
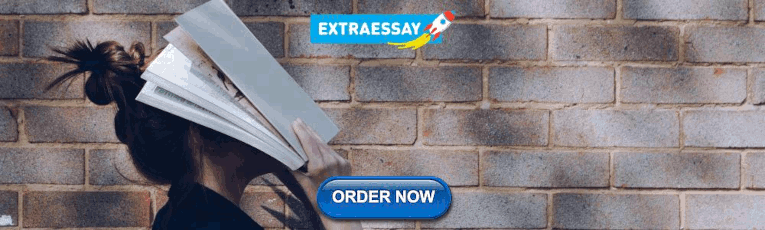
Research Topics & Ideas: Cybersecurity
50 Topic Ideas To Kickstart Your Research
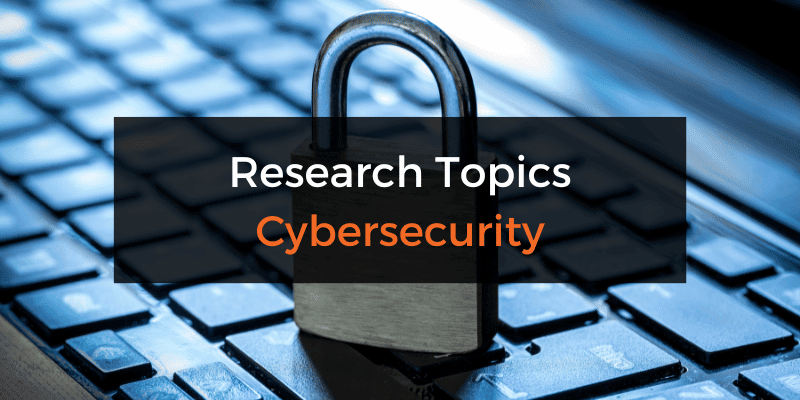
If you’re just starting out exploring cybersecurity-related topics for your dissertation, thesis or research project, you’ve come to the right place. In this post, we’ll help kickstart your research by providing a hearty list of cybersecurity-related research topics and ideas , including examples from recent studies.
PS – This is just the start…
We know it’s exciting to run through a list of research topics, but please keep in mind that this list is just a starting point . These topic ideas provided here are intentionally broad and generic , so keep in mind that you will need to develop them further. Nevertheless, they should inspire some ideas for your project.
To develop a suitable research topic, you’ll need to identify a clear and convincing research gap , and a viable plan to fill that gap. If this sounds foreign to you, check out our free research topic webinar that explores how to find and refine a high-quality research topic, from scratch. Alternatively, consider our 1-on-1 coaching service .
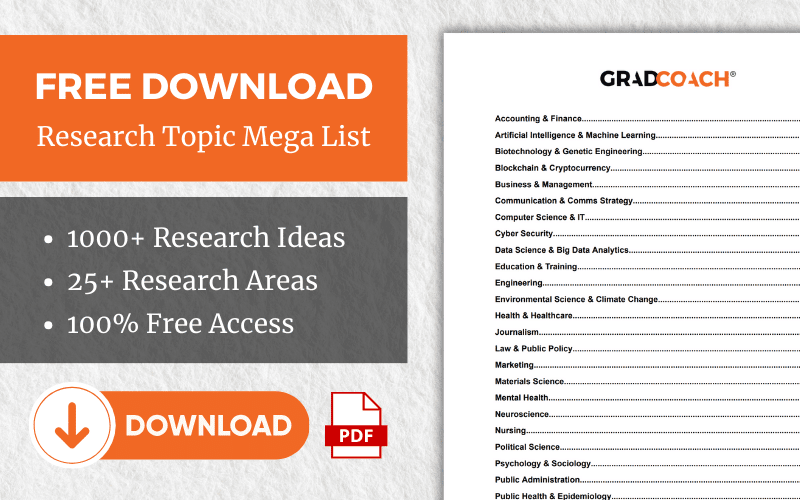
Cybersecurity-Related Research Topics
- Developing machine learning algorithms for early detection of cybersecurity threats.
- The use of artificial intelligence in optimizing network traffic for telecommunication companies.
- Investigating the impact of quantum computing on existing encryption methods.
- The application of blockchain technology in securing Internet of Things (IoT) devices.
- Developing efficient data mining techniques for large-scale social media analytics.
- The role of virtual reality in enhancing online education platforms.
- Investigating the effectiveness of various algorithms in reducing energy consumption in data centers.
- The impact of edge computing on the performance of mobile applications in remote areas.
- The application of computer vision techniques in automated medical diagnostics.
- Developing natural language processing tools for sentiment analysis in customer service.
- The use of augmented reality for training in high-risk industries like oil and gas.
- Investigating the challenges of integrating AI into legacy enterprise systems.
- The role of IT in managing supply chain disruptions during global crises.
- Developing adaptive cybersecurity strategies for small and medium-sized enterprises.
- The impact of 5G technology on the development of smart city solutions.
- The application of machine learning in personalized e-commerce recommendations.
- Investigating the use of cloud computing in improving government service delivery.
- The role of IT in enhancing sustainability in the manufacturing sector.
- Developing advanced algorithms for autonomous vehicle navigation.
- The application of biometrics in enhancing banking security systems.
- Investigating the ethical implications of facial recognition technology.
- The role of data analytics in optimizing healthcare delivery systems.
- Developing IoT solutions for efficient energy management in smart homes.
- The impact of mobile computing on the evolution of e-health services.
- The application of IT in disaster response and management.
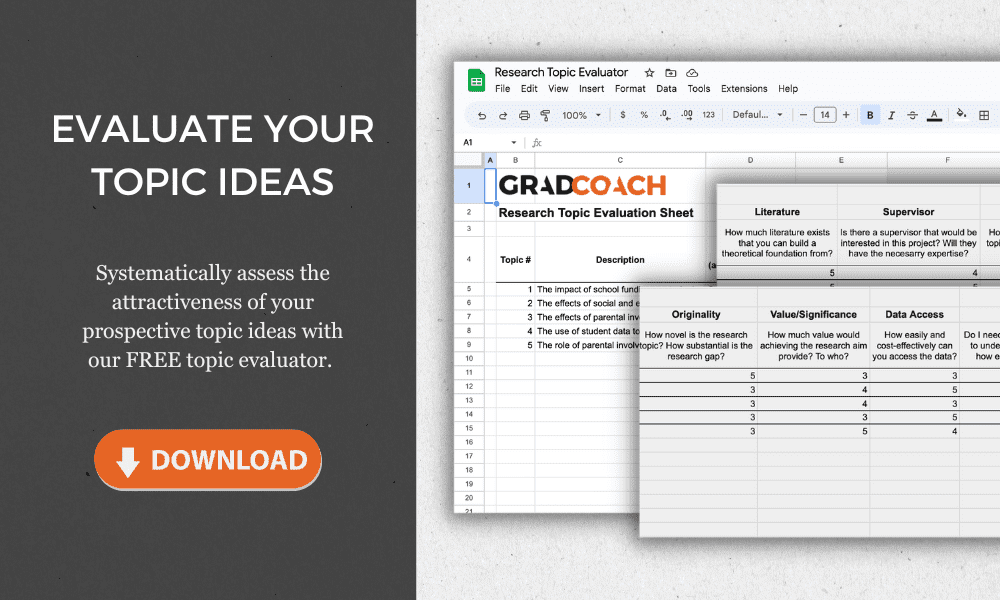
Cybersecurity Research Ideas (Continued)
- Assessing the security implications of quantum computing on modern encryption methods.
- The role of artificial intelligence in detecting and preventing phishing attacks.
- Blockchain technology in secure voting systems: opportunities and challenges.
- Cybersecurity strategies for protecting smart grids from targeted attacks.
- Developing a cyber incident response framework for small to medium-sized enterprises.
- The effectiveness of behavioural biometrics in preventing identity theft.
- Securing Internet of Things (IoT) devices in healthcare: risks and solutions.
- Analysis of cyber warfare tactics and their implications on national security.
- Exploring the ethical boundaries of offensive cybersecurity measures.
- Machine learning algorithms for predicting and mitigating DDoS attacks.
- Study of cryptocurrency-related cybercrimes: patterns and prevention strategies.
- Evaluating the impact of GDPR on data breach response strategies in the EU.
- Developing enhanced security protocols for mobile banking applications.
- An examination of cyber espionage tactics and countermeasures.
- The role of human error in cybersecurity breaches: a behavioural analysis.
- Investigating the use of deep fakes in cyber fraud: detection and prevention.
- Cloud computing security: managing risks in multi-tenant environments.
- Next-generation firewalls: evaluating performance and security features.
- The impact of 5G technology on cybersecurity strategies and policies.
- Secure coding practices: reducing vulnerabilities in software development.
- Assessing the role of cyber insurance in mitigating financial losses from cyber attacks.
- Implementing zero trust architecture in corporate networks: challenges and benefits.
- Ransomware attacks on critical infrastructure: case studies and defence strategies.
- Using big data analytics for proactive cyber threat intelligence.
- Evaluating the effectiveness of cybersecurity awareness training in organisations.
Recent Cybersecurity-Related Studies
While the ideas we’ve presented above are a decent starting point for finding a research topic, they are fairly generic and non-specific. So, it helps to look at actual studies in the cybersecurity space to see how this all comes together in practice.
Below, we’ve included a selection of recent studies to help refine your thinking. These are actual studies, so they can provide some useful insight as to what a research topic looks like in practice.
- Cyber Security Vulnerability Detection Using Natural Language Processing (Singh et al., 2022)
- Security for Cloud-Native Systems with an AI-Ops Engine (Ck et al., 2022)
- Overview of Cyber Security (Yadav, 2022)
- Exploring the Top Five Evolving Threats in Cybersecurity: An In-Depth Overview (Mijwil et al., 2023)
- Cyber Security: Strategy to Security Challenges A Review (Nistane & Sharma, 2022)
- A Review Paper on Cyber Security (K & Venkatesh, 2022)
- The Significance of Machine Learning and Deep Learning Techniques in Cybersecurity: A Comprehensive Review (Mijwil, 2023)
- Towards Artificial Intelligence-Based Cybersecurity: The Practices and ChatGPT Generated Ways to Combat Cybercrime (Mijwil et al., 2023)
- ESTABLISHING CYBERSECURITY AWARENESS OF TECHNICAL SECURITY MEASURES THROUGH A SERIOUS GAME (Harding et al., 2022)
- Efficiency Evaluation of Cyber Security Based on EBM-DEA Model (Nguyen et al., 2022)
- An Overview of the Present and Future of User Authentication (Al Kabir & Elmedany, 2022)
- Cybersecurity Enterprises Policies: A Comparative Study (Mishra et al., 2022)
- The Rise of Ransomware: A Review of Attacks, Detection Techniques, and Future Challenges (Kamil et al., 2022)
- On the scale of Cyberspace and Cybersecurity (Pathan, 2022)
- Analysis of techniques and attacking pattern in cyber security approach (Sharma et al., 2022)
- Impact of Artificial Intelligence on Information Security in Business (Alawadhi et al., 2022)
- Deployment of Artificial Intelligence with Bootstrapped Meta-Learning in Cyber Security (Sasikala & Sharma, 2022)
- Optimization of Secure Coding Practices in SDLC as Part of Cybersecurity Framework (Jakimoski et al., 2022)
- CySSS ’22: 1st International Workshop on Cybersecurity and Social Sciences (Chan-Tin & Kennison, 2022)
As you can see, these research topics are a lot more focused than the generic topic ideas we presented earlier. So, for you to develop a high-quality research topic, you’ll need to get specific and laser-focused on a specific context with specific variables of interest. In the video below, we explore some other important things you’ll need to consider when crafting your research topic.
Get 1-On-1 Help
If you’re still unsure about how to find a quality research topic, check out our Research Topic Kickstarter service, which is the perfect starting point for developing a unique, well-justified research topic.
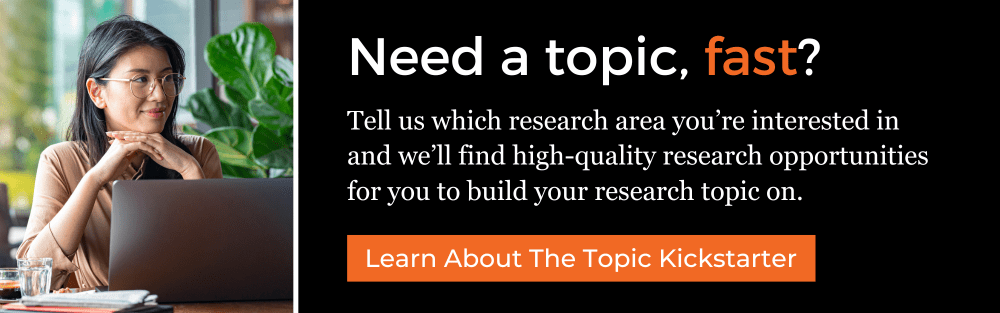
You Might Also Like:
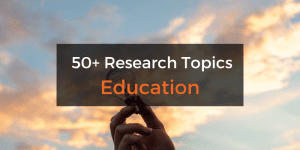
Submit a Comment Cancel reply
Your email address will not be published. Required fields are marked *
Save my name, email, and website in this browser for the next time I comment.
- Print Friendly
- Survey Paper
- Open access
- Published: 01 July 2020
Cybersecurity data science: an overview from machine learning perspective
- Iqbal H. Sarker ORCID: orcid.org/0000-0003-1740-5517 1 , 2 ,
- A. S. M. Kayes 3 ,
- Shahriar Badsha 4 ,
- Hamed Alqahtani 5 ,
- Paul Watters 3 &
- Alex Ng 3
Journal of Big Data volume 7 , Article number: 41 ( 2020 ) Cite this article
141k Accesses
238 Citations
51 Altmetric
Metrics details
In a computing context, cybersecurity is undergoing massive shifts in technology and its operations in recent days, and data science is driving the change. Extracting security incident patterns or insights from cybersecurity data and building corresponding data-driven model , is the key to make a security system automated and intelligent. To understand and analyze the actual phenomena with data, various scientific methods, machine learning techniques, processes, and systems are used, which is commonly known as data science. In this paper, we focus and briefly discuss on cybersecurity data science , where the data is being gathered from relevant cybersecurity sources, and the analytics complement the latest data-driven patterns for providing more effective security solutions. The concept of cybersecurity data science allows making the computing process more actionable and intelligent as compared to traditional ones in the domain of cybersecurity. We then discuss and summarize a number of associated research issues and future directions . Furthermore, we provide a machine learning based multi-layered framework for the purpose of cybersecurity modeling. Overall, our goal is not only to discuss cybersecurity data science and relevant methods but also to focus the applicability towards data-driven intelligent decision making for protecting the systems from cyber-attacks.
Introduction
Due to the increasing dependency on digitalization and Internet-of-Things (IoT) [ 1 ], various security incidents such as unauthorized access [ 2 ], malware attack [ 3 ], zero-day attack [ 4 ], data breach [ 5 ], denial of service (DoS) [ 2 ], social engineering or phishing [ 6 ] etc. have grown at an exponential rate in recent years. For instance, in 2010, there were less than 50 million unique malware executables known to the security community. By 2012, they were double around 100 million, and in 2019, there are more than 900 million malicious executables known to the security community, and this number is likely to grow, according to the statistics of AV-TEST institute in Germany [ 7 ]. Cybercrime and attacks can cause devastating financial losses and affect organizations and individuals as well. It’s estimated that, a data breach costs 8.19 million USD for the United States and 3.9 million USD on an average [ 8 ], and the annual cost to the global economy from cybercrime is 400 billion USD [ 9 ]. According to Juniper Research [ 10 ], the number of records breached each year to nearly triple over the next 5 years. Thus, it’s essential that organizations need to adopt and implement a strong cybersecurity approach to mitigate the loss. According to [ 11 ], the national security of a country depends on the business, government, and individual citizens having access to applications and tools which are highly secure, and the capability on detecting and eliminating such cyber-threats in a timely way. Therefore, to effectively identify various cyber incidents either previously seen or unseen, and intelligently protect the relevant systems from such cyber-attacks, is a key issue to be solved urgently.
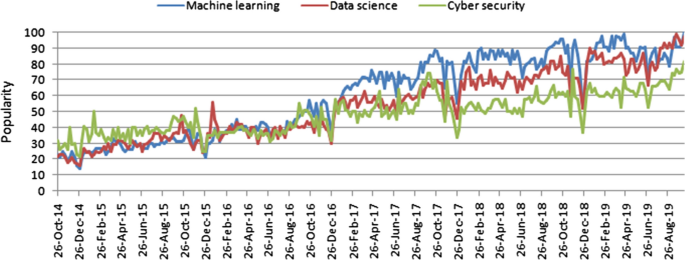
Popularity trends of data science, machine learning and cybersecurity over time, where x-axis represents the timestamp information and y axis represents the corresponding popularity values
Cybersecurity is a set of technologies and processes designed to protect computers, networks, programs and data from attack, damage, or unauthorized access [ 12 ]. In recent days, cybersecurity is undergoing massive shifts in technology and its operations in the context of computing, and data science (DS) is driving the change, where machine learning (ML), a core part of “Artificial Intelligence” (AI) can play a vital role to discover the insights from data. Machine learning can significantly change the cybersecurity landscape and data science is leading a new scientific paradigm [ 13 , 14 ]. The popularity of these related technologies is increasing day-by-day, which is shown in Fig. 1 , based on the data of the last five years collected from Google Trends [ 15 ]. The figure represents timestamp information in terms of a particular date in the x-axis and corresponding popularity in the range of 0 (minimum) to 100 (maximum) in the y-axis. As shown in Fig. 1 , the popularity indication values of these areas are less than 30 in 2014, while they exceed 70 in 2019, i.e., more than double in terms of increased popularity. In this paper, we focus on cybersecurity data science (CDS), which is broadly related to these areas in terms of security data processing techniques and intelligent decision making in real-world applications. Overall, CDS is security data-focused, applies machine learning methods to quantify cyber risks, and ultimately seeks to optimize cybersecurity operations. Thus, the purpose of this paper is for those academia and industry people who want to study and develop a data-driven smart cybersecurity model based on machine learning techniques. Therefore, great emphasis is placed on a thorough description of various types of machine learning methods, and their relations and usage in the context of cybersecurity. This paper does not describe all of the different techniques used in cybersecurity in detail; instead, it gives an overview of cybersecurity data science modeling based on artificial intelligence, particularly from machine learning perspective.
The ultimate goal of cybersecurity data science is data-driven intelligent decision making from security data for smart cybersecurity solutions. CDS represents a partial paradigm shift from traditional well-known security solutions such as firewalls, user authentication and access control, cryptography systems etc. that might not be effective according to today’s need in cyber industry [ 16 , 17 , 18 , 19 ]. The problems are these are typically handled statically by a few experienced security analysts, where data management is done in an ad-hoc manner [ 20 , 21 ]. However, as an increasing number of cybersecurity incidents in different formats mentioned above continuously appear over time, such conventional solutions have encountered limitations in mitigating such cyber risks. As a result, numerous advanced attacks are created and spread very quickly throughout the Internet. Although several researchers use various data analysis and learning techniques to build cybersecurity models that are summarized in “ Machine learning tasks in cybersecurity ” section, a comprehensive security model based on the effective discovery of security insights and latest security patterns could be more useful. To address this issue, we need to develop more flexible and efficient security mechanisms that can respond to threats and to update security policies to mitigate them intelligently in a timely manner. To achieve this goal, it is inherently required to analyze a massive amount of relevant cybersecurity data generated from various sources such as network and system sources, and to discover insights or proper security policies with minimal human intervention in an automated manner.
Analyzing cybersecurity data and building the right tools and processes to successfully protect against cybersecurity incidents goes beyond a simple set of functional requirements and knowledge about risks, threats or vulnerabilities. For effectively extracting the insights or the patterns of security incidents, several machine learning techniques, such as feature engineering, data clustering, classification, and association analysis, or neural network-based deep learning techniques can be used, which are briefly discussed in “ Machine learning tasks in cybersecurity ” section. These learning techniques are capable to find the anomalies or malicious behavior and data-driven patterns of associated security incidents to make an intelligent decision. Thus, based on the concept of data-driven decision making, we aim to focus on cybersecurity data science , where the data is being gathered from relevant cybersecurity sources such as network activity, database activity, application activity, or user activity, and the analytics complement the latest data-driven patterns for providing corresponding security solutions.
The contributions of this paper are summarized as follows.
We first make a brief discussion on the concept of cybersecurity data science and relevant methods to understand its applicability towards data-driven intelligent decision making in the domain of cybersecurity. For this purpose, we also make a review and brief discussion on different machine learning tasks in cybersecurity, and summarize various cybersecurity datasets highlighting their usage in different data-driven cyber applications.
We then discuss and summarize a number of associated research issues and future directions in the area of cybersecurity data science, that could help both the academia and industry people to further research and development in relevant application areas.
Finally, we provide a generic multi-layered framework of the cybersecurity data science model based on machine learning techniques. In this framework, we briefly discuss how the cybersecurity data science model can be used to discover useful insights from security data and making data-driven intelligent decisions to build smart cybersecurity systems.
The remainder of the paper is organized as follows. “ Background ” section summarizes background of our study and gives an overview of the related technologies of cybersecurity data science. “ Cybersecurity data science ” section defines and discusses briefly about cybersecurity data science including various categories of cyber incidents data. In “ Machine learning tasks in cybersecurity ” section, we briefly discuss various categories of machine learning techniques including their relations with cybersecurity tasks and summarize a number of machine learning based cybersecurity models in the field. “ Research issues and future directions ” section briefly discusses and highlights various research issues and future directions in the area of cybersecurity data science. In “ A multi-layered framework for smart cybersecurity services ” section, we suggest a machine learning-based framework to build cybersecurity data science model and discuss various layers with their roles. In “ Discussion ” section, we highlight several key points regarding our studies. Finally, “ Conclusion ” section concludes this paper.
In this section, we give an overview of the related technologies of cybersecurity data science including various types of cybersecurity incidents and defense strategies.
- Cybersecurity
Over the last half-century, the information and communication technology (ICT) industry has evolved greatly, which is ubiquitous and closely integrated with our modern society. Thus, protecting ICT systems and applications from cyber-attacks has been greatly concerned by the security policymakers in recent days [ 22 ]. The act of protecting ICT systems from various cyber-threats or attacks has come to be known as cybersecurity [ 9 ]. Several aspects are associated with cybersecurity: measures to protect information and communication technology; the raw data and information it contains and their processing and transmitting; associated virtual and physical elements of the systems; the degree of protection resulting from the application of those measures; and eventually the associated field of professional endeavor [ 23 ]. Craigen et al. defined “cybersecurity as a set of tools, practices, and guidelines that can be used to protect computer networks, software programs, and data from attack, damage, or unauthorized access” [ 24 ]. According to Aftergood et al. [ 12 ], “cybersecurity is a set of technologies and processes designed to protect computers, networks, programs and data from attacks and unauthorized access, alteration, or destruction”. Overall, cybersecurity concerns with the understanding of diverse cyber-attacks and devising corresponding defense strategies that preserve several properties defined as below [ 25 , 26 ].
Confidentiality is a property used to prevent the access and disclosure of information to unauthorized individuals, entities or systems.
Integrity is a property used to prevent any modification or destruction of information in an unauthorized manner.
Availability is a property used to ensure timely and reliable access of information assets and systems to an authorized entity.
The term cybersecurity applies in a variety of contexts, from business to mobile computing, and can be divided into several common categories. These are - network security that mainly focuses on securing a computer network from cyber attackers or intruders; application security that takes into account keeping the software and the devices free of risks or cyber-threats; information security that mainly considers security and the privacy of relevant data; operational security that includes the processes of handling and protecting data assets. Typical cybersecurity systems are composed of network security systems and computer security systems containing a firewall, antivirus software, or an intrusion detection system [ 27 ].
Cyberattacks and security risks
The risks typically associated with any attack, which considers three security factors, such as threats, i.e., who is attacking, vulnerabilities, i.e., the weaknesses they are attacking, and impacts, i.e., what the attack does [ 9 ]. A security incident is an act that threatens the confidentiality, integrity, or availability of information assets and systems. Several types of cybersecurity incidents that may result in security risks on an organization’s systems and networks or an individual [ 2 ]. These are:
Unauthorized access that describes the act of accessing information to network, systems or data without authorization that results in a violation of a security policy [ 2 ];
Malware known as malicious software, is any program or software that intentionally designed to cause damage to a computer, client, server, or computer network, e.g., botnets. Examples of different types of malware including computer viruses, worms, Trojan horses, adware, ransomware, spyware, malicious bots, etc. [ 3 , 26 ]; Ransom malware, or ransomware , is an emerging form of malware that prevents users from accessing their systems or personal files, or the devices, then demands an anonymous online payment in order to restore access.
Denial-of-Service is an attack meant to shut down a machine or network, making it inaccessible to its intended users by flooding the target with traffic that triggers a crash. The Denial-of-Service (DoS) attack typically uses one computer with an Internet connection, while distributed denial-of-service (DDoS) attack uses multiple computers and Internet connections to flood the targeted resource [ 2 ];
Phishing a type of social engineering , used for a broad range of malicious activities accomplished through human interactions, in which the fraudulent attempt takes part to obtain sensitive information such as banking and credit card details, login credentials, or personally identifiable information by disguising oneself as a trusted individual or entity via an electronic communication such as email, text, or instant message, etc. [ 26 ];
Zero-day attack is considered as the term that is used to describe the threat of an unknown security vulnerability for which either the patch has not been released or the application developers were unaware [ 4 , 28 ].
Beside these attacks mentioned above, privilege escalation [ 29 ], password attack [ 30 ], insider threat [ 31 ], man-in-the-middle [ 32 ], advanced persistent threat [ 33 ], SQL injection attack [ 34 ], cryptojacking attack [ 35 ], web application attack [ 30 ] etc. are well-known as security incidents in the field of cybersecurity. A data breach is another type of security incident, known as a data leak, which is involved in the unauthorized access of data by an individual, application, or service [ 5 ]. Thus, all data breaches are considered as security incidents, however, all the security incidents are not data breaches. Most data breaches occur in the banking industry involving the credit card numbers, personal information, followed by the healthcare sector and the public sector [ 36 ].
Cybersecurity defense strategies
Defense strategies are needed to protect data or information, information systems, and networks from cyber-attacks or intrusions. More granularly, they are responsible for preventing data breaches or security incidents and monitoring and reacting to intrusions, which can be defined as any kind of unauthorized activity that causes damage to an information system [ 37 ]. An intrusion detection system (IDS) is typically represented as “a device or software application that monitors a computer network or systems for malicious activity or policy violations” [ 38 ]. The traditional well-known security solutions such as anti-virus, firewalls, user authentication, access control, data encryption and cryptography systems, however might not be effective according to today’s need in the cyber industry
[ 16 , 17 , 18 , 19 ]. On the other hand, IDS resolves the issues by analyzing security data from several key points in a computer network or system [ 39 , 40 ]. Moreover, intrusion detection systems can be used to detect both internal and external attacks.
Intrusion detection systems are different categories according to the usage scope. For instance, a host-based intrusion detection system (HIDS), and network intrusion detection system (NIDS) are the most common types based on the scope of single computers to large networks. In a HIDS, the system monitors important files on an individual system, while it analyzes and monitors network connections for suspicious traffic in a NIDS. Similarly, based on methodologies, the signature-based IDS, and anomaly-based IDS are the most well-known variants [ 37 ].
Signature-based IDS : A signature can be a predefined string, pattern, or rule that corresponds to a known attack. A particular pattern is identified as the detection of corresponding attacks in a signature-based IDS. An example of a signature can be known patterns or a byte sequence in a network traffic, or sequences used by malware. To detect the attacks, anti-virus software uses such types of sequences or patterns as a signature while performing the matching operation. Signature-based IDS is also known as knowledge-based or misuse detection [ 41 ]. This technique can be efficient to process a high volume of network traffic, however, is strictly limited to the known attacks only. Thus, detecting new attacks or unseen attacks is one of the biggest challenges faced by this signature-based system.
Anomaly-based IDS : The concept of anomaly-based detection overcomes the issues of signature-based IDS discussed above. In an anomaly-based intrusion detection system, the behavior of the network is first examined to find dynamic patterns, to automatically create a data-driven model, to profile the normal behavior, and thus it detects deviations in the case of any anomalies [ 41 ]. Thus, anomaly-based IDS can be treated as a dynamic approach, which follows behavior-oriented detection. The main advantage of anomaly-based IDS is the ability to identify unknown or zero-day attacks [ 42 ]. However, the issue is that the identified anomaly or abnormal behavior is not always an indicator of intrusions. It sometimes may happen because of several factors such as policy changes or offering a new service.
In addition, a hybrid detection approach [ 43 , 44 ] that takes into account both the misuse and anomaly-based techniques discussed above can be used to detect intrusions. In a hybrid system, the misuse detection system is used for detecting known types of intrusions and anomaly detection system is used for novel attacks [ 45 ]. Beside these approaches, stateful protocol analysis can also be used to detect intrusions that identifies deviations of protocol state similarly to the anomaly-based method, however it uses predetermined universal profiles based on accepted definitions of benign activity [ 41 ]. In Table 1 , we have summarized these common approaches highlighting their pros and cons. Once the detecting has been completed, the intrusion prevention system (IPS) that is intended to prevent malicious events, can be used to mitigate the risks in different ways such as manual, providing notification, or automatic process [ 46 ]. Among these approaches, an automatic response system could be more effective as it does not involve a human interface between the detection and response systems.
- Data science
We are living in the age of data, advanced analytics, and data science, which are related to data-driven intelligent decision making. Although, the process of searching patterns or discovering hidden and interesting knowledge from data is known as data mining [ 47 ], in this paper, we use the broader term “data science” rather than data mining. The reason is that, data science, in its most fundamental form, is all about understanding of data. It involves studying, processing, and extracting valuable insights from a set of information. In addition to data mining, data analytics is also related to data science. The development of data mining, knowledge discovery, and machine learning that refers creating algorithms and program which learn on their own, together with the original data analysis and descriptive analytics from the statistical perspective, forms the general concept of “data analytics” [ 47 ]. Nowadays, many researchers use the term “data science” to describe the interdisciplinary field of data collection, preprocessing, inferring, or making decisions by analyzing the data. To understand and analyze the actual phenomena with data, various scientific methods, machine learning techniques, processes, and systems are used, which is commonly known as data science. According to Cao et al. [ 47 ] “data science is a new interdisciplinary field that synthesizes and builds on statistics, informatics, computing, communication, management, and sociology to study data and its environments, to transform data to insights and decisions by following a data-to-knowledge-to-wisdom thinking and methodology”. As a high-level statement in the context of cybersecurity, we can conclude that it is the study of security data to provide data-driven solutions for the given security problems, as known as “the science of cybersecurity data”. Figure 2 shows the typical data-to-insight-to-decision transfer at different periods and general analytic stages in data science, in terms of a variety of analytics goals (G) and approaches (A) to achieve the data-to-decision goal [ 47 ].
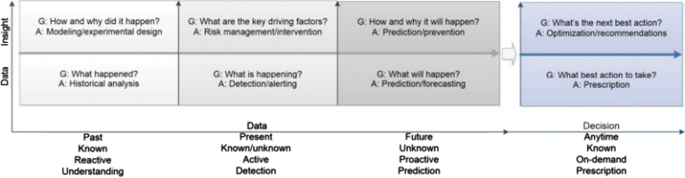
Data-to-insight-to-decision analytic stages in data science [ 47 ]
Based on the analytic power of data science including machine learning techniques, it can be a viable component of security strategies. By using data science techniques, security analysts can manipulate and analyze security data more effectively and efficiently, uncovering valuable insights from data. Thus, data science methodologies including machine learning techniques can be well utilized in the context of cybersecurity, in terms of problem understanding, gathering security data from diverse sources, preparing data to feed into the model, data-driven model building and updating, for providing smart security services, which motivates to define cybersecurity data science and to work in this research area.
Cybersecurity data science
In this section, we briefly discuss cybersecurity data science including various categories of cyber incidents data with the usage in different application areas, and the key terms and areas related to our study.
Understanding cybersecurity data
Data science is largely driven by the availability of data [ 48 ]. Datasets typically represent a collection of information records that consist of several attributes or features and related facts, in which cybersecurity data science is based on. Thus, it’s important to understand the nature of cybersecurity data containing various types of cyberattacks and relevant features. The reason is that raw security data collected from relevant cyber sources can be used to analyze the various patterns of security incidents or malicious behavior, to build a data-driven security model to achieve our goal. Several datasets exist in the area of cybersecurity including intrusion analysis, malware analysis, anomaly, fraud, or spam analysis that are used for various purposes. In Table 2 , we summarize several such datasets including their various features and attacks that are accessible on the Internet, and highlight their usage based on machine learning techniques in different cyber applications. Effectively analyzing and processing of these security features, building target machine learning-based security model according to the requirements, and eventually, data-driven decision making, could play a role to provide intelligent cybersecurity services that are discussed briefly in “ A multi-layered framework for smart cybersecurity services ” section.
Defining cybersecurity data science
Data science is transforming the world’s industries. It is critically important for the future of intelligent cybersecurity systems and services because of “security is all about data”. When we seek to detect cyber threats, we are analyzing the security data in the form of files, logs, network packets, or other relevant sources. Traditionally, security professionals didn’t use data science techniques to make detections based on these data sources. Instead, they used file hashes, custom-written rules like signatures, or manually defined heuristics [ 21 ]. Although these techniques have their own merits in several cases, it needs too much manual work to keep up with the changing cyber threat landscape. On the contrary, data science can make a massive shift in technology and its operations, where machine learning algorithms can be used to learn or extract insight of security incident patterns from the training data for their detection and prevention. For instance, to detect malware or suspicious trends, or to extract policy rules, these techniques can be used.
In recent days, the entire security industry is moving towards data science, because of its capability to transform raw data into decision making. To do this, several data-driven tasks can be associated, such as—(i) data engineering focusing practical applications of data gathering and analysis; (ii) reducing data volume that deals with filtering significant and relevant data to further analysis; (iii) discovery and detection that focuses on extracting insight or incident patterns or knowledge from data; (iv) automated models that focus on building data-driven intelligent security model; (v) targeted security alerts focusing on the generation of remarkable security alerts based on discovered knowledge that minimizes the false alerts, and (vi) resource optimization that deals with the available resources to achieve the target goals in a security system. While making data-driven decisions, behavioral analysis could also play a significant role in the domain of cybersecurity [ 81 ].
Thus, the concept of cybersecurity data science incorporates the methods and techniques of data science and machine learning as well as the behavioral analytics of various security incidents. The combination of these technologies has given birth to the term “cybersecurity data science”, which refers to collect a large amount of security event data from different sources and analyze it using machine learning technologies for detecting security risks or attacks either through the discovery of useful insights or the latest data-driven patterns. It is, however, worth remembering that cybersecurity data science is not just about a collection of machine learning algorithms, rather, a process that can help security professionals or analysts to scale and automate their security activities in a smart way and in a timely manner. Therefore, the formal definition can be as follows: “Cybersecurity data science is a research or working area existing at the intersection of cybersecurity, data science, and machine learning or artificial intelligence, which is mainly security data-focused, applies machine learning methods, attempts to quantify cyber-risks or incidents, and promotes inferential techniques to analyze behavioral patterns in security data. It also focuses on generating security response alerts, and eventually seeks for optimizing cybersecurity solutions, to build automated and intelligent cybersecurity systems.”
Table 3 highlights some key terms associated with cybersecurity data science. Overall, the outputs of cybersecurity data science are typically security data products, which can be a data-driven security model, policy rule discovery, risk or attack prediction, potential security service and recommendation, or the corresponding security system depending on the given security problem in the domain of cybersecurity. In the next section, we briefly discuss various machine learning tasks with examples within the scope of our study.
Machine learning tasks in cybersecurity
Machine learning (ML) is typically considered as a branch of “Artificial Intelligence”, which is closely related to computational statistics, data mining and analytics, data science, particularly focusing on making the computers to learn from data [ 82 , 83 ]. Thus, machine learning models typically comprise of a set of rules, methods, or complex “transfer functions” that can be applied to find interesting data patterns, or to recognize or predict behavior [ 84 ], which could play an important role in the area of cybersecurity. In the following, we discuss different methods that can be used to solve machine learning tasks and how they are related to cybersecurity tasks.
Supervised learning
Supervised learning is performed when specific targets are defined to reach from a certain set of inputs, i.e., task-driven approach. In the area of machine learning, the most popular supervised learning techniques are known as classification and regression methods [ 129 ]. These techniques are popular to classify or predict the future for a particular security problem. For instance, to predict denial-of-service attack (yes, no) or to identify different classes of network attacks such as scanning and spoofing, classification techniques can be used in the cybersecurity domain. ZeroR [ 83 ], OneR [ 130 ], Navies Bayes [ 131 ], Decision Tree [ 132 , 133 ], K-nearest neighbors [ 134 ], support vector machines [ 135 ], adaptive boosting [ 136 ], and logistic regression [ 137 ] are the well-known classification techniques. In addition, recently Sarker et al. have proposed BehavDT [ 133 ], and IntruDtree [ 106 ] classification techniques that are able to effectively build a data-driven predictive model. On the other hand, to predict the continuous or numeric value, e.g., total phishing attacks in a certain period or predicting the network packet parameters, regression techniques are useful. Regression analyses can also be used to detect the root causes of cybercrime and other types of fraud [ 138 ]. Linear regression [ 82 ], support vector regression [ 135 ] are the popular regression techniques. The main difference between classification and regression is that the output variable in the regression is numerical or continuous, while the predicted output for classification is categorical or discrete. Ensemble learning is an extension of supervised learning while mixing different simple models, e.g., Random Forest learning [ 139 ] that generates multiple decision trees to solve a particular security task.
Unsupervised learning
In unsupervised learning problems, the main task is to find patterns, structures, or knowledge in unlabeled data, i.e., data-driven approach [ 140 ]. In the area of cybersecurity, cyber-attacks like malware stays hidden in some ways, include changing their behavior dynamically and autonomously to avoid detection. Clustering techniques, a type of unsupervised learning, can help to uncover the hidden patterns and structures from the datasets, to identify indicators of such sophisticated attacks. Similarly, in identifying anomalies, policy violations, detecting, and eliminating noisy instances in data, clustering techniques can be useful. K-means [ 141 ], K-medoids [ 142 ] are the popular partitioning clustering algorithms, and single linkage [ 143 ] or complete linkage [ 144 ] are the well-known hierarchical clustering algorithms used in various application domains. Moreover, a bottom-up clustering approach proposed by Sarker et al. [ 145 ] can also be used by taking into account the data characteristics.
Besides, feature engineering tasks like optimal feature selection or extraction related to a particular security problem could be useful for further analysis [ 106 ]. Recently, Sarker et al. [ 106 ] have proposed an approach for selecting security features according to their importance score values. Moreover, Principal component analysis, linear discriminant analysis, pearson correlation analysis, or non-negative matrix factorization are the popular dimensionality reduction techniques to solve such issues [ 82 ]. Association rule learning is another example, where machine learning based policy rules can prevent cyber-attacks. In an expert system, the rules are usually manually defined by a knowledge engineer working in collaboration with a domain expert [ 37 , 140 , 146 ]. Association rule learning on the contrary, is the discovery of rules or relationships among a set of available security features or attributes in a given dataset [ 147 ]. To quantify the strength of relationships, correlation analysis can be used [ 138 ]. Many association rule mining algorithms have been proposed in the area of machine learning and data mining literature, such as logic-based [ 148 ], frequent pattern based [ 149 , 150 , 151 ], tree-based [ 152 ], etc. Recently, Sarker et al. [ 153 ] have proposed an association rule learning approach considering non-redundant generation, that can be used to discover a set of useful security policy rules. Moreover, AIS [ 147 ], Apriori [ 149 ], Apriori-TID and Apriori-Hybrid [ 149 ], FP-Tree [ 152 ], and RARM [ 154 ], and Eclat [ 155 ] are the well-known association rule learning algorithms that are capable to solve such problems by generating a set of policy rules in the domain of cybersecurity.
Neural networks and deep learning
Deep learning is a part of machine learning in the area of artificial intelligence, which is a computational model that is inspired by the biological neural networks in the human brain [ 82 ]. Artificial Neural Network (ANN) is frequently used in deep learning and the most popular neural network algorithm is backpropagation [ 82 ]. It performs learning on a multi-layer feed-forward neural network consists of an input layer, one or more hidden layers, and an output layer. The main difference between deep learning and classical machine learning is its performance on the amount of security data increases. Typically deep learning algorithms perform well when the data volumes are large, whereas machine learning algorithms perform comparatively better on small datasets [ 44 ]. In our earlier work, Sarker et al. [ 129 ], we have illustrated the effectiveness of these approaches considering contextual datasets. However, deep learning approaches mimic the human brain mechanism to interpret large amount of data or the complex data such as images, sounds and texts [ 44 , 129 ]. In terms of feature extraction to build models, deep learning reduces the effort of designing a feature extractor for each problem than the classical machine learning techniques. Beside these characteristics, deep learning typically takes a long time to train an algorithm than a machine learning algorithm, however, the test time is exactly the opposite [ 44 ]. Thus, deep learning relies more on high-performance machines with GPUs than classical machine-learning algorithms [ 44 , 156 ]. The most popular deep neural network learning models include multi-layer perceptron (MLP) [ 157 ], convolutional neural network (CNN) [ 158 ], recurrent neural network (RNN) or long-short term memory (LSTM) network [ 121 , 158 ]. In recent days, researchers use these deep learning techniques for different purposes such as detecting network intrusions, malware traffic detection and classification, etc. in the domain of cybersecurity [ 44 , 159 ].
Other learning techniques
Semi-supervised learning can be described as a hybridization of supervised and unsupervised techniques discussed above, as it works on both the labeled and unlabeled data. In the area of cybersecurity, it could be useful, when it requires to label data automatically without human intervention, to improve the performance of cybersecurity models. Reinforcement techniques are another type of machine learning that characterizes an agent by creating its own learning experiences through interacting directly with the environment, i.e., environment-driven approach, where the environment is typically formulated as a Markov decision process and take decision based on a reward function [ 160 ]. Monte Carlo learning, Q-learning, Deep Q Networks, are the most common reinforcement learning algorithms [ 161 ]. For instance, in a recent work [ 126 ], the authors present an approach for detecting botnet traffic or malicious cyber activities using reinforcement learning combining with neural network classifier. In another work [ 128 ], the authors discuss about the application of deep reinforcement learning to intrusion detection for supervised problems, where they received the best results for the Deep Q-Network algorithm. In the context of cybersecurity, genetic algorithms that use fitness, selection, crossover, and mutation for finding optimization, could also be used to solve a similar class of learning problems [ 119 ].
Various types of machine learning techniques discussed above can be useful in the domain of cybersecurity, to build an effective security model. In Table 4 , we have summarized several machine learning techniques that are used to build various types of security models for various purposes. Although these models typically represent a learning-based security model, in this paper, we aim to focus on a comprehensive cybersecurity data science model and relevant issues, in order to build a data-driven intelligent security system. In the next section, we highlight several research issues and potential solutions in the area of cybersecurity data science.
Research issues and future directions
Our study opens several research issues and challenges in the area of cybersecurity data science to extract insight from relevant data towards data-driven intelligent decision making for cybersecurity solutions. In the following, we summarize these challenges ranging from data collection to decision making.
Cybersecurity datasets : Source datasets are the primary component to work in the area of cybersecurity data science. Most of the existing datasets are old and might insufficient in terms of understanding the recent behavioral patterns of various cyber-attacks. Although the data can be transformed into a meaningful understanding level after performing several processing tasks, there is still a lack of understanding of the characteristics of recent attacks and their patterns of happening. Thus, further processing or machine learning algorithms may provide a low accuracy rate for making the target decisions. Therefore, establishing a large number of recent datasets for a particular problem domain like cyber risk prediction or intrusion detection is needed, which could be one of the major challenges in cybersecurity data science.
Handling quality problems in cybersecurity datasets : The cyber datasets might be noisy, incomplete, insignificant, imbalanced, or may contain inconsistency instances related to a particular security incident. Such problems in a data set may affect the quality of the learning process and degrade the performance of the machine learning-based models [ 162 ]. To make a data-driven intelligent decision for cybersecurity solutions, such problems in data is needed to deal effectively before building the cyber models. Therefore, understanding such problems in cyber data and effectively handling such problems using existing algorithms or newly proposed algorithm for a particular problem domain like malware analysis or intrusion detection and prevention is needed, which could be another research issue in cybersecurity data science.
Security policy rule generation : Security policy rules reference security zones and enable a user to allow, restrict, and track traffic on the network based on the corresponding user or user group, and service, or the application. The policy rules including the general and more specific rules are compared against the incoming traffic in sequence during the execution, and the rule that matches the traffic is applied. The policy rules used in most of the cybersecurity systems are static and generated by human expertise or ontology-based [ 163 , 164 ]. Although, association rule learning techniques produce rules from data, however, there is a problem of redundancy generation [ 153 ] that makes the policy rule-set complex. Therefore, understanding such problems in policy rule generation and effectively handling such problems using existing algorithms or newly proposed algorithm for a particular problem domain like access control [ 165 ] is needed, which could be another research issue in cybersecurity data science.
Hybrid learning method : Most commercial products in the cybersecurity domain contain signature-based intrusion detection techniques [ 41 ]. However, missing features or insufficient profiling can cause these techniques to miss unknown attacks. In that case, anomaly-based detection techniques or hybrid technique combining signature-based and anomaly-based can be used to overcome such issues. A hybrid technique combining multiple learning techniques or a combination of deep learning and machine-learning methods can be used to extract the target insight for a particular problem domain like intrusion detection, malware analysis, access control, etc. and make the intelligent decision for corresponding cybersecurity solutions.
Protecting the valuable security information : Another issue of a cyber data attack is the loss of extremely valuable data and information, which could be damaging for an organization. With the use of encryption or highly complex signatures, one can stop others from probing into a dataset. In such cases, cybersecurity data science can be used to build a data-driven impenetrable protocol to protect such security information. To achieve this goal, cyber analysts can develop algorithms by analyzing the history of cyberattacks to detect the most frequently targeted chunks of data. Thus, understanding such data protecting problems and designing corresponding algorithms to effectively handling these problems, could be another research issue in the area of cybersecurity data science.
Context-awareness in cybersecurity : Existing cybersecurity work mainly originates from the relevant cyber data containing several low-level features. When data mining and machine learning techniques are applied to such datasets, a related pattern can be identified that describes it properly. However, a broader contextual information [ 140 , 145 , 166 ] like temporal, spatial, relationship among events or connections, dependency can be used to decide whether there exists a suspicious activity or not. For instance, some approaches may consider individual connections as DoS attacks, while security experts might not treat them as malicious by themselves. Thus, a significant limitation of existing cybersecurity work is the lack of using the contextual information for predicting risks or attacks. Therefore, context-aware adaptive cybersecurity solutions could be another research issue in cybersecurity data science.
Feature engineering in cybersecurity : The efficiency and effectiveness of a machine learning-based security model has always been a major challenge due to the high volume of network data with a large number of traffic features. The large dimensionality of data has been addressed using several techniques such as principal component analysis (PCA) [ 167 ], singular value decomposition (SVD) [ 168 ] etc. In addition to low-level features in the datasets, the contextual relationships between suspicious activities might be relevant. Such contextual data can be stored in an ontology or taxonomy for further processing. Thus how to effectively select the optimal features or extract the significant features considering both the low-level features as well as the contextual features, for effective cybersecurity solutions could be another research issue in cybersecurity data science.
Remarkable security alert generation and prioritizing : In many cases, the cybersecurity system may not be well defined and may cause a substantial number of false alarms that are unexpected in an intelligent system. For instance, an IDS deployed in a real-world network generates around nine million alerts per day [ 169 ]. A network-based intrusion detection system typically looks at the incoming traffic for matching the associated patterns to detect risks, threats or vulnerabilities and generate security alerts. However, to respond to each such alert might not be effective as it consumes relatively huge amounts of time and resources, and consequently may result in a self-inflicted DoS. To overcome this problem, a high-level management is required that correlate the security alerts considering the current context and their logical relationship including their prioritization before reporting them to users, which could be another research issue in cybersecurity data science.
Recency analysis in cybersecurity solutions : Machine learning-based security models typically use a large amount of static data to generate data-driven decisions. Anomaly detection systems rely on constructing such a model considering normal behavior and anomaly, according to their patterns. However, normal behavior in a large and dynamic security system is not well defined and it may change over time, which can be considered as an incremental growing of dataset. The patterns in incremental datasets might be changed in several cases. This often results in a substantial number of false alarms known as false positives. Thus, a recent malicious behavioral pattern is more likely to be interesting and significant than older ones for predicting unknown attacks. Therefore, effectively using the concept of recency analysis [ 170 ] in cybersecurity solutions could be another issue in cybersecurity data science.
The most important work for an intelligent cybersecurity system is to develop an effective framework that supports data-driven decision making. In such a framework, we need to consider advanced data analysis based on machine learning techniques, so that the framework is capable to minimize these issues and to provide automated and intelligent security services. Thus, a well-designed security framework for cybersecurity data and the experimental evaluation is a very important direction and a big challenge as well. In the next section, we suggest and discuss a data-driven cybersecurity framework based on machine learning techniques considering multiple processing layers.
A multi-layered framework for smart cybersecurity services
As discussed earlier, cybersecurity data science is data-focused, applies machine learning methods, attempts to quantify cyber risks, promotes inferential techniques to analyze behavioral patterns, focuses on generating security response alerts, and eventually seeks for optimizing cybersecurity operations. Hence, we briefly discuss a multiple data processing layered framework that potentially can be used to discover security insights from the raw data to build smart cybersecurity systems, e.g., dynamic policy rule-based access control or intrusion detection and prevention system. To make a data-driven intelligent decision in the resultant cybersecurity system, understanding the security problems and the nature of corresponding security data and their vast analysis is needed. For this purpose, our suggested framework not only considers the machine learning techniques to build the security model but also takes into account the incremental learning and dynamism to keep the model up-to-date and corresponding response generation, which could be more effective and intelligent for providing the expected services. Figure 3 shows an overview of the framework, involving several processing layers, from raw security event data to services. In the following, we briefly discuss the working procedure of the framework.
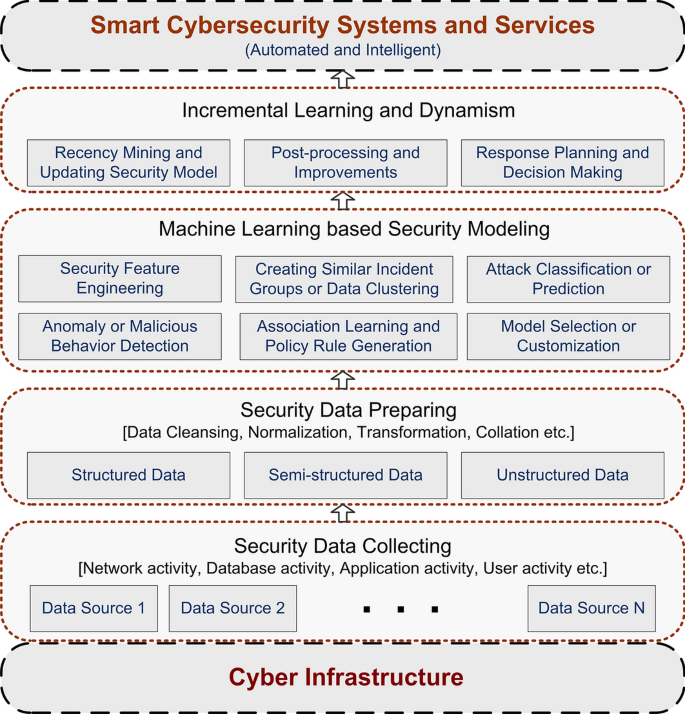
A generic multi-layered framework based on machine learning techniques for smart cybersecurity services
Security data collecting
Collecting valuable cybersecurity data is a crucial step, which forms a connecting link between security problems in cyberinfrastructure and corresponding data-driven solution steps in this framework, shown in Fig. 3 . The reason is that cyber data can serve as the source for setting up ground truth of the security model that affect the model performance. The quality and quantity of cyber data decide the feasibility and effectiveness of solving the security problem according to our goal. Thus, the concern is how to collect valuable and unique needs data for building the data-driven security models.
The general step to collect and manage security data from diverse data sources is based on a particular security problem and project within the enterprise. Data sources can be classified into several broad categories such as network, host, and hybrid [ 171 ]. Within the network infrastructure, the security system can leverage different types of security data such as IDS logs, firewall logs, network traffic data, packet data, and honeypot data, etc. for providing the target security services. For instance, a given IP is considered malicious or not, could be detected by performing data analysis utilizing the data of IP addresses and their cyber activities. In the domain of cybersecurity, the network source mentioned above is considered as the primary security event source to analyze. In the host category, it collects data from an organization’s host machines, where the data sources can be operating system logs, database access logs, web server logs, email logs, application logs, etc. Collecting data from both the network and host machines are considered a hybrid category. Overall, in a data collection layer the network activity, database activity, application activity, and user activity can be the possible security event sources in the context of cybersecurity data science.
Security data preparing
After collecting the raw security data from various sources according to the problem domain discussed above, this layer is responsible to prepare the raw data for building the model by applying various necessary processes. However, not all of the collected data contributes to the model building process in the domain of cybersecurity [ 172 ]. Therefore, the useless data should be removed from the rest of the data captured by the network sniffer. Moreover, data might be noisy, have missing or corrupted values, or have attributes of widely varying types and scales. High quality of data is necessary for achieving higher accuracy in a data-driven model, which is a process of learning a function that maps an input to an output based on example input-output pairs. Thus, it might require a procedure for data cleaning, handling missing or corrupted values. Moreover, security data features or attributes can be in different types, such as continuous, discrete, or symbolic [ 106 ]. Beyond a solid understanding of these types of data and attributes and their permissible operations, its need to preprocess the data and attributes to convert into the target type. Besides, the raw data can be in different types such as structured, semi-structured, or unstructured, etc. Thus, normalization, transformation, or collation can be useful to organize the data in a structured manner. In some cases, natural language processing techniques might be useful depending on data type and characteristics, e.g., textual contents. As both the quality and quantity of data decide the feasibility of solving the security problem, effectively pre-processing and management of data and their representation can play a significant role to build an effective security model for intelligent services.
Machine learning-based security modeling
This is the core step where insights and knowledge are extracted from data through the application of cybersecurity data science. In this section, we particularly focus on machine learning-based modeling as machine learning techniques can significantly change the cybersecurity landscape. The security features or attributes and their patterns in data are of high interest to be discovered and analyzed to extract security insights. To achieve the goal, a deeper understanding of data and machine learning-based analytical models utilizing a large number of cybersecurity data can be effective. Thus, various machine learning tasks can be involved in this model building layer according to the solution perspective. These are - security feature engineering that mainly responsible to transform raw security data into informative features that effectively represent the underlying security problem to the data-driven models. Thus, several data-processing tasks such as feature transformation and normalization, feature selection by taking into account a subset of available security features according to their correlations or importance in modeling, or feature generation and extraction by creating new brand principal components, may be involved in this module according to the security data characteristics. For instance, the chi-squared test, analysis of variance test, correlation coefficient analysis, feature importance, as well as discriminant and principal component analysis, or singular value decomposition, etc. can be used for analyzing the significance of the security features to perform the security feature engineering tasks [ 82 ].
Another significant module is security data clustering that uncovers hidden patterns and structures through huge volumes of security data, to identify where the new threats exist. It typically involves the grouping of security data with similar characteristics, which can be used to solve several cybersecurity problems such as detecting anomalies, policy violations, etc. Malicious behavior or anomaly detection module is typically responsible to identify a deviation to a known behavior, where clustering-based analysis and techniques can also be used to detect malicious behavior or anomaly detection. In the cybersecurity area, attack classification or prediction is treated as one of the most significant modules, which is responsible to build a prediction model to classify attacks or threats and to predict future for a particular security problem. To predict denial-of-service attack or a spam filter separating tasks from other messages, could be the relevant examples. Association learning or policy rule generation module can play a role to build an expert security system that comprises several IF-THEN rules that define attacks. Thus, in a problem of policy rule generation for rule-based access control system, association learning can be used as it discovers the associations or relationships among a set of available security features in a given security dataset. The popular machine learning algorithms in these categories are briefly discussed in “ Machine learning tasks in cybersecurity ” section. The module model selection or customization is responsible to choose whether it uses the existing machine learning model or needed to customize. Analyzing data and building models based on traditional machine learning or deep learning methods, could achieve acceptable results in certain cases in the domain of cybersecurity. However, in terms of effectiveness and efficiency or other performance measurements considering time complexity, generalization capacity, and most importantly the impact of the algorithm on the detection rate of a system, machine learning models are needed to customize for a specific security problem. Moreover, customizing the related techniques and data could improve the performance of the resultant security model and make it better applicable in a cybersecurity domain. The modules discussed above can work separately and combinedly depending on the target security problems.
Incremental learning and dynamism
In our framework, this layer is concerned with finalizing the resultant security model by incorporating additional intelligence according to the needs. This could be possible by further processing in several modules. For instance, the post-processing and improvement module in this layer could play a role to simplify the extracted knowledge according to the particular requirements by incorporating domain-specific knowledge. As the attack classification or prediction models based on machine learning techniques strongly rely on the training data, it can hardly be generalized to other datasets, which could be significant for some applications. To address such kind of limitations, this module is responsible to utilize the domain knowledge in the form of taxonomy or ontology to improve attack correlation in cybersecurity applications.
Another significant module recency mining and updating security model is responsible to keep the security model up-to-date for better performance by extracting the latest data-driven security patterns. The extracted knowledge discussed in the earlier layer is based on a static initial dataset considering the overall patterns in the datasets. However, such knowledge might not be guaranteed higher performance in several cases, because of incremental security data with recent patterns. In many cases, such incremental data may contain different patterns which could conflict with existing knowledge. Thus, the concept of RecencyMiner [ 170 ] on incremental security data and extracting new patterns can be more effective than the existing old patterns. The reason is that recent security patterns and rules are more likely to be significant than older ones for predicting cyber risks or attacks. Rather than processing the whole security data again, recency-based dynamic updating according to the new patterns would be more efficient in terms of processing and outcome. This could make the resultant cybersecurity model intelligent and dynamic. Finally, response planning and decision making module is responsible to make decisions based on the extracted insights and take necessary actions to prevent the system from the cyber-attacks to provide automated and intelligent services. The services might be different depending on particular requirements for a given security problem.
Overall, this framework is a generic description which potentially can be used to discover useful insights from security data, to build smart cybersecurity systems, to address complex security challenges, such as intrusion detection, access control management, detecting anomalies and fraud, or denial of service attacks, etc. in the area of cybersecurity data science.
Although several research efforts have been directed towards cybersecurity solutions, discussed in “ Background ” , “ Cybersecurity data science ”, and “ Machine learning tasks in cybersecurity ” sections in different directions, this paper presents a comprehensive view of cybersecurity data science. For this, we have conducted a literature review to understand cybersecurity data, various defense strategies including intrusion detection techniques, different types of machine learning techniques in cybersecurity tasks. Based on our discussion on existing work, several research issues related to security datasets, data quality problems, policy rule generation, learning methods, data protection, feature engineering, security alert generation, recency analysis etc. are identified that require further research attention in the domain of cybersecurity data science.
The scope of cybersecurity data science is broad. Several data-driven tasks such as intrusion detection and prevention, access control management, security policy generation, anomaly detection, spam filtering, fraud detection and prevention, various types of malware attack detection and defense strategies, etc. can be considered as the scope of cybersecurity data science. Such tasks based categorization could be helpful for security professionals including the researchers and practitioners who are interested in the domain-specific aspects of security systems [ 171 ]. The output of cybersecurity data science can be used in many application areas such as Internet of things (IoT) security [ 173 ], network security [ 174 ], cloud security [ 175 ], mobile and web applications [ 26 ], and other relevant cyber areas. Moreover, intelligent cybersecurity solutions are important for the banking industry, the healthcare sector, or the public sector, where data breaches typically occur [ 36 , 176 ]. Besides, the data-driven security solutions could also be effective in AI-based blockchain technology, where AI works with huge volumes of security event data to extract the useful insights using machine learning techniques, and block-chain as a trusted platform to store such data [ 177 ].
Although in this paper, we discuss cybersecurity data science focusing on examining raw security data to data-driven decision making for intelligent security solutions, it could also be related to big data analytics in terms of data processing and decision making. Big data deals with data sets that are too large or complex having characteristics of high data volume, velocity, and variety. Big data analytics mainly has two parts consisting of data management involving data storage, and analytics [ 178 ]. The analytics typically describe the process of analyzing such datasets to discover patterns, unknown correlations, rules, and other useful insights [ 179 ]. Thus, several advanced data analysis techniques such as AI, data mining, machine learning could play an important role in processing big data by converting big problems to small problems [ 180 ]. To do this, the potential strategies like parallelization, divide-and-conquer, incremental learning, sampling, granular computing, feature or instance selection, can be used to make better decisions, reducing costs, or enabling more efficient processing. In such cases, the concept of cybersecurity data science, particularly machine learning-based modeling could be helpful for process automation and decision making for intelligent security solutions. Moreover, researchers could consider modified algorithms or models for handing big data on parallel computing platforms like Hadoop, Storm, etc. [ 181 ].
Based on the concept of cybersecurity data science discussed in the paper, building a data-driven security model for a particular security problem and relevant empirical evaluation to measure the effectiveness and efficiency of the model, and to asses the usability in the real-world application domain could be a future work.
Motivated by the growing significance of cybersecurity and data science, and machine learning technologies, in this paper, we have discussed how cybersecurity data science applies to data-driven intelligent decision making in smart cybersecurity systems and services. We also have discussed how it can impact security data, both in terms of extracting insight of security incidents and the dataset itself. We aimed to work on cybersecurity data science by discussing the state of the art concerning security incidents data and corresponding security services. We also discussed how machine learning techniques can impact in the domain of cybersecurity, and examine the security challenges that remain. In terms of existing research, much focus has been provided on traditional security solutions, with less available work in machine learning technique based security systems. For each common technique, we have discussed relevant security research. The purpose of this article is to share an overview of the conceptualization, understanding, modeling, and thinking about cybersecurity data science.
We have further identified and discussed various key issues in security analysis to showcase the signpost of future research directions in the domain of cybersecurity data science. Based on the knowledge, we have also provided a generic multi-layered framework of cybersecurity data science model based on machine learning techniques, where the data is being gathered from diverse sources, and the analytics complement the latest data-driven patterns for providing intelligent security services. The framework consists of several main phases - security data collecting, data preparation, machine learning-based security modeling, and incremental learning and dynamism for smart cybersecurity systems and services. We specifically focused on extracting insights from security data, from setting a research design with particular attention to concepts for data-driven intelligent security solutions.
Overall, this paper aimed not only to discuss cybersecurity data science and relevant methods but also to discuss the applicability towards data-driven intelligent decision making in cybersecurity systems and services from machine learning perspectives. Our analysis and discussion can have several implications both for security researchers and practitioners. For researchers, we have highlighted several issues and directions for future research. Other areas for potential research include empirical evaluation of the suggested data-driven model, and comparative analysis with other security systems. For practitioners, the multi-layered machine learning-based model can be used as a reference in designing intelligent cybersecurity systems for organizations. We believe that our study on cybersecurity data science opens a promising path and can be used as a reference guide for both academia and industry for future research and applications in the area of cybersecurity.
Availability of data and materials
Not applicable.
Abbreviations
- Machine learning
Artificial Intelligence
Information and communication technology
Internet of Things
Distributed Denial of Service
Intrusion detection system
Intrusion prevention system
Host-based intrusion detection systems
Network Intrusion Detection Systems
Signature-based intrusion detection system
Anomaly-based intrusion detection system
Li S, Da Xu L, Zhao S. The internet of things: a survey. Inform Syst Front. 2015;17(2):243–59.
Google Scholar
Sun N, Zhang J, Rimba P, Gao S, Zhang LY, Xiang Y. Data-driven cybersecurity incident prediction: a survey. IEEE Commun Surv Tutor. 2018;21(2):1744–72.
McIntosh T, Jang-Jaccard J, Watters P, Susnjak T. The inadequacy of entropy-based ransomware detection. In: International conference on neural information processing. New York: Springer; 2019. p. 181–189
Alazab M, Venkatraman S, Watters P, Alazab M, et al. Zero-day malware detection based on supervised learning algorithms of api call signatures (2010)
Shaw A. Data breach: from notification to prevention using pci dss. Colum Soc Probs. 2009;43:517.
Gupta BB, Tewari A, Jain AK, Agrawal DP. Fighting against phishing attacks: state of the art and future challenges. Neural Comput Appl. 2017;28(12):3629–54.
Av-test institute, germany, https://www.av-test.org/en/statistics/malware/ . Accessed 20 Oct 2019.
Ibm security report, https://www.ibm.com/security/data-breach . Accessed on 20 Oct 2019.
Fischer EA. Cybersecurity issues and challenges: In brief. Congressional Research Service (2014)
Juniper research. https://www.juniperresearch.com/ . Accessed on 20 Oct 2019.
Papastergiou S, Mouratidis H, Kalogeraki E-M. Cyber security incident handling, warning and response system for the european critical information infrastructures (cybersane). In: International Conference on Engineering Applications of Neural Networks, p. 476–487 (2019). New York: Springer
Aftergood S. Cybersecurity: the cold war online. Nature. 2017;547(7661):30.
Hey AJ, Tansley S, Tolle KM, et al. The fourth paradigm: data-intensive scientific discovery. 2009;1:
Cukier K. Data, data everywhere: A special report on managing information, 2010.
Google trends. In: https://trends.google.com/trends/ , 2019.
Anwar S, Mohamad Zain J, Zolkipli MF, Inayat Z, Khan S, Anthony B, Chang V. From intrusion detection to an intrusion response system: fundamentals, requirements, and future directions. Algorithms. 2017;10(2):39.
MATH Google Scholar
Mohammadi S, Mirvaziri H, Ghazizadeh-Ahsaee M, Karimipour H. Cyber intrusion detection by combined feature selection algorithm. J Inform Sec Appl. 2019;44:80–8.
Tapiador JE, Orfila A, Ribagorda A, Ramos B. Key-recovery attacks on kids, a keyed anomaly detection system. IEEE Trans Depend Sec Comput. 2013;12(3):312–25.
Tavallaee M, Stakhanova N, Ghorbani AA. Toward credible evaluation of anomaly-based intrusion-detection methods. IEEE Transactions on Systems, Man, and Cybernetics, Part C (Applications and Reviews) 40(5), 516–524 (2010)
Foroughi F, Luksch P. Data science methodology for cybersecurity projects. arXiv preprint arXiv:1803.04219 , 2018.
Saxe J, Sanders H. Malware data science: Attack detection and attribution, 2018.
Rainie L, Anderson J, Connolly J. Cyber attacks likely to increase. Digital Life in. 2014, vol. 2025.
Fischer EA. Creating a national framework for cybersecurity: an analysis of issues and options. LIBRARY OF CONGRESS WASHINGTON DC CONGRESSIONAL RESEARCH SERVICE, 2005.
Craigen D, Diakun-Thibault N, Purse R. Defining cybersecurity. Technology Innovation. Manag Rev. 2014;4(10):13–21.
Council NR. et al. Toward a safer and more secure cyberspace, 2007.
Jang-Jaccard J, Nepal S. A survey of emerging threats in cybersecurity. J Comput Syst Sci. 2014;80(5):973–93.
MathSciNet MATH Google Scholar
Mukkamala S, Sung A, Abraham A. Cyber security challenges: Designing efficient intrusion detection systems and antivirus tools. Vemuri, V. Rao, Enhancing Computer Security with Smart Technology.(Auerbach, 2006), 125–163, 2005.
Bilge L, Dumitraş T. Before we knew it: an empirical study of zero-day attacks in the real world. In: Proceedings of the 2012 ACM conference on computer and communications security. ACM; 2012. p. 833–44.
Davi L, Dmitrienko A, Sadeghi A-R, Winandy M. Privilege escalation attacks on android. In: International conference on information security. New York: Springer; 2010. p. 346–60.
Jovičić B, Simić D. Common web application attack types and security using asp .net. ComSIS, 2006.
Warkentin M, Willison R. Behavioral and policy issues in information systems security: the insider threat. Eur J Inform Syst. 2009;18(2):101–5.
Kügler D. “man in the middle” attacks on bluetooth. In: International Conference on Financial Cryptography. New York: Springer; 2003, p. 149–61.
Virvilis N, Gritzalis D. The big four-what we did wrong in advanced persistent threat detection. In: 2013 International Conference on Availability, Reliability and Security. IEEE; 2013. p. 248–54.
Boyd SW, Keromytis AD. Sqlrand: Preventing sql injection attacks. In: International conference on applied cryptography and network security. New York: Springer; 2004. p. 292–302.
Sigler K. Crypto-jacking: how cyber-criminals are exploiting the crypto-currency boom. Comput Fraud Sec. 2018;2018(9):12–4.
2019 data breach investigations report, https://enterprise.verizon.com/resources/reports/dbir/ . Accessed 20 Oct 2019.
Khraisat A, Gondal I, Vamplew P, Kamruzzaman J. Survey of intrusion detection systems: techniques, datasets and challenges. Cybersecurity. 2019;2(1):20.
Johnson L. Computer incident response and forensics team management: conducting a successful incident response, 2013.
Brahmi I, Brahmi H, Yahia SB. A multi-agents intrusion detection system using ontology and clustering techniques. In: IFIP international conference on computer science and its applications. New York: Springer; 2015. p. 381–93.
Qu X, Yang L, Guo K, Ma L, Sun M, Ke M, Li M. A survey on the development of self-organizing maps for unsupervised intrusion detection. In: Mobile networks and applications. 2019;1–22.
Liao H-J, Lin C-HR, Lin Y-C, Tung K-Y. Intrusion detection system: a comprehensive review. J Netw Comput Appl. 2013;36(1):16–24.
Alazab A, Hobbs M, Abawajy J, Alazab M. Using feature selection for intrusion detection system. In: 2012 International symposium on communications and information technologies (ISCIT). IEEE; 2012. p. 296–301.
Viegas E, Santin AO, Franca A, Jasinski R, Pedroni VA, Oliveira LS. Towards an energy-efficient anomaly-based intrusion detection engine for embedded systems. IEEE Trans Comput. 2016;66(1):163–77.
Xin Y, Kong L, Liu Z, Chen Y, Li Y, Zhu H, Gao M, Hou H, Wang C. Machine learning and deep learning methods for cybersecurity. IEEE Access. 2018;6:35365–81.
Dutt I, Borah S, Maitra IK, Bhowmik K, Maity A, Das S. Real-time hybrid intrusion detection system using machine learning techniques. 2018, p. 885–94.
Ragsdale DJ, Carver C, Humphries JW, Pooch UW. Adaptation techniques for intrusion detection and intrusion response systems. In: Smc 2000 conference proceedings. 2000 IEEE international conference on systems, man and cybernetics.’cybernetics evolving to systems, humans, organizations, and their complex interactions’(cat. No. 0). IEEE; 2000. vol. 4, p. 2344–2349.
Cao L. Data science: challenges and directions. Commun ACM. 2017;60(8):59–68.
Rizk A, Elragal A. Data science: developing theoretical contributions in information systems via text analytics. J Big Data. 2020;7(1):1–26.
Lippmann RP, Fried DJ, Graf I, Haines JW, Kendall KR, McClung D, Weber D, Webster SE, Wyschogrod D, Cunningham RK, et al. Evaluating intrusion detection systems: The 1998 darpa off-line intrusion detection evaluation. In: Proceedings DARPA information survivability conference and exposition. DISCEX’00. IEEE; 2000. vol. 2, p. 12–26.
Kdd cup 99. http://kdd.ics.uci.edu/databases/kddcup99/kddcup99.html . Accessed 20 Oct 2019.
Tavallaee M, Bagheri E, Lu W, Ghorbani AA. A detailed analysis of the kdd cup 99 data set. In: 2009 IEEE symposium on computational intelligence for security and defense applications. IEEE; 2009. p. 1–6.
Caida ddos attack 2007 dataset. http://www.caida.org/data/ passive/ddos-20070804-dataset.xml/ . Accessed 20 Oct 2019.
Caida anonymized internet traces 2008 dataset. https://www.caida.org/data/passive/passive-2008-dataset . Accessed 20 Oct 2019.
Isot botnet dataset. https://www.uvic.ca/engineering/ece/isot/ datasets/index.php/ . Accessed 20 Oct 2019.
The honeynet project. http://www.honeynet.org/chapters/france/ . Accessed 20 Oct 2019.
Canadian institute of cybersecurity, university of new brunswick, iscx dataset, http://www.unb.ca/cic/datasets/index.html/ . Accessed 20 Oct 2019.
Shiravi A, Shiravi H, Tavallaee M, Ghorbani AA. Toward developing a systematic approach to generate benchmark datasets for intrusion detection. Comput Secur. 2012;31(3):357–74.
The ctu-13 dataset. https://stratosphereips.org/category/datasets-ctu13 . Accessed 20 Oct 2019.
Moustafa N, Slay J. Unsw-nb15: a comprehensive data set for network intrusion detection systems (unsw-nb15 network data set). In: 2015 Military Communications and Information Systems Conference (MilCIS). IEEE; 2015. p. 1–6.
Cse-cic-ids2018 [online]. available: https://www.unb.ca/cic/ datasets/ids-2018.html/ . Accessed 20 Oct 2019.
Cic-ddos2019 [online]. available: https://www.unb.ca/cic/datasets/ddos-2019.html/ . Accessed 28 Mar 2019.
Jing X, Yan Z, Jiang X, Pedrycz W. Network traffic fusion and analysis against ddos flooding attacks with a novel reversible sketch. Inform Fusion. 2019;51:100–13.
Xie M, Hu J, Yu X, Chang E. Evaluating host-based anomaly detection systems: application of the frequency-based algorithms to adfa-ld. In: International conference on network and system security. New York: Springer; 2015. p. 542–49.
Lindauer B, Glasser J, Rosen M, Wallnau KC, ExactData L. Generating test data for insider threat detectors. JoWUA. 2014;5(2):80–94.
Glasser J, Lindauer B. Bridging the gap: A pragmatic approach to generating insider threat data. In: 2013 IEEE Security and Privacy Workshops. IEEE; 2013. p. 98–104.
Enronspam. https://labs-repos.iit.demokritos.gr/skel/i-config/downloads/enron-spam/ . Accessed 20 Oct 2019.
Spamassassin. http://www.spamassassin.org/publiccorpus/ . Accessed 20 Oct 2019.
Lingspam. https://labs-repos.iit.demokritos.gr/skel/i-config/downloads/lingspampublic.tar.gz/ . Accessed 20 Oct 2019.
Alexa top sites. https://aws.amazon.com/alexa-top-sites/ . Accessed 20 Oct 2019.
Bambenek consulting—master feeds. available online: http://osint.bambenekconsulting.com/feeds/ . Accessed 20 Oct 2019.
Dgarchive. https://dgarchive.caad.fkie.fraunhofer.de/site/ . Accessed 20 Oct 2019.
Zago M, Pérez MG, Pérez GM. Umudga: A dataset for profiling algorithmically generated domain names in botnet detection. Data in Brief. 2020;105400.
Zhou Y, Jiang X. Dissecting android malware: characterization and evolution. In: 2012 IEEE Symposium on security and privacy. IEEE; 2012. p. 95–109.
Virusshare. http://virusshare.com/ . Accessed 20 Oct 2019.
Virustotal. https://virustotal.com/ . Accessed 20 Oct 2019.
Comodo. https://www.comodo.com/home/internet-security/updates/vdp/database . Accessed 20 Oct 2019.
Contagio. http://contagiodump.blogspot.com/ . Accessed 20 Oct 2019.
Kumar R, Xiaosong Z, Khan RU, Kumar J, Ahad I. Effective and explainable detection of android malware based on machine learning algorithms. In: Proceedings of the 2018 international conference on computing and artificial intelligence. ACM; 2018. p. 35–40.
Microsoft malware classification (big 2015). arXiv:org/abs/1802.10135/ . Accessed 20 Oct 2019.
Koroniotis N, Moustafa N, Sitnikova E, Turnbull B. Towards the development of realistic botnet dataset in the internet of things for network forensic analytics: bot-iot dataset. Future Gen Comput Syst. 2019;100:779–96.
McIntosh TR, Jang-Jaccard J, Watters PA. Large scale behavioral analysis of ransomware attacks. In: International conference on neural information processing. New York: Springer; 2018. p. 217–29.
Han J, Pei J, Kamber M. Data mining: concepts and techniques, 2011.
Witten IH, Frank E. Data mining: Practical machine learning tools and techniques, 2005.
Dua S, Du X. Data mining and machine learning in cybersecurity, 2016.
Kotpalliwar MV, Wajgi R. Classification of attacks using support vector machine (svm) on kddcup’99 ids database. In: 2015 Fifth international conference on communication systems and network technologies. IEEE; 2015. p. 987–90.
Pervez MS, Farid DM. Feature selection and intrusion classification in nsl-kdd cup 99 dataset employing svms. In: The 8th international conference on software, knowledge, information management and applications (SKIMA 2014). IEEE; 2014. p. 1–6.
Yan M, Liu Z. A new method of transductive svm-based network intrusion detection. In: International conference on computer and computing technologies in agriculture. New York: Springer; 2010. p. 87–95.
Li Y, Xia J, Zhang S, Yan J, Ai X, Dai K. An efficient intrusion detection system based on support vector machines and gradually feature removal method. Expert Syst Appl. 2012;39(1):424–30.
Raman MG, Somu N, Jagarapu S, Manghnani T, Selvam T, Krithivasan K, Sriram VS. An efficient intrusion detection technique based on support vector machine and improved binary gravitational search algorithm. Artificial Intelligence Review. 2019, p. 1–32.
Kokila R, Selvi ST, Govindarajan K. Ddos detection and analysis in sdn-based environment using support vector machine classifier. In: 2014 Sixth international conference on advanced computing (ICoAC). IEEE; 2014. p. 205–10.
Xie M, Hu J, Slay J. Evaluating host-based anomaly detection systems: Application of the one-class svm algorithm to adfa-ld. In: 2014 11th international conference on fuzzy systems and knowledge discovery (FSKD). IEEE; 2014. p. 978–82.
Saxena H, Richariya V. Intrusion detection in kdd99 dataset using svm-pso and feature reduction with information gain. Int J Comput Appl. 2014;98:6.
Chandrasekhar A, Raghuveer K. Confederation of fcm clustering, ann and svm techniques to implement hybrid nids using corrected kdd cup 99 dataset. In: 2014 international conference on communication and signal processing. IEEE; 2014. p. 672–76.
Shapoorifard H, Shamsinejad P. Intrusion detection using a novel hybrid method incorporating an improved knn. Int J Comput Appl. 2017;173(1):5–9.
Vishwakarma S, Sharma V, Tiwari A. An intrusion detection system using knn-aco algorithm. Int J Comput Appl. 2017;171(10):18–23.
Meng W, Li W, Kwok L-F. Design of intelligent knn-based alarm filter using knowledge-based alert verification in intrusion detection. Secur Commun Netw. 2015;8(18):3883–95.
Dada E. A hybridized svm-knn-pdapso approach to intrusion detection system. In: Proc. Fac. Seminar Ser., 2017, p. 14–21.
Sharifi AM, Amirgholipour SK, Pourebrahimi A. Intrusion detection based on joint of k-means and knn. J Converg Inform Technol. 2015;10(5):42.
Lin W-C, Ke S-W, Tsai C-F. Cann: an intrusion detection system based on combining cluster centers and nearest neighbors. Knowl Based Syst. 2015;78:13–21.
Koc L, Mazzuchi TA, Sarkani S. A network intrusion detection system based on a hidden naïve bayes multiclass classifier. Exp Syst Appl. 2012;39(18):13492–500.
Moon D, Im H, Kim I, Park JH. Dtb-ids: an intrusion detection system based on decision tree using behavior analysis for preventing apt attacks. J Supercomput. 2017;73(7):2881–95.
Ingre, B., Yadav, A., Soni, A.K.: Decision tree based intrusion detection system for nsl-kdd dataset. In: International conference on information and communication technology for intelligent systems. New York: Springer; 2017. p. 207–18.
Malik AJ, Khan FA. A hybrid technique using binary particle swarm optimization and decision tree pruning for network intrusion detection. Cluster Comput. 2018;21(1):667–80.
Relan NG, Patil DR. Implementation of network intrusion detection system using variant of decision tree algorithm. In: 2015 international conference on nascent technologies in the engineering field (ICNTE). IEEE; 2015. p. 1–5.
Rai K, Devi MS, Guleria A. Decision tree based algorithm for intrusion detection. Int J Adv Netw Appl. 2016;7(4):2828.
Sarker IH, Abushark YB, Alsolami F, Khan AI. Intrudtree: a machine learning based cyber security intrusion detection model. Symmetry. 2020;12(5):754.
Puthran S, Shah K. Intrusion detection using improved decision tree algorithm with binary and quad split. In: International symposium on security in computing and communication. New York: Springer; 2016. p. 427–438.
Balogun AO, Jimoh RG. Anomaly intrusion detection using an hybrid of decision tree and k-nearest neighbor, 2015.
Azad C, Jha VK. Genetic algorithm to solve the problem of small disjunct in the decision tree based intrusion detection system. Int J Comput Netw Inform Secur. 2015;7(8):56.
Jo S, Sung H, Ahn B. A comparative study on the performance of intrusion detection using decision tree and artificial neural network models. J Korea Soc Dig Indus Inform Manag. 2015;11(4):33–45.
Zhan J, Zulkernine M, Haque A. Random-forests-based network intrusion detection systems. IEEE Trans Syst Man Cybern C. 2008;38(5):649–59.
Tajbakhsh A, Rahmati M, Mirzaei A. Intrusion detection using fuzzy association rules. Appl Soft Comput. 2009;9(2):462–9.
Mitchell R, Chen R. Behavior rule specification-based intrusion detection for safety critical medical cyber physical systems. IEEE Trans Depend Secure Comput. 2014;12(1):16–30.
Alazab M, Venkataraman S, Watters P. Towards understanding malware behaviour by the extraction of api calls. In: 2010 second cybercrime and trustworthy computing Workshop. IEEE; 2010. p. 52–59.
Yuan Y, Kaklamanos G, Hogrefe D. A novel semi-supervised adaboost technique for network anomaly detection. In: Proceedings of the 19th ACM international conference on modeling, analysis and simulation of wireless and mobile systems. ACM; 2016. p. 111–14.
Ariu D, Tronci R, Giacinto G. Hmmpayl: an intrusion detection system based on hidden markov models. Comput Secur. 2011;30(4):221–41.
Årnes A, Valeur F, Vigna G, Kemmerer RA. Using hidden markov models to evaluate the risks of intrusions. In: International workshop on recent advances in intrusion detection. New York: Springer; 2006. p. 145–64.
Hansen JV, Lowry PB, Meservy RD, McDonald DM. Genetic programming for prevention of cyberterrorism through dynamic and evolving intrusion detection. Decis Supp Syst. 2007;43(4):1362–74.
Aslahi-Shahri B, Rahmani R, Chizari M, Maralani A, Eslami M, Golkar MJ, Ebrahimi A. A hybrid method consisting of ga and svm for intrusion detection system. Neural Comput Appl. 2016;27(6):1669–76.
Alrawashdeh K, Purdy C. Toward an online anomaly intrusion detection system based on deep learning. In: 2016 15th IEEE international conference on machine learning and applications (ICMLA). IEEE; 2016. p. 195–200.
Yin C, Zhu Y, Fei J, He X. A deep learning approach for intrusion detection using recurrent neural networks. IEEE Access. 2017;5:21954–61.
Kim J, Kim J, Thu HLT, Kim H. Long short term memory recurrent neural network classifier for intrusion detection. In: 2016 international conference on platform technology and service (PlatCon). IEEE; 2016. p. 1–5.
Almiani M, AbuGhazleh A, Al-Rahayfeh A, Atiewi S, Razaque A. Deep recurrent neural network for iot intrusion detection system. Simulation Modelling Practice and Theory. 2019;102031.
Kolosnjaji B, Zarras A, Webster G, Eckert C. Deep learning for classification of malware system call sequences. In: Australasian joint conference on artificial intelligence. New York: Springer; 2016. p. 137–49.
Wang W, Zhu M, Zeng X, Ye X, Sheng Y. Malware traffic classification using convolutional neural network for representation learning. In: 2017 international conference on information networking (ICOIN). IEEE; 2017. p. 712–17.
Alauthman M, Aslam N, Al-kasassbeh M, Khan S, Al-Qerem A, Choo K-KR. An efficient reinforcement learning-based botnet detection approach. J Netw Comput Appl. 2020;150:102479.
Blanco R, Cilla JJ, Briongos S, Malagón P, Moya JM. Applying cost-sensitive classifiers with reinforcement learning to ids. In: International conference on intelligent data engineering and automated learning. New York: Springer; 2018. p. 531–38.
Lopez-Martin M, Carro B, Sanchez-Esguevillas A. Application of deep reinforcement learning to intrusion detection for supervised problems. Exp Syst Appl. 2020;141:112963.
Sarker IH, Kayes A, Watters P. Effectiveness analysis of machine learning classification models for predicting personalized context-aware smartphone usage. J Big Data. 2019;6(1):1–28.
Holte RC. Very simple classification rules perform well on most commonly used datasets. Mach Learn. 1993;11(1):63–90.
John GH, Langley P. Estimating continuous distributions in bayesian classifiers. In: Proceedings of the eleventh conference on uncertainty in artificial intelligence. Morgan Kaufmann Publishers Inc.; 1995. p. 338–45.
Quinlan JR. C4.5: Programs for machine learning. Machine Learning, 1993.
Sarker IH, Colman A, Han J, Khan AI, Abushark YB, Salah K. Behavdt: a behavioral decision tree learning to build user-centric context-aware predictive model. Mobile Networks and Applications. 2019, p. 1–11.
Aha DW, Kibler D, Albert MK. Instance-based learning algorithms. Mach Learn. 1991;6(1):37–66.
Keerthi SS, Shevade SK, Bhattacharyya C, Murthy KRK. Improvements to platt’s smo algorithm for svm classifier design. Neural Comput. 2001;13(3):637–49.
Freund Y, Schapire RE, et al: Experiments with a new boosting algorithm. In: Icml, vol. 96, p. 148–156 (1996). Citeseer
Le Cessie S, Van Houwelingen JC. Ridge estimators in logistic regression. J Royal Stat Soc C. 1992;41(1):191–201.
Watters PA, McCombie S, Layton R, Pieprzyk J. Characterising and predicting cyber attacks using the cyber attacker model profile (camp). J Money Launder Control. 2012.
Breiman L. Random forests. Mach Learn. 2001;45(1):5–32.
Sarker IH. Context-aware rule learning from smartphone data: survey, challenges and future directions. J Big Data. 2019;6(1):95.
MacQueen J. Some methods for classification and analysis of multivariate observations. In: Fifth Berkeley symposium on mathematical statistics and probability, vol. 1, 1967.
Rokach L. A survey of clustering algorithms. In: Data Mining and Knowledge Discovery Handbook. New York: Springer; 2010. p. 269–98.
Sneath PH. The application of computers to taxonomy. J Gen Microbiol. 1957;17:1.
Sorensen T. method of establishing groups of equal amplitude in plant sociology based on similarity of species. Biol Skr. 1948;5.
Sarker IH, Colman A, Kabir MA, Han J. Individualized time-series segmentation for mining mobile phone user behavior. Comput J. 2018;61(3):349–68.
Kim G, Lee S, Kim S. A novel hybrid intrusion detection method integrating anomaly detection with misuse detection. Exp Syst Appl. 2014;41(4):1690–700.
MathSciNet Google Scholar
Agrawal R, Imieliński T, Swami A. Mining association rules between sets of items in large databases. In: ACM SIGMOD Record. ACM; 1993. vol. 22, p. 207–16.
Flach PA, Lachiche N. Confirmation-guided discovery of first-order rules with tertius. Mach Learn. 2001;42(1–2):61–95.
Agrawal R, Srikant R, et al: Fast algorithms for mining association rules. In: Proc. 20th Int. Conf. Very Large Data Bases, VLDB, 1994, vol. 1215, p. 487–99.
Houtsma M, Swami A. Set-oriented mining for association rules in relational databases. In: Proceedings of the eleventh international conference on data engineering. IEEE; 1995. p. 25–33.
Ma BLWHY. Integrating classification and association rule mining. In: Proceedings of the fourth international conference on knowledge discovery and data mining, 1998.
Han J, Pei J, Yin Y. Mining frequent patterns without candidate generation. In: ACM Sigmod Record. ACM; 2000. vol. 29, p. 1–12.
Sarker IH, Salim FD. Mining user behavioral rules from smartphone data through association analysis. In: Proceedings of the 22nd Pacific-Asia Conference on Knowledge Discovery and Data Mining (PAKDD), Melbourne, Australia. New York: Springer; 2018. p. 450–61.
Das A, Ng W-K, Woon Y-K. Rapid association rule mining. In: Proceedings of the tenth international conference on information and knowledge management. ACM; 2001. p. 474–81.
Zaki MJ. Scalable algorithms for association mining. IEEE Trans Knowl Data Eng. 2000;12(3):372–90.
Coelho IM, Coelho VN, Luz EJS, Ochi LS, Guimarães FG, Rios E. A gpu deep learning metaheuristic based model for time series forecasting. Appl Energy. 2017;201:412–8.
Van Efferen L, Ali-Eldin AM. A multi-layer perceptron approach for flow-based anomaly detection. In: 2017 International symposium on networks, computers and communications (ISNCC). IEEE; 2017. p. 1–6.
Liu H, Lang B, Liu M, Yan H. Cnn and rnn based payload classification methods for attack detection. Knowl Based Syst. 2019;163:332–41.
Berman DS, Buczak AL, Chavis JS, Corbett CL. A survey of deep learning methods for cyber security. Information. 2019;10(4):122.
Bellman R. A markovian decision process. J Math Mech. 1957;1:679–84.
Kaelbling LP, Littman ML, Moore AW. Reinforcement learning: a survey. J Artif Intell Res. 1996;4:237–85.
Sarker IH. A machine learning based robust prediction model for real-life mobile phone data. Internet of Things. 2019;5:180–93.
Kayes ASM, Han J, Colman A. OntCAAC: an ontology-based approach to context-aware access control for software services. Comput J. 2015;58(11):3000–34.
Kayes ASM, Rahayu W, Dillon T. An ontology-based approach to dynamic contextual role for pervasive access control. In: AINA 2018. IEEE Computer Society, 2018.
Colombo P, Ferrari E. Access control technologies for big data management systems: literature review and future trends. Cybersecurity. 2019;2(1):1–13.
Aleroud A, Karabatis G. Contextual information fusion for intrusion detection: a survey and taxonomy. Knowl Inform Syst. 2017;52(3):563–619.
Sarker IH, Abushark YB, Khan AI. Contextpca: Predicting context-aware smartphone apps usage based on machine learning techniques. Symmetry. 2020;12(4):499.
Madsen RE, Hansen LK, Winther O. Singular value decomposition and principal component analysis. Neural Netw. 2004;1:1–5.
Qiao L-B, Zhang B-F, Lai Z-Q, Su J-S. Mining of attack models in ids alerts from network backbone by a two-stage clustering method. In: 2012 IEEE 26th international parallel and distributed processing symposium workshops & Phd Forum. IEEE; 2012. p. 1263–9.
Sarker IH, Colman A, Han J. Recencyminer: mining recency-based personalized behavior from contextual smartphone data. J Big Data. 2019;6(1):49.
Ullah F, Babar MA. Architectural tactics for big data cybersecurity analytics systems: a review. J Syst Softw. 2019;151:81–118.
Zhao S, Leftwich K, Owens M, Magrone F, Schonemann J, Anderson B, Medhi D. I-can-mama: Integrated campus network monitoring and management. In: 2014 IEEE network operations and management symposium (NOMS). IEEE; 2014. p. 1–7.
Abomhara M, et al. Cyber security and the internet of things: vulnerabilities, threats, intruders and attacks. J Cyber Secur Mob. 2015;4(1):65–88.
Helali RGM. Data mining based network intrusion detection system: A survey. In: Novel algorithms and techniques in telecommunications and networking. New York: Springer; 2010. p. 501–505.
Ryoo J, Rizvi S, Aiken W, Kissell J. Cloud security auditing: challenges and emerging approaches. IEEE Secur Priv. 2013;12(6):68–74.
Densham B. Three cyber-security strategies to mitigate the impact of a data breach. Netw Secur. 2015;2015(1):5–8.
Salah K, Rehman MHU, Nizamuddin N, Al-Fuqaha A. Blockchain for ai: review and open research challenges. IEEE Access. 2019;7:10127–49.
Gandomi A, Haider M. Beyond the hype: big data concepts, methods, and analytics. Int J Inform Manag. 2015;35(2):137–44.
Golchha N. Big data-the information revolution. Int J Adv Res. 2015;1(12):791–4.
Hariri RH, Fredericks EM, Bowers KM. Uncertainty in big data analytics: survey, opportunities, and challenges. J Big Data. 2019;6(1):44.
Tsai C-W, Lai C-F, Chao H-C, Vasilakos AV. Big data analytics: a survey. J Big data. 2015;2(1):21.
Download references
Acknowledgements
The authors would like to thank all the reviewers for their rigorous review and comments in several revision rounds. The reviews are detailed and helpful to improve and finalize the manuscript. The authors are highly grateful to them.
Author information
Authors and affiliations.
Swinburne University of Technology, Melbourne, VIC, 3122, Australia
Iqbal H. Sarker
Chittagong University of Engineering and Technology, Chittagong, 4349, Bangladesh
La Trobe University, Melbourne, VIC, 3086, Australia
A. S. M. Kayes, Paul Watters & Alex Ng
University of Nevada, Reno, USA
Shahriar Badsha
Macquarie University, Sydney, NSW, 2109, Australia
Hamed Alqahtani
You can also search for this author in PubMed Google Scholar
Contributions
This article provides not only a discussion on cybersecurity data science and relevant methods but also to discuss the applicability towards data-driven intelligent decision making in cybersecurity systems and services. All authors read and approved the final manuscript.
Corresponding author
Correspondence to Iqbal H. Sarker .
Ethics declarations
Competing interests.
The authors declare that they have no competing interests.
Additional information
Publisher's note.
Springer Nature remains neutral with regard to jurisdictional claims in published maps and institutional affiliations.
Rights and permissions
Open Access This article is licensed under a Creative Commons Attribution 4.0 International License, which permits use, sharing, adaptation, distribution and reproduction in any medium or format, as long as you give appropriate credit to the original author(s) and the source, provide a link to the Creative Commons licence, and indicate if changes were made. The images or other third party material in this article are included in the article's Creative Commons licence, unless indicated otherwise in a credit line to the material. If material is not included in the article's Creative Commons licence and your intended use is not permitted by statutory regulation or exceeds the permitted use, you will need to obtain permission directly from the copyright holder. To view a copy of this licence, visit http://creativecommons.org/licenses/by/4.0/ .
Reprints and permissions
About this article
Cite this article.
Sarker, I.H., Kayes, A.S.M., Badsha, S. et al. Cybersecurity data science: an overview from machine learning perspective. J Big Data 7 , 41 (2020). https://doi.org/10.1186/s40537-020-00318-5
Download citation
Received : 26 October 2019
Accepted : 21 June 2020
Published : 01 July 2020
DOI : https://doi.org/10.1186/s40537-020-00318-5
Share this article
Anyone you share the following link with will be able to read this content:
Sorry, a shareable link is not currently available for this article.
Provided by the Springer Nature SharedIt content-sharing initiative
- Decision making
- Cyber-attack
- Security modeling
- Intrusion detection
- Cyber threat intelligence
Thank you for visiting nature.com. You are using a browser version with limited support for CSS. To obtain the best experience, we recommend you use a more up to date browser (or turn off compatibility mode in Internet Explorer). In the meantime, to ensure continued support, we are displaying the site without styles and JavaScript.
- View all journals
- My Account Login
- Explore content
- About the journal
- Publish with us
- Sign up for alerts
- Open access
- Published: 17 May 2023
A holistic and proactive approach to forecasting cyber threats
- Zaid Almahmoud 1 ,
- Paul D. Yoo 1 ,
- Omar Alhussein 2 ,
- Ilyas Farhat 3 &
- Ernesto Damiani 4 , 5
Scientific Reports volume 13 , Article number: 8049 ( 2023 ) Cite this article
4663 Accesses
5 Citations
2 Altmetric
Metrics details
- Computer science
- Information technology
Traditionally, cyber-attack detection relies on reactive, assistive techniques, where pattern-matching algorithms help human experts to scan system logs and network traffic for known virus or malware signatures. Recent research has introduced effective Machine Learning (ML) models for cyber-attack detection, promising to automate the task of detecting, tracking and blocking malware and intruders. Much less effort has been devoted to cyber-attack prediction, especially beyond the short-term time scale of hours and days. Approaches that can forecast attacks likely to happen in the longer term are desirable, as this gives defenders more time to develop and share defensive actions and tools. Today, long-term predictions of attack waves are mostly based on the subjective perceptiveness of experienced human experts, which can be impaired by the scarcity of cyber-security expertise. This paper introduces a novel ML-based approach that leverages unstructured big data and logs to forecast the trend of cyber-attacks at a large scale, years in advance. To this end, we put forward a framework that utilises a monthly dataset of major cyber incidents in 36 countries over the past 11 years, with new features extracted from three major categories of big data sources, namely the scientific research literature, news, blogs, and tweets. Our framework not only identifies future attack trends in an automated fashion, but also generates a threat cycle that drills down into five key phases that constitute the life cycle of all 42 known cyber threats.
Similar content being viewed by others
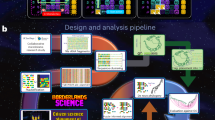
Improving microbial phylogeny with citizen science within a mass-market video game
Roman Sarrazin-Gendron, Parham Ghasemloo Gheidari, … Jérôme Waldispühl
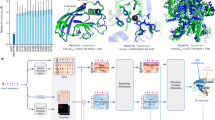
Highly accurate protein structure prediction with AlphaFold
John Jumper, Richard Evans, … Demis Hassabis
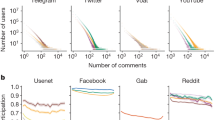
Persistent interaction patterns across social media platforms and over time
Michele Avalle, Niccolò Di Marco, … Walter Quattrociocchi
Introduction
Running a global technology infrastructure in an increasingly de-globalised world raises unprecedented security issues. In the past decade, we have witnessed waves of cyber-attacks that caused major damage to governments, organisations and enterprises, affecting their bottom lines 1 . Nevertheless, cyber-defences remained reactive in nature, involving significant overhead in terms of execution time. This latency is due to the complex pattern-matching operations required to identify the signatures of polymorphic malware 2 , which shows different behaviour each time it is run. More recently, ML-based models were introduced relying on anomaly detection algorithms. Although these models have shown a good capability to detect unknown attacks, they may classify benign behaviour as abnormal 3 , giving rise to a false alarm.
We argue that data availability can enable a proactive defense, acting before a potential threat escalates into an actual incident. Concerning non-cyber threats, including terrorism and military attacks, proactive approaches alleviate, delay, and even prevent incidents from arising in the first place. Massive software programs are available to assess the intention, potential damages, attack methods, and alternative options for a terrorist attack 4 . We claim that cyber-attacks should be no exception, and that nowadays we have the capabilities to carry out proactive, low latency cyber-defenses based on ML 5 .
Indeed, ML models can provide accurate and reliable forecasts. For example, ML models such as AlphaFold2 6 and RoseTTAFold 7 can predict a protein’s three-dimensional structure from its linear sequence. Cyber-security data, however, poses its unique challenges. Cyber-incidents are highly sensitive events and are usually kept confidential since they affect the involved organisations’ reputation. It is often difficult to keep track of these incidents, because they can go unnoticed even by the victim. It is also worth mentioning that pre-processing cyber-security data is challenging, due to characteristics such as lack of structure, diversity in format, and high rates of missing values which distort the findings.
When devising a ML-based method, one can rely on manual feature identification and engineering, or try and learn the features from raw data. In the context of cyber-incidents, there are many factors ( i.e. , potential features) that could lead to the occurrence of an attack. Wars and political conflicts between countries often lead to cyber-warfare 8 , 9 . The number of mentions of a certain attack appearing in scientific articles may correlate well with the actual incident rate. Also, cyber-attacks often take place on holidays, anniversaries and other politically significant dates 5 . Finding the right features out of unstructured big data is one of the key strands of our proposed framework.
The remainder of the paper is structured as follows. The “ Literature review ” section presents an overview of the related work and highlights the research gaps and our contributions. The “ Methods ” section describes the framework design, including the construction of the dataset and the building of the model. The “ Results ” section presents the validation results of our model, the trend analysis and forecast, and a detailed description of the developed threat cycle. Lastly, the “ Discussion ” section offers a critical evaluation of our work, highlighting its strengths and limitations, and provides recommendations for future research.
Literature review
In recent years, the literature has extensively covered different cyber threats across various application domains, and researchers have proposed several solutions to mitigate these threats. In the Social Internet of Vehicles (SIoV), one of the primary concerns is the interception and tampering of sensitive information by attackers 10 . To address this, a secure authentication protocol has been proposed that utilises confidential computing environments to ensure the privacy of vehicle-generated data. Another application domain that has been studied is the privacy of image data, specifically lane images in rural areas 11 . The proposed methodology uses Error Level Analysis (ELA) and artificial neural network (ANN) algorithms to classify lane images as genuine or fake, with the U-Net model for lane detection in bona fide images. The final images are secured using the proxy re-encryption technique with RSA and ECC algorithms, and maintained using fog computing to protect against forgery.
Another application domain that has been studied is the security of Wireless Mesh Networks (WMNs) in the context of the Internet of Things (IoT) 12 . WMNs rely on cooperative forwarding, making them vulnerable to various attacks, including packet drop/modification, badmouthing, on-off, and collusion attacks. To address this, a novel trust mechanism framework has been proposed that differentiates between legitimate and malicious nodes using direct and indirect trust computation. The framework utilises a two-hop mechanism to observe the packet forwarding behaviour of neighbours, and a weighted D-S theory to aggregate recommendations from different nodes. While these solutions have shown promising results in addressing cyber threats, it is important to anticipate the type of threat that may arise to ensure that the solutions can be effectively deployed. By proactively identifying and anticipating cyber threats, organisations can better prepare themselves to protect their systems and data from potential attacks.
While we are relatively successful in detecting and classifying cyber-attacks when they occur 13 , 14 , 15 , there has been a much more limited success in predicting them. Some studies exist on short-term predictive capability 16 , 17 , 18 , 19 , 20 , 21 , 22 , 23 , 24 , 25 , 26 , such as predicting the number or source of attacks to be expected in the next hours or days. The majority of this work performs the prediction in restricted settings ( e.g. , against a specific entity or organisation) where historical data are available 18 , 19 , 25 . Forecasting attack occurrences has been attempted by using statistical methods, especially when parametric data distributions could be assumed 16 , 17 , as well as by using ML models 20 . Other methods adopt a Bayesian setting and build event graphs suitable for estimating the conditional probability of an attack following a given chain of events 21 . Such techniques rely on libraries of predefined attack graphs: they can identify the known attack most likely to happen, but are helpless against never-experienced-before, zero-day attacks.
Other approaches try to identify potential attackers by using network entity reputation and scoring 26 . A small but growing body of research explores the fusion of heterogeneous features (warning signals) to forecast cyber-threats using ML. Warning signs may include the number of mentions of a victim organisation on Twitter 18 , mentions in news articles about the victim entity 19 , and digital traces from dark web hacker forums 20 . Our literature review is summarised in Table 1 .
Forecasting the cyber-threats that will most likely turn into attacks in the medium and long term is of significant importance. It not only gives to cyber-security agencies the time to evaluate the existing defence measures, but also assists them in identifying areas where to develop preventive solutions. Long-term prediction of cyber-threats, however, still relies on the subjective perceptions of human security experts 27 , 28 . Unlike a fully automated procedure based on quantitative metrics, the human-based approach is prone to bias based on scientific or technical interests 29 . Also, quantitative predictions are crucial to scientific objectivity 30 . In summary, we highlight the following research gaps:
Current research primarily focuses on detecting ( i.e. , reactive) rather than predicting cyber-attacks ( i.e. , proactive).
Available predictive methods for cyber-attacks are mostly limited to short-term predictions.
Current predictive methods for cyber-attacks are limited to restricted settings ( e.g. , a particular network or system).
Long-term prediction of cyber-attacks is currently performed by human experts, whose judgement is subjective and prone to bias and disagreement.
Research contributions
Our objective is to fill these research gaps by a proactive, long-term, and holistic approach to attack prediction. The proposed framework gives cyber-security agencies sufficient time to evaluate existing defence measures while also providing objective and accurate representation of the forecast. Our study is aimed at predicting the trend of cyber-attacks up to three years in advance, utilising big data sources and ML techniques. Our ML models are learned from heterogeneous features extracted from massive, unstructured data sources, namely, Hackmageddon 9 , Elsevier 31 , Twitter 32 , and Python APIs 33 . Hackmageddon provides more than 15, 000 records of global cyber-incidents since the year 2011, while Elsevier API offers access to the Scopus database, the largest abstract and citation database of peer-reviewed literature with over 27,000,000 documents 34 . The number of relevant tweets we collected is around 9 million. Our study covers 36 countries and 42 major attack types. The proposed framework not only provides the forecast and categorisation of the threats, but also generates a threat life-cycle model, whose the five key phases underlie the life cycle of all 42 known cyber-threats. The key contribution of this study consists of the following:
A novel dataset is constructed using big unstructured data ( i.e. , Hackmageddon) including news and government advisories, in addition to Elsevier, Twitter, and Python API. The dataset comprises monthly counts of cyber-attacks and other unique features, covering 42 attack types across 36 countries.
Our proactive approach offers long-term forecasting by predicting threats up to 3 years in advance.
Our approach is holistic in nature, as it does not limit itself to specific entities or regions. Instead, it provides projections of attacks across 36 countries situated in diverse parts of the world.
Our approach is completely automated and quantitative, effectively addressing the issue of bias in human predictions and providing a precise forecast.
By analysing past and predicted future data, we have classified threats into four main groups and provided a forecast of 42 attacks until 2025.
The first threat cycle is proposed, which delineates the distinct phases in the life cycle of 42 cyber-attack types.
The framework of forecasting cyber threats
The architecture of our framework for forecasting cyber threats is illustrated in Fig. 1 . As seen in the Data Sources component (l.h.s), to harness all the relevant data and extract meaningful insights, our framework utilises various sources of unstructured data. One of our main sources is Hackmageddon, which includes massive textual data on major cyber-attacks (approx. 15,334 incidents) dating back to July 2011. We refer to the monthly number of attacks in the list as the Number of Incidents (NoI). Also, Elsevier’s Application Programming Interface (API) gives access to a very large corpus of scientific articles and data sets from thousands of sources. Utilising this API, we obtained the Number of Mentions (NoM) ( e.g. , monthly) of each attack that appeared in the scientific publications. This NoM data is of particular importance as it can be used as the ground truth for attack types that do not appear in Hackmageddon. During the preliminary research phase, we examined all the potentially relevant features and noticed that wars/political conflicts are highly correlated to the number of cyber-events. These data were then extracted via Twitter API as Armed Conflict Areas/Wars (ACA). Lastly, as attacks often take place around holidays, Python’s holidays package was used to obtain the number of public holidays per month for each country, which is referred to as Public Holidays (PH).
To ensure the accuracy and quality of Hackmageddon data, we validated it using the statistics from official sources across government, academia, research institutes and technology organisations. For a ransomware example, the Cybersecurity & Infrastructure Security Agency stated in their 2021 trend report that cybersecurity authorities in the United States, Australia, and the United Kingdom observed an increase in sophisticated, high-impact ransomware incidents against critical infrastructure organisations globally 35 . The WannaCry attack in the dataset was also validated with Ghafur et al ’s 1 statement in their article: “WannaCry ransomware attack was a global epidemic that took place in May 2017”.
An example of an entry in the Hackmageddon dataset is shown in Table 2 . Each entry includes the incident date, the description of the attack, the attack type, and the target country. Data pre-processing (Fig. 1 ) focused on noise reduction through imputing missing values ( e.g. , countries), which were often observed in the earlier years. We were able to impute these values from the description column or occasionally, by looking up the entity location using Google.
The textual data were quantified via our Word Frequency Counter (WFC), which counted the number of each attack type per month as in Table 3 . Cumulative Aggregation (CA) obtained the number of attacks for all countries combined and an example of a data entry after transformation includes the month, and the number of attacks against each country (and all countries combined) for each attack type. By adding features such as NoM, ACA, and PH, we ended up having additional features that we appended to the dataset as shown in Table 4 . Our final dataset covers 42 common types of attacks in 36 countries. The full list of attacks is provided in Table 5 . The list of the countries is given in Supplementary Table S1 .
To analyse and investigate the main characteristics of our data, an exploratory analysis was conducted focusing on the visualisation and identification of key patterns such as trend and seasonality, correlated features, missing data and outliers. For seasonal data, we smoothed out the seasonality so that we could identify the trend while removing the noise in the time series 36 . The smoothing type and constants were optimised along with the ML model (see Optimisation for details). We applied Stochastic selection of Features (SoF) to find the subset of features that minimises the prediction error, and compared the univariate against the multivariate approach.
For the modelling, we built a Bayesian encoder-decoder Long Short-Term Memory (B-LSTM) network. B-LSTM models have been proposed to predict “perfect wave” events like the onset of stock market “bear” periods on the basis of multiple warning signs, each having different time dynamics 37 . Encoder-decoder architectures can manage inputs and outputs that both consist of variable-length sequences. The encoder stage encodes a sequence into a fixed-length vector representation (known as the latent representation). The decoder prompts the latent representation to predict a sequence. By applying an efficient latent representation, we train the model to consider all the useful warning information from the input sequence - regardless of its position - and disregard the noise.
Our Bayesian variation of the encoder-decoder LSTM network considers the weights of the model as random variables. This way, we extract epistemic uncertainty via (approximate) Bayesian inference, which quantifies the prediction error due to insufficient information 38 . This is an important parameter, as epistemic uncertainty can be reduced by better intelligence, i.e. , by acquiring more samples and new informative features. Details are provided in “ Bayesian long short-term memory ” section.
Our overall analytical platform learns an operational model for each attack type. Here, we evaluated the model’s performance in predicting the threat trend 36 months in advance. A newly modified symmetric Mean Absolute Percentage Error (M-SMAPE) was devised as the evaluation metric, where we added a penalty term that accounts for the trend direction. More details are provided in the “ Evaluation metrics ” section.
Feature extraction
Below, we provide the details of the process that transforms raw data into numerical features, obtaining the ground truth NoI and the additional features NoM, ACA and PH.
NoI: The number of daily incidents in Hackmageddon was transformed from the purely unstructured daily description of attacks along with the attack and country columns, to the monthly count of incidents for each attack in each country. Within the description, multiple related attacks may appear, which are not necessarily in the attack column. Let \(E_{x_i}\) denote the set of entries during the month \(x_i\) in Hackmageddon dataset. Let \(a_j\) and \(c_k\) denote the j th attack and k th country. Then NoI can be expressed as follows:
where \(Z(a_j,c_k,e)\) is a function that evaluates to 1 if \(a_j\) appears either in the description or in the attack columns of entry e and \(c_k\) appears in the country column of e . Otherwise, the function evaluates to 0. Next, we performed CA to obtain the monthly count of attacks in all countries combined for each attack type as follows:
NoM: We wrote a Python script to query Elsevier API for the number of mentions of each attack during each month 31 . The search covers the title, abstract and keywords of published research papers that are stored in Scopus database 39 . Let \(P_{x_i}\) denote the set of research papers in Scopus published during the month \(x_i\) . Also, let \(W_{p}\) denote the set of words in the title, abstract and keywords of research paper p . Then NoM can be expressed as follows:
where \(U(w,a_j)\) evaluates to 1 if \(w=a_j\) , and to 0 otherwise.
ACA: Using Twitter API in Python 32 , we wrote a query to obtain the number of tweets with keywords related to political conflicts or military attacks associated with each country during each month. The keywords used for each country are summarised in Supplementary Table S2 , representing our query. Formally, let \(T_{x_i}\) denote the set of all tweets during the month \(x_i\) . Then ACA can be expressed as follows:
where \(Q(t,c_k)\) evaluates to 1 if the query in Supplementary Table S2 evaluates to 1 given t and \(c_k\) . Otherwise, it evaluates to 0.
PH: We used the Python holidays library 33 to count the number of days that are considered public holidays in each country during each month. More formally, this can be expressed as follows:
where \(H(d,c_k)\) evaluates to 1 if the day d in the country \(c_k\) is a public holiday, and to 0 otherwise. In ( 4 ) and ( 5 ), CA was used to obtain the count for all countries combined as in ( 2 ).
Data integration
Based on Eqs. ( 1 )–( 5 ), we obtain the following columns for each month:
NoI_C: The number of incidents for each attack type in each country ( \(42 \times 36\) columns) [Hackmageddon].
NoI: The total number of incidents for each attack type (42 columns) [Hackmageddon].
NoM: The number of mentions of each attack type in research articles (42 columns) [Elsevier].
ACA_C: The number of tweets about wars and conflicts related to each country (36 columns) [Twitter].
ACA: The total number of tweets about wars and conflicts (1 column) [Twitter].
PH_C: The number of public holidays in each country (36 columns) [Python].
PH: The total number of public holidays (1 column) [Python].
In the aforementioned list of columns, the name enclosed within square brackets denotes the source of data. By matching and combining these columns, we derive our monthly dataset, wherein each row represents a distinct month. A concrete example can be found in Tables 3 and 4 , which, taken together, constitute a single observation in our dataset. The dataset can be expanded through the inclusion of other monthly features as supplementary columns. Additionally, the dataset may be augmented with further samples as additional monthly records become available. Some suggestions for extending the dataset are provided in the “ Discussion ” section.
Data smoothing
We tested multiple smoothing methods and selected the one that resulted in the model with the lowest M-SMAPE during the hyper-parameter optimisation process. The methods we tested include exponential smoothing (ES), double exponential smoothing (DES) and no smoothing (NS). Let \(\alpha \) be the smoothing constant. Then the ES formula is:
where \(D(x_{i})\) denotes the original data at month \(x_{i}\) . For the DES formula, let \(\alpha \) and \(\beta \) be the smoothing constants. We first define the level \(l(x_{i})\) and the trend \(\tau (x_{i})\) as follows:
then, DES is expressed as follows:
The smoothing constants ( \(\alpha \) and \(\beta \) ) in the aforementioned methods are chosen as the predictive results of the ML model that gives the lowest M-SMAPE during the hyper-parameter optimisation process. Supplementary Fig. S5 depicts an example for the DES result.
Bayesian long short-term memory
LSTM is a type of recurrent neural network (RNN) that uses lagged observations to forecast the future time steps 30 . It was introduced as a solution to the so-called vanishing/exploding gradient problem of traditional RNNs 40 , where the partial derivative of the loss function may suddenly approach zero at some point of the training. In LSTM, the input is passed to the network cell, which combines it with the hidden state and cell state values from previous time steps to produce the next states. The hidden state can be thought of as a short-term memory since it stores information from recent periods in a weighted manner. On the other hand, the cell state is meant to remember all the past information from previous intervals and store them in the LSTM cell. The cell state thus represents the long-term memory.
LSTM networks are well-suited for time-series forecasting, due to their proficiency in retaining both long-term and short-term temporal dependencies 41 , 42 . By leveraging their ability to capture these dependencies within cyber-attack data, LSTM networks can effectively recognise recurring patterns in the attack time-series. Moreover, the LSTM model is capable of learning intricate temporal patterns in the data and can uncover inter-correlations between various variables, making it a compelling option for multivariate time-series analysis 43 .
Given a sequence of LSTM cells, each processing a single time-step from the past, the final hidden state is encoded into a fixed-length vector. Then, a decoder uses this vector to forecast future values. Using such architecture, we can map a sequence of time steps to another sequence of time steps, where the number of steps in each sequence can be set as needed. This technique is referred to as encoder-decoder architecture.
Because we have relatively short sequences within our refined data ( e.g. , 129 monthly data points over the period from July 2011 to March 2022), it is crucial to extract the source of uncertainty, known as epistemic uncertainty 44 , which is caused by lack of knowledge. In principle, epistemic uncertainty can be reduced with more knowledge either in the form of new features or more samples. Deterministic (non-stochastic) neural network models are not adequate to this task as they provide point estimates of model parameters. Rather, we utilise a Bayesian framework to capture epistemic uncertainty. Namely, we adopt the Monte Carlo dropout method proposed by Gal et al. 45 , who showed that the use of non-random dropout neurons during ML training (and inference) provides a Bayesian approximation of the deep Gaussian processes. Specifically, during the training of our LSTM encoder-decoder network, we applied the same dropout mask at every time-step (rather than applying a dropout mask randomly from time-step to time-step). This technique, known as recurrent dropout is readily available in Keras 46 . During the inference phase, we run trained model multiple times with recurrent dropout to produce a distribution of predictive results. Such prediction is shown in Fig. 4 .
Figure 2 shows our encoder-decoder B-LSTM architecture. The hidden state and cell state are denoted respectively by \(h_{i}\) and \(C_{i}\) , while the input is denoted by \(X_{i}\) . Here, the length of the input sequence (lag) is a hyper-parameter tuned to produce the optimal model, where the output is a single time-step. The number of cells ( i.e. , the depth of each layer) is tuned as a hyper-parameter in the range between 25 and 200 cells. Moreover, we used one or two layers, tuning the number of layers to each attack type. For the univariate model we used a standard Rectified Linear Unit (ReLU) activation function, while for the multivariate model we used a Leaky ReLU. Standard ReLU computes the function \(f(x)=max(0,x)\) , thresholding the activation at zero. In the multivariate case, zero-thresholding may generate the same ReLU output for many input vectors, making the model convergence slower 47 . With Leaky ReLU, instead of defining ReLU as zero when \(x < 0\) , we introduce a negative slope \(\alpha =0.2\) . Additionally, we used recurrent dropout ( i.e. , arrows in red as shown in Fig. 2 ), where the probability of dropping out is another hyper-parameter that we tune as described above, following Gal’s method 48 . The tuned dropout value is maintained during the testing and prediction as previously mentioned. Once the final hidden vector \(h_{0}\) is produced by the encoder, the Repeat Vector layer is used as an adapter to reshape it from the bi-dimensional output of the encoder ( e.g. , \(h_{0}\) ) to the three-dimensional input expected by the decoder. The decoder processes the input and produces the hidden state, which is then passed to a dense layer to produce the final output.
Each time-step corresponds to a month in our model. Since the model is learnt to predict a single time-step (single month), we use a sliding window during the prediction phase to forecast 36 (monthly) data points. In other words, we predict a single month at each step, and the predicted value is fed back for the prediction of the following month. This concept is illustrated in the table shown in Fig. 2 . Utilising a single time-step in the model’s output minimises the size of the sliding window, which in turn allows for training with as many observations as possible with such limited data.
The difference between the univariate and multivariate B-LSTMs is that the latter carries additional features in each time-step. Thus, instead of passing a scalar input value to the network, we pass a vector of features including the ground truth at each time-step. The model predicts a vector of features as an output, from which we retrieve the ground truth, and use it along with the other predicted features as an input to predict the next time-step.
Evaluation metrics
The evaluation metric SMAPE is a percentage (or relative) error based accuracy measure that judges the prediction performance purely on how far the predicted value is from the actual value 49 . It is expressed by the following formula:
where \(F_{t}\) and \(A_{t}\) denote the predicted and actual values at time t . This metric returns a value between 0% and 100%. Given that our data has zero values in some months ( e.g. , emerging threats), the issue of division by zero may arise, a problem that often emerges when using standard MAPE (Mean Absolute Percentage Error). We find SMAPE to be resilient to this problem, since it has both the actual and predicted values in the denominator.
Recall that our model aims to predict a curve (corresponding to multiple time steps). Using plain SMAPE as the evaluation metric, the “best” model may turn out to be simply a straight line passing through the same points of the fluctuating actual curve. However, this is undesired in our case since our priority is to predict the trend direction (or slope) over its intensity or value at a certain point. We hence add a penalty term to SMAPE that we apply when the height of the predicted curve is relatively smaller than that of the actual curve. This yields the modified SMAPE (M-SMAPE). More formally, let I ( V ) be the height of the curve V , calculated as follows:
where n is the curve width or the number of data points. Let A and F denote the actual and predicted curves. We define M-SMAPE as follows:
where \(\gamma \) is a penalty constant between 0 and 1, and d is another constant \(\ge \) 1. In our experiment, we set \(\gamma \) to 0.3, and d to 3, as we found these to be reasonable values by trial and error. We note that the range of possible values of M-SMAPE is between 0% and (100 + 100 \(\gamma \) )% after this modification. By running multiple experiments we found out that the modified evaluation metric is more suitable for our scenario, and therefore was adopted for the model’s evaluation.
Optimisation
On average, our model was trained on around 67% of the refined data, which is equivalent to approximately 7.2 years. We kept the rest, approximately 33% (3 years + lag period), for validation. These percentages may slightly differ for different attack types depending on the optimal lag period selected.
For hyper-parameter optimisation, we performed a random search with 60 iterations, to obtain the set of features, smoothing methods and constants, and model’s hyper-parameters that results in the model with the lowest M-SMAPE. Random search is a simple and efficient technique for hyper-parameter optimisation, with advantages including efficiency, flexibility, robustness, and scalability. The technique has been studied extensively in the literature and was found to be superior to grid search in many cases 50 . For each set of hyper-parameters, the model was trained using the mean squared error (MSE) as the loss function, and while using ADAM as the optimisation algorithm 51 . Then, the model was validated by forecasting 3 years while using M-SMAPE as the evaluation metric, and the average performance was recorded over 3 different seeds. Once the set of hyper-parameters with the minimum M-SMAPE was obtained, we used it to train the model on the full data, after which we predicted the trend for the next 3 years (until March, 2025).
The first group of hyper-parameters is the subset of features in the case of the multivariate model. Here, we experimented with each of the 3 features separately (NoM, ACA or PH) along with the ground truth (NoI), in addition to the combination of all features. The second group is the smoothing methods and constants. The set of methods includes ES, DES and NS, as previously discussed. The set of values for the smoothing constant \(\alpha \) ranges from 0.05 to 0.7 while the set of values for the smoothing constant \(\beta \) (for DES) ranges from 0.3 to 0.7. Next is the optimisation of the lag period with values that range from 1 to 12 months. This is followed by the model’s hyper-parameters which include the learning rate with values that range from \(6\times 10^{-4}\) to \(1\times 10^{-2}\) , the number of epochs with values between 30 and 200, the number of layers in the range 1 to 2, the number of units in the range 25 to 200, and the recurrent dropout value between 0.2 and 0.5. The range of these values was obtained from the literature and the online code repositories 52 .
Validation and comparative analysis
The results of our model’s validation are provided in Fig. 3 and Table 5 . As shown in Fig. 3 , the predicted data points are well aligned with the ground truth. Our models successfully predicted the next 36 months of all the attacks’ trends with an average M-SMAPE of 0.25. Table 5 summarises the validation results of univariate and multivariate approaches using B-LSTM. The results show that with approximately 69% of all the attack types, the multivariate approach outperformed the univariate approach. As seen in Fig. 3 , the threats that have a consistent increasing or emerging trend seemed to be more suitable for the univariate approach, while threats that have a fluctuating or decreasing trend showed less validation error when using the multivariate approach. The feature of ACA resulted in the best model for 33% of all the attack types, which makes it among the three most informative features that can boost the prediction performance. The PH accounts for 17% of all the attacks followed by NoM that accounts for 12%.
We additionally compared the performance of the proposed model B-LSTM with other models namely LSTM and ARIMA. The comparison covers the univariate and multivariate approaches of LSTM and B-LSTM, with two features in the case of multivariate approach namely NoI and NoM. The comparison is in terms of the Mean Absolute Percentage Error (MAPE) when predicting four common attack types, namely DDoS, Password Attack, Malware, and Ransomware. A comparison table is provided in Supplementary Table S3 . The results illustrate the superiority of the B-LSTM model for most of the attack types.
Trends analysis
The forecast of each attack trend until the end of the first quarter of 2025 is given in Supplementary Figs. S1 – S4 . By visualising the historical data of each attack as well as the prediction for the next three years, we were able to analyse the overall trend of each attack. The attacks generally follow 4 types of trends: (1) rapidly increasing, (2) overall increasing, (3) emerging and (4) decreasing. The names of attacks for each category are provided in Fig. 4 .
The first trend category is the rapidly increasing trend (Fig. 4 a—approximately 40% of the attacks belong to this trend. We can see that the attacks belonging to this category have increased dramatically over the past 11 years. Based on the model’s prediction, some of these attacks will exhibit a steep growth until 2025. Examples include session hijacking, supply chain, account hijacking, zero-day and botnet. Some of the attacks under this category have reached their peak, have recently started stabilising, and will probably remain steady over the next 3 years. Examples include malware, targeted attack, dropper and brute force attack. Some attacks in this category, after a recent increase, are likely to level off in the next coming years. These are password attack, DNS spoofing and vulnerability-related attacks.
The second trend category is the overall increasing trend as seen in Fig. 4 b. Approximately 31% of the attacks seem to follow this trend. The attacks under this category have a slower rate of increase over the years compared to the attacks in the first category, with occasional fluctuations as can be observed in the figure. Although some of the attacks show a slight recent decline ( e.g. , malvertising, keylogger and URL manipulation), malvertising and keylogger are likely to recover and return to a steady state while URL manipulation is projected to continue a smooth decline. Other attacks typical of “cold” cyber-warfare like Advanced Persistent Threats (APT) and rootkits are already recovering from a small drop and will likely to rise to a steady state by 2025. Spyware and data breach have already reached their peak and are predicted to decline in the near future.
Next is the emerging trend as shown in Fig. 4 c. These are the attacks that started to grow significantly after the year 2016, although many of them existed much earlier. In our study, around 17% of the attacks follow this trend. Some attacks have been growing steeply and are predicted to continue this trend until 2025. These are Internet of Things (IoT) device attack and deepfake. Other attacks have also been increasing rapidly since 2016, however, are likely to slow down after 2022. These include ransomware and adversarial attacks. Interestingly, some attacks that emerged after 2016 have already reached the peak and recently started a slight decline ( e.g. , cryptojacking and WannaCry ransomware attack). It is likely that WannaCry will become relatively steady in the coming years, however, cryptojacking will probably continue to decline until 2025 thanks to the rise of proof-of-stake consensus mechanisms 53 .
The fourth and last trend category is the decreasing trend (Fig. 4 d—only 12% of the attacks follow this trend. Some attacks in this category peaked around 2012, and have been slowly decreasing since then ( e.g. , SQL Injection and defacement). The drive-by attack also peaked in 2012, however, had other local peaks in 2016 and 2018, after which it declined noticeably. Cross-site scripting (XSS) and pharming had their peak more recently compared to the other attacks, however, have been smoothly declining since then. All the attacks under this category are predicted to become relatively stable from 2023 onward, however, they are unlikely to disappear in the next 3 years.
The threat cycle
This large-scale analysis involving the historical data and the predictions for the next three years enables us to come up with a generalisable model that traces the evolution and adoption of the threats as they pass through successive stages. These stages are named by the launch, growth, maturity, trough and stability/decline. We refer to this model as The Threat Cycle (or TTC), which is depicted in Fig. 5 . In the launch phase, few incidents start appearing for a short period. This is followed by a sharp increase in terms of the number of incidents, growth and visibility as more and more cyber actors learn and adopt this new attack. Usually, the attacks in the launch phase are likely to have many variants as observed in the case of the WannaCry attack in 2017. At some point, the number of incidents reaches a peak where the attack enters the maturity phase, and the curve becomes steady for a while. Via the trough (when the attack experiences a slight decline as new security measures seem to be very effective), some attacks recover and adapt to the security defences, entering the slope of plateau, while others continue to smoothly decline although they do not completely disappear ( i.e. , slope of decline). It is worth noting that the speed of transition between the different phases may vary significantly between the attacks.
As seen in Fig. 5 , the attacks are placed on the cycle based on the slope of their current trend, while considering their historical trend and prediction. In the trough phase, we can see that the attacks will either follow the slope of plateau or the slope of decline. Based on the predicted trend in the blue zone in Fig. 4 , we were able to indicate the future direction for some of the attacks close to the split point of the trough using different colours (blue or red). Brute force, malvertising, the Distributed Denial-of-Service attack (DDoS), insider threat, WannaCry and phishing are denoted in blue meaning that these are likely on their way to the slope of plateau. In the first three phases, it is usually unclear and difficult to predict whether a particular attack will reach the plateau or decline, thus, denoted in grey.
There are some similarities and differences between TTC and the well-known Gartner hype cycle (GHC) 54 . A standard GHC is shown in a vanishing green colour in Fig. 5 . As TTC is specific to cyber threats, it has a much wider peak compared to GHC. Although both GHC and TTC have a trough phase, the threats decline slightly (while significant drop in GHC) as they exit their maturity phase, after which they recover and move to stability (slope of plateau) or decline.
Many of the attacks in the emerging category are observed in the growth phase. These include IoT device attack, deepfake and data poisoning. While ransomwares (except WannaCry) are in the growth phase, WannaCry already reached the trough, and is predicted to follow the slope of plateau. Adversarial attack has just entered the maturity stage, and cryptojacking is about to enter the trough. Although adversarial attack is generally regarded as a growing threat, interestingly, this machine-based prediction and introspection shows that it is maturing. The majority of the rapidly increasing threats are either in the growth or in the maturity phase. The attacks in the growth phase include session hijacking, supply chain, account hijacking, zero-day and botnet. The attacks in the maturity phase include malware, targeted attack, vulnerability-related attacks and Man-In-The-Middle attack (MITM). Some rapidly increasing attacks such as phishing, brute force, and DDoS are in the trough and are predicted to enter the stability. We also observe that most of the attacks in the category of overall increasing threats have passed the growth phase and are mostly branching to the slope of plateau or the slope of decline, while few are still in the maturity phase ( e.g. , spyware). All of the decreasing threats are on the slope of decline. These include XSS, pharming, drive-by, defacement and SQL injection.
Highlights and limitations
This study presents the development of a ML-based proactive approach for long-term prediction of cyber-attacks offering the ability to communicate effectively with the potential attacks and the relevant security measures in an early stage to plan for the future. This approach can contribute to the prevention of an incident by allowing more time to develop optimal defensive actions/tools in a contested cyberspace. Proactive approaches can also effectively reduce uncertainty when prioritising existing security measures or initiating new security solutions. We argue that cyber-security agencies should prioritise their resources to provide the best possible support in preventing fastest-growing attacks that appear in the launch phase of TTC or the attacks in the categories of the rapidly increasing or emerging trend as in Fig. 4 a and c based on the predictions in the coming years.
In addition, our fully automated approach is promising to overcome the well-known issues of human-based analysis, above all expertise scarcity. Given the absence of the possibility of analysing with human’s subjective bias while following a purely quantitative procedure and data, the resulting predictions are expected to have lower degree of subjectivity, leading to consistencies within the subject. By fully automating this analytic process, the results are reproducible and can potentially be explainable with help of the recent advancements in Explainable Artificial Intelligence.
Thanks to the massive data volume and wide geographic coverage of the data sources we utilised, this study covers every facet of today’s cyber-attack scenario. Our holistic approach performs the long-term prediction on the scale of 36 countries, and is not confined to a specific region. Indeed, cyberspace is limitless, and a cyber-attack on critical infrastructure in one country can affect the continent as a whole or even globally. We argue that our Threat Cycle (TTC) provides a sound basis to awareness of and investment in new security measures that could prevent attacks from taking place. We believe that our tool can enable a collective defence effort by sharing the long-term predictions and trend analysis generated via quantitative processes and data and furthering the analysis of its regional and global impacts.
Zero-day attacks exploit a previously unknown vulnerability before the developer has had a chance to release a patch or fix for the problem 55 . Zero-day attacks are particularly dangerous because they can be used to target even the most secure systems and go undetected for extended periods of time. As a result, these attacks can cause significant damage to an organisation’s reputation, financial well-being, and customer trust. Our approach takes the existing research on using ML in the field of zero-day attacks to another level, offering a more proactive solution. By leveraging the power of deep neural networks to analyse complex, high-dimensional data, our approach can help agencies to prepare ahead of time, in-order to prevent the zero-day attack from happening at the first place, a problem that there is no existing fix for it despite our ability to detect it. Our results in Fig. 4 a suggest that zero-day attack is likely to continue a steep growth until 2025. If we know this information, we can proactively invest on solutions to prevent it or slow down its rise in the future, since after all, the ML detection approaches may not be alone sufficient to reduce its effect.
A limitation of our approach is its reliance on a restricted dataset that encompasses data since 2011 only. This is due to the challenges we encountered in accessing confidential and sensitive information. Extending the prediction phase requires the model to make predictions further into the future, where there may be more variability and uncertainty. This could lead to a decrease in prediction accuracy, especially if the underlying data patterns change over time or if there are unforeseen external factors that affect the data. While not always the case, this uncertainty is highlighted by the results of the Bayesian model itself as it expresses this uncertainty through the increase of the confidence interval over time (Fig. 3 a and b). Despite incorporating the Bayesian model to tackle the epistemic uncertainty, our model could benefit substantially from additional data to acquire a comprehensive understanding of past patterns, ultimately improving its capacity to forecast long-term trends. Moreover, an augmented dataset would allow ample opportunity for testing, providing greater confidence in the model’s resilience and capability to generalise.
Further enhancements can be made to the dataset by including pivotal dates (such as anniversaries of political events and war declarations) as a feature, specifically those that experience a high frequency of cyber-attacks. Additionally, augmenting the dataset with digital traces that reflect the attackers’ intentions and motivations obtained from the dark web would be valuable. Other informative features could facilitate short-term prediction, specifically to forecast the on-set of each attack.
Future work
Moving forward, future research can focus on augmenting the dataset with additional samples and informative features to enhance the model’s performance and its ability to forecast the trend in the longer-term. Also, the work opens a new area of research that focuses on prognosticating the disparity between the trend of cyber-attacks and the associated technological solutions and other variables, with the aim of guiding research investment decisions. Subsequently, TTC could be improved by adopting another curve model that can visualise the current development of relevant security measures. The threat trend categories (Fig. 4 ) and TTC (Fig. 5 ) show how attacks will be visible in the next three years and more, however, we do not know where the relevant security measures will be. For example, data poisoning is an AI-targeted adversarial attack that attempts to manipulate the training dataset to control the prediction behaviour of a machine-learned model. From the scientific literature data ( e.g. , Scopus), we could analyse the published articles studying the data poisoning problem and identify the relevant keywords of these articles ( e.g. , Reject on Negative Impact (RONI) and Probability of Sufficiency (PS)). RONI and PS are typical methods used for detecting poisonous data by evaluating the effect of individual data points on the performance of the trained model. Likewise, the features that are informative, discriminating or uncertainty-reducing for knowing how the relevant security measures evolve exist within such online sources in the form of author’s keywords, number of citations, research funding, number of publications, etc .
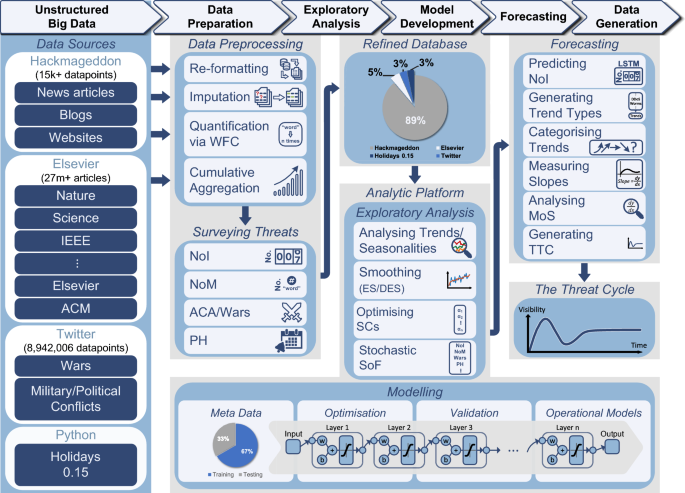
The workflow and architecture of forecasting cyber threats. The ground truth of Number of Incidents (NoI) was extracted from Hackmageddon which has over 15,000 daily records of cyber incidents worldwide over the past 11 years. Additional features were obtained including the Number of Mentions (NoM) of each attack in the scientific literature using Elsevier API which gives access to over 27 million documents. The number of tweets about Armed Conflict Areas/Wars (ACA) was also obtained using Twitter API for each country, with a total of approximately 9 million tweets. Finally, the number of Public Holidays (PH) in each country was obtained using the holidays library in Python. The data preparation phase includes data re-formatting, imputation and quantification using Word Frequency Counter (WFC) to obtain the monthly occurrence of attacks per country and Cumulative Aggregation (CA) to obtain the sum for all countries. The monthly NoM, ACA and PHs were quantified and aggregated using CA. The numerical features were then combined and stored in the refined database. The percentages in the refined database are based on the contribution of each data source. In the exploratory analysis phase, the analytic platform analyses the trend and performs data smoothing using Exponential Smoothing (ES), Double Exponential Smoothing (DES) and No Smoothing (NS). The smoothing methods and Smoothing Constants (SCs) were chosen for each attack followed by the Stochastic Selection of Features (SoF). In the model development phase, the meta data was partitioned into approximately 67% for training and 33% for testing. The models were learned using the encoder-decoder architecture of the Bayesian Long Short-Term Memory (B-LSTM). The optimisation component finds the set of hyper-parameters that minimises the error (i.e., M-SMAPE), which is then used for learning the operational models. In the forecasting phase, we used the operational models to predict the next three years’ NoIs. Analysing the predicted data, trend types were identified and attacks were categorised into four different trends. The slope of each attack was then measured and the Magnitude of Slope (MoS) was analysed. The final output is The Threat Cycle (TTC) illustrating the attacks trend, status, and direction in the next 3 years.
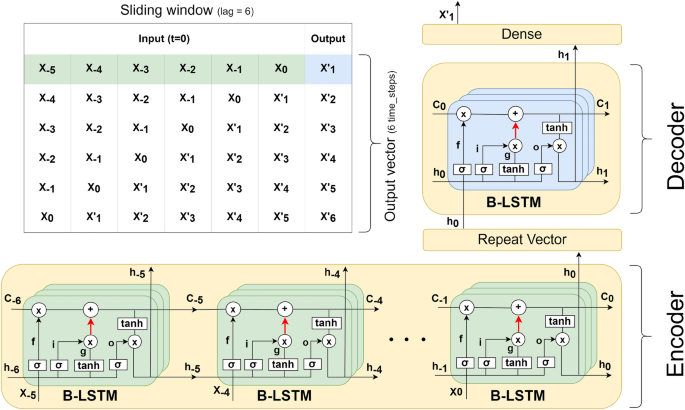
The encoder-decoder architecture of Bayesian Long Short-Term Memory (B-LSTM). \(X_{i}\) stands for the input at time-step i . \(h_{i}\) stands for the hidden state, which stores information from the recent time steps (short-term). \(C_{i}\) stands for the cell state, which stores all processed information from the past (long-term). The number of input time steps in the encoder is a variable tuned as a hyper-parameter, while the output in the decoder is a single time-step. The depth and number of layers are another set of hyper-parameters tuned during the model optimisation. The red arrows indicate a recurrent dropout maintained during the testing and prediction. The figure shows an example for an input with time lag=6 and a single layer. The final hidden state \(h_{0}\) produced by the encoder is passed to the Repeat Vector layer to convert it from 2 dimensional output to 3 dimensional input as expected by the decoder. The decoder processes the input and produces the final hidden state \(h_{1}\) . This hidden state is finally passed to a dense layer to produce the output. The table illustrates the concept of sliding window method used to forecast multiple time steps during the testing and prediction (i.e., using the output at a time-step as an input to forecast the next time-step). Using this concept, we can predict as many time steps as needed. In the table, an output vector of 6 time steps was predicted.
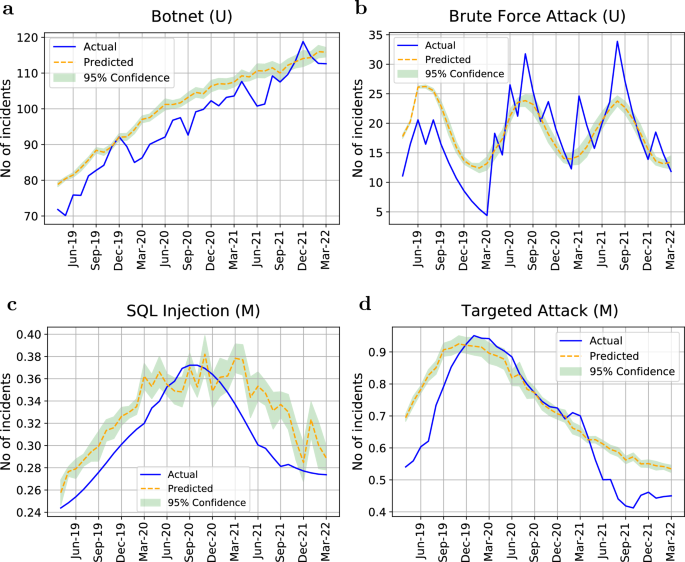
The B-LSTM validation results of predicting the number of attacks from April, 2019 to March, 2022. (U) indicates an univariate model while (M) indicates a multivariate model. ( a ) Botnet attack with M-SMAPE=0.03. ( b ) Brute force attack with M-SMAPE=0.13. ( c ) SQL injection attack with M-SMAPE=0.04 using the feature of NoM. ( d ) Targeted attack with M-SMAPE=0.06 using the feature of NoM. Y axis is normalised in the case of multivariate models to account for the different ranges of feature values.
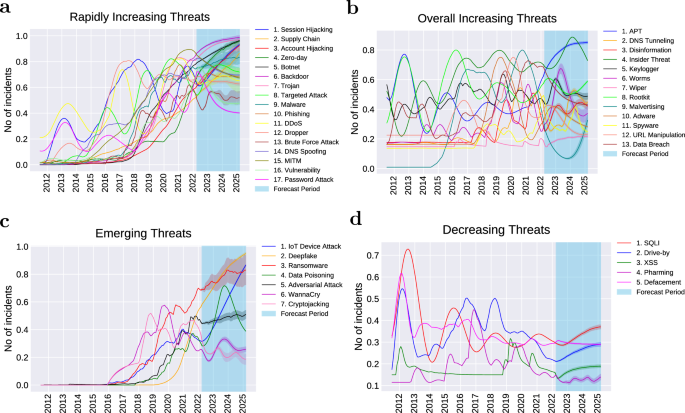
A bird’s eye view of threat trend categories. The period of the trend plots is between July, 2011 and March, 2025, with the period between April, 2022 and March, 2025 forecasted using B-LSTM. ( a ) Among rapidly increasing threats, as observed in the forecast period, some threats are predicted to continue a sharp increase until 2025 while others will probably level off. ( b ) Threats under this category have overall been increasing while fluctuating over the past 11 years. Recently, some of the overall increasing threats slightly declined however many of those are likely to recover and level off by 2025. ( c ) Emerging threats that began to appear and grow sharply after the year 2016, and are expected to continue growing at this increasing rate, while others are likely to slow down or stabilise by 2025. ( d ) Decreasing threats that peaked in the earlier years and have slowly been declining since then. This decreasing group are likely to level off however probably will not disappear in the coming 3 years. The Y axis is normalised to account for the different ranges of values across different attacks. The 95% confidence interval is shown for each threat prediction.
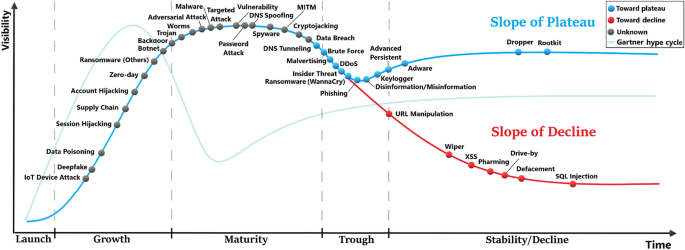
The threat cycle (TTC). The attacks go through 5 stages, namely, launch, growth, maturity trough, and stability/decline. A standard Gartner hype cycle (GHC) is shown with a vanishing green colour for a comparison to TTC. Both GHC and TTC have a peak, however, TTC’s peak is much wider with a slightly less steep curve during the growth stage. Some attacks in TTC do not recover after the trough and slide into the slope of decline. TTC captures the state of each attack in 2022, where the colour of each attack indicates which slope it would follow (e.g., plateau or decreasing) based on the predictive results until 2025. Within the trough stage, the attacks (in blue dot) are likely to arrive at the slope of plateau by 2025. The attacks (in red dot) will probably be on the slope of decline by 2025. The attacks with unknown final destination are coloured in grey.
Data availability
As requested by the journal, the data used in this paper is available to editors and reviewers upon request. The data will be made publicly available and can be accessed at the following link after the paper is published. https://github.com/zaidalmahmoud/Cyber-threat-forecast .
Ghafur, S. et al. A retrospective impact analysis of the wannacry cyberattack on the NHS. NPJ Digit. Med. 2 , 1–7 (2019).
Article Google Scholar
Alrzini, J. R. S. & Pennington, D. A review of polymorphic malware detection techniques. Int. J. Adv. Res. Eng. Technol. 11 , 1238–1247 (2020).
Google Scholar
Lazarevic, A., Ertoz, L., Kumar, V., Ozgur, A. & Srivastava, J. A comparative study of anomaly detection schemes in network intrusion detection. In: Proceedings of the 2003 SIAM International Conference on Data Mining , 25–36 (SIAM, 2003).
Kebir, O., Nouaouri, I., Rejeb, L. & Said, L. B. Atipreta: An analytical model for time-dependent prediction of terrorist attacks. Int. J. Appl. Math. Comput. Sci. 32 , 495–510 (2022).
MATH Google Scholar
Anticipating cyber attacks: There’s no abbottabad in cyber space. Infosecurity Magazine https://www.infosecurity-magazine.com/white-papers/anticipating-cyber-attacks (2015).
Jumper, J. et al. Highly accurate protein structure prediction with alphafold. Nature 596 , 583–589 (2021).
Article ADS CAS PubMed PubMed Central Google Scholar
Baek, M. et al. Accurate prediction of protein structures and interactions using a three-track neural network. Science 373 , 871–876 (2021).
Gibney, E. et al. Where is russia’s cyberwar? researchers decipher its strategy. Nature 603 , 775–776 (2022).
Article ADS CAS PubMed Google Scholar
Passeri, P. Hackmageddon data set. Hackmageddon https://www.hackmageddon.com (2022).
Chen, C.-M. et al. A provably secure key transfer protocol for the fog-enabled social internet of vehicles based on a confidential computing environment. Veh. Commun. 39 , 100567 (2023).
Nagasree, Y. et al. Preserving privacy of classified authentic satellite lane imagery using proxy re-encryption and UAV technologies. Drones 7 , 53 (2023).
Kavitha, A. et al. Security in IoT mesh networks based on trust similarity. IEEE Access 10 , 121712–121724 (2022).
Salih, A., Zeebaree, S. T., Ameen, S., Alkhyyat, A. & Shukur, H. M A survey on the role of artificial intelligence, machine learning and deep learning for cybersecurity attack detection. In: 2021 7th International Engineering Conference “Research and Innovation amid Global Pandemic” (IEC) , 61–66 (IEEE, 2021).
Ren, K., Zeng, Y., Cao, Z. & Zhang, Y. Id-rdrl: A deep reinforcement learning-based feature selection intrusion detection model. Sci. Rep. 12 , 1–18 (2022).
Liu, X. & Liu, J. Malicious traffic detection combined deep neural network with hierarchical attention mechanism. Sci. Rep. 11 , 1–15 (2021).
Werner, G., Yang, S. & McConky, K. Time series forecasting of cyber attack intensity. In Proceedings of the 12th Annual Conference on Cyber and Information Security Research , 1–3 (2017).
Werner, G., Yang, S. & McConky, K. Leveraging intra-day temporal variations to predict daily cyberattack activity. In 2018 IEEE International Conference on Intelligence and Security Informatics (ISI) , 58–63 (IEEE, 2018).
Okutan, A., Yang, S. J., McConky, K. & Werner, G. Capture: cyberattack forecasting using non-stationary features with time lags. In 2019 IEEE Conference on Communications and Network Security (CNS) , 205–213 (IEEE, 2019).
Munkhdorj, B. & Yuji, S. Cyber attack prediction using social data analysis. J. High Speed Netw. 23 , 109–135 (2017).
Goyal, P. et al. Discovering signals from web sources to predict cyber attacks. arXiv preprint arXiv:1806.03342 (2018).
Qin, X. & Lee, W. Attack plan recognition and prediction using causal networks. In 20th Annual Computer Security Applications Conference , 370–379 (IEEE, 2004).
Husák, M. & Kašpar, J. Aida framework: real-time correlation and prediction of intrusion detection alerts. In: Proceedings of the 14th international conference on availability, reliability and security , 1–8 (2019).
Liu, Y. et al. Cloudy with a chance of breach: Forecasting cyber security incidents. In: 24th USENIX Security Symposium (USENIX Security 15) , 1009–1024 (2015).
Malik, J. et al. Hybrid deep learning: An efficient reconnaissance and surveillance detection mechanism in sdn. IEEE Access 8 , 134695–134706 (2020).
Bilge, L., Han, Y. & Dell’Amico, M. Riskteller: Predicting the risk of cyber incidents. In Proceedings of the 2017 ACM SIGSAC Conference on Computer and Communications Security , 1299–1311 (2017).
Husák, M., Bartoš, V., Sokol, P. & Gajdoš, A. Predictive methods in cyber defense: Current experience and research challenges. Futur. Gener. Comput. Syst. 115 , 517–530 (2021).
Stephens, G. Cybercrime in the year 2025. Futurist 42 , 32 (2008).
Adamov, A. & Carlsson, A. The state of ransomware. Trends and mitigation techniques. In EWDTS , 1–8 (2017).
Shoufan, A. & Damiani, E. On inter-rater reliability of information security experts. J. Inf. Secur. Appl. 37 , 101–111 (2017).
Cha, Y.-O. & Hao, Y. The dawn of metamaterial engineering predicted via hyperdimensional keyword pool and memory learning. Adv. Opt. Mater. 10 , 2102444 (2022).
Article CAS Google Scholar
Elsevier research products apis. Elsevier Developer Portal https://dev.elsevier.com (2022).
Twitter api v2. Developer Platform https://developer.twitter.com/en/docs/twitter-api (2022).
holidays 0.15. PyPI. The Python Package Index https://pypi.org/project/holidays/ (2022).
Visser, M., van Eck, N. J. & Waltman, L. Large-scale comparison of bibliographic data sources: Scopus, web of science, dimensions, crossref, and microsoft academic. Quant. Sci. Stud. 2 , 20–41 (2021).
2021 trends show increased globalized threat of ransomware. Cybersecurity and Infrastructure Security Agency https://www.cisa.gov/uscert/ncas/alerts/aa22-040a (2022).
Lai, K. K., Yu, L., Wang, S. & Huang, W. Hybridizing exponential smoothing and neural network for financial time series predication. In International Conference on Computational Science , 493–500 (Springer, 2006).
Huang, B., Ding, Q., Sun, G. & Li, H. Stock prediction based on Bayesian-lstm. In Proceedings of the 2018 10th International Conference on Machine Learning and Computing , 128–133 (2018).
Mae, Y., Kumagai, W. & Kanamori, T. Uncertainty propagation for dropout-based Bayesian neural networks. Neural Netw. 144 , 394–406 (2021).
Article PubMed Google Scholar
Scopus preview. Scopus https://www.scopus.com/home.uri (2022).
Jia, P., Chen, H., Zhang, L. & Han, D. Attention-lstm based prediction model for aircraft 4-d trajectory. Sci. Rep. 12 (2022).
Chandra, R., Goyal, S. & Gupta, R. Evaluation of deep learning models for multi-step ahead time series prediction. IEEE Access 9 , 83105–83123 (2021).
Gers, F. A., Schmidhuber, J. & Cummins, F. Learning to forget: Continual prediction with lstm. Neural Comput. 12 , 2451–2471 (2000).
Article CAS PubMed Google Scholar
Sagheer, A. & Kotb, M. Unsupervised pre-training of a deep lstm-based stacked autoencoder for multivariate time series forecasting problems. Sci. Rep. 9 , 1–16 (2019).
Article ADS Google Scholar
Swiler, L. P., Paez, T. L. & Mayes, R. L. Epistemic uncertainty quantification tutorial. In Proceedings of the 27th International Modal Analysis Conference (2009).
Gal, Y. & Ghahramani, Z. Dropout as a bayesian approximation: Representing model uncertainty in deep learning. arXiv preprint arXiv:1506.02142v6 (2016).
Chollet, F. Deep Learning with Python , 2 edn. (Manning Publications, 2017).
Xu, J., Li, Z., Du, B., Zhang, M. & Liu, J. Reluplex made more practical: Leaky relu. In 2020 IEEE Symposium on Computers and Communications (ISCC) , 1–7 (IEEE, 2020).
Gal, Y., Hron, J. & Kendall, A. Concrete dropout. Adv. Neural Inf. Process. Syst. 30 (2017).
Shcherbakov, M. V. et al. A survey of forecast error measures. World Appl. Sci. J. 24 , 171–176 (2013).
Bergstra, J. & Bengio, Y. Random search for hyper-parameter optimization. J. Mach. Learn. Res. 13 (2012).
Kingma, D. P. & Ba, J. Adam: A method for stochastic optimization. arXiv preprint arXiv:1412.6980 (2014).
Krizhevsky, A., Sutskever, I. & Hinton, G. E. Imagenet classification with deep convolutional neural networks. Commun. ACM 60 , 84–90 (2017).
Shifferaw, Y. & Lemma, S. Limitations of proof of stake algorithm in blockchain: A review. Zede J. 39 , 81–95 (2021).
Dedehayir, O. & Steinert, M. The hype cycle model: A review and future directions. Technol. Forecast. Soc. Chang. 108 , 28–41 (2016).
Abri, F., Siami-Namini, S., Khanghah, M. A., Soltani, F. M. & Namin, A. S. Can machine/deep learning classifiers detect zero-day malware with high accuracy?. In 2019 IEEE International Conference on Big Data (Big Data) , 3252–3259 (IEEE, 2019).
Download references
Acknowledgements
The authors are grateful to the DASA’s machine learning team for their invaluable discussions and feedback, and special thanks to the EBTIC, British Telecom’s (BT) cyber security team for their constructive criticism on this work.
Author information
Authors and affiliations.
Department of Computer Science and Information Systems, University of London, Birkbeck College, London, United Kingdom
Zaid Almahmoud & Paul D. Yoo
Huawei Technologies Canada, Ottawa, Canada
Omar Alhussein
Department of Electrical and Computer Engineering, University of Waterloo, Waterloo, Canada
Ilyas Farhat
Department of Computer Science, Università degli Studi di Milano, Milan, Italy
Ernesto Damiani
Center for Cyber-Physical Systems (C2PS), Khalifa University, Abu Dhabi, United Arab Emirates
You can also search for this author in PubMed Google Scholar
Contributions
Z.A., P.D.Y, I.F., and E.D. were in charge of the framework design and theoretical analysis of the trend analysis and TTC. Z.A., O.A., and P.D.Y. contributed to the B-LSTM design and experiments. O.A. proposed the concepts of B-LSTM. All of the authors contributed to the discussion of the framework design and experiments, and the writing of this paper. P.D.Y. proposed the big data approach and supervised the whole project.
Corresponding author
Correspondence to Paul D. Yoo .
Ethics declarations
Competing interests.
The authors declare no competing interests.
Additional information
Publisher's note.
Springer Nature remains neutral with regard to jurisdictional claims in published maps and institutional affiliations.
Supplementary Information
Supplementary information., rights and permissions.
Open Access This article is licensed under a Creative Commons Attribution 4.0 International License, which permits use, sharing, adaptation, distribution and reproduction in any medium or format, as long as you give appropriate credit to the original author(s) and the source, provide a link to the Creative Commons licence, and indicate if changes were made. The images or other third party material in this article are included in the article's Creative Commons licence, unless indicated otherwise in a credit line to the material. If material is not included in the article's Creative Commons licence and your intended use is not permitted by statutory regulation or exceeds the permitted use, you will need to obtain permission directly from the copyright holder. To view a copy of this licence, visit http://creativecommons.org/licenses/by/4.0/ .
Reprints and permissions
About this article
Cite this article.
Almahmoud, Z., Yoo, P.D., Alhussein, O. et al. A holistic and proactive approach to forecasting cyber threats. Sci Rep 13 , 8049 (2023). https://doi.org/10.1038/s41598-023-35198-1
Download citation
Received : 21 December 2022
Accepted : 14 May 2023
Published : 17 May 2023
DOI : https://doi.org/10.1038/s41598-023-35198-1
Share this article
Anyone you share the following link with will be able to read this content:
Sorry, a shareable link is not currently available for this article.
Provided by the Springer Nature SharedIt content-sharing initiative
By submitting a comment you agree to abide by our Terms and Community Guidelines . If you find something abusive or that does not comply with our terms or guidelines please flag it as inappropriate.
Quick links
- Explore articles by subject
- Guide to authors
- Editorial policies
Sign up for the Nature Briefing: AI and Robotics newsletter — what matters in AI and robotics research, free to your inbox weekly.

- Workforce Risk Management
- Talent Matching
- Staff Augmentation
- Full Service Cyber Staffing
- Diversity Staffing
- CyberSN Platform
- View Professionals
- Build a Profile
- Join our Network
- Career Center
- 45 Cybersecurity Roles
- New to Cyber
- Leadership Team
- Podcasts and Videos
Security Researcher
Security researchers keep current with the latest cyber threats and threat actor techniques., role overview.
A Security Researcher stays informed on the current, new and emerging technology, proposed standards, and threat actors that could be used to exploit application and system vulnerabilities. They then examine its functions and present these findings to their organization or a larger audience, often creating proof of concept exploits as well. These professionals are immersed in technology with a desire to understand the inner workings of the research subject matter and other cybersecurity threats.
Security Researchers
Adversary researcher, appsec security research engineer, cloud security researcher, crypto and blockchain researcher, cyber attack researcher, cyber exploitation researcher, cyber-physical systems research engineer, cyber research advisor, cyber research analyst, cyber research scientist, cyber researcher, cybercrime research analyst, cybersecurity vulnerability researcher, embedded security researcher, exploit developer, exploit engineer, exploit researcher, exploitation analyst, exploitation and malware researcher sme, hardware exploitation researcher, insider threat researcher, mobile security researcher, network exploitation and vulnerability research analyst, research assistant cybersecurity researcher, research reverse engineering, research scientist security and privacy, researcher vulnerability exploitation, security research analyst, security research engineer, security research intern, security researcher (red team), systems analysis and exploitation researcher, technical cyber researcher, threat intelligence research engineer, threat researcher, vulnerability research engineer, vulnerability researcher, career path.

Average Salary
Responsibilities, tools & environment.
Security Researchers need a deep understanding of cybersecurity threats, exploits, and threat actor techniques involving hardware, software, networks, protocols, and architectures and their implications. They should also be able to use Static Application Security Testing (SAST) tools, debuggers, disassemblers, programming languages , and large datasets.
Certifications
[cyber_job_role_count] security researcher jobs, white papers, free courses.
Get our latest insights. Subscribe to our newsletter.

Workforce Solutions
Career development, dynamic title for modals, are you sure.
75 Cyber Security Research Topics in 2024
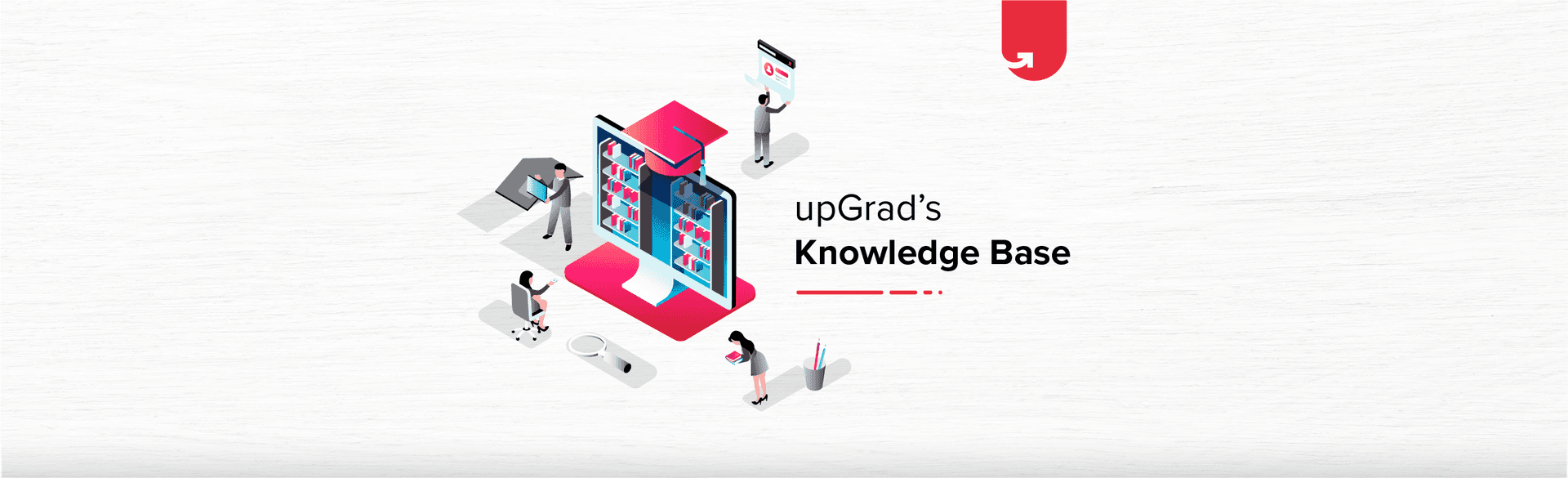
Introduction to Cybersecurity Research
Cybersecurity research aims to protect computer systems, networks, and data from unauthorised access, theft, or damage. It involves studying and developing methods and techniques to identify, understand, and mitigate cyber threats and vulnerabilities.
The field can be divided into theoretical and applied research and faces challenges such as
- Increasing complexity
- New forms of malware
- The growing sophistication of cyber attacks
On a daily basis, approximately 2,200 cyber attacks occur, with an average of one cyber attack happening every 39 seconds. This is the reason why researchers must stay up-to-date and collaborate with others in the field.
In this article, let’s discuss the different cybersecurity research topics and how they will help you become an expert in the field.
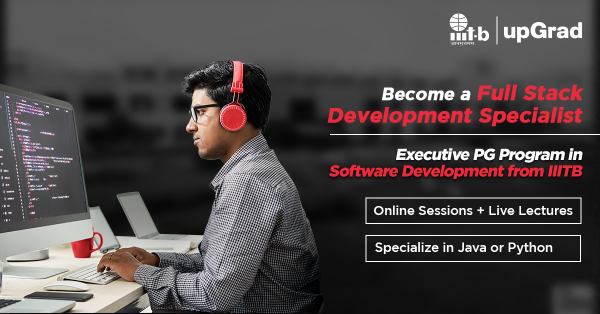
Check out our free technology courses to get an edge over the competition.
Here are some of the latest research topics in cyber security –
Emerging Cyber Threats and Vulnerabilities in 2024
Continual technological advancements lead to changes in cybersecurity trends, with data breaches, ransomware, and hacks becoming more prevalent.
- Cyber Attacks and Their Countermeasures – Discuss – This research paper will discuss various cyber attacks and their corresponding countermeasures. It aims to provide insights on how organisations can better protect themselves from cyber threats.
- Is Cryptography Necessary for Cybersecurity Applications? – Explore the role of cryptography in ensuring the confidentiality, integrity, and availability of data and information in cybersecurity. It would examine the various cryptographic techniques used in cybersecurity and their effectiveness in protecting against cyber threats.
Here are some other cyber security topics that you may consider –
- Discuss the Application of Cyber Security for Cloud-based Applications
- Data Analytics Tools in Cybersecurity
- Malware Analysis
- What Are the Behavioural Aspects of Cyber Security?
- Role of Cyber Security on Intelligent Transporation Systems
- How to Stop and Spot Different Types of Malware?
Check Out upGrad’s Software Development Courses to upskill yourself.
Machine Learning and AI in Cybersecurity Research
Machine learning and AI are research topics in cybersecurity, aiming to develop algorithms for threat detection, enhance intelligence and automate risk mitigation. However, security risks like adversarial attacks require attention.
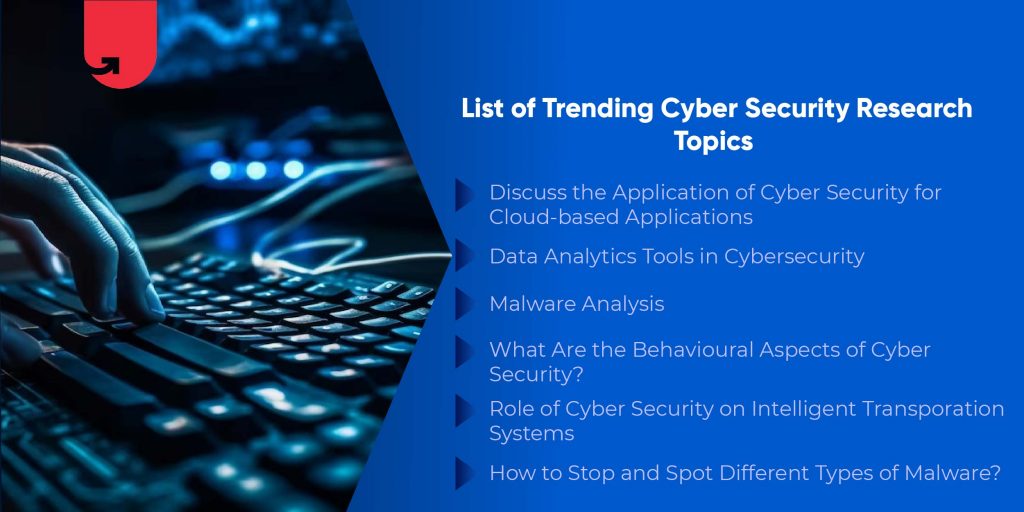
- Using AI/ML to Analyse Cyber Threats – This cyber security research paper analyses cyber threats and could include an overview of the current state of cyber threats and how AI/ML can help with threat detection and response. The paper could also discuss the challenges and limitations of using AI/ML in cybersecurity and potential areas for further research.
Here are some other topics to consider –
- Developing Cognitive Systems for Cyber Threat Detection and Response
- Developing Distributed Ai Systems to Enhance Cybersecurity
- Developing Deep Learning Architectures for Cyber Defence
- Exploring the Use of Computational Intelligence and Neuroscience in Enhancing Security and Privacy
- How is Cyber Security Relevant for Everyone? Discuss
- Discuss the Importance of Network Traffic Analysis
- How to Build an App to Break Ceasar Cipher
You can check out the Advanced Certificate Programme in Cyber Security course by upGrad, which will help students become experts in cyber security.
IoT Security and Privacy
IoT security and privacy research aim to develop secure and privacy-preserving architectures, protocols, and algorithms for IoT devices, including encryption, access control, and secure communication. The challenge is to balance security with usability while addressing the risk of cyber-attacks and compromised privacy.
- Service Orchestration and Routing for IoT – It may focus on developing efficient and secure methods for managing and routing traffic between IoT devices and services. The paper may explore different approaches for optimising service orchestration.
- Efficient Resource Management, Energy Harvesting, and Power Consumption in IoT – This paper may focus on developing strategies to improve energy use efficiency in IoT devices. This may involve investigating the use of energy harvesting technologies, optimising resource allocation and management, and exploring methods to reduce power consumption.
Here are some other cyber security project topics to consider –
- Computation and Communication Gateways for IoT
- The Miniaturisation of Sensors, Cpus, and Networks in IoT
- Big Data Analytics in IoT
- Semantic Technologies in IoT
- Virtualisation in IoT
- Privacy, Security, Trust, Identity, and Anonymity in IoT
- Heterogeneity, Dynamics, and Scale in IoT
- Consequences of Leaving Unlocked Devices Unattended
Explore our Popular Software Engineering Courses
Blockchain security: research challenges and opportunities.
Blockchain security research aims to develop secure and decentralised architectures, consensus algorithms, and privacy-preserving techniques while addressing challenges such as smart contract security and consensus manipulation. Opportunities include transparent supply chain management and decentralised identity management.
- Advanced Cryptographic Technologies in the Blockchain – Explore the latest advancements and emerging trends in cryptographic techniques used in blockchain-based systems. It could also analyse the security and privacy implications of these technologies and discuss their potential impact.
- Applications of Smart Contracts in Blockchain – Explore the various use cases and potential benefits of using smart contracts to automate and secure business processes. It could also examine the challenges and limitations of smart contracts and propose potential solutions for these issues.
Here are some other topics –
- Ensuring Data Consistency, Transparency, and Privacy in the Blockchain
- Emerging Blockchain Models for Digital Currencies
- Blockchain for Advanced Information Governance Models
- The Role of Blockchain in Future Wireless Mobile Networks
- Law and Regulation Issues in the Blockchain
- Transaction Processing and Modification in the Blockchain
- Collaboration of Big Data With Blockchain Networks
Cloud Security: Trends and Innovations in Research
Cloud security research aims to develop innovative techniques and technologies for securing cloud computing environments, including threat detection with AI, SECaaS, encryption and access control, secure backup and disaster recovery, container security, and blockchain-based solutions. The goal is to ensure the security, privacy, and integrity of cloud-based data and applications for organisations.
- Posture Management in Cloud Security – Discuss the importance of identifying and addressing vulnerabilities in cloud-based systems and strategies for maintaining a secure posture over time. This could include topics such as threat modelling, risk assessment, access control, and continuous monitoring.
- Are Cloud Services 100% Secure?
- What is the Importance of Cloud Security?
- Cloud Security Service to Identify Unauthorised User Behaviour
- Preventing Theft-of-service Attacks and Ensuring Cloud Security on Virtual Machines
- Security Requirements for Cloud Computing
- Privacy and Security of Cloud Computing
Explore Our Software Development Free Courses
Cybercrime investigations and forensics.
Cybercrime investigations and forensics involve analysing digital evidence to identify and prosecute cybercriminals, including developing new data recovery, analysis, and preservation techniques. Research also focuses on identifying cybercriminals and improving legal and regulatory frameworks for prosecuting cybercrime.
- Black Hat and White Hat Hacking: Comparison and Contrast – Explore the similarities and differences between these two approaches to hacking. It would examine the motivations and methods of both types of hackers and their impact on cybersecurity.
- Legal Requirements for Computer Forensics Laboratories
- Wireless Hacking Techniques: Emerging Technologies and Mitigation Strategies
- Cyber Crime: Current Issues and Threats
- Computer Forensics in Law Enforcement: Importance and Challenges
- Basic Procedures for Computer Forensics and Investigations
- Digital Forensic Examination of Counterfeit Documents: Techniques and Tools
- Cybersecurity and Cybercrime: Understanding the Nature and Scope
An integral part of cybercrime investigation is to learn software development. Become experts in this field with the help of upGrad’s Executive Post Graduate Programme in Software Development – Specialisation in Full Stack Development .
Cybersecurity Policy and Regulations
Cybersecurity policy and regulations research aims to develop laws, regulations, and guidelines to ensure the security and privacy of digital systems and data, including addressing gaps in existing policies, promoting international cooperation, and developing standards and best practices for cybersecurity. The goal is to protect digital systems and data while promoting innovation and growth in the digital economy.
- The Ethicality of Government Access to Citizens’ Data – Explore the ethical considerations surrounding government access to citizens’ data for surveillance and security purposes, analysing the potential risks and benefits and the legal and social implications of such access.
- The Moral Permissibility of Using Music Streaming Services – Explore the ethical implications of using music streaming services, examining issues such as intellectual property rights, artist compensation, and the environmental impact of streaming.
- Real Name Requirements on Internet Forums
- Restrictions to Prevent Domain Speculation
- Regulating Adult Content Visibility on the Internet
- Justification for Illegal Downloading
- Adapting Law Enforcement to Online Technologies
- Balancing Data Privacy With Convenience and Centralisation
- Understanding the Nature and Dangers of Cyber Terrorism
Human Factors in Cybersecurity
Human factors in cybersecurity research study how human behaviour impacts cybersecurity, including designing interfaces, developing security training, addressing user error and negligence, and examining cybersecurity’s social and cultural aspects. The goal is to improve security by mitigating human-related security risks.
- Review the Human Factors in Cybersecurity – It explores various human factors such as awareness, behaviour, training, and culture and their influence on cybersecurity, offering insights and recommendations for improving cybersecurity outcomes.
- Integrating Human Factors in Cybersecurity for Better Risk Management
- Address the Human Factors in Cybersecurity Leadership
- Human Factors in IoT Security
- Internal Vulnerabilities: the Human Factor in It Security
- Cyber Security Human Factors – the Ultimate List of Statistics and Data
In-Demand Software Development Skills
Cybersecurity education and awareness.
Cybersecurity education and awareness aims to educate individuals and organisations about potential cybersecurity threats and best practices to prevent cyber attacks. It involves promoting safe online behaviour, training on cybersecurity protocols, and raising awareness about emerging cyber threats.
- Identifying Phishing Attacks – This research paper explores various techniques and tools to identify and prevent phishing attacks, which are common types of cyber attacks that rely on social engineering tactics to trick victims into divulging sensitive information or installing malware on their devices.
- Risks of Password Reuse for Personal and Professional Accounts – Investigate the risks associated with reusing the same password across different personal and professional accounts, such as the possibility of credential stuffing attacks and the impact of compromised accounts on organisational security.
- Effective Defence Against Ransomware
- Information Access Management: Privilege and Need-to-know Access
- Protecting Sensitive Data on Removable Media
- Recognising Social Engineering Attacks
- Preventing Unauthorised Access to Secure Areas: Detecting Piggybacking and Tailgating
- E-mail Attack and Its Characteristics
- Safe Wifi Practice: Understanding VPN
With the increasing use of digital systems and networks, avoiding potential cyber-attacks is more important than ever. The 75 research topics outlined in this list offer a glimpse into the different dimensions of this important field. By focusing on these areas, researchers can make significant contributions to enhancing the security and safety of individuals, organisations, and society as a whole.
upGrad’s Master of Science in Computer Science program is one of the top courses students can complete to become experts in the field of tech and cyber security. The program covers topics such as Java Programming and other forms of software engineering which will help students understand the latest technologies and techniques used in cyber security.
The program also includes hands-on projects and case studies to ensure students have practical experience in applying these concepts. Graduates will be well-equipped to take on challenging roles in the rapidly growing field of cyber security.

Pavan Vadapalli
Something went wrong
Our Popular Software Engineering Courses
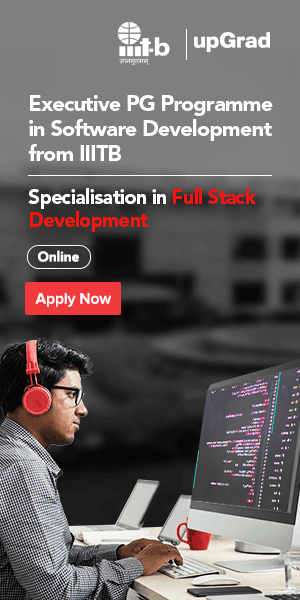
Our Trending Software Engineering Courses
- Master of Science in Computer Science from LJMU
- Executive PG Program in Software Development Specialisation in Full Stack Development from IIIT-B
- Advanced Certificate Programme in Cyber Security from IIITB
- Full Stack Software Development Bootcamp
- Software Engineering Bootcamp from upGrad
Popular Software Development Skills
- React Courses
- Javascript Courses
- Core Java Courses
- Data Structures Courses
- ReactJS Courses
- NodeJS Courses
- Blockchain Courses
- SQL Courses
- Full Stack Development Courses
- Big Data Courses
- Devops Courses
- NFT Courses
- Cyber Security Courses
- Cloud Computing Courses
- Database Design Courses
- Crypto Courses
- Python Courses
Frequently Asked Questions (FAQs)
Artificial intelligence (AI) has proved to be an effective tool in cyber defence. AI is anticipated to gain even more prominence in 2024, mainly in monitoring, resource and threat analysis, and quick response capabilities.
One area of focus is the development of secure quantum and space communications to address the increasing use of quantum technologies and space travel. Another area of research is improving data privacy.
The approach to cybersecurity is expected to change from defending against attacks to acknowledging and managing ongoing cyber risks. The focus will be on improving resilience and recovering from potential cyber incidents.
Related Programs View All
Certification
40 Hrs Live, Expert-Led Sessions
2 High-Quality Practice Exams
View Program
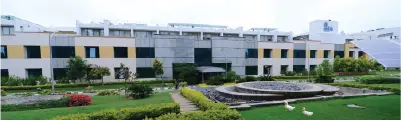
Executive PG Program
IIIT-B Alumni Status
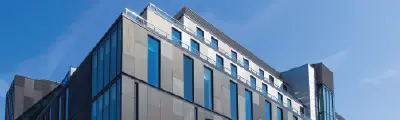
Master's Degree
40000+ Enrolled Learners
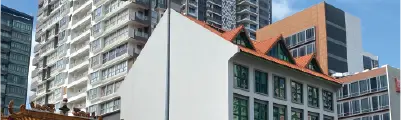
Job Assistance
32-Hr Training by Dustin Brimberry
Question Bank with 300+ Practice Qs
45 Hrs Live Expert-Led Training
Microsoft-Approved Curriculum
159+ Hours of Live Sessions
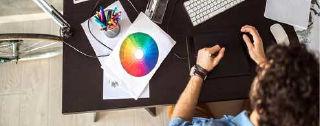
126+ Hours of Live Sessions
Fully Online
13+ Hrs Instructor-Led Sessions
Live Doubt-Solving Sessions
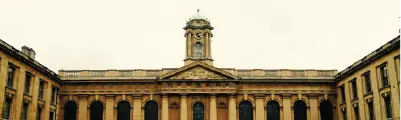
2 Unique Specialisations
300+ Hiring Partners
20+ Hrs Instructor-Led Sessions
16 Hrs Live Expert-Led Training
CLF-C02 Exam Prep Support
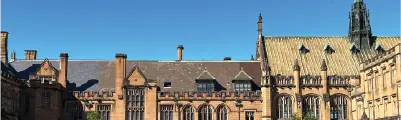
24 Hrs Live Expert-Led Training
4 Real-World Capstone Projects
17+ Hrs Instructor-Led Training
3 Real-World Capstone Projects
289 Hours of Self-Paced Learning
10+ Capstone Projects
490+ Hours Self-Paced Learning
4 Real-World Projects
690+ Hours Self-Paced Learning
Cloud Labs-Enabled Learning
288 Hours Self-Paced Learning
9 Capstone Projects
40 Hrs Live Expert-Led Sessions
2 Mock Exams, 9 Assessments
Executive PG Certification
GenAI integrated curriculum
Job Prep Support
Instructor-Led Sessions
Hands-on UI/UX
16 Hrs Live Expert-Led Sessions
12 Hrs Hand-On Practice
30+ Hrs Live Expert-Led Sessions
24+ Hrs Hands-On with Open Stack
2 Days Live, Expert-Led Sessions
34+ Hrs Instructor-Led Sessions
10 Real-World Live Projects
24 Hrs Live Expert-Led Sessions
16 Hrs Hand-On Practice
8 Hrs Instructor-Led Training
Case-Study Based Discussions
40 Hrs Instructor-Led Sessions
Hands-On Practice, Exam Support
24-Hrs Live Expert-Led Sessions
Regular Doubt-Clearing Sessions
Extensive Exam Prep Support
6 Hrs Live Expert-Led Sessions
440+ Hours Self-Paced Learning
400 Hours of Cloud Labs
15-Hrs Live Expert-Led Sessions
32 Hrs Live Expert-Led Sessions
28 Hrs Hand-On Practice
Mentorship by Industry Experts
24 Hrs Live Trainer-Led Sessions
Mentorship by Certified Trainers
GenAI Integrated Curriculum
Full Access to Digital Resources
16 Hrs Live Instructor-Led Sessions
80+ Hrs Hands-On with Cloud Labs
160+ Hours Live Instructor-Led Sessions
Hackathons and Mock Interviews
31+ Hrs Instructor-Led Sessions
120+ Hrs of Cloud Labs Access
35+ Hrs Instructor-Led Sessions
6 Real-World Live Projects
24+ Hrs Instructor-Led Training
Self-Paced Course by Nikolai Schuler
Access Digital Resources Library
300+ Hrs Live Expert-Led Training
90 Hrs Doubt Clearing Sessions
56 Hours Instructor-Led Sessions
78 Hrs Live Expert-Led Sessions
22 Hrs Live, Expert-Led Sessions
CISA Job Practice Exams
Explore Free Courses
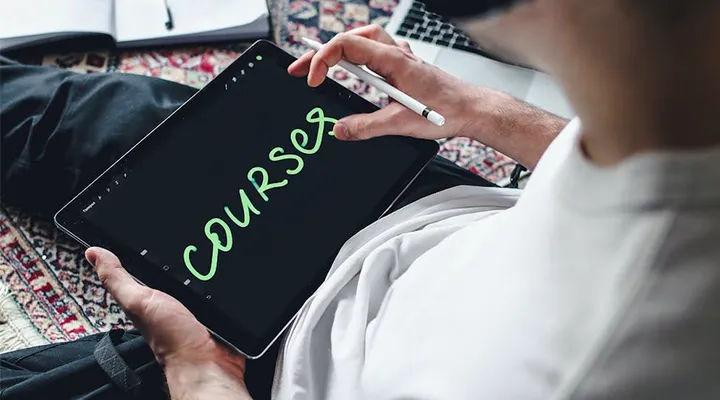
Learn more about the education system, top universities, entrance tests, course information, and employment opportunities in Canada through this course.
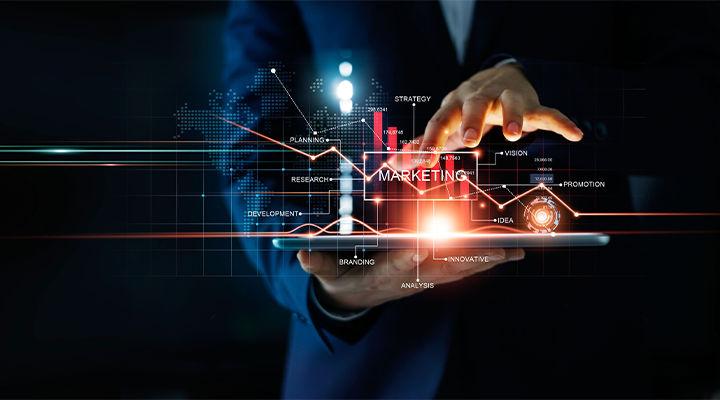
Advance your career in the field of marketing with Industry relevant free courses
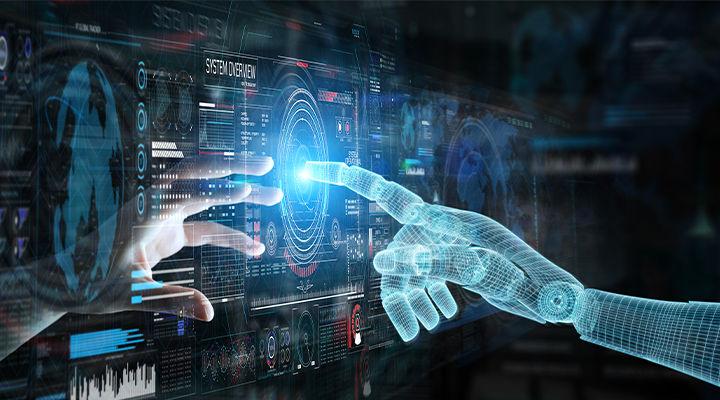
Build your foundation in one of the hottest industry of the 21st century
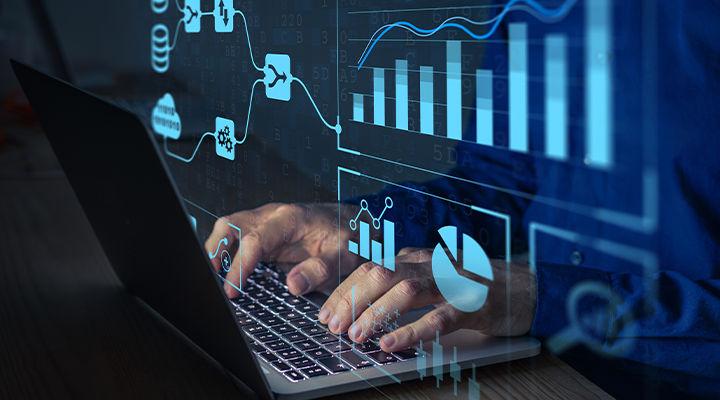
Master industry-relevant skills that are required to become a leader and drive organizational success
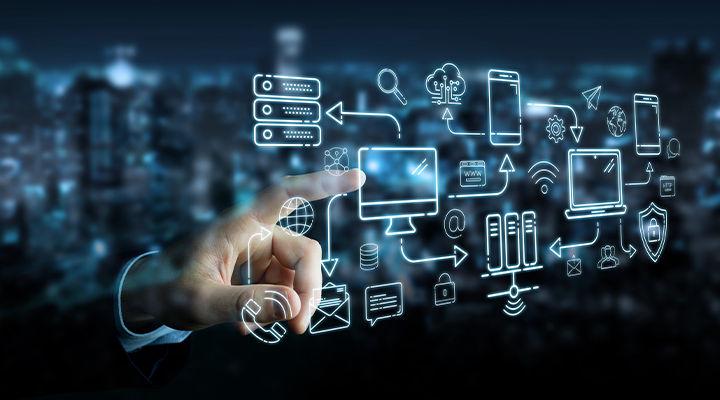
Build essential technical skills to move forward in your career in these evolving times
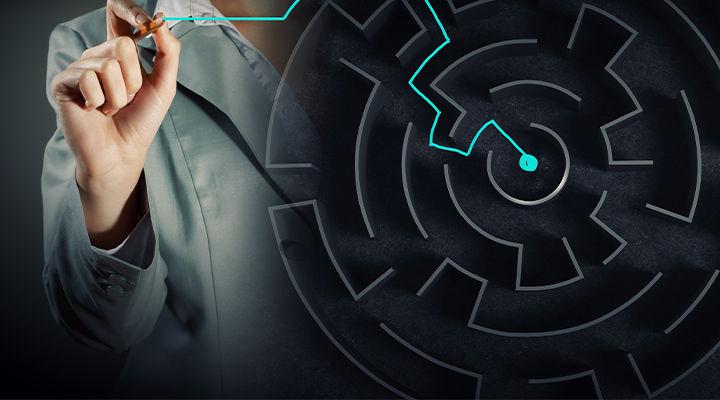
Get insights from industry leaders and career counselors and learn how to stay ahead in your career
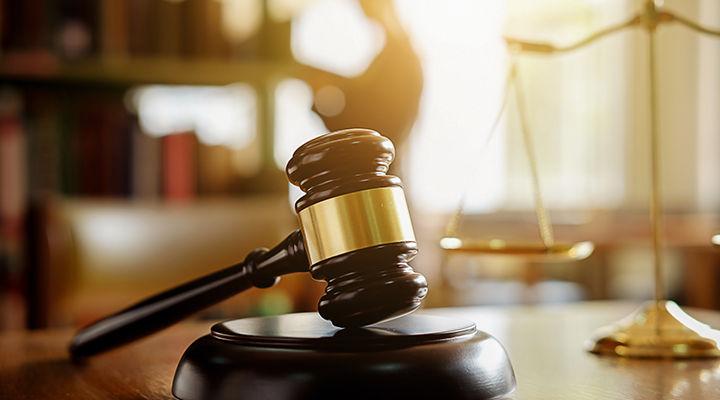
Kickstart your career in law by building a solid foundation with these relevant free courses.

Stay ahead of the curve and upskill yourself on Generative AI and ChatGPT
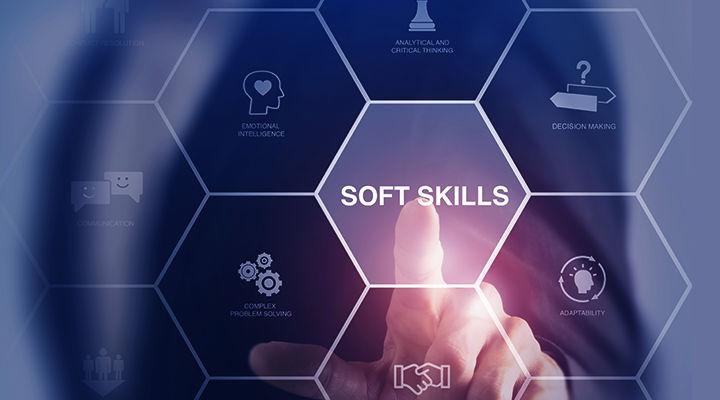
Build your confidence by learning essential soft skills to help you become an Industry ready professional.
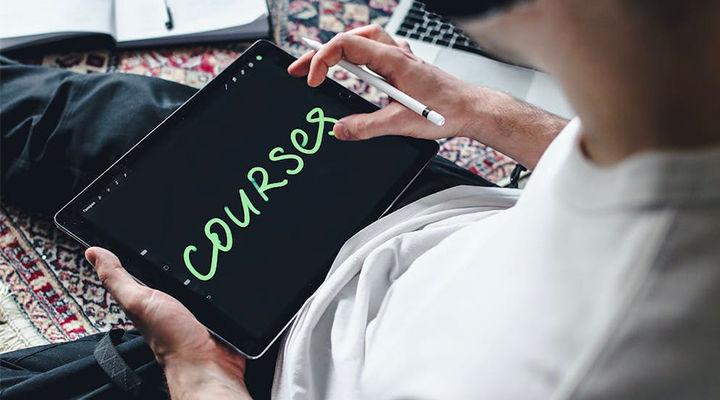
Learn more about the education system, top universities, entrance tests, course information, and employment opportunities in USA through this course.
Suggested Tutorials
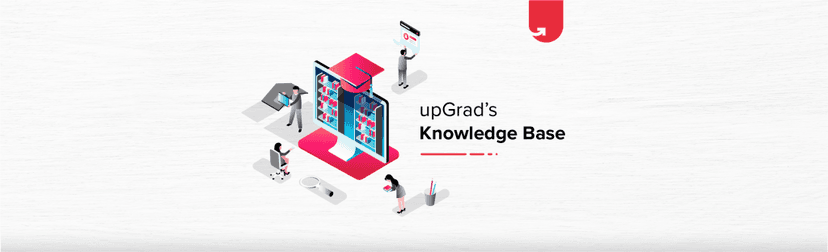
Explore Python programming with this concise tutorial, covering basics to advanced concepts for beginners and enthusiasts alike.
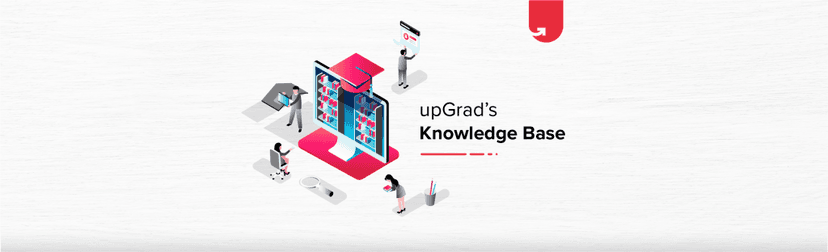
Introduction to C Programming, Learn all the C programming language concepts in this tutorial.
Suggested Blogs
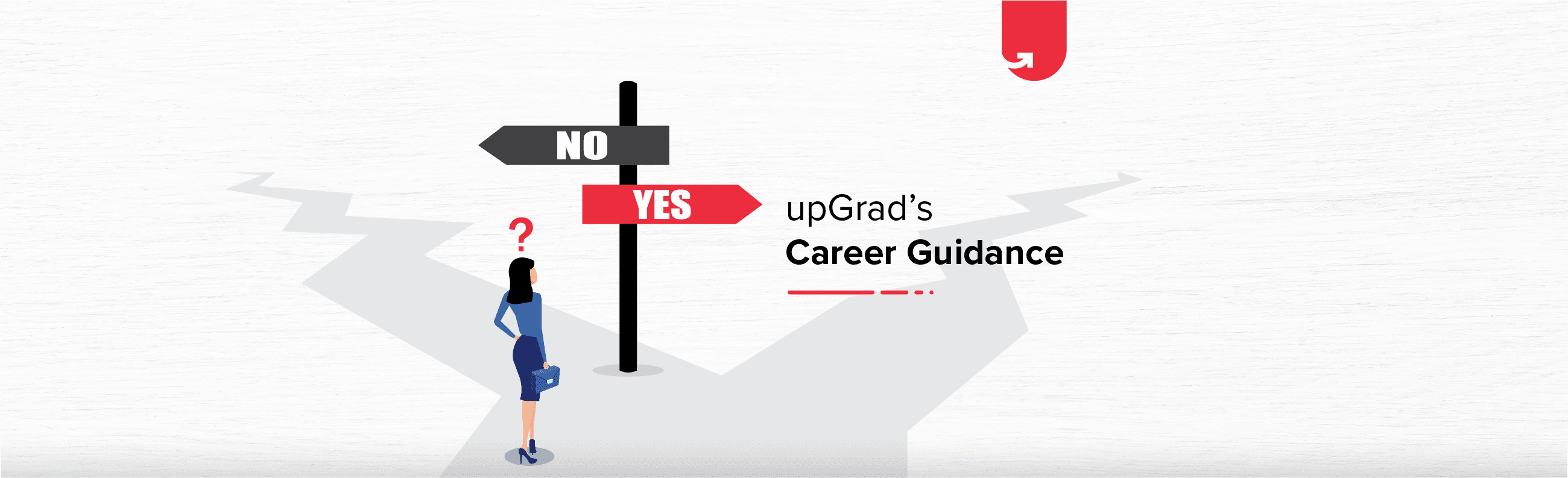
12 Apr 2024
![cyber security research tasks Scrum Master Salary in India: For Freshers & Experienced [2023]](https://www.upgrad.com/__khugblog-next/image/?url=https%3A%2F%2Fd14b9ctw0m6fid.cloudfront.net%2Fugblog%2Fwp-content%2Fuploads%2F2020%2F05%2F486.png&w=3840&q=75)
by Rohan Vats
05 Mar 2024
![cyber security research tasks SDE Developer Salary in India: For Freshers & Experienced [2024]](https://www.upgrad.com/__khugblog-next/image/?url=https%3A%2F%2Fd14b9ctw0m6fid.cloudfront.net%2Fugblog%2Fwp-content%2Fuploads%2F2020%2F05%2F482-1.png&w=3840&q=75)
by Prateek Singh
29 Feb 2024
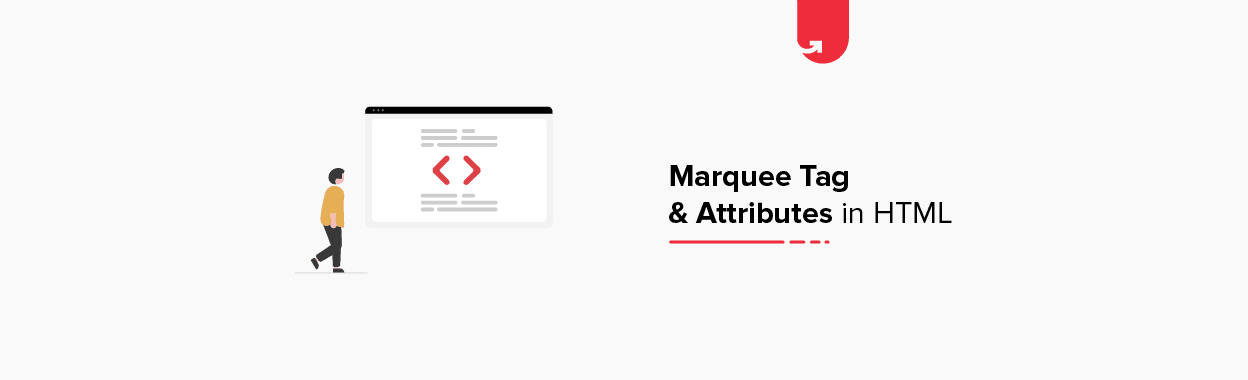
by venkatesh Rajanala
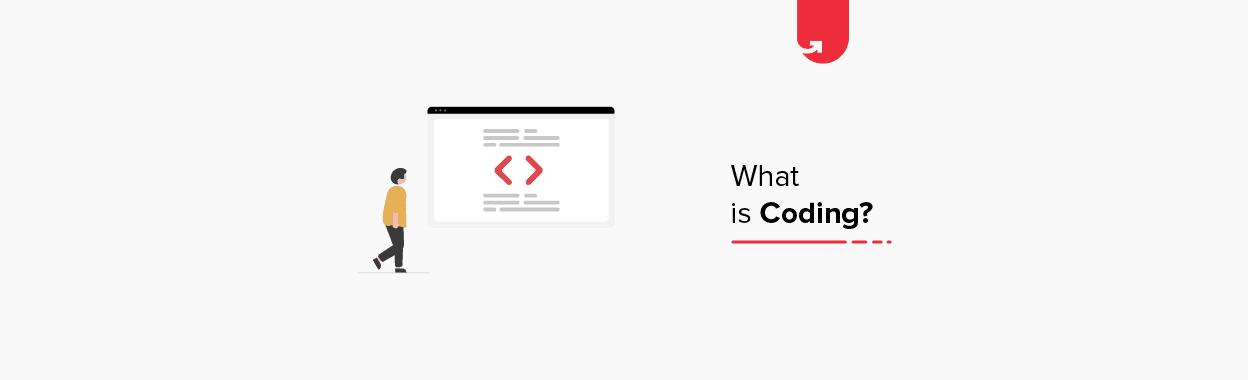
by Harish K
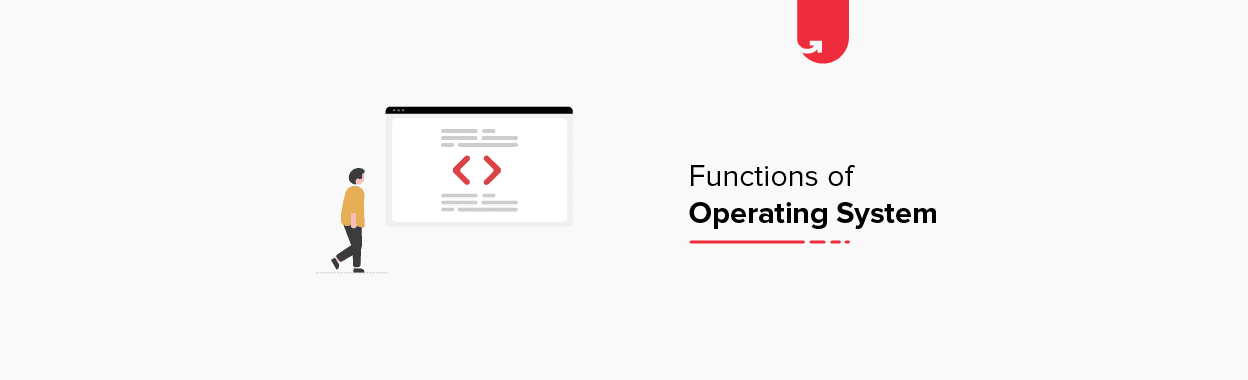
by Geetika Mathur
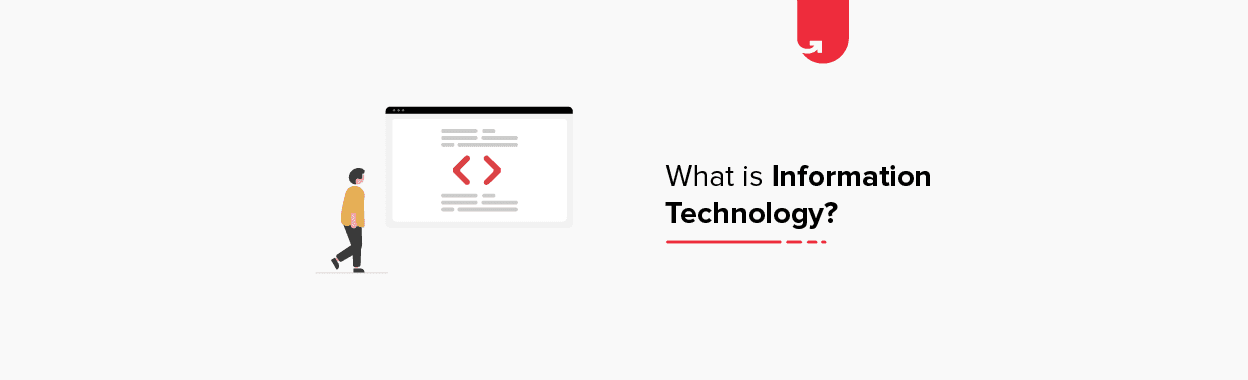
by spandita hati
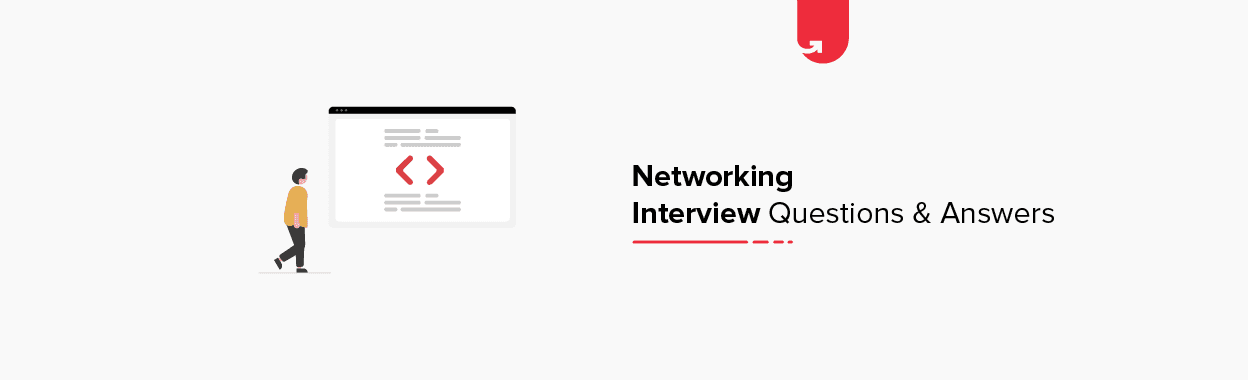
- Reference Manager
- Simple TEXT file
People also looked at
Review article, a review of cyber vigilance tasks for network defense.
- 1 Edith Cowan University, Joondalup, WA, Australia
- 2 Cyber Security Cooperative Research Centre, Perth, WA, Australia
- 3 Experimental Psychology Unit, Perth, WA, Australia
- 4 Western Australian Department of the Premier and Cabinet, Perth, WA, Australia
The capacity to sustain attention to virtual threat landscapes has led cyber security to emerge as a new and novel domain for vigilance research. However, unlike classic domains, such as driving and air traffic control and baggage security, very few vigilance tasks exist for the cyber security domain. Four essential challenges that must be overcome in the development of a modern, validated cyber vigilance task are extracted from this review of existent platforms that can be found in the literature. Firstly, it can be difficult for researchers to access confidential cyber security systems and personnel. Secondly, network defense is vastly more complex and difficult to emulate than classic vigilance domains such as driving. Thirdly, there exists no single, common software console in cyber security that a cyber vigilance task could be based on. Finally, the rapid pace of technological evolution in network defense correspondingly means that cyber vigilance tasks can become obsolete just as quickly. Understanding these challenges is imperative in advancing human factors research in cyber security.
CCS categories: Human-centered computing~Human computer interaction (HCI)~HCI design and evaluation methods.
Introduction
The weakest link in modern network defense are the natural limitations of the human operators who work in security operations centers ( Thomason, 2013 ; Cavelty, 2014 ). These limitations are neuropsychological in their origin, and mostly impact the human attentional system, which interacts with cognitive design elements of cyber security software. These elements of design include signal salience, event rate, cognitive load, and workload transitions ( Parasuraman, 1979 , 1985 ). The executive resources required to sustain vigilant attention to network defense systems are an order of magnitude greater than in classic vigilance domains, such as air traffic control, nuclear plant monitoring and baggage security ( Wickens et al., 1997 ; Hancock and Hart, 2002 ; Chappelle et al., 2013 ; Gartenberg et al., 2015 ; Reinerman-Jones et al., 2016 ). The volume, diversity, specificity, and evolution rate of threats in the cyber landscape make network defense an extremely cognitively demanding task ( D'Amico et al., 2005 ).
Classic vigilance research first involved creating a laboratory simulation of the operational sustained attention problem ( Cunningham and Freeman, 1994 ; Smith, 2016 ; Joly et al., 2017 ; Valdez, 2019 ). For example, Mackworth's (1948 , 1950) clock test was used to simulate the task demands associated with World War 2 radar operation. Because vigilance performance is task specific, the study of vigilance decrement in network defense analysts necessitates a test bed specifically designed to emulate the cognitive demands associated with real world cyber security ( Satterfield et al., 2019 ). In this regard however, a gap has been identified in the tools available to investigate cyber vigilance decrement. Specifically, a validated cyber vigilance task that probes each of Parasuraman's (1979 , 1985) parameters does not currently exist. This gap in the literature could hinder the application of wider human factors research, such as methods of tracking or intervening in vigilance decrement, from the lab into applied domains such as cyber security ( Al-Shargie et al., 2019 ; Yahya et al., 2020 ). For example, Parasuraman's (1979 , 1985) parameters of a valid vigilance tasks were derived long before modern network defense, it hence remains a similarly unexplored question if these parameters alone constitute a vigilance task valid in cyber security. Similarly, Bodala et al. (2016) demonstrated that integrating challenging features into vigilance task stimuli was a useful method of enhancing sustained attention. However, the task Bodala utilized was not designed to emulate the cognitive demands associated with modern cyber defense. Hence, it remains a standing question if the vigilance performance enhanced by greater challenge integration on Bodala's task would extend to cyber security. However, this question cannot be probed without a modern, validated cyber vigilance task in which the challenging parameters of stimuli can be controlled. The main goal of this review is therefore to understand several factors that may explain this gap in the literature, including access and confidentiality, task complexity, non-standard operating environments, and rapid obsolescence.
Situational awareness refers to the perception, comprehension, and projection of the threats within an environment across time and space ( Endsley and Kiris, 1995 ; Wickens, 2008 ). The term cyber-cognitive situational awareness specifically refers to human operators' awareness of threats distributed across virtual landscapes ( Gutzwiller et al., 2015 ). For the purposes of brevity, the term “cyber-cognitive situational awareness” is referred to here as “situational awareness.”
Network defense analysts must pay close consistent attention to Security Event Information Management Systems (SEIMs), which are used to establish and support situational awareness of cyber threat landscapes ( Komlodi et al., 2004 ; Spathoulas and Katsikas, 2010 , 2013 ; Tyworth et al., 2012 ; Albayati and Issac, 2015 ; Newcomb and Hammell, 2016 ). SEIMs summarize anomalous and potentially malicious patterns of network traffic as sets of alarms, or alerts, which analysts must individually investigate as potential cyber threats ( Barford et al., 2010 ; Spathoulas and Katsikas, 2010 , 2013 ; Gaw, 2014 ; Newcomb and Hammell, 2016 ). Analysts' capacity to sustain attention to their SEIM therefore constrains their situational awareness of the cyber threat landscape being protected ( Endsley and Kiris, 1995 ; Gutzwiller et al., 2015 ; Wickens et al., 2015 ).
Situational awareness hinges on the capacity to sustain attention to threats distributed across cyber threat landscapes ( Endsley and Kiris, 1995 ; Barford et al., 2010 ). In the context of network security, analysts use SEIMs to perceive and act on threats to protected cyber infrastructures ( Gutzwiller et al., 2015 ). SEIM threat detection is a tedious, monotonous task that requires analysts to sustain high levels of attention for prolonged periods of time ( Fathi et al., 2017 ; Nanay, 2018 ).
Distinguishing between malicious and benign SEIM alerts is not dissimilar to the search for a needle in a haystack ( Erola et al., 2017 ). Analysts sift through vast numbers of SEIM alerts, most of which are false positives, just to identify and act on a small number of malicious threats ( Sawyer et al., 2016 ). Although SEIM threat detection is initially easy to perform, analyst mistakes invariably accumulate with time spent distinguishing between malicious and benign element signals ( Sawyer et al., 2016 ). This gradual decline in sustained attention is known as vigilance decrement ; it occurs when the brain is required to sustain a high level of workload processing activity for longer than its energy reserves can support ( Sawyer et al., 2016 ). Establishing and sustaining situational awareness in a cyber security operations center, requires that analysts sustain vigilant attention to their SEIM dashboards for prolonged periods of time ( Wall and Williams, 2013 ). However, vigilance decrement has become an increasingly disruptive influence in operational network defense analysts whose role requires the use of SEIM to hunt for threats in the cyber landscape ( Chappelle et al., 2013 ; Wall and Williams, 2013 ).
Vigilance refers to the capacity an individual has to sustain conscious processing of repetitive, unpredictable stimuli without habituation or distraction ( Pradhapan et al., 2017 ). Vigilance is regarded as a state of alertness to rare and unpredictably frequent stimuli ( Pradhapan et al., 2017 ). When attention is sustained for a prolonged period, human processing limitations lead to compounding performance failures, the phenomenon known as vigilance decrement ( Sawyer and Hancock, 2018 ; Warm et al., 2018 ). For example, drivers must sustain vigilance in attuning and responding to hazards on the road ( Zheng et al., 2019 ). A driver experiencing vigilance decrement, however, will be less capable of responding to road hazards ( Gopalakrishnan, 2012 ). Hence, failure to sustain attention to road hazards is the leading cause of thousands of road deaths each year ( Gopalakrishnan, 2012 ). Depending upon the context, vigilance decrement can manifest either as an increased reaction time to detect critical signals or as a reduction in their correct detection ( Warm et al., 2018 ). For example, during World War Two, British radar operators were required to monitor their terminals over prolonged periods of time for “blips” that indicated the presence of Axis U-boats. Despite their training and motivation to avoid Axis invasion, these operators began to miss critical U-boat signals after only half an hour of monitoring ( Mackworth, 1948 , 1950 ). Mackworth (1948 , 1950) was commissioned by the Royal Air Force to study the problem, in what would become seminal vigilance research.
Mackworth (1948 , 1950) devised a “Clock Test” that simulated the Royal Air Force's radar displays. This comprised of a black pointer that traced along the circumference of a blank, featureless clock-type face in 0.3-inch increments per second. At random points during the task, the radar pointer would increment twice in a row as a way of simulating the detection of a U -boat. Mackworth (1948 , 1950) tasked observers with detecting these double jumps by pressing a button when one was seen. Despite the clarity of Mackworth's (1948 , 1950) target signals, correct detections declined by 10% in the first 30 min of the 2-h-long task. This gradual drop in correct signal detection was the first laboratory demonstration of vigilance decrement. The phenomenon has since been demonstrated as one of the most ubiquitous and consistently replicated findings in the vigilance literature ( Baker, 1959 ; Mackworth, 1968 ; Sostek, 1978 ; Parasuraman and Mouloua, 1987 ; Dember et al., 1992 ; Warm and Dember, 1998 ; Pattyn et al., 2008 ; Epling et al., 2016 ).
Laboratory vigilance tasks require correctly identifying rare target stimuli in an array for a prolonged period ( Daly et al., 2017 ). Vigilance decrement typically onsets within 15 min of sustained attention, however it has been reported in as little as 8 min under particularly demanding situations ( Helton et al., 1999 ; St John et al., 2006 ).
Vigilance decrement has only recently received recognition in the human-factors literature, as a cyber incident risk factor ( Chappelle et al., 2013 ; Mancuso et al., 2014 ). For example, network defense analysts who experience vigilance decrement will decline in their capacity to attune to, detect, and act against threats presented in a SEIM console ( McIntire et al., 2013 ). Vigilance decrement is therefore a human factor bottleneck to the protective benefit of SEIM software. That is, the cyber protection offered by SEIM software is bottlenecked by the capacity of its operators to sustain vigilant attention to the information it presents. Managing vigilance decrement first necessitates a nuanced understanding of the factors which contribute to declines in sustained attention to network defense consoles ( McIntire et al., 2013 ). This may explain why current attempts to manage vigilance decrement in the human factors literature have focused on developing unobtrusive psychophysiological monitoring methods for indicating when the capacity to sustain attention capacity begins to decline ( McIntire et al., 2013 ; Mancuso et al., 2014 ; Sawyer et al., 2016 ). However, the psychophysiological correlates of cyber vigilance decrement may not be adequately understood without an experimental test bed that accurately simulates the cognitive demands associated with modern network defense ( McIntire et al., 2013 ; Mancuso et al., 2015 ; Sawyer et al., 2016 ).
The review that follows identifies limitations in experimental platforms that could be used to conduct human-in-the-loop studies of cyber vigilance decrement, and challenges that need to be overcome to fill this gap. The only cyber vigilance tasks documented in the literature to date are owned by The United States Air Force and are outdated simulations of the demands associated with modern network defense ( McIntire et al., 2013 ; Mancuso et al., 2015 ; Sawyer et al., 2016 ). Beyond researchers, an accessible experimental test bed for human-in-the-loop studies of cyber vigilance decrement could also provide utility to business, government, and militaries, by informing training, selection, and software development standards ( Alhawari et al., 2012 ; Ormrod, 2014 ).
Review significance
As reliance on global cyber networks continues to grow, the extent of the impact of their compromise will also increase ( Ben-Asher and Gonzalez, 2015 ; Goutam, 2015 ). Ensuring the security of these systems hinges on the optimized performance of human network defenders ( Thomason, 2013 ; Cavelty, 2014 ). Lapses in network defender attention therefore have the potential to cripple the cyber infrastructure being guarded ( Thomason, 2013 ; Cavelty, 2014 ). This includes virtual and physical military assets, governmental assets, central banking networks, stock market infrastructure as well as national power and telecommunications grids ( Gordon et al., 2011 ; Jolley, 2012 ; Saltzman, 2013 ; Ormrod, 2014 ; Hicks, 2015 ; Skopik et al., 2016 ; Rajan et al., 2017 ). The integrity of these assets hinges on measuring and mitigating neurocognitive inefficiencies in network defenders' capacity to sustain vigilant attention to cyber security command and control consoles ( Maybury, 2012 ). Managing the risk associated with cyber vigilance decrement will enhance the defense of critical global cyber infrastructures ( Maybury, 2012 ; Wall and Williams, 2013 ). However, cyber vigilance tasks that allow researchers to study the decrement in network defense are not currently accessible to researchers ( Maybury, 2012 ; McIntire et al., 2013 ; Mancuso et al., 2015 ; Sawyer et al., 2016 ).
Cyber vigilance decrement
In under 20 min, a fully trained, motivated, and experienced network defense analyst's capacity to identify threats in their SEIM can begin to decline ( McIntire et al., 2013 ). From a technological perspective, this phenomenon, known as vigilance decrement, has arisen in the cyber domain due to the gradual rise in the volume, diversity and specificity of data that network analysts must process to identify and act upon threats ( D'Amico et al., 2005 ).
Cyber vigilance decrement has emerged as a defining human factor of network security ( Tian et al., 2004 ; Maybury, 2012 ; Aleem and Ryan Sprott, 2013 ; Wall and Williams, 2013 ; Franke and Brynielsson, 2014 ; Gutzwiller et al., 2015 ; Vieane et al., 2016 ). For example, prevalence denial attacks involve flooding the SEIM of a target network with huge volumes of innocuous, non-malicious signals designed to intentionally induce vigilance decrement in defense analysts ( Vieane et al., 2016 ). Once in this less attentive state, bad actors can improve their chance of implementing a successful attack on the target net work (Vieane et al., 2016) . Vigilance decrement is therefore a cyber-cognitive security vulnerability which must be studied and managed like any other vulnerability in network defense ( Tian et al., 2004 ; Aleem and Ryan Sprott, 2013 ; Wall and Williams, 2013 ; Vieane et al., 2016 ).
Existing cyber vigilance tasks
Whilst Google Scholar is not a database, it was chosen as the driving methodology for this review for its capacity to broadly scan wide breadths of academic literature ( Tong and Thomson, 2015 ). Studies were only included in this review if they presented a sustained attention task specifically designed to emulate the cognitive demands associated with operating a cyber security console, like the SEIM software that network defense analysts use to sustain situational awareness of virtual threat landscapes. This process yielded only three examples in the literature of an experimental test bed that researchers could use to study vigilance decrement in network defense ( McIntire et al., 2013 ; Mancuso et al., 2015 ; Sawyer et al., 2016 ).
The Cyber Defense Task (CDT) that McIntire et al. (2013) presented was the formative example of a cyber vigilance task in the literature. Mancuso et al. (2015) and Sawyer et al. (2016) followed soon after with their presentation of the Mancuso Cyber Defense Task (MCDT and MCDT-II). The discussion that follows presents a critical review of the CDT and MCDT. For example, the validity of these tasks as simulations of the demands associated with network defense may have declined between now and when they were published due to evolving complexity in network defense ( Gutzwiller et al., 2015 ). Rapid obsolescence of cyber vigilance tasks may also reflect the need to consider cyber-cognitive parameters of SEIM consoles which, according to Parasuraman (1979 , 1985) , influence the probability of vigilance decrement. Hence any research based on existent platforms may not generalize well beyond the lab, let alone beyond the context of military cyber defense for which they were designed.
McIntire's Cyber Defense Task (CDT)
McIntire et al.'s (2013) formative CDT aimed to psychophysiologically identify the onset of vigilance decrement in a laboratory cyber-defense task. Although successful in monitoring vigilance performance, several methodological issues make it difficult to generalize McIntire et al.'s (2013) results to operational cyber defense. For instance, McIntire et al.'s (2013) sample comprised 20 military and civilian cyber defenders who participated in four, 40-min trials of the CDT. It is possible that the civilian participants McIntire et al. (2013) sampled did not have the same motivations or stressors as the active duty subset of their sample ( Finomore et al., 2009 ). This compromise was however understandable, as cyber defense analysts are a difficult population to sample from, and the task did not require prior cyber defense training ( Zhong et al., 2003 , 2015 ; Rajivan et al., 2013 ).
The CDT was designed to simulate the cognitive demands associated with modern network defense. It is not possible to completely appraise the CDT as a cyber vigilance task, as only a brief account of the software was documented in the literature ( McIntire et al., 2013 ; Sherwood et al., 2016 ). In addition, McIntire et al. (2013) and Sherwood et al. (2016) are the only studies that have made use of the CDT, and both were sponsored by the United States Air Force Research Laboratory (AFRL). Though it cannot be confirmed, it is possible that the CDT has been retained for the AFRL's exclusive research use, which limits the degree of scientific enquiry that can be made into cyber vigilance decrement on this task.
As described in McIntire et al. (2013) , the CDT involved two subtasks that participants concurrently completed during the cyber vigilance task. The CDT's textual component required the participant to monitor and report the presence of three suspicious IP addresses and port combinations (Figure 2 in McIntire et al., 2013 ). Participants had to memorize these IP addresses beforehand and press a button to indicate when one was observed. The second component of McIntire et al.'s (2013) CDT was graphical and presented concurrently with the first textual component. Participants were presented with a live graph of simulated network traffic, which they monitored in case a threshold value, indicated by a red horizontal line, was exceeded (Figure 2 in McIntire et al., 2013 ). Participants indicated when traffic exceeded this limit by pressing a button.
McIntire et al. (2013) observed vigilance decrement in CDT performance, which also correlated with a series of ocular parameters that they recorded using an eye tracker. Participants' blink frequency and duration, eye closure percentage, pupil diameter, eccentricity, and velocity were all recorded as they performed the CDT. These measurements all correlated with changes in CDT performance over time, a result which accorded with an abundance of studies on vigilance while driving ( Thiffault and Bergeron, 2003a , b ; Tan and Zhang, 2006 ; D'Orazio et al., 2007 ; Sommer and Golz, 2010 ; Jo et al., 2014 ; Aidman et al., 2015 ; Cabrall et al., 2016 ; Zheng et al., 2019 ).
Validity concerns with the CDT
It was unclear if the ocular changes that McIntire et al. (2013) correlated with time spent on the CDT would extend beyond this laboratory analog, which is not as cognitively demanding as network defense in the real-world ( Donald, 2008 ; Reinerman-Jones et al., 2010 ; Chappelle et al., 2013 ; Hancock, 2013 ). The complexity of network defense could explain why existing cyber vigilance tasks are considered oversimplified ( Rajivan et al., 2013 ; DoD, 2014 ; Gutzwiller et al., 2016 ; Rajivan and Cooke, 2017 ). For instance, eleven key service skills are required by the United States Department of Defense network defense analysts ( DoD, 2014 ). These cores skills include cryptology, oversight and compliance, reporting, cyber security, computer science, network exploitation, and technology operations ( DoD, 2014 ). A case could be made that the CDT did require the use of reporting oversight and compliance, however eight of the 11 core skills were not built into McIntire et al.'s (2013) task. In contrast, Mackworth's (1948 , 1950) clock test accurately simulated every feature of the radar operator's task except for the presence of actual U -boats. Therefore, even by the DoD's (2014) own standard, it would be generous to suggest the CDT is a passable simplification of real-life Cyber Defense Task demands.
The brevity of McIntire et al. (2013) 40-min-long trials also make the CDT's external validity unclear. In terms of laboratory vigilance investigations, 40 min is a typical period for performing a vigilance task ( See et al., 1995 ; Helton et al., 1999 ; Warm et al., 2008 , 2009 ; See, 2014 ). However, Chappelle et al. (2013) reported that active-duty cyber-defenders work for 51 h per week, or 10.5 h per day, with extremely limited rest breaks. Thus, the demands associated with a 40-min vigilance task are not analogous to a 10.5 h work day that Chappelle et al. (2013) observed to induce clinically significant levels of stress and burnout ( O'Connell, 2012 ; Mancuso et al., 2015 ). By comparison to the rest of their day, the 40-min CDT could possibly have been a welcome respite for McIntire et al.'s (2013) the active service participants. It is hence unclear how externally valid the ocular changes that McIntire et al. (2013) associated with vigilance performance are, and how well these might extend across the standard 8–10-h shifts served by real-world cyber defenders.
The external validity of McIntire et al.'s (2013) study further suffered from insufficient control of confounding blue light exposure. A considerable proportion of the light emitted by many modern computer monitors is in the form of high-frequency blue light, and it is possible that the United States Air Force outfits their cyber defenders with these common tools ( Lockley et al., 2006 ; Hatori et al., 2017 ). Blue light suppresses melatonin and actively increases the capacity to sustain attention on vigilance tasks in a dose-dependent fashion ( Lockley et al., 2006 ; Holzman, 2010 ). Since this effect is dose-dependent, the longer cyber defenders are exposed to the blue light of their computer monitors, the greater vigilance performance could be expected to improve ( Lockley et al., 2006 ). In a real-world cyber defense setting, analysts are exposed to 1,200 times the blue light exposure than the participants in McIntire et al. (2013) . The vigilance performance enhancement provided by so much more blue light exposure may have rendered measuring the phenomenon far more than McIntire et al. (2013) suggested. Thus, the results reported by McIntire et al. (2013) may not generalize beyond the laboratory to the real-world ( Reinerman-Jones et al., 2010 ; Hancock, 2013 ).
These largely technological critiques of the CDT's validity were overshadowed by the fact that McIntire et al.'s task was not validated according to Parasuraman's (1979 , 1985) parameters of valid vigilance tasks. The first component of the CDT required that participants retain and recall three “suspicious” IP addresses from memory as they attempt each critical signal discrimination. This set of textual critical signals increased their participants' cognitive load while performing the CDT. However, because each critical CDT signal was considered in isolation, there was a gradual decline in cognitive load as time on the task increases. This is not the case in real world network defense. Operational analysts consider the alerts presented over their SEIM relative to one another within the wider virtual threat landscape ( Heeger, 1997 , 2007 ; Alserhani et al., 2010 ; Bridges, 2011 ; Majeed et al., 2019 ). For example, if a SEIM becomes flooded with benign alerts in a brief window of time, this can represent the beginning of a prevalence denial attack, as such, analysts must consider each benign alert in the context of all others presented by their system ( Sawyer et al., 2016 ; Vieane et al., 2016 ). Cognitive load hence does not decline with time on task in operational network defense, whereas it does so in McIntire et al.'s (2013) CDT. It cannot therefore be claimed that vigilance decrement underlies the performance deficits observed by McIntire et al. (2013) on the CDT with any validity.
The frequency that alerts are presented to analysts by a SEIM is known as the event, or incident, rate ( Simmons et al., 2013 ). The SEIM event rate communicates important information surrounding threatening elements distributed through the virtual threat landscape to analysts. For example, consider the rate that SEIM alerts occur at 2 am on Christmas Day against that observed at 11 am on a regular weekday. SEIM alerts are generally more frequent during the working week than during the holiday season ( Pompon et al., 2018 ; Rodriguez and Okamura, 2019 ). Therefore, if the event rate at 2 am on Christmas Day even closely approximates that which is usually seen at 11 am on a weekday, this will influence how an analyst contextualizes and subsequently actions each SEIM alert. Even if every Christmas day SEIM alert is benign, the atypical event rate would influence the level of imminent risk perceived by an analyst in the virtual threat landscape ( Vieane et al., 2016 ).
Event rate in real world network defense hence guides the way network defense analysts contextualize and then action SEIM alerts. This element of network defense was not captured by the CDT because McIntire et al. (2013) set the event rate to be a controlled variable. In an operational setting, analysts would also consider how quickly each “suspicious” IP address was presented in forming their threat level appraisal ( Simmons et al., 2013 ). This further decreases the CDT's validity as a cyber vigilance task, as a fixed event rate may have impacted analysts' cognitive engagement with each potentially critical signal. That is, McIntire et al.'s (2013) participants needed to recruit fewer executive resources at a slower rate than their operational peers. It is therefore unclear if the performance deficits observed by McIntire et al. (2013) on the CDT resembled those observed during operational network defense.
Two types of critical signal were presented in the CDT, each via a different modality. The first type of critical signal was textual, in the form of three “suspicious” IP addresses that participants had to remember ( McIntire et al., 2013 ). The second type of critical signal presented in the CDT was graphical and required no memory activation ( McIntire et al., 2013 ). Although McIntire et al. (2013) had the requisite data to compare vigilance performance between the two critical signal modalities they did not report this comparison. Had vigilance performance varied between the graphical and textual critical signals, an argument could be made that this would demonstrate CDT performance sensitivity to signal salience. However, this would have been a tenuous argument at best, as the two signals were presented in vastly different ways. The CDT's textual critical signals were presented in a simultaneous fashion, which used participants' memory resources every time a discrimination was made. Simultaneous vigilance tasks require minimal executive resource activation because critical signal discriminations are based on sequential comparative judgements ( Gartenberg et al., 2015 , 2018 ). By comparison, the CDT's graphical critical signals were presented successively. Successive vigilance tasks are associated with a degree of cognitive workload above that of simultaneous tasks because operators must retain and recall critical signal information from memory before a discrimination can be made ( Gartenberg et al., 2015 , 2018 ). The primary deficiency of the CDT was fundamentally due to not being validated according to Parasuraman's (1979 , 1985) vigilance task validity parameters. Similar deficiencies have also been found in Mancuso et al.'s (2015) Cyber Defense Task.
Mancuso et al.'s Cyber Defense Task (MCDT)
The MCDT presented network traffic logs in a waterfall display which their participants needed to read and action. Traffic logs contained four pieces of information, including two possible methods used to transmit data across the network, as well as the size, source, and destination of the transmission. A “signature” referred to a specific configuration of these four traffic log details that suggests malicious network activity. Mancuso et al.'s (2015) participants first needed to commit the details of a signature associated with a fictitious hacker to memory. They then had to identify any traffic log presented to them that matched at least three out of four items of the hacker's signature. The number of items within each log that matched the hacker's signature defined the color by which it was presented in the MCDT (Figure 1 in Mancuso et al., 2015 ). Mancuso et al. (2015) justified color coding each target to better resemble the systems used by the United States Air Force (Figure 1 in Mancuso et al., 2015 ). Logs that matched 0, 1, 2, 3, or all four elements of the hacker's signature were respectively colored, green, blue, violet, purple, and red in the MCDT. Of these, only purple and red logs were critical targets that the participant had to action.
Validity concerns with the MCDT
The MCDT was designed similarly to McIntire et al.'s (2013) CDT. For instance, the task maintained a fixed critical signal probability of 20%. However, fixed task demands such as this are difficult to generalize to real world operations ( Helton et al., 2004 ). Primarily, this is because vigilance is sensitive to task demands, and in cyber defense, these fluctuate between great extremes ( Helton et al., 2004 ; Chappelle et al., 2013 ).
Another questionable feature of the MCDT's validity is that the visual field of view is confined to a single computer monitor. In real world cyber security contexts, SEIMs require multiple monitors to portray the network's security status. Multiple monitors are pragmatically necessary due to the volume, diversity, and specificity of virtual threat data that analysts are required to handle ( D'Amico et al., 2005 ). Hence, Mancuso et al.'s (2015) limited field of view restricted the range of cyber threat stimuli that could be sampled from real world operations for use in their cyber vigilance task. This detracted from the MCDT's external validity as a cyber vigilance task.
In addition, the color coding system that Mancuso et al. (2015) incorporated into the MCDT obscured the cognitive load participants experienced when discriminating between critical and non-critical traffic logs. For example, the volume and type of information required to discriminate critical MCDT traffic logs, both with and without color coding, is compared in Figure 1 in Mancuso et al. (2015) .
Under the color coded system, participants needed to remember only two graphical elements of information, namely that the color of critical logs was indicated by red or purple ( Table 1 and Figure 1 in Mancuso et al., 2015 ). This is in contrast with a colorless MCDT, where critical signals could only be identified when the participant remembered four elements of salient threat information in the hacker's signature. Because Mancuso et al.'s (2015) participants had two ways of interpreting the MCDT's signals, this made the cognitive load associated with the task unclear. There could be no way of knowing if Mancuso et al.'s (2015) participants analyzed each traffic log based on its color alone, or if they analyzed all four threat salient elements of information. Color coding the MCDT's signals therefore detracted from its external validity. That is, rather than bolstering the MCDT's external validity, Mancuso et al.'s (2015) color coding system instead served to confound the cognitive load associated with the task.
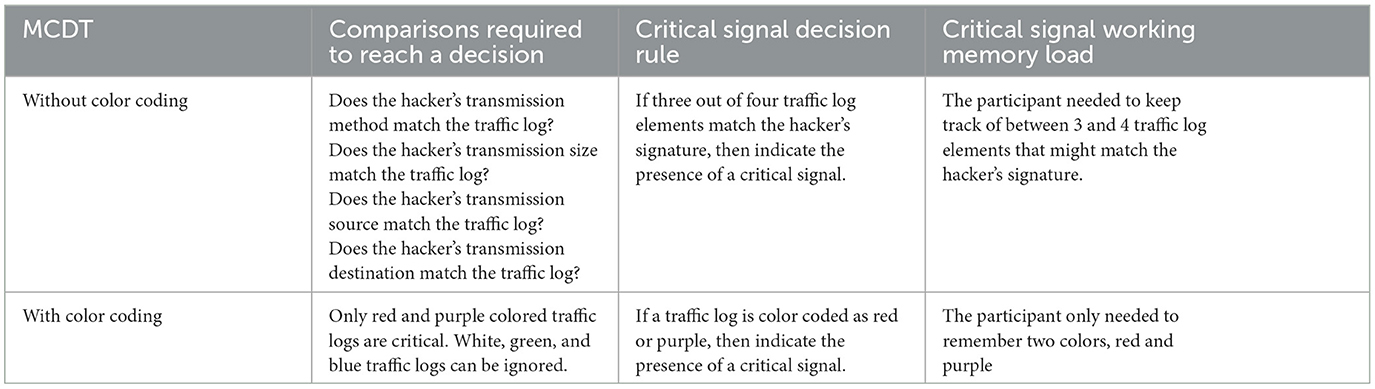
Table 1 . Comparison of the MCDT with and without color coded signals.
Sawyer et al.'s MCDT-II
Sawyer et al. (2016) used a modified form of the MCDT to investigate the impact of event rate and signal salience on cyber vigilance performance. For the purposes of discussion Sawyer et al.'s (2016) modified MCDT will be referred to as the MCDT-II. The MCDT-II presented network traffic logs to participants in a colorless waterfall display. In the original MCDT, these traffic logs detailed four threat salient pieces of information, namely, transmission method, size, source, and destination. Sawyer et al. (2016) adapted these traffic logs in the MCDT-II to include the source IP address, the source port, the destination IP address, and the destination port of each transmission (Figure 1 in Sawyer et al., 2016 ). Each network traffic log in the MCDT-II contained the IP address and communication port numbers for both the source and destination of a data transmission across a hypothetical network. Two new traffic logs appeared periodically at the top of the MCDT-II's display. The critical signal that participants needed be vigilant of was any instance in which a top row IP address and port number-pairs matched an existing traffic log already present on the display (see Figure 1 in Sawyer et al., 2016 ).
Unlike McIntire et al. (2013) and Mancuso et al. (2015) , Sawyer et al. (2016) attempted to validate their cyber vigilance task according to two of Parasuraman's (1979 , 1985) parameters, namely, event rate and signal salience. Sawyer et al. (2016) formed four experimental conditions based on two levels of event rate and signal salience, respectively ( Table 2 ). Sawyer et al. (2016) reported reductions in vigilance performance when critical MCDT-II signals were low in signal salience, slowly presented, or both. Sawyer et al. (2016) observed a gradual decline in the mean percentage of correctly identified MCDT-II signals. Moreover, in accordance with Parasuraman (1979 , 1985) , Sawyer et al. (2016) found that these reductions in performance were mediated by the signal salience and event rate of the MCDT-II.
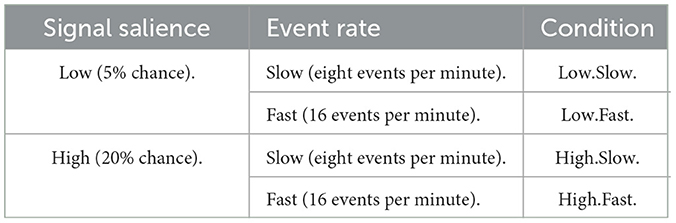
Table 2 . Levels of event rate and signal salience examined by Sawyer et al. (2016) .
With the possible exception of the High.Fast condition, Sawyer observed changes in vigilance performance that align with vigilance decrement ( Figure 1 ). Each condition Sawyer et al. (2016) tested was composed of variations in event rate and signal salience. Sawyer et al. (2016) observed that event rate had a greater influence over vigilance performance at baseline than signal salience. For example, vigilance performance under both slow conditions was higher than in the fast conditions after 10 min. However, signal salience appeared to have the greater influence by the end of the trial. For example vigilance performance in both slow and fast high signal salience condition outperformed what Sawyer et al. (2016) observed in the low signal salience condition. Sawyer et al. (2016) also reported variations in signal salience and event rate influenced trajectory of vigilance performance across all four conditions. For example, after ~30 min, Sawyer et al. (2016) reported sharp declines in the trajectory of vigilance performance observed under both low signal salience conditions ( Figure 1 ). In contrast, Sawyer et al. (2016) reported more linear declines in vigilance performance under the high signal salienc econditions. However, this linear decline varied drastically between the High.Slow and High.Fast conditions. For example, vigilance performance under the High.Fast condition only changed by 0.52% from baseline. In contrast, vigilance performance under the High.Slow condition dropped by 15.62%, which more closely approximates the average decline across all conditions, which came to ~14.85%.
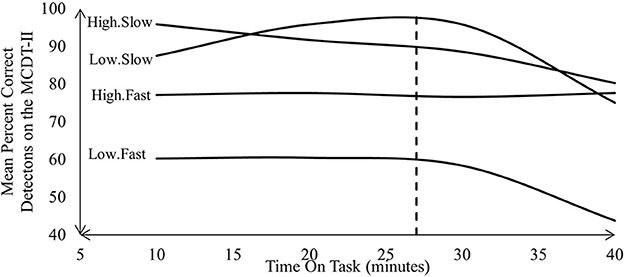
Figure 1 . MCDT-II performance Sawyer et al. (2016) reported.
Differing compositions of signal salience and event rate also resulted in clear level differences in vigilance performance. For example, vigilance performance in the Low.Fast condition was the lowest acros the entire duration of the task, and also had the lowest final final value. By the end of the task, the level of the High.Slow, Low.Slow and High.Fast vigilance performance curves all appear approximately similar at around 77.5%. The only exception to this was the value of the Low. Fast condition, which ended at almost half of all other conditions, at 43.75%. Sawyer et al. (2016) therefore demonstrated that variations in event rate and signal salience influenced the way vigilance decrement presented throughout the entire MCDT-II. Sensitivity to signal salience and event rate are just two of Parasuraman's (1979 , 1985) three parameters that characterize a valid vigilance task. Sensitivity to cognitive load was Parasuraman's (1979 , 1985) third parameter of a valid vigilance, which was a controlled variable in Sawyer et al. (2016) . The MCDT-II was therefore only partially validated as a cyber vigilance task.
Challenges of developing cyber vigilance tasks
Access and confidentiality.
Like many security sub domains, network defense analysts and their workplaces can be difficult to access for the purposes of research ( Paul, 2014 ; Gutzwiller et al., 2015 ). It can therefore be difficult to obtain details about Cyber Security Operations Centers' operational procedures or SEIM software console, as these are extremely sensitive corporate information that many enterprises would be hesitant about sharing with outsiders ( Paul, 2014 ). This information is, however, crucial to the development of a cyber vigilance task. Access and confidentiality can therefore hinder the process of designing a vigilance task that accurately parallels the operational cognitive demands of network defense ( Paul, 2014 ). In contrast, Mackworth (1948 , 1950) was able to rely on support from the Royal Air Force to create his formative clock vigilance task. For example, the Royal Air Force granted Mackworth direct access to their radar equipment and operators, at a time in history where this critical strategic information would have been closely guarded in Europe after World War Two.
Task complexity
The sheer complexity of cyber security may also explain why there are so few vigilance tasks for network defense in the literature. That is, simulating the complex demands of operational network defense is central to the development of a generalizable cyber vigilance task ( Reinerman-Jones et al., 2010 ; Hancock, 2013 ). This is because the behavioral presentation of vigilance decrement functions according to the domain specific demands of the task being performed ( Donald, 2008 ; Reinerman-Jones et al., 2010 ; Hancock, 2013 ). That is, if the demands of an operational vigilance task are not accurately captured by its laboratory analog, then the behavioral presentation of any performance decrement that occurs may not generalize to the operational setting ( Donald, 2008 ; Reinerman-Jones et al., 2010 ; Hancock, 2013 ). The predictive validity of laboratory-based vigilance research hence hinges on the degree to which task demands match what is observed operationally ( Donald, 2008 ; Reinerman-Jones et al., 2010 ; Hancock, 2013 ; Gutzwiller et al., 2015 ).
Non-standard operating environments
The absence of a validated cyber vigilance task in the literature may also be explained by the fact that network defense analysts are known to customize their work terminals. SEIMs integrate cyber threat intelligence, derived from inbound and outbound network traffic, and present this to analysts, who then action appropriate defensive responses to virtual threats ( Tresh and Kovalsky, 2018 ).
SEIMS are built according to the diverse cyber security needs of specific organizations, and are not engineered according to a common, standardized design. In contrast, Mackworth (1948 , 1950) was able to derived the clock task from real world radar display that was characterized by a standardized design. However, SEIM's are not designed according to a standardized design, and as such, it was not possible to derive a modern cyber vigilance task from a given SEIM in industry in the same way Mackworth's (1948 , 1950) clocks were based on real world radars ( Work, 2020 ).
Further complicating the challenge of designing a modern cyber vigilance task, in addition to non-standard SEIM designs, is the fact that many analysts also customize their personal workstations, a practice that produces radical differences in task performance even within the same cyber security team ( Hao et al., 2013 ). These customisations alter the cognitive load required to use a SEIM, which in turn can alter the behavioral presentation of vigilance decrement.
Rapid obsolescence
Like many technology subfields, cyber security is evolving quickly ( Gutzwiller et al., 2015 ). Moreover, the rate of evolution in cyber security is unlike the rate in any other domain in which vigilance decrement has been observed. Rapid evolution in the technological complexity of cyber security may also explain why the literature lacks a modern vigilance task for network defense. Cyber vigilance tasks can become obsolete experimental tools as quickly as the systems they have been designed to emulate ( Gutzwiller et al., 2015 ). For example, although cars vary in the design and layout of their control surfaces, driving has remained a fundamentally unchanged task for decades. In turn, driver vigilance tasks have likewise remained fundamentally the same for decades ( Milakis et al., 2015 ). Hence, unlike cyber security, the validity of driver vigilance tasks is unlikely to degrade over time, as the fundamental elements of the task are also unlikely to change significantly ( Gutzwiller et al., 2015 ).
Cyber security's rapid evolution therefore limits the long-term validity of any vigilance task designed for the space. For example, the single computer monitor used to run McIntire et al.'s (2013) cyber vigilance task shows its age. In comparison to 2013, modern network defense is too complex a task to complete on a single computer monitor, which forces analysts to divide their attention across multiple screens of information ( D'Amico et al., 2005 ; Axon et al., 2018 ). This difference in required screen real estate reflects an evolution in the volume of information that human operators are required to handle in the defense of a network. This in turn reflects growth in the level of cognitive load that analysts must sustain as they hunt for threats distributed across the virtual threat landscape. McIntire et al.'s (2013) single-screen cyber vigilance task therefore inaccurately simulated the demands associated with modern network defense. Furthermore, this suggests that the validity of cyber vigilance tasks may be sensitive to the rapid rate at which the technological tools develop in this space.
Tasks that require routine updates to remain valid are not uncommon in the psychological space. For example, the Wechsler Adult Intelligence Scale is an established psychometric instrument that requires routine updates to minimize reduced validity ( Wechsler, 2002 ). Cyber vigilance tasks might likewise require periodic updates to maintain valid simulators of network defense. Hence McIntire et al.'s (2013) CDT may have reasonably approximated the demands of network security at the time it was published. However, by the standards of modern network defense, McIntire et al.'s (2013) task is outdated. Had the CDT been updated periodically to keep up with developments in network security, this would have preserved some degree of its validity as a vigilance task.
Table 3 summarizes the various challenges McIntire et al. (2013) , Mancuso et al. (2015) , and Sawyer et al. (2016) encountered in creating a cyber vigilance task. These are challenges future researchers will need to navigate if the gap in the literature left by a modern, validated cyber vigilance task is to ever be addressed.
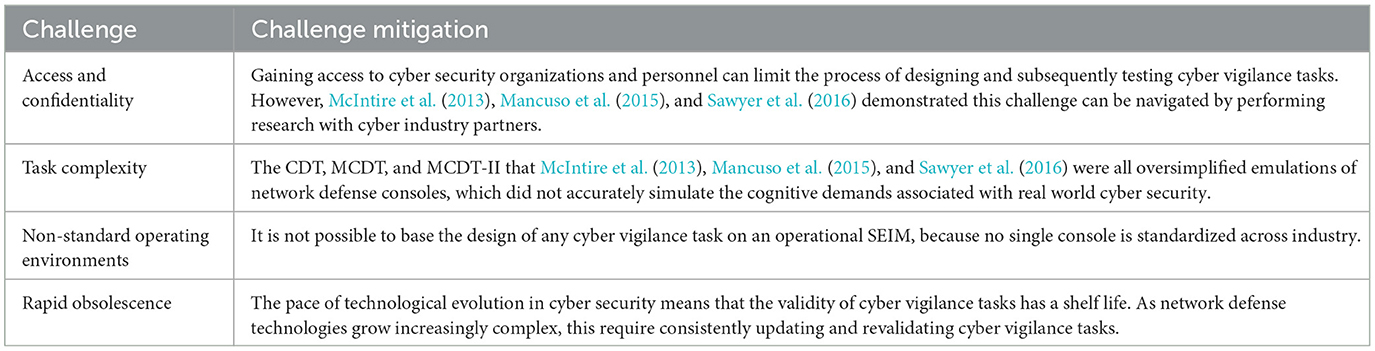
Table 3 . Cyber vigilance task creation challenges.
In closing, vigilance decrement is a cyber-cognitive vulnerability which must be better understood to manage it as a human factor security risk. However, advancing our understanding of vigilance decrement in the network defense space necessitates developing experimental testbeds that accommodate access and confidentiality, task complexity, non-standard operating environments, and rapid obsolescence. Moving forward, improving the interaction between SEIM consoles and human network defense analysts, necessitates developing an updated cyber vigilance task that is also valid according to Parasuraman's (1979 , 1985) parameters.
Author contributions
All authors listed have made a substantial, direct, and intellectual contribution to the work and approved it for publication.
This work has been supported by the Cyber Security Research Centre Limited whose activities are partially funded by the Australian Government's Cooperative Research Centres Programme.
Conflict of interest
The authors declare that the research was conducted in the absence of any commercial or financial relationships that could be construed as a potential conflict of interest.
Publisher's note
All claims expressed in this article are solely those of the authors and do not necessarily represent those of their affiliated organizations, or those of the publisher, the editors and the reviewers. Any product that may be evaluated in this article, or claim that may be made by its manufacturer, is not guaranteed or endorsed by the publisher.
Aidman, E., Chadunow, C., Johnson, K., and Reece, J. (2015). Real-time driver drowsiness feedback improves driver alertness and self-reported driving performance. Accid. Anal. Prev . 81, 8–13. doi: 10.1016/j.aap.2015.03.041
PubMed Abstract | CrossRef Full Text | Google Scholar
Albayati, M., and Issac, B. (2015). Analysis of intelligent classifiers and enhancing the detection accuracy for intrusion detection system. Int. J. Comput. Intell. Syst . 8, 841–853. doi: 10.1080/18756891.2015.1084705
Aleem, A., and Ryan Sprott, C. (2013). Let me in the cloud: Analysis of the benefit and risk assessment of cloud platform. J. Fin. Crime 20, 6–24. doi: 10.1108/13590791311287337
CrossRef Full Text | Google Scholar
Alhawari, S., Karadsheh, L., Talet, A. N., and Mansour, E. (2012). Knowledge-based risk management framework for information technology project. Int. J. Informat. Manag . 32, 50–65. doi: 10.1016/j.ijinfomgt.2011.07.002
Alserhani, F., Akhlaq, M., Awan, I. U., Cullen, A. J., and Mirchandani, P. (2010). “MARS: Multi-stage attack recognition system 2010,” in 24th IEEE International Conference on Advanced Information Networking and Applications (Perth). doi: 10.1109/AINA.2010.57
Al-Shargie, F., Tariq, U., Mir, H., Alawar, H., Babiloni, F., and Al-Nashash, H. (2019). Vigilance decrement and enhancement techniques: A review. Brain Sci . 9, 178. doi: 10.3390/brainsci9080178
Axon, L., Alahmadi, B., Nurse, J., Goldsmith, M., and Creese, S. (2018). “Sonification in security operations centres: What do security practitioners think?,” The Network and Distributed System Security (NDSS) Symposium 2018 . San Diego, CA. doi: 10.14722/usec.2018.23024
Baker, C. (1959). Attention to visual displays during a vigilance task: II. Maintaining the level of vigilance. Br. J. Psychol . 50, 30–36. doi: 10.1111/j.2044-8295.1959.tb00678.x
Barford, P., Dacier, M., Dietterich, T. G., Fredrikson, M., Giffin, J., Jajodia, S., et al. (2010). “Cyber SA: Situational awareness for cyber defense,” in Cyber Situational Awareness (Berlin: Springer), 3–13. doi: 10.1007/978-1-4419-0140-8_1
Ben-Asher, N., and Gonzalez, C. (2015). Effects of cyber security knowledge on attack detection. Comput. Hum. Behav . 48, 51–61. doi: 10.1016/j.chb.2015.01.039
Bodala, I. P., Li, J., Thakor, N. V., and Al-Nashash, H. (2016). EEG and eye tracking demonstrate vigilance enhancement with challenge integration. Front. Hum. Neurosci . 10, 273. doi: 10.3389/fnhum.2016.00273
Bridges, N. R. (2011). Predicting Vigilance Performance Under Transcranial Direct Current Stimulation (Publication Number 1047). (Masters Thesis), Wright State University, Dayton, OH . Available online at: https://corescholar.libraries.wright.edu/etd_all/1047/ (accessed March 6, 2020).
Google Scholar
Cabrall, C., Happee, R., and De Winter, J. (2016). From Mackworth's clock to the open road: A literature review on driver vigilance task operationalization. Transport. Res. F 40, 169–189. doi: 10.1016/j.trf.2016.04.001
Cavelty, M. D. (2014). Breaking the cyber-security dilemma: Aligning security needs and removing vulnerabilities. Sci. Eng. Ethics 20, 701–715. doi: 10.1007/s11948-014-9551-y
Chappelle, W., McDonald, K., Christensen, J., Prince, L., Goodman, T., Thompson, W., et al (2013). Sources of Occupational Stress and Prevalence of Burnout and Clinical Distress Among US Air Force Cyber Warfare Operators [Final Technical Report] (88ABW-2013-2089) . Available online at: https://apps.dtic.mil/dtic/tr/fulltext/u2/a584653.pdf (accessed March 6, 2020).
Cunningham, S. G., and Freeman, F. (1994). The Electrocortical Correlates of Fluctuating States of Attention During Vigilance Tasks [Contractor Report (CR)](19950008450). (NASA Contractor Report – NASA-CR-197051., NASA Contractor Report – NASA CR-197051, Issue . Available online at: https://ntrs.nasa.gov/api/citations/19950008450/downloads/19950008450.pdf (accessed March 7, 2020).
Daly, T., Murphy, J., Anglin, K., Szalma, J., Acree, M., Landsberg, C., et al. (2017). “Moving vigilance out of the laboratory: Dynamic scenarios for UAS operator vigilance training,” in Augmented Cognition. Enhancing Cognition and Behavior in Complex Human Environments (Berlin: Springer International Publishing), 20–35. doi: 10.1007/978-3-319-58625-0_2
D'Amico, A., Whitley, K., Tesone, D., O'Brien, B., and Roth, E. (2005). Achieving cyber defense situational awareness: A cognitive task analysis of information assurance analysts. Proc. Hum. Fact. Ergon. Soc. Ann. Meet . 49, 229–233. doi: 10.1177/154193120504900304
Dember, W. N., Galinsky, T. L., and Warm, J. S. (1992). The role of choice in vigilance performance. Bullet. Psychon. Soc . 30, 201–204. doi: 10.3758/BF03330441
DoD (2014). Mission Analysis for Cyber Operations of Department of Defense (E-0CD45F6) . Available online at: https://info.publicintelligence.net/DoD-CyberMissionAnalysis.pdf (accessed April 4, 2020).
Donald, F. M. (2008). The classification of vigilance tasks in the real world. Ergonomics 51, 1643–1655. doi: 10.1080/00140130802327219
D'Orazio, T., Leo, M., Guaragnella, C., and Distante, A. (2007). A visual approach for driver inattention detection. Patt. Recogn . 40, 2341–2355. doi: 10.1016/j.patcog.2007.01.018
Endsley, M., and Kiris, E. (1995). The out-of-the-loop performance problem and level of control in automation. Hum. Fact . 37, 32–64. doi: 10.1518/001872095779049543
Epling, S. L., Russell, P. N., and Helton, W. S. (2016). A new semantic vigilance task: Vigilance decrement, workload, and sensitivity to dual-task costs. Exp. Brain Res . 234, 133–139. doi: 10.1007/s00221-015-4444-0
Erola, A., Agrafiotis, I., Happa, J., Goldsmith, M., Creese, S., and Legg, P. A. (2017). “RicherPicture: Semi-automated cyber defence using context-aware data analytics,” in The 2017 International Conference On Cyber Situational Awareness, Data Analytics And Assessment (Cyber SA) (London) doi: 10.1109/CyberSA.2017.8073399
Fathi, N., Mehraban, A. H., Akbarfahimi, M., and Mirzaie, H. (2017). Validity and reliability of the test of everyday attention for children (teach) in Iranian 8-11 year old normal students. Iran. J. Psychiatr. Behav. Sci . 11, 1–7. doi: 10.5812/ijpbs.2854
Finomore, V., Matthews, G., Shaw, T., and Warm, J. (2009). Predicting vigilance: A fresh look at an old problem. Ergonomics 52, 791–808. doi: 10.1080/00140130802641627
Franke, U., and Brynielsson, J. (2014). Cyber situational awareness – A systematic review of the literature. Comput. Secur . 46, 18–31. doi: 10.1016/j.cose.2014.06.008
Gartenberg, D., Gunzelmann, G., Hassanzadeh-Behbaha, S., and Trafton, J. G. (2018). Examining the role of task requirements in the magnitude of the vigilance decrement. Front. Psychol. 9, 1504. doi: 10.3389/fpsyg.2018.01504
Gartenberg, D., Gunzelmann, G., Veksler, B. Z., and Trafton, J. G. (2015). “Improving vigilance analysis methodology: questioning the successive versus simultaneous distinction,” in Proceedings of the Human Factors and Ergonomics Society Annual Meeting (Los Angeles, CA) doi: 10.1177/1541931215591059
Gaw, T. J. (2014). ARL-VIDS Visualization Techniques: 3D Information Visualization of Network Security Events (Publication Number 882577849). (Masters Thesis), Ball State University, Muncie, IN . Available online at: http://liblink.bsu.edu/catkey/1745749 (accessed April 1, 2020).
Gopalakrishnan, S. (2012). A public health perspective of road traffic accidents. J. Fam. Med. Primary Care 1, 144–150. doi: 10.4103/2249-4863.104987
Gordon, L. A., Loeb, M. P., and Zhou, L. (2011). The impact of information security breaches: Has there been a downward shift in costs? J. Comput. Secur . 19, 33–56. doi: 10.3233/JCS-2009-0398
Goutam, R. K. (2015). Importance of cyber security. Int. J. Comput. Appl. 111, 1250. doi: 10.5120/19550-1250
Gutzwiller, R. S., Fugate, S., Sawyer, B. D., and Hancock, P. (2015). “The human factors of cyber network defense,” in Proceedings of the Human Factors and Ergonomics Society Annual Meeting. (Los Angeles, CA). doi: 10.1177/1541931215591067
Gutzwiller, R. S., Hunt, S. M., and Lange, D. S. (2016). “A task analysis toward characterizing cyber-cognitive situation awareness (CCSA) in cyber defense analysts,” in The 2016 IEEE International Multi-Disciplinary Conference on Cognitive Methods in Situation Awareness and Decision Support (CogSIMA) . (San Diego, CA). doi: 10.1109/COGSIMA.2016.7497780
Hancock, P. A. (2013). In search of vigilance: The problem of iatrogenically created psychological phenomena. Am. Psycholog . 68, 97–109. doi: 10.1037/a0030214
Hancock, P. A., and Hart, S. (2002). Defeating terrorism: What can human factors/ergonomics offer? Ergon. Design 10, 6–16. doi: 10.1177/106480460201000103
Hao, L., Healey, C. G., and Hutchinson, S. E. (2013). Flexible web visualization for alert-based network security analytics. Assoc. Comput. Machinery . doi: 10.1145/2517957.2517962
Hatori, M., Gronfier, C., Van Gelder, R. N., Bernstein, P. S., Carreras, J., Panda, S., et al. (2017). Global rise of potential health hazards caused by blue light-induced circadian disruption in modern aging societies. NPJ Aging Mechanisms Dis . 3, 1–3. doi: 10.1038/s41514-017-0010-2
Heeger, D. (1997). Signal Detection Theory. New York University . Available online at: https://www.cns.nyu.edu/~david/handouts/sdt/sdt.html (accessed May 31, 2020).
Heeger, D. (2007). Signal Detection Theory. New York University . Available online at: https://www.cns.nyu.edu/david/handouts/sdt/sdt.html (accessed May 31, 2020).
Helton, W. S., Dember, W. N., Warm, J. S., and Matthews, G. (1999). Optimism, pessimism, and false failure feedback: Effects on vigilance performance. Curr. Psychol . 18, 311–325. doi: 10.1007/s12144-999-1006-2
Helton, W. S., Shaw, T. H., Warm, J. S., Dember, G. M. W. N., and Hancock, P. A. (2004). “Demand transitions in vigilance: Effects on performance efficiency and stress,” in Human Performance, Situation Awareness, and Automation: Current Research and Trends HPSAA II, Volumes I and II , eds V. M. Mouloua and P. A. Hancock (Mahwah, NJ: Lawrence Erlbaum Associates, Inc., Publishers), 258–263.
PubMed Abstract | Google Scholar
Hicks, J. M. (2015). A Theater-Level Perspective on Cyber (0704-0188). N. D. U. Press . Available online at: https://apps.dtic.mil/dtic/tr/fulltext/u2/a618537.pdf (accessed April 3, 2020).
Holzman, D. C. (2010). What's in a color? The unique human health effects of blue light. Environ. Health Perspect . 118, 22–27. doi: 10.1289/ehp.118-a22
Jo, J., Lee, S. J., Park, K. R., Kim, I.-J., and Kim, J. (2014). Detecting driver drowsiness using feature-level fusion and user-specific classification. Expert Syst. Appl . 41, 1139–1152. doi: 10.1016/j.eswa.2013.07.108
Jolley, J. D. (2012). Article 2 and Cyber Warfare: How Do Old Rules Control the Brave New World? Available at SSRN 2128301. 2 . World Wide Organisation; Institution of Engineering and Technology. 1–16. doi: 10.5539/ilr.v2n1p1
Joly, A., Zheng, R., Kaizuka, T., and Nakano, K. (2017). Effect of drowsiness on mechanical arm admittance and driving performances. Inst. Eng. Technol. Intell. Transport Syst . 12, 220–226. doi: 10.1049/iet-its.2016.0249
Komlodi, A., Goodall, J. R., and Lutters, W. G. (2004). “An information visualization framework for intrusion detection,” in Association for Computing Machinery 2004 Conference on Human Factors in Computing Systems. (Vienna). doi: 10.1145/985921.1062935
Lockley, S. W., Evans, E. E., Scheer, F. A., Brainard, G. C., Czeisler, C. A., and Aeschbach, D. (2006). Short-wavelength sensitivity for the direct effects of light on alertness, vigilance, and the waking electroencephalogram in humans. Sleep 29, 161–168. doi: 10.1093/sleep/29.2.161
Mackworth, J. F. (1968). Vigilance, arousal, and habituation. Psychol. Rev . 4, 308–322. doi: 10.1037/h0025896
Mackworth, N. H. (1948). The breakdown of vigilance during prolonged visual search. Quart. J. Exp. Psychol . 1, 6–21. doi: 10.1080/17470214808416738
Mackworth, N. H. (1950). Researches on the measurement of human performance. J. Royal Stat. Soc. Ser. A . 113, 588–589. doi: 10.2307/2980885
Majeed, A., ur Rasool, R., Ahmad, F., Alam, M., and Javaid, N. (2019). Near-miss situation based visual analysis of SIEM rules for real time network security monitoring. J. Ambient Intell. Human. Comput . 10, 1509–1526. doi: 10.1007/s12652-018-0936-7
Mancuso, V. F., Christensen, J. C., Cowley, J., Finomore, V., Gonzalez, C., and Knott, B. (2014). “Human factors in cyber warfare II: Emerging perspectives,” in Proceedings of the Human Factors and Ergonomics Society Annual Meeting. (Chicago, IL). doi: 10.1177/1541931214581085
Mancuso, V. F., Greenlee, E. T., Funke, G., Dukes, A., Menke, L., Brown, R., et al. (2015). Augmenting cyber defender performance and workload through sonified displays. Proc. Manufact . 3, 5214–5221. doi: 10.1016/j.promfg.2015.07.589
Maybury, M. T. (2012). “Air force cyber vision 2025,” in 5th International Symposium on Resilient Control Systems . Salt Lake City, UT.
McIntire, L., McKinley, R. A., McIntire, J., Goodyear, C., and Nelson, J. (2013). Eye metrics: An alternative vigilance detector for military operators. Milit. Psychol . 25, 502–513. doi: 10.1037/mil0000011
Milakis, D., Van Arem, B., and Van Wee, B. (2015). The Ripple Effect of Automated Driving BIVEC-GIBET Transport Research Day, May 28–29. 2015, Eindhoven, The Netherlands . Available online at: http://resolver.tudelft.nl/uuid:e6ecff79-4334-4baa-a60b-3ed897587157 (accessed April 3, 2020).
Nanay, B. (2018). Perception is not all-purpose. Synthese 1, 1–12. doi: 10.1007/s11229-018-01937-5
Newcomb, E. A., and Hammell, R. J. (2016). “A fuzzy logic utility framework (FLUF) to support information assurance,” in Software Engineering Research, Management and Applications , ed R. Lee (Berlin: Springer), 33–48. doi: 10.1007/978-3-319-33903-0_3
O'Connell, M. E. (2012). Cyber security without cyber war. J. Conflict Secur. Law 17, 187–209. doi: 10.1093/jcsl/krs017
Ormrod, D. (2014). “The coordination of cyber and kinetic deception for operational effect: Attacking the C4ISR interface,” in The 2014 IEEE Military Communications Conference . Baltimore, MD.
Parasuraman, R. (1979). Memory load and event rate control sensitivity decrements in sustained attention. Science 205, 924–927. doi: 10.1126/science.472714
Parasuraman, R. (1985). “Sustained attention: A multifactorial approach,” in Attention and Performance XI, Vol. 1482 , ed M. I. Posner and M. S. Oscar (Mahwah, NJ: Lawrence Erlbaum Associates, Inc., Publishers), 493–511.
Parasuraman, R., and Mouloua, M. (1987). Interaction of signal discriminability and task type in vigilance decrement. Percept. Psychophys . 41, 17–22. doi: 10.3758/BF03208208
Pattyn, N., Neyt, X., Henderickx, D., and Soetens, E. (2008). Psychophysiological investigation of vigilance decrement: Boredom or cognitive fatigue? Physiol. Behav. 93, 369–378. doi: 10.1016/j.physbeh.2007.09.016
Paul, C. L. (2014). “Human-centered study of a network operations center: Experience report and lessons learned,” in Proceedings of the 2014 ACM Workshop on Security Information Workers (New York, NY). doi: 10.1145/2663887.2663899
Pompon, R., Walkowski, D., Boddy, S., and Levin, M. (2018). 2018 Phishing and Fraud Report - Attacks Peak During The Holidays (Phishing and Fraud Report, Issue. F. Labs). Available online at: https://www.f5.com/labs/articles/threat-intelligence/2018-phishing-and-fraud-report–attacks-peak-during-the-holidays (accessed April 3, 2020).
Pradhapan, P., Griffioen, R., Clerx, M., and Mihajlović, V. (2017). “Personalized characterization of sustained attention/vigilance in healthy children,” in Lecture Notes of the Institute for Computer Sciences, Social Informatics and Telecommunications Engineering, Vol. 181 , eds K. Giokas, L. Bokor, and F. Hopfgartner (Cham: Springer International Publishing), 271–281. doi: 10.1007/978-3-319-49655-9_35
Rajan, A. V., Ravikumar, R., and Al Shaer, M. (2017). “UAE cybercrime law and cybercrimes—An analysis,” in The 2017 International Conference on Cyber Security And Protection Of Digital Services (Cyber Security) . doi: 10.1109/CyberSecPODS.2017.8074858
Rajivan, P., and Cooke, N. (2017). “Impact of team collaboration on cybersecurity situational awareness,” in Theory and Models for Cyber Situation Awareness , eds P. Liu, S. Jajodia, and C. Wang (Cham: Springer International Publishing), 203–226. doi: 10.1007/978-3-319-61152-5_8
Rajivan, P., Janssen, M. A., and Cooke, N. J. (2013). “Agent-based model of a cyber security defense analyst team,” in Proceedings of the Human Factors and Ergonomics Society Annual Meeting (San Diego, CA), doi: 10.1177/1541931213571069
Reinerman-Jones, L., Matthews, G., and Mercado, J. E. (2016). Detection tasks in nuclear power plant operation: Vigilance decrement and physiological workload monitoring. Saf. Sci . 88, 97–107. doi: 10.1016/j.ssci.2016.05.002
Reinerman-Jones, L. E., Matthews, G., Langheim, L. K., and Warm, J. S. (2010). Selection for vigilance assignments: A review and proposed new direction. Theoret. Iss. Ergon. Sci . 12, 273–296. doi: 10.1080/14639221003622620
Rodriguez, A., and Okamura, K. (2019). “Generating real time cyber situational awareness information through social media data mining,” in 2019 IEEE 43rd Annual Computer Software and Applications Conference (COMPSAC). (Milwaukee, WI). doi: 10.1109/COMPSAC.2019.10256
Saltzman, I. (2013). Cyber posturing and the offense-defense balance. Contemp. Secur. Pol . 34, 40–63. doi: 10.1080/13523260.2013.771031
Satterfield, K., Harwood, A. E., Helton, W. S., and Shaw, T. H. (2019). Does depleting self-control result in poorer vigilance performance? Hum. Fact . 61, 415–425. doi: 10.1177/0018720818806151
Sawyer, B. D., Finomore, V. S., Funke, G. J., Matthews, G., Mancuso, V., Funke, M., et al (2016). Cyber Vigilance: The Human Factor (0704-0188) . Available online at: https://apps.dtic.mil/sti/pdfs/AD1021913.pdf (accessed April 4, 2020).
Sawyer, B. D., and Hancock, P. A. (2018). Hacking the human: The prevalence paradox in cybersecurity. Hum. Fact . 60, 597–609. doi: 10.1177/0018720818780472
See, J. E. (2014). Vigilance: A Review of the Literature and Applications to Sentry Duty (SAND2014-17929) . United States: Office of Scientific and Technical Information (OSTI). doi: 10.2172/1322275
See, J. E., Howe, S. R., Warm, J. S., and Dember, W. N. (1995). Meta-analysis of the sensitivity decrement in vigilance. Psychol. Bullet . 117, 230–249. doi: 10.1037/0033-2909.117.2.230
Sherwood, M. S., Kane, J. H., Weisend, M. P., and Parker, J. G. (2016). Enhanced control of dorsolateral prefrontal cortex neurophysiology with real-time functional magnetic resonance imaging (rt-fMRI) neurofeedback training and working memory practice. Neuroimage 124, 214–223. doi: 10.1016/j.neuroimage.2015.08.074
Simmons, C. B., Shiva, S. G., Bedi, H. S., and Shandilya, V. (2013). “ADAPT: A game inspired attack-defense and performance metric taxonomy,” in IFIP International Information Security Conference (Memphis, MS). doi: 10.1007/978-3-642-39218-4_26
Skopik, F., Settanni, G., and Fiedler, R. (2016). A problem shared is a problem halved: A survey on the dimensions of collective cyber defense through security information sharing. Comput. Secur . 60, 154–176. doi: 10.1016/j.cose.2016.04.003
Smith, M. (2016). “The Effect of Perceived Humanness in Non-Human Robot Agents on Social Facilitation in a Vigilance Task (Publication Number 10132069) . (Doctoral dissertation), George Mason University, Fairfax, VA. Available online at: https://search.proquest.com/openview/49fba8a8ccd3001dd6465ccb7bddbd70/1?pq-origsite=gscholar&cbl=18750&diss=y (accessed April 5, 2020).
Sommer, D., and Golz, M. (2010). “Evaluation of PERCLOS based current fatigue monitoring technologies,” The 2010 Annual International Conference of the IEEE Engineering in Medicine and Biology (Buenos Aires). doi: 10.1109/IEMBS.2010.5625960
Sostek, A. J. (1978). Effects of electrodermal lability and payoff instructions on vigilance performance. Psychophysiology 15, 561–568. doi: 10.1111/j.1469-8986.1978.tb03110.x
Spathoulas, G. P., and Katsikas, S. K. (2010). Reducing false positives in intrusion detection systems. Comput. Secur . 29, 35–44. doi: 10.1016/j.cose.2009.07.008
Spathoulas, G. P., and Katsikas, S. K. (2013). Enhancing IDS performance through comprehensive alert post-processing. Comput. Secur . 37, 176–196. doi: 10.1016/j.cose.2013.03.005
St John, M., Risser, M. R., and Kobus, D. A. (2006). “Toward a usable closed-loop attention management system: Predicting vigilance from minimal contact head, eye, and EEG measures,” in Proceedings of the 2nd Annual Augmented Cognition, San Franciso, CA . Available online at: http://citeseerx.ist.psu.edu/viewdoc/download?doi=10.1.1.135.1229&rep=rep1&type=pdf (accessed April 5, 2020).
Tan, H., and Zhang, Y.-J. (2006). Detecting eye blink states by tracking iris and eyelids. Pat. Recogn. Lett . 27, 667–675. doi: 10.1016/j.patrec.2005.10.005
Thiffault, P., and Bergeron, J. (2003a). Fatigue and individual differences in monotonous simulated driving. Personal. Individ. Diff . 34, 159–176. doi: 10.1016/S0191-8869(02)00119-8
Thiffault, P., and Bergeron, J. (2003b). Monotony of road environment and driver fatigue: A simulator study. Accid. Anal. Prev . 35, 381–391. doi: 10.1016/S0001-4575(02)00014-3
Thomason, S. (2013). People–The weak link in security. Glob. J. Comput. Sci. Technol .
Tian, H. T., Huang, L. S., Zhou, Z., and Luo, Y. L. (2004). “Arm up administrators: Automated vulnerability management,” in 7th International Symposium on Parallel Architectures, Algorithms and Networks, 2004. Proceedings (Hong Kong). doi: 10.1109/ISPAN.2004.1300542
Tong, M., and Thomson, C. (2015). “Developing a critical literature review for project management research,” in Designs, Methods and Practices for Research of Project Management . (London: Gower Publishing Limited; Routledge), 153–171).
Tresh, K., and Kovalsky, M. (2018). Toward Automated Information Sharing California: Cybersecurity Integration Center's approach to improve on the traditional information sharing models. Cyber Defense Rev . 3, 23–32. Available online at: https://www.jstor.org/stable/26491220
Tyworth, M., Giacobe, N. A., and Mancuso, V. (2012). Cyber situation awareness as distributed socio-cognitive work. Cyber Sens. 2012, 919338. doi: 10.1117/12.919338
Valdez, P. (2019). Homeostatic and circadian regulation of cognitive performance. Biolog. Rhythm Res . 50, 85–93. doi: 10.1080/09291016.2018.1491271
Vieane, A., Funke, G., Gutzwiller, R., Mancuso, V., Sawyer, B., and Wickens, C. (2016). “Addressing human factors gaps in cyber defense,” in Proceedings of the Human Factors and Ergonomics Society Annual Meeting (Washington, DC). doi: 10.1177/1541931213601176
Wall, D. S., and Williams, M. L. (2013). Policing cybercrime: Networked and social media technologies and the challenges for policing. Policing Soc . 23, 409–412. doi: 10.1080/10439463.2013.780222
Warm, J. S., and Dember, W. (1998). “Tests of vigilance taxonomy,” in Viewing Psychology as a Whole: The Integrative Science of William N. Dember , eds R. R. Hoffman and M. F. Sherrick (Washington, DC: American Psychological Association). doi: 10.1037/10290-004
Warm, J. S., Matthews, G., and Finomore, V. S. (2018). “Vigilance, workload, and stress,” in Performance Under Stress , eds P. A. Hancock and J. L. Szalma (Boca Raton, FL: CRC Press), 131–158.
Warm, J. S., Matthews, G., and Parasuraman, R. (2009). Cerebral hemodynamics and vigilance performance. Milit. Psychol. 21, 75–100. doi: 10.1080/08995600802554706
Warm, J. S., Parasuraman, R., and Matthews, G. (2008). Vigilance requires hard mental work and is stressful. Hum. Fact . 50, 433–441. doi: 10.1518/001872008X312152
Wechsler, D. (2002). Technical Manual (Updated) for the Wechsler Adult Intelligence Scale, 3rd ed. and Wechsler Memory Scale, 3rd ed. San Antonio: Psychological Corporation (3rd ed.) . San Antonio, TX: The Psychological Corporation.
Wickens, C. D. (2008). Situation awareness: Review of Mica Endsley's 1995 articles on situation awareness theory and measurement. Hum. Fact . 50, 397–403. doi: 10.1518/001872008X288420
Wickens, C. D., Gutzwiller, R., and Santamaria, A. (2015). Discrete task switching in overload: A meta-analyses and a model. Int. J. Hum. Comput. Stud . 79, 79–84. doi: 10.1016/j.ijhcs.2015.01.002
Wickens, C. D., Mavor, A. S., and McGee, J. (1997). Panel on Human Factors in Air Traffic Control Automation (N. A. Press, Ed.) . Washington, DC: National Research Council.
Work, J. (2020). Evaluating commercial cyber intelligence activity. Int. J. Intell. Counter Intelligence 33, 278–308. doi: 10.1080/08850607.2019.1690877
Yahya, F., Hassanin, O., Tariq, U., and Al-Nashash, H. (2020). EEG-Based Semantic Vigilance Level Classification Using Directed Connectivity Patterns and Graph Theory Analysis . World Wide Organisation; IEEE Access.
Zheng, W. L., Gao, K., Li, G., Liu, W., Liu, C., Liu, J. Q., et al. (2019). Vigilance estimation using a wearable EOG device in real driving environment. IEEE Trans. Intell. Transport. Syst . 1, 1–15. doi: 10.1109/TITS.2018.2889962
Zhong, C., Yen, J., Liu, P., Erbacher, R., Etoty, R., and Garneau, C. (2015). “ARSCA: A computer tool for tracing the cognitive processes of cyber-attack analysis,” in The 2015 IEEE International Multi-Disciplinary Conference on Cognitive Methods in Situation Awareness and Decision (Xi'an) doi: 10.1109/COGSIMA.2015.7108193
Zhong, S. C., Song, Q. F., Cheng, X. C., and Zhang, Y. (2003). “A safe mobile agent system for distributed intrusion detection,” in Proceedings of the 2003 International Conference on Machine Learning and Cybernetics (IEEE Cat. No. 03EX693) (San Diego, CA).
Keywords: vigilance, tasks, cyber defense, Security Event Information Management, vigilance decrement, sustained attention response task
Citation: Guidetti OA, Speelman C and Bouhlas P (2023) A review of cyber vigilance tasks for network defense. Front. Neuroergon. 4:1104873. doi: 10.3389/fnrgo.2023.1104873
Received: 22 November 2022; Accepted: 29 March 2023; Published: 18 April 2023.
Reviewed by:
Copyright © 2023 Guidetti, Speelman and Bouhlas. This is an open-access article distributed under the terms of the Creative Commons Attribution License (CC BY) . The use, distribution or reproduction in other forums is permitted, provided the original author(s) and the copyright owner(s) are credited and that the original publication in this journal is cited, in accordance with accepted academic practice. No use, distribution or reproduction is permitted which does not comply with these terms.
*Correspondence: Oliver Alfred Guidetti, o.guidetti@ecu.edu.au
This article is part of the Research Topic
Leveraging Neurophysiological Measures to Account for Cognitive Performance in Complex, Uncertain and Resource Limited Settings
- Privacy Policy
Buy Me a Coffee

Home » 500+ Cyber Security Research Topics
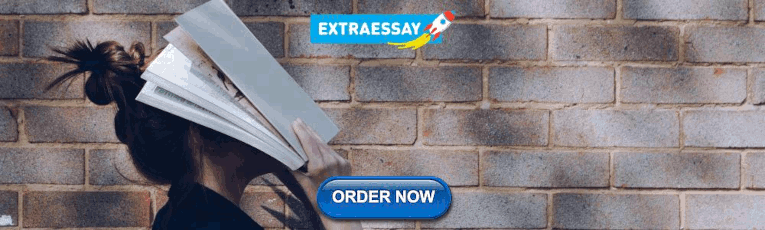
500+ Cyber Security Research Topics

Cybersecurity has become an increasingly important topic in recent years as more and more of our lives are spent online. With the rise of the digital age, there has been a corresponding increase in the number and severity of cyber attacks. As such, research into cybersecurity has become critical in order to protect individuals, businesses, and governments from these threats. In this blog post, we will explore some of the most pressing cybersecurity research topics, from the latest trends in cyber attacks to emerging technologies that can help prevent them. Whether you are a cybersecurity professional, a Master’s or Ph.D. student, or simply interested in the field, this post will provide valuable insights into the challenges and opportunities in this rapidly evolving area of study.
Cyber Security Research Topics
Cyber Security Research Topics are as follows:
- The role of machine learning in detecting cyber threats
- The impact of cloud computing on cyber security
- Cyber warfare and its effects on national security
- The rise of ransomware attacks and their prevention methods
- Evaluating the effectiveness of network intrusion detection systems
- The use of blockchain technology in enhancing cyber security
- Investigating the role of cyber security in protecting critical infrastructure
- The ethics of hacking and its implications for cyber security professionals
- Developing a secure software development lifecycle (SSDLC)
- The role of artificial intelligence in cyber security
- Evaluating the effectiveness of multi-factor authentication
- Investigating the impact of social engineering on cyber security
- The role of cyber insurance in mitigating cyber risks
- Developing secure IoT (Internet of Things) systems
- Investigating the challenges of cyber security in the healthcare industry
- Evaluating the effectiveness of penetration testing
- Investigating the impact of big data on cyber security
- The role of quantum computing in breaking current encryption methods
- Developing a secure BYOD (Bring Your Own Device) policy
- The impact of cyber security breaches on a company’s reputation
- The role of cyber security in protecting financial transactions
- Evaluating the effectiveness of anti-virus software
- The use of biometrics in enhancing cyber security
- Investigating the impact of cyber security on the supply chain
- The role of cyber security in protecting personal privacy
- Developing a secure cloud storage system
- Evaluating the effectiveness of firewall technologies
- Investigating the impact of cyber security on e-commerce
- The role of cyber security in protecting intellectual property
- Developing a secure remote access policy
- Investigating the challenges of securing mobile devices
- The role of cyber security in protecting government agencies
- Evaluating the effectiveness of cyber security training programs
- Investigating the impact of cyber security on the aviation industry
- The role of cyber security in protecting online gaming platforms
- Developing a secure password management system
- Investigating the challenges of securing smart homes
- The impact of cyber security on the automotive industry
- The role of cyber security in protecting social media platforms
- Developing a secure email system
- Evaluating the effectiveness of encryption methods
- Investigating the impact of cyber security on the hospitality industry
- The role of cyber security in protecting online education platforms
- Developing a secure backup and recovery strategy
- Investigating the challenges of securing virtual environments
- The impact of cyber security on the energy sector
- The role of cyber security in protecting online voting systems
- Developing a secure chat platform
- Investigating the impact of cyber security on the entertainment industry
- The role of cyber security in protecting online dating platforms
- Artificial Intelligence and Machine Learning in Cybersecurity
- Quantum Cryptography and Post-Quantum Cryptography
- Internet of Things (IoT) Security
- Developing a framework for cyber resilience in critical infrastructure
- Understanding the fundamentals of encryption algorithms
- Cyber security challenges for small and medium-sized businesses
- Developing secure coding practices for web applications
- Investigating the role of cyber security in protecting online privacy
- Network security protocols and their importance
- Social engineering attacks and how to prevent them
- Investigating the challenges of securing personal devices and home networks
- Developing a basic incident response plan for cyber attacks
- The impact of cyber security on the financial sector
- Understanding the role of cyber security in protecting critical infrastructure
- Mobile device security and common vulnerabilities
- Investigating the challenges of securing cloud-based systems
- Cyber security and the Internet of Things (IoT)
- Biometric authentication and its role in cyber security
- Developing secure communication protocols for online messaging platforms
- The importance of cyber security in e-commerce
- Understanding the threats and vulnerabilities associated with social media platforms
- Investigating the role of cyber security in protecting intellectual property
- The basics of malware analysis and detection
- Developing a basic cyber security awareness training program
- Understanding the threats and vulnerabilities associated with public Wi-Fi networks
- Investigating the challenges of securing online banking systems
- The importance of password management and best practices
- Cyber security and cloud computing
- Understanding the role of cyber security in protecting national security
- Investigating the challenges of securing online gaming platforms
- The basics of cyber threat intelligence
- Developing secure authentication mechanisms for online services
- The impact of cyber security on the healthcare sector
- Understanding the basics of digital forensics
- Investigating the challenges of securing smart home devices
- The role of cyber security in protecting against cyberbullying
- Developing secure file transfer protocols for sensitive information
- Understanding the challenges of securing remote work environments
- Investigating the role of cyber security in protecting against identity theft
- The basics of network intrusion detection and prevention systems
- Developing secure payment processing systems
- Understanding the role of cyber security in protecting against ransomware attacks
- Investigating the challenges of securing public transportation systems
- The basics of network segmentation and its importance in cyber security
- Developing secure user access management systems
- Understanding the challenges of securing supply chain networks
- The role of cyber security in protecting against cyber espionage
- Investigating the challenges of securing online educational platforms
- The importance of data backup and disaster recovery planning
- Developing secure email communication protocols
- Understanding the basics of threat modeling and risk assessment
- Investigating the challenges of securing online voting systems
- The role of cyber security in protecting against cyber terrorism
- Developing secure remote access protocols for corporate networks.
- Investigating the challenges of securing artificial intelligence systems
- The role of machine learning in enhancing cyber threat intelligence
- Evaluating the effectiveness of deception technologies in cyber security
- Investigating the impact of cyber security on the adoption of emerging technologies
- The role of cyber security in protecting smart cities
- Developing a risk-based approach to cyber security governance
- Investigating the impact of cyber security on economic growth and innovation
- The role of cyber security in protecting human rights in the digital age
- Developing a secure digital identity system
- Investigating the impact of cyber security on global political stability
- The role of cyber security in protecting the Internet of Things (IoT)
- Developing a secure supply chain management system
- Investigating the challenges of securing cloud-native applications
- The role of cyber security in protecting against insider threats
- Developing a secure software-defined network (SDN)
- Investigating the impact of cyber security on the adoption of mobile payments
- The role of cyber security in protecting against cyber warfare
- Developing a secure distributed ledger technology (DLT) system
- Investigating the impact of cyber security on the digital divide
- The role of cyber security in protecting against state-sponsored attacks
- Developing a secure Internet infrastructure
- Investigating the challenges of securing industrial control systems (ICS)
- Developing a secure quantum communication system
- Investigating the impact of cyber security on global trade and commerce
- Developing a secure decentralized authentication system
- Investigating the challenges of securing edge computing systems
- Developing a secure hybrid cloud system
- Investigating the impact of cyber security on the adoption of smart cities
- The role of cyber security in protecting against cyber propaganda
- Developing a secure blockchain-based voting system
- Investigating the challenges of securing cyber-physical systems (CPS)
- The role of cyber security in protecting against cyber hate speech
- Developing a secure machine learning system
- Investigating the impact of cyber security on the adoption of autonomous vehicles
- The role of cyber security in protecting against cyber stalking
- Developing a secure data-driven decision-making system
- Investigating the challenges of securing social media platforms
- The role of cyber security in protecting against cyberbullying in schools
- Developing a secure open source software ecosystem
- Investigating the impact of cyber security on the adoption of smart homes
- The role of cyber security in protecting against cyber fraud
- Developing a secure software supply chain
- Investigating the challenges of securing cloud-based healthcare systems
- The role of cyber security in protecting against cyber harassment
- Developing a secure multi-party computation system
- Investigating the impact of cyber security on the adoption of virtual and augmented reality technologies.
- Cybersecurity in Cloud Computing Environments
- Cyber Threat Intelligence and Analysis
- Blockchain Security
- Data Privacy and Protection
- Cybersecurity in Industrial Control Systems
- Mobile Device Security
- The importance of cyber security in the digital age
- The ethics of cyber security and privacy
- The role of government in regulating cyber security
- Cyber security threats and vulnerabilities in the healthcare sector
- Understanding the risks associated with social media and cyber security
- The impact of cyber security on e-commerce
- The effectiveness of cyber security awareness training programs
- The role of biometric authentication in cyber security
- The importance of password management in cyber security
- The basics of network security protocols and their importance
- The challenges of securing online gaming platforms
- The role of cyber security in protecting national security
- The impact of cyber security on the legal sector
- The ethics of cyber warfare
- The challenges of securing the Internet of Things (IoT)
- Understanding the basics of malware analysis and detection
- The challenges of securing public transportation systems
- The impact of cyber security on the insurance industry
- The role of cyber security in protecting against ransomware attacks
- The challenges of securing remote work environments
- Understanding the threats and vulnerabilities associated with social engineering attacks
- The impact of cyber security on the education sector
- Investigating the challenges of securing supply chain networks
- The challenges of securing personal devices and home networks
- The importance of secure coding practices for web applications
- The impact of cyber security on the hospitality industry
- The role of cyber security in protecting against identity theft
- The challenges of securing public Wi-Fi networks
- The importance of cyber security in protecting critical infrastructure
- The challenges of securing cloud-based storage systems
- The effectiveness of antivirus software in cyber security
- Developing secure payment processing systems.
- Cybersecurity in Healthcare
- Social Engineering and Phishing Attacks
- Cybersecurity in Autonomous Vehicles
- Cybersecurity in Smart Cities
- Cybersecurity Risk Assessment and Management
- Malware Analysis and Detection Techniques
- Cybersecurity in the Financial Sector
- Cybersecurity in Government Agencies
- Cybersecurity and Artificial Life
- Cybersecurity for Critical Infrastructure Protection
- Cybersecurity in the Education Sector
- Cybersecurity in Virtual Reality and Augmented Reality
- Cybersecurity in the Retail Industry
- Cryptocurrency Security
- Cybersecurity in Supply Chain Management
- Cybersecurity and Human Factors
- Cybersecurity in the Transportation Industry
- Cybersecurity in Gaming Environments
- Cybersecurity in Social Media Platforms
- Cybersecurity and Biometrics
- Cybersecurity and Quantum Computing
- Cybersecurity in 5G Networks
- Cybersecurity in Aviation and Aerospace Industry
- Cybersecurity in Agriculture Industry
- Cybersecurity in Space Exploration
- Cybersecurity in Military Operations
- Cybersecurity and Cloud Storage
- Cybersecurity in Software-Defined Networks
- Cybersecurity and Artificial Intelligence Ethics
- Cybersecurity and Cyber Insurance
- Cybersecurity in the Legal Industry
- Cybersecurity and Data Science
- Cybersecurity in Energy Systems
- Cybersecurity in E-commerce
- Cybersecurity in Identity Management
- Cybersecurity in Small and Medium Enterprises
- Cybersecurity in the Entertainment Industry
- Cybersecurity and the Internet of Medical Things
- Cybersecurity and the Dark Web
- Cybersecurity and Wearable Technology
- Cybersecurity in Public Safety Systems.
- Threat Intelligence for Industrial Control Systems
- Privacy Preservation in Cloud Computing
- Network Security for Critical Infrastructure
- Cryptographic Techniques for Blockchain Security
- Malware Detection and Analysis
- Cyber Threat Hunting Techniques
- Cybersecurity Risk Assessment
- Machine Learning for Cybersecurity
- Cybersecurity in Financial Institutions
- Cybersecurity for Smart Cities
- Cybersecurity in Aviation
- Cybersecurity in the Automotive Industry
- Cybersecurity in the Energy Sector
- Cybersecurity in Telecommunications
- Cybersecurity for Mobile Devices
- Biometric Authentication for Cybersecurity
- Cybersecurity for Artificial Intelligence
- Cybersecurity for Social Media Platforms
- Cybersecurity in the Gaming Industry
- Cybersecurity in the Defense Industry
- Cybersecurity for Autonomous Systems
- Cybersecurity for Quantum Computing
- Cybersecurity for Augmented Reality and Virtual Reality
- Cybersecurity in Cloud-Native Applications
- Cybersecurity for Smart Grids
- Cybersecurity in Distributed Ledger Technology
- Cybersecurity for Next-Generation Wireless Networks
- Cybersecurity for Digital Identity Management
- Cybersecurity for Open Source Software
- Cybersecurity for Smart Homes
- Cybersecurity for Smart Transportation Systems
- Cybersecurity for Cyber Physical Systems
- Cybersecurity for Critical National Infrastructure
- Cybersecurity for Smart Agriculture
- Cybersecurity for Retail Industry
- Cybersecurity for Digital Twins
- Cybersecurity for Quantum Key Distribution
- Cybersecurity for Digital Healthcare
- Cybersecurity for Smart Logistics
- Cybersecurity for Wearable Devices
- Cybersecurity for Edge Computing
- Cybersecurity for Cognitive Computing
- Cybersecurity for Industrial IoT
- Cybersecurity for Intelligent Transportation Systems
- Cybersecurity for Smart Water Management Systems
- The rise of cyber terrorism and its impact on national security
- The impact of artificial intelligence on cyber security
- Analyzing the effectiveness of biometric authentication for securing data
- The impact of social media on cyber security and privacy
- The future of cyber security in the Internet of Things (IoT) era
- The role of machine learning in detecting and preventing cyber attacks
- The effectiveness of encryption in securing sensitive data
- The impact of quantum computing on cyber security
- The rise of cyber bullying and its effects on mental health
- Investigating cyber espionage and its impact on national security
- The effectiveness of cyber insurance in mitigating cyber risks
- The role of blockchain technology in cyber security
- Investigating the effectiveness of cyber security awareness training programs
- The impact of cyber attacks on critical infrastructure
- Analyzing the effectiveness of firewalls in protecting against cyber attacks
- The impact of cyber crime on the economy
- Investigating the effectiveness of multi-factor authentication in securing data
- The future of cyber security in the age of quantum internet
- The impact of big data on cyber security
- The role of cybersecurity in the education system
- Investigating the use of deception techniques in cyber security
- The impact of cyber attacks on the healthcare industry
- The effectiveness of cyber threat intelligence in mitigating cyber risks
- The role of cyber security in protecting financial institutions
- Investigating the use of machine learning in cyber security risk assessment
- The impact of cyber attacks on the transportation industry
- The effectiveness of network segmentation in protecting against cyber attacks
- Investigating the effectiveness of biometric identification in cyber security
- The impact of cyber attacks on the hospitality industry
- The future of cyber security in the era of autonomous vehicles
- The effectiveness of intrusion detection systems in protecting against cyber attacks
- The role of cyber security in protecting small businesses
- Investigating the effectiveness of virtual private networks (VPNs) in securing data
- The impact of cyber attacks on the energy sector
- The effectiveness of cyber security regulations in mitigating cyber risks
- Investigating the use of deception technology in cyber security
- The impact of cyber attacks on the retail industry
- The effectiveness of cyber security in protecting critical infrastructure
- The role of cyber security in protecting intellectual property in the entertainment industry
- Investigating the effectiveness of intrusion prevention systems in protecting against cyber attacks
- The impact of cyber attacks on the aerospace industry
- The future of cyber security in the era of quantum computing
- The effectiveness of cyber security in protecting against ransomware attacks
- The role of cyber security in protecting personal and sensitive data
- Investigating the effectiveness of cloud security solutions in protecting against cyber attacks
- The impact of cyber attacks on the manufacturing industry
- The effective cyber security and the future of e-votingness of cyber security in protecting against social engineering attacks
- Investigating the effectiveness of end-to-end encryption in securing data
- The impact of cyber attacks on the insurance industry
- The future of cyber security in the era of artificial intelligence
- The effectiveness of cyber security in protecting against distributed denial-of-service (DDoS) attacks
- The role of cyber security in protecting against phishing attacks
- Investigating the effectiveness of user behavior analytics
- The impact of emerging technologies on cyber security
- Developing a framework for cyber threat intelligence
- The effectiveness of current cyber security measures
- Cyber security and data privacy in the age of big data
- Cloud security and virtualization technologies
- Cryptography and its role in cyber security
- Cyber security in critical infrastructure protection
- Cyber security in the Internet of Things (IoT)
- Cyber security in e-commerce and online payment systems
- Cyber security and the future of digital currencies
- The impact of social engineering on cyber security
- Cyber security and ethical hacking
- Cyber security challenges in the healthcare industry
- Cyber security and digital forensics
- Cyber security in the financial sector
- Cyber security in the transportation industry
- The impact of artificial intelligence on cyber security risks
- Cyber security and mobile devices
- Cyber security in the energy sector
- Cyber security and supply chain management
- The role of machine learning in cyber security
- Cyber security in the defense sector
- The impact of the Dark Web on cyber security
- Cyber security in social media and online communities
- Cyber security challenges in the gaming industry
- Cyber security and cloud-based applications
- The role of blockchain in cyber security
- Cyber security and the future of autonomous vehicles
- Cyber security in the education sector
- Cyber security in the aviation industry
- The impact of 5G on cyber security
- Cyber security and insider threats
- Cyber security and the legal system
- The impact of cyber security on business operations
- Cyber security and the role of human behavior
- Cyber security in the hospitality industry
- The impact of cyber security on national security
- Cyber security and the use of biometrics
- Cyber security and the role of social media influencers
- The impact of cyber security on small and medium-sized enterprises
- Cyber security and cyber insurance
- The impact of cyber security on the job market
- Cyber security and international relations
- Cyber security and the role of government policies
- The impact of cyber security on privacy laws
- Cyber security in the media and entertainment industry
- The role of cyber security in digital marketing
- Cyber security and the role of cybersecurity professionals
- Cyber security in the retail industry
- The impact of cyber security on the stock market
- Cyber security and intellectual property protection
- Cyber security and online dating
- The impact of cyber security on healthcare innovation
- Cyber security and the future of e-voting
- Cyber security and the role of open source software
- Cyber security and the use of social engineering in cyber attacks
- The impact of cyber security on the aviation industry
- Cyber security and the role of cyber security awareness training
- Cyber security and the role of cybersecurity standards and best practices
- Cyber security in the legal industry
- The impact of cyber security on human rights
- Cyber security and the role of public-private partnerships
- Cyber security and the future of e-learning
- Cyber security and the role of mobile applications
- The impact of cyber security on environmental sustainability
- Cyber security and the role of threat intelligence sharing
- Cyber security and the future of smart homes
- Cyber security and the role of cybersecurity certifications
- The impact of cyber security on international trade
- Cyber security and the role of cyber security auditing
About the author
Muhammad Hassan
Researcher, Academic Writer, Web developer
You may also like

200+ Funny Research Topics

500+ Sports Research Topics

500+ Environmental Research Topics

500+ Economics Research Topics

500+ Physics Research Topics

500+ Google Scholar Research Topics
A systematic literature review of cyber-security data repositories and performance assessment metrics for semi-supervised learning
- Open access
- Published: 06 April 2023
- Volume 1 , article number 4 , ( 2023 )
Cite this article
You have full access to this open access article
- Paul K. Mvula nAff1 ,
- Paula Branco na1 nAff1 ,
- Guy-Vincent Jourdan na1 nAff1 &
- Herna L. Viktor na1 nAff1
3434 Accesses
5 Citations
Explore all metrics
In Machine Learning, the datasets used to build models are one of the main factors limiting what these models can achieve and how good their predictive performance is. Machine Learning applications for cyber-security or computer security are numerous including cyber threat mitigation and security infrastructure enhancement through pattern recognition, real-time attack detection, and in-depth penetration testing. Therefore, for these applications in particular, the datasets used to build the models must be carefully thought to be representative of real-world data. However, because of the scarcity of labelled data and the cost of manually labelling positive examples, there is a growing corpus of literature utilizing Semi-Supervised Learning with cyber-security data repositories. In this work, we provide a comprehensive overview of publicly available data repositories and datasets used for building computer security or cyber-security systems based on Semi-Supervised Learning, where only a few labels are necessary or available for building strong models. We highlight the strengths and limitations of the data repositories and sets and provide an analysis of the performance assessment metrics used to evaluate the built models. Finally, we discuss open challenges and provide future research directions for using cyber-security datasets and evaluating models built upon them.
Similar content being viewed by others
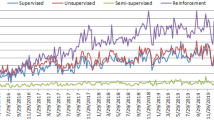
Machine Learning: Algorithms, Real-World Applications and Research Directions
Iqbal H. Sarker
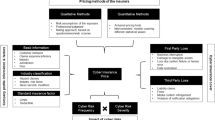
Cyber risk and cybersecurity: a systematic review of data availability
Frank Cremer, Barry Sheehan, … Stefan Materne
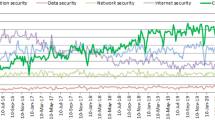
AI-Driven Cybersecurity: An Overview, Security Intelligence Modeling and Research Directions
Iqbal H. Sarker, Md Hasan Furhad & Raza Nowrozy
Avoid common mistakes on your manuscript.
1 Introduction
As a result of the significant technological advancements made throughout the years, people’s lifestyles are shifting from traditional to more electronic. This shift has resulted in an increase in cybercrimes on the Internet. Therefore, adequate measures have to be put in place to secure computer systems. Moreover, computer security or cyber-security systems must be capable of detecting and preventing cyber-attacks in real-time. The intersection of the Machine Learning (ML) and cyber-security fields has recently been rapidly growing as researchers make use of either fully labelled datasets with Supervised Learning (SL), unlabeled datasets with Unsupervised Learning (UL) or combining labelled and unlabeled data with Semi-Supervised Learning (SSL) to identify the various types of cyber-attacks. Due to the high cost and scarcity of labelled data in the cyber-security domain, SSL applications for cyber-security tasks have gained traction. Several datasets have been made available to the public to build ML-based defensive mechanisms. In ML, the quality of the output is determined by the quality of the input [ 1 ]; in other words, for ML models to generalize effectively, the datasets upon which they are built must be representative of real-world data. Therefore, surveys on the available datasets and performance evaluation metrics used to build and evaluate SSL models are required to give up-to-date information on recent cyber-security datasets and suitable performance metrics used in SSL frameworks to provide a starting point for new researchers who wish to investigate this vital subject.
Several works focusing on cyber-security provide discussions of datasets and data repositories that can be used for building ML models. For instance, Ring et al. [ 2 ] presented an extensive survey on network-based intrusion detection datasets discussing datasets containing packet-based, flow-based and neither packet- nor flow-based data while Glass-Vanderlan et al. [ 3 ] focused on Host-Based Intrusion Detection Systems (HIDS) and touched upon datasets and sources mainly related to HIDS. Other articles described datasets for (i) intrusion, malware and spam detection (e.g. [ 4 , 5 , 6 , 7 , 8 ]); (ii) network anomaly detection (e.g. [ 9 ]); or (iii) phishing URL detection (e.g. [ 10 ]). However, these works often focus on a particular cyber-security domain and do not examine in detail the characteristics of the available datasets and the performance evaluation metrics that are suitable for the various research challenges.
Because of the expanding interest in this area and the rapid speed of research, these surveys quickly become outdated; there is, therefore, an obvious need for a comprehensive survey to present the most recent datasets and evaluation metrics and their usage in the literature. To fill this gap, we present an exhaustive evaluation of the cyber-security datasets used to build SSL models. In this paper, we conduct a systematic literature review (SLR) of publicly available cyber-security datasets and performance assessment metrics used for building and evaluating SSL models. To this end, we provide a summary of datasets used to construct models for cyber-security-related tasks; the covered areas include not only network- and host-based intrusion detection, but also spam and phishing detection, Sybil and botnet detection, Internet traffic and domain name classification, malware detection and categorization, and power grid attacks detection. Additionally, we examine the performance assessment metrics used to evaluate the SSL models and discuss their usage in the selected papers. Furthermore, we provide a list of datasets, tools, and resources used to collect and analyze the data that have been made publicly available in the literature. Finally, we provide a discussion on the open research challenges and a list of observations with regard to datasets and performance metrics. This is, to the best of our knowledge, the first SLR analyzing a wide array of cyber-security datasets and performance evaluation metrics for SSL tasks, as well as providing easy access to publicly available datasets.
Our key contributions are the following:
We provide a description of the most commonly used SSL techniques.
We provide insights on the major cybercrimes for which SSL solutions have been explored.
We present a systematic literature review of the publicly available cyber-security datasets, repositories and performance evaluation metrics used.
We analyze the open challenges found in the literature and provide a set of recommendations for future research.
The remaining sections are organized as follows. Section 2 presents the definitions, important concepts, and basic assumptions of SSL, as well as a brief introduction to the methods utilized in the literature we reviewed and an overview of the different cybercrimes the included articles’ authors propose to counter. Additionally, we provide examples that highlight successful industrial deployments of ML for countering cyber threats, demonstrating the practical applications of the methods discussed in the literature. In Sect. 3 , we present the methodology we used to construct our survey and in Sect. 4 , an in-depth analysis of the publicly available datasets and the different evaluation metrics used in the selected papers is presented. Section 5 discusses the open challenges faced by the reviewed methods applying SSL for cyber-security, with respect to the datasets and evaluation metrics, presents a set of observations and the lessons learned, and highlights strategies for bridging the gap between research and practice. Finally, Sect. 6 concludes the work.
2 Background on SSL and cyber-security
Machine Learning (ML), the core subset of Artificial Intelligence (AI), may be defined as the systematic study of computer algorithms and systems that allow computer programs to automatically improve their knowledge or performance through experience [ 11 ]. It is a branch of computer science where the goal is to teach computers with sample data, i.e., training data, to make predictions or decisions on unseen data. ML algorithms can be categorized into three main types: SL, UL, and Reinforcement Learning (RL). In SL, the task, i.e., the inference of the function to map input data points from an instance space to their corresponding labels in the output space using labelled examples [ 12 , 13 ], can either be classification where the function being learned is discrete, i.e., input data points in the input space are mapped to categorical values, or regression where the function being learned is continuous, i.e., input data points are mapped to real values. In contrast to SL, in UL, there are no labels available, therefore the goal of UL algorithms is to capture important patterns or extract relationships from untagged (unlabeled) data as probability density distributions [ 14 ] and in RL, the algorithms’ goal is to attempt to maximize the feedback (reward) they are provided with. SSL conceptually stands between SL and UL, [ 15 , 16 , 17 ]. Out-of-core Learning (OL), or Incremental, or Online Learning, is a learning technique where the data becomes available in a sequential, one at a time, manner [ 18 ]. In OL, the model can learn from newly available data, in addition to making predictions from it. Information Technology (IT) security, Computer security or simply cyber-security is the protection of computer systems and networks from cyber-attacks, i.e., information disclosure, loss, theft, or damage to their hardware, software, or electronic data, as well as from the disruption or misdirection of the services they offer [ 19 ].
SSL and ML, in general, have brought significant benefits to the cyber-security domain, including improved detection capabilities, adaptive learning, automation, and threat intelligence [ 20 ] (see Sect. 2.3 for industrial examples). However, there are also challenges that need to be addressed, including the lack of quality data, adversarial attacks, model explainability, and bias and discrimination [ 21 , 22 ]. Addressing these challenges will be critical to ensuring that ML remains a useful tool in the fight against cyber threats.
In the remainder of this section, we introduce the key principles and techniques of SSL, provide a summary of cybercrimes examined in the literature, and present examples that demonstrate the potential of ML in mitigating cyber threats in the real world.
2.1 SSL concepts and methods
We will first introduce some notations. Let \(\mathcal {D}_L=(x_i,l(x_i ))_{i=1}^k\) denote a labelled dataset where each sample \((x_i,l(x_i))\) consists of data point \(x_i\) from the instance space \(\mathcal {X}\) and a target variable \(l(x_i)\) in the output space \(\mathcal {Y}\) . Let \(\mathcal {D}_U=(x_i)_{i=k+1}^{k+u}\) denote an unlabeled dataset. In SL, when \(l(x_i)\) consists of categorical values we face a classification task and when it consists of real values we have a regression task. In UL, the model is only provided with unlabeled data, i.e., \(\mathcal {D}_U\) . SL can build strong models to predict labels for unlabeled samples, but it requires \(\mathcal {D}_L\) to contain diverse samples manually labelled by domain experts, which may not only be too costly but may also contain inaccurate labels due to human mistakes. Therefore, in practice, \(u \gg k\) . On the other hand, even though UL does not require labelled samples to infer patterns, it is prone to overfitting. SSL makes use of both \(\mathcal {D}_L\) and \(\mathcal {D}_U\) to infer a function whose performance surpasses one built with either SL or UL by making use of at least one of the main learning assumptions, i.e., smoothness, low-density, manifold, [ 23 ], and cluster, [ 24 ], assumption.
The smoothness assumption is based on the notion that if two data points, \(x_1\) and \(x_2\) , lie close in the instance space, \(\mathcal {X}\) , their corresponding class labels, \(l(x_1)\) and \(l(x_2)\) , should also be close (the same), in the output space \(\mathcal {Y}\) ; the transitivity assumption, that states that if \(x_1\) lies close to \(x_2\) and \(x_2\) lies close to \(x_3\) , then \(x_1\) lies transitively close to \(x_3\) , is an important idea in the smoothness assumption because “close points in \(\mathcal {X}\) have the same label,” thus this assumption implies that if \(x_2\) is a noisy version of \(x_1\) , they should still have the same predicted label. In the low-density assumption, it is implied that data points with the same label are clustered in high-density sections of the instance space, i.e., the decision boundary must pass through a low-density region, \(\mathcal {R} \subset \mathcal {X}\) , and the probability of any data point, \(p(x_i)\) , being in the low-density region is low, i.e., \(p(x_i)\) in \(\mathcal {R}\) is low. This also verifies that the smoothness assumption is satisfied. In the manifold assumption, the instance space, \(\mathcal {X}\) , consists of one or more Riemannian manifolds \(\mathcal {M}\) on which samples share the same label. According to the cluster assumption, which can be seen as a generalization of the other three assumptions mentioned earlier [ 16 ], if data points are in the same cluster, they are likely to share the same label, and there may be several clusters constituting the same class [ 15 ].
Based on [ 16 , 25 , 26 ], the taxonomy in Fig. 1 provides a general overview of the SSL approaches which will be described in more detail in Sects. 2.1.1 and 2.1.2 . An overview of the key concepts in the taxonomy is presented next.
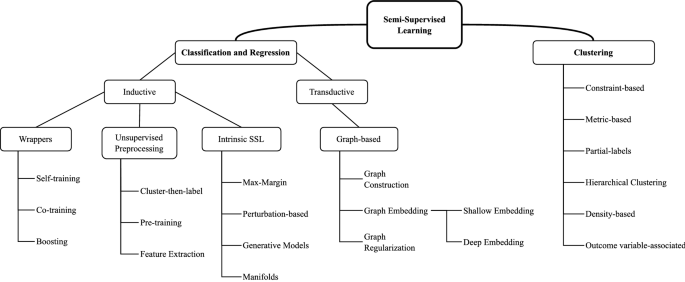
Taxonomy of SSL techniques (adapted from [ 16 , 25 , 26 ])
SS Classification and Regression methods can either be transductive or inductive [ 15 , 27 , 28 ]. In inductive SSL, the model is first built using information from \(\mathcal {D}_L\) and \(\mathcal {D}_U\) and it can then be used as one built with SL to generate predictions for previously unseen, unlabeled samples; there exists a clear distinction between a training phase and a testing phase. In transductive SSL, on the other hand, the goal is to generate labels for the unlabeled samples fed to the learner, therefore there is no clear distinction between a training and testing phase. Frequently, transductive approaches create a graph across all data points, including labelled and unlabeled, expressing the pairwise similarity of data points with weighted edges and are incapable of handling additional unseen data [ 17 ]. We group both SS Classification and Regression because they predict output values for input samples but note that most SS Classification approaches are incompatible with SS Regression, and we, therefore, specify when they may be compatible in Sect. 2.1.1 .
In the SS Clustering assumption, the learner’s goal is clustering but a small amount of knowledge is available in the form of constraints, must-link constraints (two samples must be within the same cluster) and cannot-link constraints (two data points cannot be within the same cluster). It differs from traditional clustering in the way the constraints are accommodated: either by biasing the search for relevant clusters or altering a distance or similarity metric [ 29 ]. When it is not possible for an SL method to work, even in a transductive form, because the available knowledge is too far from being representative of a target classification of the items, the cluster assumption may allow the use of the available knowledge to guide the clustering process [ 30 ]. Bair [ 25 ] provides a survey on SS Clustering methods and groups them into constraint-based, partial-labels, SS hierarchical clustering and outcome variable associated methods.
A plethora of SSL approaches have been proposed in the literature, each making use of at least one of the SSL assumptions described. The following sections briefly describe the frequently used SSL methods showing how they relate to the SSL assumptions.
2.1.1 SSL for classification and regression
We divide the classification and regression methods between the two main classes: inductive SSL and transductive SSL.
2.1.1.1 Inductive methods
The goal of inductive methods is to build a model from labelled and unlabeled data and use the model as a built-in SL (only with labelled data) to make predictions on unlabeled data. Inductive methods can further be divided into wrapper methods, unsupervised preprocessing, and intrinsically semi-supervised methods. In wrapper methods, one or more supervised-based learners are first trained based on the labelled data only, then the learner or set of learners are applied to the unlabeled data to generate pseudo-labels which are used for training in the next iterations. Pseudo-labels, \(l(x_i)\) , \(k<i<k+u\) , are simply the most confident labels produced by the learner or set of learners for a set of unlabeled samples, \(\mathcal {X}_U \subset \mathcal {D}_U\) , [ 31 ]. The wrapper methods we will consider are self-training and co-training. According to the way they make use of the unlabeled data, unsupervised preprocessing methods can be divided into feature extraction, unsupervised clustering and parameter initialization or pre-training.
2.1.1.1.1 Wrapper methods In wrapper methods, a model is first trained on labelled data to generate pseudo-labels for an unlabeled subset, \(\mathcal {X}_U \subset \mathcal {D}_U\) , then the model is iteratively re-trained, until all unlabeled data are labelled or some stopping criterion is met, with a new dataset containing both the labelled dataset, \(\mathcal {D}_L\) , and the pseudo-labels, \(l{(x_i)}\) , \(k<i<k+u\) , of the subset \(\mathcal {X}_U\) , generated in previous iterations. They are the most well-known and oldest SSL methods [ 27 , 31 ]. Wrapper methods may be used for classifictaion and regression and are divided into three categories: self-training, co-training, and boosting.
Self-training. Self-training [ 32 ] also referred to as self-learning, are wrapper methods that consist of a single base SL learner that is iteratively trained on a training set consisting of the original labelled data and the high-confidence predictions, pseudo-label, from the previous iterations. They are the most basic wrapper methods [ 31 ] and may be applied to most, if not all, SL algorithms such as Random Forests (RF) [ 33 ], Support Vector Machines (SVM) [ 34 ], etc.
Co-training. Co-training methods, [ 35 , 36 ], assume that (i) features can be split into two or more distinct sets or views; (ii) each feature subset is sufficient to train a good classifier; (iii) the views are conditionally independent given the class label. Co-training extends the principle of self-training to multiple SL learners that are each iteratively trained with the pseudo-labels from the other learners, in other words, learners “teach” each other with the added pseudo-labels to improve global performance. For co-training to work well, the sufficiency (ii) and independence (iii) assumptions should be satisfied [ 35 ]. Multi-view co-training, the basic form of co-training, constructs two learners on distinct feature sets or views. When no natural feature split is known a priori, single-view co-training may be used to build two or more weak learners with different hyper-parameters on the same feature set. There exist several approaches based on single-view co-training such as tri-training [ 37 ], co-forest [ 38 ], co-regularization [ 39 ], etc. In co-regularization, the two terms of the objective function minimize the error rate and optimize the disagreement between base learners [ 39 ].
2.1.1.1.2 Unsupervised preprocessing The unsupervised preprocessing methods use \(\mathcal {D}_U\) and \(\mathcal {D}_L\) at two different steps. The first step often consists of extraction (feature extraction) or transformation (unsupervised clustering) of the feature space or for initialization of a model’s parameters (pre-training) while the second step consists of using knowledge from \(\mathcal {D}_L\) to label the unlabeled data points in \(\mathcal {D}_U\) . We briefly describe the methods in the next points.
Feature Extraction: Feature extraction is one of the most critical steps to take in ML. It consists of extracting a set of relevant features for ML models to work. Typically, SSL feature extraction methods consist of either finding lower-dimensional feature spaces, from \(\mathcal {X}\) , without sacrificing significant amounts of information or finding lower-dimensional vector representations of highly dimensional data objects by considering the relationships between the inputs. Examples of SSL feature extraction methods are autoencoder (AE) [ 14 ] and a few of its variants, such as denoising autoencoder [ 40 ] and contractive autoencoder [ 41 ], and methods in NLP (Natural Language Processing) such as Word2Vec [ 42 ], GloVe [ 43 ], etc.
Unsupervised clustering: Also referred to as cluster-then-label methods, these methods explicitly join the SL or SSL classification or regression algorithms and UL or SSL clustering algorithms. The UL or SSL clustering algorithm first clusters all the data points, then those clusters are fed to the SL or SSL classifier or regressor for label inference [ 44 , 45 , 46 ].
2.1.1.1.3 Intrinsically semi-supervised Intrinsically semi-supervised methods are typically extensions of existing SL methods to directly include the information from unlabeled data points in the loss function. Regarding the SSL assumption they rely on, these methods can be further grouped into four categories: (i) maximum-margin methods, where the goal is to maximize the distance between data points and the decision boundary (low density-assumption), (ii) perturbation-based methods, often implemented with neural networks (NN), rely directly on the smoothness assumption (a noisy, or perturbated, version of a data point should have the same predicted label, as the original data point), (iii) manifold-based methods either explicitly or implicitly estimate the manifolds on which the data points lie and (iv) generative models whose primary goal is to infer a function that can generate samples, similar to the available samples, from random noise.
2.1.1.2 Transductive methods
A learner is said to be transductive if it only works on the labelled and unlabeled data available at training and cannot handle unseen data [ 17 ]. The goal of a transductive learner is to infer labels for an unlabeled dataset \(\mathcal {D}_U\) , using \(\mathcal {D}_L\) . If a new unlabeled data point, \(x_u \notin \mathcal {D}_U\) , is given, the learner must be reapplied, from scratch to all the data, i.e., \(\mathcal {D}_L\) , \(\mathcal {D}_U\) , and \(x_u\) . Graph-based methods, which are often transductive in nature, define a graph where the nodes are labelled and unlabeled samples in the dataset, and edges (weighted) reflect the similarity of the samples. These methods usually assume label smoothness over the graph. Graph methods are non-parametric and discriminative [ 17 ]. The defined loss function is optimized to achieve two goals: (i) for already labelled samples, from \(\mathcal {D}_L\) , the inferred labels should correspond to their true labels and (ii) the predicted labels of similar samples on the graph be the same. A transductive learner’s task may be classification or regression.
2.1.2 SSL for clustering
Semi-supervised clustering methods can be used with partially labelled data as well as other types of outcome measures. When cluster assignments, or partial labels, for a subset of the data, are known beforehand, the objective is to classify the unlabeled samples using the known cluster assignments [ 47 ], this is, in a sense, equivalent to an SL problem. When more complex relationships among the samples are known in the form of constraints, the problem becomes a generalization of the previous objective and is either called constrained clustering [ 48 ], i.e., an existing clustering method is modified to satisfy the constraints, or distance-based (metric-based) clustering, i.e., an alternative distance metric is used to satisfy the constraints [ 49 , 50 ].
Hierarchical and partitional clustering techniques are the two main types of clustering algorithms. Hierarchical clustering methods recursively locate nested clusters in either agglomerative or divisive mode. In agglomerative mode, they start with each data point in its own cluster and merge the most similar clusters successively to form a cluster hierarchy and in divisive or top-down mode, they start with all the data points in one cluster and recursively divide each cluster into smaller clusters [ 51 ]. SS Hierarchical clustering methods group samples using a tree-like architecture, known as a hierarchy. They either built separate hierarchies for must-link and cannot-link constrained samples [ 52 , 53 , 54 , 55 ] or use other types of constraints [ 56 , 57 , 58 , 59 , 60 ]. Finally, SS Clustering may be used to build clusters related to a given outcome variable [ 61 ].
We refer the interested reader to [ 16 , 25 , 26 , 29 , 62 ] for detailed descriptions of the methods mentioned in this section.
2.2 Cybercrimes
As mentioned in Sect. 1 , a cyber-attack is any offensive maneuver that targets computer systems aiming at information disclosure, theft of or damage to their hardware, software, or electronic data, as well as from the disruption or misdirection of the services they provide, and cyber-security can be defined as the protection of computer systems against cyber-attacks [ 19 ]. Cybercrimes are criminal activities that involve the use of digital technologies such as computers, smartphones, the internet, and other digital devices [ 63 ]. From a legal perspective, cybercrimes can be defined as criminal offences that involve the use of a computer or a computer network [ 64 ]. The cyber-attacks covered in this article can all be seen as specific types of cybercrime, we, therefore, use the two terms interchangeably. Note that different jurisdictions may have different laws regarding what constitutes a cybercrime or cyber-attack. Therefore, an activity that is considered a cyber-attack in one jurisdiction may not be considered a cybercrime in another jurisdiction, depending on the specific laws in each location but cybercrimes typically involve the illegal or unauthorized use of digital technologies such as computers [ 63 , 64 ]. Additionally, some activities that are not considered cyber attacks in some jurisdictions may still be considered cybercrimes if they violate specific laws related to computer systems and networks [ 63 ]. Cybercrimes may also be viewed from technical [ 65 ] and procedural [ 66 , 67 ] perspectives.
The IBM X-Force Incident Response and Intelligence Services (IRIS) estimated the profit made by a group of attackers to be over US$123 million in 2020 [ 68 ] and the Cost of a Data Breach report published in 2021 by IBM Security estimates the global average cost per incident to US$4.24 million [ 69 ]. Cybercriminals are always taking advantage of catastrophes, disasters, and hot events for their own gains. A clear example is the surge in cybercrimes of all sorts witnessed at the beginning of the pandemic.
The following subsections briefly describe the cybercrimes countered in the covered literature.
2.2.1 Network intrusion
Any unlawful action on a digital network is referred to as network intrusion. Network intrusions or breaches can be thought of as a succession of acts carried out one after the other, each dependent on the success of the last. The stages of the intrusion are sequential, beginning with reconnaissance and ending with the compromising of sensitive data [ 70 ]. These principles are useful for managing proactive measures and finding bad actors’ behaviour. Network intrusions often include the theft of valuable network resources and virtually always compromise network and/or data security [ 71 , 72 ]. Living off the land, multi-routing, buffer overwriting, covert CGI scripts, protocol-specific attacks, traffic flooding, Trojan horse malware, and worms are the most frequent intrusion attacks.
Some intruders will attempt to implant code that cracks passwords, logs keystrokes, or imitates a website in order to lead unaware users to their own. Others will infiltrate the network and steal data on a regular basis or alter websites accessible to the public with a range of messages. Intruders may get access to a computer system in a number of ways, including internally, externally, or even physically.
2.2.2 Phishing
IBM X-Force identified phishing as one of the most used attack vectors in 2021 because of their ease of use and low resource requirements [ 73 ]. Phishing is a form of cybercrime where the attackers’ aim is to trick users into revealing sensitive data, including personal information, banking, and credit card details, IDs, passwords, and more valuable information via replicas of legitimate websites of trusted organizations. Phishing attacks can be grouped into deceptive phishing and technical subterfuge [ 74 ]. Deceptive phishing is often performed via emails, SMS, calendar invitations, using telephony, etc., and technical subterfuge is the act of tricking individuals into disclosing their sensitive information through technical subterfuge by downloading malicious code into the victim’s system. We refer the reader to a recent in-depth study on phishing attacks [ 74 ].
Spam, not to be mistaken for canned meat, may be defined as unsolicited and unwanted messages, typically sent in bulk, that can take several forms such as email, text messages, phone calls, or social media messages. The content of spam messages can vary widely, but they are often commercial in nature and aim to advertise a product or service or promote a fraudulent scheme or solicit donations [ 75 ].
2.2.4 Malware
Malware or malicious software is defined as any software that intentionally executes malicious payloads on victim machines (computers, smartphones, computer networks, and so on) to cause disruptions. There exist several varieties of malware, such as computer viruses, worms, Trojan horses, ransomware, spyware, adware, rogue software, wipers, and scareware. In the 2022 Threat Intelligence Index, IBM X-Force reported that ransomware, a type of malware, was again the top attack type in 2021, although decreasing from 23%, in 2020, to 21% [ 73 ]. Defensive tactics vary depending on the type of malware, but most may be avoided by installing antivirus software and firewalls, applying regular patches to decrease zero-day threats, safeguarding networks from intrusion, performing regular backups, and isolating infected devices.
2.2.5 Other cyber-attacks
In addition to intrusions, spam, phishing and malware, we also discuss SSL applications for:
Traffic classification - traffic classification may be used to detect patterns suggestive of denial-of-service attacks, prompt automated re-allocation of network resources for priority customers, or identify customer use of network resources that in some manner violates the operator’s terms of service [ 76 ];
Sybil detection —a Sybil attack may be defined as an attack against identity in which an individual entity masquerades as numerous identities at the same time [ 77 ];
Stock market manipulation detection —market manipulation may be defined as an illegal practice in an attempt to boost or reduce stock prices by generating an illusion of an active trading [ 78 , 79 ];
Social bot detection —a social bot may be defined as a social media account that is operated by a computer algorithm to automatically generate content and interact with humans (or other bot users) on social media, in an attempt to mimic and possibly modify their behaviour [ 80 , 81 ];
Shilling attack detection —a Shilling attack is a particular type of attack in which a malicious user profile is injected into an existing collaborative filtering dataset to influence the recommender system’s outcome. The injected profiles explicitly rate items in a way that either promotes or demotes the target items [ 82 ];
Pathogenic social media account detection —Pathogenic Social Media (PSM) accounts refer to accounts that have the capability to spread harmful misinformation on social media to viral proportions. Terrorist supporters, water armies, and fake news writers are among the accounts in this category [ 83 , 84 ];
Fraud detection —in the banking industry such as credit card fraud detection. Credit card fraud may happen when unauthorized individuals obtain access to a person’s credit card information and use it to make purchases, other transactions, or open new accounts [ 85 ]; and
Detection of attacks on other platforms such as the power grid - the smart grid enables energy customers and providers to manage and generate electricity more effectively. The smart grid, like other emerging technology, raises new security issues [ 86 ].
2.3 Examples of industry deployments of ML in cyber-security
This section presents examples of successful industrial deployments of ML for countering cyber threats. The first example is “IBM X-Force Threat Management” [ 87 ], an ML platform deployed to counter cyber threats. IBM X-Force Threat Management is a cloud-based security platform that leverages ML to provide advanced threat detection and response capabilities. It analyzes massive amounts of security data, including network traffic, system logs, and user behaviour, to identify and respond to potential threats in real-time using ML algorithms. The ML models are trained on large datasets of historical security events, allowing the system to learn and adapt to new threats over time. Depending on the use case and data available, it is possible that IBM X-Force Threat Management may use a combination of ML techniques, such as SSL and Reinforcement Learning, in addition to other optimization methods for enhancing security policies. However, it should be noted that without specific information from IBM, it cannot be definitively confirmed whether these techniques are actually employed. Nonetheless, the platform has demonstrated success in detecting various types of cyber threats, including banking Trojans such as IcedID, Footnote 1 TrickBot and QakBot.
The second example is the Deep Packet Inspection (DPI) system developed by Darktrace, a cyber-security company. The system uses unsupervised ML algorithms to learn the expected behaviour of a network and detect anomalies that may indicate malicious activity. The system can also automatically respond to detected threats by initiating a range of actions, such as quarantining a device or blocking network traffic. Darktrace has deployed its DPI system in various industries, including healthcare, finance, and energy. In one instance, a UK construction company used the system to detect and respond to a ransomware attack. Footnote 2 The system identified the attack within minutes of it starting and initiated a range of responses, including blocking the attacker’s IP address and quarantining affected devices. The company was able to contain the attack and avoid paying the ransom demanded by the attackers.
Our third example is Feedzai, an ML platform that provides fraud prevention and anti-money laundering for financial institutions and businesses. Feedzai employs a variety of ML techniques, including Deep Learning and combining SL and UL (SSL), Footnote 3 to detect and prevent fraudulent activity in real-time. After partnering with a large European bank, Feedzai’s platform reduced false positives and accurately identified fraudulent activity, resulting in lower losses due to fraud. Footnote 4
Overall, IBM X-Force Threat Management, Darktrace, and Feedzai demonstrate how ML can be successfully deployed in the industry to counter cyber threats and provide advanced threat detection and response capabilities.
3 Review methodology
This section provides the details of the methodology we followed. To achieve our goal of reviewing the datasets and evaluation metrics used in the applications of SSL techniques to cyber-security, we followed the standard systematic literature review guidelines outlined in [ 88 ] for assessing the search’s completeness. The entire process was done on Covidence [ 89 ], an online tool for systematic review management and production. We first defined our three research questions shown below. These are motivated by the need to examine the efforts being made to safeguard users and computer systems against attacks using SSL. This stems from the fact that attacks are far more harmful than vulnerability scans or related operations. We intend to review the datasets as well as the evaluation metrics used in the literature identifying the cyber-attacks as soon as possible to take the necessary actions to reverse them.
With the introduction and use of SSL in cyber-security, what are the assessment metrics used to evaluate the built models?
What datasets are the proposed SSL approaches built upon? What are the most used datasets?
What are the open challenges with respect to the datasets and performance assessment metrics?
Our inclusion and exclusion criteria were then defined from the above research questions. A paper is included if it directly applies SSL for detecting at least one of the cyber-attacks mentioned in Sect. 2.2 . with enough details to address our research questions. On the other hand, a paper is excluded if (i) another paper of the same authors superseded the work, in which case the latest work is considered, (ii) it does not use SSL for the inclusion criteria and (iii) the approach is discussed at a high level, with insufficient information to fulfill the research questions. The entire process was done on Covidence [ 89 ], an online tool for systematic review management and production. We then queried IEEE Xplore and ACM Digital Library for articles having (“semi-supervised learning” AND “cyber-security”), (“semi-supervised” AND “cyber-security”) and (“semi-supervised” AND “security”) anywhere within the article.
The keywords (“semi-supervised learning” AND “cyber-security”) have been chosen because SSL has been increasingly used in cyber-security to improve the accuracy of detection and classification systems [ 90 ]. This combination has been used to find articles that specifically focus on using SSL in cyber-security tasks such as intrusion detection, malware detection, network traffic analysis, etc. Similarly, the combination of (“semi-supervised” AND “cyber-security”) has been used to find articles that discuss semi-supervised learning in a cyber-security context, even if they do not explicitly mention the phrase “semi-supervised learning”. Finally, the combination (“semi-supervised” AND “security”) has been used to broaden the search beyond just cyber-security and potentially include other domains where SSL has been applied to security-related tasks.
Note that we did not limit the search to the title, abstract or keywords because it was essential to making sure to find all the articles discussing and applying SSL methods for cyber-security for screening. The reason we chose these databases is that they are among the top databases suggested by our university library for conducting Computer Science research and they also contain papers published in top-tier venues. To complement the results obtained from IEEE Xplore and ACM Digital Library, we submitted the same search queries to Google Scholar and extracted the top 200 search results sorted by relevance. The combinations mentioned earlier and this search strategy allowed us to find articles that are relevant to using SSL in cyber-security, and gain a better understanding of how it is being/has been used to improve security systems.
As seen in Fig. 2 , in total, 1914 studies were imported for screening; 267 duplicates were automatically removed, and the remaining 1647 studies’ titles and abstracts were manually screened for relevance. Based on our inclusion and exclusion criteria, 1319 studies were found irrelevant, because they either did not discuss SSL methods or cyber-attack defences. The remaining 328 studies’ full texts were further assessed as they were either partially or fully related to our inclusion criteria, and finally, 210 relevant studies were included for data extraction. Furthermore, we used state-of-the-art surveys and review articles on SSL [ 16 , 27 ] and ML for cyber-security [ 4 ] to construct this extensive review of cyber-security datasets and performance evaluation metrics for SSL models.
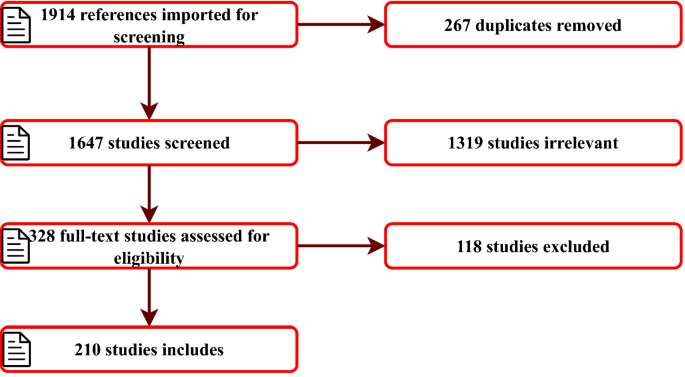
Review methodology
4 Datasets and performance assessment metrics
In this section, we summarize and analyze the public datasets and performance assessment metrics used in the selected papers.
4.1 Datasets and repositories
AI, especially ML, has proven itself a particularly useful tool in cyber-security as well as other fields of computer science and has extensively featured in the literature for cybercrime or malicious activity detection. “Cost of a Data Breach” [ 69 ], published by IBM Security, reported a US$3.81 million, or almost 80% difference between breach costs of companies with fully deployed security AI/ML and automation and companies without security AI/ML and automation. We present the public datasets used in the covered literature in this section, grouped by type of attack and show their usage in the selected papers in Figs. 3 , 4 , 5 , and 6 . Note that we acknowledge the difference between Spam and Phishing in Subsections 2.2.3 and 2.2.2 as they are different attack vectors but due to the scarcity of these datasets, we have combined them in a single section.
4.1.1 Network intrusion datasets and sources
In terms of network intrusion, we found a total of 18 public datasets and sources in the papers we reviewed. We begin by providing a brief description of each dataset; we, then, provide a summary of their main characteristics as well as some key data usage statistics.
KDD’99 and NSL-KDD . The KDD’99 dataset is a statistically preprocessed dataset which has been available since 1999 from DARPA [ 91 ], it is an updated version of the DARPA98. It is the most used dataset in the selected papers. The dataset has three components, basic, content and traffic features, making a total of 41 features for normal and simulated attack traffic. The NSL-KDD dataset, proposed by Tavallaee [ 92 ], is a version of the KDD’99 dataset in which redundant records are removed to enable the classifiers to produce unbiased results. The two datasets contain various attack types such as Neptune-DoS, pod-DoS, Smurf-DoS, and buffer-overflow. Table 1 gives a brief composition of the KDD’99 and NSL-KDD datasets.
Moore Set . The Moore Set [ 93 ] was prepared in 2005 by researchers at Intel Research. It comprises real-world traces collected by the high-performance network monitor. Each object in the Moore set represents a single flow of TCP packets between client and server, which consists of 248 characteristics. The information in the features is derived using packet header information alone, while the classification- class has been derived using content-based analysis. Table 2 shows a brief composition of the Moore Set.
LBNL2005. The Lawrence Berkeley National Laboratory (LBNL) 2005 traffic traces were collected at the LBNL/ICSI under the Enterprise Tracing Project over a period of three months in 2004 and 2005 on two routers [ 94 ]. It contains full header network traffic recorded at a medium-sized enterprise covering 22 subnets and includes trace data for a wide range of traffic including web, email, backup, and streaming media. Because the traffic traces are completely anonymized, all the packets do not have a payload. As seen in Table 3 , the LBNL trace consists of five datasets labelled: D0–D4. The “Per Tap” row specifies the number of traces collected on each monitored router port while the “Snaplen” row gives the maximum number of bytes recorded for each packet.
CAIDA Datasets . The Centre for Applied Internet Data Analysis (CAIDA), based at the University of California’s San Diego Supercomputer Center, collects a variety of data from geographically and topologically diverse locations and makes it available to the research community to the extent possible while respecting the privacy of individuals and organizations who donate data or network access. The CAIDA-DDoS Dataset [ 95 ], comprises approximately one hour of anonymized traffic from a DDoS attack on August 4, 2007 (20:50:08 UTC to 21:56:16 UTC). This type of denial-of-service attack tries to prevent access to the targeted server by using all of the server’s computational power and all of the bandwidth on the network linking the server to the Internet. The traces only include attack traffic to the victim and responses to the attack from the victim. Non-attack traffic has been eliminated to the greatest extent practicable.
Kyoto2006+ . The Kyoto2006+ is a publicly available benchmark dataset, consisting of 24 statistical features, that is built on three years of network traffic, from November 2006 to August 2009 [ 96 ]. It covers both regular servers and honeypots deployed at Kyoto University in Japan labelled as normal (no attack), attack (known attack) and unknown attack. It includes a variety of attacks performed against the honeypots such as shellcode, exploits, DoS, port scans, backscatter, and malware, shown in Table 4 . An updated version of the dataset contains additional data collected from November 2006 to December 2015 [ 97 ].
UNIBS2009 . The UNIBS-2009 trace [ 98 ], was compiled by the University of Brescia in 2009. It consists of traffic traces collected by running Tcpdump on the edge router of the university’s campus network on three consecutive working days (2009.9.30, 2009.10.1 and 2009.10.02) connecting the network to the Internet through a 100 Mbps uplink. As shown in Table 5 , the dataset supplies the true labels, and the traffic trace includes Web (HTTP and HTTPS), Mail (POP3, IMAP4, SMTP and their Secure Sockets Layer variants), Skype, P2P (BitTorrent, Edonkey), SSH (Secure Shell), FTP (File Transfer Protocol) and MSN.
UNB ISCX-2012 . The Installation Support Center of Expertise (ISCX)-2012 dataset has been prepared at the ISCX at the University of New Brunswick [ 99 ]. It is built on 7 days of network traffic, shown in Table 6 , and consists of over two million traffic packets characterized by 20 features taking nominal, integer, or float values. The dataset includes full packet payloads in pcap format.
CTU-13 . The CTU-13 dataset was compiled by the Czech Technical University [ 100 ]. It consists of botnet traffic captured in the university in 2011. The dataset includes thirteen scenarios, shown in Table 7 , covering different botnet attacks, that use a variety of protocols and performing different actions, mixed with normal traffic and background traffic. The dataset is available in the forms of unidirectional flow, bidirectional flow, and packet capture.
SCADA 2014 . The Supervisory Control And Data Acquisition (SCADA) [ 101 ] is a database proposed by Mississippi State University Key Infrastructure Protection Center in 2014 to evaluate the industrial network intrusion detection model. It is one of the standard databases in the current industrial control network intrusion detection commonly used in experiments. It includes the Gas system dataset and Water storage system dataset from the Industrial Control System network layer.
UNSW-NB15 . The UNSW-NB15 dataset was compiled in 2015 by the University of New South Wales Canberra at the School of Engineering and IT, UNSW Canberra at ADFA, using a small, emulated network over 31 h by getting normal and malicious raw network packets. It consists of nine attack types: analysis, backdoors, DoS, exploits, generic, fuzzers, reconnaissance, shell code and worms. It consists of over two million records each characterized by 49 features taking nominal, integer, or float values. The dataset’s data distribution is shown in Table 8 .
AWID 2015 . The Aegean Wi-Fi Intrusion Dataset (AWID), published in 2015 [ 102 ], comprises the largest amount of Wi-Fi network data (normal and attack) collected from real network environments. The 16 attack types can be grouped into flooding, impersonation, and injection. As seen in Table 9 , the dataset contains over 5 million samples each characterized by 154 features, representing the WLAN frame fields along with physical layer meta-data.
ISCXVPN2016 . The ISCXVPN2016 [ 103 ], published by the UNB in 2016, comprises traffic captured using Wireshark and tcpdump, generating a total amount of 28GB of data. For the VPN, an external VPN service provider connected to using OpenVPN (UDP mode) was used. To generate SFTP and FTPS traffic an external service provider and Filezilla as a client was used. Table 10 shows the data distribution in the ISCXVPN2016 dataset.
CIDDS . The Coburg Intrusion Detection Datasets (CIDDS), prepared at Coburg University of Applied Sciences (Hochschule Coburg), consist of several labelled flow-based datasets created in virtual environments using OpenStack. The CIDDS database’s most used dataset, CIDDS-001, released in 2017, covers four weeks of unidirectional traffic flows each characterized by 19 features taking nominal, integer, or float values. As seen in Table 11 , the dataset includes attacks such as DoS, port scan and SSH brute force.
CICIDS2017 . The Canadian Institute for Cyber-security Intrusion - Evaluation Dataset (CIC-IDS)-2017 was produced in an emulated network environment at the CIC [ 104 ]. It is built on 5 days (July 3 to July 7, 2017) of network traffic, shown in Table 12 , and includes a variety of most common attack types including FTP patator, SSH patator, DoS slowloris, DoS Slowhttptest, DoS Hulk, DoS GoldenEye, Heartbleed, Brute force, XSS, SQL Injection, Infiltration, Bot, DDoS (Distributed denial of service), and Port Scan each characterized by 80 features extracted using CICFlowMeter [ 103 , 105 ]. The dataset also includes full packet payloads in pcap format.
UGR’16 . The UGR’16 dataset, proposed in 2018 by Maciá-Fernández et al. [ 106 ], comprises NetFlow network traces collected from a real Tier 3 ISP network made up of several organizations’ and clients’ virtualized and hosted services including WordPress, Joomla, email, FTP, etc. NetFlow sensors were installed in the network’s border routers to capture all incoming and outgoing traffic from the ISP. As seen in Table 13 , two sets of data are provided: one for training models (calibration set) and the other for testing the models’ outputs (test set).
Kitsune2019 . The Kitsune Network Attack Dataset, Kitsune2019, has been prepared at Ben-Gurion University of the Negev, Israel and was released in May 2018 [ 107 ]. The dataset is composed of 9 files covering 9 distinct attacks situations on a commercial IP-based video surveillance system and an IoT network: OS (Operating System) Scan, Fuzzing, Video Injection, ARP Man in the Middle, Active Wiretap, SSDP Flood, SYN DoS, Secure Sockets Layer Renegotiation and Mirai Botnet. It contains 27,170,754 samples each characterized by 115 real features. The violation column in Table 14 indicates the attacker’s security violation on the network’s confidentiality (C), integrity (I), and availability (A).
NETRESEC is a software company that specializes in network security monitoring and forensics. They also maintain.pcap repository files gathered from various Internet sources [ 108 ]. It is a list of freely accessible public packet capture repositories on the Internet. Most of the websites listed on their website provide Full Packet Capture (FPC) files, however, others only provide truncated frames.
MAWI archive . The MAWI archive [ 109 ] consists of an ongoing collection of daily Internet traffic traces captured within the WIDE backbone network at several sampling points. Tcpdump is used to retrieve traffic traces, and the IP (Internet Protocol) addresses in the traces are encrypted using a modified version of Tcpdpriv (MAWI Working Group Traffic Archive ( http://www.wide.ad.jp )). The samplepoint-F consists of daily traces at the transit link of WIDE to the upstream ISP and has been in operation since 01/07/2006.
Kaggle Footnote 5 is an online data sharing and publishing platform. It includes security-based datasets such as KDD’99 and NSL-KDD. Registered users can also upload and explore data analysis models.
A breakdown of the usage of the Intrusion Detection datasets in the selected papers is shown in Fig. 3 , we also provide an overview of the Network Intrusion datasets in Table 15 . As seen in Fig. 3 , the KDD’99 dataset, despite being old and containing redundant and noisy records, is the most used of the 17 intrusion detection datasets described in this section. 45 out of the 100 selected papers used either the KDD’99 alone or in conjunction with some other intrusion detection dataset. This dataset is followed by the NSL-KDD dataset which is only a smaller version without the redundant and noisy records present in KDD’99. Additionally, none of these datasets are balanced, therefore suitable evaluation metrics should be used when evaluating models built on these datasets. We must highlight that the four most recent datasets used in the papers reviewed were already published in 2017 and 2018 and they have not been extensively explored in an SSL context. Finally, we refer the interested reader to a recent comprehensive survey of Network-based Intrusion datasets [ 2 ].
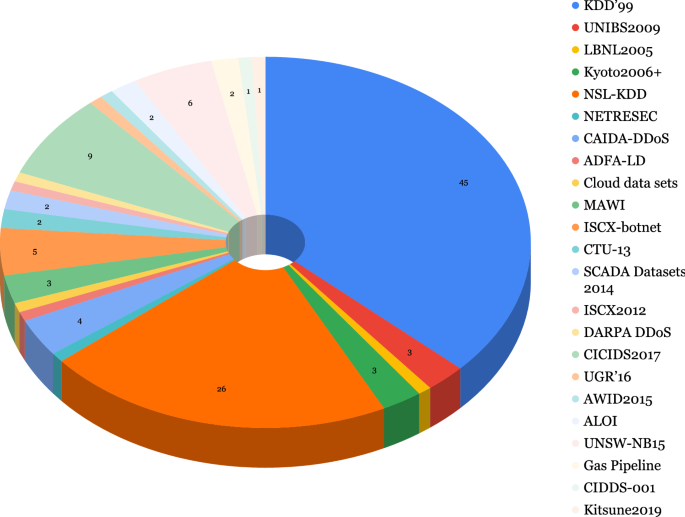
Usage of intrusion detection datasets and sources in selected papers
4.1.2 Spam and phishing datasets and sources
Spam Email . The SPAM Email Dataset contains a total of 4601 emails including 1813 spam emails and 2788 legitimate emails each characterized by 58 attributes. It was donated to the UCI Machine Learning Repository by Hewlett Packard in 1999 [ 110 ].
Ling-Spam . The Ling-Spam dataset, proposed by Androutsopoulos et al. [ 111 ] in 2000, contains both spam and legitimate emails retrieved from an email distribution list, the Linguistic list, focusing on linguistic interests around research opportunities, job postings, and software discussion. The dataset contains 2,893 different emails, of which 2,412 are genuine emails collected from the list’s digests and 481 are spam emails retrieved from one of the corpus’ authors.
WEBSPAM-UK2006 . The WEBSPAM-UK2006 dataset was obtained using a set of.UK pages downloaded by the Laboratory of Web Algorithmics of the University of Milan (Università degli Studi di Milano) and manually assessed by a group of volunteers in 2006. The dataset consists of labels, URLs and hyperlinks and HTML page contents of 77,741,046 Web pages [ 112 ].
SpamAssassin (spamassassin.apache.org). Apache SpamAssassin is an Open-Source anti-spam platform providing a filter to classify email and block spam. The SpamAssassin Public mail corpus is a selection of 6,047 emails prepared by SpamAssassin in 2006. Of the total count, there are 1,897 spam messages and 4,150 legitimate emails.
TREC2007 Public Corpus . The TREC 2007 Public Corpus contains all email messages delivered to a particular server. The server contained several accounts, fallen into disuse and several ‘honeypot’ accounts published on the web, which were used to sign up for a few services, some legitimate and some not. The TREC dataset contains 75,419 messages, of which 25,220 are legitimate emails and 50,199 are junk messages; the messages are divided into three subcorpora [ 113 ].
SMS Spam Collection . The SMS Spam Collection Dataset is a publicly available dataset created by Almeida et al. [ 114 , 115 , 116 ] in 2011. It is a labelled dataset of 5574 SMS messages, 747 spam and 4827 ham, collected from mobile phones.
“Gold standard” opinion spam . The “gold standard” opinion spam dataset was proposed by Ott et al. [ 117 ] in 2011. The corpus comprises 1,600 review texts, 800 deceptive and 800 genuine, on 20 hotels in the Chicago area. The genuine reviews were obtained from reviewing websites such as TripAdvisor, Expedia and Yelp and the deceptive ones were rendered using Amazon Mechanical Turk (AMT). In the dataset, 400 reviews are written with a negative sentimental polarity and 400 depict a positive sentimental polarity.
Spear phishing email dataset (2011) & Benign email dataset (2013) . These two datasets have been prepared by Symantec’s enterprise mail scanning service. The spear phishing email dataset contains 1,467 emails from 8 campaigns and the benign email dataset contains 14,043 emails. The emails were sent between 2011 and 2013, and have attachments, anonymous customer information and PII. The extraction process is described in [ 118 , 119 ].
MovieLens Dataset . The GroupLens Research has collected and made available rating datasets from the MovieLens website ( https://movielens.org ). The datasets were collected over various periods of time, depending on the size of the set. The MovieLens 20 M contains 20 million ratings and 465,000 tag applications applied to 27,000 movies by 138,000 users collected from January 1995 to March 2015 [ 120 ].
Netflix . The Netflix dataset Footnote 6 consists of listings of all the movies and TV shows available on Netflix, along with details such as - cast, directors, ratings, release year, duration, etc.
Twitter and Sina Weibo are two of the most influential social network media platforms in the world. Authors in the selected papers have either used crawlers or APIs to get sample data from these sources.
PhishTank , Footnote 7 DeltaPhish [ 121 ], Phish-Labls Footnote 8 and Anti-Phishing Working Group (APWG Footnote 9 ) are anti-phishing resources that publicly report phishing web pages in an effort to reduce fraud and identity theft caused by phishing and related incidents.
YELP Footnote 10 and delicious.com Footnote 11 publish crowd-sourced reviews about businesses. Similar to Twitter and Sina Weibo, APIs and crawlers may be used to extract data from these sources.
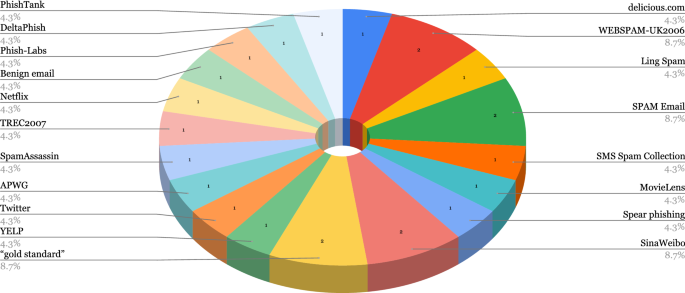
Usage of spam and phishing datasets and sources in selected papers
A breakdown of the usage of the described Spam and Phishing datasets in the selected papers is shown in Fig. 4 , we also provide an overview of the Spam and Phishing datasets in Table 16 . We observe that, in the revised works, there is no tendency towards using one or two specific datasets when tackling spam and/or phishing. In effect, the majority of the datasets are used in a single publication and only four, i.e. WEBSPAM-UK2006, Spam Email, SinaWeibo and “gold standard,” out of nineteen are used in two papers as shown in Fig. 4 . Additionally, except for the “gold standard” dataset, none of these datasets is balanced.
4.1.3 Malware datasets and sources
Georgia Tech Packed-Executable Dataset . The Georgia Tech Packed-Executable dataset [ 122 ] was published in 2008. It consists of 2598 packed viruses collected from the Malfease Project dataset (http://malfease.oarci.net), and 2231 non-packed benign executables collected from a clean installation of Windows XP Home plus several common user applications. The authors also generated 669 packed benign executables by applying 17 different executable packing tools freely available on the Internet to the executables in the Windows XP start menu. Of the 3267 packed executables in their collection, PEiD ( http://peid.has.it ), one of the most used signature-based detectors for packed executables, was able to detect only 2262 of them, whereas 1005 remained undetected. Therefore, those 1005 undetected samples were kept in the test and the train set contains 4493 samples: 2231 samples related to the non-packed benign executables and 2262 patterns related to the packed executables detected using PEiD.
The Malimg Dataset [ 123 ], proposed in 2011 by the University of California, Santa Barbara, contains 9458 malware images from 25 families.
The Malware Genome Project [ 124 ], proposed by researchers at the North Carolina State University in 2011, contains 1260 Android Malware samples belonging to 49 different malware families collected from August 2010 to October 2011.
Malheur [ 125 , 126 ], proposed in 2011, is a tool for the automatic analysis of malware behaviour in a sandbox environment.
Malicia Dataset . The Malicia dataset [ 127 , 128 ], published in 2013, comprises 11,688 malware binaries collected from 500 drive-by download servers over a period of 11 months in Windows Portable Executable format. The objective of their work was to identify hosts which spread malware in the wild and to collect samples of malware. In order to collect the samples of malware they set up a honeypot and clients in this honeypot were referring to the malware URL database for downloading and milking the website by resolving the IP address.
CTU-Malware . The CTU-Malware dataset [ 129 ], also compiled by the Czech Technical University, consists of hundreds of captures (called scenarios) of different malware communication samples. Both malware and normal samples are included in the dataset as shown in Table 17 .
In 2015, Microsoft launched the Microsoft Malware Classification Challenge , along with the release of a dataset [ 130 ] consisting of over 20,000 malware samples belonging to nine families. Each malware file includes an identifier, which is a 20-character hash value that uniquely identifies the file, and a class label, which is an integer that represents one of the nine families to which the malware may belong.
USTC-TFC2016 . The USTC-TFC2016 dataset [ 131 ], published in 2017, consists of ten types of malware traffic from public websites which were collected from a real network environment from 2011 to 2015. Along with such malicious traffic, the benign part contains ten types of normal traffic which were collected using IXIA BPS, a professional network traffic simulation equipment. The dataset’s size is 3.71 GB in the pcap format. The dataset's composition is shown in Table 18 .
CICAndMal2017 . The CICAndMal2017 android malware dataset, published in 2018 by the CIC [ 132 ], consists of four malware categories namely Adware, Ransomware, Scareware, and SMS Malware and 80 traffic features extracted using CICFlowMeter [ 103 , 105 ]. The dataset includes 5,065 benign apps from the Google play market published in 2015, 2016, and 2017 and 426 malware samples belonging to 42 unique malware families. The dataset is fully labelled and contains network traffic, logs, API/SYS calls, phone statistics, and memory dumps of malware families shown in Table 19 .
CICMalDroid2020 . Also published by the CIC in 2020, the CICMalDroid2020 dataset [ 133 , 134 ] consists of more than 17,341 Android samples from several sources collected from December 2017 to December 2018. It includes complete capture of static and dynamic features and contains samples spanning between five distinct categories: Adware, Banking malware, SMS malware, Riskware and Benign. Out of 17,341 samples, 13,077 samples ran successfully while the rest failed due to errors such as time-out, invalid APK files, and memory allocation failures. Of the 13,077 samples, 12% failed to be opened mostly due to an “unterminated string” error. From the 11,598 remaining samples, 470 extracted features comprise frequencies of system calls, binders, and composite behaviours, 139 extracted features comprise frequencies of system calls and 50,621 extracted features comprise static information, such as intent actions, permissions, permissions, sensitive APIs, receivers, etc. A brief composition of the dataset is shown in Table 20 .
VxHeavens Footnote 12 is a website dedicated to providing information about malware. The archive comprises over 17,000 programs belonging to 585 malware families (Trojan, viruses, worms).
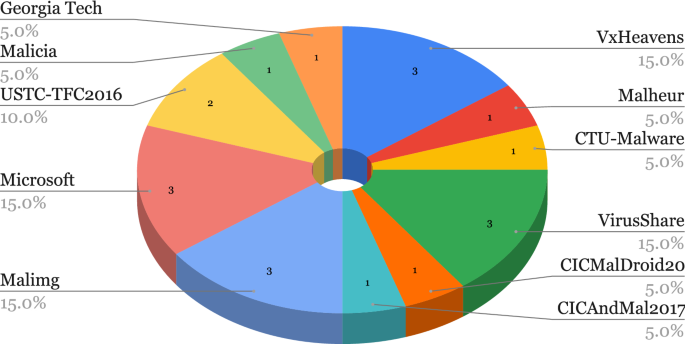
Usage of malware datasets and sources in selected papers
We provide an overview of the Malware datasets in Table 21 . In Fig. 5 , we also show a breakdown of the usage of the described Malware datasets in the selected papers. For these datasets, we observe that out of eleven datasets four have been used in three publications, one was used in two publications and the remaining six have been used only once. In addition, none of these datasets are balanced.
4.1.4 Additional datasets and sources
IEEE Test Feeders . For nearly two decades, the Distribution System Analysis (DSA) Subcommittee’s Test Feeder Working Group (TFWG) has been constructing publicly available distribution test feeders for use by academics. These test feeders aim to create distribution system models that reflect a wide range of design options and analytic issues. The 13-bus and 123-bus Feeders are part of the Test Feeder systems created in 1992 to evaluate and benchmark algorithms in solving unbalanced three-phase radial systems. The DSA Subcommittee approved them during the 2000 Power and Energy Society (PES) Summer Meeting. Schneider et al. [ 135 ] summarize the TFWG efforts and intended uses of Test Feeders.
XSSed Footnote 13 project was created in February 2007. It is an archive of cross-site scripting (XSS) vulnerable websites and provides information on things related to XSS vulnerabilities.
The NeCTAR (National eResearch Collaboration Tools and Resources) cloud platform, Footnote 14 launched in 2012 by the Australian Research Data Commons, provides Australia’s research community with fast, interactive, self-service access to large-scale computing infrastructure, software and data.
The Mobile-Sandbox [ 136 ] proposed by the University of Erlangen-Nurember, Germany, in 2014 is a static and dynamic analyzer system designed to support analysts detect malicious behaviours of malware.
Credit Card Fraud . The dataset has been collected and analyzed during a research collaboration of Worldline and the Machine Learning Group (http://mlg.ulb.ac.be) of ULB (Université Libre de Bruxelles) on big data mining and fraud detection [ 137 , 138 , 139 , 140 , 141 , 142 , 143 , 144 , 145 ]. The dataset contains transactions made by credit cards in September 2013 by European cardholders. This dataset presents transactions that occurred in two days, where we have 492 frauds out of 284,807 transactions. The dataset is highly unbalanced, the positive class (frauds) accounts for 0.172% of all transactions. It contains only numerical input variables which are the result of a PCA transformation. Unfortunately, the original features and more background information about the data are not provided due to confidentiality issues. The only features not transformed with PCA are ’Time’ and ’Amount’. Feature ’Time’ contains the seconds elapsed between each transaction and the first transaction in the dataset. The feature ’Amount’ is the transaction Amount.
Twitter ISIS Dataset . The Twitter ISIS dataset [ 84 ], published in 2018, consists of ISIS-related tweets/retweets in Arabic gathered from Feb. 2016 to May 2016. The dataset includes tweets and the associated information such as user ID, re-tweet ID, hashtags, number of followers, number of followees, content, date, and time. About 53 M tweets are collected based on the 290 hashtags such as State of the Islamic-Caliphate, and Islamic State. Table 22 provides a brief overview of the Twitter ISIS dataset composition.
Italian Retweets Timeseries . The Italian Retweets Timeseries dataset [ 146 ], published in 2019, contains temporal data of about 5,121,132 retweets from 47,947 users taken from the Italian Twittersphere published between 18/06/2018 and 01/07/2018.
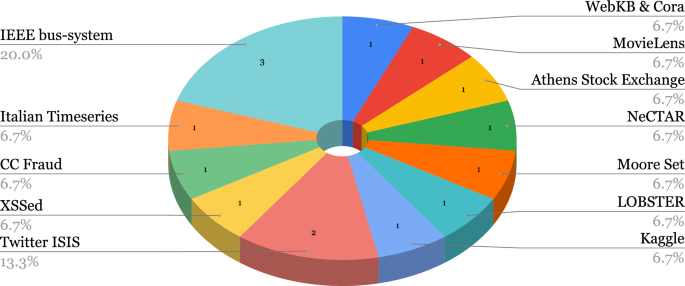
Usage of additional datasets and sources in selected papers
The breakdown of the usage of the additional datasets in the selected papers is shown in Fig. 6 .
4.2 Performance assessment metrics
Frequently, a model’s performance is evaluated by constructing a confusion matrix [ 147 ], shown in Table 23 , and calculating several metrics from the values of the confusion matrix. Table 24 shows the metrics commonly used to evaluate the performance of ML models. TP represents the true positives, the samples predicted as malicious or attacks that were truly malicious, TN the true negatives, the samples predicted as benign that were truly benign, FP the false positives, the samples predicted as attacks that were in fact benign, and FN the false negatives, the samples predicted as benign that were in fact attacks or malicious.
The accuracy score represents the fraction of correctly predicted samples, benign and malicious, and the error rate considers the misclassified samples. The accuracy metric may be misleading, especially when classes are highly imbalanced. The precision rate is the ratio of correctly predicted benign samples to all samples predicted as benign, and the sensitivity is the ratio of correctly predicted benign samples to samples to all benign samples. The Negative Predictive Value relates to the precision but considers the malicious samples; similarly, the specificity relates to the sensitivity but also considers the malicious samples. The False Positive (Negative) Rate is the ratio of malicious (benign) samples predicted as benign (malicious) to all the malicious (benign) samples. The \(F_1\) -score is the harmonic mean of the precision and recall scores. This metric aggregates two metrics to provide a more global view of the performance. The Geometric-Mean measures how balanced the prediction performances are on both the majority and minority classes.
The kappa ( \(\kappa \) ) statistic, introduced in [ 148 ], considers a model prequential accuracy, \(p_0\) , and the probability of randomly guessing a correct prediction, \(p_c\) . If the model is always correct, \(\kappa =1\) , and if the predictions are similar to random guessing, then \(\kappa =0\) . A \(\kappa < 0\) indicates less agreement than would be expected by chance alone. The Matthews Correlation Coefficient (also known as phi coefficient or mean square contingency coefficient), introduced in [ 149 ], may be seen as a discretization of the Pearson Correlation Coefficient [ 150 ], or Pearson’s r , for a binary confusion matrix. It measures the difference between predicted and actual values and returns a value between \(-1\) and \(+1\) , where \(-1\) indicates a completely incorrect classifier and 1 indicates the exact opposite.
Researchers also use graphical-based metrics to observe the performance. However, these metrics make the comparison between different models more complex. For this reason, summarizations of graphical-based metrics are used. An example of such metrics is the receiver operating characteristic curve, or ROC curve, which provides a graphical representation of a binary classifier system’s diagnostic performance when its discrimination threshold is modified. The Area Under the ROC (AUC ROC or AUROC) represents the probability that a uniformly drawn random positive sample is ranked higher than a consistently drawn random negative sample. Like the ROC, the Precision-Recall Curve (PRC) employs multiple thresholds on the model’s predictions to compute distinct scores for precision and recall. Because computing the Area Under the PRC (AUPRC) is not as straightforward as the AUROC computation process, the interested reader is referred to [ 151 ] where a review of the main solutions proposed to compute the AUPRC is presented. Finally, training time and inference time are the time required to build a model and provide predictions, respectively.
As seen in Fig. 7 , where we present a breakdown of the usage of the evaluation metrics in the selected papers, the ACC is the most used of the 15 metrics considered for evaluation in the selected papers. In 108 out of the 210 selected papers, or 22.1%, the ACC is used for evaluation. It is followed by the DR which has been used in 100 papers, or 20.5%, the PPV which has been used in 69 papers, or 14.1% and the \(F_1\) -score which has been used in 61 papers, or 12.5%. As highlighted in Sect. 4.1 , except for the “gold standard” dataset, none of the presented datasets is balanced, which points that the ACC measure is not a suitable metric for performance assessment. The DR, PPV and \(F_1\) -score, however, are more suitable metrics than the accuracy as they consider the class imbalance in datasets. In cyber-security, the DR is useful as there is a high cost associated to attacks, similarly the PPV is an important metric to consider as a low PPV indicates that benign samples or transactions are being flagged as attacks which renders the ML model useless. Due to the imbalanced nature of cyber-security datasets as seen in Sect. 4.1 , the \(F_1\) -score is a useful assessment metric as it simply balances the DR and PPV. The least used metrics are the NPV and \(\kappa \) -score, which have both been used only once in the selected papers. The NPV is proportional to the frequency of attacks in the dataset, in other words, it is sensitive to imbalanced datasets. As a result, if the prevalence of attacks in the training dataset differs from the prevalence of attacks in the actual world, the computed NPV may be inaccurate. That is, as the prevalence of attacks decreases, the NPV increases because there are more true negatives for every false negative. This is because a false negative would imply that a data point is actually an attack, which is improbable given the scarcity of attacks [ 152 ]. Similarly, the \(\kappa \) -score is also sensitive to imbalanced datasets, therefore it is not suitable in the cyber-security domain where attacks are less frequent than benign samples or transactions. Finally, the time complexity (training and inference) is only reported in 2.7% of the selected papers.
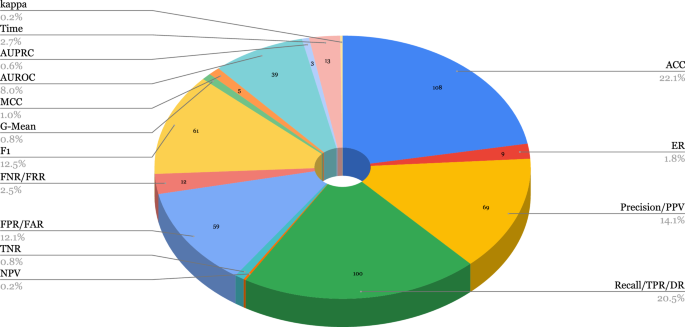
Usage of evaluation metrics in selected papers
5 Open issues and challenges
This section answers our third research question and presents the open challenges found in the literature. We cover open issues and challenges in the areas of the datasets and assessment metrics used, review the learnt lessons and recommend future research directions. Finally, we also discuss the challenge of the gap between research and practice in the field of cyber-security, particularly in the application of ML.
5.1 Datasets and repositories
In Sect. 4.1 , we have described 45 datasets, repositories and sources. We summarize the key issues found related to the datasets in this subsection.
Over 70 of the 100 reviewed articles focusing on intrusion detection used either the KDD’99 or the NSL-KDD datasets which are closed, anonymized, and outdated (over 20 years old) datasets. Similarly, the most recent Spam and Phishing email dataset used in the selected papers is from 2013. Therefore it is possible that some of the parts under consideration are no longer relevant due to changes in attack vectors and additional factors such as availability and comparability. Additionally, the use of outdated datasets hinders the ability to generalize the results to current real-world scenarios [ 153 ].
Besides being outdated, both the Spam and Phishing datasets used in the selected papers, except for the TREC and WEBSPAM-UK, contain less data when compared to the intrusion datasets. They comprise 5000 or fewer samples, with the “gold standard” dataset containing only 1600 samples.
Moreover, in addition to not only containing synthetically generated but also manually labelled data, the class imbalance in these datasets is not representative when compared to real-world scenarios, rendering the proposed approaches ineffective when applied to real data. This is one of the primary reasons why most academic methods are not implemented in practice.
As shown in Table 15 , apart from the KDD’99, NSL-KDD, UNIBS2009, AWID2015, UNSW-NB15 and UGR’16 datasets, the datasets in the selected papers are not originally split into train and test partitions, but even then, authors train and test their proposed approaches on random and narrower partitions of these datasets or train/test partitions
Most of the data collected from traffic or spam and/or phishing feeds are frequently kept private, making it impossible for other authors to reproduce results.
There are no updated, standard and public benchmark datasets for the different cyber-security problems. Due to these facts, accurate comparisons of the approaches are impossible without having to re-implement them and obtain the data from sources such as traffic or phishing feeds.
In computer science, the quality of the output is decided by the quality of the input, as stated by George Fuechsel in the concept “Garbage in, Garbage out.”. We acknowledge the limitations of the reviewed datasets and repositories and advocate the need for the development of more up-to-date, standardized, and open benchmark cyber-security datasets that reflect the current state of cyber threats and attack vectors, those datasets should also be adequately separated into training/testing and validation partitions. Additionally, we recommend that future studies should consider using multiple datasets and testing the models on a variety of scenarios to improve the generalizability of the results and allow proper evaluation, comparison, and real-world applications.
5.2 Performance assessment metrics
In Sect. 4.2 , we presented the 15 metrics used in the selected papers for assessing the performance of the SSL models built on the datasets presented in Sect. 4.1 . In this subsection, we present an overview of the significant issues identified in relation to the performance assessment metrics.
Throughout the selected papers, we have noticed that certain important assessment metrics are not used in most of the papers. For example, in [ 154 ], only the AUROC is reported, in [ 155 , 156 ], only DR and FPR or FAR are reported, and in [ 157 ] only DR and ACC are reported. This shows that authors are giving more importance to certain metrics while overlooking others, such as PPV and \(F_1\) -score, which should be used in conjunction as they consider the class imbalance in datasets.
The accuracy is a misleading metric in imbalanced settings, however, it has been used alone in [ 158 , 159 , 160 , 161 ]. Furthermore, the accuracy can be inadequate for use in the real world, where data is typically unbalanced. In light of this, it is important to conduct assessments using realistic deployment situations with unbalanced data and adequate assessment frameworks. The chosen metrics must accommodate the needs of the target audience.
Only 2.7% reported time complexity measurements, which is an important metric in the cyber-security domain where attack should be detected as soon as possible and static models often need to be rebuilt from scratch to detect unseen attacks, more importance should be given to this assessment metric as it is imperative to detect and mitigate those attacks in a timely manner.
An excessive amount of false positives may be detrimental to cyber-security because they increase the likelihood that users will ignore or dismiss alarms, leaving them vulnerable to serious cyber threats that they might otherwise have caught. The fact that out of the 210 selected papers, only 59, or 12.1%, measure the FAR–an assessment metric that should be given more weight–demonstrates that it is not being prioritized enough.
The issue of imbalanced data in cyber-security has been the subject of several recent studies. In particular, researchers have explored alternative techniques to address this issue such as cost-sensitive learning [ 162 ], which assigns higher costs to the minority class (i.e., the class with fewer instances) than the majority class to encourage the model to focus more on correctly classifying instances of the minority class, thus improving the performance on the rare class. Additional techniques include data augmentation which can be done through methods such as over-/under-sampling, ensemble methods such as bagging and boosting, or using scalar and graphical metrics which are adequate for imbalanced settings [ 163 ].
5.3 Bridging the gap between ML-based cyber-security research and practice
The field of cyber-security faces a significant challenge due to the gap between research and practice, especially in the applications of ML [ 153 , 164 ]. While several industries have successfully deployed ML-based solutions in the field of cyber-security (Sect. 2.3 ), and research has made significant advances in developing new ML algorithms, the ML algorithms developed by academia are often not practical to implement in real-world scenarios due to scalability, data availability, and regulatory compliance issues. Moreover, the lack of communication and collaboration between academic researchers and industry practitioners adds to the disconnect. As a result, several ML-based cyber-security solutions have not been widely adopted in the industry. This gap underscores the need for increased knowledge sharing and cooperation between researchers and practitioners, a better understanding of the industrial requirements and constraints from academia, as well as a good understanding of ML concepts from both academia and practitioners [ 165 , 166 ].
To address this gap, there is a need for more interdisciplinary collaboration and partnerships between academia and industry. Collaboration can help researchers better understand the practical challenges faced by practitioners, while practitioners can provide researchers with access to real-world data and feedback on the effectiveness of ML algorithms in practice [ 164 ]. Another way to bridge the gap is through the development of standardized evaluation frameworks for ML-based cyber-security solutions as discussed in Sect. 5.2 . Standardization can help ensure that ML algorithms are evaluated in a consistent and transparent manner, making it easier for practitioners to understand the effectiveness of a particular solution.
Moreover, it is important to develop ML algorithms that are explainable and interpretable. Several AI algorithms used in cyber-security and other fields, in general, are considered “black boxes” [ 167 ], meaning it can be difficult to understand how they make decisions. This lack of transparency can be a barrier to adoption, as it can be difficult for practitioners to trust and validate the results produced by these algorithms. The development of more explainable and interpretable ML algorithms can help address this issue [ 168 , 169 , 170 ].
In summary, bridging the gap between research and practice in ML-based cyber-security requires interdisciplinary collaboration, standardized evaluation frameworks, and the development of explainable and interpretable ML algorithms.
6 Conclusion
In this survey, we have reviewed the datasets, repositories and performance assessment metrics used in the state-of-the-art applications of SSL methods in the field of cyber-security, namely network intrusion detection, spam and phishing detection, malware detection and categorization, and additional cyber-security areas. Good datasets are necessary for building and evaluating strong SSL models. Our main contribution is an extensive analysis of the cyber-security datasets and repositories. This in-depth analysis attempts to assist readers in identifying datasets and sources that are appropriate for their needs. The review of the datasets reveals that the research community has recognized that there is a lack of publicly available cyber-security datasets and has recently attempted to address this gap by publishing several datasets. Because multiple research organizations are working in this field, further intrusion detection datasets and advancements can be expected in the near future.
We investigated the datasets used in the different papers applying SSL methods for cyber-attack prevention as improvements over conventional security systems and either fully SL or UL methods which would not be adequate in the cyber-security field, where labelled data is often scarce and difficult to obtain. We have reviewed the subcategories of SSL methods and provided a taxonomy based on previous studies. To the best of our knowledge, this is the first work that analyzes the datasets used in the literature applying SSL methods for intrusion, spam, phishing, and malware detection. We have also summarized multiple performance evaluation metrics used for assessing the build models. In addition, where applicable, we have provided brief descriptions, compositions and trends of the datasets used in the reviewed literature. There are no up-to-date and representative benchmark datasets available for each threat domain. However, the datasets reviewed, despite being outdated, are still heavily used in research. Furthermore, most of the publicly available datasets are either imbalanced or not initially split into train/test/validation datasets, making comparing results a tedious task. Moreover, we have outlined the primary open challenges and issues identified in the literature, highlighted strategies for bridging the gap between research and practice, and compiled a comprehensive bibliography in this area. The aforementioned issues and challenges deserve particular attention in future research. Finally, we acknowledge the potential constraints associated with literature reviews, such as limitations on search thoroughness and content selection, which may influence our research; therefore, we made our best efforts to minimize these limitations.
https://securityintelligence.com/new-banking-trojan-icedid-discovered-by-ibm-x-force-research/ .
https://darktrace.com/news/darktrace-stops-ransomware-attack-at-uk-construction-company .
https://feedzai.com/blog/machine-learning-rules-vs-models-in-anti-money-laundering-platforms/ .
https://bwnews.pr/3YEZbhg .
https://www.kaggle.com .
https://www.kaggle.com/datasets/shivamb/netflix-shows .
https://www.phishtank.com .
https://www.phishlabs.com .
https://apwg.org .
https://www.yelp.com .
https://www.delicious.com.au .
https://vxug.fakedoma.in/archive/VxHeaven/index.html .
http://www.xssed.com/archive .
http://www.nectar.org.au/ .
Babbage C. Passages from the life of a philosopher. Longman, Green, Longman, Roberts, Green. OCLC: 258982
Ring M, Wunderlich S, Scheuring D, Landes D, Hotho A. A survey of network-based intrusion detection data sets. Comput Secur. 2019;86:147–67. https://doi.org/10.1016/j.cose.2019.06.005 .
Article Google Scholar
Glass-Vanderlan TR, Iannacone MD, Vincent MS, Chen Qian, Bridges RA. A survey of intrusion detection systems leveraging host data. arXiv. 2018 . https://doi.org/10.48550/arXiv.1805.06070 .
Shaukat K, Luo S, Varadharajan V, Hameed IA, Xu M. A survey on machine learning techniques for cyber security in the last decade. IEEE Access. 2020;8:222310–54. https://doi.org/10.1109/ACCESS.2020.3041951 .
Aslan A, Samet R. A comprehensive review on malware detection approaches. IEEE Access. 2020;8:6249–71. https://doi.org/10.1109/ACCESS.2019.2963724 .
Nisioti A, Mylonas A, Yoo PD, Katos V. From intrusion detection to attacker attribution: a comprehensive survey of unsupervised methods. IEEE Commun Surv Tutor. 2018;20(4):3369–88. https://doi.org/10.1109/COMST.2018.2854724 .
Ucci D, Aniello L, Baldoni R. Survey of machine learning techniques for malware analysis. Comp Sec. 2019;81:123–47. https://doi.org/10.1016/j.cose.2018.11.001 .
Martins N, Cruz JM, Cruz T, Henriques Abreu P. Adversarial machine learning applied to intrusion and malware scenarios: a systematic review. IEEE Access. 2020;8:35403–19. https://doi.org/10.1109/ACCESS.2020.2974752 .
Bhuyan MH, Bhattacharyya DK, Kalita JK. Network anomaly detection: methods, systems and tools. IEEE Commun Surv Tutor. 2014;16(1):303–36. https://doi.org/10.1109/SURV.2013.052213.00046 .
Jalil S, Usman M. A review of phishing URL detection using machine learning classifiers. In: Arai K, Kapoor S, Bhatia R, editors. Intelligent systems and applications. Advances in intelligentadvances in intelligent systems and computing. Amsterdam: Springer; 2021. p. 646–65. https://doi.org/10.1007/978-3-030-55187-2_47 .
Chapter Google Scholar
Mitchell TM. Machine learning. McGraw-Hill series in computer science. New York: McGraw-Hill; 1997.
Google Scholar
Flach P. Machine learning: the art and science of algorithms that make sense of data. New York: Cambridge University Press; 2012.
Book MATH Google Scholar
Russell SJ, Norvig P. Artificial intelligence: a modern approach. Englewood Cliffs: Prentice Hall series in artificial intelligence. Prentice Hall; 1995.
MATH Google Scholar
Hinton GE, Sejnowski TJ, editors. Unsupervised learning: foundations of neural computation. Computational neuroscience. Cambridge: MIT Press; 1999.
Chapelle O, Schölkopf B, Zien A. Semi-supervised Learning. Adaptive computation and machine learning. Cambridge: MIT Press; 2006.
van Engelen JE, Hoos HH. A survey on semi-supervised learning. Mach Learn. 2020;109(2):373–440. https://doi.org/10.1007/s10994-019-05855-6 .
Article MathSciNet MATH Google Scholar
Zhu X. Semi-supervised learning with graphs. PhD thesis (May 2005).
Hoi SCH, Sahoo D, Lu J, Zhao P. Online learning: a comprehensive survey. arXiv:1802.02871 . 2018.
Schatz D, Bashroush R, Wall J. Towards a more representative definition of cyber security. J Digital Foren Sec Law. 2017. https://doi.org/10.15394/jdfsl.2017.1476 .
Alazab M, Tang M. Deep learning applications for cyber security. Advanced sciences and technologies for security applications. Amsterdam: Springer; 2019. https://doi.org/10.1007/978-3-030-13057-2 .
Book Google Scholar
Biggio B, Corona I, Maiorca D, Nelson B, Šrndić N, Laskov P, Giacinto G, Roli F. Evasion attacks against machine learning at test time. In: Blockeel H, Kersting K, Nijssen S, Elezn F, editors. Machine learning and knowledge discovery in databases. Lecture notes in computer science. Amsterdam: Springer; 2013. https://doi.org/10.1007/978-3-642-40994-3_25 .
Lipton ZC. The mythos of model interpretability. arXiv. 2017;10:11 . https://doi.org/10.48550/arXiv.1606.03490 .
Belkin M, Niyogi P, Sindhwani V. Manifold regularization: a geometric framework for learning from labeled and unlabeled examples. J Mach Learn Res. 2006;7(85):2399–434.
MathSciNet MATH Google Scholar
Chapelle O, Weston J, Schölkopf B. Cluster kernels for semi-supervised learning. In Becker S, Thrun S, Obermayer K, editors. Advances in neural information processing systems, vol. 15. MIT Press; 2002. 8 pp. https://doi.org/10.5555/2968618.2968693 .
Bair E. Semi-supervised clustering methods: semi-supervised clustering methods. Wiley Interdisc Rev Comput Stat. 2013;5(5):349–61. https://doi.org/10.1002/wics.1270 .
Song Z, Yang X, Xu Z, King I. Graph-based semi-supervised learning: a comprehensive review. arXiv. 2021 . https://doi.org/10.48550/arXiv.2102.13303 .
Zhu X. Semi-supervised learning literature survey, 2005;60.
Zhu X, Goldberg AB. Introduction to semi-supervised learning. Synth Lect Artific Intell Mach Learn. 2009;3(1):1–130. https://doi.org/10.2200/S00196ED1V01Y200906AIM006 .
Article MATH Google Scholar
Basu S, Bilenko M, Mooney RJ. Comparing and unifying search-based and similarity-based approaches to semi-supervised clustering. 2003;8.
Grira N, Crucianu M, Boujemaa N. Unsupervised and semi-supervised clustering: a brief survey. 12; 2004.
Triguero I, García S, Herrera F. Self-labeled techniques for semi-supervised learning: taxonomy, software and empirical study. Knowl Inf Syst. 2015;42(2):245–84. https://doi.org/10.1007/s10115-013-0706-y .
Yarowsky D. Unsupervised word sense disambiguation rivaling supervised methods. Cambridge: Association for Computational Linguistics; 1995. https://doi.org/10.3115/981658.981684 .
Breiman L. Random forests. Mach Learn. 2001;45(1):5–32. https://doi.org/10.1023/A:1010933404324 .
Vapnik VN. Statistical learning theory. Adaptive and learning systems for signal processing, communications, and control. New York: Wiley; 1998.
Blum A, Mitchell T. Combining labeled and unlabeled data with co-training. Madison: ACM Press; 1998. p. 92–100. https://doi.org/10.1145/279943.279962 .
Mitchell TM. The role of unlabeled data in supervised learning. In Larrazabal J, Miranda LAP, editors. The role of unlabeled data in supervised learning. Dordrecht: Springer Netherlands; 2004. pp 103–111
Zhou Z-H, Li M. Tri-training: exploiting unlabeled data using three classifiers. IEEE Trans Knowl Data Eng. 2005;17(11):1529–41. https://doi.org/10.1109/TKDE.2005.186 .
Li M, Zhou Z-H. Improve computer-aided diagnosis with machine learning techniques using undiagnosed samples. IEEE Trans Syst Man Cybern A Syst Human. 2007;37(6):1088–98. https://doi.org/10.1109/TSMCA.2007.904745 .
Yu S, Krishnapuram B, Rosales R, Rao RB. Bayesian co-training. J Mach Learn Res. 2011;12(80):2649–80.
Vincent P, Larochelle H, Bengio Y, Manzagol P-A. Extracting and composing robust features with denoising autoencoders. Helsinki: ACM Press; 2008. https://doi.org/10.1145/1390156.1390294 .
Rifai S, Vincent P, Muller X, Glorot X, Bengio Y. Contractive auto-encoders: explicit invariance during feature extraction. International conference on machine learning. 2011; 8.
Mikolov T, Chen K, Corrado G, Dean J. Efficient estimation of word representations in vector space. arXiv. 2013 . https://doi.org/10.48550/arXiv.1301.3781 .
Pennington J, Socher R, Manning C. Glove: global vectors for word representation. Doha: Association for Computational Linguistics; 2014. p. 1532–43. https://doi.org/10.3115/v1/D14-1162 .
Dara R, Kremer SC, Stacey DA. Clustering unlabeled data with soms improves classification of labeled real-world data. Comp Sec. 2002;3:2237–22423. https://doi.org/10.1109/IJCNN.2002.1007489 .
Demiriz A, Bennett KP, Embrechts MJ. Semi-supervised clustering using genetic algorithms. 1999, 809–814.
Goldberg A, Zhu X, Singh A, Xu Z, Nowak R. Multi-manifold semi-supervised learning. In: van Dyk, D., Welling, M. (eds.) Proceedings of the Twelth International Conference on Artificial Intelligence and Statistics. Proceedings of Machine Learning Research, vol. 5, pp. 169–176. PMLR, Hilton Clearwater Beach Resort, Clearwater Beach, Florida. 2009.
Basu S, Banerjee A, Mooney RJ. Semi-supervised clustering by seeding. International conference on machine learning. 2002.
Wagstaff K, Cardie C, Rogers S, Schrödl S. Constrained k-means clustering with background knowledge. ICML ’01. San Francisco: Morgan Kaufmann Publishers Inc.; 2001. p. 577–84. https://doi.org/10.5555/645530.655669 .
Basu S, Banerjee A, Mooney RJ. Active semi-supervision for pairwise constrained clustering. Proc Int Conf Data Mining. 2004. https://doi.org/10.1137/1.9781611972740.31 .
Klein D, Kamvar SD, Manning CD. From instance-level constraints to space-level constraints: making the most of prior knowledge in data clustering. International conference on machine learning. 2002;8.
Jain AK. Data clustering: 50 years beyond k-means. Pattern Recogn Lett. 2010;31(8):651–66. https://doi.org/10.1016/j.patrec.2009.09.011 .
Davidson I, Ravi SS. Agglomerative hierarchical clustering with constraints: theoretical and empirical results. In: Jorge AM, Torgo L, Brazdil P, Camacho R, Gama J, editors. Knowledge discovery in databases: PKDD. Berlin: Springer; 2005. p. 59–70. https://doi.org/10.1007/11564126_11 .
Davidson I, Ravi SS. Using instance-level constraints in agglomerative hierarchical clustering: theoretical and empirical results. Data Mining Knowl Discov. 2009;18(2):257–82. https://doi.org/10.1007/s10618-008-0103-4 .
Article MathSciNet Google Scholar
Miyamoto S, Terami A. Semi-supervised agglomerative hierarchical clustering algorithms with pairwise constraints. 2010; pp. 1–6.
Miyamoto S, Terami A. Constrained agglomerative hierarchical clustering algorithms with penalties. 2011, pp. 422–427.
Zhao H, Qi Z. Hierarchical agglomerative clustering with ordering constraints. IEEE. 2010. https://doi.org/10.1109/WKDD.2010.123 .
Hamasuna Y, Endo Y, Miyamoto S. Semi-supervised agglomerative hierarchical clustering with ward method using clusterwise tolerance. MDAI’11. Berlin: Springer; 2011. p. 103–13.
Hamasuna Y, Endo Y, Miyamoto S. On agglomerative hierarchical clustering using clusterwise tolerance based pairwise constraints. J Adv Comput Intell Intell Inform. 2012;16(1):174–9. https://doi.org/10.20965/jaciii.2012.p0174 .
Bade K, Nurnberger A. Personalized hierarchical clustering. Hong Kong: IEEE; 2006. p. 181–7. https://doi.org/10.1109/WI.2006.131 .
Zheng L, Li T. Semi-supervised hierarchical clustering. 2011 IEEE 11th international conference on data mining. 2011, pp. 982–991.
Bair E, Tibshirani R. Semi-supervised methods to predict patient survival from gene expression data. PLoS Biol. 2004;2(4):108. https://doi.org/10.1371/journal.pbio.0020108 .
Chong Y, Ding Y, Yan Q, Pan S. Graph-based semi-supervised learning: a review. Neurocomputing. 2020;408:216–30. https://doi.org/10.1016/j.neucom.2019.12.130 .
Moore R. Cybercrime: investigating high-technology computer crime, 2nd edn. Anderson Pub. OCLC: ocn659239788.
Sharma DSK. Cyber security: a legal perspective. 2017. https://www.ripublication.com/irph/ijcis17/ijcisv9n1_01.pdf .
Gladden M. The handbook of information security for advanced neuroprosthetics. 2017.
Daniel L, Daniel L. Digital forensics for legal professionals: understanding digital evidence from the warrant to the courtroom. Amsterdam: Elsevier; 2012. https://doi.org/10.1016/C2010-0-67122-7 .
Casey E. Handbook of digital forensics and investigation. Academic. 2010. https://doi.org/10.1016/C2009-0-01683-3 .
Security IBM. X-Force threat intelligence index. 2021;2021:50.
IBM Security: cost of a data breach report 2021. Risk quantification, 73. 2021.
Pirc J, DeSanto D, Davison I, Gragido W. 8—kill chain modeling. In: Pirc J, DeSanto D, Davison I, Gragido W (eds) Threat forecasting, pp. 115–127. Syngress.
Mukkamala S, Janoski G, Sung A. Intrusion detection using neural networks and support vector machines. In: Proceedings of the 2002 international joint conference on neural networks. IJCNN’02 (Cat. No.02CH37290), vol. 2, pp. 1702–17072. https://doi.org/10.1109/IJCNN.2002.1007774 . ISSN: 1098-7576
García-Teodoro P, Díaz-Verdejo J, Maciá-Fernández G, Vázquez E. Anomaly-based network intrusion detection: techniques, systems and challenges. 28(1): 18–28. https://doi.org/10.1016/j.cose.2008.08.003 .
Security IBM. IBM Security X-Force Threat Intelligence Index. 2022;2022:59. https://www.ibm.com/downloads/cas/ADLMYLAZ
Alkhalil Z, Hewage C, Nawaf L, Khan I. Phishing attacks: a recent comprehensive study and a new anatomy. 2021. https://doi.org/10.3389/fcomp.2021.563060 .
Jáñez-Martino F, Alaiz-Rodríguez R, González-Castro V, Fidalgo E, Alegre E. A review of spam email detection: analysis of spammer strategies and the dataset shift problem. Artif Intell Rev. 2023;56(2):1145–73. https://doi.org/10.1007/s10462-022-10195-4 .
Nguyen TTT, Armitage G. A survey of techniques for internet traffic classification using machine learning. IEEE Commun Surv Tutor. 2008;10(4):56–76. https://doi.org/10.1109/SURV.2008.080406 .
Levine BN, Shields C, Margolin NB. A survey of solutions to the sybil attack. Amherst: University of Massachusetts Amherst; 2006. p. 224.
Riyanto A, Arifin Z. Pump-dump manipulation analysis: the influence of market capitalization and its impact on stock price volatility at indonesia stock exchange. Rev Integr Bus Econ Res. 2018;7(3):129–142. https://www.proquest.com/docview/2088916427 .
Akram T, RamaKrishnan S, Naveed M. Assessing four decades of global research studies on stock market manipulations: a sceintometric analysis. J Financ Crime. 2021. https://doi.org/10.1108/JFC-08-2020-0163 .
Ferrara E, Varol O, Davis C, Menczer F, Flammini A. The rise of social bots. Commun ACM. 2016;59(7):96–104. https://doi.org/10.1145/2818717 .
Shu K, Sliva A, Wang S, Tang J, Liu H. Fake news detection on social media: a data mining perspective. ACM SIGKDD Explor Newslett. 2017;19(1):22–36. https://doi.org/10.1145/3137597.3137600 .
Sundar AP, Li F, Zou X, Gao T, Russomanno ED. Understanding shilling attacks and their detection traits: a comprehensive survey. IEEE Access. 2020;8:171703–15. https://doi.org/10.1109/ACCESS.2020.3022962 .
Alvari H, Shaabani E, Shakarian P. Early identification of pathogenic social media accounts. 2018, pp. 169–174. https://doi.org/10.1109/ISI.2018.8587339 .
Shaabani E, Guo R, Shakarian P. Detecting pathogenic social media accounts without content or network structure. South Padre Island: IEEE; 2018. p. 57–64. https://doi.org/10.1109/ICDIS.2018.00016 .
Consumer Action: Credit card fraud training manual, 12; 2009. https://www.consumer-action.org/downloads/english/2009_CCF_Lesson_Plan_web.pdf . Accessed 24 Oct 2022.
McDaniel P, McLaughlin S. Security and privacy challenges in the smart grid. 2009;7(3):75–7. https://doi.org/10.1109/MSP.2009.76 .
IBM Security: IBM security X-force threat intelligence index 2023. 2023. https://www.ibm.com/downloads/cas/DB4GL8YM
Kitchenham B, Charters S. Guidelines for performing systematic literature reviews in software engineering. 2007. https://www.elsevier.com/__data/promis_misc/525444systematicreviewsguide.pdf .
Veritas Health Innovation: Covidence, Melbourne, Australia 2022. https://www.covidence.org/
Fitriani S, Mandala S, Murti MA. Review of semi-supervised method for intrusion detection system. In: 2016 Asia Pacific Conference on Multimedia and Broadcasting (APMediaCast), pp. 36–41. https://doi.org/10.1145/382912.382914 .
Lee W, Stolfo SJ. A framework for constructing features and models for intrusion detection systems. Trans Inf Syst Secur. 2000; 3(4): 227–261. https://doi.org/10.1109/APMediaCast.2016.7878168 .
Tavallaee M, Bagheri E, Lu W, Ghorbani AA. A detailed analysis of the kdd cup 99 data set. IEEE. 2009. https://doi.org/10.1109/CISDA.2009.5356528 .
Moore AW, Zuev D. Internet traffic classification using bayesian analysis techniques, 11. 2005. https://dl.acm.org/doi/10.1145/1064212.1064220
Pang R, Allman M, Bennett M, Lee J, Paxson V, Tierney B. A first look at modern enterprise traffic. ACM Press. 2005;2005:1. https://doi.org/10.1145/1330107.1330110 .
UCSD—Center for Applied Internet Data Analysis: CAIDA DDoS 2007 Attack Dataset (2007-08-04 to 2007-08-04). IMPACT, 2007. https://www.impactcybertrust.org/dataset_view?idDataset=117
Song J, Takakura H, Okabe Y, Eto M, Inoue D, Nakao K. Statistical analysis of honeypot data and building of kyoto 2006+ dataset for nids evaluation. In: Proceedings of the First Workshop on Building Analysis Datasets and Gathering Experience Returns for Security. BADGERS ’11, pp. 29–36. Association for Computing Machinery, New York, NY, USA, 2011. https://doi.org/10.1145/1978672.1978676 .
Sangkatsanee P, Wattanapongsakorn N, Charnsripinyo C. Practical real-time intrusion detection using machine learning approaches. Comput Commun. 2011;34:2227–35. https://doi.org/10.1016/j.comcom.2011.07.001 .
Gringoli F, Salgarelli L, Dusi M, Cascarano N, Risso F, Claffy CK. Gt: picking up the truth from the ground for internet traffic. ACM SIGCOMM Comput Commun Rev. 2009;39(5):12–8. https://doi.org/10.1145/1629607.1629610 .
Shiravi A, Shiravi H, Tavallaee M, Ghorbani AA. Toward developing a systematic approach to generate benchmark datasets for intrusion detection. 2012;31(3):357–74. https://doi.org/10.1016/j.cose.2011.12.012 .
García S, Grill M, Stiborek J, Zunino A. An empirical comparison of botnet detection methods. Comp Sec. 2014;45:100–23. https://doi.org/10.1016/j.cose.2014.05.011 .
Morris T, Vaughn R, Dandass YS. A testbed for scada control system cybersecurity research and pedagogy. Oak Ridge: ACM Press; 2011. p. 1. https://doi.org/10.1145/2179298.2179327 .
Kolias C, Kambourakis G, Stavrou A, Gritzalis S. Intrusion detection in 80211 networks: empirical evaluation of threats and a public dataset. IEEE Commun Surv Tutor. 2016;18(1):184–208. https://doi.org/10.1109/COMST.2015.2402161 .
Draper-Gil G, Lashkari AH, Mamun MSI, Ghorbani AA. Characterization of encrypted and VPN traffic using time-related features, Funchal, Madeira, Portugal, pp. 407–414. https://doi.org/10.5220/0005740704070414 .
Sharafaldin I, Habibi Lashkari A, Ghorbani AA. Toward generating a new intrusion detection dataset and intrusion traffic characterization. Funchal: Science and Technology Publications; 2018. p. 108–16. https://doi.org/10.5220/0006639801080116 .
Habibi Lashkari A, Draper Gil G, Mamun M, Ghorbani A. Characterization of tor traffic using time based features. https://doi.org/10.5220/0006105602530262 .
Maciá-Fernández G, Camacho J, Magán-Carrión R, García-Teodoro P, Therón R. Ugr16: a new dataset for the evaluation of cyclostationarity-based network IDSs. 2018; 73: 411–424. https://doi.org/10.1016/j.cose.2017.11.004 .
Mirsky Y, Doitshman T, Elovici Y, Shabtai A. Kitsune: an ensemble of autoencoders for online network intrusion detection. arXiv. 2018;10:11. https://doi.org/10.48550/arXiv.1802.09089 .
Netresec: Public PCAP files for download, Olstavagen 6, 74961 Orsundsbro, Sweden. 2022. https://www.netresec.com/?page=PcapFiles .
Cho K, Mitsuya K, Kato A. Traffic data repository at the wide project, 8. 2000. https://dl.acm.org/doi/10.5555/1267724.1267775 .
Hopkins M, Reeber E, Forman G, Suermondt J. Spambase Data Set. 1999. http://archive.ics.uci.edu/ml/datasets/Spambase .
Androutsopoulos I, Koutsias J, Chandrinos KV, Paliouras G, Spyropoulos CD. An evaluation of naive bayesian anti-spam filtering. 2000. https://arxiv.org/abs/cs/0006013 .
Castillo C, Donato D, Becchetti L, Boldi P, Leonardi S, Santini M, Vigna S. A reference collection for web spam. SIGIR Forum. 2006;40:2006.
Cormack GV. Trec 2006 spam track overview. Text Retrieval Conference.2006.
Almeida TA, Gómez JM, Yamakami A. Contributions to the study of sms spam filtering: new collection and results, pp. 259–262. 2011.
Almeida TA, Hidalgo JMG, Silva TP. Towards SMS spam filtering: results under a new dataset. Int J Inf Secur Sci. 2013;2:1–18.
Hidalgo JMG, Almeida TA, Yamakami A. On the validity of a new sms spam collection. Boca Raton: IEEE; 2012. p. 240–5. https://doi.org/10.1109/ICMLA.2012.211 .
Ott M, Choi Y, Cardie C, Hancock J. Finding deceptive opinion spam by any stretch of the imagination. Proceedings of the 49th annual meeting of the association for computational linguistics: human language technologies, Portland, Oregon, USA. Association for Computational Linguistics; 2011. pp 309–319. https://aclanthology.org/P11-1032 .
Lee M, Lewis D. Clustering disparate attacks: mapping the activities of the advanced persistent threat. 22. 2011. https://www.virusbulletin.com/uploads/pdf/conference_slides/2011/Lee-VB2011.pdf .
Thonnard O, Bilge L, O’Gorman G, Kiernan S, Lee M. Industrial espionage and targeted attacks: understanding the characteristics of an escalating threat. In: Balzarotti D, Stolfo SJ, Cova M, editors. Research in attacks, intrusions, and defenses. Berlin: Springer; 2012. p. 64–85. https://doi.org/10.1007/978-3-642-33338-5_4 .
Harper FM, Konstan JA. The movielens datasets: history and context. ACM Trans Int Intell Syst. 2016;5(4):1–19. https://doi.org/10.1145/2827872 .
Corona I, Biggio B, Contini M, Piras L, Corda R, Mereu M, Mureddu G, Ariu D, Roli F. DeltaPhish: detecting phishing webpages in compromised websites. In: Foley SN, Gollmann D, Snekkenes E, editors. Computer security—ESORICS. Berlin: Springer; 2017. p. 370–88. https://doi.org/10.1007/978-3-319-66402-6_22 .
Perdisci R, Lanzi A, Lee W. Classification of packed executables for accurate computer virus detection. Pattern Recog Lett. 2008;29(14):1941–6. https://doi.org/10.1016/j.patrec.2008.06.016 .
Nataraj L, Karthikeyan S, Jacob G, Manjunath BS. Malware images: visualization and automatic classification VizSec ’11. New York: Association for llhinery; 2011. p. 1–7. https://doi.org/10.1145/2016904.2016908 .
Zhou Y, Jiang X. Dissecting android malware: characterization and evolution. San Francisco: IEEE; 2012. p. 95–109. https://doi.org/10.1109/SP.2012.16 .
Rieck K, Trinius P, Willems C, Holz T. Automatic analysis of malware behavior using machine learning. J Comput Sec. 2011;19(4):639–68. https://doi.org/10.3233/JCS-2010-0410 .
Rieck K. Malheur—automatic analysis of malware behavior. 2022. https://github.com/rieck/malheur .
Nappa A, Rafique MZ, Caballero J. Driving in the cloud: an analysis of drive-by download operations and abuse reporting. In: Rieck K, Stewin P, Seifert J-P, editors. Detection of intrusions and malware, and vulnerability assessment. Berlin: Springer; 2013. p. 1–20. https://doi.org/10.1007/978-3-642-39235-1_1 .
Nappa A, Rafique MZ, Caballero J. The malicia dataset: identification and analysis of drive-by download operations. Intl J Inf Sec. 2015;14(1):15–33. https://doi.org/10.1007/s10207-014-0248-7 .
Stratosphere: Stratosphere Laboratory Datasets. https://www.stratosphereips.org/datasets-overview . 2015. 24 Oct 2022.
Ronen R, Radu M, Feuerstein C, Yom-Tov E, Ahmadi M. Microsoft malware classification challenge. arXiv. 2018 . https://doi.org/10.48550/ARXIV.1802.10135 .
Wang W, Zhu M, Zeng X, Ye X, Sheng Y. Malware traffic classification using convolutional neural network for representation learning, pp. 712–717 (2017). https://doi.org/10.1109/ICOIN.2017.7899588 .
Lashkari AH, Kadir AFA, Taheri L, Ghorbani AA. Toward developing a systematic approach to generate benchmark android malware datasets and classification. Montreal: IEEE; 2018. p. 1–7. https://doi.org/10.1109/CCST.2018.8585560 .
Mahdavifar S, Abdul Kadir AF, Fatemi R, Alhadidi D, Ghorbani AA. Dynamic android malware category classification using semi-supervised deep learning, pp. 515–522 (2020). https://doi.org/10.1109/DASC-PICom-CBDCom-CyberSciTech49142.2020.00094 .
Mahdavifar S, Alhadidi D, Ghorbani AA. Effective and efficient hybrid android malware classification using pseudo-label stacked auto-encoder. J Netw Syst Manag. 2022;30(1):22. https://doi.org/10.1007/s10922-021-09634-4 .
Schneider KP, Mather BA, Pal BC, Ten C-W, Shirek GJ, Zhu H, Fuller JC, Pereira JLR, Ochoa LF, de Araujo LR, Dugan RC, Matthias S, Paudyal S, McDermott TE, Kersting W. Analytic considerations and design basis for the ieee distribution test feeders. IEEE Trans Power Syst. 2018;33(3):3181–8. https://doi.org/10.1109/TPWRS.2017.2760011 .
Spreitzenbarth M, Schreck T, Echtler F, Arp D, Hoffmann J. Mobile-sandbox: combining static and dynamic analysis with machine-learning techniques. Int J Inf Sec. 2015;14(2):141–53. https://doi.org/10.1007/s10207-014-0250-0 .
Carcillo F, Dal Pozzolo A, Le Borgne Y-A, Caelen O, Mazzer Y, Bontempi G. Scarff : a scalable framework for streaming credit card fraud detection with spark. Inf Fusion. 2018;41:182–94. https://doi.org/10.1016/j.inffus.2017.09.005 .
Carcillo F, Le Borgne Y-A, Caelen O, Bontempi G. Streaming active learning strategies for real-life credit card fraud detection: assessment and visualization. Int J Data Sci Anal. 2018;5(4):285–300. https://doi.org/10.1007/s41060-018-0116-z .
Carcillo F, Le Borgne Y-A, Caelen O, Kessaci Y, Oblé F, Bontempi G. Combining unsupervised and supervised learning in credit card fraud detection. Inf Sci. 2021;557:317–31. https://doi.org/10.1016/j.ins.2019.05.042 .
Dal Pozzolo A, Boracchi G, Caelen O, Alippi C, Bontempi G. Credit card fraud detection: a realistic modeling and a novel learning strategy. IEEE Trans Neural Netw Learn Syst. 2018;29(8):3784–97. https://doi.org/10.1109/TNNLS.2017.2736643 .
Dal Pozzolo A, Caelen O, Le Borgne Y-A, Waterschoot S, Bontempi G. Learned lessons in credit card fraud detection from a practitioner perspective. Expert Syst Appl. 2014;41(10):4915–28. https://doi.org/10.1016/j.eswa.2014.02.026 .
Lebichot B, Le Borgne Y-A, He-Guelton L, Oblé F, Bontempi G. Deep-learning domain adaptation techniques for credit cards fraud detection. In: Oneto L, Navarin N, Sperduti A, Anguita D, editors. Recent advances in big data and deep learning. Cham: Springer; 2020. https://doi.org/10.1016/j.eswa.2014.02.026 .
Lebichot B, Paldino GM, Siblini W, He-Guelton L, Oblé F, Bontempi G. Incremental learning strategies for credit cards fraud detection. Int J Data Sci Anal. 2021;12(2):165–74. https://doi.org/10.1007/s41060-021-00258-0 .
Pozzolo AD, Bontempi G. Adaptive machine learning for credit card fraud detection. PhD thesis. 2015.
Pozzolo AD, Caelen O, Johnson RA, Bontempi G. Calibrating probability with undersampling for unbalanced classification. Cape Town: IEEE; 2015. p. 159–66. https://doi.org/10.1109/SSCI.2015.33 .
Mazza M, Cresci S, Avvenuti M, Quattrociocchi W, Tesconi M. Italian retweets timeseries. Zenodo. 2019. https://zenodo.org/record/2653137 .
Swets JA. Measuring the accuracy of diagnostic systems. Science 1988;240(4857):1285–93. https://doi.org/10.1177/001316446002000104 .
Cohen J. A coefficient of agreement for nominal scales. Edu Psychol Meas. 1960;20(1):37–46. https://doi.org/10.1177/001316446002000104 .
Matthews BW. Comparison of the predicted and observed secondary structure of t4 phage lysozyme. Biochim Biophys Acta (BBA) Protein Struct 405(2), 442–451. https://doi.org/10.1016/0005-2795(75)90109-9 .
Pearson K. Note on regression and inheritance in the case of two parents. Proc R Soci Lond Ser. 1895;I(58):240–2.
Gaudreault J-G, Branco P, Gama J. An analysis of performance metrics for imbalanced classification. In: Soares C, Torgo L, editors. Discovery Science, vol. 12986. Berlin: Springer; 2021. p. 67–77. https://doi.org/10.1007/978-3-030-88942-5_6 .
Iverson GL. Negative predictive power. In: Kreutzer JS, DeLuca J, Caplan B, editors. Encyclopedia of clinical neuropsychology. Berlin: Springer; 2011. p. 1720–2. https://doi.org/10.1007/978-0-387-79948-3_1219 .
Bertoli GdC, Junior LAP, Verri FAN, Santos ALd, Saotome O. Bridging the gap to real-world for network intrusion detection systems with data-centric approach. 2021
Zavrak S, İskefiyeli M. Anomaly-based intrusion detection from network flow features using variational autoencoder. IEEE Access. 2020;8:108346–58. https://doi.org/10.1109/ACCESS.2020.3001350 .
Angiulli F, Argento L, Furfaro A. Exploiting n-gram location for intrusion detection. 2015 IEEE 27th International Conference on Tools with Artificial Intelligence (ICTAI), pp. 1093–1098. https://doi.org/10.1109/ICTAI.2015.155
Xian G. Cyber intrusion prevention for large-scale semi-supervised deep learning based on local and non-local regularization. IEEE Access. 2020;8:55526–39. https://doi.org/10.1109/ACCESS.2020.2981162 .
Chen L, Zhang M, Yang C-y, Sahita R. POSTER: Semi-supervised classification for dynamic android malware detection. In: Proceedings of the 2017 ACM SIGSAC Conference on Computer and Communications Security. CCS ’17, pp. 2479–2481. Association for Computing Machinery, Dallas, Texas, USA. 2017.
Zhang S, Du C. Semi-supervised deep learning based network intrusion detection. 2020, pp. 35–40.
Yao H, Fu D, Zhang P, Li M, Liu Y. Msml: a novel multilevel semi-supervised machine learning framework for intrusion detection system. IEEE Int Things J. 2019;6(2):1949–59. https://doi.org/10.1109/JIOT.2018.2873125 .
Chen C, Gong Y, Tian Y. Semi-supervised learning methods for network intrusion detection. 2008 IEEE International Conference on Systems, Man and Cybernetics, 2008, pp. 2603–2608. https://doi.org/10.1109/ICSMC.2008.4811688 .
Yang J, Yang P, Jin X, Ma Q. Multi-classification for malicious url based on improved semi-supervised algorithm. 2017 IEEE international conference on computational science and engineering (CSE) and IEEE International Conference on Embedded and Ubiquitous Computing (EUC) 2017;1:143–50. https://doi.org/10.1109/CSE-EUC.2017.34 .
Elkan C. The foundations of cost-sensitive learning. In: Proceedings of the 17th International Joint Conference on Artificial Intelligence—Volume 2. IJCAI’01, pp. 973–978. Morgan Kaufmann Publishers Inc.
Branco P, Torgo L, Ribeiro RP. A survey of predictive modeling on imbalanced domains. Comput Surv. 2017;49(2):1–50. https://doi.org/10.1145/2907070
Apruzzese G, Anderson HS, Dambra S, Freeman D, Pierazzi F, Roundy KA. “Real attackers don’t compute gradients”: bridging the gap between adversarial ML research and practice. arXiv. 2022. https://doi.org/10.48550/arXiv.2212.14315 .
Grosse K, Bieringer L, Besold TR, Biggio B, Krombholz K. “Why do so?”—a practical perspective on machine learning security. arXiv. 2022. https://doi.org/10.48550/arXiv.2207.05164 .
Bieringer L, Grosse K, Backes M, Biggio B, Krombholz K. Industrial practitioners’ mental models of adversarial machine learning, pp. 97–116. https://www.usenix.org/conference/soups2022/presentation/bieringer .
Rudin C, Radin J. Why are we using black box models in AI when we don’t need to? A lesson from an explainable AI competition.
Van Lent M, Fisher W, Mancuso M. An explainable artificial intelligence system for small-unit tactical behavior. In: Proceedings of the National Conference on Artificial Intelligence, pp. 900–907 (2004). Menlo Park, CA; Cambridge, MA; London; AAAI Press; MIT Press; 1999.
Vollert S, Atzmueller M, Theissler A. Interpretable machine learning: a brief survey from the predictive maintenance perspective. In: 2021 26th IEEE International Conference on Emerging Technologies and Factory Automation (ETFA ), pp. 01–08.
Nakagawa PI, Ferreira Pires L, Rebelo Moreira JL, Olavo Bonino L. Towards semantic description of explainable machine learning workflows. In: 2021 IEEE 25th International Enterprise Distributed Object Computing Workshop (EDOCW), pp. 236–244. ISSN: 2325-6605.
Download references
Acknowledgements
We thank the anonymous reviewers, the editor and the assistant editor for their constructive comments and suggestions. We are also thankful to Professor Daniel Amyot for providing his valuable guidance throughout the development of the literature review.
This research was supported by the Natural Sciences and Engineering Research Council of Canada, the Vector Institute, and The IBM Center for Advanced Studies (CAS) Canada within Research Project 1059.
Author information
Paul K. Mvula, Paula Branco, Guy-Vincent Jourdan & Herna L. Viktor
Present address: School of Electrical Engineering and Computer Science (EECS), University of Ottawa, 800 King Edward Avenue, Ottawa, K1N 6N5, ON, Canada
Paula Branco, Guy-Vincent Jourdan and Herna L. Viktor contributed equally to this work
Authors and Affiliations
You can also search for this author in PubMed Google Scholar
Contributions
P.M. worked on the conceptualization, methodology, software, visualization, and writing of the original draft. P.B., G.-V. J. and H.V. aided in the conceptualization, supervision, validation, reviewing and editing of the final manuscript. All authors read and approved the final manuscript.
Corresponding author
Correspondence to Paul K. Mvula .
Ethics declarations
Competing interests.
The authors would like to declare that they have no competing interests.
Additional information
Publisher's note.
Springer Nature remains neutral with regard to jurisdictional claims in published maps and institutional affiliations.
Rights and permissions
Open Access This article is licensed under a Creative Commons Attribution 4.0 International License, which permits use, sharing, adaptation, distribution and reproduction in any medium or format, as long as you give appropriate credit to the original author(s) and the source, provide a link to the Creative Commons licence, and indicate if changes were made. The images or other third party material in this article are included in the article's Creative Commons licence, unless indicated otherwise in a credit line to the material. If material is not included in the article's Creative Commons licence and your intended use is not permitted by statutory regulation or exceeds the permitted use, you will need to obtain permission directly from the copyright holder. To view a copy of this licence, visit http://creativecommons.org/licenses/by/4.0/ .
Reprints and permissions
About this article
Mvula, P.K., Branco, P., Jourdan, GV. et al. A systematic literature review of cyber-security data repositories and performance assessment metrics for semi-supervised learning. Discov Data 1 , 4 (2023). https://doi.org/10.1007/s44248-023-00003-x
Download citation
Received : 27 January 2023
Accepted : 21 March 2023
Published : 06 April 2023
DOI : https://doi.org/10.1007/s44248-023-00003-x
Share this article
Anyone you share the following link with will be able to read this content:
Sorry, a shareable link is not currently available for this article.
Provided by the Springer Nature SharedIt content-sharing initiative
- Cyber-security
- Performance metrics
- Phishing detection
- Intrusion detection
- Malware detection
- Find a journal
- Publish with us
- Track your research
For enquiries call:
+1-469-442-0620

60+ Latest Cyber Security Research Topics for 2024
Home Blog Security 60+ Latest Cyber Security Research Topics for 2024

The concept of cybersecurity refers to cracking the security mechanisms that break in dynamic environments. Implementing Cyber Security Project topics and cyber security thesis topics /ideas helps overcome attacks and take mitigation approaches to security risks and threats in real-time. Undoubtedly, it focuses on events injected into the system, data, and the whole network to attack/disturb it.
The network can be attacked in various ways, including Distributed DoS, Knowledge Disruptions, Computer Viruses / Worms, and many more. Cyber-attacks are still rising, and more are waiting to harm their targeted systems and networks. Detecting Intrusions in cybersecurity has become challenging due to their Intelligence Performance. Therefore, it may negatively affect data integrity, privacy, availability, and security.
This article aims to demonstrate the most current Cyber Security Topics for Projects and areas of research currently lacking. We will talk about cyber security research questions, cyber security research questions, cyber security topics for the project, best cyber security research topics, research titles about cyber security and web security research topics.
.jpg&w=3840&q=75)
List of Trending Cyber Security Research Topics for 2024
Digital technology has revolutionized how all businesses, large or small, work, and even governments manage their day-to-day activities, requiring organizations, corporations, and government agencies to utilize computerized systems. To protect data against online attacks or unauthorized access, cybersecurity is a priority. There are many Cyber Security Courses online where you can learn about these topics. With the rapid development of technology comes an equally rapid shift in Cyber Security Research Topics and cybersecurity trends, as data breaches, ransomware, and hacks become almost routine news items. In 2024, these will be the top cybersecurity trends.
A) Exciting Mobile Cyber Security Research Paper Topics
- The significance of continuous user authentication on mobile gadgets.
- The efficacy of different mobile security approaches.
- Detecting mobile phone hacking.
- Assessing the threat of using portable devices to access banking services.
- Cybersecurity and mobile applications.
- The vulnerabilities in wireless mobile data exchange.
- The rise of mobile malware.
- The evolution of Android malware.
- How to know you’ve been hacked on mobile.
- The impact of mobile gadgets on cybersecurity.
B) Top Computer and Software Security Topics to Research
- Learn algorithms for data encryption
- Concept of risk management security
- How to develop the best Internet security software
- What are Encrypting Viruses- How does it work?
- How does a Ransomware attack work?
- Scanning of malware on your PC
- Infiltrating a Mac OS X operating system
- What are the effects of RSA on network security ?
- How do encrypting viruses work?
- DDoS attacks on IoT devices
C) Trending Information Security Research Topics
- Why should people avoid sharing their details on Facebook?
- What is the importance of unified user profiles?
- Discuss Cookies and Privacy
- White hat and black hat hackers
- What are the most secure methods for ensuring data integrity?
- Talk about the implications of Wi-Fi hacking apps on mobile phones
- Analyze the data breaches in 2024
- Discuss digital piracy in 2024
- critical cyber-attack concepts
- Social engineering and its importance
D) Current Network Security Research Topics
- Data storage centralization
- Identify Malicious activity on a computer system.
- Firewall
- Importance of keeping updated Software
- wireless sensor network
- What are the effects of ad-hoc networks
- How can a company network be safe?
- What are Network segmentation and its applications?
- Discuss Data Loss Prevention systems
- Discuss various methods for establishing secure algorithms in a network.
- Talk about two-factor authentication
E) Best Data Security Research Topics
- Importance of backup and recovery
- Benefits of logging for applications
- Understand physical data security
- Importance of Cloud Security
- In computing, the relationship between privacy and data security
- Talk about data leaks in mobile apps
- Discuss the effects of a black hole on a network system.
F) Important Application Security Research Topics
- Detect Malicious Activity on Google Play Apps
- Dangers of XSS attacks on apps
- Discuss SQL injection attacks.
- Insecure Deserialization Effect
- Check Security protocols
G) Cybersecurity Law & Ethics Research Topics
- Strict cybersecurity laws in China
- Importance of the Cybersecurity Information Sharing Act.
- USA, UK, and other countries' cybersecurity laws
- Discuss The Pipeline Security Act in the United States
H) Recent Cyberbullying Topics
- Protecting your Online Identity and Reputation
- Online Safety
- Sexual Harassment and Sexual Bullying
- Dealing with Bullying
- Stress Center for Teens
I) Operational Security Topics
- Identify sensitive data
- Identify possible threats
- Analyze security threats and vulnerabilities
- Appraise the threat level and vulnerability risk
- Devise a plan to mitigate the threats
J) Cybercrime Topics for a Research Paper
- Crime Prevention.
- Criminal Specialization.
- Drug Courts.
- Criminal Courts.
- Criminal Justice Ethics.
- Capital Punishment.
- Community Corrections.
- Criminal Law.
Research Area in Cyber Security
The field of cyber security is extensive and constantly evolving. Its research covers a wide range of subjects, including:
- Quantum & Space
- Data Privacy
- Criminology & Law
- AI & IoT Security
How to Choose the Best Research Topics in Cyber Security
A good cybersecurity assignment heading is a skill that not everyone has, and unfortunately, not everyone has one. You might have your teacher provide you with the topics, or you might be asked to come up with your own. If you want more research topics, you can take references from Certified Ethical Hacker Certification, where you will get more hints on new topics. If you don't know where to start, here are some tips. Follow them to create compelling cybersecurity assignment topics.
1. Brainstorm
In order to select the most appropriate heading for your cybersecurity assignment, you first need to brainstorm ideas. What specific matter do you wish to explore? In this case, come up with relevant topics about the subject and select those relevant to your issue when you use our list of topics. You can also go to cyber security-oriented websites to get some ideas. Using any blog post on the internet can prove helpful if you intend to write a research paper on security threats in 2024. Creating a brainstorming list with all the keywords and cybersecurity concepts you wish to discuss is another great way to start. Once that's done, pick the topics you feel most comfortable handling. Keep in mind to stay away from common topics as much as possible.
2. Understanding the Background
In order to write a cybersecurity assignment, you need to identify two or three research paper topics. Obtain the necessary resources and review them to gain background information on your heading. This will also allow you to learn new terminologies that can be used in your title to enhance it.
3. Write a Single Topic
Make sure the subject of your cybersecurity research paper doesn't fall into either extreme. Make sure the title is neither too narrow nor too broad. Topics on either extreme will be challenging to research and write about.
4. Be Flexible
There is no rule to say that the title you choose is permanent. It is perfectly okay to change your research paper topic along the way. For example, if you find another topic on this list to better suit your research paper, consider swapping it out.
The Layout of Cybersecurity Research Guidance
It is undeniable that usability is one of cybersecurity's most important social issues today. Increasingly, security features have become standard components of our digital environment, which pervade our lives and require both novices and experts to use them. Supported by confidentiality, integrity, and availability concerns, security features have become essential components of our digital environment.
In order to make security features easily accessible to a wider population, these functions need to be highly usable. This is especially true in this context because poor usability typically translates into the inadequate application of cybersecurity tools and functionality, resulting in their limited effectiveness.
Writing Tips from Expert
Additionally, a well-planned action plan and a set of useful tools are essential for delving into Cyber Security Research Topics. Not only do these topics present a vast realm of knowledge and potential innovation, but they also have paramount importance in today's digital age. Addressing the challenges and nuances of these research areas will contribute significantly to the global cybersecurity landscape, ensuring safer digital environments for all. It's crucial to approach these topics with diligence and an open mind to uncover groundbreaking insights.
- Before you begin writing your research paper, make sure you understand the assignment.
- Your Research Paper Should Have an Engaging Topic
- Find reputable sources by doing a little research
- Precisely state your thesis on cybersecurity
- A rough outline should be developed
- Finish your paper by writing a draft
- Make sure that your bibliography is formatted correctly and cites your sources.
Discover the Power of ITIL 4 Foundation - Unleash the Potential of Your Business with this Cost-Effective Solution. Boost Efficiency, Streamline Processes, and Stay Ahead of the Competition. Learn More!
Studies in the literature have identified and recommended guidelines and recommendations for addressing security usability problems to provide highly usable security. The purpose of such papers is to consolidate existing design guidelines and define an initial core list that can be used for future reference in the field of Cyber Security Research Topics.
The researcher takes advantage of the opportunity to provide an up-to-date analysis of cybersecurity usability issues and evaluation techniques applied so far. As a result of this research paper, researchers and practitioners interested in cybersecurity systems who value human and social design elements are likely to find it useful. You can find KnowledgeHut’s Cyber Security courses online and take maximum advantage of them.
Frequently Asked Questions (FAQs)
Businesses and individuals are changing how they handle cybersecurity as technology changes rapidly - from cloud-based services to new IoT devices.
Ideally, you should have read many papers and know their structure, what information they contain, and so on if you want to write something of interest to others.
The field of cyber security is extensive and constantly evolving. Its research covers various subjects, including Quantum & Space, Data Privacy, Criminology & Law, and AI & IoT Security.
Inmates having the right to work, transportation of concealed weapons, rape and violence in prison, verdicts on plea agreements, rehab versus reform, and how reliable are eyewitnesses?

Mrinal Prakash
I am a B.Tech Student who blogs about various topics on cyber security and is specialized in web application security
Avail your free 1:1 mentorship session.
Something went wrong
Upcoming Cyber Security Batches & Dates

- Android Malware 22
- Artificial Intelligence 4
- Check Point Research Publications 368
- Cloud Security 1
- Data & Threat Intelligence 1
- Data Analysis 0
- Global Cyber Attack Reports 300
- How To Guides 11
- Ransomware 1
- Russo-Ukrainian War 1
- Security Report 1
- Threat and data analysis 0
- Threat Research 169
- Web 3.0 Security 8
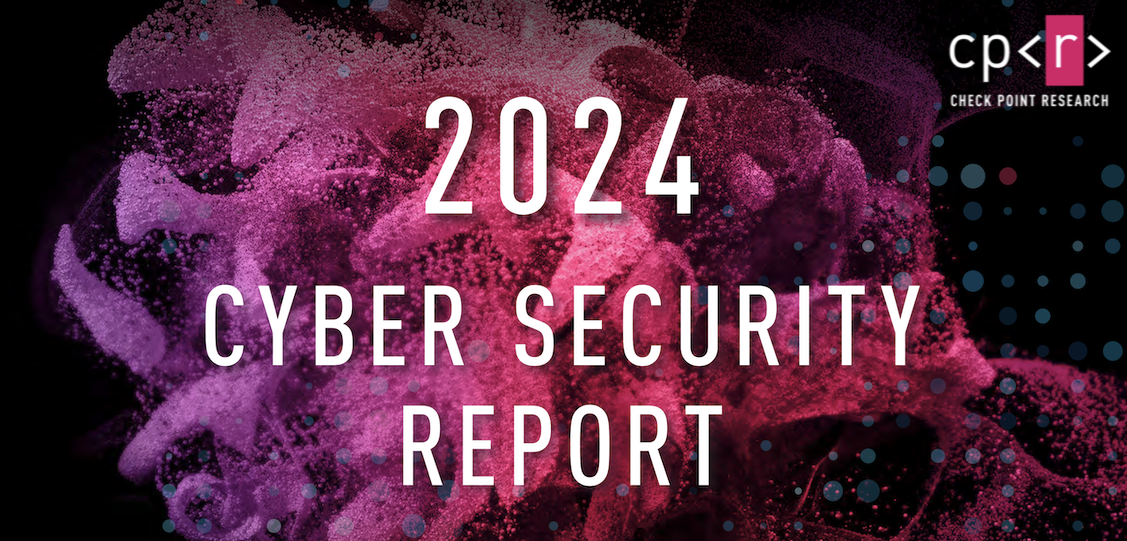
2024 Security Report: Podcast Edition
Once every year, Check Point releases an annual report reviewing the biggest events and trends in cybersecurity. In this episode we’ll break down the latest iteration, focusing on its most important parts, to catch you up on what you need to know most in 2024.
BLOGS AND PUBLICATIONS
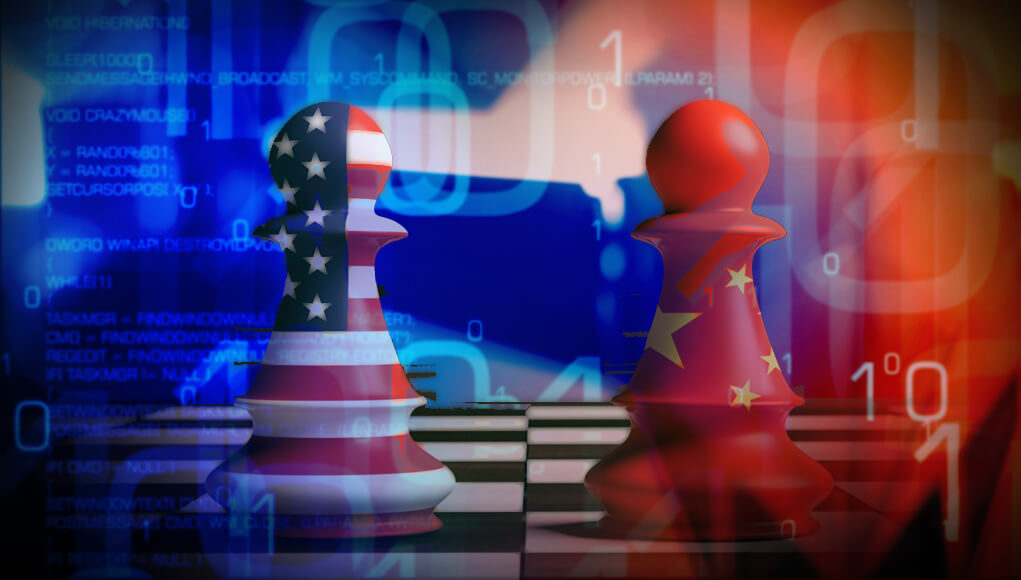
[CPRadio] UPSynergy: Chinese-American Spy vs. Spy Story
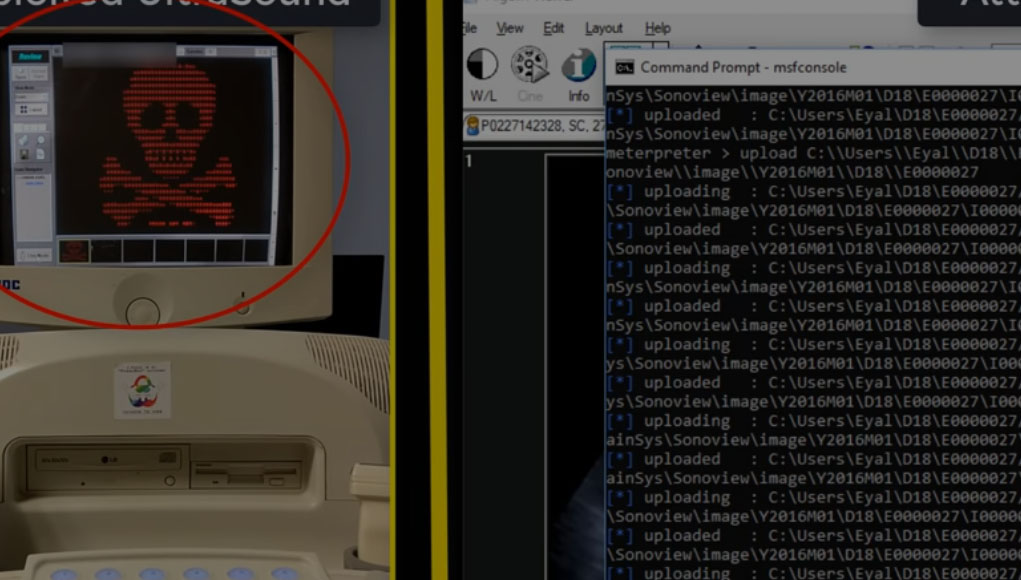
[CPRadio] UltraHack: The Security Risks of Medical IoT
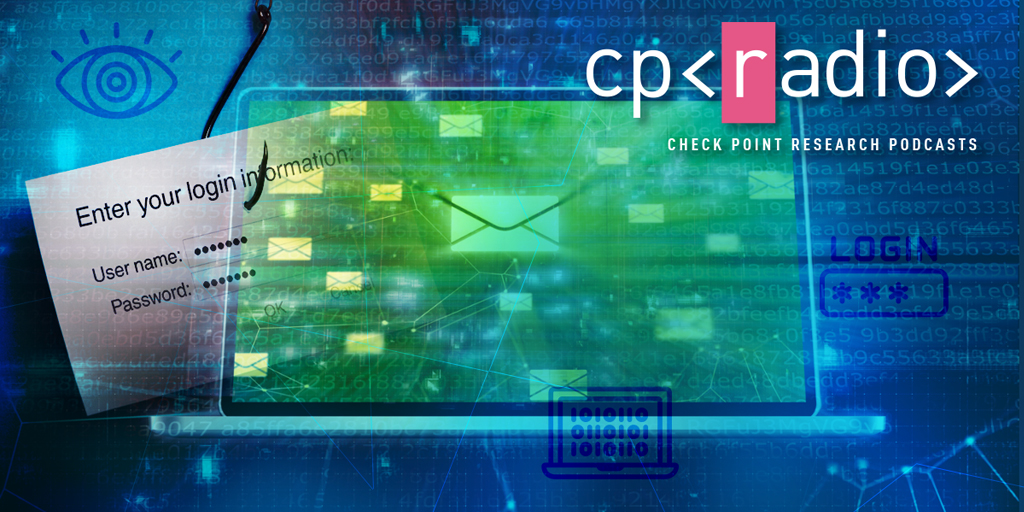
[CPRadio] Phishing in Canada
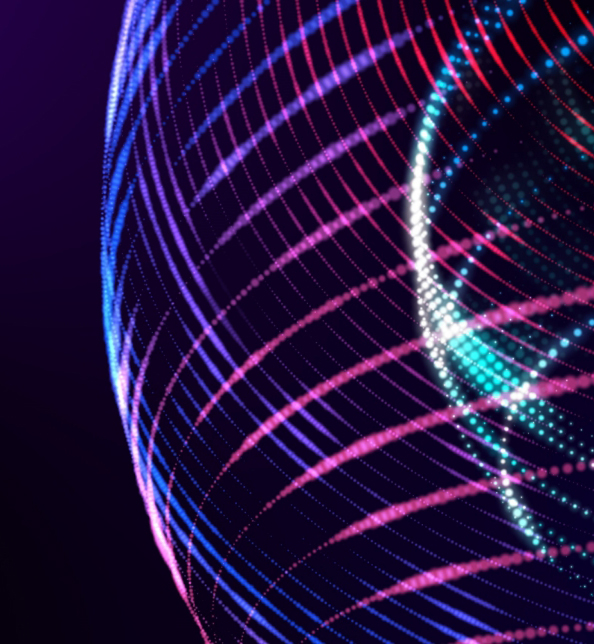
SUBSCRIBE TO CYBER INTELLIGENCE REPORTS
Country —Please choose an option— China India United States Indonesia Brazil Pakistan Nigeria Bangladesh Russia Japan Mexico Philippines Vietnam Ethiopia Egypt Germany Iran Turkey Democratic Republic of the Congo Thailand France United Kingdom Italy Burma South Africa South Korea Colombia Spain Ukraine Tanzania Kenya Argentina Algeria Poland Sudan Uganda Canada Iraq Morocco Peru Uzbekistan Saudi Arabia Malaysia Venezuela Nepal Afghanistan Yemen North Korea Ghana Mozambique Taiwan Australia Ivory Coast Syria Madagascar Angola Cameroon Sri Lanka Romania Burkina Faso Niger Kazakhstan Netherlands Chile Malawi Ecuador Guatemala Mali Cambodia Senegal Zambia Zimbabwe Chad South Sudan Belgium Cuba Tunisia Guinea Greece Portugal Rwanda Czech Republic Somalia Haiti Benin Burundi Bolivia Hungary Sweden Belarus Dominican Republic Azerbaijan Honduras Austria United Arab Emirates Israel Switzerland Tajikistan Bulgaria Hong Kong (China) Serbia Papua New Guinea Paraguay Laos Jordan El Salvador Eritrea Libya Togo Sierra Leone Nicaragua Kyrgyzstan Denmark Finland Slovakia Singapore Turkmenistan Norway Lebanon Costa Rica Central African Republic Ireland Georgia New Zealand Republic of the Congo Palestine Liberia Croatia Oman Bosnia and Herzegovina Puerto Rico Kuwait Moldov Mauritania Panama Uruguay Armenia Lithuania Albania Mongolia Jamaica Namibia Lesotho Qatar Macedonia Slovenia Botswana Latvia Gambia Kosovo Guinea-Bissau Gabon Equatorial Guinea Trinidad and Tobago Estonia Mauritius Swaziland Bahrain Timor-Leste Djibouti Cyprus Fiji Reunion (France) Guyana Comoros Bhutan Montenegro Macau (China) Solomon Islands Western Sahara Luxembourg Suriname Cape Verde Malta Guadeloupe (France) Martinique (France) Brunei Bahamas Iceland Maldives Belize Barbados French Polynesia (France) Vanuatu New Caledonia (France) French Guiana (France) Mayotte (France) Samoa Sao Tom and Principe Saint Lucia Guam (USA) Curacao (Netherlands) Saint Vincent and the Grenadines Kiribati United States Virgin Islands (USA) Grenada Tonga Aruba (Netherlands) Federated States of Micronesia Jersey (UK) Seychelles Antigua and Barbuda Isle of Man (UK) Andorra Dominica Bermuda (UK) Guernsey (UK) Greenland (Denmark) Marshall Islands American Samoa (USA) Cayman Islands (UK) Saint Kitts and Nevis Northern Mariana Islands (USA) Faroe Islands (Denmark) Sint Maarten (Netherlands) Saint Martin (France) Liechtenstein Monaco San Marino Turks and Caicos Islands (UK) Gibraltar (UK) British Virgin Islands (UK) Aland Islands (Finland) Caribbean Netherlands (Netherlands) Palau Cook Islands (NZ) Anguilla (UK) Wallis and Futuna (France) Tuvalu Nauru Saint Barthelemy (France) Saint Pierre and Miquelon (France) Montserrat (UK) Saint Helena, Ascension and Tristan da Cunha (UK) Svalbard and Jan Mayen (Norway) Falkland Islands (UK) Norfolk Island (Australia) Christmas Island (Australia) Niue (NZ) Tokelau (NZ) Vatican City Cocos (Keeling) Islands (Australia) Pitcairn Islands (UK)
We value your privacy!
BFSI uses cookies on this site. We use cookies to enable faster and easier experience for you. By continuing to visit this website you agree to our use of cookies.
- Choose your language...
- English (English)
- Spanish (Español)
- French (Français)
- German (Deutsch)
- Italian (Italiano)
- Portuguese (Português)
- Japanese (日本語)
- Chinese (中文)
- Korean (한국어)
- Taiwan (繁體中文)
- Organization Size
- Hybrid Cloud
- Zero Trust & Least Privilege
- Developer Security & Operations
- IoT Security Solutions
- Anti-Ransomware
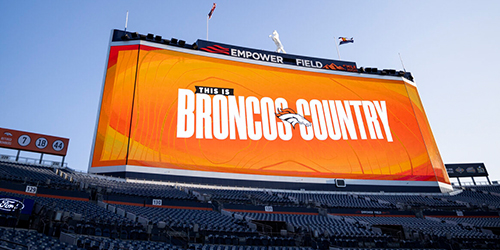
See how use cases come to life through Check Point's customer stories.
- Financial Services
- Federal Government
- State & Local Government
- Telco Service Provider
- Small & Medium Business
- Infinity Platform
- Secure the Network
- Secure the Cloud
- Secure the Workspace
- Security Operations and AI
- Platform Overview
- Infinity Core Services
- Infinity Portal Access Infinity Portal
- Infinity Platform Agreement Predictable cyber-security environments through a platform agreement
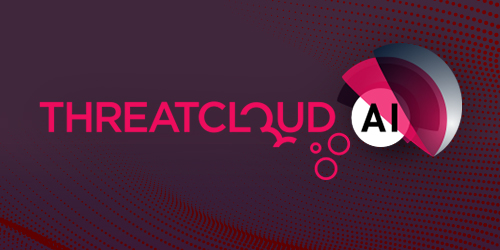
AI-Powered Threat Prevention
- Next Generation Firewall (NGFW) Security Gateway Industry-leading AI powered security gateways for modern enterprises
- SD-WAN Software Defined Wide Area networks converging security with networking
- Security Policy and Threat Management Manage firewall and security policy on a unified platform for on-premises and cloud networks
- Operational Technology and Internet of Things (IoT) Autonomous IoT/OT threat prevention with zero-trust profiling, virtual patching and segmentation
- Remote Access VPN Secure, seamless remote access to corporate networks
- Cloud Network Security Industry-leading threat prevention through cloud-native firewalls
- Cloud Native Application Protection Platform Cloud native prevention first security
- Code Security Developer centric code security
- Web Application and API Security Automated application and API security
- Email and Collaboration Security Email security including office & collaboration apps
- Endpoint Security Comprehensive endpoint protection to prevent attacks & data compromise
- Mobile Security Complete protection for the mobile workforce across all mobile devices
- SASE Unifying security with optimized internet and network connectivity
- Managed Prevention & Response Service SOC operations as a service with Infinty MDR/MPR
- Extended Prevention & Response AI-Powered, Cloud-Delivered Security Operations with Infinity XDR/XPR
- Secure Automation and Collaboration Automate response playbooks with Infinity Playblocks
- Unified Security Events and Logs as a Service Infinity Events cloud-based analysis, monitoring and reporting
- AI Powered Teammate Automated Security Admin & Incident Response with AI Copilot
- ThreatCloud AI The Brain behind Check Point’s threat prevention
- Cyber Security Risk Assessment Assess cyber security maturity and plan actionable goals
- Penetration Testing Evaluate security defenses against potential cyber attacks and threats
- Security Controls Gap Analysis (NIST CIST) Analyze technology gaps and plan solutions for improved security and ROI
- Threat Intelligence Analyzed data on cyber threats, aiding proactive security measures
- See All Infinity Global Services >
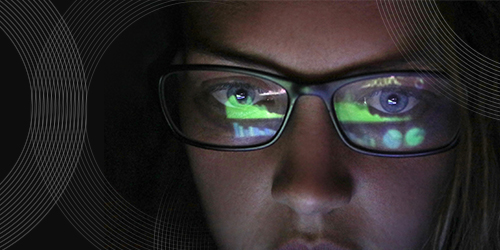
Learn hackers inside secrets and beat them at their own game
- Security Deployment & Optimization Strategic deployment and refinement of security for optimal protection
- Advanced Technical Account Management Proactive service delivered by highly skilled Cyber Security professionals
- Lifecycle Management Services Effectively maintain the lifecycle of security products and services
- Certifications & Accreditations Comprehensive cyber security training and certification programs
- CISO Training Globally recognized training for Chief Information Security Officers
- Security Awareness Empower employees with cyber security skills for work and home
- Cyber Range Simulated gamification environment for security training
- Mind Check Point Cyber Security and Awareness Programs training hub
- Incident Response Manage and mitigate security incidents with systematic response services
- Managed Detection and Response Prioritize prevention, delivering comprehensive SOC operations as a service
- Digital Forensics Comprehensive investigation and analysis of cyber incidents and attacks
- MXDR with Managed SIEM
- Managed Firewalls
- EDR with Agent Management
- Managed CNAPP
- Managed CSPM
- Support Programs Programs designed to help maximize security technology utilization
- Check Point PRO Proactive monitoring of infrastructure program offerings
- Contact Support
- Infinity Portal
- Infinity Platform Agreement
- Next Generation Firewall (NGFW) Security Gateway
- Security Policy and Threat Management
- Operational Technology and Internet of Things (IoT)
- Remote Access VPN
- Cloud Network Security
- Cloud Native Application Protection Platform
- Code Security
- Web Application and API Security
- Email and Collaboration Security
- Endpoint Security
- Mobile Security
- Managed Prevention & Response Service
- Extended Prevention & Response
- Secure Automation and Collaboration
- Unified Security Events and Logs as a Service
- AI Powered Teammate
- ThreatCloud AI
- Cyber Security Risk Assessment
- Penetration Testing
- Security Controls Gap Analysis (NIST CIST)
- Threat Intelligence
- Security Deployment & Optimization
- Advanced Technical Account Management
- Lifecycle Management Services
- Certifications & Accreditations
- CISO Training
- Security Awareness
- Cyber Range
- Incident Response
- Managed Detection and Response
- Digital Forensics
- Support Programs
- Check Point PRO
- Find a Partner
- Channel Partners
- Technology Partners
- MSSP Partners
- Azure Cloud
- Partner Portal
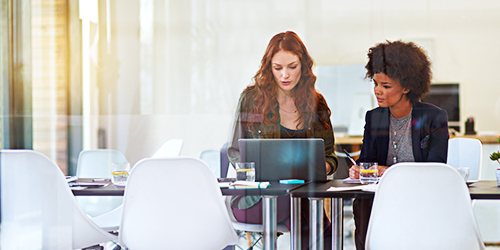
Check Point is 100% Channel. Grow Your Business with Us!
- Investor Relations
- Resource Center
- Customer Stories
- Events & Webinars
- Check Point Research
- Cyber Talk for Executives
- CheckMates Community
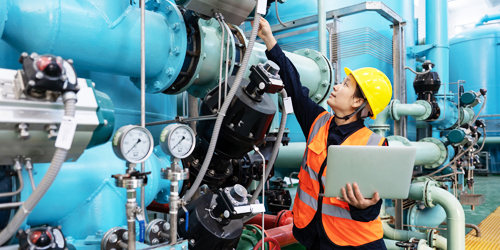
Shifting Attack Landscapes and Sectors in Q1 2024 with a 28% increase in cyber attacks globally

- Recurring increase in cyber attacks: Q1 2024 saw a marked 28% increase in the average number of cyber attacks per organization from the last quarter of 2023, though a 5% increase in Q1 YoY
- Sustained Industry Attacks focus : The Hardware Vendor industry saw a substantial rise of 37% cyber attacks YoY, as the Education/Research, Government/Military and Healthcare sector maintained their leads as the most heavily attacked sectors in Q1 2024
- Contrasting Regional Variances : The Africa region saw a notable 20% increase in cyber attacks, as opposed to Latin America, which reported a 20% decrease YoY
- Ransomware continues to surge : Europe saw a YoY 64% surge in ransomware attacks followed by Africa (18%), though North America emerged as the region most impacted by ransomware attacks with 59% out of close to 1000 published ransomware attacks from ransomware ‘shame sites’
The realm of cyber security is an ever-evolving battlefield. As we step into 2024, the shadows of 2023’s massive cyber threats still loomed, setting a precedent for what was to come. The first quarter of 2024 has seen an intriguing shift in the landscape of cyber attacks, both in frequency and in the nature of threats.
Global Cyber Security Trends for Q1 2024
In Q1 2024, Check Point Research (CPR) witnessed a notable increase in the average number of cyber attacks per organization per week, reaching 1308, marking a 5% increase from Q1 2023 and a 28% increase from the last quarter of 2023. This escalation is not just a number but a stark reminder of the persistent and evolving threat landscape, and the substantial increase from Q4 2023 accentuates a worrying trend of rapid escalation in cyber threats.
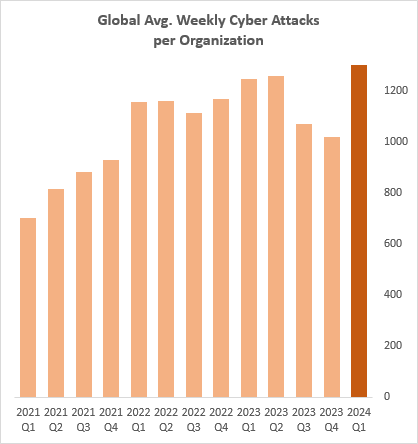
Global Attacks Per Industry
The Education/Research sector experienced a significant blow with an average of 2454 attacks per organization weekly, leading the chart in targeted industries, followed by the Government/Military (1692 attacks per week) and Healthcare (1605 attacks per organization) sectors, signalling an alarming vulnerability in sectors that are pivotal to societal function.
However, it is the substantial year-on-year increase in attacks on the Hardware Vendor industry, rising by 37%, which underlines a strategic shift in target preference by cybercriminals. This industry’s increasing reliance on hardware for IoT and smart devices makes these vendors lucrative targets for cybercriminals.
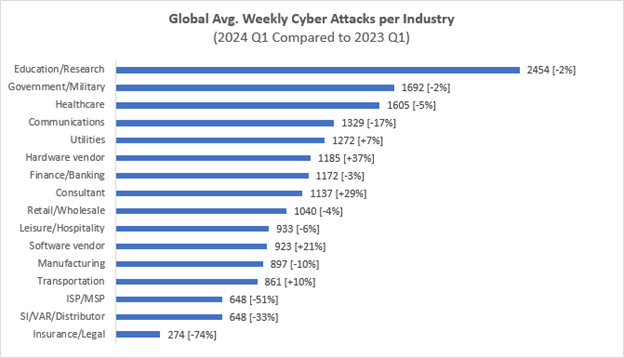
Regional Analysis of Overall Attacks
Regionally, Africa surged to the forefront with an average of 2373 attacks per week per organization, a 20% jump from the same period in 2023. In contrast, Latin America showed a 20% decline, perhaps indicating a shift in focus or improved defensive measures in the region; another reason could be a temporary shift in focus by cybercriminals on other more vulnerable regions across the world. The data also revealed a nuanced picture of varying intensities and types of cyber threats in different regions, underscoring the complex and dynamic nature of cyber warfare.
Ransomware Attack Insights per Region and Industry
In Q1 2024, North America was the region most impacted by Ransomware attacks, accounting for 59% out of close to 1000 published ransomware attacks*, followed by Europe (24%) and APAC (12%). The largest increase in reported attacks compared to Q1 2023 was seen in Europe, with a significant 64% increase. This significant increase could be attributed to factors such as increased digitization of services and regulatory environments that may make organizations more vulnerable or visible targets. In contrast, the North America saw a 16% increase, indicating a sustained focus by attackers on this region.
The most impacted Industry globally was the Manufacturing sector, accounting for 29% of published ransomware attacks and having almost double the amount of reported attacked YoY, followed by the Healthcare industry with 11% of the attacks (and 63% increase YoY), and Retail/Wholesale with 8% of the attacks.
The Communications sector saw the highest increase YoY in ransomware attacks with 177%, though it constituted only 4% of the published attacks in the quarter. The Communications sector’s surge in cyberattacks YOY could have been fueled by rapid digital transformation, integrating technologies like 5G and IoT, which expand vulnerabilities, while its critical role and handling of sensitive data make it a prime target for diverse threats, including state-sponsored espionage and data theft. The Manufacturing sector had the second highest increase in ransomware attacks with 96% YoY, and is a common prime target due to its heavy reliance on interconnected technology and weakened security capabilities due to the usage of legacy industrial technologies.
(*) This section features information derived from ransomware “shame sites” operated by double-extortion ransomware groups which posted the names and information of victims. The data from these shame sites carries its own biases, but still provides valuable insights into the ransomware ecosystem.
Practical Organization Strategies
Businesses must adopt a multi-faceted approach to cyber security, encompassing robust data backups, frequent cyber awareness training, timely security patches, strong user authentication, and advanced anti-ransomware solutions. Proactive engagement with AI-powered defenses can significantly bolster an organization’s resilience against these threats.
In response to these escalating threats which are becoming more sophisticated, advancements in defense techniques especially in threat detection and analysis and spotting anomalies and new attack patterns early, particularly in AI, have become pivotal. For instance, Check Point’s ThreatCloud AI, which underpins all its solutions, leverages AI and big data to counter sophisticated threats while minimizing false positives. It processes vast amounts of data and indicators of compromise daily. A practical example of its effectiveness is in handling zero-day attacks: a malicious link identified in the US is instantly blocked and this intelligence is shared globally, allowing a similar attack in Australia to be thwarted within seconds, averting potential harm.
The Drive to Defend Continues
The first quarter of 2024 has underscored the need for adaptive cybersecurity strategies to combat the evolving threat landscape. The increased attacks on specific industries and regions, coupled with the complexity of ransomware tactics, highlight the necessity for comprehensive and prevention-first approaches to cybersecurity. As we continue to navigate this challenging terrain, awareness, preparedness, and innovation in defense strategies remain our strongest allies.
You may also like
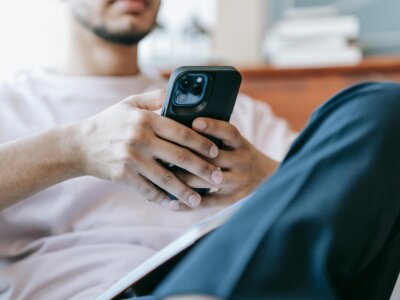
Not So Private After All: How Dating Apps Can Reveal Your Exact Location
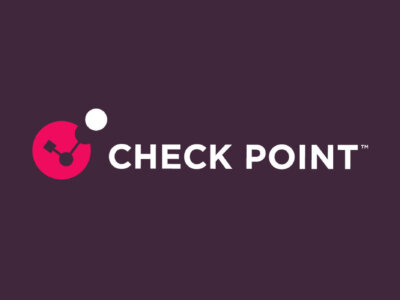
Agent Tesla Targeting United States & Australia: Revealing the Attackers’ Identities
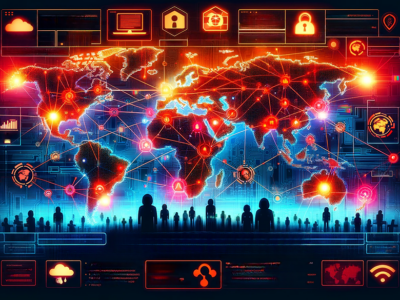
Beyond Imagining – How AI is actively used in election campaigns around the world
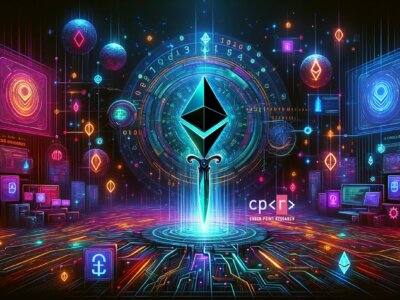
The Hidden Risks Within Ethereum’s CREATE2 Function: A Guide to Navigating Blockchain Security
Our approach
- Responsibility
- Infrastructure
- Try Meta AI
CYBERSECEVAL 2: A Wide-Ranging Cybersecurity Evaluation Suite for Large Language Models
April 18, 2024
Large language models (LLMs) introduce new security risks, but there are few comprehensive evaluation suites to measure and reduce these risks. We present CYBERSECEVAL 2, a novel benchmark to quantify LLM security risks and capabilities. We introduce two new areas for testing: prompt injection and code interpreter abuse. We evaluated multiple state of the art (SOTA) LLMs, including GPT-4, Mistral, Meta Llama 3 70B-Instruct, and Code Llama. Our results show conditioning away risk of attack remains an unsolved problem; for example, all tested models showed between 25% and 50% successful prompt injection tests. Our code is open source and can be used to evaluate other LLMs. We further introduce the safety-utility tradeoff : conditioning an LLM to reject unsafe prompts can cause the LLM to falsely reject answering benign prompts, which lowers utility. We propose quantifying this tradeoff using False Refusal Rate (FRR). As an illustration, we introduce a novel test set to quantify FRR for cyberattack helpfulness risk. We find many LLMs able to successfully comply with “borderline” benign requests while still rejecting most unsafe requests. Finally, we quantify the utility of LLMs for automating a core cybersecurity task, that of exploiting software vulnerabilities. This is important because the offensive capabilities of LLMs are of intense interest; we quantify this by creating novel test sets for four representative problems. We find that models with coding capabilities perform better than those without, but that further work is needed for LLMs to become proficient at exploit generation. Our code is open source and can be used to evaluate other LLMs.
GenAI Cybersec Team
Manish Bhatt
Sahana Chennabasappa
Cyrus Nikolaidis
Daniel Song
Shengye Wan
Faizan Ahmad
Cornelius Aschermann
Yaohui Chen
Dhaval Kapil
David Molnar
Spencer Whitman
Joshua Saxe

Help Us Pioneer The Future of AI
We share our open source frameworks, tools, libraries, and models for everything from research exploration to large-scale production deployment..
Product experiences
Foundational models
Latest news
Meta © 2024
More From Forbes
The endless possibilities of genai in cybersecurity transformation.

- Share to Facebook
- Share to Twitter
- Share to Linkedin
Tony is CEO at CyberProof and is a CISO at UST. CyberProof, a UST company, is an advanced managed detection and response provider.
AI is hardly the new kid on the block in cybersecurity. From the use of simple algorithms to machine learning and deep learning, most of us in the tech industry have seen AI used to great effect across a wide range of use cases.
With GenAI, in particular, we’re focusing on AI models that learn the patterns of language to generate its own content, including code . In many ways, this kind of AI is still in its infancy in terms of potential uses, especially for cybersecurity.
The Future Is Now
In 2023, when ChatGPT hit the headlines, Forrester discussed the potential benefits for cybersecurity (paywall) across three categories:
• Content creation , supported by GenAI, can allow teams to quickly summarize incidents for reporting, generating human-readable case descriptions and acting as coding assistants for developers.
Bitcoin Suddenly Braced For A 35 Trillion Halving Price Earthquake
New google play biometrics warning issued to all android users, apple watch series 9 hits all time low special offer price.
• Behavior prediction capabilities could predict privacy risks and attacker activities, plan attack scenarios and suggest remediation.
• Knowledge articulation would communicate information in a more human-friendly way, such as for querying the environment or creating product documentation.
Fast forward 18 months, and these benefits have moved beyond the realm of potential. GenAI as an AI-powered collaborator, what Microsoft calls “Copilot” and Google calls “Duet,” is having a measurable impact across core areas of the business, including:
• Incident response: Security teams who spend time hard coding and fine-tuning alerts and response processes now benefit from learning functions that identify relevance, investigate active incidents faster and augment threat-hunting capabilities.
• Compliance: Compliance is an ever-changing beast that varies between regions. GenAI can automatically collect relevant audit logs, investigate compliance risks and continuously validate that policies are in alignment with compliance mandates.
• Threat intelligence: GenAI is being used to simulate attacks ahead of time—to uncover vulnerabilities and identify exploitable zero days. Teams are also automating the distribution of threat intelligence to support information-sharing goals.
• CISO and executive communication: Visibility and transparency are everything for the C-suite. GenAI can create thorough incident summary drafts, build detailed threat prevention plans and report upward to executive stakeholders at a regular cadence.
The Value Of GenAI In Context
These kinds of broad GenAI capabilities are already in place, and within six months, I believe they will be broadly adopted.
However, enterprise environments are always going to be a target, and if you’re ready to take GenAI further than the basics to increase resilience, there is a clear opportunity here to adapt GenAI models to contextually fit your business needs.
This can be done using methods such as grounding , which tethers a model’s output to your specific data. With this approach, you are reducing the chance of inaccuracies or hallucinations, anchoring the model’s responses to relevant data and enhancing the trustworthiness of any content the model generates, including how applicable it is to your business and its specific needs.
When a model is grounded in context to the enterprise, its current architecture, its current vulnerabilities, its regional and industry-targeted compliance needs and its security policies and controls, the business gains can be immense. They include:
Enabling Analyst Productivity
While many companies are busy building LLM chatbots, which take users out of their workflow to augment analyst capabilities, I believe the true value of GenAI for security lies in addressing tasks that were previously part of the analyst workflow. This could be anything from writing draft incident response reports and completing “what if” analysis to querying logs. Routine, low-risk security tasks can even be automated from end to end.
To add further efficiencies, when GenAI gathers information from a broad range of sources and delivers it in plain language, analysts can adapt this to quarterlies and add their recommendations to save time.
Addressing The Skills Gap
Gartner believes that by 2025, half of all workplace cybersecurity incidents will happen because of “a lack of talent, or human failure.”
With GenAI at the product wheel, instead of training analysts on dozens of tools, they can be trained on security use cases instead. This accelerates time to value with new hires and allows novice and intermediate analysts to upskill with ease. Microsoft research shows that when using Copilot for Security, analysts were 26% faster across all tasks . That is the equivalent of adding an analyst to every team of four.
In addition, coaching and in-context recommendations offer personalized security awareness training across the business—making security part of business as usual.
Boosting Collaboration
Many security teams today work in functional silos, with teams such as incident response, threat intelligence, vulnerability scanning and threat hunting working independently of one another. GenAI can offer better and faster cross-functional collaboration, with information sharing at a scale allowing teams to monitor, preempt and respond to threats across their specific data estate.
Stakeholders can work collaboratively to ensure they are minimizing the potential impact on the business, its customers, and its reputation and streamlining everything from prevention to containment and response.
Sidestepping Challenges To Leverage GenAI For Success
To meet this potential, creating corporate governance for the use of AI is essential. A starting point for policies includes:
• Overreliance on AI: AI can make decisions that are unexplainable, fabricated or lack human judgment. System outputs should be questioned and never be considered infallible.
• Data security and compliance: AI data access, including transport and storage (for example training data), should be included in all data classification and protection requirements.
• Targeted AI attacks: How are you protecting the AI from malware or DDoS attacks? Many AI tools are in their earliest experimentation and research stages. This means the maturity of vulnerability testing is likely to be low.
GenAI is evolving rapidly, and codifying internal governance allows your security teams to stay two steps ahead, making the use of GenAI consistent across the business, and identifying risk ahead of time.
Forbes Technology Council is an invitation-only community for world-class CIOs, CTOs and technology executives. Do I qualify?
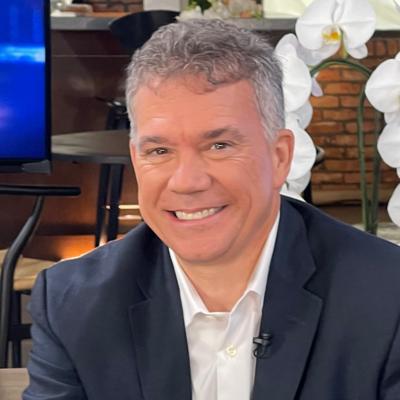
- Editorial Standards
- Reprints & Permissions

World-first “Cybercrime Index” ranks countries by cybercrime threat level
Following three years of intensive research, an international team of researchers have compiled the first ever ‘World Cybercrime Index’, which identifies the globe’s key cybercrime hotspots by ranking the most significant sources of cybercrime at a national level.
The Index, published today in the journal PLOS ONE , shows that a relatively small number of countries house the greatest cybercriminal threat. Russia tops the list, followed by Ukraine, China, the USA, Nigeria, and Romania. The UK comes in at number eight.
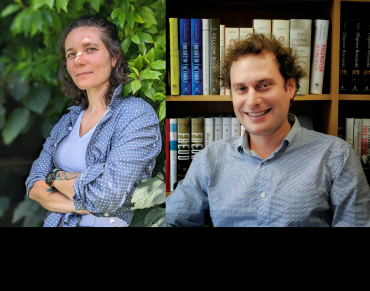
‘The research that underpins the Index will help remove the veil of anonymity around cybercriminal offenders, and we hope that it will aid the fight against the growing threat of profit-driven cybercrime,’ Dr Bruce said.
‘We now have a deeper understanding of the geography of cybercrime, and how different countries specialise in different types of cybercrime.’
‘By continuing to collect this data, we’ll be able to monitor the emergence of any new hotspots and it is possible early interventions could be made in at-risk countries before a serious cybercrime problem even develops.’
The data that underpins the Index was gathered through a survey of 92 leading cybercrime experts from around the world who are involved in cybercrime intelligence gathering and investigations. The survey asked the experts to consider five major categories of cybercrime*, nominate the countries that they consider to be the most significant sources of each of these types of cybercrime, and then rank each country according to the impact, professionalism, and technical skill of its cybercriminals.
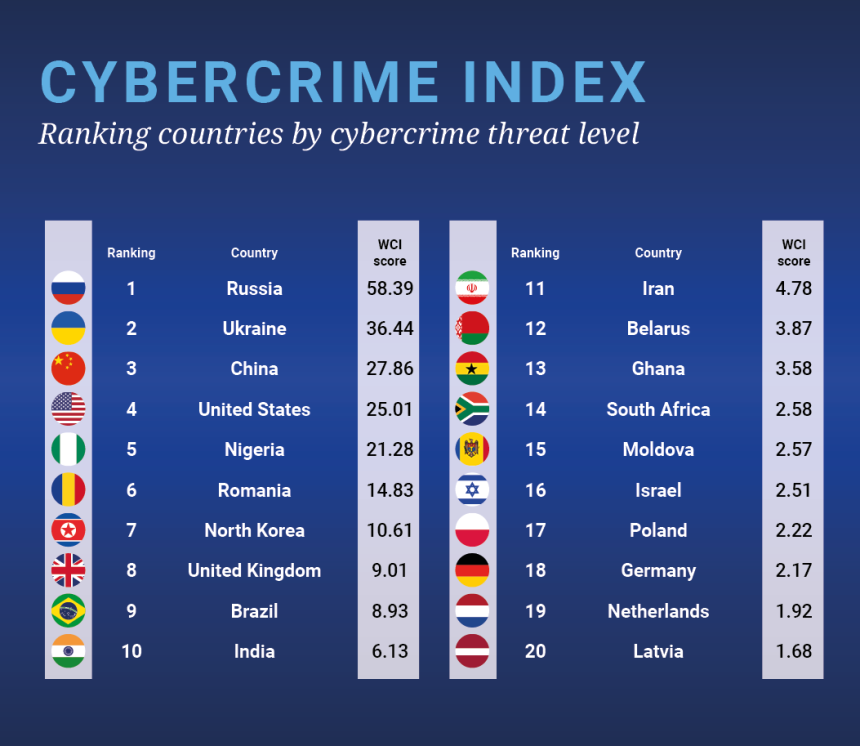
Co-author Associate Professor Jonathan Lusthaus , from the University of Oxford’s Department of Sociology and Oxford School of Global and Area Studies, said cybercrime has largely been an invisible phenomenon because offenders often mask their physical locations by hiding behind fake profiles and technical protections.
'Due to the illicit and anonymous nature of their activities, cybercriminals cannot be easily accessed or reliably surveyed. They are actively hiding. If you try to use technical data to map their location, you will also fail, as cybercriminals bounce their attacks around internet infrastructure across the world. The best means we have to draw a picture of where these offenders are actually located is to survey those whose job it is to track these people,' Dr Lusthaus said.
Figuring out why some countries are cybercrime hotspots, and others aren't, is the next stage of the research. There are existing theories about why some countries have become hubs of cybercriminal activity - for example, that a technically skilled workforce with few employment opportunities may turn to illicit activity to make ends meet - which we'll be able to test against our global data set. Dr Miranda Bruce Department of Sociology, University of Oxford and UNSW Canberra
Co-author of the study, Professor Federico Varese from Sciences Po in France, said the World Cybercrime Index is the first step in a broader aim to understand the local dimensions of cybercrime production across the world.
‘We are hoping to expand the study so that we can determine whether national characteristics like educational attainment, internet penetration, GDP, or levels of corruption are associated with cybercrime. Many people think that cybercrime is global and fluid, but this study supports the view that, much like forms of organised crime, it is embedded within particular contexts,’ Professor Varese said.
The World Cybercrime Index has been developed as a joint partnership between the University of Oxford and UNSW and has also been funded by CRIMGOV , a European Union-supported project based at the University of Oxford and Sciences Po. The other co-authors of the study include Professor Ridhi Kashyap from the University of Oxford and Professor Nigel Phair from Monash University.
The study ‘Mapping the global geography of cybercrime with the World Cybercrime Index’ has been published in the journal PLOS ONE .
*The five major categories of cybercrime assessed by the study were:
1. Technical products/services (e.g. malware coding, botnet access, access to compromised systems, tool production).
2. Attacks and extortion (e.g. denial-of-service attacks, ransomware).
3. Data/identity theft (e.g. hacking, phishing, account compromises, credit card comprises).
4. Scams (e.g. advance fee fraud, business email compromise, online auction fraud).
5. Cashing out/money laundering (e.g. credit card fraud, money mules, illicit virtual currency platforms).

DISCOVER MORE
- Support Oxford's research
- Partner with Oxford on research
- Study at Oxford
- Research jobs at Oxford
You can view all news or browse by category
Cookie Acknowledgement
This website uses cookies to collect information to improve your browsing experience. Please review our Privacy Statement for more information.

- College of Engineering
- News Center
Auburn’s McCrary Institute and Oak Ridge National Laboratory to partner on first regional cybersecurity center to protect the nation’s electricity grid
Published: Apr 18, 2024 10:00 AM
By Taylor Bright
Auburn University’s McCrary Institute for Cyber and Critical Infrastructure Security was awarded a $10 million Department of Energy grant in partnership with Oak Ridge National Laboratory (ORNL) to create a pilot regional cybersecurity research and operations center to protect the electric power grid against cyber attacks. The total value of the project is $12.5 million, with the additional $2.5 million coming from Auburn University and other strategic partners. The center, officially named the Southeast Region Cybersecurity Collaboration Center (SERC3), will bring together experts from the private sector, academia and government to share information and generate innovative real-world solutions to protect the nation’s power grid and other key sectors. It will include a mock utility command center to train participants in real-time cyber defense.
“Auburn University is proud to be at the forefront of this important field as we work against one of the greatest threats the country and the business sector will face in the future,” said Steve Taylor, Auburn University’s senior vice president for research and economic development. “The center will conduct critical research and provide real operational solutions to protect all of us as we address these challenges. We are thankful to Oak Ridge National Laboratory for partnering with us and Rep. Mike Rogers for his support in securing funding for this critical program.”
The center will run experiments with industry partners in a research lab environment to support integration of new and existing security software and hardware into operational environments. Research labs will be established at Auburn University, housed at the Samuel Ginn College of Engineering, and at the Oak Ridge National Laboratory in Oak Ridge, Tennessee.
“We are excited to work with Auburn on this important national mission,” said Oak Ridge National Laboratory Director Stephen Streiffer. “We’re combining our capabilities to partner with industry, develop new security technologies and transfer those technologies to industry, all while developing the workforce that will operate these enhanced systems.”
Workforce and skills development will be a core role of Auburn’s in this partnership.
“This project provides an exciting opportunity for our college and our students,” said Mario Eden, dean of the Samuel Ginn College of Engineering. “Our students will get hands-on experience in a real-world environment. We have a proven track record of innovation and this project perfectly aligns with our mission to provide the best student-centered engineering experience in America and expand our engineering knowledge through research.”
With an emphasis on critical infrastructure, the research will help utilities across the nation become more resilient to the increasing threat of cyberattacks. Puesh M. Kumar, director of the Department of Energy’s Office of Cybersecurity, Energy Security and Emergency Response (CESER), praised the collaboration between organizations. “I applaud Auburn University and Oak Ridge National Laboratory’s collaborative effort to advance grid cybersecurity,” Kumar said. “Everyone must come together – industry, the national laboratories, academia, as well as State and Federal governments – if we are to succeed against the growing cyber threats facing the U.S. energy sector from malicious actors and nation-states like the People’s Republic of China. This partnership is a critical example of that.”
“We know that adversaries want the ability to disrupt our energy infrastructure, which could be devastating for our communities,” said Moe Khaleel, associate laboratory director for National Security Sciences at ORNL. “SERC3 will focus on establishing regional partnerships and developing science-based solutions to mitigate these threats – and keep everyone’s lights on.”
Frank Cilluffo, director of the McCrary Institute, said the project is at the core of what the institute does.
“A secure and resilient grid is a national and regional imperative,” Cilluffo said. “Spearheaded by James Goosby at McCrary and Tricia Schulz at Oak Ridge, we will create new research to rapidly identify, share and mitigate cybersecurity risks while we train the future workforce we need to keep us safe.”
Related Links
The center, officially named the Southeast Region Cybersecurity Collaboration Center (SERC3), will bring together experts from the private sector, academia and government to share information and generate innovative real-world solutions to protect the nation’s power grid and other key sectors.
Featured Faculty
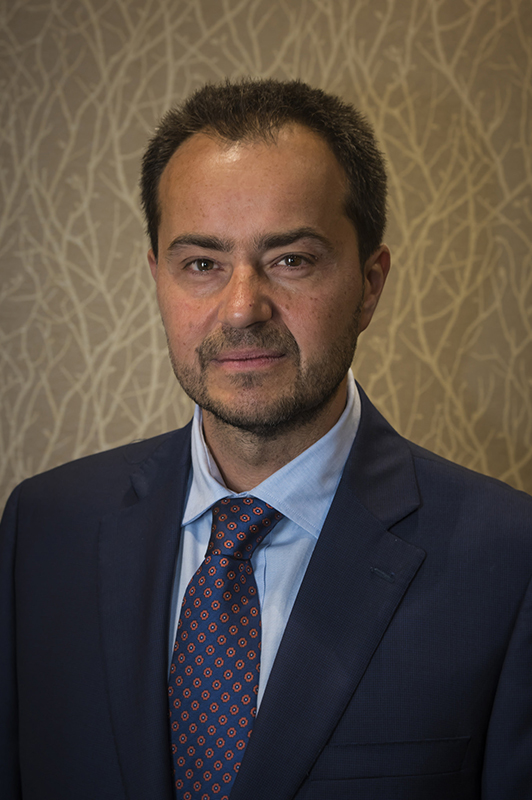
McCrary Institute
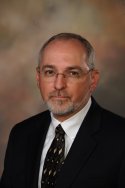
Biosystems Engineering
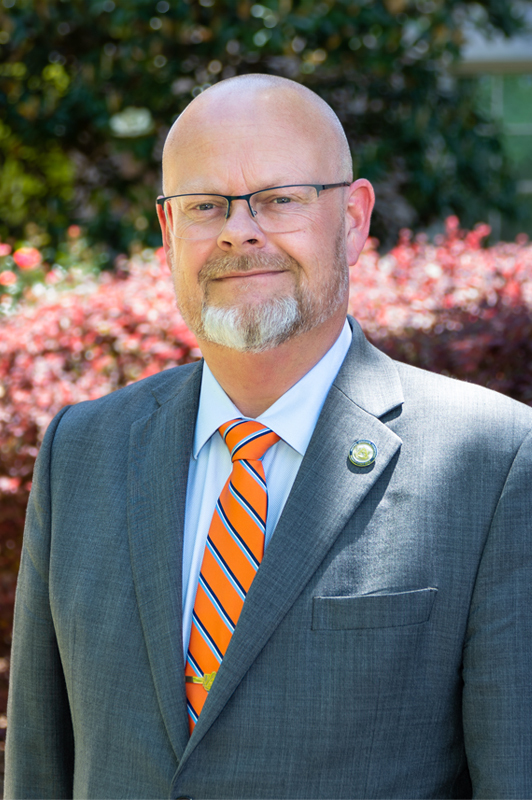
Recent Headlines
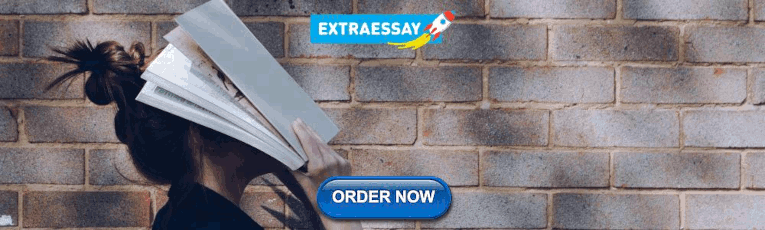
IMAGES
VIDEO
COMMENTS
Cyber Security Research with a focus on the design, development, integration, and deployment of cutting-edge tools, techniques, and systems to support cyber operations. Full time. Starting salary: $69,287 - $122,459. Bachelor's degree. Opportunities for domestic travel are possible.
Cybersecurity analysts are often the first line of defense against cybercrime. Cybersecurity analysts protect computer networks from cyberattacks and unauthorized access. They do this by trying to anticipate and defend against cyber threats, and responding to security breaches when they do happen. In this job, you play a key role in protecting ...
The study is conducted based on a comprehensive study to present the use of artificial intelligence (AI) in cyber security to automate tasks, improve decision-making, and detect threats more effectively than traditional methods. ... Artificial intelligence in cyber security: research advances, challenges, and opportunities. Artif. Intell.
Security researchers study malicious programs such as malware and the processes they use to exploit systems, and then use that insight to address and eliminate vulnerabilities. They compile threat intelligence and analytics, and create data-driven solutions or propose recommended actions that can protect against these malicious programs. They ...
If you're still unsure about how to find a quality research topic, check out our Research Topic Kickstarter service, which is the perfect starting point for developing a unique, well-justified research topic. A comprehensive list of cybersecurity-related research topics. Includes 100% free access to a webinar and research topic evaluator.
Each of these working groups focuses on a unique topic or aspect of cloud security, including AI, IoT, DevSecOps, and much more. Then, every month, research publications created by these working groups and reviewed by the industry are released on the CSA website, free for anyone to download and read. In this article, we've defined 12 CSA ...
In a computing context, cybersecurity is undergoing massive shifts in technology and its operations in recent days, and data science is driving the change. Extracting security incident patterns or insights from cybersecurity data and building corresponding data-driven model, is the key to make a security system automated and intelligent. To understand and analyze the actual phenomena with data ...
As a cybersecurity analyst, you're tasked with protecting your company's hardware, software, and networks from theft, loss, or unauthorised access. At a small company or organisation, you might expect to perform a variety of cybersecurity tasks. At larger organisations, you might specialise as one part of a larger security team.
Artificial intelligence (AI) is one of the key technologies of the Fourth Industrial Revolution (or Industry 4.0), which can be used for the protection of Internet-connected systems from cyber threats, attacks, damage, or unauthorized access. To intelligently solve today's various cybersecurity issues, popular AI techniques involving machine learning and deep learning methods, the concept of ...
Recent research has introduced effective Machine Learning (ML) models for cyber-attack detection, promising to automate the task of detecting, tracking and blocking malware and intruders.
Security Researchers need a deep understanding of cybersecurity threats, exploits, and threat actor techniques involving hardware, software, networks, protocols, and architectures and their implications. They should also be able to use Static Application Security Testing (SAST) tools, debuggers, disassemblers, programming languages , and large ...
In recent times, there have been attempts to leverage artificial intelligence (AI) techniques in a broad range of cyber security applications. Therefore, this paper surveys the existing literature (comprising 54 papers mainly published between 2016 and 2020) on the applications of AI in user access authentication, network situation awareness, dangerous behavior monitoring, and abnormal traffic ...
Cybersecurity research aims to protect computer systems, networks, and data from unauthorised access, theft, or damage. It involves studying and developing methods and techniques to identify, understand, and mitigate cyber threats and vulnerabilities. The field can be divided into theoretical and applied research and faces challenges such as.
Artificial intelligence (AI) is a powerful technology that helps cybersecurity teams automate repetitive tasks, accelerate threat detection and response, and improve the accuracy of their actions to strengthen the security posture against various security issues and cyberattacks.
The capacity to sustain attention to virtual threat landscapes has led cyber security to emerge as a new and novel domain for vigilance research. However, unlike classic domains, such as driving and air traffic control and baggage security, very few vigilance tasks exist for the cyber security domain. Four essential challenges that must be overcome in the development of a modern, validated ...
Next, in Section 4, we elucidate the cybersecurity tasks orthogonal to threat detection that can exploit the capabilities of ML to analyze unstructured data. In contrast to detection problems that require (costly) labels, raw data are abundant in cybersecurity and can also be exploited via ML. ... 6.3 Usable Security Research (Scientific Community)
Cybersecurity is a significant concern for businesses worldwide, as cybercriminals target business data and system resources. Cyber threat intelligence (CTI) enhances organizational cybersecurity resilience by obtaining, processing, evaluating, and disseminating information about potential risks and opportunities inside the cyber domain. This research investigates how companies can employ CTI ...
Therefore, the security tasks and functions of each country are increasingly affected by cyberspace ... Cyber-security policy may require that "when the risk of disclosure of confidential ... Manz D.O. (Eds.), Research Methods for Cyber Security, Syngress (2017), pp. 33-62 (Chapter 2) View PDF View article Google Scholar. Furnell and Shah ...
Cyber Security Research Topics. Cyber Security Research Topics are as follows: The role of machine learning in detecting cyber threats. The impact of cloud computing on cyber security. Cyber warfare and its effects on national security. The rise of ransomware attacks and their prevention methods.
In Machine Learning, the datasets used to build models are one of the main factors limiting what these models can achieve and how good their predictive performance is. Machine Learning applications for cyber-security or computer security are numerous including cyber threat mitigation and security infrastructure enhancement through pattern recognition, real-time attack detection, and in-depth ...
The term cybersecurity refers to a set of technologies, processes and. practices to protect and defend networks, devices, software and data. from attack, damage or unauthorized access [1 ...
The concept of cybersecurity refers to cracking the security mechanisms that break in dynamic environments. Implementing Cyber Security Project topics and cyber security thesis topics/ideas helps overcome attacks and take mitigation approaches to security risks and threats in real-time. Undoubtedly, it focuses on events injected into the system, data, and the whole network to attack/disturb it.
This paper review 27 articles on cyber security and cybercrimes and it showed that cyber security is a complex task that relies on domain knowledge and requires cognitive abilities to determine ...
2024 Security Report: Podcast Edition. April 18, 2024. Once every year, Check Point releases an annual report reviewing the biggest events and trends in cybersecurity. In this episode we'll break down the latest iteration, focusing on its most important parts, to catch you up on what you need to know most in 2024.
Recurring increase in cyber attacks: Q1 2024 saw a marked 28% increase in the average number of cyber attacks per organization from the last quarter of 2023, though a 5% increase in Q1 YoY Sustained Industry Attacks focus: The Hardware Vendor industry saw a substantial rise of 37% cyber attacks YoY, as the Education/Research, Government/Military and Healthcare sector maintained their leads as ...
The "Absolute Security Cyber Resilience Risk Index 2024," will help CISOs and other security and risk professionals to learn about what Cyber Resilience is, the security and risk benefits it provides, whether risk factors impacting resilience are present in their environments, and how to take mitigation steps. With access to telemetry from more ...
Large language models (LLMs) introduce new security risks, but there are few comprehensive evaluation suites to measure and reduce these risks. We present CYBERSECEVAL 2, a novel benchmark to quantify LLM security risks and capabilities. We introduce two new areas for testing: prompt injection and code interpreter abuse.
Microsoft research shows that when using Copilot for Security, analysts were 26% faster across all tasks. That is the equivalent of adding an analyst to every team of four. That is the equivalent ...
Co-author of the study, Dr Miranda Bruce from the University of Oxford and UNSW Canberra said the study will enable the public and private sectors to focus their resources on key cybercrime hubs and spend less time and funds on cybercrime countermeasures in countries where the problem is not as significant. 'The research that underpins the Index will help remove the veil of anonymity around ...
Auburn University's McCrary Institute for Cyber and Critical Infrastructure Security was awarded a $10 million Department of Energy grant in partnership with Oak Ridge National Laboratory (ORNL) to create a pilot regional cybersecurity research and operations center to protect the electric power grid against cyber attacks. The total value of the project is $12.5 million, with the additional ...