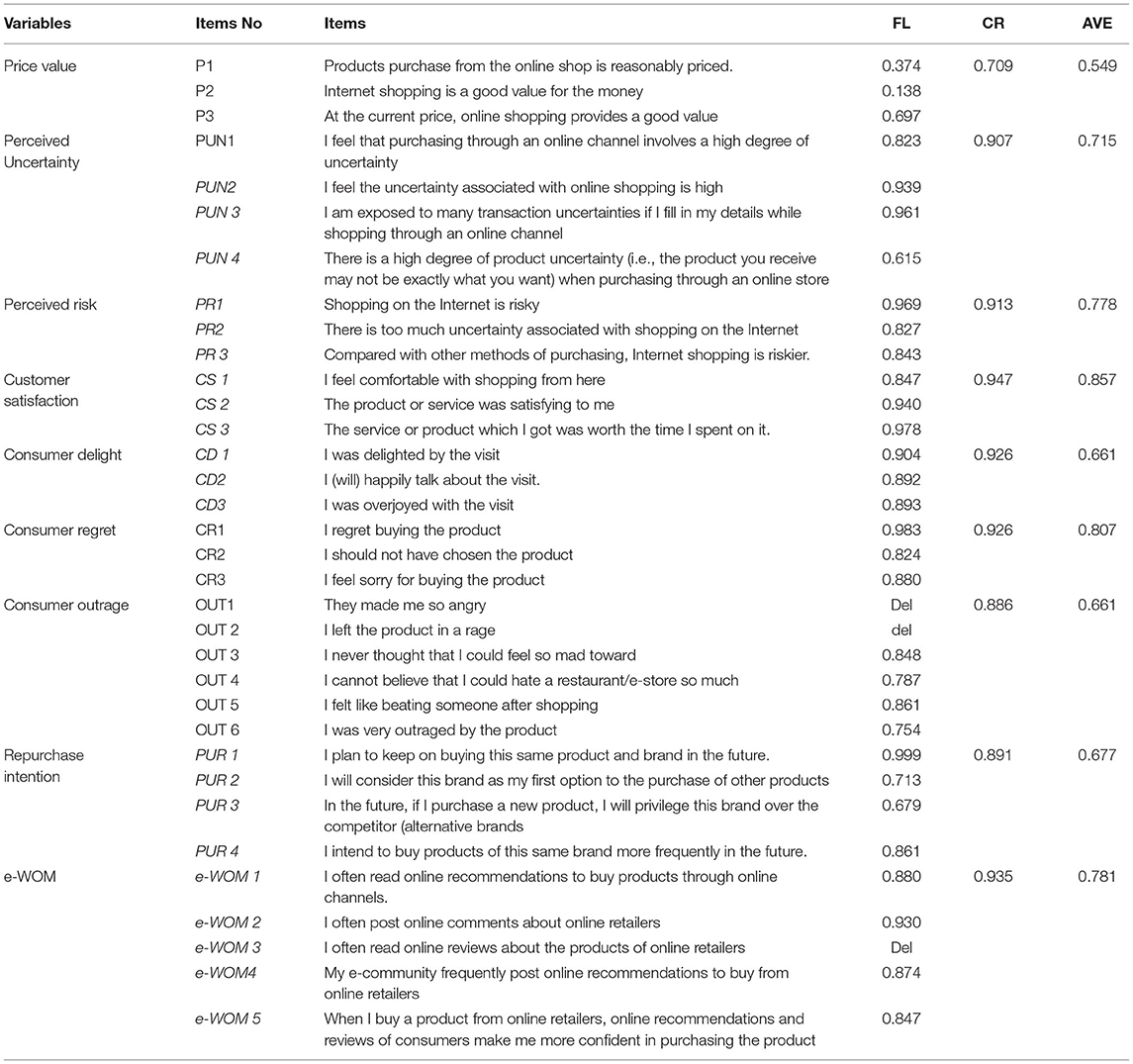
Table 2 . Reliability and convergent validity.
Discriminant Validity
Discriminant validity is evaluated based on two conditions that are required to evaluate it. First, the correlation between the conceptual model variables should be <0.85 ( Kline, 2005 ). Second, the AVE square value must be less than the value of the conceptual model ( Fornell and Larcker, 1981 ). Table 3 depicts the discriminant validity of the construct of the study.
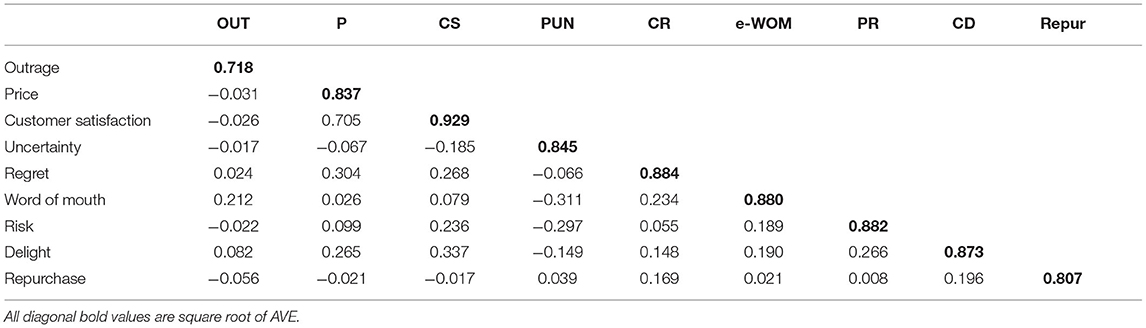
Table 3 . Discriminant validity.
Multi-Group Invariance Tests
Multi-group confirmatory factor analysis was conducted as the pre-requisites for the measurement model. The multi-group analysis was used to investigate a variety of invariance tests. Different invariance tests were performed to guarantee the items working precisely in the same manner in all the groups. In this research, the following are the model fit indexes, that is, CMIN/dF =2.992 CFI = 0.915, TLI = 0.906, and RMSEA = 0.071. Byrne (2010) and Teo et al. (2009) stated that CFI gives more accurate results, especially when comparing variables in different groups.
Hypotheses Testing
Scanning electron microscope technique was used to run and test the proposed hypotheses for the conceptual model. First, all the hypotheses proposed were checked, from which eight were initially accepted. Later, the multi-group test was utilized to test the proposed hypotheses and compare the shopping experience from direct e-store with indirect e-store and consumer perception with actual experience. Table 4 explains this in detail.
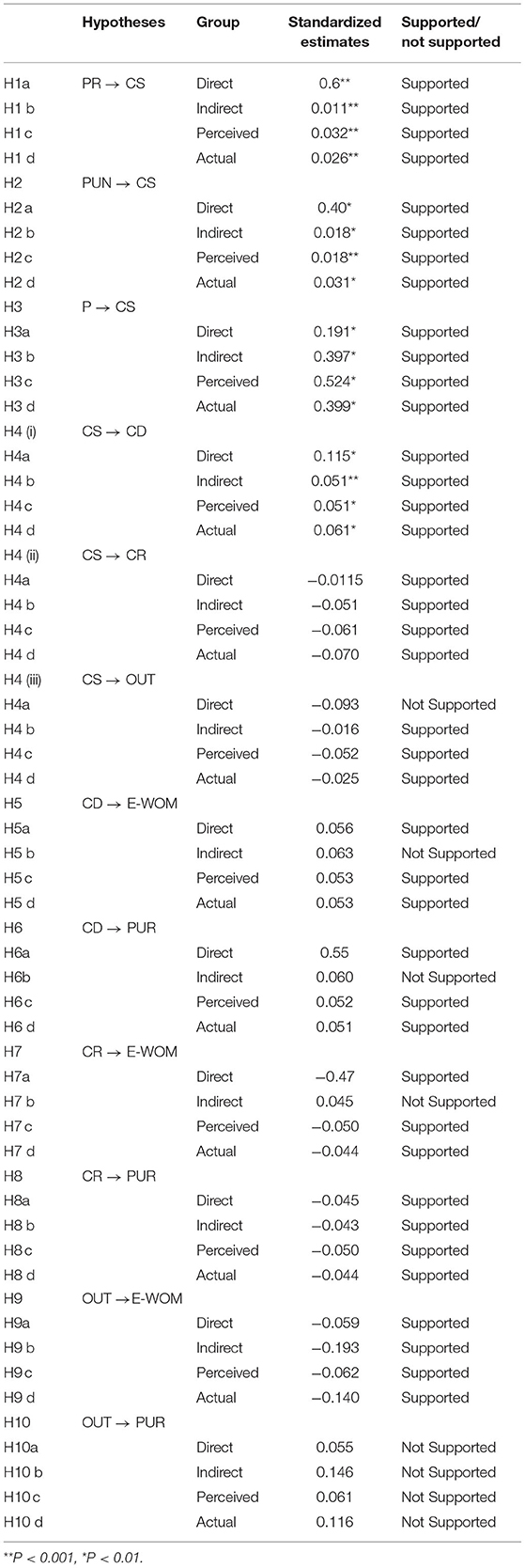
Table 4 . Hypotheses results.
Discussion and Implications
This research offers a remarkable number of facts for practitioners. This study can benefit marketing strategists by reducing the perceived risk, decreasing the intensity of perceived uncertainty, stabilizing the price, enhancing consumer satisfaction, promoting delighting consumers, accepting the negative behavior of the consumers, consumer retention, and establishing a positive e-WOM.
Reducing Risks
Certain factors play a role in antecedents of consumer satisfaction; they are particularly those that resist consumers to shop from any online channel, neither direct e-store nor indirect e-store. Perceived risk, perceived uncertainty, and the price are some of those antecedents that play a significant role in affecting the degree of satisfaction of the consumers, resulting in either to retain a consumer or to outrage a consumer. This study aligns with the existing literature. Tandon et al. (2016) ; Bonnin (2020) and Pandey et al. (2020) showed that consumers seek to shop from an e-store without bearing any risk. Consumers feel more confident about an e-store when the perceived risk is less than shopping from traditional ones as consumers want to feel optimistic about their decision. Second, e-vendors should ensure that the quality of a product is up to the mark and according to the consumer needs. Therefore, vendors should offer complete details about the product/service and its risks to the consumers. Moreover, this study suggests that e-stores must align the visuals of a product with its actual appearance. This would help them to increase customer satisfaction and confidence in the e-store.
Focus on Consumer Satisfaction
Consumer satisfaction is the deal-breaker factor in the online sector. Literature ( Shamsudin et al., 2018 ; Hassan et al., 2019 ) showed that organizations prioritize their consumers by fulfilling their requirements and required assistance. As a result, consumers are more confident and become satisfied consumers in the long run. This study adds to the literature that the degree of satisfaction of the consumers plays an essential role in shopping from an e-store. Consumers feel more confident in shopping from a direct e-store than an indirect e-store as the difference in the perception of consumers and the actual experience varies. Therefore, online vendors should focus on satisfying their consumers as it plays a remarkable role in retaining consumers.
Value Consumer Emotions
Online, retaining, and satisfying consumers are the most vital factor that directly affects the organization. This research aligns with the existing literature ( Jalonen and Jussila, 2016 ; Hechler and Kessler, 2018 ; Coetzee and Coetzee, 2019 ); when the retailer successfully fulfills its requirements, the consumer gets delighted repeating his choice to repurchase. On the other hand, if the online retailer fails to serve the consumer, the consumer regrets and, in extreme cases, becomes outraged about his decision. The negative emotions of the consumers threaten the company from many perspectives, as the company loses its consumer and its reputation in the market is affected. Therefore, first, market practitioners should avoid ignoring the requirements of consumers. Second, online vendors should pay special attention to the feedback of the consumers and assure them that they are valued.
Consumer Retention
The ultimate goal is to retain its consumers, but e-vendors should make proper strategies to satisfy their consumers as far as the online sector is concerned. The earlier studies of Zhang et al. (2015) and Ariffin et al. (2016) contributed to the literature that consumer satisfaction is a significant aspect in retaining a consumer. This research has also suggested that the satisfaction of the consumers plays a vital role in retaining them. Moreover, online shoppers provide the fastest spread of the right WOM about the product/ service. Second, consumers should feel valued and committed to vendors.
Pre- and Post-buying Behavior
This study contributed to a conceptual model that deals with consumer pre- and post-purchase behavior from the direct and indirect e-stores. With the help of experimental design, this study has reported its finding, highlighted how a satisfied customer is delightful and shares e-WOM, and showed repurchase intention. However, if the customer is not satisfied with the flip of a coin, he may feel regretted or outraged and cannot share e-WOM or have a repurchase intention.
Conclusions
This research concludes that online shopping has boomed during this COVID-19 pandemic period, as the lockdown prolonged in both the developed and the developing countries. The study further supports the difference between shopping from a direct e-store and an indirect e-store. The perception of the consumers shopping from direct e-store is more confident, and their degree of satisfaction is much higher, as the actual experience of the consumers aligns with their perceptions. Instead, consumers feel dissatisfied or outraged to choose an indirect e-store for shopping. Indirect e-store makes false promises and guarantees to its buyers, and eventually, when the consumers experience the product, it is against their perception.
This research fills the literature gap about the antecedents that lead to online shopping growth in the developing countries. This study aligns with Hechler and Kessler's (2018) earlier research, which stated that dissatisfied consumers threaten the reputation of the organization. Furthermore, Klaus and Maklan (2013) , Lemon and Verhoef (2016) suggested that handling the experience and satisfaction of the buyers plays a significant role in surviving among its competitors. Grange et al. (2019) recommended that e-commerce develops and attracts consumers by fulfilling their needs and requirements quickly. This study aligned with the existing literature by adding factors influencing the shopping preferences of the consumers from an e-store.
Limitations and Future Research
Despite its significant findings, this research has some limitations and scope for future research. First, this research only examined a few risks involved in online shopping. Future research studies should analyze other risks, for example, quality risk and privacy risk. Second, this study focused on shopping through direct e-stores and indirect e-stores. Future research can implement a conceptual model of a specific brand. Third, this study can be implemented in other sectors, for example, tourism, and hospitality. Fourth, it may be fascinating to look at other fundamentals, such as age, gender, education, relation with the retailer, or the degree of involvement with online shopping to differentiate other factors.
The proposed framework can be utilized in other developing countries, as every country faces different problems according to its growth and development. The model can be examined among specific direct e-stores to compare new customers and loyal customers. Future studies can explore indirect relationships along with adding mediators and moderators in the proposed model.
Data Availability Statement
The original contributions presented in the study are included in the article, further inquiries can be directed to the corresponding author.
Ethics Statement
The studies involving human participants were reviewed and approved by This study involving human participants was reviewed and approved by the Ethics Committee of the Department of Management Sciences, Riphah International University, Faisalabad Campus, Faisalabad, Pakistan. The participants provided their written informed consent to participate in this study. The patients/participants provided their written informed consent to participate in this study.
Author Contributions
AS contributed to the conceptualization and writing the first draft of the research. JU contributed to visualizing and supervising the research. All authors who contributed to the manuscript read and approved the submitted version.
Conflict of Interest
The authors declare that the research was conducted in the absence of any commercial or financial relationships that could be construed as a potential conflict of interest.
Publisher's Note
All claims expressed in this article are solely those of the authors and do not necessarily represent those of their affiliated organizations, or those of the publisher, the editors and the reviewers. Any product that may be evaluated in this article, or claim that may be made by its manufacturer, is not guaranteed or endorsed by the publisher.
Afzal, S., Chandio, A. K., Shaikh, S., Bhand, M., and Ghumro, B. A. (2013). Factors behind brand switching in cellular networks. Int. J. Asian Soc. Science 3, 299–307.
Google Scholar
Ahmad, W., Kim, W. G., Choi, H. M., and Ul Haq, J. (2021). Modeling behavioral intention to use travel reservation apps: a cross-cultural examination between US and China. J. Retail. Consum. Serv. 63:102689. doi: 10.1016/j.jretconser.2021.102689
CrossRef Full Text | Google Scholar
Alharthey, B. (2020). The role of online trust in forming online shopping intentions. Int. J. Online Market. 10, 32–57. doi: 10.4018/IJOM.2020010103
Anderson, E. W., and Sullivan, M. W. (1993). The antecedents and consequences of customer satisfaction for firms. Market. Sci. 12, 125–143. doi: 10.1287/mksc.12.2.125
Ariffin, S., Yusof, J. M., Putit, L., and Shah, M. I. A. (2016). Factors influencing perceived quality and repurchase intention towards green products. Proc. Econ. Finan. 37, 391–396. doi: 10.1016/S2212-5671(16)30142-3
Ashoer, M., and Said, S. (2016). “The impact of perceived risk on consumer purchase intention in Indonesia; a social commerce study,” in Proceeding of the International Conference on Accounting, Management, Economics and Social Sciences . 1–13.
Aslam, W., Arif, I., Farhat, K., and Khursheed, M. (2018). The role of customer trust, service quality and value dimensions in determining satisfaction and loyalty: an Empirical study of mobile telecommunication industry in Pakistan. Market-TrŽište 30, 177–194. doi: 10.22598/mt/2018.30.2.177
Balaji, M. S., Khong, K. W., and Chong, A. Y. L. (2016). Determinants of negative word-of-mouth communication using social networking sites. Inform. Manage. 53, 528–540. doi: 10.1016/j.im.2015.12.002
Bechwati, N. N., and Xia, L. (2003). Do computers sweat? the impact of perceived effort of online decision aids on consumers' satisfaction with the decision process. J. Consum. Psychol. 13, 139–148. doi: 10.1207/S15327663JCP13-1andamp;2_12
Bonnin, G. (2020). The roles of perceived risk, attractiveness of the online store and familiarity with AR in the influence of AR on patronage intention. J. Retail. Consum. Serv. 52:101938. doi: 10.1016/j.jretconser.2019.101938
Butt, M. M., Rose, S., Wilkins, S., and Haq, J. U. (2017). MNCs and religious influences in global markets: drivers of consumer-based halal brand equity. Int. Market. Rev . 12:277. doi: 10.1108/IMR-12-2015-0277
Byrne, B. M. (2010). Structural Equation Modeling With AMOS: Basic Concepts, Applications, and Programming , 2nd Edn. New York, NY: Routledge.
Cai, R., and Chi, C. G. Q. (2018). The impacts of complaint efforts on customer satisfaction and loyalty. Serv. Industr. J. 38, 1095–1115. doi: 10.1080/02642069.2018.1429415
Coetzee, A., and Coetzee, J. (2019). Service quality and attitudinal loyalty: the mediating effect of delight on retail banking relationships. Glob. Bus. Econ. Rev. 21, 120–138. doi: 10.1504/GBER.2019.096856
Crotts, J. C., and Magnini, V. P. (2011). The customer delight construct: is surprise essential? Ann. Tourism Res. 38, 719–722. doi: 10.1016/j.annals.2010.03.004
De Vos, J. (2020). The effect of COVID-19 and subsequent social distancing on travel behavior. Transport. Res. Interdisciplin. Perspect. 5:100121. doi: 10.1016/j.trip.2020.100121
PubMed Abstract | CrossRef Full Text | Google Scholar
Dodds, W. B., Monroe, K. B., and Grewal, D. (1991). Effects of price, brand, and store information on buyers' product evaluations. J. Market. Res. 28, 307–319. doi: 10.1177/002224379102800305
Durmaz, Y., Demira,g, B., and Çavuşoglu, S. (2020). Influence of regret and regret reversing effort on dissatisfaction and repurchase intention after purchasing fashion products. Preprints. doi: 10.20944/preprints202003.0280.v1
Ellsworth, P. C., and Smith, C. A. (1988). Shades of joy: patterns of appraisal differentiating pleasant emotions. Cogn. Emot. 2, 301–331. doi: 10.1080/02699938808412702
Escobar-Sierra, M., García-Cardona, A., and Vera Acevedo, L. D. (2021). How moral outrage affects consumer's perceived values of socially irresponsible companies. Cogent Bus. Manage. 8:1888668. doi: 10.1080/23311975.2021.1888668
Filieri, R., Galati, F., and Raguseo, E. (2021). The impact of service attributes and category on eWOM helpfulness: an investigation of extremely negative and positive ratings using latent semantic analytics and regression analysis. Comput. Human Behav. 114:106527. doi: 10.1016/j.chb.2020.106527
Finn, A. (2012). Customer delight: distinct construct or zone of nonlinear response to customer satisfaction? J. Serv. Res. 15, 99–110. doi: 10.1177/1094670511425698
Fornell, C., and Larcker, D. F. (1981). Evaluating structural equation models with unobservable variables and measurement error. J. Market. Res. 18, 39–50. doi: 10.1177/002224378101800104
Goyette, I., Ricard, L., Bergeron, J., and Marticotte, F. (2010). e-WOM Scale: word-of-mouth measurement scale for e-services context. Can. J. Admin. Sci. 27, 5–23. doi: 10.1002/cjas.129
Gozukara, E., Ozyer, Y., and Kocoglu, I. (2014). The moderating effects of perceived use and perceived risk in online shopping. J. Glob. Strateg. Manage. 16, 67–81. doi: 10.20460/JGSM.2014815643
Grange, C., Benbasat, I., and Burton-Jones, A. (2019). With a little help from my friends: Cultivating serendipity in online shopping environments. Inf. Manage . 56, 225–235.
Grewal, D., Levy, M., and Kumar, V. (2009). Customer experience management in retailing: An organizing framework. J. Retail. 85, 1–14. doi: 10.1016/j.jretai.2009.01.001
Guzel, M., Sezen, B., and Alniacik, U. (2020). Drivers and consequences of customer participation into value co-creation: a field experiment. J. Product Brand Manage. doi: 10.1108/JPBM-04-2020-2847
Hair, J. F., Anderson, R. E., Tatham, R. L., and Black, W. C. (1998). Multivariate Data Analysis . 5th ed., Hoboken, NJ: Prentice-Hall.
Hair, J. F., Celsi, M., Ortinau, D. J., and Bush, R. P. (2010). Essentials of Marketing Research , Vol. 2. New York, NY: McGraw-Hill/Irwin.
Hair, J. F., Ringle, C. M., and Sarstedt, M. (2011). PLS-SEM: indeed a silver bullet. J. Market. Theor. Pract. 19, 139–152. doi: 10.2753/MTP1069-6679190202
Hassan, S., Shamsudin, M. F., and Mustapha, I. (2019). The effect of service quality and corporate image on student satisfaction and loyalty in TVET higher learning institutes (HLIs). J. Tech. Educ. Train. 11:4.
Hechler, S., and Kessler, T. (2018). On the difference between moral outrage and empathic anger: anger about wrongful deeds or harmful consequences. J. Exp. Soc. Psychol. 76, 270–282. doi: 10.1016/j.jesp.2018.03.005
Hosany, S. (2012). Appraisal determinants of tourist emotional responses. J. Trav. Res. 51, 303–314. doi: 10.1177/0047287511410320
Ishfaq, M., Nazir, M. S., Qamar, M. A. J., and Usman, M. (2020). Cognitive bias and the Extraversion personality shaping the behavior of investors. Front. Psychol. 11:556506. doi: 10.3389/fpsyg.2020.556506
Jain, S. (2021). Examining the moderating role of perceived risk and web atmospherics in online luxury purchase intention. J. Fash. Market. Manage. Int. J. 05:89. doi: 10.1108/JFMM-05-2020-0089
Jalonen, H., and Jussila, J. (2016). “Developing a conceptual model for the relationship between social media behavior, negative consumer emotions and brand disloyalty,” in Conference on e-Business, e-Services and e-Society (Cham: Springer), 134–145. doi: 10.1007/978-3-319-45234-0_13
Jin, N., Line, N. D., and Merkebu, J. (2016). The impact of brand prestige on trust, perceived risk, satisfaction, and loyalty in upscale restaurants. J. Hospital. Market. Manage. 25, 523–546. doi: 10.1080/19368623.2015.1063469
Johnson, M. S., Sivadas, E., and Garbarino, E. (2008). Customer satisfaction, perceived risk and affective commitment: an investigation of directions of influence. J. Serv. Market. 5:120. doi: 10.1108/08876040810889120
Kähr, A., Nyffenegger, B., Krohmer, H., and Hoyer, W. D. (2016). When hostile consumers wreak havoc on your brand: the phenomenon of consumer brand sabotage. J. Mark. 80, 25–41. doi: 10.1509/jm.15.0006
Kim, D. J., Ferrin, D. L., and Rao, H. R. (2008). A trust-based consumer decision-making model in electronic commerce: the role of trust, perceived risk, and their antecedents. Decis. Support Syst. 44, 544–564. doi: 10.1016/j.dss.2007.07.001
Kim, M., Vogt, C. A., and Knutson, B. J. (2015). Relationships among customer satisfaction, delight, and loyalty in the hospitality industry. J. Hospital. Tourism Res. 39, 170–197. doi: 10.1177/1096348012471376
Klaus, P. P., and Maklan, S. (2013). Towards a better measure of customer experience. Int. J. Market Res. 55, 227–246. doi: 10.2501/IJMR-2013-021
Kline, R. B. (2005). Principles and Practice of Structural Equation Modeling , 2nd Edn. New York, NY: Guilford Press.
Kumar, A., Chaudhuri, D., Bhardwaj, D., and Mishra, P. (2020). Impulse buying and post-purchase regret: a study of shopping behaviour for the purchase of grocery products. Int. J. Manage. 11:57. doi: 10.34218/IJM.11.12.2020.057
Kumar, A., and Kim, Y. K. (2014). The store-as-a-brand strategy: the effect of store environment on customer responses. J. Retail. Consum. Serv. 21, 685–695. doi: 10.1016/j.jretconser.2014.04.008
Lee, S. H., and Cotte, J. (2009). Post-purchase Consumer Regret: Conceptualization and Development of the PPCR Scale . ACR North American Advances.
Lemon, K. N., and Verhoef, P. C. (2016). Understanding customer experience throughout the customer journey. J. Market. 80, 69–96. doi: 10.1509/jm.15.0420
Li, S., Zhou, K., Sun, Y., Rao, L. L., Zheng, R., and Liang, Z. Y. (2010). Anticipated regret, risk perception, or both: which is most likely responsible for our intention to gamble? J. Gambl. Stud. 26, 105–116. doi: 10.1007/s10899-009-9149-5
Liao, C., Lin, H. N., Luo, M. M., and Chea, S. (2017). Factors influencing online shoppers' repurchase intentions: the roles of satisfaction and regret. Inform. Manage. 54, 651–668. doi: 10.1016/j.im.2016.12.005
Lindenmeier, J., Schleer, C., and Pricl, D. (2012). Consumer outrage: emotional reactions to unethical corporate behavior. J. Bus. Res. 65, 1364–1373. doi: 10.1016/j.jbusres.2011.09.022
Liu, M. W., and Keh, H. T. (2015). Consumer delight and outrage: scale development and validation. J. Serv. Theory Pract . 25, 680–699. doi: 10.1108/JSTP-08-2014-0178
Loureiro, S. M. C., and Kastenholz, E. (2011). Corporate reputation, satisfaction, delight, and loyalty towards rural lodging units in Portugal. Int. J. Hospital. Manage. 30, 575–583. doi: 10.1016/j.ijhm.2010.10.007
Ludwig, N. L., Heidenreich, S., Kraemer, T., and Gouthier, M. (2017). Customer delight: universal remedy or a double-edged sword? J. Serv. Theory Pract . 8:197. doi: 10.1108/JSTP-08-2015-0197
Ma, J., Gao, J., Scott, N., and Ding, P. (2013). Customer delight from theme park experiences: the antecedents of delight based on cognitive appraisal theory. Ann. Tour. Res. 42, 359–381. doi: 10.1016/j.annals.2013.02.018
Malhotra, N., Hall, J., Shaw, M., and Oppenheim, P. (2006). Marketing Research: An Applied Orientation . Melbourne, VC: Pearson Education Australia.
Mamuaya, N. C., and Pandowo, A. (2020). Determinants of customer satisfaction and its implications on word of mouth in e-commerce industry: case study in Indonesia. Asia Pacific J. Manage. Educ. 3, 16–27. doi: 10.32535/apjme.v3i1.740
Mattila, A. S., and Ro, H. (2008). Discrete negative emotions and customer dissatisfaction responses in a casual restaurant setting. J. Hosp. Tour. Res. 32, 89–107. doi: 10.1177/1096348007309570
Mendez, J. L., Oubina, J., and Rubio, N. (2008). Expert quality evaluation and price of store vs. manufacturer brands: an analysis of the Spanish mass market. J. Retail. Consum. Serv. 15, 144–155. doi: 10.1016/j.jretconser.2007.11.003
Mikulić, J., Kreši,ć, D., and Šerić, M. (2021). The factor structure of medical tourist satisfaction: exploring key drivers of choice, delight, and frustration. J. Hospital. Tour. Res. 1177:1096348020987273. doi: 10.1177/1096348020987273
Moors, A., Boddez, Y., and De Houwer, J. (2017). The power of goal-directed processes in the causation of emotional and other actions. Emot. Rev. 9, 310–318. doi: 10.1177/1754073916669595
Nassauer, S. (2020). Walmart sales surge as Coronavirus drives Americans to stockpile. Wall Street J . Availale online at: https://www.wsj.com/articles/walmart-sales-surge-as-coronavirus-drivesamericans-to-stockpile-11589888464?mod=hp_lead_pos5 (accessed on May 18, 2020).
Oliver, R. L. (1989). Processing of the satisfaction response in consumption. J. Consum. Satisfact. Dissatisfact. Complain. Behav. 2, 1–26.
Oliver, R. L., and DeSarbo, W. S. (1988). Response determinants in satisfaction judgments. J. Consum. Res. 14, 495–507. doi: 10.1086/209131
Oliver, R. L., Rust, R. T., and Varki, S. (1997). Customer delight: foundations, findings, and managerial insight. J. Retail. 73:311. doi: 10.1016/S0022-4359(97)90021-X
Olotewo, J. (2017). Examining the antecedents of in-store and online purchasing behavior: a case of Nigeria. J. Market. Res. Case Stud. 15, 1–16. doi: 10.5171/2017.668316
Pandey, N., Tripathi, A., Jain, D., and Roy, S. (2020). Does price tolerance depend upon the type of product in e-retailing? role of customer satisfaction, trust, loyalty, and perceived value. J. Strateg. Market. 28, 522–541. doi: 10.1080/0965254X.2019.1569109
Parasuraman, A., Ball, J., Aksoy, L., Keiningham, T. L., and Zaki, M. (2020). More than a feeling? toward a theory of customer delight. J. Serv. Manage . 3:34. doi: 10.1108/JOSM-03-2019-0094
Park, E. O., Chung, K. H., and Shin, J. I. (2010). The relationship among internal marketing, internal customer satisfaction, organizational commitment and performance. Product. Rev. 24, 199–232. doi: 10.15843/kpapr.24.2.201006.199
CrossRef Full Text
Pavlou, P. A., Liang, H., and Xue, Y. (2007). Understanding and mitigating uncertainty in online exchange relationships: a principal-agent perspective. MIS Q. 105–136. doi: 10.2307/25148783
Rahmadini, Y., and Halim, R. E. (2018). The “Influence of social media towards emotions, brand relationship quality, and word of Mouth (WOM) on Concert's Attendees in Indonesia,” in MATEC Web of Conferences (EDP Sciences) , 05058.
Redman, R. (2020). “Online grocery sales to grow 40% in 2020,” in Supermarket News . Available online at: https://www.supermarketnews.com/onlineretail/online-grocery-sales-grow-40-2020 (accessed on May 21, 2020).
Rizal, H., Yussof, S., Amin, H., and Chen-Jung, K. (2018). EWOM towards homestays lodging: extending the information system success model. J. Hosp. Tour. Technol. doi: 10.1108/JHTT-12-2016-0084
Roseman, I. J. (1984). Cognitive determinants of emotion: a structural theory. Rev. Person. Soc. Psychol. 5, 11–36.
Roseman, I. J. (2013). Author reply: on the frontiers of appraisal theory. Emot. Rev. 5, 187–188. doi: 10.1177/1754073912469592
Rossolov, A., Rossolova, H., and Holguín-Veras, J. (2021). Online and in-store purchase behavior: shopping channel choice in a developing economy. Transportation 20, 1–37. doi: 10.1007/s11116-020-10163-3
Salancik, G. R., and Pfeffer, J. (1978). Uncertainty, secrecy, and the choice of similar others. Soc. Psychol. 23, 246–255. doi: 10.2307/3033561
Saleh, M. A. H. (2016). Website design, technological expertise, demographics, and consumer's e-purchase transactions. Int. J. Market. Stud. 8, 125–138. doi: 10.5539/ijms.v8n1p125
Scherer, K. R. (1997). The role of culture in emotion-antecedent appraisal. J. Pers. Soc. Psychol. 73:902. doi: 10.1037/0022-3514.73.5.902
Schneider, B., and Bowen, D. E. (1999). Understanding customer delight and outrage. Sloan Manage. Rev. 41, 35–45. doi: 10.1016/S0022-4359(01)00035–45
Shamsudin, M. F., Razak, A. A., and Salem, M. A. (2018). The role of customer interactions towards customer satisfaction in theme parks experience. Opcion 34, 546–558.
Shim, S., Eastlick, M. A., Lotz, S. L., and Warrington, P. (2001). An online prepurchase intentions model: the role of intention to search: best overall paper award—the Sixth Triennial AMS/ACRA Retailing Conference, 2000? J. Retail. 77, 397–416. doi: 10.1016/S0022-4359(01)00051-3
Smith, C. A., and Ellsworth, P. C. (1985). Patterns of cognitive appraisal in emotion. J. Pers. Soc. Psychol . 48:813.
Tandon, A., Aakash, A., and Aggarwal, A. G. (2020). Impact of EWOM, website quality, and product satisfaction on customer satisfaction and repurchase intention: moderating role of shipping and handling. Int. J. Syst. Assur. Eng. Manage. 54, 1–8. doi: 10.1007/s13198-020-00954-3
Tandon, U. (2021). Predictors of online shopping in India: an empirical investigation. J. Market. Anal. 9, 65–79. doi: 10.1057/s41270-020-00084-6
Tandon, U., Kiran, R., and Sah, A. N. (2016). Understanding online shopping adoption in India: unified theory of acceptance and use of technology 2 (UTAUT2) with perceived risk application. Serv. Sci. 8, 420–437. doi: 10.1287/serv.2016.0154
Tarhini, A., Alalwan, A. A., Al-Qirim, N., and Algharabat, R. (2021). “An analysis of the factors influencing the adoption of online shopping,” in Research Anthology on E-Commerce Adoption, Models, and Applications for Modern Business (Pennsylvania: IGI Global), 363–384.
Tarofder, A. K., Nikhashemi, S. R., Azam, S. F., Selvantharan, P., and Haque, A. (2016). The mediating influence of service failure explanation on customer repurchase intention through customers satisfaction. Int. J. Qual. Serv. Sci. 4:44. doi: 10.1108/IJQSS-04-2015-0044
Taylor, S. A., and Baker, T. L. (1994). An assessment of the relationship between service quality and customer satisfaction in the formation of consumers' purchase intentions. J. Retail. 70, 163–178. doi: 10.1016/0022-4359(94)90013-2
Teo, T., Lee, C. B., Chai, C. S., and Wong, S. L. (2009). Assessing the intention to use technology among preservice teachers in Singapore and Malaysia: a multigroup invariance analysis of the technology acceptance model (TAM). Comput. Educ. 53, 1000–1009. doi: 10.1016/j.compedu.2009.05.017
Thibaut, J. W., and Kelley, H. H. (2017). The Social Psychology of Groups . Routledge. doi: 10.4324/9781315135007
Torres, E. N., Milman, A., and Park, S. (2019). Customer delight and outrage in theme parks: a roller coaster of emotions. Int. J. Hospital. Tour. Administr. 16, 1–23. doi: 10.1080/15256480.2019.1641455
Tsiros, M., and Mittal, V. (2000). Regret: a model of its antecedents and consequences in consumer decision making. J. Consum. Res. 26, 401–417. doi: 10.1086/209571
Tzeng, S. Y., Ertz, M., Jo, M. S., and Sarigöll,ü, E. (2021). Factors affecting customer satisfaction on online shopping holiday. Market. Intell. Plann. 8:346. doi: 10.1108/MIP-08-2020-0346
Ul Haq, J., and Bonn, M. A. (2018). Understanding millennial perceptions of human and nonhuman brands. Int. Hospital. Rev . 9:14. doi: 10.1108/IHR-09-2018-0014
Unal, S., and Aydin, H. (2016). Evaluation of consumer regret in terms of perceived risk and repurchase intention. J. Glob. Strateg. Manage. 2, 31–31. doi: 10.20460/JGSM.20161024354
Venkatesh, V., Thong, J. Y., and Xu, X. (2012). Consumer acceptance and use of information technology: extending the unified theory of acceptance and use of technology. MIS Q. 157–178. doi: 10.2307/41410412
Wai, K., Dastane, O., Johari, Z., and Ismail, N. B. (2019). Perceived risk factors affecting consumers' online shopping behaviour. J. Asian Financ. Econ. Bus. 6, 246–260. doi: 10.13106/jafeb.2019.vol6.no4.249
Wang, C. Y., and Mattila, A. S. (2011). A cross-cultural comparison of perceived informational fairness with service failure explanations. J. Serv. Market . 25, 429–439. doi: 10.1108/08876041111161023
Wang, X. (2011). The effect of unrelated supporting service quality on consumer delight, satisfaction, and repurchase intentions. J. Serv. Res. 14, 149–163. doi: 10.1177/1094670511400722
Westbrook, R. A., and Oliver, R. L. (1991). The dimensionality of consumption emotion patterns and consumer satisfaction. J. Consum. Res. 18, 84–91. doi: 10.1086/209243
Whelan, J., and Dawar, N. (2014). Attributions of blame following a product-harm crisis depend on consumers' attachment styles. Mark. Lett. 27, 285–294. doi: 10.1007/s11002-014-9340-z
Woodside, A. G., Frey, L. L., and Daly, R. T. (1989). Linking service quality, customer satisfaction, and behavio. Mark. Health Serv. 9:5. doi: 10.1016/S0022-4359(01)0009-5
Wu, R., and Wang, C. L. (2017). The asymmetric impact of other-blame regret versus self-blame regret on negative word of mouth: empirical evidence from China. Eur. J. Market. doi: 10.1108/EJM-06-2015-0322
Yang, Y., Gong, Y., Land, L. P. W., and Chesney, T. (2020). Understanding the effects of physical experience and information integration on consumer use of online to offline commerce. Int. J. Inf. Manage. 51:102046. doi: 10.1016/j.ijinfomgt.2019.102046
Yap, B. W., and Khong, K. W. (2006). Examining the effects of customer service management (CSM) on perceived business performance via structural equation modelling. Appl. Stochast. Models Bus. Indus. 22, 587–605. doi: 10.1002/asmb.648
Zeelenberg, M., and Pieters, R. (2007). A theory of regret regulation 1.0. J. Consum. Psychol. 17, 3–18. doi: 10.1207/s15327663jcp1701_3
Zeithaml, V. A., Berry, L. L., and Parasuraman, A. (1996). The behavioral consequences of service quality. J. Market. 60, 31–46. doi: 10.1177/002224299606000203
Zhang, H. (2017). Understanding the Consumption Experience of Chinese Tourists: Assessing the Effect of Audience Involvement, Flow and Delight on Electronic Word-of-mouth (eWOM) (Doctoral dissertation).
Zhang, Z., Ye, Q., Song, H., and Liu, T. (2015). The structure of customer satisfaction with cruise-line services: an empirical investigation based on online word of mouth. Curr. Issues Tourism . 18, 450–464.
Keywords: consumer perception, online shopping, actual experiences, customer satisfaction, direct shopping, perceived risk, delight, outrage
Citation: Rao YH, Saleem A, Saeed W and Ul Haq J (2021) Online Consumer Satisfaction During COVID-19: Perspective of a Developing Country. Front. Psychol. 12:751854. doi: 10.3389/fpsyg.2021.751854
Received: 02 August 2021; Accepted: 30 August 2021; Published: 01 October 2021.
Reviewed by:
Copyright © 2021 Rao, Saleem, Saeed and Ul Haq. This is an open-access article distributed under the terms of the Creative Commons Attribution License (CC BY) . The use, distribution or reproduction in other forums is permitted, provided the original author(s) and the copyright owner(s) are credited and that the original publication in this journal is cited, in accordance with accepted academic practice. No use, distribution or reproduction is permitted which does not comply with these terms.
*Correspondence: Junaid Ul Haq, junaid041@yahoo.com
Disclaimer: All claims expressed in this article are solely those of the authors and do not necessarily represent those of their affiliated organizations, or those of the publisher, the editors and the reviewers. Any product that may be evaluated in this article or claim that may be made by its manufacturer is not guaranteed or endorsed by the publisher.
Marketing research on Mobile apps: past, present and future
- Review Paper
- Published: 08 November 2021
- Volume 50 , pages 195–225, ( 2022 )
Cite this article
- Lara Stocchi 1 ,
- Naser Pourazad 2 ,
- Nina Michaelidou 3 ,
- Arry Tanusondjaja 1 &
- Paul Harrigan 4
51k Accesses
48 Citations
12 Altmetric
Explore all metrics
We present an integrative review of existing marketing research on mobile apps, clarifying and expanding what is known around how apps shape customer experiences and value across iterative customer journeys, leading to the attainment of competitive advantage, via apps (in instances of apps attached to an existing brand) and for apps (when the app is the brand). To synthetize relevant knowledge, we integrate different conceptual bases into a unified framework, which simplifies the results of an in-depth bibliographic analysis of 471 studies. The synthesis advances marketing research by combining customer experience, customer journey, value creation and co-creation, digital customer orientation, market orientation, and competitive advantage. This integration of knowledge also furthers scientific marketing research on apps, facilitating future developments on the topic and promoting expertise exchange between academia and industry.
Similar content being viewed by others
Mobile Applications, the Economics of
Understanding Perceived Values and Behavioral Effects of Mobile Apps: An Abstract
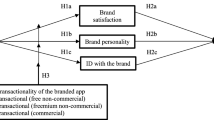
The role of transactionality of mobile branded apps in brand experience and its impact on loyalty
Avoid common mistakes on your manuscript.
Introduction
Mobile apps, or apps in short, have been defined as the ultimate marketing vehicle (Watson, McCarthy and Rowley 2013 ) and a staple promotional tactic (Rohm, Gao, Sultan and Pagani 2012 ) to attract business ‘on the go’ (Fang 2019 ). They yield great potential for customer engagement due to specific characteristics (e.g., vividness, novelty and built-in features, see Kim, Lin and Sung 2013 ), supporting one-to-one and one-to-many interactions (Watson et al. 2013 ) and facilitating exchanges without time or location-based restrictions (Alnawas and Aburub 2016 ). In essence, apps translate communication efforts into interactive customer experiences heightening cognitive, emotional and behavioral responses (Kim and Yu 2016 ). For example, apps support value-generating activities such as making purchases and accessing information (Natarajan, Balasubramanian and Kasilingam 2017 ). Accordingly, apps offer firms multiple opportunities to achieve marketing objectives, influencing and shaping the customer journey (Wang, Kim and Malthouse 2016a ). Overall, apps also allow firms to realize a digital customer orientation and to attain competitive advantages through the provision of superior customer experiences (Kopalle, Kumar and Subramaniam 2020 ).
Over the last decade, the popularity of apps continued to increase (currently, there are more than 2.87 million apps available, Buildfire 2021 ) and, although apps’ growth has gradually slowed down, they remain at the heart of digital marketing strategies, impacting economies worldwide (Arora, Hofstede and Mahajan 2017 ). For instance, in the US, apps drive about 60% of digital media consumption (Fang 2019 ) and 90% of the top 100 global brands offer one or more apps (Tseng and Lee 2018 ). Apps also generate significant economic results thanks to attaining prolonged media exposure and consumer spending. For example, the TikTok app generates over one billion video views every day (Influencer Marketing Hub 2018 ; Iqbal 2019 ) and has attracted $50 million in consumer spending last year, on top of advertising revenues (Williams 2020 ). The global health and financial crisis caused by the COVID-19 pandemic further illustrates the pivotal role apps play in facilitating business survival and reigniting customer experiences—see the instance of the Zoom app, which generated $2.6 billion revenue in 2020 (Sensortower 2020 ).
An increase in academic research on apps has matched their growth in popularity. Marketing is no exception to this trend; however, it lacks a state-of-the-art integrative review , which hinders the advancement of this field of inquiry. Integrative reviews offer new insights as a result of synthesis and critique, and are crucial for new knowledge generation (Elsbach and van Knippenberg 2020 ). Importantly, integrative reviews form the basis for justification or validation of established knowledge (MacInnis 2011 ); they also “identify new ways of conceiving a given field or phenomenon” (Post, Sarala, Gattrell and Prescott 2020 , p.354). Moreover, in addition to their substantiative theoretical contribution, integrative reviews typically facilitate the exchange of knowledge between academia and industry. Based on this reasoning, the present study has two research objectives. The first objective ( RO1 ) is to synthesize existing research on apps to sharpen scholarly understanding of their key role in marketing and customer experiences. To do so, we review established findings through the theoretical lens of the customer journey (Lemon and Verhoef 2016 ), which we modify and extend to establish conceptual links with digital customer orientation , market orientation and competitive advantage . As illustrated, the factor that connects these concepts and explicates apps’ relevance to marketing is value (including value co-creation ). The second objective ( RO2 ) involves offering a series of directions for future research based on priority knowledge gaps. The ultimate goal is to define future research paths for marketing scholars, while promoting knowledge and data exchange between academia and practice.
Comprehensively, this study constitutes the most extensive attempt in the marketing literature to integrate and review the full breadth of publications on apps and significantly differs from existing reviews (e.g., Ström, Vendel and Bredican 2014 ; Nysveen, Pedersen and Skard 2015 ). In particular, we synthesize 471 bibliometric sources, maintaining a clearer delineation between mobile technologies in general vs. apps. Our review also covers all types of apps and includes a unified conceptual framework—two further limitations of prior attempts (e.g., Tyrväinen and Karjaluato 2019 ; Mondal and Chakrabarti 2019 ).
Review approach
In line with past studies (e.g., Groenewald 2004 ; Mkono 2013 ), we used a semi-inductive approach to integrate and review marketing knowledge on apps. Specifically, as appropriate when reviewing fields that are not yet stabilized (Roma and Ragaglia 2016 ), we first conducted a bibliometric analysis to identify relevant sources, mapping the overall knowledge field via quantitative assessment of authors, references and citations (Culnan et al. 1990 ). We followed the same procedure as Samiee and Chabowski ( 2012 ), which begins with identifying keywords. In this regard, we drew upon extant literature on apps (e.g., Mondal and Chakrabarti 2019 ) to collate sources, which contained in the title, abstract or keywords any of the following terms: mobile application(s), mobile app(s), mobile phone application(s), mobile phone app(s), smartphone application(s), smartphone app(s), and apps(s). This selection aligns with past studies (e.g., Radler 2018 ) and reflects synonyms of apps used in real life. We also narrowed down the bibliometric data to sources with a clear marketing focus by screening for terms such as marketing , consumer or customer in the title, abstract or keywords. At times, this approach resulted in the inclusion of sources outside the confines of marketing research (e.g., technology and information system and/or management). Following a similar protocol to others (e.g., Wang, Zhao and Wang 2015 ; Mondal and Chakrabarti 2019 ), we located all sources from the Scopus database, concentrating on articles published in the last two decades—a timeframe, which captures seminal studies and more recent research. The second step of the review process involved screening all bibliometric sources to identify recurring themes and established findings. Following recent guidelines for developing insightful reviews (Hulland and Houston 2020 ), this intuitive review step also entailed locating and examining additional bibliometric sources not included in the initial data frame. The Web Appendix describes all sources examined (471) and a full-length bibliography.
Superordinate theoretical lens
To present the outcomes of our integrative review, we modify and expand the scope of the customer journey by Lemon and Verhoef ( 2016 ). This framework is applicable to different consumption contexts and simplifies the complexity resulting from seemingly disconnected theoretical bases. Moreover, it serves as a useful basis to understand and manage customer experiences . Customer experiences combine cognitive, emotional, behavioral, sensory and social aspects related to distinct consumption stages and touchpoints (see Verhoef, Lemon, Parasuraman, Roggeveen, Tsiros and Schlesinger 2009 ; Becker and Jaakkola 2020 ). In relation to apps, McLean, Al-Nabhani and Wilson ( 2018 ) highlight that the experiential (or journey) factor has been neglected thus far. This knowledge void is surprising, since apps are considered catalysts of ‘new’ customer experiences due to being a unique source of customer value . Nonetheless, apps call for critical modifications of Lemon and Verhoef’s ( 2016 ) framework, as follows.
First, we synthesize research across three journey stages: pre-adoption , adoption and post-adoption. Footnote 1 The pre-adoption stage concerns customer experiences and decision-making before app adoption, which shape consumer predispositions toward the app. In more detail, this stage captures the theoretical links between positive consumer attitudes, individual characteristics and the intention to download, adopt or use the app; it also includes firm/brand-initiated strategies to enhance consumer predispositions. The adoption stage includes customer experiences inherent to the continuation of the consumer decision-making process past initial predispositions, which signal app download and use . Experiences arise from firm/brand-initiated strategies and associated consumer reactions; they also originate from consumer characteristics likely to impact the choice of an app. Moreover, this stage includes activities that signify adoption such as using the app (e.g., mobile shopping). The post-adoption stage involves all customer experiences following adoption and resulting from ongoing app usage such as stickiness —i.e., the intention to continue using the app and frequency of app usage (Racherla, Furner and Babb 2012 ); and engagement (e.g., Kim et al. 2013 ; Wu 2015 ; Fang 2017 )—i.e., “a customer’s voluntary resource contribution to a firm’s marketing function, going beyond financial patronage” (Harmeling, Moffett, Arnold and Carlson 2017 , p.312). This final stage also includes relevant outcomes for the app and for the brand behind the app such as brand loyalty and customer satisfaction.
In line with Lemon and Verhoef’s ( 2016 ) assumptions, we contend the distinction between the three customer journey stages to be conceptually and practically fluid. Specifically, the adoption and post-adoption stages are blurred by a seamless feedback loop , since the decision-making process underpinning app adoption is likely to start from pre-adoption predispositions, and to be re-lived during activities that signify adoption whilst also shaping crucial post-adoption outcomes. However, for practical purposes, we distinguished bibliometric sources related to each stage by focusing on the focal concept or key dependent variable discussed in each source. For instance, we considered studies on intentions to adopt the app for pre-adoption; studies on app usage were examined for the adoption stage; and studies on stickiness and engagement were reviewed in relation to post-adoption.
A second modification of the original framework concerns the touchpoints. In more detail, we integrate brand-owned and partner-owned touchpoints , which are designed, managed and controlled by the firm and/or other partners (e.g., developers and app stores) due to the unique business model of app stores (Jung, Baek and Lee 2012 ); and consumer-owned and social touchpoints , which are out of the firm’s direct control, highlighting the extraordinary level of direct consumer involvement with apps through seamless feedback mechanisms—e.g., through customer ratings and reviews. Based on apps’ ubiquitous nature (Tojib and Tsarenko 2012 ), we also consider these touchpoints as “ always-on” points of interaction with a pervasive impact across all stages. For instance, taking the app’s marketing mix as an example (a key brand and partner-owned touchpoint), we assume it to impact consumer initial predispositions toward the app (pre-adoption); app usage (adoption) and consumer responses to the app (post-adoption).
Finally, to further enhance the theoretical and managerial contributions made, we expand the framework’s scope by linking it to customer orientation and competitive advantage via the broad notion of customer value . Kopalle et al. ( 2020 ) clarify that any brand or firm can harvest market opportunities by embracing a digital customer orientation . Digital customer orientation occurs “when the customization and enrichment of the experience delivered by a firm is in real-time and based on the in-use feedback from customers” (Kopalle et al. p. 115). This definition requires a platform for information sharing, real-time insights and context-driven value creation and co-creation . Apps are ideal platforms, as consumers can easily act as integrators of value and resources throughout the customer journey. For example, the business model of app stores hinges on user feedback and information exchange across the supply chain, extending the scope of apps to a broad service delivery network (Tax, McCutcheon and Wilkinson 2013 ). Apps are also viewed as dynamic packages of service provision (see Piccoli, Brohman, Watson and Parasuraman 2009 ), or ‘bundles’ of stimuli, functionalities and experiences that facilitate value creation and co-creation inherent to the appscape (Kumar, Purani and Viswanathan 2018 ; Lee 2018b ). Finally, apps are a pivotal source of hyper-contextualized consumer insights, which can be turned into market intelligence (Tong et al. 2020 ). By making consumer insights and market intelligence part of inter-functional coordination and strategic implementation (Narver and Slater 1990 ; Deshpandé, Farley and Webster 1993 ; Lafferty and Hult 2001 ), firms can consistently deliver superior customer value, attaining market orientation and competitive advantages via apps (when the app is linked to an existing brand) and for apps (when the app is the brand) .
Pre-adoption stage
Empirical research on the pre-adoption stage is abundant and focuses on two aspects that initiate the consumer decision-making process shaping consumer predispositions toward the app, driving the intention to download and/or adopt an app over other alternatives: the technological features and benefits consumers seek; and specific individual consumer characteristics . In contrast, research exploring different strategies for encouraging apps adoption is scarce. Table 1 summarizes existing theoretical approaches inherent to this stage, together with future research themes and examples of priority research questions.
Initiation of the consumer decision-making process
Technological features and benefits sought.
Extant research extensively documents technological features and benefits that consumers seek in apps, using the Technology Adoption Model (TAM) (Davis, Bagozzi and Warshaw 1989 ) and modifications of it, including conceptual models that combine technology adoption with Diffusion of Innovation Theory (Rogers 2005) and Uses and Gratification (U&G) theory (Mcguire 1974 , Eighmey and McCord 1998 ). In particular, past research consistently highlights the following key pre-adoption drivers. First, incentives of technology adoption such as usefulness, ease of use and enjoyment—all confirmed to enhance consumer positive attitudes and/or evaluations of an app, thus underpinning the intention to download and/or adopt the app (Bruner and Kumar 2005 ; Hong and Tam 2006 ; Karaiskos, Drossos, Tsiaousis, Giaglis and Fouskas 2012 ; Ko, Kim and Lee 2009 ; Maity 2010 ; Wang and Li 2012 ; Kim, Yoon and Han 2016b ; Li 2018 ; Stocchi, Michaelidou and Micevski 2019 ). Second, numerous studies stress the importance of value perceptions (Peng, Chen and Wen 2014 ; Zhu, So and Hudson 2017 ; Zolkepli, Mukhiar and Tan 2020 ), especially perceptions of convenience (Kim, Park and Oh 2008 ; Kang, Mun and Johnson 2015 ); novelty, accuracy and precision (Ho 2012 ); locatability (i.e., identifiability in space and time); and, more broadly, apps’ quality (Noh and Lee 2016 ). Studies also highlight apps’ potential to create positive consumer predispositions via personalization (Tan and Chou 2008 ; Wang and Li 2012 ; Watson et al. 2013 ; Li 2018 ); pleasant aesthetics (Stocchi et al. 2019 ; Kumar et al. 2018 ; Lee and Kim 2019 ); and the perceived monetary value (Hong and Tam 2006 ; Kim et al. 2008 ; Venkatesh, Thong and Xu 2012 ), which often counterbalances effort expectancy (Kang et al. 2015 ). Third, past research often explains the pre-adoption decision-making process via concentrating on medium characteristics such as apps’ compatibility, controllability, connectivity and service availability (Kim et al. 2008 ; Ko et al. 2009 ; Lu, Yang, Chau and Cao 2011 ; Mallat, Rossi, Tuunainen and Öörni 2009 ; Tan and Chou 2008 ; Wu and Wang 2005 ); and medium richness (Lee, Cheung and Chen 2007 ). Similarly, other research focuses on consumer’s positive attitudes resulting evaluations of the technology provider such as reputation (Chandra, Srivastava and Theng 2010 ) and communicativeness (Khalifa, Cheng and Shen 2012 ); or network factors including synergies with other channels (Kim et al. 2008 ) and app popularity (Picoto, Duarte and Pinto 2019 ).
The same technological features and benefits discussed so far are recurrently mentioned within industry reports explaining how to attract app users (e.g., IBM Cloud Education 2020 ; Babich 2017 ; Payne 2021 ). Nonetheless, there is a limited understanding of which combinations of technological features and benefits sought most impact the intention to download and/or adopt an app. Such insights could originate from experimental studies shedding light on how consumers choose an app over alternatives. There is also scope for longitudinal analyses of which technological features most impact app market performance.
Individual characteristics
Several marketing studies catalogue individual consumer characteristics that drive the intention to adopt an app, stemming from a combination of personality traits theory (McCrae, Costa 1987 ; John and Srivastava 1999 ), consumer involvement theory (Richins and Bloch 1986 ; Mittal 1989 ), and the Theory of Planned Behavior (TPB) and the Theory of Reasoned Action (TRA) (Ajzen 1991 ; Azjen 1980 ). Combining these theories, it is possible to identify the following recurring drivers. First, we find studies highlighting the relevance of generic factors likely to influence consumer pre-dispositions at the early stages of any decision-making process, such as consumer involvement (Taylor and Levin 2014 ), inertia (Wang, Ou and Chen 2019 ), consumer experience (Lee and Kim 2019 ; Kim et al. 2013 ) and past behavior (Atkinson 2013 ; Ho 2012 ; Kang et al. 2015 ). We also find research highlighting the impact of behavioral control and self-efficacy (Kleijnen, de Ruyter and Wetzels 2007 ; Maity 2010 ; Sripalawat, Thongmak and Ngramyarn 2011 ; Wang, Lin and Luarn 2006 ), social norm (Hong and Tam 2006 ; Karaiskos et al. 2012 ; Lu et al. 2007 , 2008 ) and motives (Bruner and Kumar 2005 ). Second, we find research remarking the importance of key individual differences, such as consumer demographics (Yang 2005 ; Carter and Yeo 2016 ; Veríssimo 2018 ; Hur, Lee and Choo 2017 ), lifestyle (Kim and Lee 2018 ), personality (Xu, Peak and Prybutok 2015 ; Frey, Xu and Ilic 2017 ) and individual traits like innovativeness (Lu, Wang and Yu 2007 ; Liu, Yu and Wang 2008 ; Hur et al. 2017 ; Karjaluoto, Shaikh, Saarijärvi and Saraniemi 2019 ), optimism (Kumar and Mukherjee 2013 ) and mavenism (Atkinson 2013 ).
Despite the great emphasis on these aspects, there is a scope for new studies examining their implications for apps avoidance (i.e., not wanting to adopt an app) and apps resistance (i.e., opposing or postponing app adoption). Furthermore, it is crucial to investigate apps’ re-adoption , since many apps are downloaded but abandoned shortly afterwards (Baek and Yoo 2018 ). Such new research endeavors can shed further light on app abandonment caused by sampling (Roggeveen, Grewal and Schweiger 2020 ), meeting industry needs. In fact, industry reports lament that only one in four users use apps one day after the download, and within three months after download over 70% of the app users have churned (Kim 2019 ).
Route to introduction (strategies for encouraging app adoption)
Existing research exploring strategies that encourage app adoption primarily draws from industry trends, as opposed to empirical evidence or conceptual work (see Zhao and Balagué 2015 ). Hence, the need for new frameworks outlining and evaluating strategies for apps’ introduction is pressing. In particular, there is scope for empirical studies assessing the effectiveness of alternative market introduction strategies for different app types. For example, future research on pre-adoption of apps linked to existing brands could compare apps against other brand touchpoints (see also Peng et al. 2014 ; Wang et al. 2016a ). Similarly, future research on pre-adoption of standalone apps could concentrate on appraising the implications of the app’s marketing mix (discussed later on in this integrative review).
Adoption stage
The adoption stage of the customer journey for apps and via apps covers the continuation of the consumer decision-making process until app adoption, including any activities that signify adoption—e.g., behaviors resulting from using the app such as mobile shopping and in-app purchases . Table 2 combines theoretical approaches used to explore these aspects; it also lists key themes for future research, alongside examples of unanswered questions.
Continuation of the consumer decision-making process
Past studies focus on technological features and benefits sought, or on individual consumer characteristics also in relation to the pre-adoption stage. In relation to the first aspect, many scholars confirm the importance of the same pre-adoption drivers (e.g., ease of use, usefulness and enjoyment), either directly or via attitudes and/or intentions (see Gao, Rohm, Sultan and Pagani 2013 ; Huang, Lin and Chuang 2007 ; Koenig-Lewis, Marquet, Palmer and Zhao 2015 ; Veríssimo 2018 ; Stocchi, Pourazad and Michaelidou 2020a ). Past research also highlights new drivers such as mobility value (i.e., the combination of convenience, expediency and immediacy, see Huang et al. 2007 ) and ubiquity (i.e., the possibility to access products and services “anytime, anywhere”, see Tojib and Tsarenko 2012 ). Other drivers of app adoption and/or use include trust (Chong, Chan and Ooi 2012 ), device compatibility (Wu and Wang 2005 ), app price (Malhotra and Malhotra 2009 ), provider reputation (Chandra et al. 2010 ), consumer experiential learning (Grant and O’Donohoe 2007 ) and perceived media flow (Wu and Ye 2013 ). In terms of individual characteristics, extant studies confirm the same range of factors as in pre-adoption (Mort and Drennan 2007 ; Bhave, Jain and Roy 2013 ; Byun, Chiu and Bae 2018 ; Taylor, Voelker and Pentina 2011 ; Yang 2013 ; Kang et al. 2015 ). Research also highlights the importance of consumer motives (Jin and Villegas 2008 ), social influence (Chong et al. 2012 ), attachment with the device (Rohm, Gao, Sultan and Pagani 2012 ) and self-to-app connection (Newman, Wachter and White 2018 ). Additionally, some studies reveal further important individual level factors such as consumer innovativeness (Lewis et al. 2015), consumer knowledge (Koenig-Lewis et al. 2015 ), personality (Pentina, Zhang, Bata and Chen 2016 ; Fang 2017 ) and a sense of self (Scholz and Duffy 2018 ). Moreover, several studies uncover new drivers such as escapism (Grant and O’Donohoe 2007 ), playfulness and drive stimulation (Mahatanankoon, Wen and Lim 2005 ). There are also studies highlighting the importance of usage values (Liu, Zhao and Li 2017 ) and advantages (Zolkepli et al. 2020 ; Newman, Wachter and White 2018 ; Arya, Sethi and Paul 2019 ), including information needs (Alavi and Ahuja 2016 ) and usage preferences (Doub, Levin, Heath and LeVangie ( 2018 ); Cheng, Fang, Hong and Yang 2017 ) such as browsing (Kim, Kim, Choi and Trivedi 2017 ).
The range of theoretical approaches underpinning the research mentioned so far is broad. For example, we find theories not explored for pre-adoption like experiential learning theory (Kolb 1984 ), media flow theory (Wu and Ye 2013 ), motivation theory (Herzberg, Mausner and Bloch-Snyderman 1959 ) and the self-concept (Sirgy 1982 ). Nonetheless, a common aspect connecting these seemingly disparate theoretical bases is the notion of value. Specifically, there is an emphasis on different types of consumer values (e.g., utilitarian and hedonic) assumed to encourage the shift from the intention to adopt an app to actual adoption and/or use. Although this assumption is plausible and empirically sound, there is scope for new investigations outlining the consumer decision-making process resulting in app adoption and/or use in greater detail. For instance, scholars could adapt conceptual frameworks explicating how consumers evaluate brands for choice (e.g., Keller 1993 ).
Behaviors that signal adoption
The industry distinguishes 33 app categories in the Google Play store and 24 categories in the Apple’s App Store, out of which popular app categories (i.e., categories with an uptake greater than 3%) include apps linked to retailers, games and lifestyle apps (Think Mobile 2021 ). Considering these popular app categories, two key behaviors signaling adoption echo the focus of extant marketing studies: mobile shopping via apps and in-app purchasing .
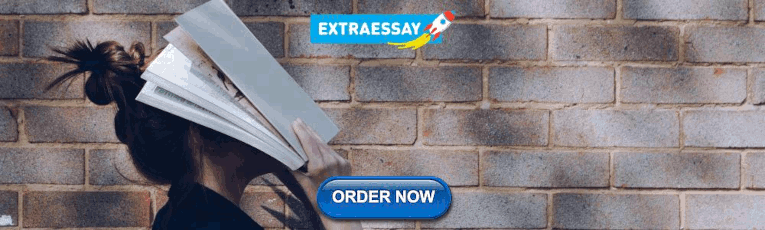
Mobile shopping
Past research clarifies the factors that encourage purchasing via the app and the intention to purchase the brand powering the app (when the app is linked to an existing offline or online brand). In terms of factors that encourage purchases via the app vs. other channels, extant studies identify the importance of positive customer experiences , especially the speed of transactions, security and user-friendliness that apps can provide (Buellingen and Woerter 2004 ; Figge 2014 ); consumer participation, flexibility and technology quality (Mäki and Kokko 2017 ; Dacko 2017 ); location awareness and interactivity (Wang et al. 2016a ); and access to information and promotions (Magrath and McCormick 2013 ). Extant research also discusses the relevance of the customer’s overall interest in the app (Taylor and Levin 2014 ) and specific apps’ attributes (e.g., ease of use and connection with the self) as drivers of the intention to purchase via the app over the physical store (Newman et al. 2018 ), often due to heightening buying impulses (Wu and Ye 2013 ; Chadha, Alavi and Ahuja 2017 ). Finally, past studies highlight two factors that underpin the intention to purchase the brand powering the app: the provision of holistic brand experiences (Wang and Li 2012 ; Kim and Yu 2016 ; Chen 2017 ; Fang 2017 ) and and app usability (i.e., “the extent to which a mobile app can be used to achieve a specified task effectively during brand-consumer interactions” Baek and Yoo 2018 , p. 72).
Considering the above, more research is needed to clarify how purchases via apps occur, including any facilitating or inhibiting factors, as these strongly correspond with industry priorities. Indeed, industry experts call for more insights on how personalized content and push notifications might encourage purchasing via the app (Anblicks 2017 ; Tariq 2020 ). Such future research extensions could also reinforce rather scattered theoretical bases, which primarily include expectancy theory (Vroom 1964 ), motivation theory (Herzberg et al. 1959 ), Uses and Gratifications (U&G) theory (Mcguire 1974 ) and customer satisfaction theory (Churchill Jr. and Surprenant 1982 ). Finally, in terms of apps attached to existing brands, future research could evaluate the impact on brand sales and/or other brand performance indicators. For example, future studies could consider the effects of apps as a tool to enhance a brand’s availability in consumer’s memory, ultimately impacting brand purchase intentions (see Sharp 2010 ; Romaniuk and Sharp 2016 ).
In-app purchasing
Research predicting in-app purchases highlights, as key drivers, perceived app value (i.e., quality, value for money, social and emotional value—see Hsu and Lin 2015 ; and Hsiao and Chen 2016 ) and features of the app that motivate app use (Stocchi, Michaelidou, Pourazad and Micevski 2018 ; Stocchi et al. 2019 ). Extant studies also remark the importance of personality traits such as bargain proneness, frugality and extraversion (Dinsmore, Swani and Dugan 2017 ), and price sensitivity , which Natarajan et al. ( 2017 ) found to alter perceptions of risk, usefulness, enjoyment and personal innovativeness, via customer satisfaction (see also Kübler, Pauwels, Yildrim and Fandrich 2018 ). Since the conceptual focus and scope of extant studies is somewhat confined, future research could expand the theoretical bases used by considering established patterns and regularities in buying behavior (see the work of Sharp 2010 ; and Romaniuk and Sharp 2016 ).
Post-adoption stage
The post-adoption stage concerns two aspects: ongoing or continued app usage, explored through the notions of stickiness and engagement ; and outcomes of app adoption for the app itself and for the brand behind the app , as applicable. Table 3 maps extant theoretical approaches vs. outstanding research themes and priorities linked to these aspects, with examples of research questions yet to be explored (Fig. 1 ).
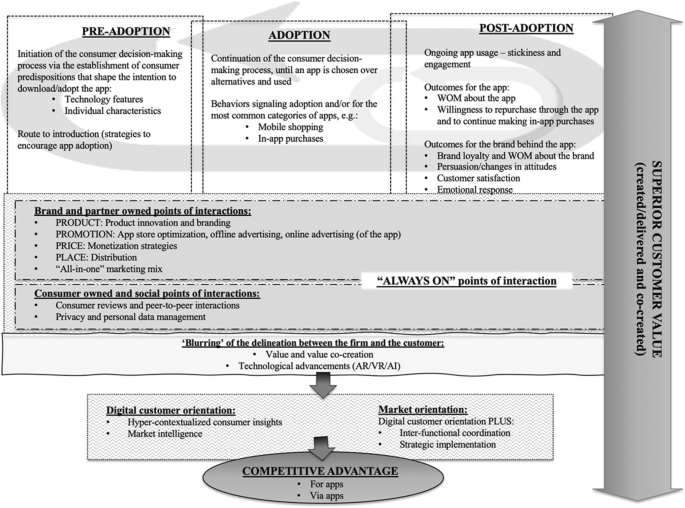
Unified theoretical framework
Ongoing (continued) app usage
App stickiness.
Racherla, Furner and Babb ( 2012 ) and Furner, Racherla and Babb ( 2014 ) link app stickiness to telepresence , which comprises two dimensions: vividness and interactivity . Vividness influences a medium’s ability to induce a sense of presence resulting from its breadth (sensory dimensions and cues) and depth (quality of presentation). Interactivity is the extent to which users can modify the medium’s form and content in real-time. Similarly, Chang ( 2015 ) and Xu et al. ( 2015 ) explore loyalty towards apps focusing on perceived value and customer satisfaction. Other studies concentrate on the continued intention to use an app , highlighting the importance of consumer perceptions of apps’ features (Kim, Baek, Kim and Yoo 2016 ), especially design, functionality and social features (Tarute, Nikou and Gatautis 2017a ). For example, Tseng and Lee ( 2018 ) confirm that improving loyalty towards branded apps can be achieved through an affective path (i.e., bolstering functional, experiential, symbolic and monetary benefits) and a utilitarian path (i.e., emphasizing system and information quality). Similarly, Alalwan ( 2020 ) links performance expectancy and hedonic motivation to the continued intention to use apps.
The above studies draw upon different theoretical bases, albeit consistently highlighting the importance of value perceptions resulting from customer experiences. Nonetheless, past research bears two recurring issues: inconsistent conceptualizations and measurements, and the conflation with other prominent notions such as app engagement. These two issues could be turned into future research providing a unified definition and measure of app stickiness. Future research could also explore the outcomes of app stickiness, clarifying if it can improve apps’ market performance and survival chances. Lastly, there is scope for longitudinal studies examining fluctuations in app stickiness, especially pre and post app modifications. Notably, these future endeavors all yield significant synergies with current industry practices and trends (see App Radar 2019 , The Manifest 2018 ).
App engagement
According to Kim et al. ( 2013 ) and Wang et al. ( 2016b ), app engagement can be understood as the sum of motivational experiences (see also Calder and Malthouse 2008 ) that connect the consumer to the app. Similarly, Dovaliene, Masiulyte and Piligrimiene ( 2015 ) and Dovaliene, Piligrimiene and Masiulyte ( 2016 ) theorize consumer engagement with apps as a mixture of cognitive, emotional and behavioral aspects (see also Jain and Viswanathan 2015 ), while Noh and Lee ( 2016 ) link consumer intention to engage with apps to perceptions of quality . Adapting Calder, Malthouse and Schaedel’s ( 2009 ) measure of media engagement , Wu ( 2015 ) confirms that effort expectancy, performance expectancy, social influence and consumer-brand identification underpin consumer engagement, which then drives the intention to continue app usage. In contrast, Kim and Baek ( 2018 ) use Kilger and Romer’s ( 2007 ) measure of media engagement to evaluate branded apps engagement. This approach closely aligns with Eigenraam, Eelen, van Lin, and Verlegh’s ( 2018 ) definition of digital engagement , which captures consumers’ tendency to conduct various tasks beyond usage of branded services, displaying behaviors that signal engagement. In a similar vein, Tarute, Nikou and Gatatuis ( 2017a ) modify Hollebeek, Glynn and Brodie’s ( 2014 ) work and contend that engagement with apps originates from the intensity of individual participation and motivation (see also Vivek, Beatty and Morgan 2012 ). Stocchi et al. ( 2018 ) explore consumer motives for engaging with apps, while Fang, Zhao, Wen and Wang ( 2017 ) consider branded apps’ characteristics that underpin psychological engagement (i.e., a highly subjective state characterized by deep focus, concentration and absorption), assumed to drive behavioral engagement (i.e., the consumer intention to engage with the branded app). Past studies also analyze consumer engagement behaviors (i.e., manifestations towards the brand or the firm beyond purchase that strengthen the consumer-brand relationship and generate value, see van Doorn, Lemon, Mittal, Nass, Pick, Pirner and Verhoef 2010 ). For example, Viswanathan, Hollebeek, Malthouse, Maslowska, Kim and Xie ( 2017 ) infer app engagement from the behavior changes of customers enrolled in the loyalty program. Gill, Sridhar and Grewal ( 2017 ) return similar findings for B2B apps. Lee ( 2018b ) and van Heerde, Dinner and Neslin ( 2019 ) highlight that consumer engagement behaviors have a strong bearing on brand loyalty. Finally, Chen ( 2017 ) and Fang ( 2017 ) predict engagement with the brand powering the app.
In essence, existing research on apps’ engagement presents contrasting assumptions and conceptualizations, which place emphasis on different cognitive and psychological aspects resulting from an evaluation of the benefits (and thus values) that apps offer. Therefore, there is scope for a unified definition and measurement of app engagement combining diverging theoretical perspectives such as motivation theory (Herzberg et al. 1959 ), flow theory (Wu and Ye 2013 ), transportation theory (Green and Brock 2000 ), media engagement theory (Kilger and Romer 2007 , Calder and Malthouse 2008 ) and the Customer, Value, Satisfaction and Loyalty (VSL) framework (Lam, Shankar, Erramilli and Murthy 2004 ; Yang and Peterson 2004 ). Meeting recurring industry priorities (Beard 2020 ; Marchick 2014 ; Facebook 2021 ), future research could aso explore disengagement —i.e., when consumers de-escalate the frequency of app usage (see also Wang et al. 2016c ), as well as the link between app engagement and other apps performance indicators such as downloads.
Outcomes for the app
Extant research exploring the outcomes of app adoption for the app itself concentrates on two key aspects: the willingness to spread word-of-mouth (WOM) about the app and the willingness to re-purchase via the app . For example, Furner, Racherla et al. (2014) attribute consumer willingness to spread positive WOM about mobile apps to the app’s stickiness. In a similar vein, Baek and Yoo ( 2018 ) link branded apps’ continued usage intention to branded apps’ referral intentions. Embracing a different conceptual angle, Xu et al. ( 2015 ) highlight the link between perceptions of app value, satisfaction with the app, loyalty towards the app and WOM about the app, which the authors consider to be a form of experiential computing . Other studies attribute the consumer’s inclination to recommend apps to the level of app loyalty resulting from perceptions of value (Chang 2015 ) or service quality (Chopdar and Sivakumar 2018 ). On occasion, past research explores specific characteristics of branded apps likely to entice WOM such as usefulness (Kim et al. 2016 ), ease of use and personal connection (Newman et al. 2018 ), and utilitarian and hedonic benefits (Stocchi et al. 2018 ). In terms of the willingness to re-purchase via the app and other mobile shopping changes, Kim et al. ( 2015 ), Wang, Xiang, Law and Ki ( 2016a ) and Gill et al. ( 2017 ) demonstrate that using an app increases spending over time. In light of these findings, research on the outcomes of app adoption for the app reveals substantial scope for expansion. In particular, future research could explore the underlying mechanisms linking perceptions of value (especially value in use), satisfaction with the app and outcomes beyond the standard chain of effects leading to WOM and/or other forms of loyalty toward the app.
Outcomes for the brand behind the app
Research exploring the outcomes for the brand behind the app covers a wide range of conceptual bases, including persuasion theory (Petty and Cacioppo 1986 ), involvement theory (Richins and Bloch 1986 ; Mittal 1989 ), self-congruence theory (Aaker 1999 ; Sirgy, Lee, Johar and Tidwell 2008 ) and consumer-brand relationship theory (Fournier 1998 ). Nonetheless, given the theoretical and managerial relevance of these aspects, there is ample scope for new marketing knowledge, as follows.
Brand loyalty
Lin and Wang ( 2006 ) theorize brand loyalty as the outcome of perceived value, customer satisfaction, trust and habits inherent to m-commerce apps. Similarly, Kim and Yu ( 2016 ) evaluate the extent to which branded apps can drive brand loyalty through the provision of a continuous brand experience, which they defined as “sensation, feelings, cognition and behavioral responses evoked by brand-related stimuli that are all a part of a brand’s design, identity, packaging, communication, and environment” (p.52). Embracing a slightly different focus, Baek and Yoo ( 2018 ) focus on branded apps’ usability, seen as conceptually woven into the user experience. Therefore, building upon these past studies and their implications, future research could focus on the psychological mechanisms that increment brand loyalty via app usage. For example, keeping in mind the established conventions of how brands grow (Sharp 2010 ; Romaniuk and Sharp 2016 ), there is scope for investigating app characteristics likely to enhance brand loyalty for different customer segments. There is also scope for research exploring the reverse effect, i.e. studies evaluating the impact of brand loyalty on app performance.
Willingness to spread WOM about the brand
Kim and Yu ( 2016 ) attribute consumer’s willingness to spread positive WOM about the brand powering an app to the holistic brand experience resulting from using the app. Similarly, Sarkar, Sarkar, Sreejesh and Anusree ( 2018 ) link positive WOM about retailers to the use of related apps. To revamp scholarly and managerial attention around this theme, future studies could establish a connection with the latest online WOM research (e.g., Ismagilova, Slade, Rana and Dwivedi 2019 ; Sanchez, Abril and Haenlein 2020 ; Rosario, de Valck and Sotgiu 2020 ). Such studies could also consider instances whereby buzz about the brand might impact app performance.
Wang et al. ( 2016a ) present a series of theoretical reflections concerning the persuasive nature of branded apps, highlighting apps’ ability to trigger frequent context-based brand recall. Bellman, Potter, Treleaven-Hassard, Robinson and Varan ( 2011 ) add that branded apps can persuade consumers by increasing interest in the brand powering the app (purchase intention) and in the product category (product involvement). At the same time, Ahmed, Beard and Yoon ( 2016 ) remark that apps’ persuasive potential originates from vividness, novelty, and multi-platforming opportunities (see also Kim et al. 2013 ). Similarly, Alnawas and Aburub ( 2016 ) and Seitz and Aldebasi ( 2016 ) attribute apps’ persuasiveness to the benefits offered, which can be cognitive (information acquisition), social integrative (connecting with others), personal integrative (self-value bolstering) and hedonic (e.g., escapism). More recently, Lee ( 2018a ) examines the dual route to persuasion for apps, including argument quality (central route) and source credibility (peripheral route), while van Noort and van Reijmersdal ( 2019 ) evaluate cognitive and affective brand responses to apps.
In line with the above, apps’ persuasive power is widely established, a trend that is also apparent in mobile advertising trends (via apps and in-apps), which continue to overtake desktop advertising (eMarketer 2019). Nonetheless, there is scope for new knowledge evaluating the outcomes of advertising via apps beyond attitude change and brand purchase intentions (see Ahmed et al. 2016 ), explicitly appraising apps’ effects on brand recall and brand recognition (see Ström et al. 2014 ; van Noort and Reijmersdal 2019 ). There is also scope for replications and extensions of Bellman et al.’s ( 2011 ) seminal work, bringing neuroscience into marketing research on apps. For example, future research could determine the most persuasive app features for different consumer segments. It is equally paramount to consider the effects of deploying apps compared to other advertising channels. Such comparisons could evaluate synergies between apps and other digital media (especially social media), guiding firms in advertising platform choices whilst avoiding unduly media duplication. Future studies could also explore the impact of brand advertising on app performance. These future investigations are relevant to the industry, as apps are considered superior advertising channels than websites (Deshdeep 2021 ).
Customer satisfaction
Lin and Wang ( 2006 ) attribute customer satisfaction to perceptions of app value and consumer trust. Subsequent studies often refer to these original findings, albeit returning either too simplistic (Lee, Tsao and Chang 2015 ) or too intricate research frameworks (Xu et al. 2015 ), or frameworks not focused on the prediction of customer satisfaction (Natarajan et al. 2017 ). Other studies concentrate on utilitarian and hedonic benefits that apps offer vs. non-monetary sacrifices such as privacy surrender (Alnawas and Aburub 2016 ). In contrast, Alalwan ( 2020 ) considers online reviews, performance expectancy, hedonic motivation and price value. Among studies exploring perceptions of value and customer satisfaction, Chang ( 2015 ) looks at emotional and social values, app quality and value for money. Likewise, Rezaei and Valaei ( 2017 ) find that experiential values (i.e., service excellence, customer return on investment, aesthetics and playfulness) positively influence satisfaction. In contrast, Iyer, Davari and Mukherjee ( 2018 ) find that both functional and hedonic values positively influence consumer satisfaction from the branded app, while social values have a negative impact (see also Karjaluoto et al. 2019 ).
Considering the above and, more generally, the pivotal role of perceptions of value seen in extant research on pre-adoption and adoption, there is limited ground for additional endeavors exploring these aspects. However, there is a need for research clarifying how to measure service quality for apps and evaluating the differences with other non-digital sources of customer satisfaction . In fact, only two studies have explored these aspects, proposing inconsistent models. Specifically, Demir and Aydinli ( 2016 ) outline seven dimensions of service quality for instant messaging apps (communication, data transferring, distinctive features aesthetics, security, feedback, and networking), while Trivedi and Trivedi ( 2018 ) explore the antecedents of satisfaction with fashion apps adding other perceived quality dimensions. There is also scope for new research exploring the on-going effects of attaining brand engagement via apps, expanding the exploratory work by Chen ( 2017 ) on brands active on WeChat. Finally, it is worth exploring instances whereby customer satisfaction with the brand and brand engagement might influence app performance.
Emotional response toward the brand
When interacting with mobile technologies, users often experience strong emotional responses, which can result in the willingness to act without thinking (McRae, Carrabis, Carrabis and Hamel 2013 ). Indeed, van Noort and van Reijmersdal ( 2019 ) show that entertaining apps heighten affective brand responses and, according to Arya et al. ( 2019 ), consumers might become brand vocals. Moreover, apps can trigger emotional connections between the consumer and the brand, on the basis of self-congruence (Iyer et al. 2018 ; Kim and Baek 2018 ; Yang 2016 ) or self-app connection , arising from personalized consumption experiences that turn apps into digital manifestations of one’s preferences, desires and needs (Newman et al. 2018 ). Apps can also lead to brand attachment (i.e., an emotional bond between the consumer and the brand); brand identification (i.e., overlap between the consumer and the brand, see Peng et al. 2014 ); brand affect (i.e., deep emotions towards the brand, see Sarkar et al. 2018 ); brand love (i.e., a romantic connection between the brand and the consumer, see Baena 2016 ); and brand warmth (i.e., the belief that a brand is friendly, trustworthy and truthful, see Fang 2019 ). Building upon these findings, there is an opportunity to examine the cognitive and affective brand responses that result from using different types of apps (see also van Noort and van Reijmersdal 2019 ) and how these might impact app performance. Such studies could return relevant insights useful to the identification of strategies for market survival and attaining a competitive advantage for apps through building strong connections with consumers.
“Always on” points of interaction
Research linked to brand and partner-owned , and consumer-owned and social “always on” points of interaction is nascent, yet very important to understand how to shape positive and interative customer journeys with apps and via apps. Table 4 integrates extant conceptual approaches, which include the Innovation Diffusion Theory (Rogers 1995 ); personality traits theory (McCrae, Costa 1987 ; John and Srivastava 1999 ) and value network theory (Peppard and Rylander 2006 ). It also highlights key priority future research themes and questions.
Brand and partner owned “always on” points of interaction
In accordance with Tong, Luo and Xu ( 2020 ), brand and partner owned “always on” points of interaction are linked to the four standard elements of the marketing mix , as follows.
Product (including innovation and branding)
Existing research exploring how apps promote innovation and how to innovate apps is very limited. A few noteworthy exceptions include studies about apps used in specific industries such as construction and higher education—see Lu, Mao, Wang and Hu ( 2015 ); Wattanapisit, Teo, Wattanapisit, Teoh, Woo and Ng ( 2020 ); Liu, Mathrani and Mbachu ( 2019 ); and Pechenkina ( 2017 ). However, product innovation research often discusses it in relation to technological developments (Toivonen and Tuominen 2009 ). Therefore, since mobile technologies are subject to ongoing and rapid technological advancements (Lamberton and Stephen 2016 ), there is scope for new research empirically evaluating the impact of innovating apps’ technological features. For example, with the advent of apps involving augmented and virtual reality, there is room for studies quantifying the effect of these advancements on downloads and engagement and mobile shopping (in app and via the app). More broadly, more research is needed to reveal the mechanisms through which apps catalyze innovation to generate value for different stakeholders (Snyder, Witell, Gustafsson, Fombelle and Kristensson 2016 ; Shankar, Kleijnen, Ramanathan, Rizley, Holland and Morrissey 2016 ). Indeed, it has been argued that apps facilitate the establishment of two-way dialogues between the end-user and key stakeholders (Wong, Peko, Sundaram, and Piramuthu 2016 ).
Similarly to extant research on app innovation and innovation via apps, studies exploring apps as a branded digital offering or studies clarifying the implications of branding apps are also limited. This is surprising, since Sultan and Rohm ( 2005 ) define apps as a ‘ brand in the hand ’. Similarly, Smutkupt, Krairit and Esichaikul ( 2010 ) and Urban and Sultan ( 2015 ) argue that mobile technologies offer excellent opportunities for enhancing a brand’s image. Moreover, explicit links between apps and branding objectives appeared in the literature following Bellman et al.’s ( 2011 ) formal definition of branded apps and Taivalsaari and Mikkonen’s ( 2015 ) definition of ‘ brandification ’ of apps—i.e., custom-built native apps that enable seamless customer experiences. For example, Stocchi, Guerini and Michaelidou, ( 2017 ) link the image of branded apps to their market penetration, while Stocchi, Ludwichowska, Fuller and Gregoric ( 2020a ) propose and validate a simple brand equity framework for apps (c.f. Keller 1993 ). Accordingly, there is room for new empirical research exploring the implications of branding apps. For instance, future studies could explore the implications of branding and/or extending apps and thus apps’ portfolio management, which is crucial for navigating increasing app competition (Jung et al. 2012 ). The literature is also missing clarity on what information consumers hold in memory in relation to apps, and how these memories impact knowledge of the app and of the brand powering the app (see also van Noort and van Rejmersdal 2019 ).
Adding to the above, the industry discusses several practices to promote apps (Saxena 2020 ; Fedorychak 2019 )—e.g., App store optimization via keywords and the inclusion of screenshots and videos for greater conversion rate (Karagkiozidou, Ziakis, Vlachopoulou and Kyrkoudis 2019 ; Padilla-Piernas et al. 2019 ), or the use of push notifications (Srivastava 2017 ; Clearbridge Mobile 2019 ). At the same time, some studies highlight the power of promoting apps via influencers (Hu, Zhang and Wang 2019 ) or via leveraging user reviews and ratings (Ickin, Petersen and Gonzalez-Huerta 2017 ; Kübler et al. 2018 ; Numminen and Sällberg 2017 ; Hyrynsalmi, Seppänen, Aarikka-Stenroos, Suominen, Järveläinen and Harkke 2015 ; Liu, Au and Choi 2014 ). Nonetheless, there is a limited understanding of the implication and effectiveness of promoting apps via these methods. In particular, there is limited knowledge on the effects of advertising apps offline (e.g., via TV advertisements) and online (e.g., on social media or display advertising).
Research on pricing strategies for apps is a line of enquiry of its own merit, which started with Dinsmore, Dugan and Wright’s ( 2016 ) work exploring the effectiveness of monetary vs. nonmonetary (e.g., data provision) tactics to cue an app’s novelty; and Dinsmore, Swani and Dugan’s ( 2017 ) research testing whether personality traits drive the willingness to pay for apps and the willingness to make in-app purchases (see also Natarajan et al. 2017 and Kübler et al. 2018 studies on the implications of price sensitivity for app success). More recently, Arora et al. ( 2017 ) clarify that the presence of a free version of the app (sampling) reduces the speed of adoption, and Appel, Libai, Muller and Shachar ( 2020 ) also discuss issues inherent to apps’ sampling. Nonetheless, there is scope for more research on improving apps’ monetization and on maximizing the chance of market survival. For instance, future research could evaluate the trade-off between apps’ pricing strategies and other marketing mix elements, especially apps’ advertising and promotion. There are also opportunities for experimental research evaluating the effects of different monetization tactics for different app types. Lastly, although freemium pricing strategies (i.e., free basic app version with subsequent payable upgrades, Arora et al. 2017 ) are very common, they may not always be a feasible option. Likewise, the decision to market apps at a price may be quite counterproductive in light of the multitude of free alternatives.
Distribution
Although often exceeding the confines of marketing research, there is established knowledge concerning the distribution of apps. For example, Cuadrado and Dueñas ( 2012 ) stress the importance of the value network, which includes providers, consumers, platforms, telecommunications, social networks and remote service providers. Within this network, critical factors include feedback, innovation, service quality, device compatibility, ready-to-use services and interfaces (e.g., for data storage, security, automatic updates, notifications and billing), and developers’ diversity. Jung et al. ( 2012 ) highlight the relevance of the profit-sharing model of apps’ stores and the review mechanisms, which counteract low entry barriers. Oh and Min ( 2015 ) also emphasize the importance of app stores given the increasing pressure for monetization, while Wang, Lai and Chang ( 2016b ) explore different strategies for app competition. At the same time, Roma and Ragaglia ( 2016 ) revealed differences in monetization effectiveness across the two leading app stores (Google Play and Apple’s AppStore). Finally, Martin, Sarro, Jia, Zhang and Harman ( 2017 ) consider app stores as a channel for communications and feedback crucial to market survival. Hence, although extant research has established that the distribution of apps is bound to the app store’s business model, the need for research clarifying app store’s role in the competitive success of apps is pressing. In particular, future studies could introduce new paradigms for supply chain management and channel integration based on gathering and sharing large amounts of highly-contextualized consumer insights.
Different marketing mix configurations
Besides significant expansions of research considering the four elements of the marketing mix for apps, there is scope for studies exploring different marketing mix configurations. For example, according to Tong et al. ( 2020 ), mobile technologies’ marketing mix includes an element of prediction (i.e., the elaboration of considerable amounts of consumer insights), with all elements of the marketing mix enriched by opportunities for personalization . Moreover, since apps are ‘all-in-one’ gateways (Grewal, Hulland, Kopalle and Karahanna 2020 ) for the asynchronous provision of products and services whereby promotion and distribution are often combined, future research could determine the extent to which apps’ marketing mix elements are somewhat conflated.
Consumer-owned and social “always on” points of interaction
Consumer reviews and peer-to-peer interactions.
Although lacking in explicit theoretical grounding, past research confirms that consumer reviews reflect users’ experience with the app, questions and bug reports (Genc-Nayebi and Abran 2017 ). Indeed, reviews influence the decision to install and use an app (Ickin et al. 2017 ; Jung et al. 2012 ; Kübler et al. 2018 ; Numminen and Sällberg 2017 ), and the willingness to purchase an app (Huang and Korfiatis 2015 ; Hyrynsalmi et al. 2015 ; Liu et al. 2014 ). Past studies also highlight the impact of negative reviews (Huang and Korfiatis 2015 ), linking the volume and valence of reviews to app’s sales (Hyrynsalmi et al. 2015 ; Liang, Li, Yang and Wang 2015 ). Nonetheless, there is scope for future research exploring the impact of peer-to-peer interactions, embracing new conceptual perspectives such as social contagion (Iyengar, Van den Bulte and Valente 2011 ) and network effects theory (Katona, Zubcsek and Sarvary 2011 ). Future research could also examine apps’ role as catalyst of online communities, meeting industry calls for more clarity on how to attain synergies between apps and other crucial aspects of digital marketing (e.g., social media). Finally, from a methodological point of view, there is scope for qualitative research evaluating the social and personal implications of consumer views on apps, adopting lesser explored conceptual lenses such as the notion of the extended self (Belk 1988 ) or product symbolism (Elliott 1997 ; Richins 1994 ). Second, given the obvious differences in the uptake and popularity of apps across different areas of the world, this is a paramount line of future enquiry to evaluate likely cultural differences across all elements of the customer journey. For instance, future studies could evaluate the effects of standard cultural variations in basic demographic features such as age and gender (see McCrae 2002 ) and the impact of country-level cultural orientations (e.g., in line with Hofstede’s traits, see Johnson, Kulesa, Cho and Shavitt 2005 ) across all stages of the customer journey with apps, since they are known to impact individual responses and behaviours in numerous settings. Similarly, future studies could examine the impact of individual-level differences linked to specific personality traits that characterise certain cultures across the full customer journey with apps. This is a promising future research avenues, since personality traits have numerous psychological implications (e.g., in terms of cognitive styles—see Oyserman, Coon and Kemmelmeier 2002 , and cognitive processes—see Nisbett, Peng, Choi and Norenzayan 2001 ).
Privacy and personal data management
Privacy in mobile marketing practices is often seen as a result of perceived benefits, which mitigate perceptions of risks and personal data management concerns (Grewal et al. 2020 ). In line with this view, past studies describe privacy as a risk that impacts the intention to use mobile commerce (Wu and Wang 2005 ) and specific types of apps such as banking apps (Koenig-Lewis et al. 2015 ). Similarly, Sultan, Rohm and Gao ( 2009 ) examine privacy in relation to the risk inherent to mobile marketing acceptance, and Gao et al. ( 2013 ) identify privacy as a potential loss when adopting mobile devices. In contrast, Lu et al. ( 2007 ) consider privacy, security and opting out as reflections of trust in wireless environments, a view that led studies evaluating privacy in relation to apps theorize it as a key driver of adoption and/or usage resulting from consumer trust—see Morosan and DeFranco ( 2015 , 2016 ). Indeed, Miluzzo, Lane, Lu and Campbell ( 2010 ) stress the significance of enabling users to control privacy settings . As a result of such contrasting assumptions, besides exacerbating the lack of clarity surrounding privacy in the broader marketing literature (Tan, Qin, Kim and Hsu 2012 ), extant research provides limited insights on the implications of privacy, loss of privacy and security (i.e., privacy risk) for apps. Hence, there is a clear need for future research clarifying the notion of app privacy—a need, which matches important transnational industry trends to create clear guidelines for personal data collection and usage (see the key issues highlighted in the GDPR guidelines, Gdpr-info.eu 2018). Above all, exploring the acceptable trade-off between apps’ functionality and ubiquity for the secure management of consumer personal data are promising areas of future research. To explore these aspects, future studies could draw upon relevant unexplored conceptual bases such as social justice (Tyler 2020 ) and ethics theory (Yoon 2011 ).
‘Blurring’ of the delineation between the firm and the customer
For the customer journey stages and “always on” points of interaction to translate into a digital customer orientation, it is essential to consider extant knowledge that explores apps’ potential in attenuating the divide between the firm and the customer, shaping unique customer experiences; for example, via value creation and co-creation , and consumer response to app technological advancements . Table 5 lists existing theoretical approaches deployed to investigate these aspects, together with priority future research themes and questions worth exploring.
Value creation and co-creation
As previously discussed, the role of perceptions of values in the pre-adoption decision-making process, and in promoting the continuation of the cognitive, affective and behavioral processes inherent to adoption and post-adoption is well-established. Moreover, conceptual research (e.g., Zhao and Balagué 2015 ) clearly highlights apps’ great potential for value creation . Nonetheless, with a few exceptions (e.g., Ehrenhard, Wijnhoven, van den Broek and Stagno 2017 ; Kristensson 2019 ; Lei, Ye, Wang and Law 2020 ), explicit conceptual and/or empirical assessments of apps’ effectiveness for value creation are limited. This is surprising, since Larivière et al. ( 2013 ) suggest that mobile touchpoints trigger a fusion of value , which can simultaneously benefit shoppers, employees and companies. Moreover, Lei et al. ( 2020 ) show that, in hospitality, apps facilitate value co-creation by virtue of media richness. A possible reason for the marketing research scarcity in this domain could be the use of a narrow range of theoretical bases. In particular, besides the use of the Dynamic Business Capabilities (DBC) theory (Wheeler 2002 ), channel expansion theory (Carlson and Zmud 1999 ) and generic theoretical frameworks evaluating the links between perceptions of value and customer satisfaction (Lin and Wang 2006 ), there is an absence of research adapting standard customer value theories (e.g., Woodside, Golfetto and Gilbert 2008 ) and value fusion theory (e.g., Larivière et al. 2013 ). There is also scope for research clarifying how apps facilitate value co-creation and the marketing potential of co-created apps —i.e., apps shaped through the direct involvement of consumers (see Gokgoz, Ataman and van Bruggen 2021). Indeed, Dellaert ( 2019 ) contends that consumer co-production plays a fundamental role in making companies rethink the value creation process. This view matches the service-dominant logic (see Vargo and Lusch 2004 ; Zhang, Lu and Kizildag 2017 ), whereby consumers use resources available to them to experience and co-create value (Grönroos 2019 ). Thus, scholars could research antecedents and outcomes of value creation and co-creation via apps, exploring in detail the appscape (see also Tran, Mai and Taylor 2021 ). More research is also warranted to understand how apps are used during value exchanges (e.g., in shopping centers, see Rauschnabel et al. 2019 ) and after value exchanges (e.g., to mitigate purchase regret, see Wedel et al. 2020 ).
Technological advancements
Extant research contends that technological advancements such as Artificial Intelligence (AI), Augmented Reality (AR) and Virtual Reality (VR) in apps provide highly customized experiences, impacting consumer preferences and behaviors (Huang and Rust 2017 ; Pantano and Pizzi 2020 ). For example, AR-enabled apps improve consumer perceptions of utilitarian and hedonic benefits (Nikhashemi et al. 2021 ), encourage positive attitudes (Yaoyuneyong et al. 2016 ; Wedel et al. 2020 ), and boost purchase intentions and WOM (Yaoyuneyong et al. 2016 ) through enjoyment (Rauschnabel et al. 2019 ). Similarly, VR apps elicit positive brand affect by provoking strong sensory reactions such as perceptions of tangibility via haptic vibrations (Wedel et al. 2020 ). Additionally, through the use of anthropomorphic cues (i.e., human traits assigned to computers, see Nass and Moon 2000 ), apps enhance user interactions (Alnawas and Aburub 2016 ) thanks to a humanized customer experience, which influences how consumers perceive the brand attached to the app (van Esch et al. 2019 ; Olson and Mourey 2019 ) and increases trust irrespective of privacy concerns (van Esch et al. 2019 ; Ha et al. 2020 ). Although on par with current industry trends (the global VR/AR app market is considered one of the most rapidly growing domains of software development see Unity Developed 2021), this stream of research has not exhaustively evaluated the effects of apps’ technological advancements on consumer experiences. Arguably, this knowledge void is caused by dated theoretical bases such as the diffusion of innovation (Rogers 1995 ), the Uses and Gratification (U&G) theory (Mcguire 1974 , Eighmey and McCord 1998 ) and the Technology Continuance Theory (TCT) (Liao, Palvia and Chen 2009 ). Hence, future research could embrace new theoretical angles like the physical and psychological continuity theory (Lacewing 2010 ), teletransportation theory (Langford and Ramachandran 2013 ) and service prototyping theory (Razek et al. 2018 ).
Digital customer orientation and competitive advantage
- Digital customer orientation
Hyper-contextualized consumer insights
The pervasive nature of mobile technologies generates unprecedented opportunities for hyper-contextualized consumer insights , which include “at which locations consumers are using their mobiles (where), what times they are looking for products (when), how they search for information and complete purchases (how), and whether they are alone or with someone else when using mobile devices (with whom)” (Tong et al. 2020 , p. 64). Indeed, due to their built-in features, apps allow gathering, storing, and using these insights, as documented in empirical studies highlighting synergies between apps and CRM (Wang et al. 2016c ; Lee 2018a ; Newman et al. 2018 ). Intuitively, the provision of these insights potentially facilitates the realization of digital customer orientation. Nonetheless, as Table 5 shows, the marketing literature is yet to explicitly explore these aspects. Above all, there is room for future research documenting the strategic relevance of consumer insights generated via apps vs. other digital hubs such as web analytics and social media analytics. Moreover, there is scope for evaluating additional implications of information sharing and real-time insights in relation to app personalization . Specifically, apps can enable consumers accessing customized information, strengthening consumer relationships via the provision of superior experiences (Kang and Namkung 2019 ). However, although studies have considered apps’ personalization potential in frameworks aimed at predicting other aspects of the customer journey (see Tan and Chou 2008 ; Wang and Li 2012 ; Watson et al. 2013 ; Li 2018 ), more research is needed to esplicitly evaluate the trade-off between personalization and privacy loss. Furthermore, since market segmentation constitutes a key premise to understand and satisfy consumer needs based on relevant insights (e.g., Cooil, Aksoy and Keiningham 2008 ), there is scope for studying segmentation of apps’ users. In this regard, using cluster analysis, Doub et al. ( 2018 ) and Alavi and Ahuja ( 2016 ) detect distinct segments in relation to the use of certain types of apps (e.g., for food shopping and mobile banking). In contrast, Kim and Lee ( 2018 ) focus on psychographic segmentation of app users, and Kim, Lee and Park ( 2016 ) introduce a user-centric service map and a framework for user-value analysis. Finally, Liu et al. ( 2017 ) and Chen, Zhang and Zhao ( 2017 ) use the Recency, Frequency, Monetary (RFM) approach. Nonetheless, future studies could explore alternative angles such as behavioral segmentation (e.g., delineating between different types of apps’ users based on the usage occasions and frequency of use) and intent-based segmentation (e.g., distinguishing consumers based on stage of customer journey). There is also potential for determining if segments identified in bricks and mortar contexts exhibit different patterns of app usage.
Market intelligence
Thus far, there are only two key studies with a clear focus on market intelligence and competing dynamics. In more detail, using panel data, Jung, Kim and Chan-Olmsted (2014) examine habits and repertoires for different app types by adapting known audience behavior and media concentration benchmarks; and Lee and Raghu ( 2014 ) highlight that app competition is configured as a long-tail market (i.e., many choices and low search costs). Therefore, there are multiple avenues for future research advancements in relation to market intelligence (Shapiro 1988 ) (see Table 5 ). Above all, there is significant scope for more empirical efforts outlining app competition dynamics, ascertaining likely differences for dissimilar app categories (or sub-markets), and introducing metrics and methods to evaluate app return on investment (see also Gill et al. 2017 ). These future research endeavors match industry priorities and concerns; indeed, as Dinsmore et al. ( 2017 ) state: “…more than 60% of app developers are ‘below the app poverty line’, meaning they generate less than $500 a month from their apps […] and a mere 24% of developers are able to directly monetize their products by charging a fee in exchange for download” (p.227).
- Competitive advantage
Lafferty and Hult ( 2001 ) attribute the theoretical foundations of market orientation and thus the attainment of competitive advantage to four factors: customer orientation ; the strategic use of consumer insights and market intelligence ; inter-functional coordination ; and strategic implementation . Having already discussed the first two factors, we now concentrate on the latter two, synthesizing the new marketing knowledge required to clarify how to attain a competitive advantage via apps and for apps (see again Table 5 ).
Inter-functional coordination
An essential premise of market orientation is the effective dissemination of consumer insights and market intelligence across the organizational functions (Lafferty and Hult 2001 ), striving for the coordination needed to deliver superior customer value (Narver and Slater 1990 ). Unfortunately, extant marketing research on apps that relates to this matter is currently missing. Therefore, there is potential for examining apps from the perspective of organizational behaviors (see Cadogan 2012 ), exploring the role of market-orientated behaviors (e.g., product design excellence, see Cyr, Head and Ivanov 2006) in the development, launch and strategic management of apps. For example, future research could evaluate the effects of different managerial approaches, different levels of digital marketing knowledge and the implications of a firm’s overall digital marketing strategy. New studies could also examine the underlying effects of market-level conditions such as market dynamism (i.e., rapid changes in consumer needs and preferences, see again Cadogan 2012 ).
Strategic implementation
A final foundation of market orientation and pre-condition for attaining competitive advantage is the strategic use of the information in decision-making (Lafferty and Hult 2001 ), especially within individual business units (Ruekert 1992 ). It also concerns a significant degree of organizational responsiveness to exogenous factors such as market competition (Kohli and Jaworski 1990 ). Unfortunately, there is a void on these aspects in the marketing literature on apps. Hence, there is scope for new knowledge uncovering different pathways leading to competitive advantage by deploying apps and for the app. Such studies could seek to determine differences across different industries and businesses. There is also scope for studies quantifying the impact for apps on business growth. Finally, the evaluation of synergies with other crucial strategic aspects, especially attribution marketing, marketing analytics and, more broadly, a firm’s digital marketing strategy, represents a fruitful area of future research.
Conclusions, contributions and limitations
We presented an integrative review of existing marketing knowledge on apps spanning two decades of research and hundreds of studies. The synthesis has been mapped against a meta-theoretical focus (see also Becker and Jaakkola 2020 ), which integrates core marketing notions such as the customer journey, digital customer orientation and, importantly, value creation and co-creation. The integration of these aspects modifies and expands Lemon and Verhoef’s ( 2016 ) customer journey, further enhancing the contribution made in reconciling current views and assumptions. Moreover, the meta-theoretical lens used highlighted significant knowledge voids that need to be addressed to move marketing research on apps forward—an outcome that meets the first key research objective of this study. The synthesis also revealed synergies vs. disconnections between industry trends and academic research on the topic of apps, fulfilling the second research objective. The resulting conceptual and practical contributions are as follows.
Summary of theoretical contributions
Apps can enhance consumer perceptions of value from the early stages of the customer journey. In fact, the decision-making process characterizing the pre-adoption and adoption stages hinges on consumer evaluations of perceived benefits that apps can offer, alongside individual characteristics shaping the chains of effects linking attitudes, intentions and behavioral outcomes signaling adoption. Although more research is needed to better understand potential differences in these mechanisms for different types of apps and different consumer segments, the trigger of positive customer experiences and journeys lies in ensuring that the consumer sees value in the app as a channel to access products and services, and as a two-way platform for seamless interactions. Moreover, at the early stages of the customer journey, different marketing strategies play a crucial role; yet little is known in relation to them. On the contrary, a lot is understood in relation to the value of apps post-adoption as the ultimate marketing vehicle, albeit primarily in instances whereby the app is attached to an existing brand. Therefore, new theoretical and empirical evidence is needed to clarify outcomes for standalone apps beyond mobile shopping implications. In fact, considering existing marketing research on “always on” points of interaction, substantial gaps emerge in relation to apps’ marketing mix—an aspect that is vital for the provision of positive customer experiences and rewarding journeys, and for the creation (and co-creation) of value.
Nonetheless, there are clear opportunities for turning customer journeys for apps and via apps into a competitive advantage. These include realizing a digital marketing orientation, leveraging apps’ power to provide hyper-contextualized consumer insights and personalization opportunities, and harvesting the potential of technological advancements (e.g., VR/AR and AI). There are also ample opportunities for gathering strategically relevant market insights beyond the business model imposed by app stores. In this instance, the key to unlock apps’ potential for the attainment of competitive advantage lies in elevating the digital customer orientation to an all-encompassing market orientation, whereby the consumer insights and market intelligence acquired are shared across organizational functions (beyond marketing) and turned into the input of innovative business strategies. As this integrative review reveals, extant knowledge concerning these aspects is missing and needs to be created to move this field of marketing research on apps forward.
Summary of managerial contributions
Marketing practice relating to apps is ever-evolving. However, a great deal of strategies already in use and guidelines for market success often hinge on opinions, learn-by-doing and, we dare to say, blindly following trends and hypes. Scholarly marketing research can play a vital role in remedying this tendency, as long as extant and well-established findings are clearly communicated and readily available to practitioners. In this regard, our integrative review provides highly simplified summaries that can inform businesses on how to plan app launches and successfully integrate apps into business strategies. In particular, the critical synthesis of marketing knowledge presented serves as a nomological map to understand the depth of existing scholarly research on apps yielding managerial relevance. We stress that these findings often match or complement industry assumptions; in other instances, however, discrepancies emerge alongside missing know-how. Hence, a key practical implication of our integrative synthesis lies in providing a roadmap for addressing these inconsistencies, revealing great scope for more synergy between academia and the industry. Ultimately, it is auspicious to see an increase in information and data porosity through the involvement of the industry in future lines of inquiry mapped in this review. Indeed, for the research directions outlined, access to data and the monitoring of market trends are essential. Likewise, harvesting apps’ full economic potential hinges on accessing rigorous scientific findings.
Upon reading this integrative review, we envision managers of businesses deploying apps to support existing brands and managers of businesses whereby the app is the brand to embrace important strategic guidelines that emerged such as: (i) the role apps play in the media ecosystem and/or as a marketing channel, ensuring consumers enjoy seamless value-generating experiences; (ii) the importance of marketing apps via offering clear benefits that match strategic priorities of a business; and (iii) the existence of untapped strategic power for apps for the attainment of competitive advantage, especially upon gathering and using consumer insights and market intelligence above and beyond the marketing function.
Limitations and general future research directions
Our review approach entailed a combination of bibliometric analysis and a more intuitive process whereby research themes were detected and iteratively refined. Although considerable alignment emerged between these two steps, the approach inevitably resulted in some arbitrary choices. For instance, we did not focus on aspects involving the development and supply of apps; similarly, technological aspects of apps’ programing and design were not considered. Therefore, future research could pursue alternative routes such as presenting a meta-analysis of the extant empirical findings. Moreover, the reconciliation of views from academia and industry has been fulfilled by juxtaposing industry trends and assumptions with the summaries of findings extracted from the body of scholarly work reviewed. Future studies could present more explicit analyses of industry views, such as conducting primary research involving managers and app developers. Finally, future development of the outcomes of this integrative review calls for a more detailed evaluation of interdisciplinary links, detecting and exploring in more detail the connections between marketing knowledge and other relevant fields such as information technology, information management and organizational behavior.
In comparison to Lemon and Verhoef’s ( 2016 ) original framework, the use of the word ‘adoption’ is based on the logic that most apps are initially available to consumers at no cost. As such, there is often no ‘purchase’ per se; rather, the focal event that starts the customer journey is the series of customer experiences that lead to adopting the technology. The focus on adoption also combines the strategic firm/brand perspective and the consumer perspective (see Becker and Jaakkola 2020 ).
Aaker, J. L. (1999). The malleable self: The role of self-expression in persuasion. Journal of Marketing Research, 36 (1), 45–57.
Google Scholar
Ahmed, R., Beard, F., & Yoon, D. (2016). Examining and extending advertising's dual mediation hypothesis to a branded mobile phone app. Journal of Interactive Advertising, 16 , 133–144.
Ajzen, I. (1991). The theory of planned behavior. Organizational Behavior and Human Decision Processes, 50 (2), 179–211.
Alalwan, A. A. (2020). Mobile food ordering apps: An empirical study of the factors affecting customer e-satisfaction and continued intention to reuse. International Journal of Information Management, 50 , 28–44.
Alavi, S., & Ahuja, V. (2016). An empirical segmentation of users of mobile banking apps. Journal of Internet Commerce, 15 , 390–407.
Alnawas, I., & Aburub, F. (2016). The effect of benefits generated from interacting with branded mobile apps on consumer satisfaction and purchase intentions. Journal of Retailing and Consumer Services, 31 , 313–322.
Anblicks (2017). 9 Advantages of mobile apps over responsive eCommerce websites . Retrieved March 27, 2021 from https://anblicks-inc.medium.com/9-advantages-of-mobile-apps-over-responsive-ecommerce-websites-6aed1e6db0d.
App Radar (2019). 6 App metrics you absolutely need to measure . Retrieved March 27, 2021 from https://appradar.com/blog/6-app-metrics-you-absolutely-need-to-measure
Appel, G., Libai, B., Muller, E., & Shachar, R. (2020). On the monetization of mobile apps. International Journal of Research in Marketing, 37 , 93–107.
Arora, S., Hofstede, F. T., & Mahajan, V. (2017). The implications of offering free versions for the performance of paid mobile apps. Journal of Marketing, 81 , 62–78.
Arya, V., Sethi, D., & Paul, J. (2019). Does digital footprint act as a digital asset?–enhancing brand experience through remarketing. International Journal of Information Management, 49 , 142–156.
Atkinson, L. (2013). Smart shoppers? Using QR codes and ‘green’ smartphone apps to mobilize sustainable consumption in the retail environment. International Journal of Consumer Studies, 37 , 387–393.
Azjen, I. (1980). Understanding attitudes and predicting social behavior. Englewood Cliffs .
Babich (2017). Mobile app onboarding: The do’s and the don’ts . Retrieved March 27, 2021 from https://www.shopify.com/partners/blog/mobile-app-onboarding .
Baek, T. H., & Yoo, C. Y. (2018). Branded app usability: Conceptualization, measurement, and prediction of consumer loyalty. Journal of Advertising, 47 , 70–82.
Baena, V. (2016). Online and mobile marketing strategies as drivers of brand love in sports teams: Findings from real Madrid. International Journal of Sports Marketing and Sponsorship, 17 , 202–218.
Beard (2020). The challenge facing app developers: Engaging users . Retrieved March 27, 2021 from https://www.psychologytoday.com/us/blog/lab-real-world/202008/the-challenge-facing-app-developers-engaging-users
Becker, L., & Jaakkola, E. (2020). Customer experience: Fundamental premises and implications for research. Journal of the Academy of Marketing Science, 48 , 630–648.
Belk, R. W. (1988). Possessions and the extended self. Journal of Consumer Research, 15 , 139–168.
Bellman, S., Potter, R. F., Treleaven-Hassard, S., Robinson, J. A., & Varan, D. (2011). The effectiveness of branded mobile phone apps. Journal of Interactive Marketing, 25 , 191–200.
Bhave, K., Jain, V., & Roy, S. (2013). Understanding the orientation of gen Y toward mobile applications and in-app advertising in India. International Journal of Mobile Marketing, 8 , 62–71.
Blair, I. (2021). 2021. Mobile app download and usage statistics Retrieved March 30, 2021 from https://buildfire.com/app-statistics/
Boritz, J. E. (2005). IS practitioners' views on core concepts of information integrity. International Journal of Accounting Information Systems, 6 (4), 260–279.
Bowlby, J. (1982). Attachment and loss: Retrospect and prospect. American Journal of Orthopsychiatry, 52 (4), 664.
Brakus, J. J., Schmitt, B. H., & Zarantonello, L. (2009). Brand experience: What is it? How is it measured? Does it affect loyalty? Journal of Marketing, 73 (3), 52–68.
Bruner, G., & Kumar, A. (2005). Explaining consumer acceptance of handheld internet devices. Journal of Business Research, 58 , 553–558.
Buellingen, F., & Woerter, M. (2004). Development perspectives, firm strategies and applications in mobile commerce. Journal of Business Research, 57 , 1402–1408.
Buildfire. (2021). Mobile app download statistics & usage statistics. Retrieved July 26 2021 from https://buildfire.com/app-statistics/ .
Byun, H., Chiu, W., & Bae, J. (2018). Exploring the adoption of sports brand apps: An application of the modified technology acceptance model. International Journal of Asian Business and Information Management, 9 , 52–65.
Cadogan, J. W. (2012). International marketing, strategic orientations and business success: Reflections on the path ahead. International Marketing Review, 29 , 340–348.
Calder, B. J., Malthouse, E. C., & Schaedel, U. (2009). An experimental study of the relationship between online engagement and advertising effectiveness. Journal of Interactive Marketing, 23 , 321–331.
Calder, B.-J., & Malthouse, E.-C. (2008). Media engagement and advertising effectiveness. In B. J. Calder (Ed.), Kellogg on advertising and media (pp. 1–36). Wiley.
Carlson, J. R., & Zmud, R. W. (1999). Channel expansion theory and the experiential nature of media richness perceptions. Academy of Management Journal, 42 (2), 153–170.
Carter, S., & Yeo, A. C. (2016). Mobile apps usage by Malaysian business undergraduates and postgraduates: Implications for consumer behaviour theory and marketing practice. Internet Research, 26 , 733–757.
Chadha, P., Alavi, S., & Ahuja, V. (2017). Mobile shopping apps: Functionalities, consumer adoption, and usage. International Journal of Cyber Behavior, Psychology and Learning, 7 , 40–55.
Chandra, S., Srivastava, S. C., & Theng, Y. (2010). Evaluating the role of trust in consumer adoption on mobile payment systems: An empirical analysis. Communications of the Association for Information Systems, 27 , 561–588.
Chang, C. (2015). Exploring Mobile application customer loyalty: The moderating effect of use contexts. Telecommunications Policy, 39 , 678–690.
Chen, Q., Zhang, M., & Zhao, X. (2017). Analysing customer behaviour in mobile app usage. Industrial Management & Data Systems, 117 , 425–438.
Chen, Y. R. (2017). Perceived values of branded mobile media, consumer engagement, business-consumer relationship quality and purchase intention: A study of WeChat in China. Public Relations Review, 43 , 945–954.
Cheng, H. K., & Tang, Q. C. (2010). Free trial or no free trial: Optimal software product design with network effects. European Journal of Operational Research, 205 (2), 437–447.
Cheng, X., Fang, L., Hong, X., & Yang, L. (2017). Exploiting mobile big data: Sources, features, and applications. IEEE Network, 31 , 72–79.
Chong, A. Y. L., Chan, F. T. S., & Ooi, K. (2012). Predicting consumer decisions to adopt mobile commerce: Cross country empirical examination between China and Malaysia. Decision Support Systems, 53 , 34–43.
Chopdar, P. K., & Sivakumar, V. J. (2018). Understanding psychological contract violation and its consequences on mobile shopping applications use in a developing country context. Journal of Indian Business Research, 10 , 208–231.
Churchill Jr., G. A., & Surprenant, C. (1982). An investigation into the determinants of customer satisfaction. Journal of Marketing Research, 19 (4), 491–504.
Clearbridge Mobile. (2019). A step-by-step guide to marketing your mobile app . Retrieved June 1 st , 2020 from https://clearbridgemobile.com/step-by-step-guide-marketing-mobile-app/ .
Cooil, B., Aksoy, L., & Keiningham, T. L. (2008). Approaches to customer segmentation. Journal of Relationship Marketing, 6 , 9–39.
Cuadrado, F., & Dueñas, J. C. (2012). Mobile application stores: Success factors, existing approaches, and future developments. IEEE Communications Magazine, 50 , 160–167.
Culnan, M. J., O'Reilly III, C. A., & Chatman, J. A. (1990). Intellectual structure of research in organizational behavior, 1972–1984: A cocitation analysis. Journal of the American Society for Information Science, 41 (6), 453–458.
Cyr, D., Head, M., & Ivanov, A. (2006). Design aesthetics leading to m-loyalty in mobile commerce. Information & Management, 43 , 950–963.
Dacko, S. G. (2017). Enabling smart retail settings via mobile augmented reality shopping apps. Technological Forecasting and Social Change, 124 , 243–256.
Datta, H., Foubert, B., & Van Heerde, H. J. (2015). The challenge of retaining customers acquired with free trials. Journal of Marketing Research, 52 (2), 217–234.
Davis, F. D., Bagozzi, R. P., & Warshaw, P. R. (1989). User acceptance of computer technology: A comparison of two theoretical models. Management Science, 35 (8), 982–1003.
Dellaert, B. G. (2019). The consumer production journey: Marketing to consumers as co-producers in the sharing economy. Journal of the Academy of Marketing Science, 47 (2), 238–254.
DeLone, W. H., & McLean, E. R. (1992). Information systems success: The quest for the dependent variable. Information Systems Research, 3 (1), 60–95.
Demir, A., & Aydinli, C. (2016). Exploring the quality dimensions of mobile instant messaging applications and effects of them on customer satisfaction. International Journal of Computer Theory and Applications, 9 , 1–15.
Deshdeep (2021). App or website? 10 reasons why apps are better . Retrieved March 27, 2021 from https://vwo.com/blog/10-reasons-mobile-apps-are-better/ .
Deshpandé, R., Farley, J. U., & Webster Jr., F. E. (1993). Corporate culture, customer orientation, and innovativeness in Japanese firms: A quadrad analysis. Journal of Marketing, 57 , 23–37.
Dinsmore, J. B., Dugan, R. G., & Wright, S. A. (2016). Monetary vs. nonmonetary prices: Differences in product evaluations due to pricing strategies within mobile applications. Journal of Strategic Marketing, 24 (3–4), 227–240.
Dinsmore, J. B., Swani, K., & Dugan, R. G. (2017). To ‘free’ or not to ‘free’: Trait predictors of mobile app purchasing tendencies. Psychology & Marketing, 34 , 227–244.
Doub, A. E., Levin, A., Heath, C. E., & LeVangie, K. (2018). Mobile app-etite: Consumer attitudes towards and use of mobile technology in the context of eating behaviour. Journal of Direct and Digital Marketing Practice, 17 , 114–129.
Dovaliene, A., Masiulyte, A., & Piligrimiene, Z. (2015). The relations between customer engagement, perceived value and satisfaction: The case of mobile applications. Procedia-Social and Behavioral Sciences, 213 , 659–664.
Dovaliene, A., Piligrimiene, Z., & Masiulyte, A. (2016). Factors influencing customer engagement in mobile applications. Engineering Economics, 27 (2), 205–212.
Ehrenhard, M., Wijnhoven, F., van den Broek, T., & Stagno, M. Z. (2017). Unlocking how start-ups create business value with mobile applications: Development of an app-enabled business innovation cycle. Technological Forecasting and Social Change, 115 , 26–36.
Eigenraam, A., Eelen, J., van Lin, A., & Verlegh, P. (2018). A consumer-based taxonomy of digital customer engagement practices. Journal of Interactive Marketing, 44 , 102–121.
Eighmey, J., & McCord, L. (1998). Adding value in the information age: Uses and gratifications of sites on the world wide web. Journal of Business Research, 41 (3), 187–194.
Elliott, R. L. (1997). Existential consumption and irrational desire. European Journal of Marketing, 31 , 285–296.
Elsbach, K. D., & van Knippenberg, D. (2020). Creating high-impact literature reviews: An argument for ‘integrative reviews’. Journal of Management Studies, 57 , 1277–1289.
eMarketer (2019), US digital ad spending on selected channels, 2019-2023, Retrieved March 30, 2021 from https://www.emarketer.com/chart/231153/us-digital-ad-spending-on-select-channels-2019-2023-billions/ .
Facebook (2021). Accelerate your app's growth with these two strategies . Retrieved March 27 , 2021 from https://www.facebook.com/business/help/1571320809813662?id=1858550721111595 .
Fang, J., Zhao, Z., Wen, C., & Wang, R. (2017). Design and performance attributes driving mobile travel application engagement. International Journal of Information Management, 37 , 269–283.
Fang, Y. (2017). Beyond the usefulness of branded applications: Insights from consumer–brand engagement and self-construal perspectives. Psychology & Marketing, 34 , 40–58.
Fang, Y. (2019). An app a day keeps a customer connected: Explicating loyalty to brands and branded applications through the lens of affordance and service-dominant logic. Information & Management, 56 , 377–391.
Fedorychak, V. (2019). 17 efficient ways to promote a mobile app that you need to know . Retrieved June 1 st , 2020 from https://lvivity.com/efficient-ways-to-promote-a-mobile-app .
Figge, S. (2014). Situation-dependent services—A challenge for Mobile network operators. Journal of Business Research, 57 , 1416–1422.
Fournier, S. (1998). Consumers and their brands: Developing relationship theory in consumer research. Journal of Consumer Research, 24 (4), 343–373.
Frey, R. M., Xu, R., & Ilic, A. (2017). Mobile app adoption in different life stages: An empirical analysis. Pervasive and Mobile Computing, 40 , 512–527.
Furner, C. P., Racherla, P., & Babb, J. S. (2014). Mobile app stickiness (MASS) and Mobile interactivity: A conceptual model. The Marketing Review, 14 , 163–188.
Gao, T. T., Rohm, A. J., Sultan, F., & Pagani, M. (2013). Consumers un-tethered: A three-market empirical study of consumers’ mobile marketing acceptance. Journal of Business Research, 66 , 2536–2544.
Genc-Nayebi, N., & Abran, A. (2017). A systematic literature review: Opinion mining studies from mobile Appstore user reviews. Journal of Systems Software, 125 , 207–219.
Gill, M., Sridhar, S., & Grewal, R. (2017). Return on engagement initiatives: A study of a business-to-business mobile app. Journal of Marketing, 81 , 45–66.
Gokgoz, Z. A., Ataman, M. B., & van Bruggen, G. H. (2021). There’s an app for that! Understanding the drivers of mobile application downloads. Journal of Business Research, 123 , 423–437.
Goldsmith, R. E., & Newell, S. J. (1997). Innovativeness and price sensitivity: Managerial, theoretical and methodological issues. Journal of Product & Brand Management, 6 (3), 163–174.
Grant, I., & O’Donohoe, S. (2007). Why toung consumers are not open to mobile marketing communication. International Journal of Advertising, 26 , 223–246.
Green, M. C., & Brock, T. C. (2000). The role of transportation in the persuasiveness of public narratives. Journal of Personality and Social Psychology, 79 (5), 701.
Grewal, D., Hulland, J., Kopalle, P. K., & Karahanna, E. (2020). The future of technology and marketing: A multidisciplinary perspective. Journal of the Academy of Marketing Science, 48 , 1–8.
Groenewald, T. (2004). A phenomenological research design illustrated. International Journal of Qualitative Methods, 3 , 42–55.
Grönroos, C. (2019). Reforming public services: Does service logic have anything to offer? Public Management Review, 21 (5), 775–788.
Ha, Q. A., Chen, J. V., Uy, H. U., & Capistrano, E. P. (2020). Exploring the privacy concerns in using intelligent virtual assistants under perspectives of information sensitivity and anthropomorphism. International journal of human–computer interaction , 1-16.
Harmeling, C. M., Moffett, J. W., Arnold, M. J., & Carlson, B. D. (2017). Toward a theory of customer engagement marketing. Journal of the Academy of Marketing Science, 45 , 312–335.
Herzberg, F., Mausner, B., & Bloch- Snyderman, B. (1959). The motivation to work . Transaction Publishers.
Ho, S. Y. (2012). The effects of location personalization on individuals’ intention to use mobile services. Decision Support Systems, 53 , 802–812.
Hollebeek, L. D., Glynn, M. S., & Brodie, R. J. (2014). Consumer brand engagement in social media: Conceptualization, scale development and validation. Journal of Interactive Marketing, 28 , 149–165.
Hong, S., & Tam, K. Y. (2006). Understanding the adoption of multipurpose information appliances: The case of mobile data services. Information Systems Research, 17 , 162–179.
Hornbæk, K. (2006). Current practice in measuring usability: Challenges to usability studies and research. International Journal of Human-Computer Studies, 64 (2), 79–102.
Howard, J. A., & Sheth, J. N. (1969). The theory of buyer behavior (no. 658.834 H6).
Hsiao, K., & Chen, C. (2016). What drives in-app purchase intention for mobile games? An examination of perceived values and loyalty. Electronic Commerce Research and Applications, 16 , 18–29.
Hsu, C., & Lin, J. C. (2015). What drives purchase intention for paid mobile apps?–an expectation confirmation model with perceived value. Electronic Commerce Research and Applications, 14 , 45–57.
Hu, H., Zhang, D., & Wang, C. (2019). Impact of social media influencers' endorsement on application adoption: A trust transfer perspective. Social Behavior and Personality: An International Journal, 47 , 1–12.
Huang, G., & Korfiatis, N. (2015). Trying before buying: The moderating role of online reviews in trial attitude formation toward mobile applications. International Journal of Electronic Commerce, 19 , 77–111.
Huang, J., Lin, Y., & Chuang, S. (2007). Elucidating user behavior of mobile learning: A perspective of the extended technology acceptance model. The Electronic Library, 25 , 585–598.
Huang, M. H., & Rust, R. T. (2017). Technology-driven service strategy. Journal of the Academy of Marketing Science, 45 (6), 906–924.
Hughes, A. M. (1996). The complete database marketer: Second-generation strategies and techniques for tapping the power of your customer database . McGraw-Hill.
Hur, H. J., Lee, H. K., & Choo, H. J. (2017). Understanding usage intention in innovative mobile app service: Comparison between millennial and mature consumers. Computers in Human Behavior, 73 , 353–361.
Hulland, J., & Houston, M. B. (2020). Why systematic review papers and meta-analyses matter: An introduction to the special issue on generalizations in marketing. Journal of Academy of Market Science, 48 , 351–359. https://doi.org/10.1007/s11747-020-00721-7
Article Google Scholar
Hyrynsalmi, S., Seppänen, M., Aarikka-Stenroos, L., Suominen, A., Järveläinen, J., & Harkke, V. (2015). Busting myths of electronic word of mouth: The relationship between customer ratings and the sales of mobile applications. Journal of Theoretical and Applied Electronic Commerce Research, 10 , 1–18.
IBM Cloud Education (2020). Mobile Application Development . Retrieved March 27, 2021 from https://www.ibm.com/cloud/learn/mobile-application-development-explained .
Ickin, S., Petersen, K., & Gonzalez-Huerta, J. (2017). Why do users install and delete apps? A survey study. In A. Ojala, H. Olsson, & K. Werder (Eds.), Lecture notes in business information processing (pp. 186–191). Springer.
Influencer Marketing Hub (2018). 50 TikTok stats that will blow your mind . Retrieved June 1 st , 2020 from https://influencermarketinghub.com/tiktok-stats/ .
Intersoft Consulting (2021). Key issues, Retrieved March 27, 2021 from https://gdpr-info.eu/issues/ .
Iqbal, M. (2019). TikTok revenue and usage statistics—Business of apps . Retrieved June 1 st , 2020 from https://www.businessofapps.com/data/tik-tok-statistics/ .
Ismagilova, E., Slade, E. L., Rana, N., & Dwivedi, Y. K. (2019). The effect of electronic word of mouth communications on intention to buy: A meta-analysis. Information Systems Frontiers , 1–24.
Iyengar, R., Van den Bulte, C., & Valente, T. W. (2011). Opinion leadership and social contagion in new product diffusion. Marketing Science, 30 , 195–212.
Iyer, P., Davari, A., & Mukherjee, A. (2018). Investigating the effectiveness of retailers’ mobile applications in determining customer satisfaction and repatronage intentions? A congruency perspective. Journal of Retailing and Consumer Services, 22 , 235–243.
Jain, V., & Viswanathan, V. (2015). Choosing and using mobile apps: A conceptual framework for generation Y. Journal of Customer Behaviour, 14 (4), 295–309.
Jin, C., & Villegas, J. (2008). Mobile phone users’ behaviors: The motivation factors of the mobile phone user. International Journal of Mobile Marketing, 3 , 4–11.
John, O. P., & Srivastava, S. (1999). The big-five trait taxonomy: History, measurement, and theoretical perspectives, 2, 102–138 . University of California.
Johnson, T., Kulesa, P., Cho, Y., & Shavitt, S. (2005). The relation between culture and response styles: Evidence from 19 countries. Journal of Cross-Cultural Psychology, 36 , 264–277.
Joo, J., & Sang, Y. (2013). Exploring Koreans’ smartphone usage: An integrated model of the technology acceptance model and uses and gratifications theory. Computers in Human Behavior, 29 (6), 2512–2518.
Jung, E., Baek, C., & Lee, J. D. (2012). Product survival analysis for the app store. Marketing Letters, 23 , 929–941.
Jung, J., Kim, Y., & Chan-Olmsted, S. (2014). Measuring usage concentration of smartphone applications: Selective repertoire in a marketplace of choices. Mobile Media & Communication, 2 , 352–368.
Kang, J. M., Mun, J. M., & Johnson, K. K. P. (2015). In-store mobile usage: Downloading and usage intention toward mobile location-based retail apps. Computers in Human Behavior, 46 , 210–217.
Kang, J., & Namkung, Y. (2019). The role of personalization on continuance intention in food service mobile apps. International Journal of Contemporary Hospitality Management, 31 , 734–752.
Karagkiozidou, M., Ziakis, C., Vlachopoulou, M., & Kyrkoudis, T. (2019). App store optimization factors for effective mobile app ranking. Strategic Innovative Marketing and Tourism , 479–486.
Karaiskos, D. C., Drossos, D. A., Tsiaousis, A. S., Giaglis, G. M., & Fouskas, K. G. (2012). Affective and social determinants of mobile data services adoption. Behaviour & Information Technology, 31 , 209–219.
Karjaluoto, H, Shaikh, A. A., Saarijärvi, H., & Saraniemi, S. (2019). How perceived value drives the use of mobile financial services apps. International Journal of Information Management, 47, 252–261.
Katona, Z., Zubcsek, P. P., & Sarvary, M. (2011). Network effects and personal influences: The diffusion of an online social network. Journal of Marketing Research, 48 , 425–443.
Keller, K. L. (1993). Conceptualizing, measuring, and managing customer-based brand equity. Journal of Marketing, 57 , 1–22.
Khalifa, M., Cheng, S. K. N., & Shen, K. N. (2012). Adoption of Mobile commerce: A confidence model. Journal of Computer Information Systems, 53 , 14–22.
Kilger, M., & Romer, E. (2007). Do measures of media engagement correlate with product purchase likelihood? Journal of Advertising Research, 47 , 213–214.
Kim (2019). Why do bad user retention rates happen to good mobile apps? Retrieved March 27, 2021 from https://www.appcues.com/blog/mobile-app-user-retention .
Kim, E., Lin, J., & Sung, Y. (2013). To app or not to app: Engaging consumers via branded mobile apps. Journal of Interactive Advertising, 13 , 53–65.
Kim, G. S., Park, S., & Oh, J. (2008). An examination of factors influencing consumer adoption of short message service (SMS). Psychology & Marketing, 25 , 769–786.
Kim, J., & Lee, K. H. (2018). Influences of motivations and lifestyles on intentions to use smartphone applications. International Journal of Advertising, 37 , 385–401.
Kim, J., & Yu, E. A. (2016). The holistic brand experience of branded mobile applications affects brand loyalty. Social Behavior and Personality, 44 , 77–88.
Kim, J., Lee, S., & Park, Y. (2016a). A visual context-based market analysis of mobile application services. Management Decision, 54 , 2106–2132.
Kim, M., Kim, J., Choi, J., & Trivedi, M. (2017). Mobile shopping through applications: Understanding application possession and mobile purchase. Journal of Interactive Marketing, 39 , 55–68.
Kim, S. C., Yoon, D., & Han, E. K. (2016b). Antecedents of mobile app usage among smartphone users. Journal of Marketing Communications, 22 , 653–670.
Kim, S. J., Wang, R. J. H, & Malthouse, E. C. (2015). The effects of adopting and using a brand’s mobile application on customers’ subsequent purchase behavior. Journal of Interactive Marketing , 31, 28–41.
Kim, S., & Baek, T. H. (2018). Examining the antecedents and consequences of mobile app engagement. Telematics and Informatics, 35 , 148–158.
Kim, S., Baek, T. H., Kim, Y., & Yoo, K. (2016c). Factors affecting stickiness and word of mouth in mobile applications. Journal of Research in Interactive Marketing, 10 , 177–192.
Kleijnen, M., Ruyter, K. D., & Wetzels, M. (2007). An assessment of value creation in mobile service delivery and the moderating role of time consciousness. Journal of Retailing, 83 , 33–46.
Ko, E., Kim, E. Y., & Lee, E. K. (2009). Modelling consumer adoption of mobile shopping for fashion products in Korea. Psychology & Marketing, 26 , 669–687.
Koenig-Lewis, N., Marquet, M., Palmer, A., & Zhao, A. L. (2015). Enjoyment and social influence: Predicting mobile payment adoption. The Service Industries Journal, 35 , 537–554.
Kohli, A. K., & Jaworski, B. J. (1990). Market orientation: The construct, research propositions, and managerial implications. Journal of Marketing, 54 , 1–18.
Kolb, D. (1984). Experiential learning: Experience as the source of learning and development . Prentice Hall.
Kolb, D. A., Boyatzis, R. E., & Mainemelis, C. (2001). Experiential learning theory: Previous research and new directions. Perspectives on Thinking, Learning, and Cognitive Styles, 1 (8), 227–247.
Kopalle, P. K., Kumar, V., & Subramaniam, M. (2020). How legacy firms can embrace the digital ecosystem via digital customer orientation. Journal of the Academy of Marketing Science, 48 , 114–131.
Kristensson, P. (2019). Future service technologies and value creation. Journal of Services Marketing, 33 (4), 502–506.
Kübler, R., Pauwels, K., Yildirim, G., & Fandrich, T. (2018). App popularity: Where in the world are consumers most sensitive to price and user ratings? Journal of Marketing, 82 , 20–44.
Kumar, A., & Mukherjee, A. (2013). Shop while you talk: Determinants of purchase intentions through a mobile device. International Journal of Mobile Marketing, 8 , 23–37.
Kumar, D. S., Purani, K., & Viswanathan, S. A. (2018). Influences of ‘appscape’ on mobile app adoption and m-loyalty. Journal of Retailing and Consumer Services, 45 , 132–141.
Lacewing, M. (2010). Personal identity: Physical and psychological continuity theories.
Lafferty, B. A., & Hult, G. T. M. (2001). A synthesis of contemporary market orientation perspectives. European Journal of Marketing, 35 , 92–109.
Lam, S. Y., Shankar, V., Erramilli, M. K., & Murthy, B. (2004). Customer value, satisfaction, loyalty, and switching costs: An illustration from a business-to-business service context. Journal of the Academy of Marketing Science, 32 (3), 293–311.
Lamberton, C., & Stephen, A. T. (2016). A thematic exploration of digital, social media, and mobile marketing research's evolution from 2000 to 2015 and an agenda for future research. Journal of Marketing, 80 , 146–172.
Langford, S., & Ramachandran, M. (2013). The products of fission, fusion, and teletransportation: An occasional identity theorist's perspective. Australasian Journal of Philosophy, 91 (1), 105–117.
Larivière, B., Joosten, H., Malthouse, E. C., Birgelen, M. C., Aksoy, P., Kunz, W. H., & Huang, M. (2013). Value fusion: The blending of consumer and firm value in the distinctive context of mobile technologies and social media. Journal of Service Management, 24 , 268–293.
Laurent, G., & Kapferer, J. N. (1985). Measuring consumer involvement profiles. Journal of Marketing Research, 22 (1), 41–53.
Lavidge, R. J., & Steiner, G. A. (1961). A model for predictive measurements of advertising effectiveness. Journal of Marketing, 25 (6), 59–62.
Lee, C., Tsao, C., & Chang, W. C. (2015). The relationship between attitude toward using and customer satisfaction with mobile application services. Journal of Enterprise Information Management, 28 , 680–697.
Lee, G., & Raghu, T. S. (2014). Determinants of mobile apps' success: Evidence from the app store market. Journal of Management Information Systems, 31 , 133–170.
Lee, M. K. O., Cheung, C. M. K., & Chen, Z. (2007). Understanding user acceptance of multimedia messaging services: An empirical study. Journal of American Society of Information Science & Technology, 58 , 2066–2077.
Lee, S. A. (2018a). Enhancing customers’ continued mobile app use in the service industry. Journal of Services Marketing, 32 (6), 680–691.
Lee, S. A. (2018b). M-servicescape: Effects of the hotel mobile app servicescape preferences on customer response. Journal of Hospitality and Tourism Technology, 9 , 172–187.
Lee, Y. H., Hsieh, Y. C., & Hsu, C. N. (2011). Adding innovation diffusion theory to the technology acceptance model: Supporting employees' intentions to use e-learning systems. Journal of Educational Technology & Society, 14 (4), 124–137.
Lee, Y., & Kim, H. (2019). Consumer need for mobile app atmospherics and its relationships to shopper responses. Journal of Retailing and Consumer Services, 51 , 437–442.
Lei, S. I., Ye, S., Wang, D., & Law, R. (2020). Engaging customers in value co-creation through mobile instant messaging in the tourism and hospitality industry. Journal of Hospitality & Tourism Research, 44 (2), 229–251.
Lemon, K. N., & Verhoef, P. C. (2016). Understanding customer experience throughout the customer journey. Journal of Marketing, 80 , 69–96.
Li, C. (2018). Consumer behavior in switching between membership cards and mobile applications: The case of Starbucks. Computers in Human Behavior, 84 , 171–184.
Liang, T., Li, X., Yang, C., & Wang, M. (2015). What in consumer reviews affects the sales of mobile apps: A multi-facet sentiment analysis approach. International Journal of Electronic Commerce, 20 , 236–260.
Liao, C., Palvia, P., & Chen, J. L. (2009). Information technology adoption behavior life cycle: Toward a technology continuance theory (TCT). International Journal of Information Management, 29 (4), 309–320.
Lin, H. H., & Wang, Y. S. (2006). An examination of the determinants of customer loyalty in mobile commerce contexts. Information & Management, 43 (3), 271–282.
Liu, C. Z., Au, Y. A, & Choi, H. S. (2014). Effects of freemium strategy in the mobile app market: An empirical study of Google play. Journal of Management Information Systems, 31, 326–354.
Liu, F., Zhao, S., & Li, Y. (2017). How many, how often, and how new? A mulitvariate profiling of mobile app users. Journal of Retailing and Consumer Services, 38 , 71–80.
Liu, T., Mathrani, A., & Mbachu, J. (2019). Benefits and barriers in uptake of mobile apps in New Zealand construction industry: What top and middle management perceive. Facilities, 37 , 254–265.
Lu, H., & Hsiao, K. (2010). The influence of extro/introversion on the intention to pay for social networking sites. Information and Management, 47 , 150–157.
Lu, J., Liu, C., Yu, C., & Wang, K. (2008). Determinants of accepting wireless mobile data services in China. Information & Management, 45 , 52–64.
Lu, J., Mao, Z., Wang, M., & Hu, L. (2015). Goodbye maps, hello apps? Exploring the influential determinants of travel app adoption. Current Issues in Tourism, 18 , 1059–1079.
Lu, J., Wang, L., & Yu, C. (2007). Is TAM for wireless mobile data services applicable in China? A survey report from Zhejiang, China. International Journal of Mobile Communications, 5 , 11–31.
Lu, Y., Yang, S., Chau, P. Y. K., & Cao, Y. (2011). Dynamics between the trust transfer process and intention to use mobile payment services: A cross-environment perspective. Information & Management, 48 , 393–403.
MacInnis, D. J. (2011). Framework for conceptual contributions in marketing. Journal of Marketing, 75 , 136–154.
Magrath, V., & McCormick, H. (2013). Marketing design elements of mobile fashion retail apps. Journal of Fashion Marketing and Management: An International Journal, 17 , 115–134.
Mahatanankoon, P. H., Wen, J., & Lim, B. (2005). Consumer-based m-commerce: Exploring consumer perception of mobile applications. Computer Standards & Interfaces, 27 , 347–357.
Maity, M. (2010). Critical factors of consumer decision-making on m-commerce: A qualitative study in the United States. International Journal of Mobile Marketing, 5 , 87–101.
Mäki, M., & Kokko, T. (2017). The use of mobile applications in shopping: A focus on customer experience. International Journal of E-Services and Mobile Applications, 9 , 59–74.
Malhotra, A., & Malhotra, C. K. (2009). A relevancy-based services view for driving adoption of wireless web services in the U.S. Communications of the ACM, 52 , 130–134.
Mallat, N., Rossi, M., Tuunainen, V. K., & Öörni, A. (2009). The impact of use context on mobile services acceptance: The case of mobile ticketing. Information & Management, 46 , 190–195.
Marchick (2014). Engagement and Revenue Up with Mobile Apps. Retrieved March 27, 2021 from https://medium.com/@the_manifest/14-key-metrics-you-need-to-track-for-your-mobile-app-75e48714039f .
Martin, W., Sarro, F., Jia, Y., Zhang, Y., & Harman, M. (2017). A survey of app store analysis for software engineering. IEEE Transactions on Software Engineering, 43 , 817–847.
McCrae, R. R., & Costa, P. T. (1987). Validation of the five-factor model of personality across instruments and observers. Journal of Personality and Social Psychology, 52 (1), 81.
Mcguire, W. J. (1974). Psychological motives and communication gratification. In J. G. Blumler & E. Katz (Eds.), The uses of mass communications . Sage Publications.
McLean, G., Al-Nabhani, K., & Wilson, A. (2018). Developing a mobile applications customer experience model (MACE)-implications for retailers. Journal of Business Research, 85 , 325–336.
McRae, E., Carrabis, J., Carrabis, S., & Hamel, S. (2013). Case study: Emotional response on mobile platforms - want to be loved? Go mobile! International Journal of Mobile Marketing, 8 , 55–66.
McCrae, R. R. (2002). NEO-PI-R data from 36 cultures: Further intercultural comparisons. In R. R. McCrae, R. R. & J. Allik (Eds.), The five-factor model of personality across cultures (pp. 105–126). : Kluwer Academic/Plenum.
Miluzzo, E., Lane, N.D., Lu, H., & Campbell, A. T. (2010). Research in the app store era: Experiences from the CenceMe app deployment on the iPhone. UbiComp proceedings , Copenhagen, 4 .
Mittal, B. (1989). Measuring purchase-decision involvement. Psychology & Marketing, 6 (2), 147–162.
Miyazaki, A. D., & Fernandez, A. (2000). Internet privacy and security: An examination of online retailer disclosures. Journal of Public Policy & Marketing, 19 (1), 54–61.
Mkono, M. (2013). Hot and cool authentication: A Netnographic illustration. Annals of Tourism Research, 41 , 215–218.
Mondal, J., & Chakrabarti, S. (2019). Emerging phenomena of the branded app: A systematic literature review, strategies, and future research directions. Journal of Interactive Advertising, 19 , 148–167.
Morosan, C., & DeFranco, A. (2015). Disclosing personal information via hotel apps: A privacy calculus perspective. International Journal of Hospitality Management, 47 , 120–130.
Morosan, C., & DeFranco, A. (2016). Modeling guests’ intentions to use mobile apps in hotels: The roles of personalization, privacy, and involvement. International Journal of Contemporary Hospitality Management, 28 , 1968–1991.
Mort, G. M. S., & Drennan, J. (2007). Mobile communications: A study of factors influencing consumer use of m-services. Journal of Advertising Research, 47 , 302–312.
Narver, J. C., & Slater, S. F. (1990). The effect of a market orientation on business profitability. Journal of Marketing, 54 , 20–35.
Nass, C., & Moon, Y. (2000). Machines and mindlessness: Social responses to computers. Journal of Social Issues, 56 (1), 81–103.
Natarajan, T., Balasubramanian, S. A., & Kasilingam, D. L. (2017). Understanding the intention to use mobile shopping applications and its influence on price sensitivity. Journal of Retailing and Consumer Services, 37 , 8–22.
Newman, C. L., Wachter, K., & White, A. (2018). Bricks or clicks? Understanding consumer usage of retail mobile apps. Journal of Services Marketing, 32 , 211–222.
Nikhashemi, S. R., Knight, H. H., Nusair, K., & Liat, C. B. (2021). Augmented reality in smart retailing: A (n)(a) symmetric approach to continuous intention to use retail brands’ mobile AR apps. Journal of Retailing and Consumer Services, 60 , 102464.
Nisbett, R. E., Peng, K., Choi, I., & Norenzayan, A. (2001). Culture and systems of thought: Holistic versus analytic cognition. Psychological Review, 108 , 291–310.
Noh, M. J., & Lee, K. T. (2016). An analysis of the relationship between quality and user acceptance in smartphone apps. Information Systems and e-Bus Management, 14 , 273–291.
Numminen, E., & Sällberg, H. (2017). The impact of online ratings on downloads of free Mobile apps. 11 th European conference on information systems management ECISM , Genoa , 225–232.
Nysveen, H., Pedersen, P.E. and Skard, S.E. (2015), A review of mobile services research: Research gaps and suggestions for future research on mobile apps , working paper 01/15, NHH, Brage.
Oh, Y. K., & Min, J. (2015). The mediating role of popularity rank on the relationship between advertising and in-app purchase sales in mobile application market. Journal of Applied Business Research, 31 , 1311–1322.
Olson, J., & A Mourey, J. (2019). Greater expectations: Anthropomorphic products must be warm and competent... or else. ACR North American Advances .
Oyserman, D., Coon, H. M., & Kemmelmeier, M. (2002). Rethinking individualism and collectivism: Evaluation of theoretical assumptions and meta-analysis. Psychological Bulletin, 128 , 3–72.
Padilla-Piernas, J. M., Parra-Meroño, M. C., & Beltrán-Bueno, M. A. (2019). The importance of app store optimization (ASO) for hospitality applications. Digital and Social Marketing , 151–161.
Pantano, E., & Pizzi, G. (2020). Forecasting artificial intelligence on online customer assistance: Evidence from chatbot patents analysis. Journal of Retailing and Consumer Services, 55 , 102096.
Payne (2021). How to Launch a Mobile App in 2021 [Complete Guide] . Retrieved March 27, 2021 from https://blog.hubspot.com/blog/tabid/6307/bid/31277/a-marketer-s-complete-guide-to-launching-mobile-apps.aspx .
Pechenkina, E. (2017). Developing a typology of mobile apps in higher education: A national case-study. Australasian Journal of Educational Technology, 33 , 134–146.
Peng, K., Chen, Y., & Wen, K. (2014). Brand relationship, consumption values and branded app adoption. Industrial Management & Data Systems, 114 , 1131–1143.
Pentina, I., Zhang, L., Bata, H., & Chen, Y. (2016). Exploring privacy paradox in information sensitive mobile apps adoption: A cross-cultural comparison. Computers in Human Behavior, 65 , 409–419.
Peppard, J., & Rylander, A. (2006). From value chain to value network: Insights for mobile operators. European Management Journal, 24 (2–3), 128–141.
Petty, R. E., & Cacioppo, J. T. (1986). The elaboration likelihood model of persuasion. In Communication and persuasion (pp. 1–24). Springer.
Piccoli, G., Brohman, M. K., Watson, R. T., & Parasuraman, A. (2009). Process completeness: Strategies for aligning service systems with customers’ service needs. Business Horizons, 52 , 367–376.
Picoto, W. N., Duarte, R., & Pinto, I. (2019). Uncovering top-ranking factors for mobile apps through a multimethod approach. Journal of Business Research, 101 , 668–674.
Post, C., Sarala, R., Gattrell, C., & Prescott, J. E. (2020). Advancing theory with review articles. Journal of Management Studies, 57 , 351–376.
Racherla, P, Furner, C., & Babb, J. (2012). Conceptualizing the implications of mobile app usage and stickiness: A research agenda. Available at SSRN 2187056.
Radler, V. M. (2018). 20 years of brand personality: A bibliometric review and research agenda. Journal of Brand Management, 25 , 370–383.
Rauschnabel, P. A., Felix, R., & Hinsch, C. (2019). Augmented reality marketing: How mobile AR-apps can improve brands through inspiration. Journal of Retailing and Consumer Services, 49 , 43–53.
Razek, A. R. A., van Husen, C., Pallot, M., & Richir, S. (2018, April). A comparative study on conventional versus immersive service prototyping (VR, AR, MR). In proceedings of the virtual reality international conference-Laval virtual, 1-10).
Rezaei, S., & Valaei, N. (2017). Crafting experiential value via smartphone Apps Channel. Marketing, Intelligence & Planning, 35 , 688–702.
Richins, M. L. (1994). Valuing things: The public and private meanings of possessions. Journal of Consumer Research, 21 , 504–521.
Richins, M. L., & Bloch, P. H. (1986). After the new wears off: The temporal context of product involvement. Journal of Consumer Research, 13 (2), 280–285.
Robinson, S. L. (1996). Trust and breach of the psychological contract. Administrative Science Quarterly , 574–599.
Rogers, E. M. (1995). Diffusion of innovations (4th ed.). Free Press.
Rogers, E. M. (2010). Diffusion of innovations . Simon and Schuster.
Roggeveen, A. L., Grewal, D., & Schweiger, E. B. (2020). The DAST framework for retail atmospherics: The impact of in-and out-of-store retail journey touchpoints on the customer experience. Journal of Retailing, 96 (1), 128–137.
Rohm, A. J., Gao, T., Sultan, F., & Pagani, M. (2012). Brand in the hand: A cross-market investigation of consumer acceptance of mobile marketing. Business Horizons, 55 , 485–493.
Roma, P., & Ragaglia, D. (2016). Revenue models, in-app purchase, and the app performance: Evidence from apple’s app store and google play. Electronic Commerce Research and Applications, 17 , 173–190.
Romaniuk, J., & Sharp, B. (2016). How brands grow part 2 . Oxford University Press.
Rook, D. W., & Fisher, R. J. (1995). Normative influences on impulsive buying behavior. Journal of Consumer Research, 22 (3), 305–313.
Rosario, A. B., de Valck, K., & Sotgiue, F. (2020). Conceptualizing the electronic word-of-mouth process: What we know and need to know about e-WOM creation, exposure, and evaluation. Journal of the Academy of Marketing Science, 48 , 422–448.
Ruekert, R. W. (1992). Developing a market orientation: An organizational strategy perspective. International Journal of Research in Marketing, 9 , 225–245.
Rust, R. T., & Huang, M. H. (2014). The service revolution and the transformation of marketing science. Marketing Science, 33 (2), 206–221.
Samiee, S., & Chabowski, B. R. (2012). Knowledge structure in international marketing: A multi-method bibliometric analysis. Journal of the Academy of Marketing Science, 40 (2), 364–386.
Sanchez, J., Carmen, A., & Haenlein, M. (2020). Competitive spill over elasticities of electronic word of mouth: An application to the soft drink industry. Journal of the Academy of Marketing Science, 48 , 270–287.
Sarkar, A., Sarkar, J. G., Sreejesh, S., & Anusree, M. R. (2018). A qualitative investigation of e-tail brand affect. Marketing Intelligence & Planning, 36 , 365–380.
Saxena, P. (2020). Effective mobile app promotion strategies that start-ups must consider . Retrieved June 1 st , 2020 from https://appinventiv.com/blog/mobile-app-promotion-guide .
Scholz, J., & Duffy, K. (2018). We are at home: How augmented reality reshapes mobile marketing and consumer-brand relationships. Journal of Retailing and Consumer Services, 44 , 11–23.
Seitz, V. A., & Aldebasi, N. M. (2016). The effectiveness of branded mobile apps on user’s brand attitudes and purchase intentions. Review of Economic and Business Studies, 9 , 141–154.
Sensortower (2020). Top grossing apps | US | top app store ratings for iOS . Retrieved June 1 st , 2020 from https://sensortower.com/ios/rankings/top/iphone/us/all-categories?date=2020-06-02/ .
Shankar, V., Kleijnen, M., Ramanathan, S., Rizley, R., Holland, S., & Morrissey, S. (2016). Mobile shopper marketing: Key issues, current insights, and future research avenues. Journal of Interactive Marketing, 34 , 37–48.
Shapiro, B. P. (1988). What the hell is market oriented? Harvard Business Review, 66 , 119–125.
Sharp, B. (2010). How brands grow . Oxford University Press.
Sharp, B., Wright, M., & Goodhardt, G. (2002). Purchase loyalty is polarised into either repertoire or subscription patterns. Australasian Marketing Journal, 10 , 7–20.
Sirgy, M. J. (1982). Self-concept in consumer behavior: A critical review. Journal of Consumer Research, 9 (3), 287–300.
Sirgy, M. J., Lee, D. J., Johar, J. Y., & Tidwell, J. (2008). Effect of self-congruity with sponsorship on brand loyalty. Journal of Business Research, 61 (10), 1091–1097.
Smutkupt, P., Krairit, D., & Esichaikul, V. (2010). Mobile marketing: Implications for marketing strategies. International Journal of Mobile Marketing, 5 (2).
Snyder, H., Witell, L., Gustafsson, A., Fombelle, P., & Kristensson, P. (2016). Identifying categories of service innovations: A review and synthesis of the literature. Journal of Business Research, 69 , 2401–2408.
Sripalawat, J., Thongmak, M., & Ngramyarn, A. (2011). M-banking in metropolitan Bangkok and a comparison with other countries. Journal of Computer Information Systems, 51 , 67–76.
Srivastava, V. (2017). 71 ways to promote your mobile app for free . Retrieved June 1 st , 2020 from https://www.appypie.com/how-to-promote-your-mobile-app/ .
Statista. (2020). Number of apps available in leading app stores as of 1st quarter 2020. Retrieved June 2 nd , 2020 from https://www.statista.com/statistics/276623/number-of-apps-available-in-leading-app-stores/ .
Steuer, J. (1992). Defining virtual reality: Dimensions determining telepresence. Journal of Communication, 42 (4), 73–93.
Stocchi, L., Guerini, C., & Michaelidou, N. (2017). When are apps worth paying for? How marketers can analyze the market performance of mobile apps. Journal of Advertising Research, 57 , 260–271.
Stocchi, L., Ludwichowska, G., Fuller, R., & Gregoric, A. (2020a). Customer-based brand equity for branded apps: A simple research framework. Journal of marketing communications , 1-30.
Stocchi, L., Michaelidou, N., & Micevski, M. (2019). Drivers and outcomes of branded mobile app usage intention. Journal of Product & Brand Management, 28 , 28–49.
Stocchi, L., Michaelidou, N., Pourazad, N., & Micevski, M. (2018). The rules of engagement: How to motivate consumers to engage with branded mobile apps. Journal of Marketing Management, 341 , 1196–1226.
Stocchi, L., Pourazad, N., & Michaelidou, N. (2020b). Identification of two decision-making paths underpinning the continued use of branded apps. Psychology & Marketing , 1–16.
Ström, R., Vendel, M., & Bredican, J. (2014). Mobile marketing: A literature review on its value for consumers and retailers. Journal of Retailing and Consumer Services, 21 , 1001–1012.
Sultan, F., & Rohm, A. J. (2005). The coming era of 'brand in the hand' marketing. MIT Sloan Management Review, 47 , 83–90.
Sultan, F., Rohm, A. J., & Gao, T. T. (2009). Factors influencing consumer acceptance of mobile marketing: A two-country study of youth markets. Journal of Interactive Marketing, 23 , 308–320.
Sussman, S. W., & Siegal, W. S. (2003). Informational influence in organizations: An integrated approach to knowledge adoption. Information Systems Research, 14 (1), 47–65.
Svendsen, G. B., Johnsen, J. A. K., Almås-Sørensen, L., & Vittersø, J. (2013). Personality and technology acceptance: The influence of personality factors on the core constructs of the technology acceptance model. Behaviour & Information Technology, 32 (4), 323–334.
Taivalsaari, A., & Mikkonen, T. (2015). From apps to liquid multi-device software. Procedia Computer Science, 56 , 34–40.
Tan, F. B., & Chou, J. P. C. (2008). The relationship between mobile service quality, perceived technology compatibility, and users’ perceived playfulness in the context of mobile information and entertainment services. International Journal of Human–Computer Interaction, 24 , 649–671.
Tan, X., Qin, L., Kim, Y., & Hsu, J. (2012). Impact of privacy concern in social networking web sites. Internet Research, 22 , 211–233.
Tao, D., Shao, F., Wang, H., Yan, M., & Qu, X. (2020). Integrating usability and social cognitive theories with the technology acceptance model to understand young users’ acceptance of a health information portal. Health Informatics Journal, 26 (2), 1347–1362.
Tariq (2020). 4 steps for increasing your app's user engagement and retention . Retrieved March 27, 2021 from https://www.entrepreneur.com/article/353470 .
Tarute, A., Nikou, S., & Gatautis, R. (2017a). Mobile application driven consumer engagement. Telematics and Informatics, 34 (4), 145–156.
Tarute, A., Shahrokh, N., & Gatautis, R. (2017b). Mobile application driven consumer engagement. Telematics and Informatics, 34 , 145–156.
Tax, S. S., McCutcheon, D., & Wilkinson, I. F. (2013). The service delivery network (SDN): A customer-centric perspective of the customer journey. Journal of Service Research, 16 , 454–470.
Taylor, D. G., & Levin, M. (2014). Predicting mobile app usage for purchasing and information-sharing. International Journal of Retail & Distribution Management, 42 , 759–774.
Taylor, D. G., Voelker, T. A., & Pentina, I. (2011). Mobile application adoption by young adults: A social network perspective. International Journal of Mobile Marketing, 6 , 60–70.
The Manifest (2018). 14 key metrics you need to track for your mobile app . Retrieved March 27, 2021 from https://medium.com/@the_manifest/14-key-metrics-you-need-to-track-for-your-mobile-app-75e48714039f .
Think Mobile (2021). What are the popular types and categories of apps . Retrieved July 3 rd , 2021 from https://thinkmobiles.com/blog/popular-types-of-apps/ .
Thomson, M., MacInnis, D. J., & Park, C. W. (2005). The ties that bind: Measuring the strength of consumers’ emotional attachments to brands. Journal of Consumer Psychology, 15 (1), 77–91.
Toivonen, M., & Tuominen, T. (2009). Emergence of innovations in services. Service Industries Journal, 29 , 887–902.
Tojib, D., & Tsarenko, Y. (2012). Post-adoption modeling of advanced mobile service use. Journal of Business Research, 65 , 922–928.
Tong, S., Luo, X., & Xu, B. (2020). Personalized mobile marketing strategies. Journal of the Academy of Marketing Science, 48 , 64–78.
Tran, T. P., Mai, E. S., & Taylor, E. C. (2021). Enhancing brand equity of branded mobile apps via motivations: A service-dominant logic perspective. Journal of Business Research, 125 , 239–251.
Trivedi, J. P., & Trivedi, H. (2018). Investigating the factors that make a fashion app successful: The moderating role of personalization. Journal of Internet Commerce, 17 , 170–187.
Tseng, T. H., & Lee, C. T. (2018). Facilitation of consumer loyalty toward branded applications: The dual-route perspective. Telematics and Informatics, 35 , 1297–1309.
Turley, L. W., & Milliman, R. E. (2000). Atmospheric effects on shopping behavior: A review of the experimental evidence. Journal of Business Research, 49 (2), 193–211.
Tyler, T. R. (2020). Social justice: Outcome and procedure. International Journal of Psychology, 35 , 117–125.
Tyrväinen, O., & Karjaluoto, H. (2019). A systematic literature review and analysis of mobile retailing adoption. Journal of Internet Commerce, 18 , 221–247.
Unity Developers (2021). 2021 is a Year of a Substantial Rise of AR and VR Apps. Retrieved on 24 th July from https://unitydevelopers.co.uk/2021-is-a-year-of-a-substantial-rise-of-ar-and-vr-apps/ .
Urban, G. L., & Sultan, F. (2015). The case for 'benevolent' mobile apps. MIT Sloan Management Review, 56 (2), 31.
van Doorn, J., Lemon, K. N., Mittal, V., Nass, S., Pick, D., Pirner, P., & Verhoef, P. C. (2010). Customer engagement behavior: Theoretical foundations and research directions. Journal of Service Research, 13 , 253–266.
van Esch, P., Arli, D., Gheshlaghi, M. H., Andonopoulos, V., von der Heidt, T., & Northey, G. (2019). Anthropomorphism and augmented reality in the retail environment. Journal of Retailing and Consumer Services, 49 , 35–42.
van Heerde, H. J., Dinner, I., & Neslin, S. A. (2019). Engaging the unengaged customer: The value of a retailer mobile app. International Journal of Research in Marketing, 36 (3), 420–438.
van Noort, G., & van Reijmersdal, E. A. (2019). Branded apps: Explaining effects of brands' mobile phone applications on brand responses. Journal of Interactive Marketing, 45 , 16–26.
Vargo, S. L., & Lusch, R. F. (2004). Evolving to a new dominant logic for marketing. Journal of Marketing, 68 , 1–17.
Venkatesh, V., Morris, M. G., Davis, G. B., & Davis, F. D. (2003). User acceptance of information technology: Toward a unified view. MIS quarterly , 425-478.
Venkatesh, V., Thong, J. Y. L., & Xu, X. (2012). Consumer acceptance and use of information technology: Extending the unified theory of acceptance and use of technology. MIS Quarterly, 36 , 157–178.
Verhoef, P. C., Lemon, K. N., Parasuraman, A., Roggeveen, A., Tsiros, M., & Schlesinger, L. A. (2009). Customer experience creation: Determinants, dynamics and management strategies. Journal of Retailing, 85 , 31–41.
Veríssimo, J. M. C. (2018). Usage intensity of mobile medical apps: A tale of two methods. Journal of Business Research, 89 , 442–447.
Viswanathan, V., Hollebeek, L., Malthouse, E., Maslowska, E., Kim, S. J., & Xie, W. (2017). The dynamics of consumer engagement with mobile technologies. Service Science, 9 , 36–49.
Vivek, S. D., Beatty, S. E., & Morgan, R. M. (2012). Customer engagement: Exploring customer relationships beyond purchase. Journal of Marketing Theory and Practice, 20 , 122–146.
Vroom, V. H. (1964). Work and motivation . Wiley and Sons.
Wang, B., Kim, S.-J., & Malthouse, E. (2016a). Branded apps and mobile platforms as new tools for advertising. In R. Brown, V. Jones, & M. Wang (Eds.), The new advertising: Branding, content, and consumer relationships in the data-driven social media era . Praeger.
Wang, D., Xiang, Z., Law, R., & Ki, T. P. (2016b). Assessing hotel-related smartphone apps using online reviews. Journal of Hospitality Marketing & Management, 25 , 291–313.
Wang, J., Lai, J., & Chang, C. (2016c). Modeling and analysis for mobile application services: The perspective of mobile network operators. Technological Forecasting and Social Change, 111 , 146–163.
Wang, W., & Li, H. (2012). Factors influencing mobile services adoption: A brand–equity perspective. Internet Research, 22 , 142–179.
Wang, W., Ou, W., & Chen, W. (2019). The impact of inertia and user satisfaction on the continuance intentions to use mobile communication applications: A mobile service quality perspective. International Journal of Information Management, 44 , 178–193.
Wang, Y., Lin, H., & Luarn, P. (2006). Predicting consumer intention to use mobile service. Information Systems Journal, 16 , 157–179.
Wang, Z., Zhao, H., & Wang, Y. (2015). Social networks in marketing research 2001–2014: A co-word analysis. Scientometrics, 105 , 65–82.
Watson, C., McCarthy, J., & Rowley, J. (2013). Consumer attitudes towards mobile marketing in the smart phone era. International Journal of Information Management, 33 , 840–849.
Wattanapisit, A., Teo, C. H., Wattanapisit, S., Teoh, E., Woo, W. J., & Ng, C. J. (2020). Can mobile health apps replace GPs? A scoping review of comparisons between mobile apps and GP tasks. BMC Medical Informatics and Decision Making, 20.
Weber, E. U., Blais, A. R., & Betz, N. E. (2002). A domain-specific risk-attitude scale: Measuring risk perceptions and risk behaviors. Journal of Behavioral Decision Making, 15 (4), 263–290.
Wedel, M., Bigné, E., & Zhang, J. (2020). Virtual and augmented reality: Advancing research in consumer marketing. International Journal of Research in Marketing, 37 (3), 443–465.
Wei, J., Vinnikova, A., Lu, L., & Xu, J. (2020). Understanding and predicting the adoption of fitness mobile apps: Evidence from China. Health Communication , 1–12.
Wheeler, B. C. (2002). NEBIC: A dynamic capabilities theory for assessing net-enablement. Information Systems Research, 13 (2), 125–146.
Williams, K (2020). TikTok Was Installed More Than 738 Million Times in 2019, 44% of Its All-Time Downloads , Retrieved 1 st June, 2020 from https://sensortower.com/blog/tiktok-revenue-downloads-2019/ .
Wong, T. Y. T., Peko, G., Sundaram, D., & Piramuthu, S. (2016). Mobile environments and innovation co-creation processes & ecosystems. Information & Management, 53 , 336–344.
Woodside, A. G., Golfetto, F., & Gibbert, M. (2008). Customer value: Theory, research, and practice. In Creating and managing superior customer value . Emerald Group Publishing Limited.
Wu, J., & Wang, S. C. (2005). What drives mobile commerce? An empirical evaluation of the revised technology acceptance model. Information & Management, 42 , 719–729.
Wu, L, & Ye, Y. (2013). Understanding Impulsive buying behavior in mobile commerce. PACIS 2013 Proceedings, 142, https://aisel.aisnet.org/pacis2013/142 .
Wu, L. (2015). Factors of continually using branded mobile apps: The central role of app engagement. International Journal of Internet Marketing and Advertising, 9 , 303–320.
Xu, C., Peak, D., & Prybutok, V. (2015). A customer value, satisfaction, and loyalty perspective of mobile application recommendations. Decision Support Systems, 79 , 171–183.
Yang, B. (2016). A link between consumer empathy and brand attachment on branded mobile apps: The moderating effect of ideal self-congruence. Indian Journal of Science & Technology, 9 , 1–9.
Yang, H. C. (2013). Bon appètit for apps: Young American consumers’ acceptance of mobile applications. Journal of Computer Information Systems, 53 , 85–96.
Yang, K. C. C. (2005). Exploring factors affecting the adoption of mobile commerce in Singapore. Telematics & Informatics, 22 , 257–277.
Yang, Z., & Peterson, R. T. (2004). Customer perceived value, satisfaction, and loyalty: The role of switching costs. Psychology & Marketing, 21 (10), 799–822.
Yaoyuneyong, G., Foster, J., Johnson, E., & Johnson, D. (2016). Augmented reality marketing: Consumer preferences and attitudes toward hypermedia print ads. Journal of Interactive Advertising, 16 (1), 16–30.
Yoo, C., Park, J., & MacInnis, D. J. (1998). Effects of store characteristics and in-store emotional experiences on store attitude. Journal of Business Research, 42 (3), 253–263.
Yoo, Y. (2010). Computing in everyday life: A call for research on experiential computing. MIS Quarterly , 213–231.
Yoon, C. (2011). Theory of planned behavior and ethics theory in digital piracy: An integrated model. Journal of Business Ethics, 100 , 405–417.
Zaichkowsky, J. L. (1986). Conceptualizing involvement. Journal of Advertising, 15 (2), 4–34.
Zhang, T., Lu, C., & Kizildag, M. (2017). Engaging generation Y to co-create through mobile technology. International Journal of Electronic Commerce, 21 , 489–516.
Zhao, Z., & Balagué, C. (2015). Designing branded mobile apps: Fundamentals and recommendations. Business Horizons, 58 , 305–315.
Zhu, G., So, K. F., & Hudson, S. (2017). Inside the sharing economy: Understanding consumer motivations behind the adoption of mobile applications. International Journal of Contemporary Hospitality Management, 29 , 2218–2239.
Zolkepli, I. A., Mukhiar, S. N. S., Tan, C. (2020). Mobile consumer behaviour on apps usage: The effects of perceived values, rating, and cost. Journal of marketing communications (published electronically April 3), DOI: 10.1080/13527266.2020.1749108.
Download references
Acknowledgements
The authors wish to thank Maria Flutsch and Chandler Meakins for assisting with the management of the references. They also would like to thank Bryony Jardine for her assistance in the bibliometric analysis that underpins this study. Finally, the authors dedicate this work to Lucas Taousakis, who came to this world amid the first round of revisions and is the son of the lead author.
Author information
Authors and affiliations.
UniSA Business, University of South Australia (UniSA), Adelaide, South Australia
Lara Stocchi & Arry Tanusondjaja
College of Business, Government and Law, Flinders University, Adelaide, South Australia
Naser Pourazad
School of Business and Economics, Loughborough University, Leicestershire, UK
Nina Michaelidou
UWA Business School, University of Western Australia (UWA), Perth, Western Australia
Paul Harrigan
You can also search for this author in PubMed Google Scholar
Corresponding author
Correspondence to Lara Stocchi .
Additional information
Publisher’s note.
Springer Nature remains neutral with regard to jurisdictional claims in published maps and institutional affiliations.
Shailendra Jain served as Area Editor for this article
Rights and permissions
Reprints and permissions
About this article
Stocchi, L., Pourazad, N., Michaelidou, N. et al. Marketing research on Mobile apps: past, present and future. J. of the Acad. Mark. Sci. 50 , 195–225 (2022). https://doi.org/10.1007/s11747-021-00815-w
Download citation
Received : 12 October 2020
Accepted : 25 September 2021
Published : 08 November 2021
Issue Date : March 2022
DOI : https://doi.org/10.1007/s11747-021-00815-w
Share this article
Anyone you share the following link with will be able to read this content:
Sorry, a shareable link is not currently available for this article.
Provided by the Springer Nature SharedIt content-sharing initiative
- Mobile applications
- Mobile apps
- Customer journey
- Customer experience
- Find a journal
- Publish with us
- Track your research
Online Grocery Shopping Adoption: A Systematic Literature Review
Ieee account.
- Change Username/Password
- Update Address
Purchase Details
- Payment Options
- Order History
- View Purchased Documents
Profile Information
- Communications Preferences
- Profession and Education
- Technical Interests
- US & Canada: +1 800 678 4333
- Worldwide: +1 732 981 0060
- Contact & Support
- About IEEE Xplore
- Accessibility
- Terms of Use
- Nondiscrimination Policy
- Privacy & Opting Out of Cookies
A not-for-profit organization, IEEE is the world's largest technical professional organization dedicated to advancing technology for the benefit of humanity. © Copyright 2024 IEEE - All rights reserved. Use of this web site signifies your agreement to the terms and conditions.
Academia.edu no longer supports Internet Explorer.
To browse Academia.edu and the wider internet faster and more securely, please take a few seconds to upgrade your browser .
Enter the email address you signed up with and we'll email you a reset link.
- We're Hiring!
- Help Center
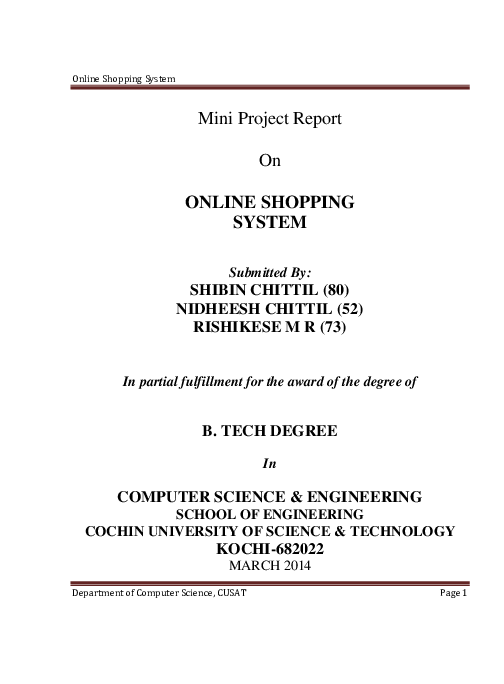
Mini Project Report On ONLINE SHOPPING SYSTEM

Related Papers
swarna prabha jena
Now a day’s Android are the best and the most popular operating systems for Smartphones. Smartphone comprise of an important features through which user can easily distribute applications via online market store. Usually, customers in retail stores stand in a queue to do the billing of the products they want to buy which makes the customers to wait for a long time till they reach to the billing person. As in this modern and fastidious world, each minute is valuable for us so, the Mobile Shopping application saves our estimable time by making the billing process faster. This paper composed of a new mobile Shop Application practiced for an Android Smartphones, meant for the sales persons of big retail stores. In this Android application, data is stored using SQLite which is a type of embedded database and is stored within a single file on a disk.
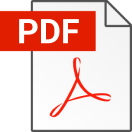
International Journal for Research in Applied Science & Engineering Technology (IJRASET)
IJRASET Publication
A Online Shopping for Retail Sector for B2C Collaboration using Android Studio is an app which allows merchants in developing counters to advertise and sell their goods. This would permit rural communities to make their wares available to the rest of the world. The objective of this project is to create a portal which would allow product information to be updated securely using a mobile device and will allow users to buy form the merchant. The main concern is given to local store to expand their business and work. In the future the internet will become whole and soul to the business fields, each and every trade is going to be done through it so this app may be helpful to the business person in this running world. The project E-commerce for SME's, is aimed to investigate the business potential of Internet for Small and Medium sized Enterprises (SME's) mainly in the UK and identifying the benefits and problems brought by the new medium. The Internet is offering SMEs a number of new opportunities that are cost effective and reliable (to a certain extent), thereby for the first time, providing them with an opportunity to compete with their larger counterparts. This project discusses the problems that SME's face while using or trying to use this technology. Problems, which are mainly cost, and skill based.
International Journal of Scientific Research in Science, Engineering and Technology IJSRSET
This paper describes the design and development of an android shopping application for city. It elaborates and shows offers and deals of particular malls and local market. Application shows offers but also provide analytics to the seller. Whole application is organized on cloud. The three-tier architecture includes front-end, middle-ware and back-end. The front-end level consists of location-based mobile shopping application for android mobile devices, for purchasing miscellaneous products from malls and nearby local markets. Front-end level also displays association among the purchased items. The middle-ware level offers a web service to generate JSON (JavaScript Object Notation) yield from the relational database. It exchanges info and data between application and servers in cloud. The back-end level offers the Apache Tomcat Web server and MySQL database. This application is based on cloud that provides application as a service to user.
Ronnel Cataluña
International Conference on eBusiness, eCommerce, eManagement, eLearning and eGovernance 2014
Android is Google's latest open source software platform for mobile devices which has already attained enormous popularity. The purpose of this paper is to describe the development of mobile application for shopping mall using Android platform. A prototype was developed for the shoppers of Bashundhara Shopping Mall of Bangladesh. This prototype will serve as a framework for any such applications (apps). The paper presents a practical demonstration of how to integrate shops' information, such as names, categories, locations, descriptions, floor layout and so forth, with map module via an android application. A summary of survey results of the related literature and projects have also been included. Critical Evaluation of the prototype along with future research and development plan has been revealed. The paper will serve as a guideline for the researchers and developers to introduce and develop similar apps.
Dr. Subash Chandra Bose J
ABSTRACT: The customer turning their faith on purchasing their project in online.But the real visualization and product reliability can’tbe seen in online.Hence this paper deals with a virtualization of the road and shops where the person can directly go search shop and purchase it in the real time and also customer can directly buy the product in the retail shop and also it gains the navigation of the information.
Faria Soroni
International Journal of Engineering and Advanced Technology
Indra Yogaswara
Buying and selling mobile phones which located at Raya Kedondong Street Waylima Subdistrict, Pesawaran District is a store that is engaged to develop, improving, and promoting the store so that it can be known outside the region. So with this, it is necessary to give easy service to the customers who are far from reach. It can be realized by (E-Commerce). Then an application system was built to help the service information about buying and selling mobile phones which uses the SDLC method, that will produce an information system related to buying and selling mobile phones. With this application, it will help the seller in managing data of ordering goods, customer data, and facilitate consumers in finding information about prices and brands of mobile phones.
Vasilyn Manalo
— Mobile and e-commerce applications are tools for accessing the Internet and for buying products and services. These applications are constantly evolving due to the high rate of technological advances being made. This paper provides a new perspective on the types of applications that can be used. It describes and analyses device requirements, provides a literature review of important aspects of mobile devices that can use such applications and the requirements of websites designed for m-commerce. The design and security aspects of mobile devices are also investigated. As an alternative to existing m-commerce applications, this paper also investigates the characteristics and potential of the PhoneGap cross-mobile platform application. The results suggest that effective mobile applications do exist for various Smartphones, and web applications on mobile devices should be effective. PhoneGap and Spree applications can communicate using JSON instead of the XML language. Android simulators can be used for ensuring proper functionality and for compiling the applications.
Journal of Computer and Communications
Günay Gültekin
RELATED PAPERS
TERMURAH !! 0817-1770-1571 MAXINDO, Perusahaan Ekspedisi di Jakarta Timur
Maxindo Ekspedisi
Agen Kompor Kaca Progas
Tér és Társadalom
Györgyi Barta
Recherches féministes
Lucie Lequin
Xix Salao De Iniciacao Cientifica E Tecnologica
Mara von Mühlen
Current Zoology
Elisabetta Palagi
Signos Filosoficos
alejandro gonzalez
Cadernos de Estudos Lingüísticos
solange vereza
Joseph Joseph
APOKALYPSIS, PARA PIANO E VIOLINO: MEMORIAL DE COMPOSIÇÃO
Thiago Busch
Journal of Medical Imaging and Radiation Sciences
Hazel Neser
Proceedings of the National Academy of Sciences of the United States of America
Mayfong Mayxay
Katalin Ujhelyi
Scientific Journal of Kurdistan University of Medical Sciences
Afshin Maleki
World Journal of Surgical Oncology
Hiroharu Yamashita
International Immunology
andres sebastian ojeda hidalgo
Journal of Economic Development
Shahriar kabir
International Journal of Creative Research Thought
Nishita Jataniya , Twinkle Rajyaguru
Gabriela Castaño
Solid State Ionics
Masaki Matsui
JRSKT: Jurnal Riset Sains dan Kimia Terapan
eka rizki amelia
Revista Ibero-Latinoamericana de Seguros
Andrés Orión Álvarez
Marta Portugal
yyjugf hfgerfd
Computers and Electronics in Agriculture
Remigio Berruto
- We're Hiring!
- Help Center
- Find new research papers in:
- Health Sciences
- Earth Sciences
- Cognitive Science
- Mathematics
- Computer Science
- Academia ©2024
Numbers, Facts and Trends Shaping Your World
Read our research on:
Full Topic List
Regions & Countries
- Publications
- Our Methods
- Short Reads
- Tools & Resources
Read Our Research On:
About 1 in 5 U.S. teens who’ve heard of ChatGPT have used it for schoolwork
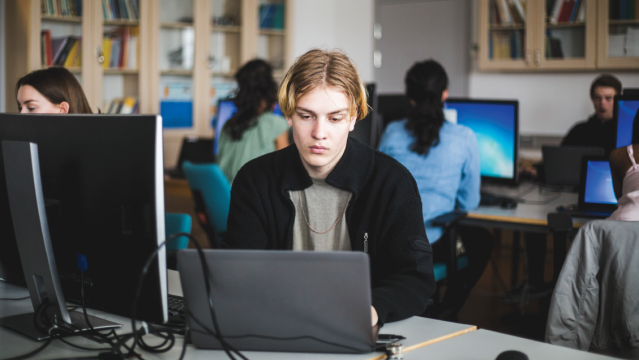
Roughly one-in-five teenagers who have heard of ChatGPT say they have used it to help them do their schoolwork, according to a new Pew Research Center survey of U.S. teens ages 13 to 17. With a majority of teens having heard of ChatGPT, that amounts to 13% of all U.S. teens who have used the generative artificial intelligence (AI) chatbot in their schoolwork.
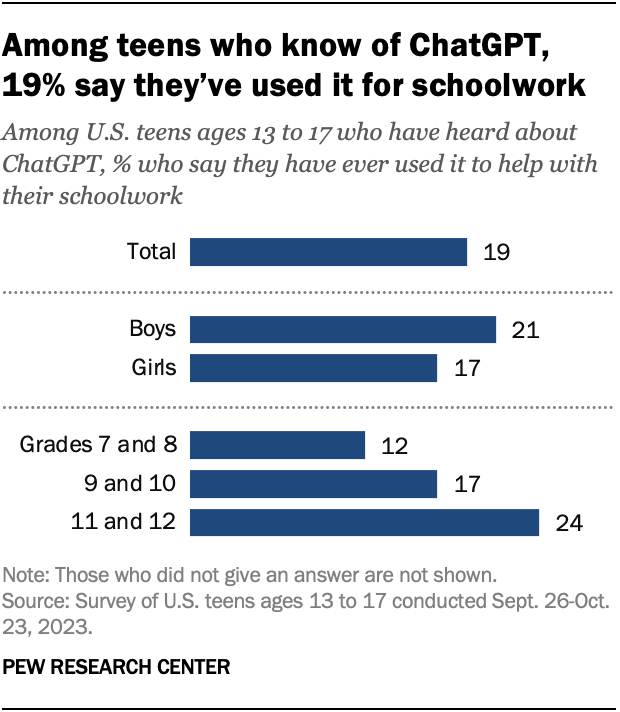
Teens in higher grade levels are particularly likely to have used the chatbot to help them with schoolwork. About one-quarter of 11th and 12th graders who have heard of ChatGPT say they have done this. This share drops to 17% among 9th and 10th graders and 12% among 7th and 8th graders.
There is no significant difference between teen boys and girls who have used ChatGPT in this way.
The introduction of ChatGPT last year has led to much discussion about its role in schools , especially whether schools should integrate the new technology into the classroom or ban it .
Pew Research Center conducted this analysis to understand American teens’ use and understanding of ChatGPT in the school setting.
The Center conducted an online survey of 1,453 U.S. teens from Sept. 26 to Oct. 23, 2023, via Ipsos. Ipsos recruited the teens via their parents, who were part of its KnowledgePanel . The KnowledgePanel is a probability-based web panel recruited primarily through national, random sampling of residential addresses. The survey was weighted to be representative of U.S. teens ages 13 to 17 who live with their parents by age, gender, race and ethnicity, household income, and other categories.
This research was reviewed and approved by an external institutional review board (IRB), Advarra, an independent committee of experts specializing in helping to protect the rights of research participants.
Here are the questions used for this analysis , along with responses, and its methodology .
Teens’ awareness of ChatGPT
Overall, two-thirds of U.S. teens say they have heard of ChatGPT, including 23% who have heard a lot about it. But awareness varies by race and ethnicity, as well as by household income:
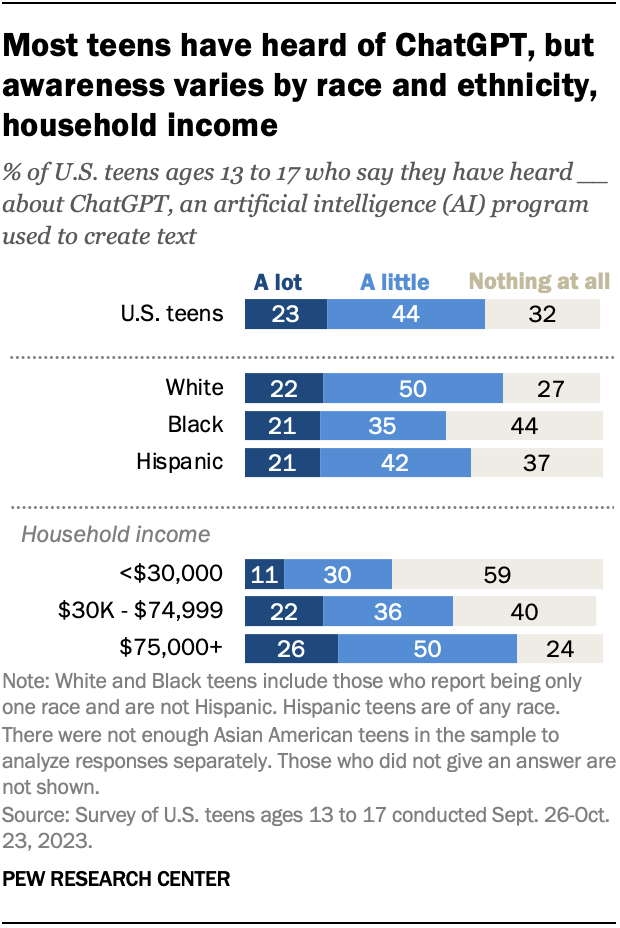
- 72% of White teens say they’ve heard at least a little about ChatGPT, compared with 63% of Hispanic teens and 56% of Black teens.
- 75% of teens living in households that make $75,000 or more annually have heard of ChatGPT. Much smaller shares in households with incomes between $30,000 and $74,999 (58%) and less than $30,000 (41%) say the same.
Teens who are more aware of ChatGPT are more likely to use it for schoolwork. Roughly a third of teens who have heard a lot about ChatGPT (36%) have used it for schoolwork, far higher than the 10% among those who have heard a little about it.
When do teens think it’s OK for students to use ChatGPT?
For teens, whether it is – or is not – acceptable for students to use ChatGPT depends on what it is being used for.
There is a fair amount of support for using the chatbot to explore a topic. Roughly seven-in-ten teens who have heard of ChatGPT say it’s acceptable to use when they are researching something new, while 13% say it is not acceptable.
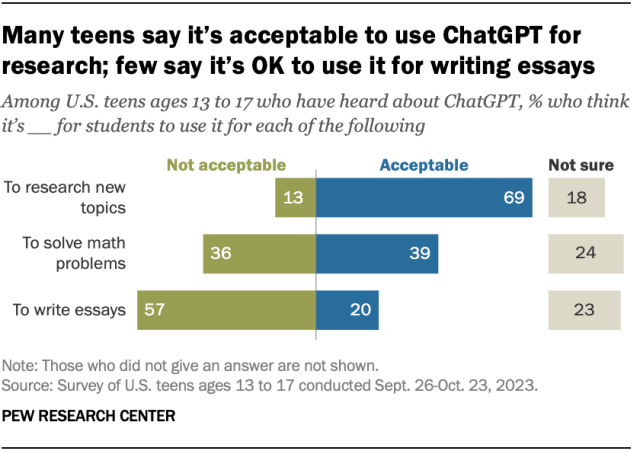
However, there is much less support for using ChatGPT to do the work itself. Just one-in-five teens who have heard of ChatGPT say it’s acceptable to use it to write essays, while 57% say it is not acceptable. And 39% say it’s acceptable to use ChatGPT to solve math problems, while a similar share of teens (36%) say it’s not acceptable.
Some teens are uncertain about whether it’s acceptable to use ChatGPT for these tasks. Between 18% and 24% say they aren’t sure whether these are acceptable use cases for ChatGPT.
Those who have heard a lot about ChatGPT are more likely than those who have only heard a little about it to say it’s acceptable to use the chatbot to research topics, solve math problems and write essays. For instance, 54% of teens who have heard a lot about ChatGPT say it’s acceptable to use it to solve math problems, compared with 32% among those who have heard a little about it.
Note: Here are the questions used for this analysis , along with responses, and its methodology .
- Artificial Intelligence
- Technology Adoption
- Teens & Tech
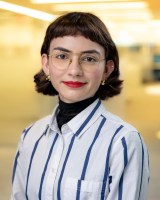
Olivia Sidoti is a research assistant focusing on internet and technology research at Pew Research Center
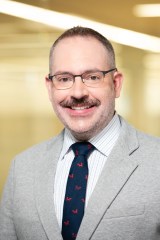
Jeffrey Gottfried is an associate director focusing on internet and technology research at Pew Research Center
Many Americans think generative AI programs should credit the sources they rely on
Americans’ use of chatgpt is ticking up, but few trust its election information, q&a: how we used large language models to identify guests on popular podcasts, striking findings from 2023, what the data says about americans’ views of artificial intelligence, most popular.
1615 L St. NW, Suite 800 Washington, DC 20036 USA (+1) 202-419-4300 | Main (+1) 202-857-8562 | Fax (+1) 202-419-4372 | Media Inquiries
Research Topics
- Age & Generations
- Coronavirus (COVID-19)
- Economy & Work
- Family & Relationships
- Gender & LGBTQ
- Immigration & Migration
- International Affairs
- Internet & Technology
- Methodological Research
- News Habits & Media
- Non-U.S. Governments
- Other Topics
- Politics & Policy
- Race & Ethnicity
- Email Newsletters
ABOUT PEW RESEARCH CENTER Pew Research Center is a nonpartisan fact tank that informs the public about the issues, attitudes and trends shaping the world. It conducts public opinion polling, demographic research, media content analysis and other empirical social science research. Pew Research Center does not take policy positions. It is a subsidiary of The Pew Charitable Trusts .
Copyright 2024 Pew Research Center
Terms & Conditions
Privacy Policy
Cookie Settings
Reprints, Permissions & Use Policy
Haney vs. Garcia Pay-Per-View: Here’s How To Watch the Boxing Livestream Online
A highly-anticipated matchup pits two superstar boxers in the ring tonight.
By Rudie Obias
Rudie Obias
- Traveling for the Miami Grand Prix? Here Are the Best Places to Stay for the Formula One Event 2 days ago
- How to Watch New Episodes of ‘Bluey’ and the ‘Bluey’ Movie Special, ‘The Sign’ 4 days ago
- Haney vs. Garcia Pay-Per-View: Here’s How To Watch the Boxing Livestream Online 4 days ago

If you purchase an independently reviewed product or service through a link on our website, Variety may receive an affiliate commission.
After meeting six times at the amateur levels (the pair split the contests 3-3), Devin “The Dream” Haney was set to defend his WBC Super Lightweight Title tonight against his rival Ryan “Super Ry” Garcia. But after Garcia failed to make weight, the superstar boxer will no longer be qualified to win Haney’s belt should he be victorious. Still, the fight will go on as scheduled, and boxing fans are in for a treat.
Popular on Variety
Not a subscriber? You can sign up for a 30-day free trial to access the PPV feed , as well as more than 100 live fights. Subscribers can also access fight replays, highlights and documentaries. After the free trial is finished, you can cancel the service with a 30-day notice, or keep watching for $19.99/month. You’re essentially getting two months for the price of one.
If you’d like to watch Haney vs. Garcia without a DAZN subscription, you can purchase access to the PPV fight for $79.99 as a standalone event.
DAZN subscribers can also watch Haney vs. Garcia prelims online free.
Although the bout is formidable, Haney is the clear favorite — especially with a record of 31-0 with 15 knockouts. However, Garcia is no slouch himself with a record of 24-1 with 20 KOs. And with a few spicy exchanges during the fight’s press conference , it’s shaping up to be a hot one in Brooklyn.
Main Fight Card, 8 p.m. ET/5 p.m. PT
- Super Lightweight: Devin Haney (champion) vs. Ryan Garcia — WBC Super Lightweight Title
- Super Flyweight: John Ramirez vs. David Jimenez — WBA Interim Flyweight Title
- Super Lightweight: Arnold Barboza Jr. vs. Sean McComb
- Super Middleweight: Bektemir Melikuziev vs. Pierre Dibombe
- Light middleweight: Charles Conwell vs. Nathaniel Gallimore
Devin Haney vs. Ryan Garcia pay-per-view is streamable on DAZN on Saturday, April 20, at 8 p.m. ET/5 p.m. PT with the main event at 11 p.m. ET/8 p.m. PT.
More From Our Brands
Kanye west announces ‘yeezy porn’ amid reports of adult film company, no kidding, swizz beatz owns a camel-racing team—and it could win him $21 million, sue bird joins seattle storm ownership group, be tough on dirt but gentle on your body with the best soaps for sensitive skin, dead boy detectives showrunners talk potential sandman crossovers and supernatural easter eggs, verify it's you, please log in.
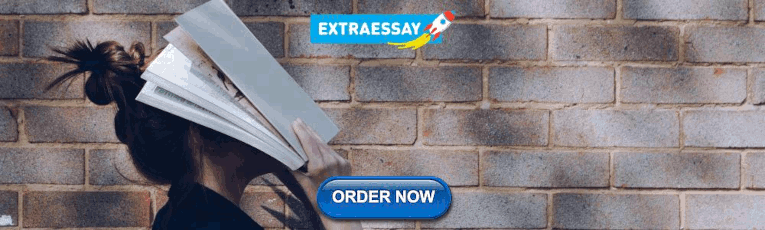
IMAGES
VIDEO
COMMENTS
Research offers some indication that the online customers' shopping experience (OCSE) can be a strong predictor of online impulsive buying behavior, but there is not much empirical support available to form a holistic understanding; whether, and indeed how, the effects of the OCSE on online impulsive buying behavior are affected by customers' attitudinal loyalty and self-control are not well ...
This paper extends the existing knowledge of the online shopping behaviour of consumers in the e-commerce context, thus revealing and sharing insights that are relevant to individuals and businesses with a common interest in the theoretical implications, including the managerial strategies that would be suitable for growing B2C e-commerce in ...
The author found that the main factors that affect online shopping are convenience and attractive pricing/discount. Advertising and recommendations were among the least effective. In the study by Lian and Yen (2014), authors tested the two dimensions (drivers and barriers) that might affect intention to purchase online.
The research paper focuses on the development of two scales to measure the applicability of the technology acceptance model and shopping motives behind the use of mobile shopping apps. Qualitative ...
Purpose. The purpose of this paper is to examine the influence of several intrinsic motivations driving consumers' intention to buy using a mobile app, namely: shopping gamification, focussed attention, shopping enjoyment and socialness, through the mediating role of shopping engagement. The online shopping experience is investigated in its ...
This article attempts to take stock of this environment to critically assess the research gaps in the domain and provide future research directions. Applying a well-grounded systematic methodology following the TCCM (theory, context, characteristics and methodology) framework, 197 online consumer shopping behaviour articles were reviewed.
Introduction. The growth of e-commerce is rapidly changing consumers' shopping habits and shaping the future of the retail industry. Many factors are driving this growth, including the proliferation of smartphones, tablets, and other portable devices; increased consumer confidence in online shopping; and the retailers' increased investments in the online channel and digital marketing budgets.
The primary research objectives were to determine whether the respondents were demonstrating a significant increase in the amount of usage of online shopping through mobile phone app before and during the Covid-19 pandemic time and also to determine whether there was a difference Małgorzata Wiścicka-Fernando et al. / Procedia Computer ...
Introduction. Online shopping is the act of buying a product or service through any e-stores with the help of any website or app. Tarhini et al. (2021) stated that shopping through online channels is actively progressing due to the opportunity to save time and effort. Furthermore, online shopping varies from direct e-store and indirect e-store about their perception against the actual experience.
A final dataset of 893 valid and complete questionnaires was used for the empirical analysis. 893 surveys were collected with 60.5% male. Table 2 shows the main demographics of the sample and a list of the main retailers used for their online shopping: Taobao (63%), Tmall (15%) and JD.com (12%) are the most used.
Online shopping is a process whereby consumers directly buy goods, services etc. from a. seller without an intermediary service over the Internet. Shoppers can visit web stores. from the comfort ...
Mobile apps, or apps in short, have been defined as the ultimate marketing vehicle (Watson, McCarthy and Rowley 2013) and a staple promotional tactic (Rohm, Gao, Sultan and Pagani 2012) to attract business 'on the go' (Fang 2019).They yield great potential for customer engagement due to specific characteristics (e.g., vividness, novelty and built-in features, see Kim, Lin and Sung 2013 ...
Online shopping is a form of electronic commerce which allows consumers to directly buy goods. or services from a sell er over the Internet using a web browser. The online stores are using ...
In the study by Lian and Yen ( 2014 ), authors tested the two dimensions (drivers and barriers) that might affect intention to purchase online. Drivers consisted of performance expectation, effort expectation, social influence and facilitating conditions. Usage, value, risk, tradition and image were all among barriers.
Academia.edu is a platform for academics to share research papers. CONSUMERS' BUYING BEHAVIOR ON ONLINE SHOPPING: AN UTAUT AND LUM MODEL APPROACH ... The researcher also recommends to them to do some deeper research about online shopping in order for them to know and learn more about this topic, since the researchers Senior High School ...
Segmentating Customers in Online Stores from Factors that Affect the Customer's Intention to Purchase., (pp. 383-388). Kim, H., Song, J., 2010. The Quality of Word-of Mouth in the Online Shopping Mall. Journal of Research in Interactive Marketing, 4(4), 376- 390. Kim, S., Jones, C., 2009. Online Shopping and Moderating Role of Offline Brand Trust.
Americans are incorporating a wide range of digital tools and platforms into their purchasing decisions and buying habits, according to a Pew Research Center survey of U.S. adults. The survey finds that roughly eight-in-ten Americans are now online shoppers: 79% have made an online purchase of any type, while 51% have bought something using a ...
E-Commerce is the Digital Technology of buying or selling products online. In 2020, E-Commerce of India is predicted to bounce from certain billion of its income to tremendous increase in total income which is highest in the world. The sector of online purchase is prominent by the penetration of mobile, increasing internet, growing facility of e-payments among users has improved the purchase ...
This article presents a Systematic Literature Review or SLR of the emerging `e-grocery adoption' research scope. It proposes grocery application adoption or online grocery shopping adoption that enriches marketing study. This study aims to draft and critically reexamine the article in the features of the grocery application adoption area. The SLR delivered 38 studies presenting jointly to ...
Contact No. 9909877266. [email protected]. Abstract. All of the businesses today as w e see are done over the internet and anything which is not there. is meant to be wiped off. E commerce ...
In the second quarter of 2020, for instance, e-commerce sales totaled $205.3 billion, up 55% from the $132.3 billion recorded a year earlier. In the fourth quarter of 2020, e-commerce accounted for 16.7% of all retail sales, still the record-high share. That share fell back as stores reopened and consumers gradually resumed their old shopping ...
The project objective is to deliver the online shopping application into android platform. Online shopping is the process whereby consumers directly buy goods or services from a seller in real-time, without an intermediary service, over the Internet. It is a form of electronic commerce.
Age gaps are modest when it comes to shopping on a tablet. Still, those ages 30 and older are more likely than those 18 to 29 to say they ever use a tablet to purchase things online (30% vs. 20%). Experiences shopping on a desktop or laptop computer do not statistically differ across age groups. Online shopping habits also vary by household income.
During t he survey period, around 33 percent of banking consumers aged 27 to 37. stated that they used mobile apps for online shopping payments in India. [13] The current e-commerce penetration in ...
Pew Research Center conducted this analysis to understand American teens' use and understanding of ChatGPT in the school setting. The Center conducted an online survey of 1,453 U.S. teens from Sept. 26 to Oct. 23, 2023, via Ipsos. Ipsos recruited the teens via their parents, who were part of its KnowledgePanel. The KnowledgePanel is a ...
Devin Haney vs. Ryan Garcia pay-per-view is streamable on DAZN on Saturday, April 20, at 8 p.m. ET/5 p.m. PT with the main event at 11 p.m. ET/8 p.m. PT. Watch Haney vs. Garcia PPV on DAZN $69.99 ...
Proposed system and Objectives. The proposed Online Grocery shopping is an intelligent multistage mobile application that helps customers shop. and delivers the needed items quickly and safely. It ...