
An official website of the United States government
The .gov means it’s official. Federal government websites often end in .gov or .mil. Before sharing sensitive information, make sure you’re on a federal government site.
The site is secure. The https:// ensures that you are connecting to the official website and that any information you provide is encrypted and transmitted securely.
- Publications
- Account settings
Preview improvements coming to the PMC website in October 2024. Learn More or Try it out now .
- Advanced Search
- Journal List
- Europe PMC Author Manuscripts
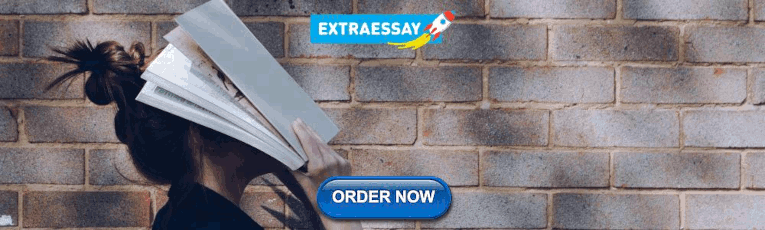
Effect of Speaking Environment on Speech Production and Perception
Peter howell.
1) Division for Psychology and Language Sciences, Faculty of Life Sciences, University College, London
Environments affect speaking and listening performance. This contribution reviews some of the main ways in which all sounds are affected by the environment they propagate into. These influences are used to assess how environments affect speakers and listeners. The article concludes with a brief consideration of factors that designers may wish to take into account to address the effects.
1. Introduction
This work considers how environments affect speech behavior. The principal effect environments have on produced speech is that they modify its acoustic structure and, when a speaker hears this altered speech, it can influence the properties of the speech that is uttered. The changes to the speech sound and noise sources that are present in the environment can affect how listeners process the speech sounds. This contribution starts with a brief review of the way environments alter sounds. It then considers how psychologists have determined how the environment influences speech production and perception. Where speech production and/or perception are adversely affected, designers may wish to modify the environment to reduce the impact of these influences.
2. How environments affect sounds
In free field conditions there are no walls or objects to affect sound as there are in enclosed spaces. When a sound enters an enclosed space or a space with obstacles, it is affected in several ways. Three ways in which sounds are affected by environments are discussed: timing, frequency and intensity structure.
The temporal properties of sounds are altered in different ways which depend on the dimensions of the room, its contents and the material on the walls. Perhaps the most obvious effect is when an echo occurs. This is a single repetition of the sound source caused by the sound-wave coming against an opposing surface which reflects it. Echoes occur when there is more than one repetition. Some buildings (such as mosques) have been specifically designed to enhance echoes.
The time the echoes arrive after the direct sound depends on the room’s dimensions. The delay between the original sound and the first echo can be calculated using the distance between the sound source and the reflecting object divided by the speed of sound. For example, if a wall is 17 meters from a sound source and the speed of sound is 340 meters per second, the echo will be heard at the origin after a delay of 0.1 seconds (2 × 17/340 seconds). The strength of an echo depends on room contents and dimensions. Strength is specified in dB sound pressure level relative to the directly transmitted wave.
Psychologists often use a procedure where a speaker hears the sound of his or her voice after a delay (commonly called delayed auditory feedback, DAF, which is discussed further in see section 3.1). It is often assumed that the delayed signal is the only sound the speaker hears. Although the voice may be heavily attenuated, it is unlikely that the speaker does not hear any of the direct voice (i.e. at zero delay). Thus the speaker hears a mixture of sound with no delay and after one or more echo.
2.2 Frequency
Rooms are resonant structures though the material in the room damps these resonances to different extents. Each room has its particular frequency response. Sounds are filtered in their passage through a room space (some frequencies pass easily into the room and others do not). For speakers using amplification signals, the equipment itself can alter the frequency content.
2.3 Intensity
Effects such as echoes, discussed earlier, and amplifiers affect the intensity of any perceived or produced sound. A sound traveling directly from its source to a receiver will be attenuated as distance increases, which will be affected by the transmission medium. In real environments, sound sources will enter environments where there may be other noises (including echoes from past sounds). Speech production is affected when there are sounds in the environment other than that which the speaker makes. These extraneous sounds disrupt the speaker’s intensity control. Section 3.3 describes how a speaker’s vocal intensity depends on whether they are speaking while hearing their own speech or other noises. The behavior of a reception device (including a listener) that needs to detect or identify a speech sound will be affected when there are extraneous sound sources (in psychoacoustics, the extra sounds are said to mask the signal; see section 4.1). Another example of how extraneous sounds affect listeners is the cocktail party phenomenon where a listener needs to distinguish one voice so as to be able to follow a conversation. Extraneous sounds can be other voices or non-speech noises.
3. How environments affect speech production
The effects environments have on speech production are considered before the effects on perception. This is because if the speaker is present in the environment, listening performance will be affected by how speech is changed by the environment as well as by the way the environment affects listening. The listener will receive the altered sound the speaker makes and the environment will affect processing by the listener too.
During the 1950s, the rapid growth in phone use raised interest in how hearing a delayed sound affected speech control ( CCITT, 1989a , 1989b ). Speaking along with a delayed version of the voice (DAF) is an on-going problem in telephony with the introduction of cellular phones and satellite technology. Many of the findings have relevance for speaking in rooms with echo. It was found that speaking along with a delayed version of the voice (DAF) caused drawling (usually on the medial vowels), led to a Lombard effect (increased voice level), while pitch became monotone, speech errors arose and messages took longer to complete than messages produced in normal listening conditions ( Fairbanks, 1955 ). It should be noted that in addition to time alterations, telephones can transmit a limited range of frequencies, and the voice can be masked by noise on the equipment.
One question that arises is whether the delayed sound during DAF has to be speech to produce the disruptions to fluent speakers’ speech? Howell and Archer (1984) addressed this question by transforming speech into a noise that had the same temporal structure as speech, but none of the phonetic content. Then they delayed the noise sound and compared performance of this with performance under standard DAF. The two conditions produced equivalent disruption over a range of delays. This suggests that the DAF signal does not need to be a speech sound to affect control in the same way as observed under DAF. It appears from these results that speech does not go through the speech comprehension system where its message content is determined and which is then used as feedback to the linguistic processes. The disruption could arise instead if asynchronous inputs affect operation of lower level mechanisms involved in motor control.
Not all speakers are adversely affected by DAF. For instance, fluent speakers vary in their susceptibility to DAF. Howell and Archer (1984) went on to show that susceptibility depended on loudness of the voice, which determined level of feedback (in their experiments feedback could be speech or non-speech noises). A second surprising finding was that the fluency of people who stutter improved when they were played DAF. Researchers who investigated the fluency-enhancing effects of DAF on people who stutter in the 1950s and 1960s include Nessel (1958) , Soderberg (1960) , Chase et al., (1961) , Lotzmann (1961) , Neelley (1961) , Goldiamond (1965) , Ham and Steer (1967) and Curlee and Perkins (1969) .
A further important claim that was made at this time that was embraced by several eminent workers was that DAF produces similar effects in fluent speakers to those that people who stutter ordinarily experience - in particular drawling and speech errors. This prompted Lee (1951) to refer to DAF as a form of ‘simulated’ stutter. In an extension of this point of view, Cherry and Sayers (1956) used DAF as a way of simulating stuttering in fluent speakers to establish the basis of the problem. They generated two different sources of sound that are heard whilst speaking normally (the sound transmitted over air and that transmitted through bone). They then examined separately which of these ‘feedback’ components led to increased stuttering rates in fluent speakers when each of them was delayed. The bone-conducted component seemed to be particularly effective in increasing ‘simulated’ stuttering; and they proposed that this source of feedback also led to the problem in speakers who stutter. They then designed a therapy that involved playing noise to speakers who stutter that was intended to mask out the problematic bone-conducted component of vocal ‘feedback’. They reported that fluency improved when the voice was masked in this way. Although there is some disagreement about whether speakers who stutter have problems processing bone-conducted sounds ( Howell & Powell, 1984 ), the effects of masking sounds on the fluency of speakers who stutter has not been disputed. In summary, altering speech timing affects the behavior of fluent speakers adversely but can improve the speech of speakers who stutter.
3.2 Frequency
There are relatively few studies on what effects altering the frequency content of speech has on speech control which is unfortunate as those that there are can be extrapolated to environments that affect the frequency content of sound. Early studies examined the effect of filtering speech ( Garber & Moller, 1979 ). Elman (1981) examined the effects of shifting the speech spectrum (frequency shifted feedback, FSF) on voice pitch, and reported that speakers partially compensate for these shifts. That is, speakers shifted their voice pitch in the opposite direction to the shift made by the experimenter. Subsequent studies have shown that FSF also has little effect on voice level ( Howell, 1990 ). The incomplete compensation for shifts in frequency of voice pitch in fluent speakers has also been confirmed ( Burnett et al., 1997 ), as well as being reported for upward shifts in speakers who stutter ( Natke et al., 2001 ) although no compensation occurs for downward shifts in people who stutter ( Natke et al., 2001 ).
Howell et al. (1987) created a frequency-shifted version of the speaker’s voice that was synchronous with the speaker’s voice, and assessed its effects on speakers who stutter. These authors used a speed-changing method (that produces a frequency shift in the same way that playing a tape recorder at different speeds does). The method they developed produces a virtually synchronous frequency shift. Other features to note about FSF are that the signal level in the shifted version varies with speech level (when speakers produce low intensity sounds, the FSF is also low in intensity, and vice versa). Also, no sound occurs when the speaker is silent (the latter is a feature that is shared with the Edinburgh masker). The two preceding factors limit the noise dose the speaker receives.
The effects on fluency of this (almost real-time) alteration was a marked improvement in fluency in people who stutter even when speakers were instructed to speak at normal rate. The first study reported by Howell et al. (1987) showed that FSF resulted in more fluent speech than DAF or a portable device called the Edinburgh masker ( Dewar et al., 1979 ). Later studies have argued that FSF does not produce speech that is superior to DAF speech at short delays ( Kalinowski et al., 1993 ; Macleod et al., 1995 ). However, these studies have used fast Fourier transform (FFT) techniques to produce frequency shifts. FFT techniques produce significant delays that are somewhat variable ( Howell & Sackin, 2002 ). Therefore, the studies that claim FSF has the same effect on fluency as DAF have compared FSF plus a short delay, with short-delay DAF. Thus the delay they include under FSF may account for why these studies failed to find a difference between it and DAF, whereas Howell et al. (1987) did. Kalinowski’s group claims the paucity of secondary effects on aspects of speech control other than fluency makes FSF acoustically ‘invisible’ on speakers who stutter (and they maintain that the same applies to short-duration DAF). They also claim that the minimal changes in speech control under these two forms of altered sound lead speakers to produce fluent, or near fluent, speech ( Kalinowski & Dayalu, 2002 ).
A second important point about the Howell et al. (1987) study was that, as mentioned, the effects on fluency were observed even though speakers were told to speak at a normal rate. Therefore, to the extent to which they obeyed instructions, the effects of FSF seem to be independent of rate. This argues against Costello-Ingham’s (1993) view that altered feedback techniques (DAF in particular) work on people who stutter because they slow overall speech rate. Direct tests of whether fluency-enhancing effects occur when speech rate is varied were made by Kalinowski et al. (1996) for DAF, and by Hargrave et al. (1994) , and Natke et al. (2001) for FSF. These studies reported that fluency was enhanced whether or not rate was slow (relative to normal speaking conditions). One proviso about the Kalinowski studies is that a global measure of speech rate was taken. It is possible for speakers to speed up global (mean) speech rate while, at the same time, reducing rate locally within an utterance. See Howell and Sackin (2000) for an empirical study that shows fluent speakers display local slowing in singing and local and global slowing under FSF. Until local measures are taken under FSF in people who stutter, it cannot be firmly concluded whether fluency changes are associated with rate change or not, since the speakers might have increased global rate but reduced local rate around the points where disfluencies would have occurred ( Howell & Sackin, 2000 ).
In Howell et al.’s (1987) fourth experiment, the effects of presenting FSF at sound onset only (where speakers who stutter have most problems) were compared with those in continuous FSF speech. The effects on fluency did not differ significantly between the two conditions, suggesting that having FSF at sound onset only was as effective as having it on throughout the utterance. This shows that it may be possible to get as much enhancement in fluency when alteration is made to selected areas in an utterance as opposed for when alteration is made to the whole utterance.
Another factor of interest is that Kalinowski’s group has investigated how FSF operates in more natural environments such as over the telephone ( Zimmerman, et al., 1997 ), or when speakers have to speak in front of audiences ( Armson et al., 1997 ). They reported that, in both these environments, there are marked improvements in fluency and, therefore, that these procedures may operate in natural environments.
3.3 Intensity
Speaking is affected when the voice is amplified ( Fletcher et al., 1918 ) or when noise is present ( Lombard, 1911 ). Laboratory studies have shown that when voice level is amplified, speakers reduce voice level and when voice level is reduced, they increase it (called the Fletcher effect). Conversely, when noise level increases, speakers increase their voice level and when noise level reduces, speakers reduce their voice level (called the Lombard effect). It is possible that these compensations could be the result of a negative feedback mechanism for regulating voice level. If speakers need to hear their voice to control it but cannot do so, either because noise level is high or voice level is low, they compensate by increasing level. Speakers would compensate in the opposite way if their speech is too loud (low noise level or when the voice is amplified). Note, however, that explanations other than a feedback account, are also possible. For instance, Lane and Tranel (1971) discuss the view that voice level changes are made so that the audience, rather than the speaker himself or herself, does not receive speech at too high or too low a level.
Speakers who stutter change their voice level in the same direction as fluent speakers when noise is present and when their voice is amplified or attenuated ( Howell, 1990 ). The effects of non-speech noises on the fluency of speakers who stutter has been examined. In one imaginative study, Sutton and Chase (1961) arranged whether noise was on or off using a voice-activated relay while subjects read aloud. They compared the fluency-enhancing effects of noise that was on continuously, noise that was presented only while the speaker was speaking and noise presented only during the silent periods between speech. They found that all these conditions were equally effective. It appears from this that the operative effect is not simply masking as there is no sound to mask when noise is presented during silent periods. However, Webster and Lubker (1968) pointed out that voice-activated relays take time to operate and so some noise would have been present at the onset of words. Therefore a masking effect cannot be ruled out. Portable masking devices such as the Edinburgh masker ( Dewar et al., 1979 ) have been developed for treating stuttering.
3.4 Cognitive influences of speaking environment
Non-auditory influences can affect a speaker’s behavior too. The view that speakers can adapt their speech when they know something about the audience has been discussed in connection with Lane and Tranel’s interpretation of the Lombard effect. Another practically important example of non-auditory effects is the clear speech phenomenon. When producing clear speech, speakers can make a conscious effort about how to control their voice, overriding environmental influences. It has been shown that if speakers speak clearly, there are substantial intelligibility gains relative to conversational speech for hearing impaired individuals ( Picheny et al., 1989 ). Clear speech differs from conversational speech in a variety of ways, including speaking rate, consonant power and the occurrence of phonological phenomena ( Picheny et al., 1989 ). Speakers frequently make attempts to speak clearly in auditoria, although the influences of this have not been studied much outside the hearing impaired field. An exception is the work of Lindblom (1990) who has embodied the idea in his H and H theory that speakers place themselves on a continuum between clear and casual speech based on the perceived importance of getting the message across.
4. How environments affect speech perception
Topics were arranged in parallel ways in the two previous sections. Perceptual studies have been conducted under perceptual themes rather than parameters that have been manipulated to simulate the effects which occur in real environments. It should be borne in mind that in the case where the speaker, as well as listener, is in the same space, the sound the perceptual system is dealing with will also have been changed by the environment. In this section, a selection of the perceptual factors that affect listeners is outlined.
4.1 Masking
When there are noises (speech or non-speech) in the environment these sounds act as maskers. The effects of masking on listeners’ performance have been studied extensively and would take many volumes to describe fully. Here the important effects of masking on listeners’ performance are merely noted.
4.2 Clear speech
The studies on clear speech described in the preceding section were undertaken with the intention of establishing what benefit these would have to listeners (in the MIT group’s work, specifically what effect they would have on hearing impaired listeners). Intelligibility tests suggest around 10% more test words can be identified when speech is spoken clearly.
4.3 Location of an object in space
Speakers can localize the origin of a sound in a room. Researchers have examined cues to locales in controlled environments (not room specific) although some important influences that operate in room environments have been studied. A brief description of binaural and monaural cues is given and then some effects that operate in rooms are described.
Sound localization is a listener’s ability to identify the location or origin of a detected sound usually in a three-dimensional space (although localization has also been studied in virtual environments). Binaural cues (using both ears) are important in localization ability. The time of arrival at the two ears is different for a sound which is not directly in front of the listener because the length of the path to the near ear is less than that to the far ear. This time delay is the primary binaural cue to sound localization and is called the interaural time difference (ITD).
A secondary binaural cue is the reduction in loudness when the sound reaches the far ear. This is called the interaural intensity difference (IID). IID is frequency dependent as low frequency sounds can bend round the head. Thus IID cues operate at these frequencies whilst high frequencies are blocked by the head and never reach the far ear (IID does not operate for these frequencies). Note that these cues will only aid in localizing the sound source’s azimuth (the angle between the source and the sagittal plane), not its elevation (the angle between the source and the horizontal plane through both ears).
Monaural localization depends primarily on the filtering effects of external structures like the head, shoulders, torso, and outer ear or pinna. The sound frequencies are filtered depending on the angle from which they strike the various external structures. The main such effect arises from the pinna notch, which arises when the pinna attenuates frequencies in a narrow frequency band. The band of frequencies in the notch depends on the angle from which the sound strikes the outer ear and provides information about the direction of the source.
It has already been mentioned that sound intensity decays with increasing distance form the source. Thus intensity provides a cue to distance but generally speaking, this is not a reliable cue, because it is not known how loud the sound source is. However in the case of a familiar sound such as speech, there is an implicit knowledge of how loud the sound source should be, which enables a rough distance judgment to be made.
Echoes provide reasonable cues to the distance of a sound source, in particular because the strength of echoes does not depend on the distance of the source, while the strength of the sound that arrives directly from the sound source becomes weaker with distance. As a result, the ratio of direct-to-echo strength alters the quality of the sound. In this way consistent, although not very accurate, distance judgments are possible.
The final topic discussed is the precedence effect ( Wallach et al., 1949 ). This states that only the first of multiple identical sounds is used to determine the sound’s location. Echoes, which otherwise could cause confusion about locale, are effectively ignored by the listener’s perceptual system.
4.4 Auditory stream segregation
A large amount of work has been done on auditory stream segregation since the publication of Bregman’s (1990) book. Obviously, one cannot hope to do justice to this in a short paper like this. The main difference between this approach and classic psychoacoustics is in the emphasis placed on top-down cognitive influences. Listeners use a lot of stored information on what they know about the structure of sounds to interpret incoming sounds.
One example is the harmonic sieve model which collects together those frequency components that belong to a particular sound source ( Duifhuis et al., 1982 ). Voiced speech has a harmonic structure. The basic idea behind a harmonic sieve is that if the auditory system performs a spectral analysis and only those frequencies near to harmonics are taken, only the components from a single speaker would be obtained and other sounds would be sieved out. In this case, listeners make use of information about harmonic structure to segregate sounds.
The auditory stream segregation approach makes extensive use of Gestalt notions. Two related examples from Darwin (1984) and Nakajima et al. (2000) that involve the Gestalt notion of capture are discussed briefly. Darwin’s studies showed that a tone that starts or stops at a different time from a steady state vowel was less likely to be heard as part of that vowel than if it was simultaneous with it. In Nakajima et al.’s study, the stimuli consisted of two glides that crossed each other at a point in time (one of which started before and finished after the shorter glide). The shorter glide was continuous, but the longer one was interrupted at the crossover point. However listeners perceived this in the opposite way (the longer one was perceived as continuous and the shorter one as interrupted). This powerful illusion again points to the importance of the Gestalt grouping notions.
4.5 Cognitive influences on listeners
As with speech production, there are also cognitive influences in the environment that affect listeners. The ideas stemming form Bregman’s work include cognitive influences. The linguistic context is one factor that affects speech dysfluencies produced by fluent speakers ( Shriberg, 2001 ). Judgments about sounds are also affected by what the listener sees. One example of this is the well-known McGurk effect ( McGurk & MacDonald, 1976 ). This is an illusion in which a listener sees a video of a speaker saying the syllable /ga/ whilst hearing the syllable /ba/. The initial plosive in these sounds has different places of articulation (velar and bilabial respectively). Listeners do not report either of these sounds, but report hearing /da/ which has a place of articulation intermediate between /ga/ and /ba/. Another example is where a visual object that moves in synchrony with the sound source (e.g. a ventriloquist’s dummy) biases a sound’s judged location. The ventriloquism effect has been studied by asking for judgments about sound source location when dummy loudspeakers are visible ( Radeau & Bertelson, 1976 ).
5. Conclusion including considerations about room design
This short review does not claim to be comprehensive but, hopefully, raises some considerations about how speakers will be affected by environments with different acoustic properties, and some of the perceptual mechanisms that listeners have available to offset the deleterious effects of some of these influences. Delay and intensity are disruptive on fluent speakers, but frequency alterations less so. Speakers who stutter show similar responses to fluent speakers in these environments although the manipulations alleviate their fluency problem (FSF, DAF and masking of the voice). This shows that the way in which environments affect sound is not necessarily bad for all types of speaker.
Studies are needed which examine together the changes a speaker makes in an environment and how a listener in that same environment processes the altered speech. Speaking clearly can potentially offset poor acoustic characteristics in environments, although this needs to be checked. The precedence effect suggests that the disruptive effects of echoes can be reduced by listeners’ perceptual mechanisms. Listeners may use harmonic sieve’s to help them track a single voice in noisy environments. Besides these mechanisms that offset problems, there are cases where speakers can be misled (McGurk and ventriloquism effects).
Acknowledgements
This work was supported by grant 072639 from the Wellcome Trust to Peter Howell.
- Armson J, Foote S, Witt C, Kalinowski J, Stuart A. Effect of frequency altered feedback and audience size on stuttering. Europ. J. Disord. Comm. 1997; 32 :359–366. [ PubMed ] [ Google Scholar ]
- Bregman A. Auditory stream segregation. Cambride MA: MIT press; 1990. [ Google Scholar ]
- Burnett TA, Senner JE, Larson CR. Voice F0 responses to pitch-shifted auditory feedback: A preliminary study. J. Voice. 1997; 11 :202–211. [ PubMed ] [ Google Scholar ]
- CCITT (1989a) Interactions between sidetone and echo. CCITT - International Telegraph and Telephone Consultative Committee, Contribution, com XII, no BB.
- CCITT (1989b) Experiments on short-term delay and echo in conversation. CCITT - International Telegraph and Telephone Consultative Committee, Contribution, com XII, no AA.
- Chase RA, Sutton S, Rapin I. Sensory feedback influences on motor performance. J. Aud. Res. 1961; 1 :212–223. [ Google Scholar ]
- Cherry C, Sayers B. Experiments upon the total inhibition of stammering by external control and some clinical results. J. Psychosomat. Res. 1956; 1 :233–246. [ PubMed ] [ Google Scholar ]
- Costello-Ingham JC. Current status of stuttering and behavior modification - 1. Recent trends in the application of behavior application in children and adults. J. Fluency Disord. 1993; 18 :27–44. [ Google Scholar ]
- Curlee RF, Perkins WH. Conversational rate control for stuttering. J. Speech Hearing Disord. 1969; 34 :245–250. [ PubMed ] [ Google Scholar ]
- Darwin CJ. Perceiving vowels in the presence of another sound: Constraints on formant perception. J. Acoust. Soc. Amer. 1984; 70 :1636–1651. [ PubMed ] [ Google Scholar ]
- Dewar A, Dewar AW, Austin WTS, Brash HM. The long-term use of an automatically triggered auditory feedback masking device in the treatment of stammering. Brit. J. Disord. Comm. 1979; 14 :219–229. [ Google Scholar ]
- Duifhuis H, Willems LF, Sluyter RJ. Measurement of pitch in speech: An implementation of Goldstein’s theory of pitch perception. J. Acoust. Soc. Amer. 1982; 71 :1568–1580. [ PubMed ] [ Google Scholar ]
- Elman JL. Effects of frequency-shifted on the pitch of vocal productions. J. Acoust. Soc. Amer. 1981; 70 :45–50. [ PubMed ] [ Google Scholar ]
- Fairbanks G. Selected vocal effects of delayed auditory feedback. J. Speech Hear. Disord. 1955; 20 :333–345. [ PubMed ] [ Google Scholar ]
- Fletcher H, Raff GM, Parmley F. Study of the effects of different sidetones in the telephone set. Western Electrical Company; 1918. Report no. 19412, Case no. 120622. [ Google Scholar ]
- Garber S, Moller K. The effects of feedback filtering on nasalization in normal and hypernasal speakers. J. Speech Hear. Res. 1979; 22 :321–333. [ PubMed ] [ Google Scholar ]
- Goldiamond I. Stuttering and fluency as manipulatable operant response classes. In: Krasner L, Ullman L, editors. Research in behavior modification. New York: Holt, Rhinehart and Winston; 1965. pp. 106–156. [ Google Scholar ]
- Ham R, Steer MD. Certain effects of alterations in auditory feedback. Folia Phoniat. 1967; 19 :53–62. [ PubMed ] [ Google Scholar ]
- Hargrave S, Kalinowski J, Stuart A, Armson J, Jones K. Effect of frequency-altered feedback on stuttering frequency at normal and fast speech rates. J. Speech Hear. Res. 1994; 37 :1313–1319. [ PubMed ] [ Google Scholar ]
- Howell P. Changes in voice level caused by several forms of altered feedback in normal speakers and stutterers. Lang. Speech. 1990; 33 :325–338. [ PubMed ] [ Google Scholar ]
- Howell P, Archer A. Susceptibility to the effects of delayed auditory feedback. Percep. Psychophys. 1984; 36 :296–302. [ PubMed ] [ Google Scholar ]
- Howell P, El-Yaniv N, Powell DJ. Factors affecting fluency in stutterers when speaking under altered auditory feedback. In: Peters H, Hulstijn W, editors. Speech Motor Dynamics in Stuttering. New York: Springer Press; 1987. pp. 361–369. [ Google Scholar ]
- Howell P, Powell DJ. Hearing your voice through bone and air: Implications for explanations of stuttering behaviour from studies of normal speakers. J. Fluency Disord. 1984; 9 :247–264. [ Google Scholar ]
- Howell P, Sackin S. Speech rate manipulation and its effects on fluency reversal in children who stutter. J. Developmental Phys. Disab. 2000; 12 :291–315. [ PMC free article ] [ PubMed ] [ Google Scholar ]
- Howell P, Sackin S. Timing interference to speech in altered listening conditions. J. Acous. Soc. Amer. 2002; 111 :2842–2852. [ PMC free article ] [ PubMed ] [ Google Scholar ]
- Kalinowski J, Armson J, Roland-Mieszkowski M, Stuart A, Gracco V. Effects of alterations in auditory feedback and speech rate on stuttering frequency. Lang. Speech. 1993; 36 :1–16. [ PubMed ] [ Google Scholar ]
- Kalinowski J, Dayalu V. A common element in the immediate inducement of effortless, natural-sounding, fluent speech in stutterers: “The Second Speech Signal” Med. Hypoth. 2002; 58 :61–66. [ PubMed ] [ Google Scholar ]
- Kalinowski J, Stuart A, Sark S, Armson J. Stuttering amelioration at various auditory feedback delays and speech rates. Eu. J. Disord. Comm. 1996; 31 :259–269. [ PubMed ] [ Google Scholar ]
- Lane HL, Tranel B. The Lombard sign and the role of hearing in speech. J. Speech Hear. Res. 1971; 14 :677–709. [ Google Scholar ]
- Lee BS. Artificial stutter. J. Speech Hearing Disord. 1951; 15 :53–55. [ PubMed ] [ Google Scholar ]
- Lindblom BB. Explaining phonetic variation: A sketch of the H and H theory. In: Hardcastle WJ, Marchal A, editors. Speech production and speech modeling. Dordrecht: Kluwer; 1990. pp. 403–439. [ Google Scholar ]
- Lombard E. Le signe de l’elevation de la voix. Annales des Maladies de l’Oreille, du Larynx, du Nez et du Pharynx. 1911; 37 :101–119. [ Google Scholar ]
- Lotzmann G. Zur Anwedung variierter verzogerungszeiten bei balbuties. Folia Phoniat. Logoped. 1961; 13 :276–312. [ Google Scholar ]
- Macleod J, Kalinowski J, Stuart A, Armson J. Effect of single and combined altered auditory feedback on stuttering frequency at two speech rates. J. Comm. Disord. 1995; 28 :217–228. [ PubMed ] [ Google Scholar ]
- McGurk H, MacDonald J. Hearing lips and seeing voices. Nature. 1976; 264 :746–748. [ PubMed ] [ Google Scholar ]
- Nakajima Y, Sasaki T, Kanafuka K, Miyamoto A, Remijn G, ten Hoopen G. Illusory recouplings of onsets and terminations of glide tone components. Percept. Psychophys. 2000; 62 :1413–1425. [ PubMed ] [ Google Scholar ]
- Natke U, Grosser J, Kalveram KT. Fluency, fundamental frequency, and speech rate under frequency shifted auditory feedback in stuttering and nonstuttering persons. J. Fluency Disord. 2001; 26 :227–241. [ Google Scholar ]
- Neelley JN. A study of the speech behaviors of stutterers and nonstutterers under normal and delayed auditory feedback. J. Speech Hearing Disord. Monog. 1961; 7 :63–82. [ PubMed ] [ Google Scholar ]
- Nessel E. Die verzogerte Sprachruckkopplung (Lee Effect) bei Stotteren. Folia Phoniat. 1958; 10 :199–204. [ PubMed ] [ Google Scholar ]
- Picheny MA, Durlach NI, Braida LD. Speaking clearly for the hard of hearing II: Acoustic characteristics of clear and conversational speech. J. Speech Hearing Res. 1989; 29 :434–446. [ PubMed ] [ Google Scholar ]
- Radeau M, Bertelson P. The effects of a textured visual field on modality dominance in a ventriloquism situation. Percept Psychophy. 1976; 20 :227–235. [ Google Scholar ]
- Shriberg E. To ‘errrr’ is human: Ecology and acoustics of speech disfluencies. J. Int. Phonetic Assoc. 2001; 31 :153–169. [ Google Scholar ]
- Soderberg GA. A study of the effects of delayed side-tone on four aspects of stutterers’ speech during oral reading and spontaneous speech. Speech Monog. 1960; 27 :252–253. [ Google Scholar ]
- Sutton S, Chase RA. White noise and stuttering. J. Speech Hear. Res. 1961; 4 :72. [ Google Scholar ]
- Wallach H, Newman EB, Rosenzweig MR. The precedence effect in binaural unmasking. Amer. J. Psych. 1949; 62 :315–336. [ PubMed ] [ Google Scholar ]
- Webster RL, Lubker B. Masking of auditory feedback in stutterers’ speech. J. Speech Hear. Res. 1968; 11 :221–222. [ PubMed ] [ Google Scholar ]
- Zimmerman S, Kalinowski J, Stuart A, Rastatter MP. Effect of altered auditory feedback on people who stutter during scripted telephone conversations. J. Speech, Lang. Hear. Res. 1997; 40 :1130–1134. [ PubMed ] [ Google Scholar ]
Gaze and speech behavior in parent–child interactions: The role of conflict and cooperation
- Open access
- Published: 02 December 2021
- Volume 42 , pages 12129–12150, ( 2023 )
Cite this article
You have full access to this open access article
- Gijs A. Holleman ORCID: orcid.org/0000-0002-6443-6212 1 ,
- Ignace T. C. Hooge 1 ,
- Jorg Huijding 2 ,
- Maja Deković 2 ,
- Chantal Kemner 1 &
- Roy S. Hessels 1
2494 Accesses
3 Citations
Explore all metrics
A primary mode of human social behavior is face-to-face interaction. In this study, we investigated the characteristics of gaze and its relation to speech behavior during video-mediated face-to-face interactions between parents and their preadolescent children. 81 parent–child dyads engaged in conversations about cooperative and conflictive family topics. We used a dual-eye tracking setup that is capable of concurrently recording eye movements, frontal video, and audio from two conversational partners. Our results show that children spoke more in the cooperation-scenario whereas parents spoke more in the conflict-scenario. Parents gazed slightly more at the eyes of their children in the conflict-scenario compared to the cooperation-scenario. Both parents and children looked more at the other's mouth region while listening compared to while speaking. Results are discussed in terms of the role that parents and children take during cooperative and conflictive interactions and how gaze behavior may support and coordinate such interactions.
Similar content being viewed by others
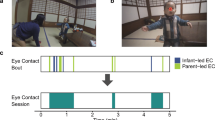
Eye tracking in an everyday environment reveals the interpersonal distance that affords infant-parent gaze communication
Hiroki Yamamoto, Atsushi Sato & Shoji Itakura
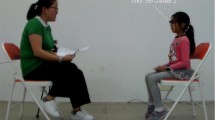
Characteristics of Visual Fixation in Chinese Children with Autism During Face-to-Face Conversations
Zhong Zhao, Haiming Tang, … Jianping Lu
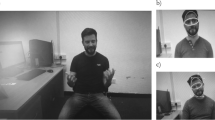
Eye gaze During Semi-naturalistic Face-to-Face Interactions in Autism
Alasdair Iain Ross, Jason Chan & Christian Ryan
Avoid common mistakes on your manuscript.
Introduction
A primary mode of human social behavior is face-to-face interaction. This is the “central ecological niche” where languages are learned and most language use occurs (Holler & Levinson, 2019 , p. 639). Face-to-face interactions are characterized by a variety of verbal and nonverbal behaviors, such as speech, gazing, facial displays, and gestures. Since the 1960s, researchers have extensively investigated the coordination and regulation of these behaviors (Duncan & Fiske, 2015 ; Kelly et al., 2010 ; Kendon, 1967 ). A paramount discovery is that gaze and speech behavior are closely coupled during face-to-face interactions. Although some patterns of speech behavior during face-to-face interactions, such as in turn-taking, are common across different languages and cultures (Stivers et al., 2009 ), the role of gaze behavior in interaction seems to be culturally- as well as contextually-dependent (Foddy, 1978 ; Haensel et al., 2017 , 2020 ; Hessels, 2020 ; Kleinke, 1986 ; Patterson, 1982 ; Rossano et al., 2009 ; Schofield et al., 2008 ). Observational studies on gaze behavior during interaction have been conducted in many different interpersonal contexts, such as interactions between adults, parents and infants, parents and children, as well as clinical interviews and conversations with typically and atypically developing children (Argyle & Cook, 1976 ; Arnold et al., 2000 ; Ashear & Snortum, 1971 ; Berger & Cunningham, 1981 ; Cipolli et al., 1989 ; Kendon, 1967 ; Levine & Sutton-Smith, 1973 ; Mirenda et al., 1983 ). More recently, new eye-tracking techniques have been developed to measure gaze behavior of individuals during face-to-face interactions with higher spatial and temporal resolution (Hessels et al., 2019 ; Ho et al., 2015 ; Rogers et al., 2018 ). However, these techniques have not been used to study the relation between speech and gaze in parent–child conversations.
Parent–child interactions provide a rich social context to investigate various aspects of social interaction, such as patterns of verbal and nonverbal behavior during face-to-face communication. Parent–child interactions are crucial for children’s social, emotional, and cognitive development (Branje, 2018 ; Carpendale & Lewis, 2004 ; Gauvain, 2001 ). Also, the ways in which parents and children interact changes significantly from childhood to adolescence. In infancy and childhood, parent–child interactions play an important role in children’s socialization, which involves the acquisition of language and social skills, as well as the internalization of social norms and values (Dunn & Slomkowski, 1992 ; Gauvain, 2001 ). In adolescence, parent–child interactions are often centered around relational changes in the hierarchical nature of the parent–child relationship, which typically consist of frequent conflicts about parental authority, child autonomy, responsibilities, and appropriate behavior (Laursen & Collins, 2009 ; Smetana, 2011 ). According to Branje ( 2018 , p. 171), “parent-adolescent conflicts are adaptive for relational development when parents and adolescents can switch flexibly between a range of positive and negative emotion s.” As children move through adolescence, they become more independent from their parents and start to challenge parents’ authority and decisions. In turn, parents need to react to these changes and renegotiate their role as a parent. How parents adapt to these changes (e.g. permissive, supporting, or authoritarian parenting styles) may have a significant impact on the social and emotional well-being of the child (Smokowski et al., 2015 ; Tucker et al., 2003 ). Children in this period become progressively aware of the perspectives and opinions of other people in their social environment (e.g. peers, classmates, teachers) and relationships with peers become more important to one’s social identity. In turn, parents’ authority and control over the decisions and actions of the child changes as the child moves from childhood to adolescence (Steinberg, 2001 ).
In this study, we investigated gaze behavior and its relation to speech in the context of parent–child interactions. We focus on the role of conflict and cooperation between parents and their preadolescent children (age range: 8 – 11 years) and how these interpersonal dynamics may be reflected in patterns of gaze and speech behavior. We chose this period because it marks the beginning of the transition from middle childhood to early adolescence. In this period, parents still hold sway over their children’s decisions and actions, however, the relational changes between children and parents start to become increasingly more prominent (e.g. striving for autonomy, disengagement from parental control), which is highly relevant to the study of conflict and cooperation in parent–child relationships (Branje, 2018 ; De Goede et al., 2009 ; Dunn & Slomkowski, 1992 ; Steinberg, 2001 ). Specifically, we are interested in patterns of gaze and speech behavior as a function of cooperative and conflicting conversation topics, to which parent–child interactions are ideally suited. We focus primarily on gaze behavior because of its importance for perception – does one need to look at another person’s face in order to perceive certain aspects of it? – and its relation to speech in face-to-face interactions (Hessels, 2020 ; Holler & Levinson, 2019 ; Kendon, 1967 ). Furthermore, the role of gaze in face-to-face interactions has previously been linked with various interpersonal dynamics, such as intimacy and affiliation, but also with social control, dominance, and authority (for a review, see Kleinke, 1986 ), which is relevant to the social context of the parent–child relationship. Although no eye-tracking studies to our knowledge have investigated the role of gaze behavior and its relation to speech in parent–child conversations, several studies have addressed the role of gaze behavior in face and speech perception and functions of gaze during conversational exchanges. Because these lines of research are directly relevant to our current study, we will briefly review important findings from this literature.
Where Do People Look at Each Other’s Faces?
Faces carry information that is crucial for social interaction. By looking at other people’s faces one may explore and detect certain aspects of those faces such as facial identity, emotional expression, gaze direction, and cognitive state (Hessels, 2020 ; Jack & Schyns, 2017 ). A well-established finding, ever since the classic eye-tracking studies by Buswell ( 1935 ) and Yarbus ( 1967 ), is that humans have a bias for looking at human faces and especially the eyes (see e.g. Birmingham et al., 2009 ; Hessels, 2020 ; Itier et al., 2007 ). This bias already seems to be present in early infancy (Farroni et al., 2002 ; Frank et al., 2009 ; Gliga et al., 2009 ). In a recent review, Hessels ( 2020 ) describes that where humans look at faces differs between, for example, when the face is moving, talking, expressing emotion, or when particular tasks or viewing conditions are imposed by researchers (e.g. face or emotion recognition, speech perception, restricted viewing). Moreover, recent eye-tracking research has shown that individuals exhibit large but stable differences in gaze behavior to faces (Arizpe et al., 2017 ; Kanan et al., 2015 ; Mehoudar et al., 2014 ; Peterson & Eckstein, 2013 ; Peterson et al., 2016 ). That is, some people tend to fixate mainly on the eye or brow region while others tend to fixate the nose or mouth area. Thus, what region of the face is looked at by an observer will likely depend on the conditions of the experimental context and on particular characteristics of the individual observer.
Previous eye-tracking research on gaze behavior to faces has mostly been conducted using static images or videos of faces presented to participants on a computer screen. However, some researchers have questioned whether gaze behavior under such conditions adequately reflects how people look at others in social situations, e.g. when there is a potential for interaction (Laidlaw et al., 2011 ; Risko et al., 2012 , 2016 ). For example, Laidlaw et al. ( 2011 ) showed that when participants were seated in a waiting room, they looked less at a confederate who was physically present compared to when that person was displayed on a video monitor. While this discovery has led researchers to question the presumed ‘automaticity’ of humans to look at faces and eyes, this situation may primarily pertain to potential interactions . That is, situations where social interaction is possible but can be avoided, for example, in some public spaces and on the street (see also Foulsham et al., 2011 ; Hessels, et al., 2020a , 2020b ; Rubo et al., 2020 ). Other studies have shown that, once engaged in actual interaction , such as in conversation, people tend to look at other people’s faces and its features (Freeth et al., 2013 ; Hessels et al., 2019 ; Rogers et al., 2018 ). One may thus expect that parents and children will also primarily look at each other’s faces during conversational interactions, but where on the face they will mostly look likely differs between individuals and may be closely related to what the face is doing (e.g. speaking, moving, expressing emotion) and to the social context of the interaction.
Where Do People Look at Each Other’s Faces During Conversations?
In (early) observational work (e.g., Argyle & Cook, 1976 ; Beattie & Bogle, 1982 ; Foddy, 1978 ; Kendon, 1967 ), researchers have often studied gaze behavior in face-to-face conversations by manually coding interactants’ gaze behavior from video recordings. These observational studies (i.e. not using an eye tracker) of two-person conversations have shown that speakers tend to equally gaze at or away from listeners, whereas listeners gaze longer at speakers with only occasional glances away from the speaker in between (Duncan & Fiske, 2015 ; Kendon, 1967 ; Rossano et al., 2009 ). Yet, the observational techniques used in these studies have limited reliability and validity to distinguish gaze direction at different regions of the face (Beattie & Bogle, 1982 ), which is of crucial importance for research on face-scanning behavior (see also Hessels, 2020 , p. 869). Conversely, eye-tracking studies on gaze behavior to faces have used videos of talking faces, instead of actual interactions (e.g. Foulsham & Sanderson, 2013 ; Vatikiotis-Bateson et al., 1998 ; Võ et al., 2012 ). Most eye-tracking studies have therefore only been concerned with where people look at faces while listening to another person speak. Võ et al. ( 2012 ), for example, presented observers with close-up video clips of people being interviewed. They found that overall, participants gazed at the eyes, nose, and mouth equally often. However, more fixations to the mouth and fewer to the eyes occurred when the face was talking than when the face was not talking. This finding converges with the well-established finding that visual information from the human face may influence, or enhance how speech is perceived (Sumby & Pollack, 1954 ). A well-known example is the McGurk effect (McGurk & MacDonald, 1976 ), where one’s perception of auditory speech syllables can be modulated by the mouth and lip movements from a talking face.
Only recently have researchers begun to use eye-tracking technology to measure where people look at each other’s faces when engaged in interactive conversational exchanges (Hessels et al., 2019 ; Ho et al., 2015 ; Rogers et al., 2018 ). Rogers et al. ( 2018 ), for example, used wearable eye trackers to measure where two people looked at each other while engaged in short “getting acquainted” conversations. They found that participants gazed away from the face of one’s partner for about 10% of the total conversation duration when listening, and about 29% when speaking (cf. Kendon, 1967 ). When participants gazed at their partner’s face, they looked primarily at the eyes and mouth region. Specifically, Rogers et al. ( 2018 ) reported that, on average, participants looked slightly more at the mouth area while listening compared to when they were speaking; a difference of approximately 5 percentage points of the time that they were looking at the other person’s face. In a different eye-tracking study, Hessels et al. ( 2019 ) investigated gaze behavior of participants engaged in a face-to-face interaction with a confederate. They observed that when participants listened to a confederate’s story, their gaze was directed at the facial features (e.g. eyes, nose, and mouth regions) for a longer total duration, as well as more often per second, compared to when speaking themselves. However, they did not find that participants looked proportionally longer at the mouth while listening compared to speaking, as in Rogers et al. ( 2018 ). One reason for this difference could be that participants in the Hessels et al. ( 2019 ) study did not need to exchange speaking turns as they were specifically tasked to wait for the confederate to end his story. The small differences in these two studies may then be explained if turn-transitions are associated with looking at the mouth.
In sum, it has been well established that gaze to faces during conversations is dependent on speaker-state: who is speaking or who is being addressed. Based on eye-tracking studies with videos of talking faces, it has often been suggested that gaze will be directed more at the mouth while listening to someone speak, as looking at the mouth area may be beneficial (but not necessary) for speech perception (see e.g. Buchan et al., 2007 ; Vatikiotis-Bateson et al., 1998 ; Võ et al., 2012 ). Recent dual eye-tracking studies on the role of gaze behavior in two-person conversations (Hessels et al., 2019 ; Rogers et al., 2018 ) have found no, or only small, differences in gaze to specific facial features (e.g. eyes, mouth) during episodes of speaking and listening. We expect to observe a similar pattern for parents and children as well.
Present study
In this study, we investigated speech and gaze behavior during conversational interactions between a parent and their child. Parent–child dyads engaged in two conversations about potential disagreements (conflict) and agreements (cooperation) on common family topics, given their importance and frequent occurrence within the social context of the parent–child relationship (Branje, 2018 ; Dixon et al., 2008 ; Laursen & Collins, 2004 ; Steinberg, 2001 ). We investigated (1) the similarities and differences between parents and children’s speech and gaze behavior during face-to-face interaction, (2) whether patterns of speech and gaze behavior in parent–child conversations are related to the nature of the conversation (conflictive versus cooperative topics), and (3) whether gaze behavior to faces is related to whether someone is speaking or listening. To engage parents and children in conflictive and cooperative conversations, we used two age-appropriate semi-structured conversation-scenarios. This method, which is considered a ‘gold standard’ in the field, has extensively been used by researchers to assess various aspects of the parent–child relationship, e.g. attachment, interpersonal affect, relational quality, parental style, and child compliance (Aspland & Gardner, 2003 ; Ehrlich et al., 2016 ; Scott et al., 2011 ). To investigate the relation between speech and gaze in parent–child conversations, we needed a setup capable of concurrently recording eye movements and audio from two conversational partners with enough spatial accuracy to distinguish gaze to regions of the face. To this end, we used a video-based dual eye-tracking setup by Hessels et al. ( 2017 ) that fulfills these criteria. Based on previous literature, we expected that parents and children on average looked predominantly at each other's faces, but that participants would exhibit substantial individual differences in what region of the face they looked at most (eyes, nose, mouth). Moreover, we expected that gaze behavior was related to whether subjects were speaking or listening. We may expect that when listening gaze is directed more at the mouth region, given its potential benefits for speech perception and turn-taking. Regarding the conflict and cooperative scenarios, we had no prior expectations.
Participants
81 parent–child dyads (total n = 162) participated in this study. All participants were also part of the YOUth study, a prospective cohort study about social and cognitive development with two entry points: Baby & Child and Child & Adolescent (Onland-Moret et al., 2020 ) . The YOUth study recruits participants who live in Utrecht and its neighboring communities. The YOUth study commenced in 2015 and is still ongoing. To be eligible for participation in our study, children needed to be aged between 8 and 11 years at the moment of the first visit (which was the same as the general inclusion criteria of the Child & Adolescent cohort). Participants also had to have a good understanding of the Dutch language. Parents needed to sign the informed consent form for the general cohort study, and for this additional eye-tracking study. Participants of the YOUth study received an additional information letter and informed consent form for this study prior to the first visit to the lab. Participants were not eligible to participate if the child was mentally or physically unable to perform the tasks, if parents didn’t sign the informed consent forms, or if a sibling was already participating in the same cohort. A complete overview of the in-and-exclusion criteria for the YOUth study are described in Onland-Moret et al. ( 2020 ).
For this study, a subset of participants from the first wave of the Child & Adolescent cohort were recruited. Children’s mean age was 9.34 (age range: 8–10 years) and 55 children were female (67%). Parents’ mean age was 42.11 (age range: 33–56) and 64 were female (79%). A complete overview with descriptive statistics of the participants’ age and gender is given in the results section (Table 1 ). We also acquired additional information about the families’ households, which is based on demographic data from seventy-six families. For five families, household demographics were not (yet) available (e.g., parents did not complete the demographics survey of the YOUth study). The average family/household size in our sample was 4.27 residents (sd = 0.71). Seventy children from our sample lived with two parents or caregivers (92.1%). Seven children had no siblings (9.2%), forty-two children had one sibling (55.3%), twenty-three children had two siblings (30.2%), and four children had three siblings (5.3%). Two parents/caregivers lived together with the children of their partner and one family/household lived together with an au pair.
We also checked how our sample compared to the rest of the YOUth study’s sample in terms of parents’ educational level, used here as a simplified proxy of social-economic status (SES). In our subset of participants, we found that most parents achieved at least middle-to-higher educational levels, which is representative of the general YOUth study population. For a detailed discussion of SES in the YOUth study population, see Fakkel et al. ( 2020 ). All participants received an information brochure at home in which this study was explained. Participants could then decide whether they wanted to participate in this additional study aside from the general testing program. All participants were included at their first visit to the lab and parents provided written informed consent for themselves as well as on behalf of their children. This study was approved by the Medical Research Ethics Committee of the University Medical Center Utrecht and is registered under protocol number 19–051/M.
A dual eye-tracking setup (see Fig. 1 a) was used to record gaze of two interactors simultaneously. Each person was displayed to the other by means of a monitor and a half-silvered mirror (see Fig. 1 b). The cameras behind the half-silvered mirrors were Logitech webcams (recording at 30 Hz at a resolution of 800 by 600 pixels). The live video-feeds were presented at a resolution of 1024 by 768 pixels in the center of a 1680 by 1050 pixels computer screen and concurrently recorded to disk. Two SMI RED eye trackers running at 120 Hz recorded participants’ eye movements (see Fig. 1 b). A stimulus computer running Ubuntu 12.04 LTS handled the live video-connection and signaled to the eye-tracker computers to start and stop recording eye movements (for a more detailed explanation of this setup, see Hessels et al., 2017 ).
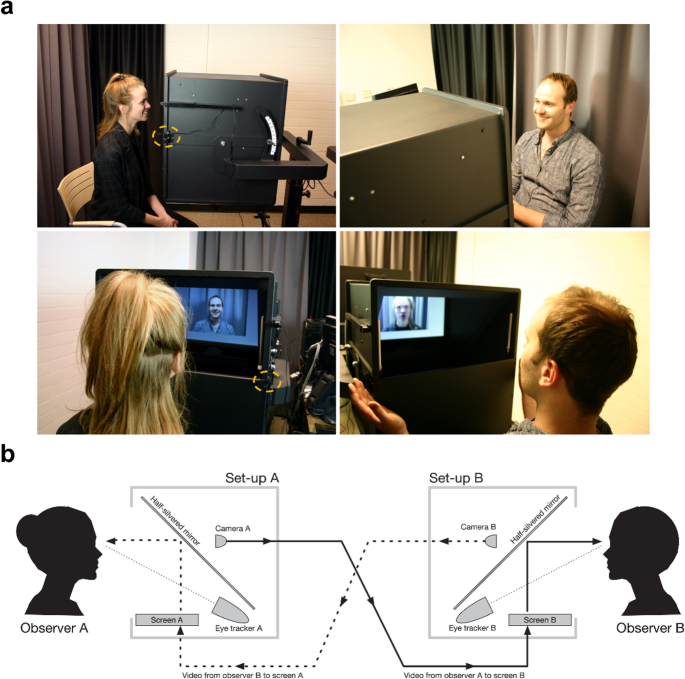
Overview of the dual eye-tracking setup. a Staged photographs of two interactors in the dual eye-tracking setup. b A schematic overview of the setup, reproduced from Hessels et al. ( 2018b ).
Audio was recorded using a set of AKG C417-PP Lavalier-microphones which were connected to a Behringer Xenyx 1204-USB audio panel. Each microphone was attached to the front of each setup (see the dashed orange circles on the left panels in Fig. 1 a). We used Audacity v. 2.3.3 running on a separate computer (Ubuntu 18.04.2 LTS) to record audio. In a stereo recording the signal of the parent was panned to the left channel and the signal of the child was panned to the right channel. Upon recording start, a 100 ms pulse was sent from the parallel port of the stimulus computer to the audio panel to be recorded. This resulted in a two-peak signal we used to synchronize the audio recordings to the beginning and end of the video and eye-tracking recordings. Audio recordings were saved to disk as 44,100 Hz 32-bit stereo WAVE files. We describe in detail how the data were processed for the final analyses in the Signal processing section below.
Upon entering the laboratory, a general instruction was read out by the experimenter (author GAH). This instruction consisted of a brief explanation of the two conversation-scenarios and the general experimental procedure. Participants were asked not to touch any equipment during the experiment (e.g. the screen, microphones, eye trackers). Because the experimenter needed to start and stop the video-feed after approximately five minutes for each conversation, he explained that he would remain present during the measurements to operate the computers. After the general instruction, participants were positioned in the dual eye-tracking setup. Participants were seated in front of one of the metal boxes at either end of the setup (containing the screens and eye trackers) such that their eyes were at the same height as the webcams behind the half-silvered mirrors using height-adjustable chairs. The distance of participants’ eyes to the eye tracker was approximately 70 cm and the distance from eyes to the screen was approximately 81 cm. After positioning, the experimenter briefly explained the calibration procedure. The eye tracker of the parent was calibrated first using a 5-point calibration sequence followed by a 4-point calibration validation. We aimed for a systematic error (validation-accuracy) below 1° in both the horizontal and vertical direction (they are returned separately by iViewX ). However, if for some reason, a sufficiently low systematic error could not be obtained, the experimenter continued anyway (see Section 11 how these recordings were handled). After calibrating the parent’s eye tracker, we continued with the child’s eye tracker. After the calibration procedure, the experimenter briefly repeated the task-instructions and explained that he would initiate the video-feed after a countdown. The experimenter repeated that he would stop recording the conversation after approximately five minutes. The experimenter did not speak or intervene during the conversation, only if participants questioned him directly, or when participants had changed their position too much. In the latter case, this was readily visible from the iViewX software which graphically and numerically displays the online gaze position signals of the eye trackers. If participants slouched too much, the incoming gaze position signals would disappear or show abnormal values. In such instances, the experimenter would ask the participants to sit more upright until the gaze position signals were being recorded properly again.
Conflict-Scenario
For the first conversation, children and their parents were instructed to discuss a family issue about which they have had a recent disagreement. The goal of the conflict-scenario was to discuss the topic of disagreement and to try to agree on possible solutions for the future. To assist the participants in finding a suitable topic, the experimenter provided a list with common topics of disagreements between parents and children. The list included topics such as screen time, bedtime, homework, and household chores (see Appendix 1 for a complete overview). The main criteria for the conflict-scenario were that the topic should be about a recent disagreement, preferably in the last month. If no suitable topic could be found on the list or could be agreed upon, the parent and child were asked to come up with a topic of their own. Some parent–child dyads could not decide at all or requested to skip the conflict-task altogether. Note that for the final analyses, we only included dyads that completed both scenarios (see Fig. 1 and Table 1 ). After participants had agreed on a topic, the experimenter explained that they should try to talk solely about the chosen topic for approximately 5 min and not digress.
Cooperation-Scenario
For the second conversation, participants were instructed to plan a party together (e.g. birthday or family gathering). The goal of the cooperation-scenario was to encourage a cooperative interaction between the parent and child. Participants were instructed to discuss for what occasion they want to organize a party and what kinds of activities they want to do. Importantly, participants had to negotiate the details and thus needed to collaborate to come up with a suitable party plan. Participants were instructed to discuss the party plan for approximately 5 min. Prior to the second conversation, the experimenter checked the participants’ positioning in front of the eye trackers and whether the eye trackers and microphones were still recording properly. In some cases, the experimenter re-calibrated the eye trackers if participants had changed position, or if the eye tracker did not work for whatever reason. Note that we always started with the conflict-scenario and ended with the cooperation-scenario because we reasoned this would be more pleasant for the children.
After the cooperation-scenario, the experimenter thanked the parents and children for their participation and the child received a small gift. The experimenter also asked how the participants had experienced the experiment, and if they were left with any questions about the goal of the experiment.
Signal Processing
To prepare the eye-tracking, audio, and video signals for the main analyses, we conducted several signal processing steps (e.g. synchronization, classification). In the following sections, we describe these separate steps. Readers who do not wish to consider all the technical and methodological details of the present study may wish to proceed to the Results, Section 12 .
Synchronization of eye-tracking signals and video recordings . By using timestamps produced by the stimulus computer, the eye-tracking signal was automatically trimmed to the start and end of the experimental trial. Next, the eye-tracking signal was downsampled from 120 to 30 Hz to correspond to the frame rate of the video. In the downsampling procedure, we averaged the position signals of four samples to produce a new sample. This caused the signal-to-noise ratio to increase by a factor of 2 (√4) due to the square root law.
Construction of Areas of Interest (AOI) and AOI assignment of gaze position. To determine where and when participants looked at each other’s faces, we mapped gaze coordinates unto the frontal video-recordings. Because participants moved and rotated their faces and bodies during the conversations, we used an AOI construction method that can efficiently and effectively deal with an enormous number of images, namely the thousands of video frames produced in this experiment. This method consists of the fully automated Limited Radius Voronoi Tessellation procedure to construct Areas-of-Interest (AOIs) for facial features in dynamic videos (Hessels et al., 2016 ). Briefly, this procedure assigns each gaze position to one of the four facial features (left eye, right eye, nose, or mouth) based on the closest distance to the facial feature. If this minimal distance exceeds the limited radius, gaze position was assigned to the background AOI (see Fig. 2 ). This background area consists of the background and small parts of the upper body of the participant visible in the video. In our study, the LRVT-radius was set to 4° Footnote 1 (200 pixels). The LRVT method is partly data-driven, resulting in smaller AOIs on the children’s faces compared to AOIs on the parents’ faces. We quantified the AOI size by the AOI span. The AOI span is defined as the mean distance from each AOI cell center to the cell center of its closest neighbor (see Hessels et al., 2016 , p. 1701). The average AOI-span for parents’ faces was 1.76° and an average AOI span for children’s faces was 1.6°.
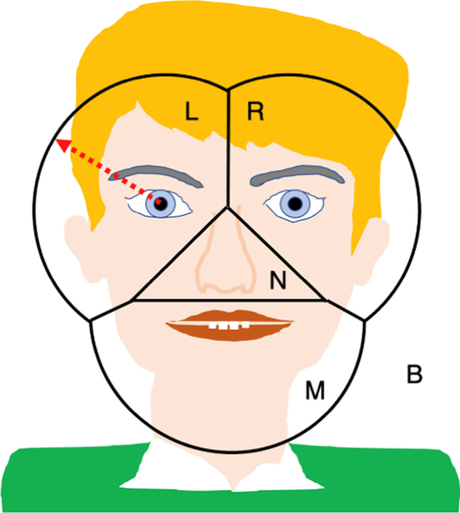
An example for computer-generated AOIs (Hessels, et al., 2018a ) for the left eye (L), right eye (R), nose (N), and mouth (M). The AOI for the left eye, for example, is the area closest to the left eye center but not further away from the center than the bounded radius of 4° (denoted with a red arrow). The background AOI (B) encompasses the background, the upper body of the participant and a small part of the top of the head
From gaze data to dwells. After individual gaze samples were mapped unto AOIs (see previous section), we computed ‘dwells’, defined here as the time spent looking at a particular face AOI (e.g., eyes, mouth). We operationalized a single dwell as the period between when the participants’ gaze position entered the AOI radius until gaze position exited the AOI, providing that the duration was at least 120 ms (i.e., four consecutive video frames). For further details, see Hessels et al. ( 2018b , p. 7).
From raw audio recordings to speaker categories.
Trimming to prepare audio for synchronization with video and eye-tracking. In a self-written audio visualization script in MATLAB, we manually marked the timepoints of the characteristic two-peak synchronization pulse sent by the stimulus computer (see Section 7 ), which indicated the start and stop of the two conversations at high temporal resolution (timing accuracy < 1 ms). Then we trimmed the audio files based on these start-and-stop timepoints. Next, the trimmed stereo files (left channel – the parent, right channel—the child) were split into two mono signals, and the first conversation (conflict) and the second conversation (cooperation) were separated. Finally, the audio signal was downsampled to 1000 Hz and converted into an absolute signal. As a result, we produced four audio files per parent–child dyad.
Determination of speech samples. Speech episodes (as an estimator for who was speaking) were operationalized as follows. First, the absolute audio signal was smoothed with a Savitsky-Golay filter (order 4; window 500 ms). Then, samples were labelled silent when the amplitude was smaller than 1.2 times the median amplitude of the whole filtered signal. Then, we computed the standard deviation of the amplitude of the silent samples. Subsequently, a sample was labelled as a speech sample if the amplitude exceeded the mean plus 2 times the standard deviation of the amplitude of the silent samples.
Removing crosstalk . Because the microphones were in the same room, we observed crosstalk in the audio channels. That is, we sometimes heard that parent speech was present in the audio recording channel of the child and vice versa. The child’s channel suffered more from crosstalk than the parent’s channel. To deal with this, we first equalized the speech signals of the parent and child by making them on average equally loud (by using the average speech amplitudes of the single episodes) Then, we identified episodes of potential crosstalk by selecting the episodes that contained a signal in the channel of both speakers. We removed crosstalk with the following rule: We assigned a crosstalk episode to X if the amplitude of the signal in the X’s channel was 3.33 times larger than in the Y’s channel. The value 3.33 was derived empirically. If a crosstalk episode was assigned to the child, it was removed from the parent’s channel and vice versa.
Determination of speech episodes. From the labelled samples, we determined speech episodes. Each speech episode is characterized by an onset and offset time, mean amplitude and duration.
Removing short speech and short silence episodes . We removed speech episodes shorter than 400 ms followed by the removal of silence episodes shorter than 100 ms.
Assigning speech labels to single samples . To link the speech signal to the eye-tracking signal in a later stage, we assigned speech labels to each sample of the speech signal of the parent–child dyad. For each timestamp, we produced a label for one of the following categories: parent speech, child speech, speech overlap and silence (no one speaks).
Combining speech and gaze behavior. The speech signal was combined with the gaze signal as follows. First, we upsampled the gaze signal (with AOI labels) from 30 to 1000 Hz by interpolation to match the sampling frequency of the audio signal. Each sample in the combined signal contained a classification of speaker categories (child speaks, parent speaks, both are speaking, no one speaks) and gaze location on the face for both child and parent (eyes, nose, mouth, background). Not all recordings produced valid eye-tracking data (eye-tracking data loss), valid AOIs for all video frames (e.g. due to extreme head rotations construction of AOIs is impossible), or valid dwells (i.e. dwells longer than 120 ms). These invalid cases were marked in our combined audio/gaze database. This is not necessarily problematic for data analysis, because we also conducted analyses on parts or combinations of parts of the data (e.g. speech analysis only).
Measures of speech and gaze. In this study, we mainly report relative measures of speech and gaze behavior because the total recording durations differed across dyads and conversations. We computed relative total speech durations as a descriptor of speech behavior and relative total dwell times as a descriptor of gaze behavior. To obtain relative measures, we determined the total duration for each speaker category (i.e. parent speech, child speech, overlap, no one speaks) and the total duration of dwells (with AOI labels eyes, nose, mouth, background) and then divided these durations by the total duration of the recording.
Eye-Tracking Data Quality and Exclusion
We first assessed the quality of the eye-tracking data, which is crucial for the validity of an eye-tracking study (Holmqvist et al., 2012 ). High-quality eye-tracking data is typically obtained when subjects are restrained with a chinrest/headrest to maintain equal viewing distance and minimize head movements. However, in the context of our face-to-face conversations, subjects could talk, gesture, move their face, head, and upper body. Although the dual eye-tracking setup used was specifically designed to allow for these behaviors, other eye-tracking studies have also demonstrated that such behaviors may negatively affect eye-tracking data quality (Hessels et al., 2015 ; Holleman et al., 2019 ; Niehorster et al., 2018 ). Moreover, young children may pose additional problems, such as excessive movement or noncompliance (Hessels & Hooge, 2019 ). We computed several commonly used eye-tracking data quality estimates, namely: accuracy (or systematic error) , precision (or variable error), and data loss (or missing data).
First, we assessed accuracy. The average validation accuracy of parents’ recordings was 0.98°. For the children’s recordings it was 1.48°. We set an exclusion criterion of 1° for the 2d-validation accuracy. Second, we determined precision by computing the sample-to-sample root mean square deviation (s2s-RMS) of the gaze-position signal. We then divided the s2s-RMS values for every participant by the AOI span (see Section Signal Processing ). This measure can range from 0 to infinity. A precision/AOI-span value of 1 means that precision is equal to the AOI span. In other words, a value of 1 means that the sample-to-sample variation of the gaze-position signal is equal to the average distance between AOIs. If the precision/AOI-span is larger than 1 this means that one cannot reliably map gaze position to an AOI. Therefore, we decided to exclude measurements in which the average precision/AOI span exceeded 1. Also, this measure accounts for differences between the recordings in the magnitude of the AOI spans in relation to the recorded gaze position. We also calculated periods of data loss – i.e. when the eye tracker did not report gaze position coordinates. Data loss is a slightly more complicated measure in the context of our study, given that data loss may coincide with talking and movement (Holleman et al., 2019 ). For example, it is well-known that some people gaze away more when speaking compared to when listening (Hessels et al., 2019 ; Kendon, 1967 ). Therefore, any exclusion based on data loss may selectively remove participants that spoke relatively more. For that reason, we did not exclude participants based on data loss but conducted separate sensitivity analyses for all our main findings as a function of a data loss exclusion criterion (see Appendix 3).
Based on the criteria for accuracy and precision, we determined how many measurements were suitable for further analyses. Figure 2 and Table 1 depict an overview of the eye-tracking data quality assessment and how many participants were excluded for further analyses based on exclusion criteria described above. Out of 81 parent–child dyads who participated, 73 dyads completed the experiment (i.e. participated in both conversation-scenarios). Out of this set, we had eye-tracking data of sufficient quality for 40 parents and 13 children. Descriptive statistics of the participants are given in Table 1 . Note that although the quality of the eye-tracking data is known to be worse for children, it was particularly problematic in our study as we needed data of sufficient quality for both conversations to answer our research questions. Although many more participants had at least one good measurement, applying our data quality criteria to both conversations for every parent–child dyad resulted in these substantial exclusion rates (Fig. 3 )
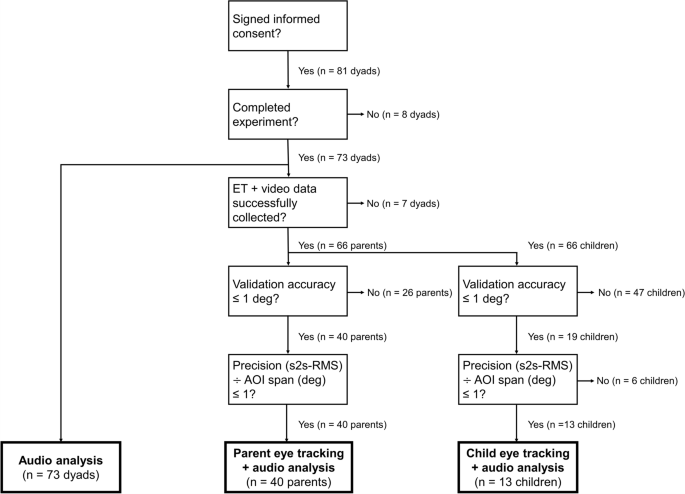
Flowchart of eye-tracking data quality assessment and exclusion criteria
Main Analyses
We present three main analyses in which we address (1) the similarities and differences between parents and children’s speech and gaze behavior during face-to-face interaction, (2) whether patterns of speech and gaze behavior in parent–child conversations are related to the topics of conversation (conflictive versus cooperative), and (3) whether gaze behavior to faces is related to whether someone is speaking or listening. For all our figures and statistical descriptions, we used detailed visualizations and bootstrapping techniques provided by Rousselet et al. ( 2017 ). Specifically, we used the Harrell-Davis estimator to compute 95% confidence intervals around the medians of each distribution with the MATLAB function decilespbci . The number of bootstrap samples was set to 2,000. If these 95% CIs do not overlap with the zero-difference line, we concluded that, statistically, the numerical difference is meaningful (or 'significant') as the 0 is not included in 95% CI around the median. We based this analysis strategy on Rousselet et al. ( 2017 ), who showed that non-parametric bootstrapping methods combined with clear visualisations may be more informative than a frequentist t-test only. Moreover, the bootstrapping technique is less susceptible to e.g. deviations from normality than regular t-tests.
Speech Behavior
In this section, we report parents’ and children’s speech behavior over the course of the two conversation-scenarios: conflict and cooperation. We wanted to know whether parents and children differed in how much they spoke when discussing potential (dis)agreements. For the analyses of speech behavior, we used the Audio-only dataset (see Table 1 ), which consisted of 73 parent–child dyads who completed both conversations. The average duration of the conflict-scenario was 281.25 s ( sd = 27.78 s) and the average duration of the cooperation-scenario was 297.37 s ( sd = 28.69 s).
Similarities and Differences Between Parents and Children
Figure 4 depicts parents’ and children’s relative total speech durations (i.e. how much they spoke as a percentage of the total conversation) across the two conversation-scenarios. We estimated relative speech durations of four speaker-categories: child speaks, parent speaks, both speak (‘overlap’), and no one speaks (‘none’). As is visible from Fig. 4 (left panels) both parents and children varied substantially in how much they spoke in total over the course of the two conversations. For example, the range of relative speaking durations ranged from less than 5% for some individuals to nearly 50% of the total conversation duration. Overall, parents spoke for a longer total time compared to children, regardless of the conversation scenario. The median relative speaking duration for parents was 39.19%, 95% CI [37.69% – 40.53%] in the conflict-scenario and 34.64%, 95% CI [32.11% – 37.14%] in the cooperation-scenario, whereas the median relative speaking duration for children in the conflict-scenario was 18.51%, 95% CI [16.65% – 20.34%] and 23.59%, 95% CI [21.91% – 26.23%] in the cooperation-scenario.
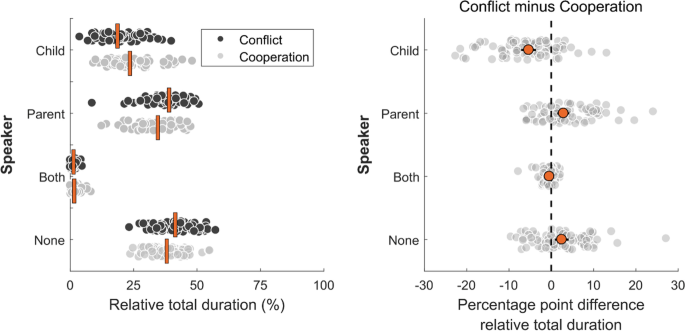
Left panel. Speech behavior of 73 parent–child dyads for the two conversation-scenarios (conflict and cooperation). Dark grey markers represent the relative total speech duration (as a percentage) of each participant during the conflict-scenario and light grey markers represent the relative total speech duration of each participant during the cooperation-scenario. The vertical orange stripes represent the median relative speech durations per speaker-category. Right panel. Difference-scores of speech behavior (conflict minus cooperation). Difference-scores were computed by subtracting participants’ relative speech durations in the cooperation-scenario from the conflict-scenario for every speaker-category. Light grey markers represent individual difference scores (as percentage point difference in relative total duration). The orange markers represent the median difference-score of the relative speech durations and the error bars (barely visible) represent 95% confidence intervals of the median, both of which were obtained through bootstrapping using the MATLAB-function decilespcbi provided by Rousselet et al. ( 2017 ). The vertical dashed line represents a zero-difference line. Negative difference scores indicate that the participant spoke less in the conflict-scenario than in the cooperation-scenario (and vice versa for positive difference scores)
Speech Behavior as a Function of Conversation Scenario
To compare how individual parents’ and children’s total speech durations differed between the two conversation-scenarios, we computed a difference-score for each participant by subtracting their relative total speech duration in the cooperation-scenario from their relative total speech duration in the conflict-scenario, see Fig. 4 (right panel). A negative difference-score (i.e. value on the left side of the zero-difference line) means that the participant spoke less in the conflict-scenario compared than in the cooperation-scenario and a positive difference-score (i.e. value on the right side of the zero-difference line) means that the participant spoke more in the conflict-scenario compared to how much they spoke in the cooperation-scenario. As is visible from Fig. 4 (right panel), parents spoke more during the conflict-scenario than during the cooperation-scenario, as indicated by a positive median difference in relative total speaking duration of 2.91 percentage points (pp), 95% CI [1.42 pp – 4.27 pp]. Conversely, children spoke more in the cooperation-scenario compared to the conflict-scenario, as indicated by a negative median difference in relative total speaking duration of -5.32 pp, 95% CI [-7.15 pp – -3.60 pp]. Finally, there was slightly more silence (i.e. neither parent or child was speaking) during the conflict-conversation compared with the cooperation-scenario, as shown by the positive median difference-score of 2.50 pp, 95% CI [0.86 pp – 4.10 pp] for the ‘none’ speaker-category.
Gaze Behavior
In this section, we report parents’ and children’s gaze behavior to facial features in relation to the conversation-scenarios. We analyzed whether and how parents and children differed in where they looked at the other’s faces when discussing (dis)agreements. Based on previous eye-tracking studies on face scanning behavior (e.g. Mehoudar et al., 2014 ; Peterson et al., 2016 ; Rogers et al., 2018 ), we expected that parents and children will look predominantly at each other's faces, but that participants may exhibit large individual differences in where at the face is looked at most (eyes, nose, or mouth). We had no expectations regarding gaze behavior as a function of conversation-scenario. For the analyses of gaze behavior, eye-tracking data of 40 parents and 13 children were used (see Table 1 ).
First, we analyzed the individual differences in parents’ and children’s gaze behavior to facial features. Figure 5 (upper panels) depicts relative total dwell times to face AOIs (e.g. eyes, nose, mouth) and the background AOI as a function of conversation-scenario (conflict and cooperation). As is visible from Fig. 5 , both parents (top left panel) and children (top right panel) varied greatly in the total duration that they looked at each other’s faces, as indicated by the large range in relative total dwell times to different face AOIs (0 to approximately 50–75% of total looking time for the eyes and mouth AOI). This was regardless of the conversation scenario. Large individual differences in gaze behavior to facial features matches our expectation based on previous research. A smaller range in relative total dwell time to the background AOI was observed, ranging from 0 to approximately 15% of total available looking time. To investigate the consistency of participants’ gaze behavior to facial features across the two conversations, we computed Spearman rank correlations for both parents’ and children’s relative total dwell time on face AOIs and the background AOI. For the parents, we found a high level of consistency in relative total dwell time across conversation scenarios for all AOIs (eyes AOI p = 0.73, p < 0.00001; nose AOI ρ = 0.73, p < 0.00001; mouth AOI ρ = 0.81, p < 0.00001; background AOI ρ = 0.72, p < 0.00001). For the children, we also found a high level of consistency in relative total dwell time across conversation scenarios for most AOIs (eyes AOI ρ = 0.80, p = 0.001; mouth AOI ρ = 0.78, p = 0.002; background AOI ρ = 0.78, p = 0.002), but slightly less so for the nose AOI (ρ = 0.43, p = 0.140). These Spearman correlations show that individuals were consistent in where they looked at on average on the other person’s face across the two conversations. One difference that stands out from Fig. 5 is that parents had higher median relative dwell times for all face AOIs compared with those of the children. However, we cannot conclude that this is a meaningful difference as data loss was generally much higher for the children than for the parents (see Appendix 2).
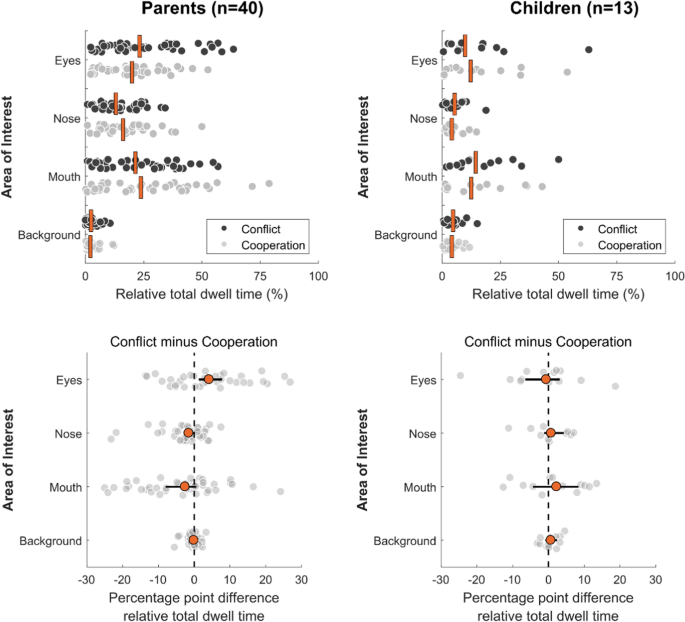
Gaze behavior as a function of conversation-scenario. Distributions of gaze behavior to the facial feature (eyes, nose, mouth) and background AOIs for parents (left panels) and children (right panels). Upper panels. Relative total dwell times (as a percentage) as a function of conversation-scenario (conflict and cooperation). Dark grey markers represent the relative total dwell time of each participant during the conflict-scenario and light grey markers represent the relative total dwell time of each participant during the cooperation-scenario. The vertical orange stripes represent the median relative total dwell time per AOI-category. Lower panels. Distributions of difference-scores of relative total dwell time to the AOIs (conflict minus cooperation). Difference-scores were computed by subtracting participants’ relative total dwell time to the AOIs in the cooperation-scenario from the conflict-scenario. Light grey markers represent individual difference scores (as percentage point difference in relative total dwell time). The orange markers represent the median difference-score of the relative total dwell times and the error bars represent 95% confidence intervals of the median, both of which were obtained through bootstrapping using the MATLAB-function decilespcbi provided by Rousselet et al. ( 2017 ). The vertical dashed line represents a zero-difference line. Negative difference scores indicate that the participant gazed more at a particular AOI in the cooperation-scenario than in the conflict-scenario (and vice versa for positive difference scores)
Gaze Behavior as a Function of Conversation Scenario
Next, we analyzed whether parents and children differed in what regions of each other’s face they looked at as a function of the conversation-scenario (conflict and cooperation). To investigate this, we compared the within-subject differences in gaze behavior to facial features of parents and children across the two scenarios. We computed the individual and median difference-scores in relative total dwell time per AOI, by subtracting participants’ relative total dwell time to face AOIs in the cooperation-scenario from the conflict-scenario. As is visible from Fig. 5 , parents (lower left panel) gazed slightly more at the child’s eyes AOI during the conflict-scenario, as indicated by a positive median difference-score of 4.15 percentage points (pp), 95% CI [1.28 pp – 7.78 pp] and a non-overlapping error bar with the zero-difference line. Also, it seemed that parents looked slightly more at the mouth AOI during the cooperation-scenario, as indicated by a negative median difference-score of -2.54 pp, 95% CI [-7.99 pp – 0.55 pp]. However, notice that the confidence interval overlaps slightly with the zero-difference line. For the children, no differences were observed between their relative total dwell times to face AOIs as a function of the conflict and cooperation scenario.
Gaze Behavior to Faces and its Relation to Speech
In this section, we report parents’ and children’s gaze behavior to facial features in relation to speech behavior. We wanted to know where participants looked at the other’s face during episodes of speaking and listening. Based on previous literature, we may expect that participants will gaze slightly more at the mouth when listening, and more at the eyes when speaking (Rogers et al., 2018 ). For these analyses, we computed relative total dwell times to AOIs as a function of speaker-state, by summing all dwells per AOI (eyes, nose, mouth, background) for all the classified episodes of self-speech (participant is speaking) and other-speech (other person is speaking) per conversation scenario (conflict, cooperation). Note that this excludes episodes of overlap or silence. Then we computed a relative measure of total dwell time per AOI category during self-speech and other-speech by dividing the total duration of dwells by the total duration of the conversation. Finally, we averaged relative total dwell times across the two scenarios.
Figure 6 (upper panels) depicts participants’ relative total dwell times to the AOIs as a function of self-speech and other-speech. As is visible from Fig. 6 (upper panels), individual parents and children varied substantially in where they looked at the different face AOIs (eyes, nose, mouth, background), regardless of speaker-state. For most parents and children, relative total dwell times to face AOIs ranged somewhere between 0 and 50%, regardless of speaker-state, and some individuals gazed at a particular face AOI for more than 50–75% of the time. Furthermore, total dwell times to the background-AOI were substantially lower on average, ranging between 0 and 18% of the total duration of available looking time during self-speech and other-speech. Again, parents had higher median relative dwell times for all face AOIs, but as stated previously, we cannot conclude that this is a meaningful difference as data loss was generally higher for the children than for the parents (see Appendix 2).
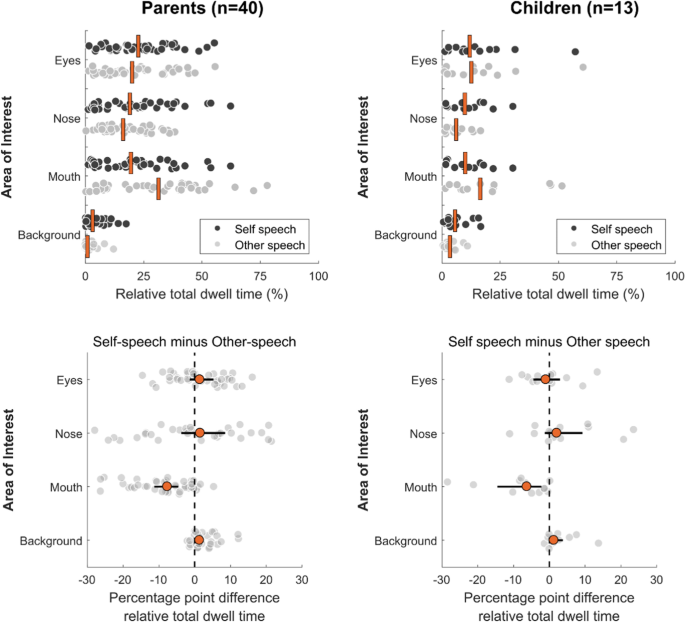
Gaze behavior as a function of speaker-state. Distributions of gaze behavior to the facial feature and background AOIs for parents (left panels) and children (right panels). Upper panels. Relative total dwell times (as a percentage) as a function of self-speech and other-speech. Dark grey markers represent the relative total dwell time to face AOIs (eyes, nose, mouth) and the background AOI of each participant when speaking and light grey markers represent the relative total dwell time to facial features of one participant when the other person was speaking). The vertical orange stripes represent the median relative total dwell time per AOI. Lower panels. Distributions of difference-scores of relative total dwell time to the AOIs (self-speech minus other-speech). Difference-scores were computed by subtracting participants’ relative total dwell time to face AOIs during other-speech from their relative total dwell time during speaking. Light grey markers represent individual difference scores. The orange markers represent the median difference-score of the relative total dwell times and the error bars represent 95% confidence intervals of the median, both of which were obtained through bootstrapping using the MATLAB-function decilespcbi provided by Rousselet et al. ( 2017 ). The vertical dashed line represents a zero-difference line. Positive difference scores indicate that the participant gazed more at particular AOI during episodes of self-speech. Negative difference scores indicate that the participant gazed more at a particular AOI during episodes of other-speech
Next, to compare how parents and children looked at the other’s face during episodes of self-speech and other-speech we computed individual within-subject difference-scores by subtracting the relative total dwell times during other-speech from relative total dwell times during self-speech (see Fig. 6 , lower panels). Note that a negative difference-score indicates that the participant gazed more at a particular AOI during other-speech compared to self-speech, and a positive difference-score means the participant gazed more at a certain facial feature during episodes of self-speech. For both the single parent (n = 40) and single child (n = 13) data sets, a negative median difference-score was observed for gazing at the mouth AOI. We found a median difference-score in total dwell time on the mouth AOI for the parents of -7.64 percentage points (pp), 95% CI [-11.27 pp – -4.58 pp], and for the children the median difference-score of total dwell time on the mouth AOI was -6.28 pp, 95% CI -14.12 pp – -2.39 pp]. Thus, overall, participants slightly gazed more at the mouth of the other when listening compared to when they were speaking themselves.
In this study, we investigated the role of gaze behavior to faces during conversations between parents and their preadolescent children about conflictive and cooperative topics. The following research questions were formulated: 1) What are the similarities and differences between parents’ and children’s speech and gaze behavior during face-to-face interaction? For example, we were interested in how much parents and children spoke over the course of the interaction, and what regions of the face they looked at during the conversations. 2) Are patterns of speech and gaze behavior in parent–child conversations related to the topics of conversation (conflictive versus cooperative)? 3) Is gaze behavior to faces during parent–child interaction related to who is speaking or listening? To estimate gaze behavior to facial features during parent–child interactions, we used a dual eye-tracking setup to obtain audio recordings, frontal videos, and gaze position of parents and their children engaged in conflict and cooperation conversations. We first briefly recap the results regarding the similarities and differences in speech and gaze behavior as a function of the two conversational scenarios, and then we recap the results regarding the relation between gaze and speech behavior. As this study represents a first attempt to study gaze behavior to facial features during parent–child interactions using a dual eye-tracking setup, we will also consider several limitations and possibilities of this technology for the study of parent–child interactions.
Summary and Interpretation of Results
Regarding our research questions on the similarities and differences in speech behavior between parents and children, and whether patterns of speech behavior were related to the conversation topic, we found clear differences in how much parents and children spoke across the two conversations. Overall, parents spoke more than children regardless of the conversation-scenario. Parents spoke more in the conflict-scenario compared with how much they spoke in the cooperation-scenario, while children spoke more in the cooperation-scenario compared to how much they spoke in the conflict-scenario. Finally, there was more silence during the conflict-scenario (neither the parent nor child was speaking).
The results on speech behavior clearly show that the two conversation-scenarios (conflict and cooperation) substantially influenced the dynamics of the interaction. This is likely due to how the two conversation-scenarios differ with regards to the role that the parent and the child take. When planning the party together (cooperation scenario), the parent and the child are more egalitarian partners, as they both recognize that their wishes and ideas carry the same weight. In the conflict situation, however, the topics that were chosen to discuss mostly concerned the child’s behaviors that the parent considered undesirable (e.g. not cleaning one's own room, not listening to the parents, fighting with a brother or sister). In such situations, the parent tends to take a lead, assert their authority, and consequently speaks more (Moed et al., 2015 ). The child, on the other hand, recognizes the parent’s authority in such matters and mostly such conflicts are resolved by the child giving in to the parent’s demands (see Laursen & Collins, 2009 for a review). This submissive role is reflected in children speaking less in the conflict discussion. Moreover, the conflict discussion likely elicits more tension and uncomfortable feelings in children than in the cooperation scenario (Thomas et al., 2017 ), which might be an additional explanation for their smaller contribution to the conflict discussion with parents. As the nature of parent–child conflicts changes significantly across various stages of development (Dunn & Slomkowski, 1992 ; Laursen & Collins, 2009 ; Steinberg, 2001 ), one may expect that patterns of gaze and speech behavior are different for parent–child conflicts in early or late childhood, or in early adolescence and late adolescence. Our participant sample consisted of preadolescent children (8–10 years) which somewhat precedes the relational changes in parental authority and child autonomy in adolescence. As such, we would expect that the contribution of children in the conversation would increase as they move through adolescence and the parent–child relationship becomes more egalitarian.
Regarding our research question on the similarities and differences in gaze behavior to faces of parents and children, we found substantial individual differences for both parents and children in what region of the other’s face was looked at most. In line with previous research with adults (Arizpe et al., 2017 ; Peterson et al., 2016 ; Rogers et al., 2018 ), we found that some parents and children looked most at the eyes while others looked at different regions of the face more equally (eyes, nose, and mouth). This was the case regardless of the conversation-scenario. While parents seemed to look more at the faces of their children than vice versa, this could be due to differences in data loss between parents and children (see Appendix 2).
Furthermore, we investigated whether gaze behavior to faces was related to the topic of the conversation. We did not find any differences in where children gazed at the parent’s face as a function of the conversation topic. Interestingly, we did find that on average parents gazed more at the child’s eyes during the conflict-scenario than in the cooperation-scenario. One reason may be that increased eye gaze asserts dominance and social status (Kleinke, 1986 ; Patterson, 1982 ). In this sense, increased gaze at the other person’s eyes may serve as a nonverbal emphasis on a particular verbal message to persuade another person, or to press a particular response from that person (Timney & London, 1973 ). Thus, increased eye gaze from the parent may signal authority while negotiating a family disagreement and persuade the child towards some goal or solution, at least in our conflict-scenario. However, increased gaze to the eyes has also been associated with the expression of affiliation and intimacy (Kleinke, 1986 ; Patterson, 1982 ). Thus, it could also be that parents looked more at the child’s eyes in the conflict-scenario because they wanted to express more intimacy while negotiating a potentially conflicting topic of discussion. Our findings do not distinguish between these two potential explanations. If increased gaze to the eyes of the child during the conflict conversation would indeed be the result of social control exercised by the parent, one may expect that the difference scores in gaze to eyes between the two conversations are correlated with some index of parental authority. If, on the other hand, increased gaze to the eyes would be an expression of intimacy, one may expect that the difference scores correlate with some measure of intimacy or interpersonal reactivity. Moreover, it would be interesting to investigate whether parents purposively exert authority or intimacy by means of increased gaze to the eyes when discussing a conflicting topic with their children.
It is important to emphasize that, although the semi-structured conversation paradigm used in this study is designed to elicit ‘conflict’ and ‘cooperation’ dynamics in parent–child interactions, both conversation scenarios could contain elements of collaboration, disagreement, and compromise. This was especially clear from listening to the content of the conversations. The ‘conflict’ conversations could contain both disagreement and collaboration, as parents and children often needed to collaborate to come up with a solution to their disagreement, for example, by settling for a compromise between the wishes of both parent and child. Also, we did not observe any ‘extreme’ conversations, e.g., in which participants raised their voices or yelled. Furthermore, the ‘cooperation’ conversation also could contain disagreements. Often, the parents did not agree with the ideas of their child, nor did children always comply with the demands of their parent. For example, the goal to organize a party in the ‘cooperation’ conversation occasionally led to disagreement, as some ideas of the child for the party plan (e.g., how many friends to invite, what activities to do) were not accepted by the parents. In other words, the distinction between ‘conflict’ and ‘cooperation’ as general labels to describe the content of interaction is not always clear cut. Nevertheless, the different scenarios did result in differences in patterns of speech and gaze behavior.
Finally, we also investigated whether gaze to facial features in parent–child interactions is dependent on speaker-state, because previous eye-tracking studies suggest that the region of the face people look at during conversations depends on whether they are speaking or listening (Rogers et al., 2018 ). Both parents and children looked more at the mouth region while listening than while speaking. This is in accordance with several non-interactive eye-tracking studies, which have shown that observers presented with videos of talking faces tend to gaze more at the mouth area, especially under noisy conditions or when tasked to report what is being said (Buchan et al., 2007 ; Vatikiotis-Bateson et al., 1998 ; Võ et al., 2012 ). Similar to Rogers et al. ( 2018 )’s interactive eye tracking study, we found that both parents and children gazed slightly more at the mouth when the other person spoke compared to when they were speaking themselves, although these differences were small on average (i.e. approximately 5–10% of total looking time), but see also Hessels et al. ( 2019 ). In our study, we found that differences in mouth-looking between speaking and listening were always (or almost always) in the same direction, although the magnitude of this difference varied between individuals. Increased gaze at the mouth when listening to speech may be explained by the fact that people look at the visual cues from mouth and lip movements to support speech perception (Sumby & Pollack, 1954 ; Vatikiotis-Bateson et al., 1998 ).
Possibilities, Problems, and Future Directions of Eye-Tracking to Study Gaze Behavior in Parent–Child Interactions
Our study is one of the first to use eye-tracking to study gaze to facial features and its relation to speech behavior in parent–child interactions. Previous research has often been conducted using observational techniques (i.e. which lack in reliability and validity to distinguish gaze to specific facial features), or has been limited to non-interactive eye-tracking procedures with photographs and videos of faces (Risko et al., 2016 ). As such, the main contribution of our study is empirical, by describing patterns of gaze and speech behavior during different types of conversations (conflict or cooperation). Moreover, the specific interpersonal context (parent–child interaction) is relevant for child development and has not been studied in this manner before. We focused on how aggregate speech and gaze behavior (e.g. total speech durations and dwell times) differed between parents and children and as a function of conversation topic and speaker-state. In future research, it will be useful to investigate the moment-to-moment characteristics of speech and gaze during parent–child interactions, for example, by looking at transitions between face AOIs as a function of speaker-state and conversation-scenarios. Also, it may be interesting to investigate to what extent patterns of verbal and nonverbal behavior are indicative of parents’ conversational style and conflict resolution strategy (Beaumont & Wagner, 2004 ; Moed et al., 2015 ), and how this is related to children’s adjustment, emotional reactivity, and social competence (Junge et al., 2020 ; Moed et al., 2017 ). For example, functional conflict resolution is typically characterized by validation, support, listening, and expressing positive or neutral affect, whereas dysfunctional conflict styles consist of negative affect, criticism, and hostility (Laursen & Hafen, 2010 ; Moed et al., 2017 ). We showed that parents look longer at the eyes during the conflict-scenario, but to discern whether this could also be exemplary of either functional or dysfunctional conflict resolution strategies would require in depth sequential analyses of speech content, voice affect, gaze, and facial expressions. Although this may seem like a daunting task, it may be a worthwhile approach to provide new and crucial insights into distinguishing different types of conflict styles in parent–child interactions.
This study exemplifies how dual eye-tracking technology can be used to objectively measure gaze behavior to faces during full-fledged interactions. However, there are also many challenges and limitations of eye-tracking when applied to the context of human interaction (for a recent review, see Valtakari et al., 2021 ). In this study, a lot of eye tracking data was lost during the measurements and many recordings were lacking in precision and accuracy. Therefore, we had to exclude many participants based on data quality criteria (see Fig. 3 ). Due to the limited sample size for some analyses in this study, we are hesitant to claim that these results would generalize to all parent–child dyads. Given the behaviors of interest in this study, we wanted participants to be relatively unrestrained during face-to-face conversations. This came at the cost of lower data quality, especially for the children. Perhaps, if participants would have had more time to practice and get experienced with the dual eye-tracking setup this may have improved data quality. However, this would consequently require more time-investment by both parents, children, and researchers. Researchers who aim to conduct interactive eye-tracking studies with children should be aware of these additional difficulties.
Another point to emphasize is that face-to-face interactions are clearly not the ideal setting for the technical performance of most eye-tracking systems. In the dual eye-tracking setup used in this study, gazing away from the other person’s face could potentially be recorded as gaze directed at the background area (i.e., not looking at the face), but if participants turned their heads away too much from the screen the eye tracker could no longer track participants’ gaze position. Also, a gaze shift back towards the screen after the eye tracker loses track of the gaze position signal does not always coincide with a smooth and instantaneous recovery of the gaze position signal (Hessels et al., 2015 ; Niehorster et al., 2018 ). Such problems occur specifically for remote eye trackers (i.e., eye trackers positioned at a distance from the participant). Other researchers have used wearable eye-tracker systems to study gaze behavior during face-to-face interactions. While wearable eye-trackers do not necessarily suffer from the same problem of losing track of someone’s gaze due to looking at and away from the other person, wearable eye-trackers have different limitations, such as a lack of accuracy to distinguish gaze position on different regions of the face. Also, slippage of the head-worn eye tracker may occur when people are speaking or smiling for example, which can result in a loss of accuracy (Niehorster et al., 2020 ). Such technical limitations thereby constrain what kind of research questions can feasibly be investigated by researchers interested in gaze behavior in interactive situations (for a recent review, see Valtakari et al., 2021 ).
Assuming that some of the technological and methodological limitations of measuring gaze in full-fledged interactions can be overcome (e.g., issues with data quality), how could the use of dual eye-tracking technology benefit future studies on the role of gaze behavior during parent–child interactions? Firstly, a more fine-grained analysis of parents’ and children’s gaze behavior could shed new light on some of the interpersonal dynamics of parent–child relationships. Observational techniques (i.e., manual coding from video-recordings) used to estimate gaze position may lack the reliability and precision to distinguish specific aspects of gaze in interaction, e.g., what regions of the face are looked at and how these are related with other behaviors, such as speaking, listening, and turn-taking. Secondly, many studies have relied heavily on self-reports to assess the parent–child relationship, e.g., parents’ and children’s perceptions about their relationship quality, the intensity and frequency of parent–child conflicts across different ages and stages of development, etc. While studies using self-reports have provided valuable insights into the general structure and relational changes of parent–child interactions (Branje, 2018 ; Mastrotheodoros et al., 2020 ; Smetana, 2011 ), such methods do not directly investigate the behavioral and interpersonal dynamics of parent–child interactions in the ‘heat of the moment’. We think that dual eye-tracking technology, in combination with algorithms to classify face and pose, speech content, and voice affect, could be the key to further understanding parent–child interactions in terms of, for example, parents’ and children’s conversational style and conflict resolution strategies (Beaumont & Wagner, 2004 ; Dixon et al., 2008 ; Thomas et al., 2017 ), as well as individual and interpersonal differences in emotion regulation and social competence (Hutchinson et al., 2019 ; Junge et al., 2020 ; Moed et al., 2015 , 2017 ; Speer et al., 2007 ; Woody et al., 2020 ).
In this study we investigated the role of conflict and cooperation in parent–child interactions. We showed how patterns of speech and gaze behavior (i.e. how much talking was going on, where parents and children looked at each other’s face during interaction) were modulated by topic of conversation (conflict, cooperation) and participant role (speaker, listener). Interpersonal dynamics of the social context were reflected in patterns of speech and gaze behavior, but varied substantially across individuals. Some individuals looked primarily at the eyes or mouth region, while others gazed at different facial features more equally over the course of the conversations. These individual differences were largely consistent across the two conversations, suggesting that individuals also exhibit stable, idiosyncratic face scanning patterns in face-to-face interactions (Arizpe et al., 2017 ; Peterson et al., 2016 ; Rogers et al., 2018 ).
All visual angles in our study are reported under the assumption that participants were seated at approximately 81 cm distance from the screen and in the center of the camera image.
Argyle, M., & Cook, M. (1976). Gaze and mutual gaze . Cambridge University Press.
Google Scholar
Arizpe, J., Walsh, V., Yovel, G., & Baker, C. I. (2017). The categories, frequencies, and stability of idiosyncratic eye-movement patterns to faces. Vision Research, 141 , 191–203. https://doi.org/10.1016/j.visres.2016.10.013
Article PubMed Google Scholar
Arnold, A., Semple, R. J., Beale, I., & Fletcher-Flinn, C. M. (2000). Eye contact in children’s social interactions: What is normal behaviour? Journal of Intellectual and Developmental Disability, 25 (3), 207–216. https://doi.org/10.1080/13269780050144271
Article Google Scholar
Ashear, V., & Snortum, J. R. (1971). Eye contact in children as a function of age, sex, social and intellective variables. Developmental Psychology, 4 (3), 479. https://doi.org/10.1037/h0030974
Aspland, H., & Gardner, F. (2003). Observational measures of parent-child interaction: An introductory review. Child and Adolescent Mental Health, 8 (3), 136–143. https://doi.org/10.1111/1475-3588.00061
Beattie, G. W., & Bogle, G. (1982). The reliability and validity of different video-recording techniques used for analysing gaze in dyadic interaction. British Journal of Social Psychology, 21 (1), 34–35. https://doi.org/10.1111/j.2044-8309.1982.tb00509.x
Beaumont, S. L., & Wagner, S. L. (2004). Adolescent-parent verbal conflict: The roles of conversational styles and disgust emotions. Journal of Language and Social Psychology, 23 (3), 338–368. https://doi.org/10.1177/0261927X04266813
Berger, J., & Cunningham, C. C. (1981). The development of eye contact between mothers and normal versus Down’s syndrome infants. Developmental Psychology, 17 (5), 678. https://doi.org/10.1037/0012-1649.17.5.678
Birmingham, E., Bischof, W. F., & Kingstone, A. (2009). Saliency does not account for fixations to eyes within social scenes. Vision Research, 49 (24), 2992–3000. https://doi.org/10.1016/j.visres.2009.09.014
Branje, S. (2018). Development of parent–adolescent relationships: Conflict interactions as a mechanism of change. Child Development Perspectives, 12 (3), 171–176. https://doi.org/10.1111/cdep.12278
Buchan, J. N., Paré, M., & Munhall, K. G. (2007). Spatial statistics of gaze fixations during dynamic face processing. Social Neuroscience, 2 (1), 1–13. https://doi.org/10.1080/17470910601043644
Buswell, G. T. (1935). How people look at pictures: A study of the psychology and perception in art . Chicago University Press.
Carpendale, J. I., & Lewis, C. (2004). Constructing an understanding of mind: The development of children’s social understanding within social interaction. Behavioral and Brain Sciences, 27 (1), 79–96. https://doi.org/10.1017/S0140525X04000032
Cipolli, C., Sancini, M., Tuozzi, G., Bolzani, R., Mutinelli, P., Flamigni, C., & Porcu, E. (1989). Gaze and eye-contact with anorexic adolescents. British Journal of Medical Psychology, 62 (4), 365–369. https://doi.org/10.1111/j.2044-8341.1989.tb02846.x
De Goede, I. H., Branje, S. J., & Meeus, W. H. (2009). Developmental changes in adolescents’ perceptions of relationships with their parents. Journal of Youth and Adolescence, 38 (1), 75–88. https://doi.org/10.1007/s10964-008-9286-7
Dixon, S. V., Graber, J. A., & Brooks-Gunn, J. (2008). The roles of respect for parental authority and parenting practices in parent-child conflict among African American, Latino, and European American families. Journal of Family Psychology, 22 (1), 1. https://doi.org/10.1037/0893-3200.22.1.1
Article PubMed PubMed Central Google Scholar
Duncan, S., & Fiske, D. W. (2015). Face-to-face interaction: Research, methods, and theory . Routledge. (1977)
Dunn, J., & Slomkowski, C. (1992). Conflict and the development of social understanding. In C. U. Schantz & W. W. Hartup (Eds), Conflict in Child and Adolescent Development (1992). Cambridge University Press.
Ehrlich, K. B., Richards, J. M., Lejuez, C., & Cassidy, J. (2016). When parents and adolescents disagree about disagreeing: Observed parent–adolescent communication predicts informant discrepancies about conflict. Journal of Research on Adolescence, 26 (3), 380–389. https://doi.org/10.1111/jora.12197
Fakkel, M., Peeters, M., Lugtig, P., Zondervan-Zwijnenburg, M. A. J., Blok, E., White, T., & Vollebergh, W. A. M. (2020). Testing sampling bias in estimates of adolescent social competence and behavioral control. Developmental Cognitive Neuroscience, 46, .
Farroni, T., Csibra, G., Simion, F., & Johnson, M. H. (2002). Eye contact detection in humans from birth. Proceedings of the National Academy of Sciences, 99 (14), 9602–9605. https://doi.org/10.1073/pnas.152159999
Foddy, M. (1978). Patterns of gaze in cooperative and competitive negotiation. Human Relations, 31 (11), 925–938. https://doi.org/10.1177/001872677803101101
Foulsham, T., & Sanderson, L. A. (2013). Look who’s talking? Sound changes gaze behaviour in a dynamic social scene. Visual Cognition, 21 (7), 922–944.
Foulsham, T., Walker, E., & Kingstone, A. (2011). The where, what and when of gaze allocation in the lab and the natural environment. Vision Research, 51 (17), 1920–1931. https://doi.org/10.1016/j.visres.2011.07.002
Frank, M. C., Vul, E., & Johnson, S. P. (2009). Development of infants’ attention to faces during the first year. Cognition, 110 (2), 160–170. https://doi.org/10.1016/j.cognition.2008.11.010
Freeth, M., Foulsham, T., & Kingstone, A. (2013). What affects social attention? Social presence, eye contact and autistic traits. PLoS ONE, 8 (1), e53286. https://doi.org/10.1371/journal.pone.0053286
Gauvain, M. (2001). The social context of cognitive development . Guilford Press.
Gliga, T., Elsabbagh, M., Andravizou, A., & Johnson, M. (2009). Faces attract infants’ attention in complex displays. Infancy, 14 (5), 550–562. https://doi.org/10.1080/15250000903144199
Haensel, J. X., Danvers, M., Ishikawa, M., Itakura, S., Tucciarelli, R., Smith, T. J., & Senju, A. (2020). Culture modulates face scanning during dyadic social interactions. Scientific Reports, 10 (1), 1–11. https://doi.org/10.1038/s41598-020-58802-0
Haensel, J. X., Smith, T. J., & Senju, A. (2017). Cultural differences in face scanning during live face-to-face interactions using head-mounted eye-tracking. Journal of Vision, 17 (10), 835–835. https://doi.org/10.1038/s41598-020-58802-0
Hessels, R. S. (2020). How does gaze to faces support face-to-face interaction? A review and perspective. Psychonomic Bulletin & Review , 1-26. https://doi.org/10.3758/s13423-020-01715-w
Hessels, R. S., Benjamins, J. S., Cornelissen, T. H. W., & Hooge, I. T. C. (2018a). A validation of automatically-generated Areas-of-Interest in videos of a face for eye-tracking research. Frontiers in Psychology, 9 , 1367. https://doi.org/10.3389/fpsyg.2018.01367
Hessels, R. S., Benjamins, J. S., van Doorn, A. J., Koenderink, J. J., Holleman, G. A., & Hooge, I. T. C. (2020a). Looking behavior and potential human interactions during locomotion. Journal of Vision, 20 (10), 5–5. https://doi.org/10.1167/jov.20.10.5
Hessels, R. S., Cornelissen, T. H., Hooge, I. T. C., & Kemner, C. (2017). Gaze behavior to faces during dyadic interaction. Canadian Journal of Experimental Psychology/revue Canadienne De Psychologie Expérimentale, 71 (3), 226–242. https://doi.org/10.1037/cep0000113
Hessels, R. S., Cornelissen, T. H. W., Kemner, C., & Hooge, I. T. C. (2015). Qualitative tests of remote eyetracker recovery and performance during head rotation. Behavior Research Methods, 47 (3), 848–859. https://doi.org/10.3758/s13428-014-0507-6
Hessels, R. S., Holleman, G. A., Cornelissen, T. H. W., Hooge, I. T. C., & Kemner, C. (2018b). Eye contact takes two–autistic and social anxiety traits predict gaze behavior in dyadic interaction. Journal of Experimental Psychopathology , 9 (2), jep. 062917. https://doi.org/10.5127/jep.062917
Hessels, R. S., Holleman, G. A., Kingstone, A., Hooge, I. T. C., & Kemner, C. (2019). Gaze allocation in face-to-face communication is affected primarily by task structure and social context, not stimulus-driven factors. Cognition, 184 , 28–43. https://doi.org/10.1016/j.cognition.2018.12.005
Hessels, R. S., & Hooge, I. T. C. (2019). Eye tracking in developmental cognitive neuroscience–The good, the bad and the ugly. Developmental Cognitive Neuroscience, 40 , 100710. https://doi.org/10.1016/j.dcn.2019.100710
Hessels, R. S., Kemner, C., van den Boomen, C., & Hooge, I. T. C. (2016). The area-of-interest problem in eyetracking research: A noise-robust solution for face and sparse stimuli. Behavior Research Methods, 48 (4), 1694–1712. https://doi.org/10.3758/s13428-015-0676-y
Hessels, R. S., van Doorn, A. J., Benjamins, J. S., Holleman, G. A., & Hooge, I. T. C. (2020b). Task-related gaze control in human crowd navigation. Attention, Perception, & Psychophysics , 1-20. https://doi.org/10.3758/s13414-019-01952-9
Ho, S., Foulsham, T., & Kingstone, A. (2015). Speaking and listening with the eyes: Gaze signaling during dyadic interactions. PLoS ONE, 10 (8), e0136905. https://doi.org/10.1371/journal.pone.0136905
Holleman, G. A., Hessels, R. S., Kemner, C., & Hooge, I. T. C. (2019). Eye Tracking During Interactive Face Perception: Does Speech Affect Eye-Tracking Data Quality? European Conference on Visual Perception [ECVP] , Leuven, Belgium.
Holler, J., & Levinson, S. C. (2019). Multimodal language processing in human communication. Trends in Cognitive Sciences, 23 (8), 639–652. https://doi.org/10.1016/j.tics.2019.05.006
Holmqvist, K., Nyström, M., & Mulvey, F. (2012). Eye tracker data quality: What it is and how to measure it. Proceedings of the Symposium on Eye Tracking Research and Applications . https://doi.org/10.1145/2168556.2168563
Hutchinson, E. A., Rosen, D., Allen, K., Price, R. B., Amole, M., & Silk, J. S. (2019). Adolescent gaze-directed attention during parent–child conflict: The effects of depressive symptoms and parent–child relationship quality. Child Psychiatry & Human Development, 50 (3), 483–493. https://doi.org/10.1007/s10578-018-0856-y
Itier, R. J., Villate, C., & Ryan, J. D. (2007). Eyes always attract attention but gaze orienting is task-dependent: Evidence from eye movement monitoring. Neuropsychologia, 45 (5), 1019–1028. https://doi.org/10.1016/j.neuropsychologia.2006.09.004
Jack, R. E., & Schyns, P. G. (2017). Toward a social psychophysics of face communication. Annual Review of Psychology, 68 , 269–297. https://doi.org/10.1146/annurev-psych-010416-044242
Junge, C., Valkenburg, P. M., Deković, M., & Branje, S. (2020). The building blocks of social competence: Contributions of the Consortium of Individual Development. Developmental Cognitive Neuroscience, 45 , 100861. https://doi.org/10.1016/j.dcn.2020.100861
Kanan, C., Bseiso, D. N., Ray, N. A., Hsiao, J. H., & Cottrell, G. W. (2015). Humans have idiosyncratic and task-specific scanpaths for judging faces. Vision Research, 108 , 67–76. https://doi.org/10.1016/j.visres.2015.01.013
Kelly, S. D., Özyürek, A., & Maris, E. (2010). Two sides of the same coin: Speech and gesture mutually interact to enhance comprehension. Psychological Science, 21 (2), 260–267. https://doi.org/10.1177/0956797609357327
Kendon, A. (1967). Some functions of gaze-direction in social interaction. Acta Psychologica, 26 , 22–63. https://doi.org/10.1016/0001-6918(67)90005-4
Kleinke, C. L. (1986). Gaze and eye contact: A research review. Psychological Bulletin, 100 (1), 78. https://doi.org/10.1037/0033-2909.100.1.78
Laidlaw, K. E., Foulsham, T., Kuhn, G., & Kingstone, A. (2011). Potential social interactions are important to social attention. Proceedings of the National Academy of Sciences, 108 (14), 5548–5553. https://doi.org/10.1073/pnas.1017022108
Laursen, B., & Collins, W. A. (2004). Parent-child communication during adolescence. The Routledge Handbook of Family Communication, 2 , 333–348.
Laursen, B., & Collins, W. A. (2009). Parent-child relationships during adolescence. In R. M. Lerner & L. Steinberg (Eds.), Handbook of Adolescent Psychology: Contextual Influences on Adolescent Development (pp. 3–42). John Wiley & Sons, Inc. https://doi.org/10.1002/9780470479193.adlpsy002002
Laursen, B., & Hafen, C. A. (2010). Future directions in the study of close relationships: Conflict is bad (except when it’s not). Social Development, 19 (4), 858–872. https://doi.org/10.1111/j.1467-9507.2009.00546.x
Levine, M. H., & Sutton-Smith, B. (1973). Effects of age, sex, and task on visual behavior during dyadic interaction. Developmental Psychology, 9 (3), 400. https://doi.org/10.1037/h0034929
Mastrotheodoros, S., Van der Graaff, J., Deković, M., Meeus, W. H., & Branje, S. (2020). Parent–adolescent conflict across adolescence: Trajectories of informant discrepancies and associations with personality types. Journal of Youth and Adolescence, 49 (1), 119–135. https://doi.org/10.1007/s10964-019-01054-7
McGurk, H., & MacDonald, J. (1976). Hearing lips and seeing voices. Nature, 264 (5588), 746–748. https://doi.org/10.1038/264746a0
Mehoudar, E., Arizpe, J., Baker, C. I., & Yovel, G. (2014). Faces in the eye of the beholder: Unique and stable eye scanning patterns of individual observers. Journal of Vision, 14 (7), 6–6. https://doi.org/10.1167/14.7.6
Mirenda, P. L., Donnellan, A. M., & Yoder, D. E. (1983). Gaze behavior: A new look at an old problem. Journal of Autism and Developmental Disorders, 13 (4), 397–409. https://doi.org/10.1007/BF01531588
Moed, A., Dix, T., Anderson, E. R., & Greene, S. M. (2017). Expressing negative emotions to children: Mothers’ aversion sensitivity and children’s adjustment. Journal of Family Psychology, 31 (2), 224. https://doi.org/10.1037/fam0000239
Moed, A., Gershoff, E. T., Eisenberg, N., Hofer, C., Losoya, S., Spinrad, T. L., & Liew, J. (2015). Parent–adolescent conflict as sequences of reciprocal negative emotion: Links with conflict resolution and adolescents’ behavior problems. Journal of Youth and Adolescence, 44 (8), 1607–1622. https://doi.org/10.1007/s10964-014-0209-5
Niehorster, D. C., Cornelissen, T. H. W., Holmqvist, K., Hooge, I. T. C., & Hessels, R. S. (2018). What to expect from your remote eye-tracker when participants are unrestrained. Behavior Research Methods, 50 (1), 213–227. https://doi.org/10.3758/s13428-017-0863-0
Niehorster, D. C., Santini, T., Hessels, R. S., Hooge, I. T., Kasneci, E., & Nyström, M. (2020). The impact of slippage on the data quality of head-worn eye trackers. Behavior Research Methods , 1-21. https://doi.org/10.3758/s13428-019-01307-0
Onland-Moret, N. C., Buizer-Voskamp, J. E., Albers, M. E., Brouwer, R. M., Buimer, E. E., Hessels, R. S., . . . Mandl, R. C. (2020). The YOUth study: rationale, Design, and study procedures. Developmental Cognitive Neuroscience , 100868. https://doi.org/10.1016/j.dcn.2020.100868
Patterson, M. L. (1982). A sequential functional model of nonverbal exchange. Psychological Review, 89 (3), 231. https://doi.org/10.1037/0033-295X.89.3.231
Peterson, M. F., & Eckstein, M. P. (2013). Individual differences in eye movements during face identification reflect observer-specific optimal points of fixation. Psychological Science, 24 (7), 1216–1225. https://doi.org/10.1177/0956797612471684
Peterson, M. F., Lin, J., Zaun, I., & Kanwisher, N. (2016). Individual differences in face-looking behavior generalize from the lab to the world. Journal of Vision, 16 (7), 12–12. https://doi.org/10.1167/16.7.12
Risko, E. F., Laidlaw, K., Freeth, M., Foulsham, T., & Kingstone, A. (2012). Social attention with real versus reel stimuli: Toward an empirical approach to concerns about ecological validity. Frontiers in Human Neuroscience, 6 , 143. https://doi.org/10.3389/fnhum.2012.00143
Risko, E. F., Richardson, D. C., & Kingstone, A. (2016). Breaking the fourth wall of cognitive science: Real-world social attention and the dual function of gaze. Current Directions in Psychological Science, 25 (1), 70–74. https://doi.org/10.1177/0963721415617806
Rogers, S. L., Speelman, C. P., Guidetti, O., & Longmuir, M. (2018). Using dual eye tracking to uncover personal gaze patterns during social interaction. Scientific Reports, 8 (1), 1–9. https://doi.org/10.1038/s41598-018-22726-7
Rossano, F., Brown, P., & Levinson, S. C. (2009). Gaze, questioning and culture. In J. Sidnell (Ed.), Conversation Analysis: Comparative Perspectives, 27 (pp. 187–249). Cambridge University Press.
Chapter Google Scholar
Rousselet, G. A., Pernet, C. R., & Wilcox, R. R. (2017). Beyond differences in means: Robust graphical methods to compare two groups in neuroscience. European Journal of Neuroscience, 46 (2), 1738–1748. https://doi.org/10.1111/ejn.13610
Rubo, M., Huestegge, L., & Gamer, M. (2020). Social anxiety modulates visual exploration in real life–but not in the laboratory. British Journal of Psychology, 111 (2), 233–245. https://doi.org/10.1111/bjop.12396
Schofield, T. J., Parke, R. D., Castaneda, E. K., & Coltrane, S. (2008). Patterns of gaze between parents and children in European American and Mexican American families. Journal of Nonverbal Behavior, 32 (3), 171–186. https://doi.org/10.1007/s10919-008-0049-7
Scott, S., Briskman, J., Woolgar, M., Humayun, S., & O’Connor, T. G. (2011). Attachment in adolescence: Overlap with parenting and unique prediction of behavioural adjustment. Journal of Child Psychology and Psychiatry, 52 (10), 1052–1062. https://doi.org/10.1111/j.1469-7610.2011.02453.x
Smetana, J. G. (2011). Adolescents’ social reasoning and relationships with parents: Conflicts and coordinations within and across domains. In E. Amsel & J. Smetana (Eds.), Adolescent Vulnerabilities and Opportunities: Constructivist and Developmental Perspectives (pp. 139–158). Cambridge University Press.
Smokowski, P. R., Bacallao, M. L., Cotter, K. L., & Evans, C. B. (2015). The effects of positive and negative parenting practices on adolescent mental health outcomes in a multicultural sample of rural youth. Child Psychiatry & Human Development, 46 (3), 333–345. https://doi.org/10.1007/s10578-014-0474-2
Speer, L. L., Cook, A. E., McMahon, W. M., & Clark, E. (2007). Face processing in children with autism: Effects of stimulus contents and type. Autism, 11 (3), 265–277. https://doi.org/10.1177/1362361307076925
Steinberg, L. (2001). We know some things: Parent–adolescent relationships in retrospect and prospect. Journal of Research on Adolescence, 11 (1), 1–19. https://doi.org/10.1111/1532-7795.00001
Stivers, T., Enfield, N. J., Brown, P., Englert, C., Hayashi, M., Heinemann, T., . . . Yoon, K.-E. (2009). Universals and cultural variation in turn-taking in conversation. Proceedings of the National Academy of Sciences , 106 (26), 10587-10592. https://doi.org/10.1073/pnas.0903616106
Sumby, W. H., & Pollack, I. (1954). Visual contribution to speech intelligibility in noise. The Journal of the Acoustical Society of America, 26 (2), 212–215. https://doi.org/10.1121/1.1907309
Thomas, S. A., Wilson, T., Jain, A., Deros, D. E., Um, M., Hurwitz, J., . . . Dunn, E. J. (2017). Toward developing laboratory-based parent–adolescent conflict discussion tasks that consistently elicit adolescent conflict-related stress responses: Support from physiology and observed behavior. Journal of Child and Family Studies , 26 (12), 3288-3302. https://doi.org/10.1007/s10826-017-0844-z
Timney, B., & London, H. (1973). Body language concomitants of persuasiveness and persuasibility in dyadic interaction. International Journal of Group Tensions, 3 (3–4), 48–67.
Tucker, C. J., McHale, S. M., & Crouter, A. C. (2003). Conflict resolution: Links with adolescents’ family relationships and individual well-being. Journal of Family Issues, 24 (6), 715–736. https://doi.org/10.1177/0192513X03251181
Valtakari, N. V., Hooge, I. T., Viktorsson, C., Nyström, P., Falck-Ytter, T., & Hessels, R. S. (2021). Eye tracking in human interaction: Possibilities and limitations. Behavior Research Methods , 1-17. https://doi.org/10.3758/s13428-020-01517-x
Vatikiotis-Bateson, E., Eigsti, I.-M., Yano, S., & Munhall, K. G. (1998). Eye movement of perceivers during audiovisualspeech perception. Perception & Psychophysics, 60 (6), 926–940. https://doi.org/10.3758/BF03211929
Võ, M.L.-H., Smith, T. J., Mital, P. K., & Henderson, J. M. (2012). Do the eyes really have it? Dynamic allocation of attention when viewing moving faces. Journal of Vision, 12 (13), 3–3. https://doi.org/10.1167/12.13.3
Woody, M. L., Price, R. B., Amole, M., Hutchinson, E., Benoit Allen, K., & Silk, J. S. (2020). Using mobile eye-tracking technology to examine adolescent daughters’ attention to maternal affect during a conflict discussion. Developmental Psychobiology . https://doi.org/10.1002/dev.22024
Yarbus, A. L. (1967). Eye movements during perception of complex objects. In Eye Movements and Vision (pp. 171–211). Springer.
Download references
Author information
Authors and affiliations.
Department of Experimental Psychology, Helmholtz Institute, Utrecht University, Utrecht, The Netherlands
Gijs A. Holleman, Ignace T. C. Hooge, Chantal Kemner & Roy S. Hessels
Department of Clinical Child and Family Studies, Utrecht University, Utrecht, The Netherlands
Jorg Huijding & Maja Deković
You can also search for this author in PubMed Google Scholar
Corresponding author
Correspondence to Gijs A. Holleman .
Ethics declarations
All the authors declare that they have no conflict of interest. All the procedures performed in this study were in accordance with the ethical standards of the institutional and/or national research committee and with the 1964 Helsinki Declaration and its later amendments or comparable ethical standards. This study was approved by the Medical Research Ethics Committee of the University Medical Center Utrecht and is registered under protocol number 19–051/M. Informed consent was obtained from all participants included in the study (parents provided written informed consent for themselves as well as on behalf of their children).
Data Availability
The data that support the findings of this study are available on reasonable request from the corresponding author GH. The data are not publicly available due to restrictions on their containing information that could compromise the privacy of research participants.
Additional information
Publisher’s note.
Springer Nature remains neutral with regard to jurisdictional claims in published maps and institutional affiliations.
Appendix 1: Conversation Topics
Transportation, using bicycle or car
Money or valuable things
Not being at home, or being too much at home
Table manners, being rude
Screen time, TV, computer, phone
Behavior in class or at school
School performance
Different opinions
Future plans
Gossip, secrets
Being annoying
Associating with others
Being on time, cancelling appointments
Leisure time
(Not) doing something when asked
Cleaning room
Appearance, clothing
School grades
Being honest
Household chores
(Not) sharing problems
Appendix 2: Three Types of Data Loss
Data loss is a problem in eye-tracking research and in our study it was expected due to relatively unrestrained behavior of the participants. Here we are interested in what were the most important sources of data loss, and how they were related to our main analyses. We identified at least three types of data loss in our study, namely: 1) samples with no eye-tracking data (i.e. no gaze position recorded), 2) samples with no AOI labels assigned to all video frames (i.e. no AOI data), and 3) samples that did not count as a dwell (i.e. shorter than 120 ms). As is visible from Fig. 7 , we found that missing eye-tracking data (i.e. no gaze position recorded) was the most prominent source of data loss in our study. Moreover, we found that total amount of time without eye-tracking data was much higher for children than parents. This may explain why relative total dwell times to the facial and background AOIs were generally higher for parents than for children (see 10 , Section 14 ).
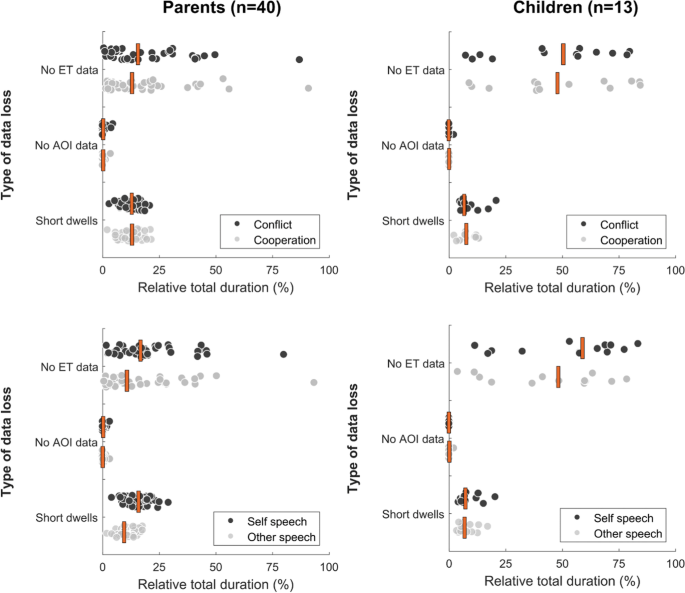
Three types of data loss: no ET data, no AOI data, and short dwells. Left panels depict data loss measures for the parents and right panels for the children. Relative total duration (as a percentage) without eye-tracking data (i.e. no gaze position recorded), without AOI data, or with dwells shorter than 120 ms (i.e. invalid dwells) are depicted on the x-axis. Upper panels depict data loss measures as a function of conversation-scenario (conflict and cooperation). Lower panels depict data loss measures as a function of speaker-state (self-speech and other-speech). Each marker represents data from one participant. The vertical orange stripes represent the median relative total dwell time per data loss type
Appendix 3: Data Loss Exclusion Criteria Does Not Affect Main Findings
As stated, we expected that eye-tracking data loss was especially likely to occur while speaking. Therefore, we did not exclude participants based on a general data loss criterion, as this may selectively exclude participants that spoke relatively more. Therefore, we needed to validate that not excluding participants based on a data loss criterion did not affect our main findings. In this section, we present two separate sensitivity analyses for two of our main findings.
The first sensitivity analysis pertains to our finding that parents looked more at the eyes AOI during the conflict-scenario. We validated whether this finding was not affected by exclusion of participants based on a data loss criterion. Figure 8 depicts an outcome measure as a function of the data loss criterion. The data loss criterion used here was the percentage of time during listening without eye-tracking data, as this does not include data loss due to excessive looking away (as in speaking). On the y-axis the percentage point difference in total dwell time at the eyes AOI is depicted. On the x-axis a data loss criterion is depicted (i.e. as a % of missing ET data). As is visible from this figure, the positive median percentage point difference in looking at the eyes AOI between conflict and cooperation (see 10 , Section 14 ) was not affected by a data loss criterion, as its median and 95% confidence intervals were consistently above zero. In other words, the direction of the percentage point difference is not related to the data loss exclusion criterion. Thus, the finding that parents looked more at their children’s eyes in the conflict conversation does not depend on whether we exclude participants based on data loss.
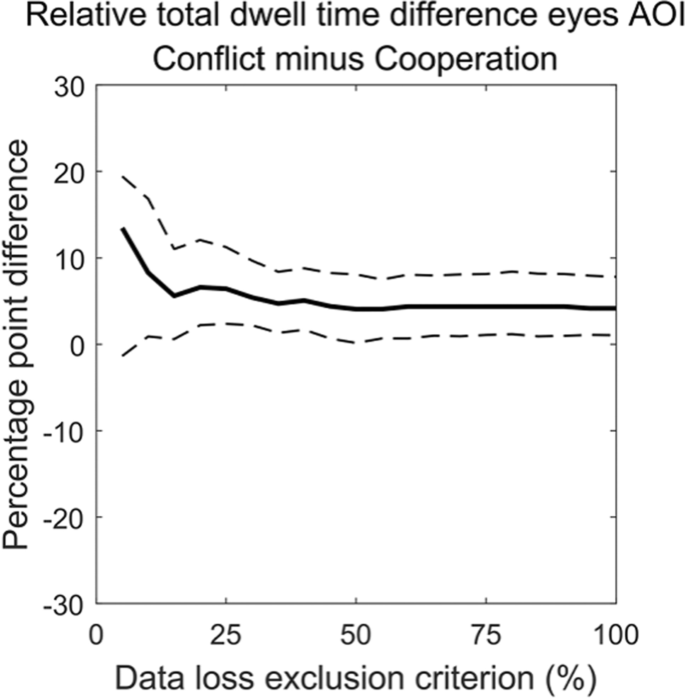
Relative total dwell time difference to the eyes AOI between conflict and cooperation scenario. In this case, we validated whether the positive median percentage point difference in parents’ total dwell time to the eyes AOI was affected by data loss. Eye-tracking data loss was operationalized as the relative total duration during which no eye-tracking data was recorded. Participants were excluded if data loss exceeded the value on the x-axis for both conflict and cooperation conversations. Lower values of the data loss exclusion criterion represent a stricter exclusion policy (at 0% all participants are excluded and at 100% no participants are excluded). The solid black line represents the median percentage point difference, and the dashed black lines represent the 95% confidence intervals of the median, both of which were obtained through bootstrapping using the MATLAB-function decilespcbi provided by Rousselet et al. ( 2017 )
The second sensitivity analysis pertains to our finding that both parents and children looked more at the mouth AOI during episodes of other-speech. We validated whether this finding was not affected by exclusion based on a data loss criterion. Figure 9 depicts the percentage point difference in total dwell time to the mouth AOI between self-speech and other-speech episodes for the parents (left panel) and children (right panel) as a function of the same data loss exclusion criterion. On the y-axis the percentage point difference in total dwell time at the mouth AOI is depicted. On the x-axis a data loss criterion is depicted (i.e. as a percentage of missing ET data). As is visible from this figure, the negative median percentage point difference in looking at the mouth AOI between self-speech and other-speech (see 10 , Section 14 ) was not affected by a data loss criterion, as its median and 95% confidence intervals were consistently below zero. Thus, the direction of the percentage point difference is not related to the data loss exclusion criterion. Thus, the finding that participants looked more at the other’s mouth during other-speech does not depend on whether we exclude participants based on data loss.
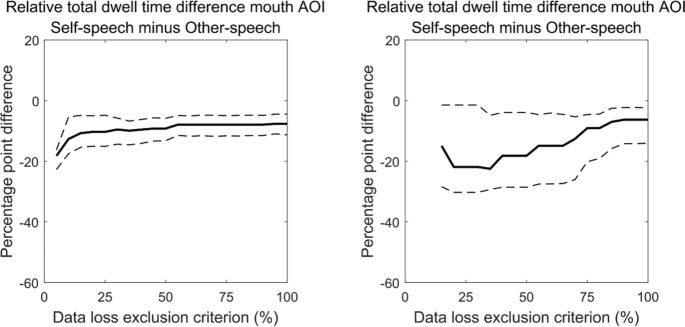
Relative total dwell time difference to the mouth AOI between self-speech and other-speech. In this case, we validated whether the negative median percentage point difference in parents’ (left panel) and children’s (right panel) relative total dwell time to the mouth was affected by data loss. Eye-tracking data loss was operationalized as the relative total duration during which no eye-tracking data was recorded during periods of other-speech. Participants were excluded if the eye-tracking data loss during periods of other-speech exceeded the value on the x-axis (eye-tracking data loss was averaged over the two conversations). Lower values of the data loss exclusion criterion represent a stricter exclusion policy (at 0% all participants are excluded and at 100% no participants are excluded). The solid black line represents the median percentage point difference, and the dashed black lines represent the 95% confidence intervals of the median, both of which were obtained through bootstrapping using the MATLAB-function decilespcbi provided by Rousselet et al. ( 2017 )
Rights and permissions
Open Access This article is licensed under a Creative Commons Attribution 4.0 International License, which permits use, sharing, adaptation, distribution and reproduction in any medium or format, as long as you give appropriate credit to the original author(s) and the source, provide a link to the Creative Commons licence, and indicate if changes were made. The images or other third party material in this article are included in the article's Creative Commons licence, unless indicated otherwise in a credit line to the material. If material is not included in the article's Creative Commons licence and your intended use is not permitted by statutory regulation or exceeds the permitted use, you will need to obtain permission directly from the copyright holder. To view a copy of this licence, visit http://creativecommons.org/licenses/by/4.0/ .
Reprints and permissions
About this article
Holleman, G.A., Hooge, I.T.C., Huijding, J. et al. Gaze and speech behavior in parent–child interactions: The role of conflict and cooperation. Curr Psychol 42 , 12129–12150 (2023). https://doi.org/10.1007/s12144-021-02532-7
Download citation
Accepted : 14 November 2021
Published : 02 December 2021
Issue Date : May 2023
DOI : https://doi.org/10.1007/s12144-021-02532-7
Share this article
Anyone you share the following link with will be able to read this content:
Sorry, a shareable link is not currently available for this article.
Provided by the Springer Nature SharedIt content-sharing initiative
- Dual eye-tracking
- Parent–child interaction
- Cooperation
- Find a journal
- Publish with us
- Track your research
Thank you for visiting nature.com. You are using a browser version with limited support for CSS. To obtain the best experience, we recommend you use a more up to date browser (or turn off compatibility mode in Internet Explorer). In the meantime, to ensure continued support, we are displaying the site without styles and JavaScript.
- View all journals
- My Account Login
- Explore content
- About the journal
- Publish with us
- Sign up for alerts
- Open access
- Published: 18 July 2019
Speech, movement, and gaze behaviours during dyadic conversation in noise
- Lauren V. Hadley 1 ,
- W. Owen Brimijoin 1 &
- William M. Whitmer ORCID: orcid.org/0000-0001-8618-6851 1
Scientific Reports volume 9 , Article number: 10451 ( 2019 ) Cite this article
4258 Accesses
41 Citations
8 Altmetric
Metrics details
- Behavioural ecology
- Human behaviour
How do people have conversations in noise and make themselves understood? While many previous studies have investigated speaking and listening in isolation, this study focuses on the behaviour of pairs of individuals in an ecologically valid context. Specifically, we report the fine-grained dynamics of natural conversation between interlocutors of varying hearing ability (n = 30), addressing how different levels of background noise affect speech, movement, and gaze behaviours. We found that as noise increased, people spoke louder and moved closer together, although these behaviours provided relatively small acoustic benefit (0.32 dB speech level increase per 1 dB noise increase). We also found that increased noise led to shorter utterances and increased gaze to the speaker’s mouth. Surprisingly, interlocutors did not make use of potentially beneficial head orientations. While participants were able to sustain conversation in noise of up to 72 dB, changes in conversation structure suggested increased difficulty at 78 dB, with a significant decrease in turn-taking success. Understanding these natural conversation behaviours could inform broader models of interpersonal communication, and be applied to the development of new communication technologies. Furthermore, comparing these findings with those from isolation paradigms demonstrates the importance of investigating social processes in ecologically valid multi-person situations.
Similar content being viewed by others
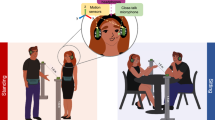
Behavioral dynamics of conversation, (mis)communication and coordination in noisy environments
Kelly Miles, Adam Weisser, … Joerg M. Buchholz
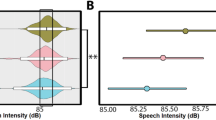
Speakers exhibit a multimodal Lombard effect in noise
James Trujillo, Asli Özyürek, … Linda Drijvers
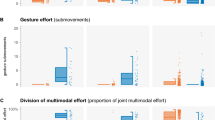
The multimodal nature of communicative efficiency in social interaction
Marlou Rasenberg, Wim Pouw, … Mark Dingemanse
Introduction
Taking part in a conversation is a complex task that requires individuals to both comprehend the speech of a partner, and produce their own comprehensible speech. Conversing effectively requires quick alternation between these processes, with the intervals between turns (i.e. between playing a listening and a speaking role) often being under 250 ms 1 . The challenge is increased in noisy environments such as cafés or restaurants, which tax interdependent sensory and cognitive skills 2 . These situations are particularly demanding for people with hearing impairment (HI), and they often shun environments in which they may fail to keep up 3 . However, speakers and listeners can draw on a variety of behavioural strategies to aid communication in such environments. In this paper we investigate the strategies individuals use spontaneously when conversing with a partner in noise, in order to identify the strategies that are spontaneously employed to facilitate communication in such contexts.
In a conversation, the speaker’s aim is to convey information in an intelligible manner. Previous studies of talking in noise have shown that speakers do this by modifying the acoustic parameters of their speech, and their speech patterns, both when producing speech in isolation and when speaking in conversation. In terms of acoustic parameters, speakers in noisy environments increase their vocal intensity and adjust the spectrum of their speech 4 , 5 , which improve intelligibility for listeners 5 , 6 . In terms of speech patterns, speakers in noisy environments produce longer utterances 7 and speak the utterances that they do produce more slowly (i.e., producing fewer syllables per second), thereby giving listeners more time to process spoken information 8 , 9 . Finally, it has been shown that in noise, speakers include more (and potentially longer) pauses 8 . While this could reflect a strategic adjustment to aid listener processing (and has been interpreted as such), it could alternatively be the result of missed turn-switches.
The listener’s aim in a conversation, on the other hand, is to comprehend the speaker’s message. This is facilitated by being able to hear the speaker better, or by receiving additional, non-auditory, cues conveying message content. To hear the speaker better, listeners can orient their ear to increase signal strength 10 , 11 , with best results when they turn 30 degrees away from the sound source 12 . Indeed listeners with unilateral hearing impairment have been found to adjust their head to increase the speech signal in adverse listening conditions, though they may be particularly aware of the impact of orientation on their hearing 13 . While highly variable, even normal hearing listeners have been shown to adjust their head movements when speech becomes difficult to hear 12 , though this may be reduced by competing visual information 11 , and few listeners reach an optimal orientation 12 , 13 , 14 . Decreasing the distance between interlocutors would also allow a listener to better hear a speaker by increasing the signal-to-noise ratio. In terms of non-auditory cues, listeners show remarkable consistency in directing their gaze toward an active speaker 15 , 16 , and experience benefit from seeing them while they talk. For example, seeing a speaker’s head movement improves speech intelligibility 17 , while seeing their lip movements improves both speech intelligibility 18 , 19 and speech detection 20 . Hence visual cues provide valuable additional information for processing speech successfully.
It is clear that a variety of strategies are available to the speaker and listener experiencing difficulty in noisy environments. But strikingly, the majority of studies investigating speaking and listening strategies have removed the social context in which these behaviours most often occur. Studies taking this isolationist approach involve speakers producing scripted utterances for a microphone in an otherwise empty room 4 , 6 , 9 , and listeners being presented with such pre-recorded speech in a similarly desolate context 6 , 12 , 13 , 17 , 18 , 20 , 21 . It is notable that the behaviours that are used to facilitate interpersonal understanding are investigated in isolated, offline, paradigms. While such work provides insight into the strategies that people can use to facilitate speaking and listening in noisy environments, it is yet to be determined whether such strategies are used spontaneously in an interactive context. Several studies attempting to address this question have used multi-person paradigms with highly constrained information-sharing 5 , 7 , 8 , 19 , and have often focused on only one modality of behaviour, removing the possibility of investigating how strategies occur in combination.
In line with the broader shift toward addressing interaction using ecologically valid contexts that involve mutual adaptation 22 , 23 , we investigate dyadic conversation behaviour in dyads approximately matched in age and hearing loss, in noisy environments. Specifically, we focus on the speech, head movement, and gaze behaviours of people with varying hearing ability conversing without hearing aids in speech-shaped background noise fluctuating between 54 dB and 78 dB (see Fig. 1 ). We hypothesise that in higher levels of noise, speakers will increase their speech level and utterance duration, as well as increasing the duration of pauses between turns. We also hypothesise that listeners will orient their head to improve reception of the auditory signal (even if they do not reach the optimal 30 degree orientation), and that they will increase their gaze toward the talker - specifically the talker’s mouth. Finally, we anticipate that individuals will move toward each other to optimise exchange of information. By investigating the broad array of behaviours that HI individuals use while holding real conversations in different levels of noise, we extend prior work on individual speaking and listening to an interactive setting, and address how multiple strategies are used concurrently.
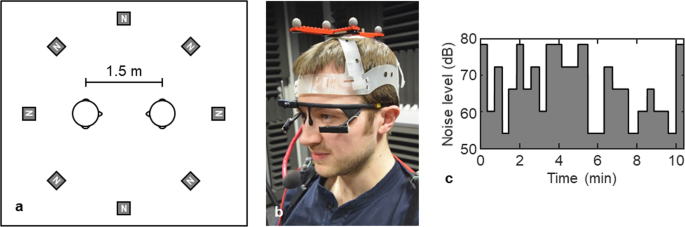
Experimental set-up (example of a non-participating individual). Panel a shows the participant setup within the sound attenuated room, showing the loudspeakers (N) presenting noise throughout each trial. Panel b shows the equipment setup including motion tracking crown, eye-tracking glasses and microphone. Panel c shows an example of the noise levels (54–78 dB in 6 dB increments) as a function of time during an example conversation trial.
Average speech level significantly increased as noise level increased (F(2.075,60.182) = 271.72, p < 0.001, ηp2 = 0.90). Participants spoke on average 1.9 dB more loudly with each 6 dB increase in noise; i.e. they increased vocal level by 0.31 dB per 1 dB noise level increase (see Fig. 2a ). Increasing noise level also led to significantly shorter utterances, (F(1.85,53.52) = 5.48, p = 0. 0.008, ηp2 = 0.16; see Fig. 2b ), and significantly shorter median inter-speaker pauses (F(2.48,34.76) = 7.37, p = 0.001, ηp2 = 0.35, see Fig. 2c ). The overall mean of these inter-speaker pauses was 247 ms, comparable to previous turn-taking results for English speakers (236 ms) 1 .
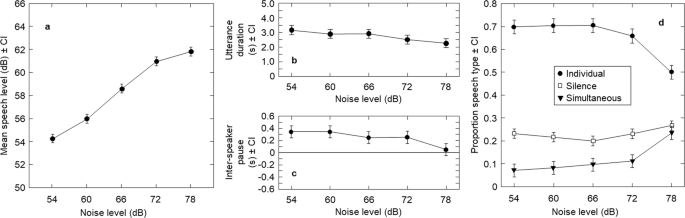
Speech adjustments by noise level. Panel a shows mean speech level by noise level. Panel b shows utterance duration by noise level, and panel c shows inter-speaker pause duration by noise level. Panel d shows proportion of time for individual speech, overlapping speech, and silence, by noise level. All error bars show 95% within-subject confidence intervals.
Noise level also affected conversational structure, showing an interaction with speech type – i.e., individual or overlapping (F(1.40,19.56) = 31.38, p < 0.001). There was a significant effect of noise level on both types of speech (individual: F(1.55,21.64) = 33.54, p < 0.001, ηp2 = 0.71; overlapping: F(1.36,19.03) = 22.10, p < 0.001, ηp2 = 0.61). Pairwise comparisons showed that that in comparison to all quieter levels, when background noise was at its loudest there was a lower proportion of individual speech ( p s < 0.001), alongside a higher proportion of speech overlap ( p s < 0.007). See Fig. 2d .
In terms of head position, interlocutors moved toward each other with increasing noise level (F(1.39,19.42) = 8.71, p = 0.004, ηp2 = 0.38; see Fig. 3a ). On average, interlocutors decreased interpersonal distance by 10 mm for each 6 dB noise increase, equivalent to a 0.01 dB speech level increase per 1 dB noise level increase. Interlocutors showed a mean head angle of +2.1° from centre across conditions, indicating a slight turn of the left ear towards the partner, and listeners’ variability was affected by noise level (F(3.00,87.10) = 2.93, p = 0.04, ηp2 = 0.09; see Fig. 3c ), with post-hoc tests showing a marginal increase between 54 dB and 78 dB ( p = 0.07).
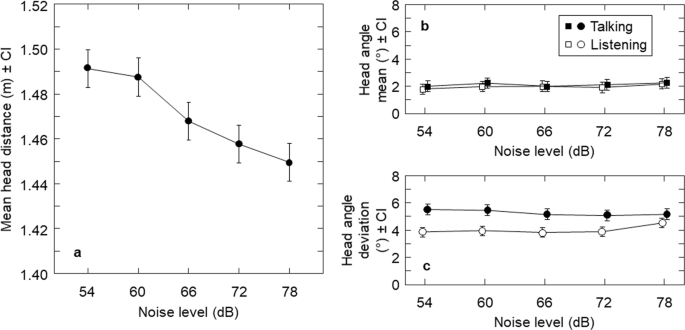
Movement adjustments by noise level. Panel a shows interpersonal distance by noise level. Panel b shows head (yaw) angle means, and panel c shows head (yaw) angle standard deviations, during periods of talking and listening by noise level. All error bars show 95% within-subject confidence intervals.
Listeners focused on their partner’s face (defined as 10° above to 10° below the height of the tragi, with a horizontal span of 20°) for an average of 88% of each trial (see Fig. 4 ). People spent a different proportion of time focused on the mouth (10° zone below the tragi) compared to the eyes (10° zone above the tragi) (F(1,116) = 8.38, p = 0.007, ηp2 = 0.22), and how much time they spent attending to the mouth vs eyes varied by noise level (F(4,116) = 11.92, p < 0.001, ηp2 = 0.29). As noise level increased, participants spent less time focused on their partner’s eyes (F(4,116) = 13.70, p < 0.001, ηp2 = 0.32) and more time focused on their partner’s mouth (F(4,116) = 7.38; p < 0.001, ηp2 = 0.20).
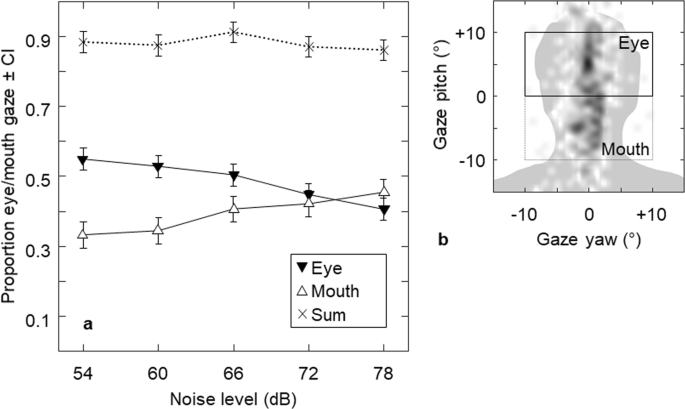
Gaze adjustments by noise level. Panel a shows proportion of listening time spent oriented toward the eye region, the mouth region, and the sum of the two, by noise level. Error bars show 95% within-subject confidence intervals. Panel b shows an example gaze pattern, with darker areas indicating more gaze time to illustrate how the gaze data was split into eye and mouth regions.
Combination
If interlocutors start with an interpersonal distance of 1.5 m, and with each 6 dB noise increase they move 10 mm closer as well as speaking 1.9 dB louder. The combined acoustic benefit of these strategies amounts to 0.32 dB per 1 dB noise increase.
In this study, we measured speech parameters (such as speech level, turn duration, and inter-speaker pause), head movement, and gaze, to comprehensively investigate the strategies spontaneously used by individuals holding conversations in noisy environments. We have shown that while individuals employ potentially beneficial strategies during increased background noise (i.e. by increasing speech level and decreasing interpersonal distance), these adjustments only partially compensate for the increase in noise level. Indeed such behaviours amount to only 0.32 dB benefit per 1 dB noise increase. Other potentially beneficial strategies included using slightly shorter utterances, and increasing looks to the speaker’s mouth. While conversation structure remained constant until the noise level reached 72 dB, with minimal speech overlap and a high proportion of individual speech, a significant increase of overlapping speech at 78 dB suggests that at this level such strategies were not enough to avoid the turn-taking structure of conversation breaking down.
These findings demonstrate that the strategies people use during an interactive conversation are not the same as those used when speaking or listening in an empty laboratory, or even during an interactive task if it is highly constrained. For example, we did not find speakers to increase utterance duration with noise (which could indicate slower speech), as found in the interaction study of Beechey et al . 7 . Several possibilities could explain this difference. It is notable that the task given to our participants was relatively free, in comparison to a path-finding task, and so they may have chosen to change the content of their speech as opposed to slowing their production rate. Furthermore, Beechey et al . varied noise level with simulated environment, and these environments changed between, rather than within, trials. This design may have led participants to employ different strategies depending on the environment, rather than adjusting their use of strategies depending on noise level. The interesting prospect that strategy adjustment is based on noise level, while strategy selection is based on other parameters of the background noise, should be tested systematically in future.
Our data also showed inter-speaker pauses to shorten rather than lengthen. While shorter utterances may have simplified information processing for the listener, increasing pause duration would have provided further benefit. However, it is possible that prior findings of increased pausing in noise are a result of turn-switch difficulties, as opposed to being a strategy used to facilitate listener processing. Finally, we saw no use of head orientation to improve audibility, and report small changes in speech level and interpersonal distance. We suggest that this is because during an interactive conversation, interlocutors must deal with two conflicting goals: (1) facilitating communication, and (2) facilitating interpersonal connection. While strategies to achieve goal 1 have been addressed using isolation paradigms, goal 2 may mediate these strategies as well as eliciting other, purely social, behaviours. Hence interactive paradigms are essential to better understand natural conversation behaviours.
It is likely that while many behaviours reported in this study were used with the goal of improving communication, they may have been modified according to the social situation. For example, interlocutors did speak louder and move towards each other, but not enough to compensate for the background noise increase. Such apparent inefficiency could relate to the social inappropriateness of shouting to a conversation partner or invading another individual’s space. Head orientation strategies may have been avoided for similar reasons; since the optimal head orientation for audibility is 30° 12 , requiring listeners to turn their head somewhat away from their partner, it is possible that social constraints led individuals to avoid adjusting their head orientation. Alternatively, individuals may not have been aware of the SNR benefits of this strategy, and it is possible that with the noise surrounding the listeners any changes in speech-to-noise ratio elicited by re-orientation were not noticeable 24 . It should be noted, however, that listeners did increase their looks toward their partner’s mouth in higher background noise levels, potentially indicating prioritisation of the visual cues gained by looking directly to the mouth over the acoustic cues provided by turning the head.
While attempting to provide an ecologically valid conversation experience, the experimental situation may also have somewhat affected strategy use. The restriction that participants should not move the position of their chairs may have contributed to their minimal movement toward each other (although notably, chairs are often fixed in position). In addition, the use of speech-shaped noise may have masked the partner’s speech more strongly in the temporal domain than typical noises experienced in the background of everyday life (e.g., competing speech exhibiting envelope dips), reducing benefit from strategy use. Finally, the fact that conversing participants did not initially know each other may have impacted their behaviour; individuals may use different/better compensatory behaviours during conversations with familiar than unfamiliar partners. Yet while individuals may be comfortable to verbalise their difficulty when talking to familiar partners, it is perhaps most critical to understand what they do in situations when they are not; indeed daily life is full of conversations with unfamiliar interlocutors: from the postman to the barista. As it is clear that the behaviours that individuals spontaneously use while conversing in noise do not provide a high level of acoustic benefit, further work could investigate whether training could be implemented to allow individuals to take advantage of potentially useful strategies (such as learning to orient the head for maximal signal-to-noise benefit).
Future work could also begin addressing how conversation behaviours differ depending on the type of background noise, and how such behaviours are modified with increasing hearing impairment. In this study we used speech-shaped noise, and the constant masking may have made conversation particularly difficult. When listening against a background of other talkers, individuals may be able to ‘listen in the gaps’ to ameliorate difficulty, reducing reliance on facilitatory strategies. Furthermore, when participants do employ strategies, they may rely more strongly on those that increase signal-to-noise ratio to take advantage of dips in the masker (such as decreasing interpersonal distance or optimising head orientation). Investigating conversation behaviours in different sorts of background noise, such as babble, could be a valuable extension of this work. It is also important to note that this study was run with participants of varying hearing ability, centring around mild hearing loss. While this reflects typical hearing of individuals in the age range tested, a next step could be to investigate whether more severe hearing impairment leads to greater reliance on the strategies reported, or the uptake of new ones, as well as how strategy use is impacted by use of hearing aids.
This work highlights the importance of measuring social processes, and particularly listening behaviours, in multi-person contexts. By providing a comprehensive record of conversation behaviours across multiple modalities while engaged in challenging conversation situations, our findings could be used to hone models of interpersonal communication, for example addressing how visual and auditory cues are used simultaneously. These findings could also be exploited in new communication technologies to improve user benefit. For example, we show that gaze is well-focused on the partner, while head orientation is offset by several degrees. Such information indicates the potential value of taking gaze direction into account in hearing aid design 25 . The raw dataset is available as Supplementary Material for such purposes.
We have shown how people behave during real conversations in noise in stationary chairs, behaviour that differs notably from that occurring when speaking or listening in isolation. We report inefficient use of behaviours that have the potential to provide high levels of acoustic benefit (e.g., increasing speech level and decreasing interpersonal distance), as well as possible prioritisation of behaviours providing alternative benefits (e.g., shortening utterances and increasing gaze to toward a speaker’s mouth). We also show that individuals seemingly sustain conversation even in high levels of background noise (up to 72 dB), although an increase in overlapping speech indicates potential break-down of conversational turn-taking past this point. This work provides a first multimodal investigation of interactive conversation between individuals in noise, and is critically important for the field of communication technology. By understanding the strategies used by dyads conversing in challenging conditions, technological innovations can begin to include processing strategies that work with, rather than against, natural behaviours.
Participants
Thirty unacquainted participants were divided into fifteen mixed-gender dyads (age mean = 61 years, age SD = 11 years; better-ear four-frequency pure-tone average (FFPTA across 0.5, 1, 2, and 4 kHz) mean = 22 dB HL, FFPTA SD = 12 dB HL). Within the available sample, participants were matched on age (difference mean = 6 years, difference SD = 5 years) and hearing asymmetry across ears (difference mean = 3 dB HL, difference SD = 2 dB HL). We also measured the difference in hearing loss between members of a pair (difference mean = 7 dB HL, difference SD = 6 dB HL). Each participant was paid £10 for taking part. This study was approved by the West of Scotland Research Ethics Committee (09/S0704/12). Methods were carried out in accordance with these ethical guidelines.
Materials and task
Participants were seated in the centre of a ring of eight equidistantly spaced loudspeakers (Tannoy VX-6) in a sound attenuated room (4.3 × 4.7 × 2.6 m; see Fig. 1a ). The loudspeakers each presented a different extract of steady-state noise with a spectrum equal to the long-term-average speech spectrum generated from data of Byrne and colleagues 26 , which includes recordings of male and female speakers across 12 languages. As noise levels in communal spaces are often over 70 dB 27 , 28 , we presented background noise continuously at 54, 60, 66, 72, or 78 dB, in 15–25 s segments with no gap between sequential levels. The complete counterbalancing of level ordering was determined using a paired de Bruijn sequence (individually sequenced for each trial 29 ), and smoothing was applied for 10 ms between segments (see Fig. 1c ). Each level was therefore presented five times, and hence each conversation lasted between 6 minutes 30 seconds and 10 minutes 50 seconds.
Vicon Tracker software was used to capture head-motion data, sampling at 100 Hz using a commercial infrared camera system (Vicon Bonita B-10 cameras fitted with wide angle lenses). Eight cameras were spaced around the room (one in each corner, plus one in the centre of each wall) to track 9-mm diameter reflective spheres that were arranged into uniquely identifiable ‘objects’ and attached to crowns on the head. Participant coordinates were measured in both Cartesian and polar space, calibrated to the centre of the floor. Temporal sampling rate was 100 Hz and spatial resolution was under 0.01°. Note that head position was recorded at the centre of the head (i.e. between the ears in line with the bridge of the nose) through reference to a pair of removable motion tracking goggles, as opposed to being recorded at the centre of the crown. Eye movement was recorded using 60 Hz Pupil Labs binocular eye trackers in 3D pupil detection mode and calibrated using a 5-point grid. The right eye was recorded in all participants except those that reported specific vision problems in that eye (two participants). Speech was recorded using a gooseneck microphone attached to the motion tracking crown approximately 6 cm from the participant’s mouth (see Fig. 1b ).
The experiment was controlled using Matlab, which determined loudspeaker output, recorded motion capture data in Euler coordinates, and recorded eye angle data. Matlab was also used to trigger changes in the presentation level of the background noise by sending the requested level (dB SPL) in the form of an 8-bit integer. The Max/MSP visual programming language was used to receive and convert this trigger to dB, which controlled the playback of an 8-channel speech-shaped noise wav file. The first of these triggers also initiated the capture of speech signals from the microphones. All audio was run at 16 bits and 44.1 kHz sample rate, I/O was handled with a Ferrofish A-16 driven by an RME MadiFace XT on the host computer.
Participants were introduced and taken into a sound attenuated room and seated face-to-face at a distance of 1.5 m (they were asked not to alter chair position). The motion tracking crowns with lapel microphones attached via a gooseneck were then fitted. Participants then each put on a pair of eye-tracking glasses and were individually calibrated in front of a 92 × 74 cm monitor at a distance of 125 cm. Hearing aids were not worn during the experiment. In total, setup took approximately 40 minutes.
Each dyad held three conversations (i.e. three trials), each lasting approximately 9 minutes. The conversation topics focused on: films, close-call incidents, and the resolution of an ethical dilemma. In the film conversation 30 , participants were asked to discuss what they liked to watch. In the close-call conversation 31 , participants were asked to discuss a near miss: a time something bad almost happened but that worked out in the end. In the ethical dilemma conversation, participants were asked to come to a joint decision regarding the Balloon task 32 . In the Balloon task, participants must choose who to sacrifice from a descending hot air balloon between a scientist on the brink of curing cancer, a pregnant woman, and the pilot (her husband). Order of conversation topics was counterbalanced.
Participants were told that they should try to keep conversing the entire time that the experimenter was outside of the room, and that background noise would play from the surrounding speakers throughout. In between each conversation, the experimenter went into the room to confirm that participants were happy to continue, give them their next topic, and perform an eye tracker drift correction.
Data from one dyad was removed due to a motion tracking error (3 trials). Of the remaining 45 trials, a technical fault led to the loss of one audio recording, hence this trial was also removed. Analysis was conducted on the remaining 44 trials. Analyses were run using repeated-measures ANOVA on participant data averaged across all instances of each level across all conversation trials. Greenhouse-Geisser correction was used when assumptions of sphericity were violated (SPSS, v24). We also report partial eta squared values to indicate effect size (ηp2), for which a value of 0.01 is considered small and 0.26 is considered large 33 . Confidence intervals (95%) were calculated from the subject × condition interaction term 34 .
Periods of speech were detected from average root mean square (RMS) amplitude using an automated algorithm dependent on a manually-selected RMS threshold across a rolling window. A smoothing value of 0.1 Hz and a pause allowance of 1.25 s were used (due to few pauses being greater than 1.25 s in conversation) 35 , 36 . This allowed speech to be defined as periods during which an individual’s microphone recording was above threshold, and listening to be defined as periods during which the other individual’s microphone recordings were above threshold. Analyses of speech level were run only across the times that individuals were determined to be speaking, as opposed to over the entire recordings. Note that while microphones did pick up a small amount of the background noise, this amounted to a mean RMS level increase of only 0.39 dB for each 6 dB increase, and reported levels are corrected. Utterance duration was calculated across all speech segments with a duration of over 50 ms (to remove clicks). Any utterances that spanned a noise level transition were excluded from duration analyses.
Prior to analysis, eye tracking data was transformed to the Vicon axes (as opposed to the position to the eye camera) using the validation data from the start of the experiment. Drift was then corrected at the start of each trial through reference to the other participant’s head centre. Eye angle data was then added to head movement to generate gaze coordinates. Head angle and gaze angle were calculated in relation to the centre of the other participant’s head (i.e., oriented directly towards the other participant would be 0° pitch and yaw).
Anonymised data analysed during this study is included in Supplementary Information. Identifiable speech data is shared as binary data coding indicating when the recorded audio was over vs under threshold, i.e. when speech occurred.
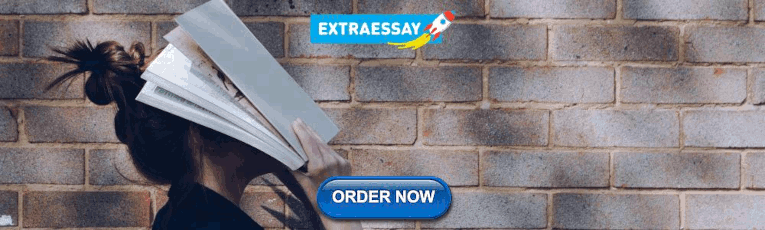
Informed consent
Informed consent was obtained from each participant prior to initiation of the study. Informed consent was also obtained for publication of images of non-participating individuals in an open-access journal.
Data Availability
Anonymised data analysed during this study is included in Supplementary Information. Identifiable speech data is shared as binary data coding when the recorded audio was over vs under threshold, i.e. when speech occurred.
Stivers, T. et al . Universals and cultural variation in turn-taking in conversation. Proc. Natl. Acad. Sci. USA 106 , 10587–92 (2009).
Article ADS CAS Google Scholar
Pichora‐Fuller, M. K., Schneider, B. A. & Daneman, M. How young and old adults listen to and remember speech in noise. J. Acoust. Soc. Am. 97 , 593–608 (1995).
Article ADS Google Scholar
Strawbridge, W. J., Wallhagen, M. I., Shema, S. J. & Kaplan, G. A. Negative Consequences of Hearing Impairment in Old Age. Gerontologist 40 , 320–326 (2000).
Article CAS Google Scholar
Junqua, J. C., Fincke, S. & Field, K. The Lombard effect: a reflex to better communicate with others in noise. Proc. 1999 IEEE Int. Conf. Acoust. Speech, Signal Process. 4 , 2083–2086 (1999).
Article Google Scholar
Garnier, M., Henrich, N. & Dubois, D. Influence of Sound Immersion and Communicative Interaction on the Lombard Effect. J. Speech Lang. Hear. Res. 53 , 588 (2010).
Pittman, A. L. & Wiley, T. L. Recognition of Speech Produced in Noise. J. Speech Lang. Hear. Res. 44 , 487–496 (2001).
Beechey, T., Buchholz, J. M. & Keidser, G. Measuring communication difficulty through effortful speech production during conversation. Speech Commun. 100 , 18–29 (2018).
Hazan, V. & Pettinato, M. The emergence of rhythmic strategies for clarifying speech: variation of syllable rate and pausing in adults, children and teenagers. In Proceedings of the 10th international seminar on speech production 178–181 (2014).
Davis, C., Kim, J., Grauwinkel, K. & Mixdorff, H. Lombard speech: Auditory (A), Visual (V) and AV effects. In Proceedings of the Third International Conference on Speech Prosody 248–252 (2006).
Blauert, J. Spatial hearing: the psychophysics of human sound localization (1983).
Kock, W. E. Binaural Localization and Masking. J. Acoust. Soc. Am. 22 , 801–804 (1950).
Grange, J. et al . Turn an ear to hear: How hearing-impaired listeners can exploit head orientation to enhance their speech intelligibility in noisy social settings. In Proceedings of the International Symposium on Auditory and Audiological Research 9–16 (2018).
Brimijoin, W. O., McShefferty, D. & Akeroyd, M. A. Undirected head movements of listeners with asymmetrical hearing impairment during a speech-in-noise task. Hear. Res. 283 , 162–168 (2012).
Jelfs, S., Culling, J. F. & Lavandier, M. Revision and validation of a binaural model for speech intelligibility in noise. Hear. Res. 275 , 96–104 (2011).
Vertegaal, R., Slagter, R., Van der Veer, G. & Nijholt, A. Eye gaze patterns in conversations: there is more to conversational agents than meets the eyes. In Proceedings of the SIGCHI conference on Human factors in computing systems 301–308 (2001).
Bavelas, J. B., Coates, L. & Johnson, T. Listener Responses as a Collaborative Process: The Role of Gaze. J. Commun. 52 , 566–580 (2002).
Munhall, K. G., Jones, J. A., Callan, D. E., Kuratate, T. & Vatikiotis-Bateson, E. Visual Prosody and Speech Intelligibility. Psychol. Sci. 15 , 133–137 (2004).
Schwartz, J.-L., Berthommier, F. & Savariaux, C. Seeing to hear better: evidence for early audio-visual interactions in speech identification. Cognition 93 , B69–B78 (2004).
Sumby, W. H. & Pollack, I. Visual Contribution to Speech Intelligibility in Noise. J. Acoust. Soc. Am. 26 , 212–215 (1954).
Grant, K. W. & Seitz, P.-F. The use of visible speech cues for improving auditory detection of spoken sentences. J. Acoust. Soc. Am. 108 , 1197–1208 (2000).
Grange, J. A. & Culling, J. F. The benefit of head orientation to speech intelligibility in noise. J. Acoust. Soc. Am. 139 , 703–712 (2016).
Schilbach, L. On the relationship of online and offline social cognition. Front. Hum. Neurosci. 8 , 278 (2014).
Schilbach, L. Eye to eye, face to face and brain to brain: novel approaches to study the behavioral dynamics and neural mechanisms of social interactions. Curr. Opin. Behav. Sci. 3 , 130–135 (2015).
McShefferty, D., Whitmer, W. M. & Akeroyd, M. A. The Just-Noticeable Difference in Speech-to-Noise Ratio. Trends Hear. 19 , 1–9 (2015).
Google Scholar
Hládek, Ľ., Porr, B. & Brimijoin, W. O. Real-time estimation of horizontal gaze angle by saccade integration using in-ear electrooculography. PLoS One 13 , e0190420 (2018).
Byrne, D. et al . An international comparison of long‐term average speech spectra. J. Acoust. Soc. Am. 96 , 2108–2120 (1994).
Lebo, C. P. et al . Restaurant noise, hearing loss, and hearing aids. West. J. Med. 161 , 45–9 (1994).
CAS PubMed PubMed Central Google Scholar
Gershon, R. R. M., Neitzel, R., Barrera, M. A. & Akram, M. Pilot Survey of Subway and Bus Stop Noise Levels. J. Urban Heal. 83 , 802–812 (2006).
De Bruijn, N. G. A Combinatorial Problem. In Koninklijke Nederlandse Akademie Wetenschappen 49 , 758–764 (1946).
MathSciNet MATH Google Scholar
Rimé, B. The elimination of visible behaviour from social interactions: Effects on verbal, nonverbal and interpersonal variables. Eur. J. Soc. Psychol. 12 , 113–129 (1982).
Bavelas, J. B., Coates, L. & Johnson, T. Listeners as co-narrators. J. Pers. Soc. Psychol. 79 , 941–952 (2000).
Healey, P. G., Purver, M., King, J., Ginzburg, J. & Mills, G. J. Experimenting with clarification in dialogue. Proc. Annu. Meet. Cogn. Sci. Soc . 25 (2003).
Perdices, M. Null Hypothesis Significance Testing, p-values, Effects Sizes and Confidence Intervals. Brain Impair. 19 , 70–80 (2018).
Loftus, G. R. & Masson, M. E. J. Using confidence intervals in within-subject designs. Psychon. Bull. Rev. 1 , 476–490 (1994).
Heldner, M. & Edlund, J. Pauses, gaps and overlaps in conversations. J. Phon. 38 , 555–568 (2010).
Campione, E. & Véronis, J. A large-scale multilingual study of silent pause duration. In Proceedings of the first international conference on speech prosody (Speech prosody 2002) 199–202 (2002).
Download references
Acknowledgements
This work was supported by funding from the Medical Research Council (Grant Number MR/S003576/1); and the Chief Scientist Office of the Scottish Government.
Author information
Authors and affiliations.
Hearing Sciences – Scottish Section, Division of Clinical Neuroscience, University of Nottingham, Glasgow, UK
Lauren V. Hadley, W. Owen Brimijoin & William M. Whitmer
You can also search for this author in PubMed Google Scholar
Contributions
W.M.W. and W.O.B. developed the study concept. All authors contributed to the study design. L.V.H. performed testing and data collection. All authors contributed to data processing, analysis, and interpretation. L.V.H drafted the manuscript, and W.M.W. and W.O.B. provided critical revisions. All authors approved the final version of the manuscript for submission.
Corresponding author
Correspondence to Lauren V. Hadley .
Ethics declarations
Competing interests.
The authors declare no competing interests.
Additional information
Publisher’s note: Springer Nature remains neutral with regard to jurisdictional claims in published maps and institutional affiliations.
Supplementary information
Rights and permissions.
Open Access This article is licensed under a Creative Commons Attribution 4.0 International License, which permits use, sharing, adaptation, distribution and reproduction in any medium or format, as long as you give appropriate credit to the original author(s) and the source, provide a link to the Creative Commons license, and indicate if changes were made. The images or other third party material in this article are included in the article’s Creative Commons license, unless indicated otherwise in a credit line to the material. If material is not included in the article’s Creative Commons license and your intended use is not permitted by statutory regulation or exceeds the permitted use, you will need to obtain permission directly from the copyright holder. To view a copy of this license, visit http://creativecommons.org/licenses/by/4.0/ .
Reprints and permissions
About this article
Cite this article.
Hadley, L.V., Brimijoin, W.O. & Whitmer, W.M. Speech, movement, and gaze behaviours during dyadic conversation in noise. Sci Rep 9 , 10451 (2019). https://doi.org/10.1038/s41598-019-46416-0
Download citation
Received : 20 August 2018
Accepted : 20 June 2019
Published : 18 July 2019
DOI : https://doi.org/10.1038/s41598-019-46416-0
Share this article
Anyone you share the following link with will be able to read this content:
Sorry, a shareable link is not currently available for this article.
Provided by the Springer Nature SharedIt content-sharing initiative
This article is cited by
- Kelly Miles
- Adam Weisser
- Joerg M. Buchholz
Scientific Reports (2023)
Use of artificial neural networks to assess train horn noise at a railway level crossing in India
- Boddu Sudhir Kumar
- Venkaiah Chowdary
Environmental Monitoring and Assessment (2023)
By submitting a comment you agree to abide by our Terms and Community Guidelines . If you find something abusive or that does not comply with our terms or guidelines please flag it as inappropriate.
Quick links
- Explore articles by subject
- Guide to authors
- Editorial policies
Sign up for the Nature Briefing newsletter — what matters in science, free to your inbox daily.


11 Behaviors of a Mindful Speaker (Plus 8 Techniques)
Do you sometimes feel detached when conversing or envy those who master public speaking effortlessly? This often indicates a need for mindful speaking, a concept that demands total presence in your dialogues.
Mindful speaking involves conscious attention to your words, their delivery, and reception. It values listening and acknowledges the impact of every spoken word or silence.
As a mindful speaker, one communicates with intent, lucidity, and compassion. The words, tone, and body language align to effectively convey the message. The goal is to ensure comprehension, not just audibility.
This piece explores 11 behaviors of mindful speaking that can enhance communication, enrich relationships, and foster personal and professional growth. These behaviors prove beneficial whether you’re delivering a speech, engaging in difficult dialogues, or simply conversing with a friend.
What Is a Mindful Speaker?
Mindful speaking is all about using mindfulness to be present and fully engaged in your conversations. It’s about listening deeply , speaking authentically, and responding thoughtfully.
It’s about being aware of your words, your tone, and your body language, and how these can impact others. But more than that, it’s about creating a sense of respect and understanding in our interactions, creating a space where everyone feels heard and valued.

Why Is Mindful Speaking So Important?
Well, think about it. Communication is at the heart of our relationships , our work, and our daily interactions. When we communicate mindfully, we can improve our relationships, increase our effectiveness at work, and even boost our own mental well-being.
The 11 Behaviors of a Mindful Speaker
So, if you’re ready to transform your communication and bring more mindfulness into your life, keep reading. I’ve got some practical tips and insights just for you.

Behavior 1: Be More Precise in Your Speech
Speaking clearly and thoughtfully is key. When we’re nervous or unsure, we might talk fast or lose our train of thought, making it hard for others to understand us. To avoid this, take a moment to pause and breathe before you speak.
Choose your words carefully to accurately express your thoughts. It’s better to take a bit more time to ensure your message is clear, rather than rushing and leaving everyone confused. Remember, effective communication is about clarity, not speed.
Behavior 2: Simplicity and Clarity
Simplicity and clarity go hand in hand with precision. Mindful speakers avoid unnecessary jargon and complex language. They try to make their message as simple and clear as possible, making it easier for others to understand. Remember, the goal of communication is not to sound smart but to be understood.
Behavior 3: Mindful Pace
A mindful speaker also pays attention to the pace of their speech. Speaking too fast can make it difficult for others to keep up while speaking too slowly can make the conversation drag.
A mindful pace means speaking slowly enough to be clear, but quickly enough to keep the conversation flowing. It’s about finding a balance that feels comfortable and natural. A little tip I use is to gently tap my foot while speaking. This uses the mind-body connection to create a speech metronome to keep you speaking at a steady pace.
Behavior 4: Embrace Silence
We often see silence as something to be avoided in conversation, but mindful speakers understand the value of embracing silence.
Pauses can give us time to gather our thoughts, process what’s been said, and respond more thoughtfully. Plus, when you pause, you create a sense of authority and intent with the words you say. Instead of rushing to fill every silence, try to sit with it, and see what it has to teach you.
Behavior 5: Active Listening
Active listening is a key part of mindful speaking. This means fully focusing on the other person, showing that you’re interested and engaged.
It’s about more than just hearing the words – it’s about understanding the emotions and intentions behind them. Active listening shows respect and empathy and can help to build stronger, more meaningful connections.
Behavior 6: Self-Observation
Mindful speaking also involves self-observation. This means being aware of our own speech patterns, body language, and emotional reactions. It might feel weird at first but recording yourself practicing speech is a great way to learn which areas to work on to improve your speech.
By paying attention to ourselves, we can become more aware of our habits and tendencies, and make conscious choices to improve our communication.
Behavior 7: Non-Judgmental Attitude
A non-judgmental attitude is another important aspect of mindful speaking. This means accepting others as they are, without trying to change them or judge them. It’s about understanding that everyone has their own perspective and that it’s okay to disagree.
Sometimes because of our assumptions or prejudices, we might misinterpret what’s being said, so listening and being mindful can make sure you’re getting the right message.
A non-judgmental attitude promotes open and honest communication and helps to build trust and respect.
Behavior 8: Emotional Awareness
Emotional awareness is also crucial for mindful speaking. This means being aware of our own emotions, as well as the emotions of others. It’s about understanding how emotions can influence our communication and learning to manage them effectively.
Instead of just focusing on the words people are saying, you also pay attention to their tone of voice, their body language, and the emotions they’re expressing.
Emotional awareness can help us to respond to others in a more empathetic and understanding way.
Behavior 9: Empathy and Understanding
Empathy and understanding are at the heart of mindful speaking. This means trying to see things from the other person’s perspective, and showing that you care about their feelings. It’s about validating their experiences, and showing them that they’re not alone.
Instead of quickly offering advice, you first try to understand their feelings and perspective.
Empathy and understanding can help to deepen our connections and make our conversations more meaningful.
Behavior 10: Respect for Others’ Perspectives
Mindful speakers show respect for others’ perspectives. They understand that everyone has their own unique experiences and viewpoints, and they value this diversity. They listen with an open mind, and they’re willing to learn from others.
As a mindful speaker, you understand that everyone has their own unique viewpoints shaped by their life experiences.
Respect for others’ perspectives fosters a sense of equality and mutual understanding in our conversations.
Behavior 11: Openness to Feedback
Finally, openness to feedback is a key behavior of a mindful speaker. This means being willing to hear and consider others’ opinions and suggestions, even if they’re different from our own. It’s about being open to learning and growing, and recognizing that we don’t have all the answers.
As a mindful speaker, you’re not just interested in expressing your own views, but also in hearing what others have to say.
Openness to feedback shows humility and a commitment to continuous improvement.
By using these 11 behaviors, you can become a more mindful speaker, improving your communication and strengthening your relationships. Remember, mindful speaking is a journey, not a destination. So be patient with yourself, and celebrate your progress along the way.

Techniques to Speak More Mindfully
Technique 1: grounding yourself and powerful posture.
The way you carry yourself on stage can make a world of difference in your public speaking. Grounding yourself and adopting a powerful posture can help you feel more confident and composed. Here’s how to do it:
- Stand with your feet hip-width apart and distribute your weight evenly between both legs.
- Keep your knees slightly bent to maintain flexibility.
- Allow your arms to hang naturally by your sides.
- Lengthen your spine and lift your chest, creating a sense of openness.
- Keep your chin parallel to the floor and relax your facial muscles.
By practicing this grounding technique, you’ll create a solid foundation for your speech, helping you feel more in control and at ease while on stage.
Technique 2: Embracing Presence and Single-Tasking
One of the keys to effective public speaking is being fully present and focused on your message. When you’re engaged in the moment, your audience will be more likely to connect with you and your message. To embrace presence and single-tasking:
- Set an intention to stay fully present during your speech.
- Avoid distractions like checking your phone or thinking about what’s next on your agenda.
- Be mindful of your breath as a way to anchor yourself in the present moment.
Remember, single-tasking and staying present can take practice, so be patient with yourself as you work on this skill.
Technique 3: Preparing with Conscious Breathing and 4/8 Breath
Your breath plays a crucial role in your voice and speech delivery. Practicing conscious breathing and the 4/8 breath technique can help you stay calm and focused while speaking. Here’s how:
- Find a quiet space before your speech and close your eyes.
- Take a deep breath in through your nose for four counts.
- Exhale through your mouth for eight counts.
- Repeat this process several times, focusing on your breath and allowing any tension to melt away.
This breathing technique can help you center yourself and prepare for your speech with a calm, focused mindset.
Technique 4: Making Effective Eye Contact and Connecting with Your Audience
Establishing genuine connections with your audience is crucial for impactful public speaking. One way to do this is through effective eye contact. Here’s how:
- Scan the room and make eye contact with individual audience members.
- Hold eye contact for a few seconds before moving on to the next person.
- Remember to include people sitting in different areas of the room, not just those in the front rows.
By making eye contact and including your audience in your personal space, you’ll create a sense of connection that can enhance your speech’s effectiveness.
Technique 5: Practicing Self-Compassion and Facing Your Fears
Being kind to yourself and facing your fears are essential aspects of overcoming public speaking anxiety. To practice self-compassion:
- Acknowledge any feelings of fear or anxiety that arise.
- Remind yourself that it’s normal to feel nervous before a speech.
- Treat yourself with kindness and understanding, just as you would a friend who was feeling anxious.
By embracing your fears and practicing self-compassion, you’ll be better equipped to handle the challenges of public speaking.
Technique 6: Becoming Embodied and Mindful of Your Body
Connecting your mind with your body is an essential aspect of mindful public speaking. To become embodied:
- Take a few moments before your speech to tune into your body.
- Notice any areas of tension or discomfort and breathe into those spaces.
- As you speak, stay aware of your body’s sensations and movements.
By being mindful of your body, you’ll be more in tune with your emotions and physical presence, which can enhance your overall performance.
Technique 7: Prioritizing Connection Over Content
While the content of your speech is important, it’s the connection with your audience that truly makes an impact. To prioritize connection:
- Focus on engaging your audience through storytelling, examples, and personal anecdotes.
- Adapt your speech based on the audience’s reactions and feedback.
- Remember that it’s okay to deviate from your script if it helps strengthen the connection with your audience.
By making connection a priority, you’ll create a more memorable and impactful experience for your audience.
Technique 8: Enjoying the Moment and Being Gentle with Yourself
Lastly, it’s essential to enjoy the public speaking experience and be gentle with yourself. Here’s how:
- Embrace the adrenaline rush and excitement that comes with public speaking.
- Remind yourself that you’re doing your best and that it’s okay to make mistakes.
- Celebrate your accomplishments and growth as a speaker.
By cultivating a positive mindset and being gentle with yourself, you’ll be more likely to enjoy the public speaking experience and continue to grow in your skills.
The Impact of Mindful Speaking
On personal relationships.
Mindful speaking can have a profound impact on our personal relationships. By speaking with precision, clarity, and empathy, we can foster deeper connections with our loved ones.
Mindful speaking allows us to express our feelings honestly and openly, reducing misunderstandings and promoting mutual understanding. It shows our loved ones that we value and respect them, strengthening our bonds and enriching our relationships.
On Professional Relationships
In our professional relationships, mindful speaking can enhance our effectiveness and credibility. By communicating clearly and respectfully, we can build trust and rapport with our colleagues and clients.
Mindful speaking can also help us to navigate difficult conversations and conflicts, promoting a more harmonious and productive work environment. Whether we’re leading a team, negotiating a deal, or presenting to a client, mindful speaking can help us to succeed.
On Self-Development
Mindful speaking also contributes to our self-development. By practicing mindful speaking, we can become more self-aware, and understand our communication habits and patterns.
This awareness can help us to improve our communication skills, manage our emotions more effectively, and become more empathetic and understanding. Mindful speaking is not just about improving our conversations, but about becoming better, more mindful individuals.
The Takeaway
Mindful speaking is a powerful tool that can transform our conversations, relationships, and even our understanding of ourselves. By focusing on precision in speech, simplicity, mindful pace, embracing silence, active listening, self-observation, a non-judgmental attitude, emotional awareness, empathy, respect for others’ perspectives, and openness to feedback, we can become more effective communicators and listeners.
But remember, becoming a mindful speaker isn’t about achieving perfection. It’s a journey of continuous learning and growth. So, be patient with yourself, celebrate your progress, and keep striving to bring more mindfulness into your conversations. The benefits you’ll reap in your personal and professional life will be well worth the effort.
Yes, mindfulness can significantly improve your public speaking experience. It helps you manage anxiety, maintain focus, create a deeper connection with your audience, and make better decisions regarding your presentation. By incorporating mindfulness into your preparation and delivery, you can become a more confident and effective speaker.
Practicing mindfulness in speech involves being present and fully engaged with your audience. You can achieve this by focusing on your breath, connecting with your thoughts and feelings, and being attentive to your audience’s reactions. Additionally, incorporating storytelling, anecdotes, humor, and thought-provoking questions can help create a more mindful and engaging presentation.
To incorporate mindfulness into your preparation, start by connecting with yourself and understanding your strengths, challenges, and motivations. Then, research your audience to tailor your presentation to their wants and needs. Finally, practice mindfulness exercises, such as deep breathing and meditation, to help manage anxiety and stay focused during your presentation.
Absolutely! Mindfulness techniques, such as focusing on your breath and grounding yourself in the present moment, can help you manage stage fright by reducing anxiety and promoting a sense of calm. Regular practice of these techniques can lead to increased confidence and reduced nervousness when speaking in public.
The time it takes to see the benefits of mindfulness in public speaking varies from person to person. Some individuals may notice improvements after just a few mindfulness exercises or meditation sessions, while others may require more practice. It’s essential to be patient and consistent in your mindfulness practice to experience the full benefits in your public speaking endeavors.
Eddie Doru is a Certified Meditation Teacher and founder of ZenGuided.com. He is a devoted meditation and mindfulness practitioner and teacher dedicated to helping individuals achieve inner peace and well-being in today's fast-paced world. With over six years of daily meditation experience, Eddie's teachings are rooted in personal practice and ongoing learning, including workshops, retreats, and engagement with meditation communities. Residing in North London with his wife, Eddie continually deepens his understanding of meditation, mindfulness, and well-being, sharing these invaluable insights with the global audience through ZenGuided.com.
Similar Posts

Is Meditation a Skill? 10 Benefits of Mindful Mastery
Meditation is more than just a relaxation technique; it’s a journey of self-discovery and inner peace. It’s a practice…

5 Habits That Could Ruin Your Life: How to Identify and Break Them
We all have habits – some good, some not so good. But there are certain bad habits that could…

Is Meditation Worth It? Does It Really Work?
Is meditation worth it? Is it the magic pill for a cluttered mind, or just another overhyped fad? Some…

Mindfulness for Preschoolers: Techniques and Activities to Help Kids Thrive

There is no doubt about it: each day in my classroom presents a unique set of challenges. But the…

What is Japa Meditation and 7 Powerful Steps to Practice It?
“What is Japa meditation?” you ask as you sip your third coffee of the day, half-heartedly scrolling through your…

Living for Yourself: 7 Ways To Take Back Your Life
Living for yourself has got a reputation for sounding bad. It seems to suggest that you’re being selfish if…
Leave a Reply Cancel reply
Your email address will not be published. Required fields are marked *
Save my name, email, and website in this browser for the next time I comment.

1 Minute Speech on Behaviour In English
Respected Principal, teachers and my dear friends, a wonderful morning to all of you. Today on this special occasion, I would like to speak some words on the topic- Behaviour.
Behaviour is the way in which one acts or presents themselves. It consists of a person’s unique mannerisms, ways of speech, habits and more. The way in which one behaves can tell you a lot about the kind of person they are, and help us understand their character better. Good behaviour always attracts others and makes one seem approachable and nice. It is an expression of good character and morals.
Similarly, bad behaviour is repulsive to people and has no positive consequences. However, outward behaviour can also be deceptive, and we must never judge a book solely by its cover. Still, behaviour is an important factor that establishes one’s position in society.
To conclude, we must always try to be on our best behaviour! Thank you for listening to me so attentively.
Related Posts:
- Raag Darbari Summary & Notes Part 3 by Shrilal Shukla
- Of Friendship Essay | Summary by Francis Bacon
- Random Disease Generator [Fake & Real]
- A Grammarian's Funeral by Robert Browning Summary
- Random Funny Joke Generator [with Answers]
- Random Idiom Generator

- Self & Career Exploration
- Blue Chip Leadership Experience
- Experiential Learning
- Research Experiences
- Transferable Skills
- Functional Skills
- Resume, CV & Cover Letter
- Online Profiles
- Networking & Relationship Building
- Internships
- Interviewing
- Offer Evaluation & Negotiation
- Career Core by Kaplan
- Arts & Media
- Commerce & Management
- Data & Technology
- Education & Social Services
- Engineering & Infrastructure
- Environment & Resources
- Global Impact & Public Service
- Health & Biosciences
- Law & Justice
- Research & Academia
- Recent Alumni
- Other Alumni Interest Areas
- People of Color
- First Generation
- International
- Faculty & Staff
- Parents & Families
Comprehensive Speech and Therapy Center
Behavior technician.
- Share This: Share Behavior Technician on Facebook Share Behavior Technician on LinkedIn Share Behavior Technician on X
We are hiring!
CSTC is locally owned and operated in Jackson, MI since 2004. Providing habilitative and rehabilitative services to children and adults with a variety of developmental and neurological disorders both in clinic and within the home in your area!
We are looking for Behavior Techs/ABA Techs to join our team. Looking for part time, with the opportunity to advance to full time, based on the needs of the business. Hours of operation are M-F 8:00-6:30pm. Behavior technicians/ABA Techs provide treatment plans for children with autism. As a behavior technician, you’ll work under a BCBA to create the most impactful changes for each client. A degree in a related field (social work, psychology, education, etc) is preferred but not required. Will consider candidate with high school diploma only if a strong work history or experience with children is present.
Qualifications
Minimum of a high school diploma or GED equivalent. Preferred degree, coursework or training in a related field, and on the job training in Applied behavioral analysis
Demonstrate the ability to communicate receptively and expressively to effectively complete job duties
Must be able to navigate an electronic device to complete daily responsibilities including emails, inputting documentation, and data collected
Must have a valid driver’s license
Must have reliable transportation and be willing and able to drive self between the clinic and assigned job location (client’s home environment, school, community)
Physical Qualifications
Must be able to lift up to 50 pounds
Must be able to lift and carry clients with adaptive equipment
Must be able to assume and maintain a variety of postures (kneeling, squatting, crawling, sitting, standing) for extended periods of time
Must be willing and able to utilize quick body movements to assist in behavior management strategies outlined within the client’s treatment plan
Competencies
Complete the Registered Behavior Technician Task List
Responsibilities
Read, understand and Implement Individualized Treatment Plans (ITPs) under the direction of the behavior analysts/consultants
Must be physically present at the assigned job location, which may include home, school, and community placements
Demonstrates understanding and use of ABA principles to teach the client to acquire language, academics, self-help and social skills according to the ITP
Applies behavioral principles consistently in all interactions with clients
Actively participates in staff training programs
Actively participates in family meetings/trainings regarding the ITP and provides data/suggestions for changes in plans
Collects data during sessions, reports the condition and behavior of the clients and maintain daily records
Functions as an active member of the treatment team
Provides co-treatment with other specialists as necessary
Maintains a caseload scheduled at 90%+ productivity, with a target of 80% actual productivity
517-750-4777 WWW.THERAPYJACKSON.COM
1001 LAURENCE PARK PLACE, JACKSON MI
We respectfully acknowledge the University of Arizona is on the land and territories of Indigenous peoples. Today, Arizona is home to 22 federally recognized tribes, with Tucson being home to the O'odham and the Yaqui. Committed to diversity and inclusion, the University strives to build sustainable relationships with sovereign Native Nations and Indigenous communities through education offerings, partnerships, and community service.
Forensic Psychiatrist Identifies Physical Indicators of Trump’s Cognitive Decline: Alterations in Movement and Gait
D onald Trump’s evident challenges with speech, language, and cognitive function continue to exacerbate, as highlighted during a recent rally in Ohio where he exhibited a disjointed manner of speech, veering off-topic, and making puzzling statements such as claiming Joe Biden defeated Barack Obama in swing states.
This pattern of behavior is not an isolated incident but rather indicative of broader issues affecting Trump’s ability to communicate and process information, suggesting a potential underlying neurological condition.
Psychiatrist Dr. John Gartner, known for his contributions to the book “The Dangerous Case of Donald Trump,” has repeatedly raised concerns about Trump’s cognitive and communicative difficulties. Despite these warnings, there has been a lack of acknowledgment from many in the media and political spheres, with attention often diverted to trivialities like Biden’s occasional verbal slip-ups.
However, Gartner asserts that Trump’s behavior is indicative of a more serious issue, suggesting a stark contrast between the cognitive decline observed in Biden and the concerning symptoms displayed by Trump.
Gartner’s concerns are echoed by hundreds of medical professionals who have signed a petition diagnosing Trump with probable dementia. The implications of Trump’s deteriorating mental faculties extend beyond national borders, raising the alarming prospect of a man with diminishing cognitive abilities wielding the immense power of the presidency, including control over nuclear weapons.
Despite these glaring signs, mainstream media outlets have largely overlooked Trump’s cognitive decline, opting instead for a focus on superficial aspects of political discourse. However, there are exceptions, such as Jennifer Rubin of The Washington Post, who has addressed Trump’s physical and cognitive changes.
To gain further insight into Trump’s behavior, Dr. Elizabeth Zoffman, a forensic psychiatrist, suggests that Trump’s symptoms align with Behavioral Variant Fronto-Temporal Dementia (FTD). This diagnosis is based on observed changes in Trump’s speech patterns, cognitive focus, pronunciation difficulties, tangential thinking, repetition of words, and social behavior.
Zoffman emphasizes the importance of a comprehensive evaluation by neurologists specializing in FTD to confirm the diagnosis.
While some may question the danger posed by individuals with FTD, Zoffman clarifies that it does not inherently make someone dangerous. However, the associated disinhibition may exacerbate existing personality traits, potentially leading to socially inappropriate behavior and harmful rhetoric, as seen in Trump’s dehumanizing statements toward marginalized groups.
Zoffman dispels misconceptions about dementia, emphasizing that it encompasses various neurodegenerative disorders, including Alzheimer’s Disease and FTD. She stresses the need to consider all available observations collectively to form a comprehensive understanding of Trump’s condition.
Addressing skepticism surrounding her decision to speak out, Zoffman acknowledges societal biases against female experts but emphasizes the importance of contributing to an objective evaluation of Trump’s health alongside other professionals.
In contrast to Trump, President Biden’s cognitive abilities appear intact, as evidenced by his recent State of the Union Address. Despite occasional pauses due to his lifelong struggle with stuttering, Biden’s speech was coherent, articulate, and devoid of tangential digressions or disinhibited behavior.
Regarding Trump’s prognosis, Zoffman warns that FTD is a progressive disorder leading to deterioration and early death. Therefore, a thorough assessment by experts specializing in neurodegenerative disorders is imperative to determine the extent of Trump’s condition and its potential implications.
Must Read Posts:
- US and Japan Advocate for Ban on Nuclear Weapons in Outer Space
- What is “We Deserve Better,” the organization Owen Jones departed Labour to join?
- McCarthy’s Seat to Remain Vacant for Two Months
Recently arrested Morgan Wallen says he's "not proud" of behavior
Country music star Morgan Wallen says he’s “not proud” of his behavior and accepts responsibility after being charged following accusations that he threw a chair off the rooftop of a six-story bar
NASHVILLE, Tenn. -- Country music star Morgan Wallen, who faces charges stemming in part from accusations that he threw a chair off the rooftop of a six-story bar, says he’s “not proud” of his behavior and accepts responsibility.
The “One Thing at a Time” singer responded publicly Friday night on social media to his arrest in Nashville two weeks ago. He faces a May 3 court date after being charged with three felony counts of reckless endangerment and one misdemeanor count of disorderly conduct, court records show.
An arrest affidavit said the chair at Chief’s bar landed about 3 feet (1 meter) from officers, who talked to witnesses and reviewed security footage. Witnesses told officers that they watched Wallen pick up a chair, throw it over the roof and laugh about it. Wallen was booked early April 8 and released.
“I didn’t feel right publicly checking in until I made amends with some folks. I’ve touched base with Nashville law enforcement, my family, and the good people at Chief’s. I’m not proud of my behavior, and I accept responsibility,” Wallen wrote on X, formerly Twitter .
Wallen, one of the biggest names in contemporary country, is currently on a stadium tour, including a concert scheduled for Saturday at Vaught-Hemingway Stadium in Oxford, Mississippi.
“I have the utmost respect for the officers working every day to keep us all safe. Regarding my tour, there will be no change,” his message said, signed ”-MW.”
The “One Thing at a Time” album spent 16 weeks at the top of the Billboard 200 in 2023 and was the most-consumed album in the U.S. last year. Top 10 hits from the album included “Last Night,” “You Proof” and “Thinkin’ Bout Me.”
In 2021, the country singer was suspended indefinitely from his label after video surfaced of him shouting a racial slur. In 2020, he was arrested on public intoxication and disorderly conduct charges after being kicked out of Kid Rock’s bar in downtown Nashville.
Top Stories
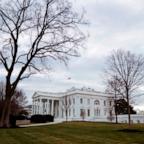
State law takes US a step closer to popular vote deciding presidential elections
- Apr 21, 6:23 AM
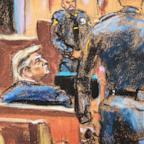
Trump hush money trial: Judge sets opening statements for Monday
- Apr 19, 5:15 PM
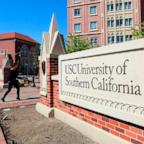
USC cancels all commencement speakers after canceled valedictorian speech
- Apr 19, 10:02 PM
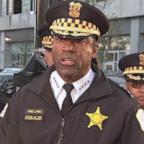
Police officer gunned down, car taken as he drove home from work: Officials
- 26 minutes ago
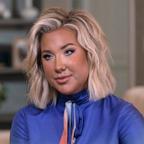
Savannah Chrisley talks about the fate of her parents Todd and Julie
- Apr 19, 6:48 PM
ABC News Live
24/7 coverage of breaking news and live events
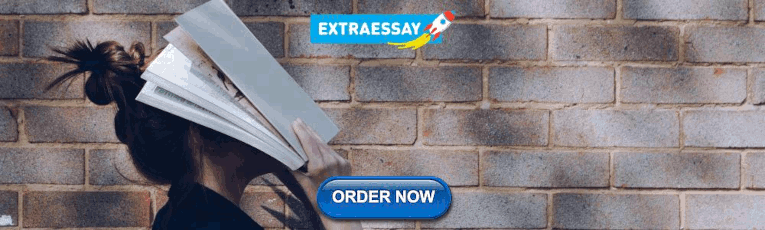
IMAGES
VIDEO
COMMENTS
What behavior analysis can offer language researchers and speech-language pathologists (SLPs) is a coherent and parsimonious interpretation of speech consistent with experimentally established scientific principles of learning that has immediate practical applications. The purpose of this paper is to illustrate a general behavioral approach to ...
PurposeMany speech-language pathologists (SLPs) experience challenging behaviors during service delivery and also report minimal training in effective behavior management strategies. ... Speech-language pathologists' behavior management training and reported experiences with challenging behavior. Communication Disorders Quarterly. https://doi ...
Understanding behaviour as communication Negative behaviour in children and young people with SLCN could mean: The risks of not supporting speech, language and communication needs Unidentified and unsupported SLCN put children and young people at risk of a range of negative outcomes in relation to behaviour:
Functional speech-language behavior is evoked and strengthened in a unit in which antecedent and consequent stimuli occur in temporal proximity to an instance of a speaker's topographic behavior and combine to become functional communication (see Sautter & LeBlanc, 2006). Therefore, its description, to be useful for treatment planning, must ...
Abstract. Conversational entrainment, also known as alignment, accommodation, convergence, and coordination, is broadly defined as similarity of communicative behavior between interlocutors. Within current literature, specific terminology, definitions, and measurement approaches are wide-ranging and highly variable.
We compared six acoustic measures of participant speech behavior across conversational task and partner. Results: Linear mixed-effects models demonstrated significant differences between speech feature measures in informational and relational conversations. ... Journal of Speech, Language, and Hearing Research, 62(2), 470-484. https://doi.org ...
Abstract. A hierarchical view of the central nervous system suggests the principle that " lower level " activities are often recruited for utilization by subsequently evolved functions. In this view, speech, as distinguished from language, is a more automatic process in part responsive to perceptual input. It both shapes and is shaped by those ...
Speech, language and communication needs and mental health: the experiences of speech and language therapists and mental health professionals. Annabel Hancock, 1 Sarah Northcott, 2 Hannah Hobson, 3 and Michael Clarke 1 ... emotional well‐being and challenging behaviour. Findings suggest that there are organizational limitations in the fields ...
This work considers how environments affect speech behavior. The principal effect environments have on produced speech is that they modify its acoustic structure and, when a speaker hears this altered speech, it can influence the properties of the speech that is uttered. The changes to the speech sound and noise sources that are present in the ...
In this paper, we focus specifically on speech entrainment, which we define as the interdependent similarity of speech behaviors between interlocutors. Within this realm, similarity of interlocutor behavior may be interpreted in several different ways. It is not surprising, therefore, that specific definitions and measurement approaches used in ...
speech behavior are closely coupled during face-to-face interactions. Although some patterns of speech behavior during face-to-face interactions, such as in turn-taking, are common across dierent languages and cultures (Stivers et al., 2009), the role of gaze behavior in interaction seems to be culturally- as well as contextually-dependent (Foddy,
Speech disorders affect a person's ability to produce sounds that create words, and they can make verbal communication more difficult. Types of speech disorder include stuttering, apraxia, and ...
A primary mode of human social behavior is face-to-face interaction. In this study, we investigated the characteristics of gaze and its relation to speech behavior during video-mediated face-to-face interactions between parents and their preadolescent children. 81 parent-child dyads engaged in conversations about cooperative and conflictive family topics. We used a dual-eye tracking setup ...
In fact, in voice analysis, the choice of the vocal task can be crucial. Generally, tasks such as reading, free speech, interviews, commenting of images, dialogues, and sustained vowels are investigated. Anyway, it is important to highlight that a task-dependent behaviour of F 0 was observed in some speech-related features [46, 53].
We found that as noise increased, people spoke louder and moved closer together, although these behaviours provided relatively small acoustic benefit (0.32 dB speech level increase per 1 dB noise ...
This study aims at exploring the speech behavior as individually and phonetically different settings and actions of speech organs using the kinematic data on the motor behavior for speech production. To do so, a novel method based on speech recognition techniques was employed to reveal articulatory-to-acoustic processes, which we call called ...
Behavior 3: Mindful Pace. A mindful speaker also pays attention to the pace of their speech. Speaking too fast can make it difficult for others to keep up while speaking too slowly can make the conversation drag. A mindful pace means speaking slowly enough to be clear, but quickly enough to keep the conversation flowing.
It enlists the social and psychological characteristics of the speech act, aspects and purposes of communication. So, the main purpose of the study is the research of "communication-behaviour" connection through the prism of speech act components and the SPEAKING model. The research methods contain the componential analysis of the speech ...
Speech behavior is a potential key fact or in the study of personalized speech synthesis, speaker identification, and . emotional analysis.
To increase organization and cohesion in speech entrainment research, we provide: • An expanded version of an earlier framework to categorize types of speech entrainment. • A literature review showing how current literature fits into the framework. • A discussion of how the framework can be used to unify research efforts.
Knowledge about speech behaviour, speech impact, as well as the ability to use speech strategies and tactics can help achieve definite goals and increase communication efficiency. The study of ...
Contamination: fusing ideas into one another. Accelerated thinking: rapid flow and increased volume of speech. Flight of ideas: losing track of where a thought is going. Inhibited thinking: slow ...
Another way that speech and language delays play a role in childhood behavior problems relates to a child's ability to have an inner monologue. Research suggests that an inner monologue, much like an inner voice, helps children decide how to respond in different situations. Children with a language delay may be lacking this inner voice.
Behaviour is the way in which one acts or presents themselves. It consists of a person's unique mannerisms, ways of speech, habits and more. The way in which one behaves can tell you a lot about the kind of person they are, and help us understand their character better. Good behaviour always attracts others and makes one seem approachable and ...
As a behavior technician, you'll work under a BCBA to create the most impactful changes for each client. A degree in a related field (social work, psychology, education, etc) is preferred but not required. Will consider candidate with high school diploma only if a strong work history or experience with children is present. Qualifications.
A noisy peacock which has evaded capture while roaming the streets for six months has finally been rescued. The exotic bird, named Saataj by locals, has been living in Gravesend, Kent crossing a ...
This diagnosis is based on observed changes in Trump's speech patterns, cognitive focus, pronunciation difficulties, tangential thinking, repetition of words, and social behavior.
The Associated Press. NASHVILLE, Tenn. -- Country music star Morgan Wallen, who faces charges stemming in part from accusations that he threw a chair off the rooftop of a six-story bar, says he ...