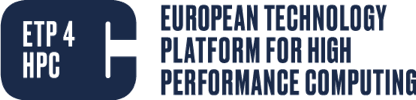
- MEMBER AREA

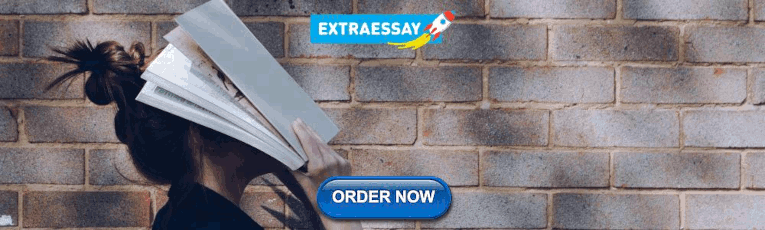
Strategic Research Agenda (SRA)
Current edition is SRA 5 , the fifth edition of the SRA, published in October 2022. Read it online below or download the PDF .
What is the SRA?
The roadmapping of the European HPC technology research is the main aim of our Association and the ETP4HPC Strategic Research Agenda (SRA) is our key deliverable. We have now issued five instances of our SRA, which have served as the basis for the HPC R&D Work Programmes to date. We make this SRA available to the EuroHPC JU and in particular its RIAG (Research and Innovation Advisory Group) with a view to basing the European HPC technology research programme on the findings of this SRA.
For SRA5, we have organised the experts - representing the members of ETP4HPC - who would like to contribute to this SRA into the following Working Groups.
ETP4HPC Strategic Research Agenda 5 (SRA 5) Working Groups
- System Architecture - leaders Laurent Cargemel (Atos), Estela Suarez (JSC), Herbert Cornelius (Megware)
- System Hardware Components - leaders Marc Duranton (CEA - HiPEAC), Sakir Sezer (NVIDIA), Craig Prunty (SIPEARL)
- System Software and Management - leaders Pascale Rosse-Laurent (Atos), Maria Perez (UPM - BDVA), Manolis Marazakis (FORTH)
- Programming Environment - leaders Guy Lonsdale (Scapos), Paul Carpenter (BSC), Gabriel Antoniu (INRIA - BDVA)
- I/O & Storage - leaders Andre Brinkmann (JGU - Mainz), Sai Narasimhamurthy (Seagate)
- Mathematics & Algorithms - leaders Dirk Pleiter (KTH), Utz-Uwe Haus (HPE)
- Application Co-design - leaders Erwin Laure (MPCDF), Andreas Wierse (SICOS)
- Centre-to-edge-framework - leaders Jens Krueger (Fraunhofer), Hans-Christian Hoppe (Scapos)
(the following two groups are new in SRA5)
- Quantum for HPC - leaders Valeria Bartsch (Fraunhofer), Cyril Allouche (Atos), Walter Ries (IBM Research), Kristel Michelsen (JSC)
- Non-conventional HPC Architectures - leaders Johannes Schemmel (KIP-HD), Steve Furber (University of Manchester, Oskar Mencer (Maxeler), Tobias Becker (Maxeler), Robert Haas (IBM Research)
While preparing SRA5, ETP4HPC regularly issued White Papers, short documents tackling technical issues pertaining to European HPC. The findings of the White Papers were re-used in the SRA and others streams of work such as the TransContinuum Initiative . The full list of White papers is available here>>
A bit of history
ETP4HPC maintains the SRA on a continuous basis. The first SRA was issued in 2013 and we published an update (SRA 2) in November 2015. SRA 3 was published in December 2017, and our latest SRA 4 at the beginning of 2020.
The SRA is the work of experts associated with ETP4HPC. We also welcome comments from other stakeholders and experts. In order to facilitate this process we open Public Calls for Comments .
The SRA reflects the needs of the European HPC Technology providers and other members of the European HPC ecosystem, i.e.: PRACE (academic users), industrial HPC end users, Independent Software Vendors. We have also used the conclusions made available by other initiatives such as HiPEAC, EESI and PlanetHPC. We also talk to the Big Data community (represented by the Big Data Value Association).
The roadmap is developed through a series of internal and external workshops. A team of ETP4HPC experts supervise and manage the process, whilst the technical areas are developed by Working Groups led by members of ETP4HPC. All members of ETP4HPC can participate in the development of the SRA.
- Strategic Research Agenda
- ETP4HPC White Papers
- Processing in Memory
- Integrated Ecosystems for Edge-Cloud-HPC Continuum
- Task-Based Performance Portability in HPC
- Quantum for HPC
- HPC for Urgent Decision-Making
- Heterogeneous High Performance Computing
- Federated HPC, cloud and data infrastructures
- Unconventional HPC Architectures
- Modular Supercomputing Architecture
- SRA 4 (2020)
- HPC in Digital Continuum
- HPC Vision 2019 - Blueprint
- ETP4HPC and Big Data
- SRA 3 (2017)
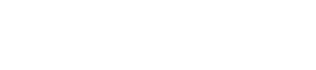
- Privacy Policy

- Open access
- Published: 28 January 2019
Strategic research agenda for biomedical imaging
The european institute for biomedical imaging research (eibir).
Insights into Imaging volume 10 , Article number: 7 ( 2019 ) Cite this article
6493 Accesses
6 Citations
5 Altmetric
Metrics details
This Strategic Research Agenda identifies current challenges and needs in healthcare, illustrates how biomedical imaging and derived data can help to address these, and aims to stimulate dedicated research funding efforts.
Medicine is currently moving towards a more tailored, patient-centric approach by providing personalised solutions for the individual patient. Innovation in biomedical imaging plays a key role in this process as it addresses the current needs for individualised prevention, treatment, therapy response monitoring, and image-guided surgery.
The use of non-invasive biomarkers facilitates better therapy prediction and monitoring, leading to improved patient outcomes. Innovative diagnostic imaging technologies provide information about disease characteristics which, coupled with biological, genetic and -omics data, will contribute to an individualised diagnosis and therapy approach.
In the emerging field of theranostics, imaging tools together with therapeutic agents enable the selection of best treatments and allow tailored therapeutic interventions.
For prenatal monitoring, the use of innovative imaging technologies can ensure an early detection of malfunctions or disease.
The application of biomedical imaging for diagnosis and management of lifestyle-induced diseases will help to avoid disease development through lifestyle changes.
Artificial intelligence and machine learning in imaging will facilitate the improvement of image interpretation and lead to better disease prediction and therapy planning.
As biomedical imaging technologies and analysis of existing imaging data provide solutions to current challenges and needs in healthcare, appropriate funding for dedicated research is needed to implement the innovative approaches for the wellbeing of citizens and patients.
Innovative biomedical imaging plays a crucial role in personalised medicine, disease prevention and therapy monitoring
Innovative biomedical imaging technologies and analysis of existing imaging data provide solutions to current challenges and needs in healthcare
Appropriate funding for biomedical imaging research is necessary to implement innovative approaches addressing citizens’ and patients’ needs
Introduction
Medicine and healthcare in general are experiencing a major change, moving from reactive to proactive approaches by providing predictive, preventive and personalised medical solutions for the individual patient. Particularly, the concept of personalised medicine is promoted by the European Commission as it addresses the challenges of ineffective treatment and rising healthcare costs with patient-centred prevention and treatment plans. Personalised and precision medicine has a huge growth potential, which provides Europe’s healthcare industry with the opportunity to further expand its leading position, contributing to economic growth and job creation.
Furthermore, Europe needs research, innovation and applications for the benefit of all. In this context, areas of research have to be prioritised and research programmes should focus on high-impact outcomes [ 1 ]. In its recently launched work programmes [ 2 ], the European Commission has identified a number of healthcare challenges, e.g. the rising costs of health and care or the influence of external environmental factors including climate change on health, that need to be addressed by Europe’s research community to develop sustainable solutions that will help to overcome those challenges, improve the treatment of patients and lead to better health and wellbeing for European citizens. The development and adoption of good-quality and safe e-services can ensure that the health systems of Member States become more efficient and sustainable [ 3 ].
However, as stated in the European Council’s conclusions on Health in the Digital Society [ 4 ], new opportunities are arising from big data and improved data analytics capabilities, yet barriers to scaling up the potential in digital health and connected care still need to be overcome. These include the dominance of data silos, lack of interoperability and of common standards for measuring clinical and patient reported outcomes, and limited access and use of large databases for research and innovation purposes. Moreover, a lack of funding and financial incentives exists, which together with market fragmentation in the EU and across the spectrum of services further impedes innovation. Progress in implementing data-driven digital solutions in healthcare requires rigorous validation and testing of new solutions’ clinical effectiveness.
It is the aim of this Strategic Research Agenda for Biomedical Imaging to:
Identify the current challenges and needs in medicine and healthcare
Illustrate how biomedical imaging can help address these challenges
Stimulate dedicated research funding efforts
Innovative imaging technologies are now allowing researchers to visualise, characterise and measure biological and molecular phenomena with a hitherto unattainable precision. For the first time in history, hallmark processes of human disease, such as tumourigenesis or molecular deposits, can be visualised in real time. Imaging technologies and computerised radiomics allow breakthrough discoveries and their application in healthcare and are thus the central tool driving fundamental research in biomedicine. Technological innovation in biomedical imaging has been growing in the last decades and significant advances in the research fields of photonics, physics, chemistry and computing are often quickly translated into instrumentation or procedures for biomedical imaging. This may accelerate medical advances, but also calls for a need to adapt existing and to implement new methods and care pathways for better patient outcomes.
Biomedical imaging is a key component in personalised medicine [ 5 ]:
Personalised prevention will rely on non-invasive or minimally invasive image-based screening programmes [ 5 ]
Structural, functional, physiological and molecular imaging biomarkers affect decisions on the type and intensity of treatment [ 5 ]
Treatment response assessment with imaging biomarkers will improve personalised and targeted treatment [ 5 ]
Imaging-supported non- or minimally invasive intervention integrates precision diagnosis and personalised treatment [ 5 ] and will contribute to value-based healthcare [ 6 ]
Moreover, biomedical imaging is a forerunner in the digital transformation of healthcare and a leading discipline in the adoption of new innovative solutions like deep learning and artificial intelligence. Digital health is essential for ensuring that breakthroughs or innovations in clinical research are translated into practice cost-effectively.
The European Institute for Biomedical Imaging Research (EIBIR) is a non-profit organisation with a network of more than 80 leading European research institutions and 11 European scientific societies related to biomedical imaging as shareholders. EIBIR is committed to coordinate and support the development of biomedical imaging technologies and the dissemination of knowledge with the ultimate goal of improving the diagnosis, treatment and prevention of disease. It supports research networking activities and plays a key role in spreading good practice and promoting common initiatives and interoperability in the field of biomedical imaging research ( www.eibir.org ).
EIBIR, together with its members and shareholder organisations, has identified five main challenges which biomedical imaging can help overcome:
Challenge 1 : Meeting the healthcare demands of Europe’s population through personalised disease prevention and therapy monitoring facilitated by medical imaging
Challenge 2 : Developing new disease-specific, targeted and image-guided therapies
Challenge 3 : Contributing to a healthy start by delivering early and improved information on foetal health and prenatal growth, and preventing the development of anomalies using advanced imaging technologies
Challenge 4 : Providing accurate assessments of the impact of lifestyle and environmental factors on health supported by medical imaging
Challenge 5 : Making Europe the world leader in machine-learning and artificial intelligence in medical imaging by exploiting existing data and expertise to implement digital solutions after rigorous clinical validation.
Challenge 1: Meeting the healthcare demands of Europe’s population through personalised disease prevention and therapy monitoring facilitated by medical imaging
Precision medicine has emerged as a novel healthcare paradigm during the past decade.
The approach for risk prediction and disease prevention taking into account individual gene variability as well as environmental and lifestyle factors involves the integration of information from multiple sources to achieve patient population stratification and provide more specific diagnoses, focused treatment and better response assessment. Deep phenotyping in combination with genetic, biochemical and physiologic biomarkers allows prediction and early assessment of disease. The emerging field of radiomics links genotypic information to phenotypic disease manifestations using imaging. Radiomics can greatly contribute to patient-tailored prevention and care.
Special attention should be devoted to the development of safe and more specific contrast agents, and the optimisation of acquisition procedures to the characteristics of the treated patient/pathology enabling precision radiology.
Clinically oriented research on quality and safety of medical imaging is an essential aspect when advancing precision radiology. In 2017, five EIBIR shareholder organisations established the European Alliance for Medical Radiation Protection Research (EURAMED; www.euramed.eu ) and published a strategic research agenda for radiation protection in medicine [ 7 ], highlighting the research needs in this field and encouraging sustainable European efforts.
Imaging biomarkers are objectively measurable indicators of biological processes and can be used for the prediction of patient outcome (regardless of therapy) and response to specific therapies, and for response assessment (monitoring) [ 8 ]. The use of imaging biomarkers is non-invasive—a clear advantage over invasive biopsies [ 8 ]. Another advantage is the assessment and quantification of cellular targets for the entire disease burden, avoiding sampling errors that can occur with heterogeneous expressions, as well as the potential for serial studies of the in vivo effects of a drug or action on the target [ 8 ]. In addition, imaging biomarkers can unravel specific localised biological pathways and enable therapeutic targeting of such specific biomolecular mechanisms [ 8 ].
Joint efforts between research institutions and technology vendors should lead to the implementation of standards in image acquisition, production, archiving and distribution, and ethical use of sensitive data. Such approaches are a prerequisite for sharing large imaging data sets across Europe and for the discovery, adoption and wider use of predictive, prognostic and diagnostic imaging biomarkers. The European Imaging Biomarker Alliance (EIBALL) is a joint initiative operating in conjunction with EIBIR and in collaboration with the Quantitative Imaging Biomarkers Alliance (QIBA) and the European Organisation for Research and Treatment of Cancer (EORTC) for the development and validation of new imaging biomarkers.
Examples addressing Challenge 1
Example 1: brca1/2 carriers, enhanced imaging-based monitoring for breast and ovarian cancer (conventional mammography vs. contrast-enhanced mri).
Persons carrying mutations in the BRCA1/2 gene are more likely to develop breast cancer (men and women), ovarian cancer in women and prostate cancer in men. Genetic screening of high-risk families allows the possibility of early detection of disease or indeed prevention. There is now compelling evidence that MRI is the most appropriate screening test for breast cancer in BRCA1/2 carriers. However, screening programmes are predominantly based on mammography. Future screening programmes are needed to fully evaluate the position of MRI in screening women in high-risk categories. In ovarian cancer (Fig. 1 ), but also in other cancers such as prostate cancer, biomarkers of early disease in BRCA carriers, such as circulating DNA, are highly likely to form the basis of future screening in BRCA carriers in conjunction with imaging tests, including ultrasound (US) and MRI. Imaging has been highly successful in determining the likelihood of malignancy in ovarian and prostate masses. Research into translating this to screening practice is of utmost importance, particularly in relation to early detection of disease and treatment stratification by combining circulating and imaging biomarkers.
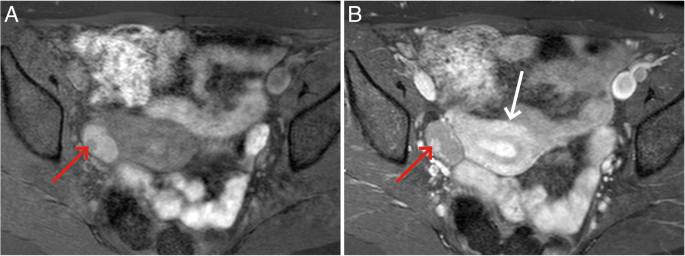
MRI scan of early-stage 1A high-grade serous ovarian cancer. The BRCA1-positive patient was identified through ultrasound screening, confirmed by MRI. The right ovary contains as cyst with an enhancing nodule (red arrows) demonstrated on the T1 fat-saturated image before ( a ) and after ( b ) contrast administration. The nodule enhances brightly, similar to the adjacent uterine myometrium (white arrow). Detection of ovarian cancer at this early stage is associated with a significantly improved 5-year survival rate compared with later stages of disease (courtesy: A. Rockall)
Example 2: Development of arterial spin labelling as a brain imaging biomarker
Arterial spin labelling (ASL) to measure perfusion has been at the forefront of physiological imaging without the use of contrast agents for more than three decades now. In spite of its obvious advantages in numerous diseases, such as brain tumours (Fig. 2 ), dementia or stroke, ASL has remained underused. In particular, it has been shown [ 9 ] that relative tumour blood flow values can be used as an independent biomarker of glioma grading, while the same biomarker can accurately distinguish pseudo-progression from true progression, thereby providing a very important tool for therapy monitoring [ 9 ]. In 2014, a landmark paper established a roadmap for all manufacturers and users of this technology. Based on this, an on-going effort is taking place to establish a jointly developed EIBALL-QIBA profile detailing guidelines on how to use ASL in clinical practice. As part of this initiative, a commercial ASL calibration phantom was recently launched, together with an online calibration tool. For ASL to finally become accepted, it will be critical for new studies to show the repeatability and reproducibility of the assessment of perfusion over time and its increased usefulness in many clinical neurological affections.
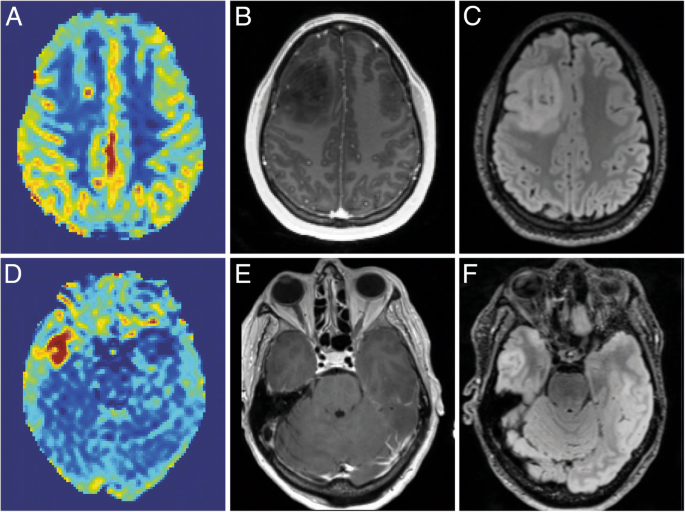
a – c Example of a 24-year-old female patient, with oligodendroglioma (grade II) in the left frontal lobe. a Cerebral blood flow, imaged by ASL. Red indicates high blood flow. b T1-Gd and c FLAIR. d – f Example of a 63-year-old female patient with GBM (grade IV) in the right temporal lobe. d Cerebral blood flow, imaged by ASL. Red indicates high blood flow. e T1 post Gd. f FLAIR. A clear difference in tumour blood flow can be seen between both patients (courtesy: A. Alsaedi, S. Bisdas)
Challenge 2: Developing new disease-specific, targeted and image-guided therapies
Biomedical imaging is an indispensable tool in personalised medicine providing information on localisation, extent, homogeneity and aggressiveness of disease.
Diagnostic imaging procedures are increasingly used to support individualised and targeted treatment and represent a mainstay of the progress of personalised medicine [ 10 ]. This may include:
The use of an imaging technology to monitor a therapy [ 10 ]
The administration of an imaging agent acting as a drug surrogate to predict the biodistribution of the drug [ 10 ]
The co-administration of both a drug and an imaging agent to assess in real time the delivery/release of the therapeutic agent at the pathological site [ 10 ]
The use of an imaging agent to guide surgeons or interventional radiologists in a (minimally) invasive procedure [ 10 ].
Recent advances in molecular biology proved that drug response is often a result of different genetic alterations, but can also arise from micro-environmental and microbiome exposure. Altered environmental and genetic factors can facilitate cancer progression and may also influence drug effectiveness, if gene mutations are involved in drug metabolism. A better understanding of the disease biology as well as molecular changes and altered signalling pathways will enable the identification of patients who may benefit from such treatments. It will catalyse the development of targeted therapies which counter the influence of specific molecular drivers contributing to the development and spread of disease, providing the foundation of precision medicine.
Theranostics is a novel emerging field of treatment in which diagnostic imaging tools coupled with therapeutic agents allow precise targeting of a disease at a molecular level. Theranostics enables tailored interventions, further personalising healthcare practices to individual patients for whom a standard therapy is not suitable. However, more work is needed to identify novel theranostic combinations. New diagnostic tracers can be tailored to the specific needs of stratified patient groups or to rare diseases for which other therapeutic approaches are not yet available.
For most currently available radionuclide therapies, a fixed amount of radioactivity is administered, regardless of patient weight or body surface area. Although these empirical dosing methods are well-established, safe and effective, the theranostic approach offers the possibility for an upfront evaluation of the biodistribution, quantification and calculation of the absorbed dose to the target volumes and critical organs. The development of a personalised dosimetry-based approach might further improve the outcome and cost benefit of radionuclide therapies. Although dosimetry is essential for the development of radiopharmaceuticals, its potential and clinical use to tailor the administered amount of radioactivity for each individual patient still needs to be established and evaluated by randomised controlled trials comparing dosimetry-based versus fixed-activity approaches.
Examples addressing Challenge 2
Example 3: theranostics for treatment of metastatic pancreatic cancer.
Figure 3 shows an example of theranostics in a patient with a metastatic pancreatic neuroendocrine tumour. In the first panel, staging with 68 Ga-Dotatate-PET shows a high expression of somatostatin receptors in the pancreatic tumour, locoregional lymph nodes and liver metastases. Panel b shows evaluation with SPECT after treatment with 177 Lu-Dotatate, confirming adequate targeting of the tumour sites. The end-of-treatment 68 Ga-Dotatate-PET scan (panel c) shows a partial remission, particularly of the metastases in the liver. A recent phase III study shows that treatment with 177 Lu-Dotatate results in a significantly higher response rate and longer progression-free survival than high-dose octreotide among patients with advanced midgut neuroendocrine tumours. A first interim analysis shows preliminary evidence of an overall survival benefit as well [ 11 ]. Similar theranostic approaches, such as 68 Ga-/ 177 Lu-PSMA in prostate cancer, are currently under evaluation in clinical trials with promising preliminary results.
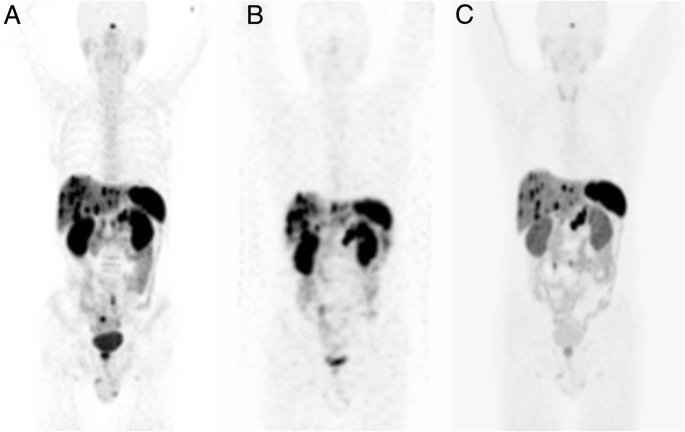
Theranostics in a patient with a metastatic pancreatic neuroendocrine tumour. a 68 Ga-Dotatate-PET scan for staging. b SPECT evaluation after treatment. c End-of-treatment 68 Ga-Dotatate-PET scan (courtesy of J. Kunikowska, Warsaw)
Example 4: Cell recruitment to detect and target cancer for therapy
Recent studies have shown that it is possible to use fibroblast recruitment as a tool for detection of cancer and targeted therapy [ 12 ]. Cells like fibroblasts labelled with a fluorescent reporter can be used as beacons for guidance of biopsy or surgery, as well as for therapy (Fig. 4 ). This figure illustrates study results that show fibroblasts migrate towards cancer cells. Additionally, a therapeutic effect could be seen in this study. Delivery of the labelled cells stopped the production of ascitic fluid in the abdomen in an orthotropic model of ovarian cancer metastasis and prolonged survival in mice.
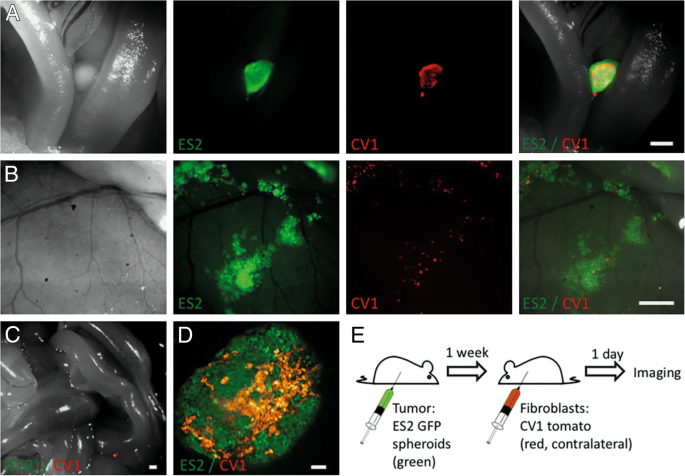
Fluorescence imaging of a mouse showing fibroblast (red) migration from injection site to location of tumour cells (green). ( a ) Fibroblast recruitment to tumour injection site next to intensine. ( b ) Fibroblast recruitment to tumour patches attached to the peritoneal wall. ( c ) Fibroblast scattered in the abdomen of a control mouse without tumour injection. ( d ) High resolution image of fibroblasts recruited to a tumour. ( e ) experimental design (green) [ 12 ]
Challenge 3: Contributing to a healthy start by delivering improved information on foetal health and prenatal growth and preventing development of anomalies using advanced imaging technologies
Biomedical imaging plays an important role in assessing foetal and prenatal health.
The placenta is one of the most important organs influencing not just the health of a woman and her foetus during pregnancy, but also the lifelong health of both. When it malfunctions, serious problems such as gestational diabetes, preterm labour and stillbirth can occur. Placental dysfunction may also lead to health problems later in life for both mother and child. The early diagnosis of foetal malformations and growth anomalies has the potential to improve foetal prognosis by granting a treatment plan and by making specialist units and treatments from birth accessible. The diagnostic accuracy of ultrasound (US), the currently most-used imaging modality, is however limited.
Novel imaging technologies should be used in clinical settings. The use of advanced US tools (e.g. elastography), optoacoustic imaging and quantitative MRI can be essential for the assessment of placenta function, transport of nutrients, oxygen, and therapeutics across the placenta while also detecting aberrations in foetal development from implantation to birth. Studies should focus on maternal and foetal risk models including genetic, infectious and environmental influences for disease prevention.
Enhanced prenatal monitoring has the potential for early detection and intervention to reduce maternal and foetal morbidity and mortality. Furthermore, among the diseases and anomalies encountered in high-risk patients during pregnancy, e.g. preeclampsia, intrauterine growth restriction, multi-foetal pregnancies, increased maternal age and infection (e.g. ZIKA virus) can be diagnosed and monitored by imaging. In general it can be expected that further advances in imaging hold a striking promise for the study of the placenta and the foetus and emerging technologies can be translated to other organs and open new research avenues for the benefit of human health.
Examples addressing Challenge 3
Example 5: foetal and perinatal imaging (us and mri).
Imaging techniques for prenatal imaging should be developed to identify in utero foetal growth disturbance, including foetal growth restriction and movement disorders. Normal foetal movement in the womb is critical to normal musculoskeletal development, and novel imaging strategies can play a critical part in diagnosis and monitoring of these diseases. For example, a spectrum of techniques including US elastography, micro CT, quantitative CT and MRI can be used to develop models of normal foetal movement (Fig. 5 ), against which diseases such as developmental hip dysplasia and foetal akinesia can be assessed. Early diagnosis and recognition will help reduce the huge societal and financial impact these diseases have on chronic musculoskeletal conditions in childhood, in combination with neonatal screening programmes for, e.g. hip dysplasia. The understanding and modelling of relationships between concurrent developments such as movement, skeletal system and the brain are key to the exploitation of perinatal imaging.
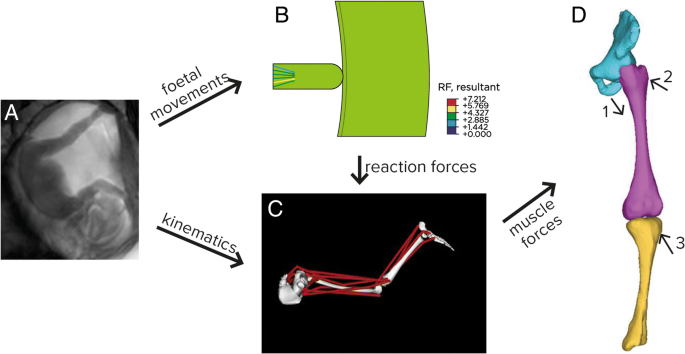
Computational pipeline for developing stress modelling of foetal movements. Foetal joint movements are tracked in utero ( a ), with finite element modelling of reaction forces ( b ) combined with musculoskeletal modelling to predict muscle forces ( c ) which are then applied to finite element models of foetal geometries ( d ). Adapted from [ 21 ]
Example 6: BOLD imaging of the placenta
While structural imaging of the foetus and the placenta are technically established, though not widely available, the assessment of placental function during pregnancy is currently a field of research. The gas and nutrient exchange between the mother’s and the foetus’ blood circulation via the placenta is essential for the development of the foetus. Early delivery is recommended for placental dysfunction but has to be weighed against the risks of preterm birth. Oxygen exchange is a most relevant function of the placenta and, due to the high blood volume methods adopted from functional brain imaging with MRI, has been proposed to evaluate placental function (Fig. 6 ). During an oxygen challenge, the change in blood oxygen level-dependent (BOLD) signal is measured. In particular, the time constant of the related oxygen change on the foetal blood circuit side of the placenta has been shown in early studies to be related to birth weight or foetal brain and liver volumes. Biomarkers derived from US measurements of the placenta and foetus have not correlated similarly well to postnatal pathologic assessment of the placenta as BOLD MRI variations. The quantitative determination of placental oxygenation remains a challenge since the BOLD signal depends on many parameters that are difficult to assess non-invasively.
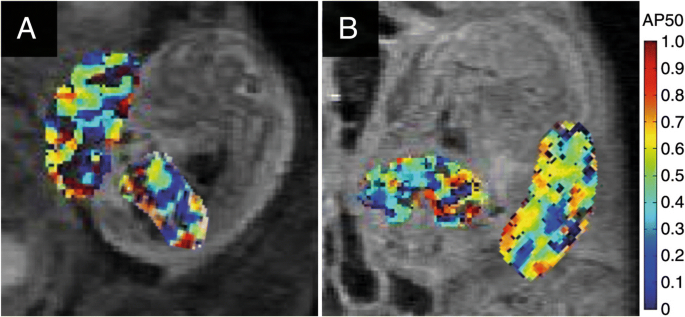
BOLD imaging analysis of pregnant mice. Representative spatial distribution maps of the oxygen-haemoglobin dissociation inside the placenta and foetal liver on days 14.5 ( a ) and 17.5 ( b ) show distribution and variability [ 22 ]
Challenge 4: Providing accurate assessment of lifestyle and environmental impact factors on health supported by medical imaging
The impact of lifestyle and environmental factors can be assessed by medical imaging.
According to the WHO [ 13 ], 60% of the factors contributing to individual health and quality of life are correlated with lifestyle. Millions of people follow an unhealthy lifestyle and encounter illness, disability and even death because of it. Problems such as metabolic diseases, joint and skeletal pathologies, cardiovascular diseases, hypertension and violence can be caused by an unhealthy lifestyle. The relationship between lifestyle and health should be considered as established. Also the interaction between human health and the environment has been extensively studied and environmental risks have been proven to significantly impact human health, either directly by exposing people to harmful agents, or indirectly by disrupting life-sustaining ecosystems. Although the exact contribution of environmental factors to the development of disease and death cannot be precisely determined, the WHO has estimated that 13 million deaths annually are attributable to preventable environmental causes [ 14 ].
The timely use of diagnostic imaging tools as a preventive measure, followed by lifestyle changes, can prevent disease development [ 15 ]. Due to the increasing prevalence of obesity and its metabolic manifestations, it is important to obtain patient-specific quantitative body composition knowledge to predict and prevent disease [ 16 ]. The role of metabolically active tissues such as brown adipose tissue needs to be studied with new imaging methods to measure both mass and metabolic activity, enabling accurate assessments of energy turnover under normal and stimulated conditions. The identification of management strategies in cardiovascular diseases (CVDs) is of utmost importance, as the WHO projected that in 10 years from now more than 23 million people will die annually due to CVDs.
The use of biomedical imaging is essential due to its strategic advantages in diagnostic and therapeutic decision-making and as it delivers inputs for different stages of disease management, including prediction, screening, early diagnosis, staging, prognosis and follow-up. Deep phenotyping using imaging in combination with information acquired from wearable sensors has the significant potential to monitor subjects and patients during daily activities, providing information on external factors influencing biological processes at different levels.
Examples addressing Challenge 4
Example 7: body fat imaging with quantification.
The increase in obesity and metabolic-related diseases is one the challenges for global healthcare systems. Fat accumulation in organs and muscles is a strong biomarker of diabetes, the metabolic syndrome and obesity. A comprehensive understanding of the volume, distribution and quality of fat and muscles in the human body is important for diagnosis and treatment of illness. There is a need to develop automated segmentation and quantification of muscle and fat tissue volumes in the body (Fig. 7 ) which can be addressed by MRI. This technique has the potential to foster links between endocrine and cancer MRI disciplines and to develop biomarkers of morbidity in cancer survivors and links between disease entities such as body fat, diabetes, cancer and sarcopenia. In addition, the amount of subcutaneous and visceral adipose tissue in the abdomen plays an essential role in the determination of health risks.
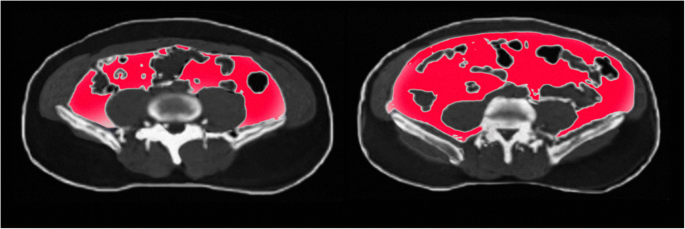
Men matched for the same BMI and total body fat: Differing ‘adiposity phenotypes’ regarding visceral obesity and subcutaneous obesity and with different risk profiles (courtesy: A. Persson)
Example 8: MR phenotyping in diabetes and cardiovascular diseases
Imaging plays a highly promising role in individualised risk assessment, particularly regarding population-relevant diseases such as diabetes mellitus and hypertension. In subjects with established diabetes or hypertension, there is strong evidence that the level of detectable subclinical disease burden, such as an altered myocardial perfusion and/or delayed enhancement as assessed by MRI, is highly variable among patients and has strong prognostic relevance beyond left and right ventricular function for the occurrence of cardiovascular events. Thus, a more personalised risk stratification is clearly attainable. Less is known in subjects with prediabetes or pre-hypertensive stages of the disease, although there is early evidence that prediabetes is associated with increased subclinical disease burden, such as carotid plaque volume and arterial stiffness by ultrasound, changes of left ventricular function by echocardiography, or findings on native or contrast-enhanced cardiac MRI (Fig. 8 ). However, these observations lack a prospective design or reference group, comprise significant selection bias or are not generalisable to a preventive setting [ 17 ]. In order to confirm these early findings and establish their role in improving clinical care, further cross-sectional and longitudinal research in specific cohorts of patients is strongly warranted [ 17 ]. This may include prospective or nested cohort studies and randomised diagnostic trials [ 17 ]. As such, medical imaging may also add to the understanding of the relationship between changes in the phenotype and the individual predisposition for metabolic and cardiovascular diseases or the interplay with neurovascular diseases from a pathophysiology point of view. Accurate MRI evaluation of fat deposits within the liver and pancreas are also critical hallmarks of the metabolic syndrome.
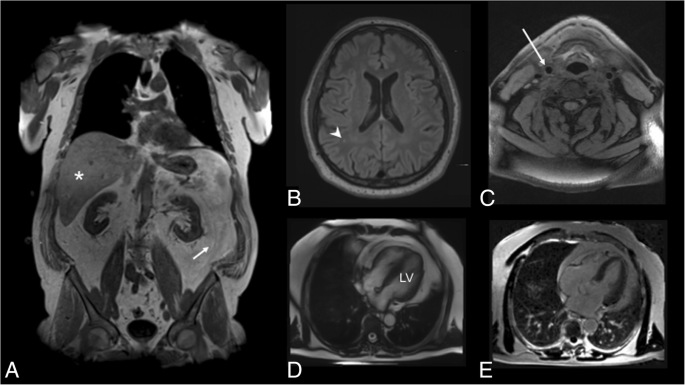
Imaging findings in a 61-year-old male indicating extensive subclinical disease burden. a Two-point DIXON T1-weighted sequence for the assessment of visceral adipose tissue volume from the femoral head to the cardiac apex (arrow) indicating high levels of fat as well as hepatic proton density fat fraction (asterisk, measured on multi-echo VIBE T1-weighted sequences). b Fluid-attenuated inversion recovery sequences demonstrating mild white matter lesions (arrowhead). c Atherosclerotic carotid plaque was determined on black-blood T1-weighted fat-suppressed sequences in the common carotid artery (arrow), the carotid bulb and the proximal internal carotid artery. d Cine-SSFP sequences were evaluated for the calculation of volume and mass left ventricle (LV). e late gadolinium enhancement was detected on fast-low-single-shot inversion recovery sequence four-chamber view [ 23 ]
Challenge 5: Making Europe the world leader in machine learning and artificial intelligence in medical imaging by exploiting existing data and expertise to implement digital solutions after rigorous clinical validation
Machine learning and artificial intelligence can substantially improve disease diagnostics and prediction.
Medical image data has been accumulating exponentially since the introduction of digital radiology in the 1990s. For this reason, being already completely computerised, radiology is bound to be the first of all medical specialties embracing the use of machine-learning and artificial intelligence. By 2020, global medical data is expected to double every 73 days, and on average, each person generates one petabyte of health-related data across his or her lifetime [ 18 ]. Medical images are a substantial portion of all medical data, estimated to amount to 30% of all data storage [ 19 ]. The amount of data being collected exceeds our capacity to interpret them, especially when integrating multiple modalities from vastly different fields such as radiology, metabolomics and genetics.
Available medical imaging data can be a highly valuable resource for research on diagnostics, epidemiology and drug development. This has led to a higher interest in the development of data-driven models based on machine learning (ML) and artificial intelligence (AI) [ 18 ]. Deep learning, based on artificial neural networks, emerged in recent years as a powerful tool for ML and promises to reshape the future of AI. Increasing computational power allows ML, and AI in general, to more accurately identify and generate semantic interpretations from medical imaging data, supporting diagnoses.
Interpreting images can be highly subjective. ML and AI can be used to interpret images more consistently, as well as document metadata and perform data entry. ML techniques not only have significant potential to improve diagnoses from medical images, but may also improve disease prediction, decision-making, treatment planning and treatment response predictions [ 20 ].
Currently, ML and AI in medical imaging are not being used to their full extent. Beyond supporting diagnoses, ML and AI can reduce cost by cutting scan times, automating post-processing and reducing computation times for model-based image interpretation. Furthermore, ML and AI can accelerate drug discovery and leverage data from multiple sources (including sources other than medical images) for better care coordination.
Examples addressing Challenge 5
Example 9: identifying novel phenotypes in lung diseases.
A key ability of ML is the exploitation of complex features drawn from multiple modalities and across the entire patient. Current finding and diagnostic categories in lung diseases such as fibrosis are lacking in terms of repeatability and their ability to predict outcome and treatment response. Machine learning can contribute by identifying those patterns that can be extracted with high stability and reliability from clinical imaging data (Fig. 9 ). It can connect these measurements with patient information such as smoking history, or age. Finally, it can mine the resulting descriptions for reliable predictors linking current patient status with outcome and risk that is relevant for individual treatment decisions to identify predictive markers. Initial results point to the power of machine learning in this direction, and we expect dramatic changes in both the characteristics we use for prognosis as well as the way we use them to treat an individual through the emerging integration of machine learning in the development of diagnostic tools, and the day-to-day clinical decision support.
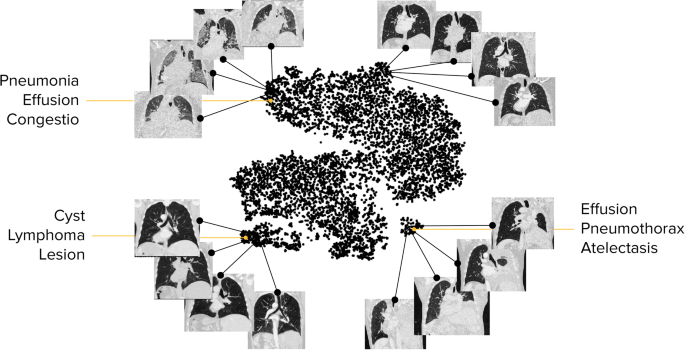
The landscape of lung disease patients based on their CT image data. The distribution illustrates clusters of patients with similar imaging characteristics confirmed in reported findings. It is a step towards the identification of phenotypes in large-scale medical imaging data. [ 24 ]
Example 10: Automation of detection and measurements
A wealth of known markers are tedious and time-consuming to extract from clinical imaging data. The widening gap between the rapidly increasing amounts of imaging data acquired during clinical routine, and the available number of radiologists who can read images, requires an increase of efficiency, and the delegation of tasks to machine learning-based algorithms. While the expert focuses on integrating individual measurements into a comprehensive assessment of the patient, the machine can reduce the time needed to screen hundreds of image slices. Examples comprising vast amounts of imaging data in need of analysis to improve disease diagnosis and prediction include the detection of nodules in lung imaging, polyps in CT-colonography, measurement of brain structures in neurological disease and evaluation of tumour characteristics for diagnosis and therapy outcome prediction (Fig. 10 ).
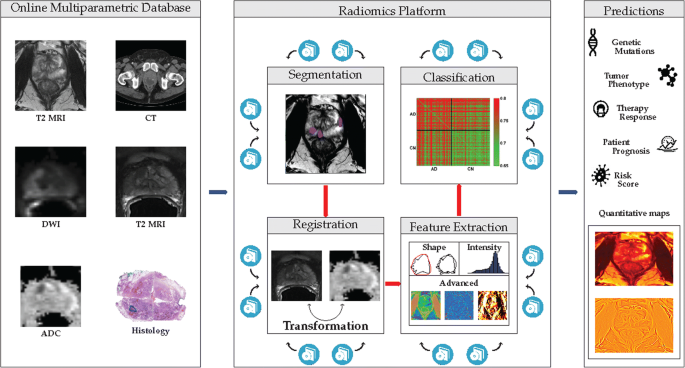
Radiomics pipeline, with the aim to link imaging features to clinically relevant parameters such as tumour subtype, patient prognosis and therapy outcome prediction (Courtesy Martijn Starmans and Stefan Klein, Rotterdam)
Overcoming challenges in healthcare through biomedical imaging research
The EIBIR Strategic Research Agenda for Biomedical Imaging identifies research areas critical to overcoming current challenges in healthcare:
Innovative biomedical imaging plays a key role in field of personalised medicine as it addresses the current needs for individualised prevention, treatment, therapy response monitoring and image-guided surgery. In the area of disease prevention and therapy monitoring, the use of non-invasive biomarkers will lead to improved patient outcome, therapy prediction and monitoring.
Innovative diagnostic imaging technologies and procedures provide information about disease characteristics and, coupled with biological, genetic and -omics data, will contribute to an individualised diagnosis and consecutive targeted therapy approach. In the emerging field of theranostics, imaging tools together with therapeutic agents allow for tailored therapeutic interventions and the selection of most effective treatments.
In the area of prenatal monitoring, the use of advanced imaging technologies with higher diagnostic accuracy will ensure the early detection of malfunctions or disease and benefit maternal and foetal health providing a healthy start in life.
The use of biomedical imaging for diagnosis and management of lifestyle-induced diseases followed by lifestyle changes will help to prevent disease development.
The advanced application of Artificial Intelligence and Machine Learning in imaging data will help to improve image interpretation and lead to better disease prediction, decision making and treatment planning.
In summary, biomedical imaging has the proven potential to address the major challenges in healthcare and to contribute to the wellbeing of European citizens and patients.
To realise this potential, investments in biomedical imaging research are needed to enable the translation of innovative solutions into clinical practice. Moreover, the appropriate funding will ensure that the European research community stays in the forefront of medical science.
Decision makers are invited to consider this document in the definition of research topics strategically recommendable for financing in the next decades.
Supporting societies
This Strategic Research Agenda for Biomedical Imaging was developed by EIBIR with the support and input of its shareholder societies:
European Society of Radiology (ESR)
The ESR is a non-profit organisation representing the general interests of radiology in Europe ( www.myesr.org ). The aims of ESR are to serve the healthcare needs of the general public through the support of science, teaching and research and the quality of service in the field of radiology as well as the promotion and coordination of the scientific, philanthropic, intellectual and professional activities of radiology in all European countries. The ESR has over 80,800 individual members as well as 109 member societies of which 47 are European national radiology societies, 15 are European radiological subspecialty societies and European allied sciences and 47 non-European national radiology societies.
Cardiovascular and Interventional Radiological Society of Europe (CIRSE)
CIRSE is a non-profit, educational and scientific association aiming to improve patient care through the support of teaching, science, research and clinical practice in the field of cardiovascular and interventional radiology (IR) ( www.cirse.org ). CIRSE aims to support education and further research in IR, as well as ensuring excellent patient safety and timely access to IR therapies. CIRSE also actively collaborates with and supports other scientific, educational, governmental, professional, national and international organisations considered useful to the aims of the Society.
European Coordination Committee of the Radiological, Electromedical and Healthcare IT Industry (COCIR)
COCIR is the European Trade Association representing the medical imaging, radiotherapy, health ICT and electromedical industries ( www.cocir.org ). COCIR is a non-profit association and provides a wide range of services on regulatory, technical, market intelligence, environmental, standardisation, international and legal affairs. COCIR promotes harmonisation of regulatory frameworks, supported by state-of-the-art international standards. Its industry provides safe and high-quality products and services, which contribute to reducing health inequalities and enhance cost efficiency in healthcare systems.
European Association of Nuclear Medicine (EANM)
EANM is the umbrella organisation representing nuclear medicine in Europe and represents 40 National Member Societies, approximately 3200 individual members and around 30,000 professionals working in nuclear medicine in Europe ( www.eanm.org ). EANM aims to advance science and education in nuclear medicine for the benefit of public health, relating to the diagnosis, treatment, research and prevention of diseases through the use of unsealed radioactive substances and the properties of stable nuclides in medicine, throughout Europe.
European Federation of Organisations for Medical Physics (EFOMP)
The EFOMP serves as an umbrella organisation representing 34 national member and affiliated organisations of more than 8100 physicists and engineers working in the field of medical physics in Europe ( www.efomp.org ). EFOMP aims to harmonise and advance medical physics in both its professional clinical and scientific expression throughout Europe by bringing about and maintaining systematic exchange of professional and scientific information, through the formulation of common policies, and by promoting education and training programmes.
European Federation of Radiographer Societies (EFRS)
The EFRS is a non-profit umbrella organisation representing 39 professional societies and 60 educational institutions representing over 100,000 radiographers across Europe ( www.efrs.eu ). The aims of the EFRS are to represent, promote and develop the profession of radiography in Europe, across medical imaging, nuclear medicine and radiotherapy areas of radiography practice.
European Organisation for Research and Treatment of Cancer (EORTC)
EORTC is an independent, non-profit cancer research organisation, with the mission to coordinate and conduct international translational and clinical research to improve the standard of cancer treatment for patients ( www.eortc.org ). EORTC aims ultimately to increase people’s survival and quality of life by testing new therapeutic strategies based on existing drugs, surgery and radiotherapy and also helps develop new drugs and approaches in partnership with the pharmaceutical industry and in patients’ best interests.
European Society for Magnetic Resonance in Medicine and Biology (ESMRMB)
ESMRMB is a non-profit society, which aims to support educational activities and research in the widest sense in the field defined by the society’s name ( www.esmrmb.org ). The ESMRMB is open to physicians, engineers, scientists and other individuals who are interested in the developments or the introduction of magnetic resonance techniques in the fields of medicine and biology.
European Society of Paediatric Radiology (ESPR)
ESPR aims to organise and bring together physicians involved in the field of paediatric imaging, to contribute to the progress of paediatric imaging particularly within but also outside Europe, to encourage training and education with other branches of medical imaging and paediatrics in clinical, scientific, education and research fields ( www.espr.org ).
European Society for Radiotherapy and Oncology (ESTRO)
ESTRO is a non-profit scientific organisation representing radiation oncologists, medical physicists, radiobiologists and radiation therapists with over 5000 members both within and outside Europe ( www.estro.org ). ESTRO aims to foster the role of radiation oncology in order to improve patient care in the multimodality treatment of cancer by promoting innovation, research and dissemination of science through its congresses, special meetings, educational courses and publications.
European Society of Medical Imaging Informatics (EuSOMII)
EuSoMII is a professional healthcare organisation that provides its members and the radiological community with up-to-date information on the latest innovations and achievements in medical imaging informatics by supporting education, research and events related to the top-tier software in radiology ( www.eusomii.pro ).
European Commission (2017) Shaping our Future. European Commission, Brussels Available via https://ec.europa.eu/info/events/shaping-our-future-2017-jul-03_en . Accessed 30 Aug 2018
European Commission (2017) 2018 Commission work programme – key documents. European Commission, Brussels Available via https://ec.europa.eu/info/publications/2018-commission-work-programme-key-documents_en . Accessed 30 Aug 2018
European Commission (2017) EU Council adopts conclusions on digital health care. European Commission, Brussels Available via https://ec.europa.eu/digital-single-market/en/news/eu-council-adopts-conclusions-digital-health-care
Google Scholar
Council of the European Union (2017) Employment, Social Policy, Health and Consumer Affairs Council Meeting on 8 December 2017. Available via http://data.consilium.europa.eu/doc/document/ST-14078-2017-INIT/en/pdf
European Society of Radiology (2011) Medical imaging in personalised medicine: a white paper of the research committee of the European Society of Radiology (ESR). Insights Imaging. https://doi.org/10.1007/s13244-011-0125-0
Alseidi A, Doniselli F, Jaeger HR, Golay X, Bisdas S (2018) The value of arterial spin labelling in adults glioma grading: systematic review and meta-analysis. Submitted manuscript
European Association of Nuclear Medicine (EANM); European Federation of Organizations for Medical Physics (EFOMP); European Federation of Radiographer Societies (EFRS); European Society of Radiology (ESR); European Society for Radiotherapy and Oncology (ESTRO) (2017) Common strategic research agenda for radiation protection in medicine. Insights Imaging. 8:183–i197. https://doi.org/10.1007/s13244-016-0538-x
Aamdal S, Dittrich C, Schellens J, et al. (2012) A paradigm shift in early drug development: individualizing to more patient benefit. Ann Oncol 23:ix32–ix33. https://doi.org/10.1093/annonc/mds374
Article Google Scholar
Calmon R, Puget S, Varlet P, et al. (2018) Cerebral blood flow changes after radiation therapy identifies pseudoprogression in diffuse intrinsic pontine gliomas. Neuro Oncol. https://doi.org/10.1093/neuonc/nox227
Geninatti Crich S, Terreno E, Aime S (2017) Nano-sized and other improved reporters for magnetic resonance imaging of angiogenesis. Adv Drug Deliv Rev . https://doi.org/10.1016/j.addr.2017.08.004
Ozdemir NY, Arslan SA, Sendur MA (2017) 177Lu-Dotatate for midgut neuroendocrine tumors. N Engl J Med. https://doi.org/10.1056/NEJMc1701616
Oren R, Addadi Y, Narunsky H et al. (2016) Fibroblast recruitment as a tool for ovarian cancer detection and targeted therapy. Int J Cancer. https://doi.org/10.1002/ijc.30209
(2009) The WHO cross-national study of health behavior in school aged children from 35 countries: findings from 2001–2002. J Sch Health. https://doi.org/10.1111/j.1746-1561.2004.tb07933.x
Prüss-Üstün A, Corvalán CF, World Health Organization (2006) Preventing disease through healthy environments: towards an estimate of the environmental burden of disease. World Health Organization Available online: http://www.who.int/iris/handle/10665/43457
Douglas PS, Cerqueira MD,Berman DS et al. (2016) The future of cardiac imaging: report of a think tank convened by the American College of Cardiology. JACC Cardiovasc Imaging. https://doi.org/10.1016/j.jcmg.2016.02.027
Segula D (2014) Complications of obesity in adults: a short review of the literature. Malawi Med J 26(1):20–24
CAS PubMed PubMed Central Google Scholar
Bamberg F, Hetterich H, Rospleszcz S et al. (2017) Subclinical disease burden as assessed by whole-body MRI in subjects with prediabetes, subjects with diabetes, and normal control subjects from the general population: the KORA-MRI study. Diabetes. https://doi.org/10.2337/db16-0630
IBM Watson Health, University of Iowa, Carver College of Medicine (2015) IBM and partners to transform personal health with Watson and Open cloud [press release]. Available online: https://www-03.ibm.com/press/us/en/pressrelease/46580.wss
European Union High level Expert Group on Scientific Data (2010) Riding the wave: how Europe can gain from the rising tide of scientific data. Available online: http://ec.europa.eu/information_society/newsroom/cf/document.cfm?action=display&doc_id=707
Ravi D, Wong C, Deligianni F et al. (2017) Deep learning for health informatics. IEEE J Biomed Health Inform. https://doi.org/10.1109/JBHI.2016.2636665
Verbruggen SW, Kainz B, Shelmerdine SC et al. (2018) Stresses and strains on the human fetal skeleton during development. J R Soc Interface. https://doi.org/10.1098/rsif.2017.0593
Avni R, Golani O, Akselrod-Ballin A et al. (2016) MR imaging–derived oxygen-hemoglobin dissociation curves and fetal-placental oxygen-hemoglobin affinities. Radiology. https://doi.org/10.1148/radiol.2015150721
Storz C, Rospleszcz S, Lorbeer R et al. (2018) Phenotypic multiorgan involvement of subclinical disease as quantified by magnetic resonance imaging in subjects with prediabetes, diabetes, and normal glucose tolerance. Invest Radiol. https://doi.org/10.1097/RLI.0000000000000451
Hofmanninger J, Krenn M, Holzer M, Schlegl T, Prosch H, Langs G (2016) Unsupervised identification of clinically relevant clusters in routine imaging data. In: Ourselin S, Joskowicz L, Sabuncu M, Unal G, Wells W (eds) Medical image computing and computer-assisted intervention – MICCAI 2016. MICCAI 2016. Lecture notes in computer science. https://doi.org/10.1007/978-3-319-46720-7_23
Download references
Acknowledgements
The European Institute for Biomedical Imaging Research would like to thank the following people for their in-depth review of the SRA and suggestions: Silvio Aime, Angel Alberich, Anja Almen, Owen J Arthurs, Henryk Barthel, Olivier Clément, Michael Crean, Nandita de Souza, Florian Demuth, Marc Dewey, Vincent Dousset, Alejandro Frangi, Casper Garos, Xavier Golay, Peter Gordebeke, Matthias Günther, Horst Hahn, Monika Hierath, Christoph Hoeschen, Myriam Hunink, Hans-Ulrich Kauczor, Gabriel Krestin, Katharina Krischak, Georg Langs, Yan Liu, Luis Marti-Bonmati, Celso Matos, Ulrike Mayerhofer-Sebera, Jonathan McNulty, Kristoff Muylle, Michal Neeman, Wiro Niessen, Konstantin Nikolaou, Philippe Pereira, Anders Persson, Antonio Pifferi, Katrine Riklund, Andrea Rockall, Karen Rosendahl, Francesco Sardanelli, Steven Sourbron, Oliver Speck, Vincenzo Valentini and Pamela Zolda.
Author information
Authors and affiliations.
- Silvio Aime
- , Angel Alberich
- , Anja Almen
- , Owen J Arthurs
- , Henryk Barthel
- , Olivier Clément
- , Michael Crean
- , Nandita de Souza
- , Florian Demuth
- , Marc Dewey
- , Vincent Dousset
- , Alejandro Frangi
- , Casper Garos
- , Xavier Golay
- , Peter Gordebeke
- , Matthias Günther
- , Horst Hahn
- , Monika Hierath
- , Christoph Hoeschen
- , Myriam Hunink
- , Hans-Ulrich Kauczor
- , Gabriel Krestin
- , Katharina Krischak
- , Georg Langs
- , Luis Marti-Bonmati
- , Celso Matos
- , Ulrike Mayerhofer-Sebera
- , Jonathan McNulty
- , Kristoff Muylle
- , Michal Neeman
- , Wiro Niessen
- , Konstantin Nikolaou
- , Philippe Pereira
- , Anders Persson
- , Antonio Pifferi
- , Katrine Riklund
- , Andrea Rockall
- , Karen Rosendahl
- , Francesco Sardanelli
- , Steven Sourbron
- , Oliver Speck
- , Vincenzo Valentini
- & Pamela Zolda
Contributions
The author read and approved the final manuscript.
Ethics declarations
Competing interests.
The author declares no competing interests.
Publisher’s Note
Springer Nature remains neutral with regard to jurisdictional claims in published maps and institutional affiliations.
Rights and permissions
Open Access This article is distributed under the terms of the Creative Commons Attribution 4.0 International License ( http://creativecommons.org/licenses/by/4.0/ ), which permits unrestricted use, distribution, and reproduction in any medium, provided you give appropriate credit to the original author(s) and the source, provide a link to the Creative Commons license, and indicate if changes were made.
Reprints and permissions
About this article
Cite this article.
The European Institute for Biomedical Imaging Research (EIBIR). Strategic research agenda for biomedical imaging. Insights Imaging 10 , 7 (2019). https://doi.org/10.1186/s13244-019-0684-z
Download citation
Received : 09 October 2018
Accepted : 03 January 2019
Published : 28 January 2019
DOI : https://doi.org/10.1186/s13244-019-0684-z
Share this article
Anyone you share the following link with will be able to read this content:
Sorry, a shareable link is not currently available for this article.
Provided by the Springer Nature SharedIt content-sharing initiative
- Precision medicine
- Preventive medicine
- Diagnostic imaging
- Artificial intelligence
The relationship between academics’ strategic research agendas and their preferences for basic research, applied research, or experimental development
- Published: 15 July 2022
- Volume 127 , pages 4191–4225, ( 2022 )
Cite this article
- J. M. Santos 1 ,
- H. Horta ORCID: orcid.org/0000-0001-6814-1393 2 &
- H. Luna 2
3785 Accesses
1 Altmetric
Explore all metrics
In this study, we assess the association between academics’ research agendas and their preferences for basic research, applied research, or experimental development. Using a sample of Mexican academics working in some of the country’s most research-oriented universities, we identify three clusters. The largest is composed of applied research-oriented academics, the second largest is composed of basic research-oriented academics, and the smallest is composed of academics who engage in both basic and applied research, and experimental development. The strategic research agendas of the three clusters are distinguished from each other along four main dimensions: Divergence, Discovery, Mentor Influence, and Social Orientation. These findings show that strategic research agendas are associated with preferences for basic research, applied research, or experimental development, but only to some extent. We also extend the Multi-Dimensional Research Agendas Inventory – Revised, a widely used instrument for measuring strategic research agendas, by adding a new dimension, “Government,” and validating the instrument in a new context. We also make the scale available in Spanish for use by academics, practitioners, managers, and administrators in Spanish-speaking countries.
Similar content being viewed by others
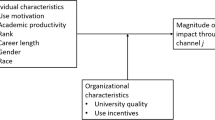
How do academic public administration and public policy researchers affect policymaking? Functional groupings from survey data
John P. Nelson, Barry Bozeman, … Spencer L. Lindsay
Will the Center Hold? What Research Centers Do to Universities and to Societal Challenges
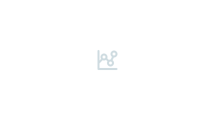
Are the strategic research agendas of researchers in the social sciences determinants of research productivity?
João M. Santos, Hugo Horta & Huan Li
Avoid common mistakes on your manuscript.
Introduction
The categorization of research activities into basic research, applied research, and experimental development was formalized by the influential Frascati Manual , published by the Organization for Economic Cooperation and Development (OECD) in 1963 (Godin, 2006 ). The Frascati Manual was a response to the need to differentiate types of research, given the changes in the development and funding of research in the second half of the twentieth century (Schauz, 2014 ). According to the Frascati Manual , the main purpose of basic research is the advancement of knowledge regardless of any particular use or application. Applied research has a problem-solving rationale and is oriented towards practical objectives, whereas experimental development involves the use of research knowledge to generate new products and services or improve current ones (OECD, 2015 ).
Studies on the evolution and purposes of basic and applied research, and experimental development, have been carried out. These often examined sectors and organizations that privilege one research focus over the other, public policies that promote research specialization and/or broadness, and how changes in science and technology influence these research categories (e.g., Fan et al., 2021 ; Larivière et al., 2018 ; Salter & Martin, 2001 ). Some studies are intellectual discussions of the benefits, challenges, and meaning of categorizing research into basic, applied, and experimental development, and of the feasibility of developing other categorization systems (e.g., Godin, 2002 ). However, despite critiques, the original OECD categorization is still generally accepted and frequently used in policymaking, managerial, and academic circles (Roll-Hansen, 2017 ; Schauz, 2014 ). Some studies have linked this research categorization to career-related incentives and the evaluation of academic research. The orientation towards applicability, problem solving, and impact has become explicit in national research projects funded by governments and in the evaluation criteria used by universities (Marques et al., 2017 ). This has led to concern that the focus on applicability in funding and evaluation has created extrinsic incentives and constraints on academic research and has encouraged a shift from basic to applied research in many North American and European countries (e.g., Zapp & Powell, 2017 ). Footnote 1 However, studies have shown that instead of shifting from basic to applied research, academics have adapted by carrying out both types (Bentley et al., 2015 ), using a complex mix of the two (Gulbrandsen & Kyvik, 2010 ).
Despite all the studies mentioned above, the preference of individual academics for basic research, applied research, or experimental development is still understudied in the literature. It is known that academics with work experience outside academia tend to prefer applied research and experimental development; it is unsurprising that they bring problems and ideas from their employment experiences to their academic work practices (Gulbrandsen & Thune, 2017 ). Full professors, although continuing to engage in basic research, also tend to be more engaged in knowledge exchange and commercialization activities, and therefore conduct more applied research and experimental development than assistant and associate professors (Gulbrandsen & Smeby, 2005 ). Recent research shows that choosing to focus on either basic research or applied research requires academics to make a trade-off between publications and innovations; only a few, known as ambidextrous scholars, manage to balance basic and applied research by relying on network dynamics and collaboration (Werker & Hopp, 2020 ). Academic women tend to be overrepresented in applied research fields and underrepresented in basic research (Abreu & Grinevich, 2016 ). In other words, male academics are more engaged in basic research that contributes to scientific progress and female academics are more engaged in applied research that addresses social issues and development (Zhang et al., 2021 ). Academics in the humanities and natural sciences are more likely to engage in basic research, those in the social and health sciences are more likely to have mixed research focuses, and those in the fields of engineering tend to engage in applied research and experimental development (Gulbrandsen & Kyvik, 2010 ). Similarly, the analysis of research orientation in 15 countries by Bentley et al. ( 2015 ) notes that although there are inter-country differences, academics who specialize in basic research consistently work in settings where applied research is not emphasized, obtain less funding, and are less engaged with social problems. These findings highlight the role of national and institutional policies in shaping academics’ research. It also highlights the role of academics as agents; they use the interactions between their educational and professional backgrounds and structural factors to shape their research strategies and define their identities as researchers (see Sapir, 2017 ).
The studies discussed above provide valuable insights into the question of who adopts specific research focuses in what settings, but an important gap can be identified: the extent to which academics’ strategic research agendas (SRAs) Footnote 2 relate to individual preferences for basic research, applied research, and experimental development. Understanding the relationship between SRAs and research preferences is important because studies have shown that SRAs are associated with personal attributes, such as gender (Santos et al., 2021 ), concepts of research (Santos & Horta, 2020 ), thinking styles (Santos et al., 2020 ), and choice of collaborators (Horta et al., 2021 ). This means that an academic’s SRA is related to research processes, but it is also imbued with the cognitive, judgmental, and decision-making traits of the researcher. The latter have been understudied in relation to research focuses, and therefore, in this paper, we assess the association between academics’ research agendas and their preferences for basic research, applied research, or experimental development. This leads to this study’s research questions:
Are there archetypes in terms of academics’ preference for basic research, applied research, and experimental development?
Are academics’ SRAs associated with their preference for basic research, applied research, and experimental development?
This second question in particular allows us to examine how individual academics’ beliefs, wants, and planning with regards to research are shaped by their predispositions and values (see Mallon et al., 2005 ) and how these are related to the pursuit of research activities in a spectrum that has basic research on one end and applied research/experimental development on the other (Bentley et al., 2015 ). The analysis uses a dataset of Mexican academics working in some of the most research-oriented universities in the country. This sample is relevant because most research on academics engaging in basic research, applied research, and experimental development has been conducted using samples from countries with developed scientific systems. One exception is the country comparison of Bentley et al. ( 2015 ), but they conduct a broad inter-country comparison and do not focus on developing scientific systems. Moreover, our dataset of academic researchers in Mexican universities allows us to achieve a second aim: validating the Multi-Dimensional Research Agendas Inventory – Revised (MDRAI-R) in a new context (see the following section and Horta & Santos, 2020 ). The replicability of the MDRAI-R, its translation into Spanish, and the addition of a new dimension, “Government,” represent further developments of this instrument, which can inform future research and managerial practices.
Data and method
Sras and the mdrai-r.
We use the MDRAI-R (Horta & Santos, 2020 ), which is an instrument designed to characterize SRAs. It has been widely used in conceptual and empirical studies. It measures eight main dimensions, some of which have sub-dimensions, of the strategic choices and preferences in researchers’ SRAs.
The first dimension, Scientific Ambition, is related to striving for prestige and peer recognition, and the related drive to publish scientific articles (i.e., the need to produce and disseminate knowledge), which are pivotal criteria in contemporary academic careers. The second dimension, Divergence, is a preference for expansion into multiple fields of knowledge, and engagement in multidisciplinary research, a key feature of current science, especially in pioneering topics where single-disciplinary perspectives are insufficient. The third dimension, Discovery, is a preference for topics that have the potential to lead to new scientific discoveries and breakthroughs, a type of research that is typically high risk, high reward. The fourth dimension, Tolerance to Low Funding (TTLF), is the willingness of an academic to engage in research projects with little to no sources of funding. The fifth dimension, Collaboration, represents both the opportunity and the willingness to participate in collaborative ventures. The sixth dimension, Mentor Influence, represents the degree to which an academic’s research is influenced by his or her mentor, typically the Ph.D. supervisor. The Academia Driven dimension measures the extent to which a person’s research agenda is influenced by institutional missions and goals, which may be either scientific communities that the academic identifies with or the university where he or she works. The eighth dimension, Society Driven, represents the degree to which the academic’s research agenda is oriented toward tackling societal problems, and the degree to which consultation with non-academics shapes the research agenda.
In this study, we add a new dimension to the MDRAI-R: the Government dimension, which measures the degree of perceived support (by academics and researchers) that the government provides to different knowledge activities. The introduction of this dimension is relevant because of the increasing influence of public policies and the associated research funding on the academics’ research orientation (Gläser & Laudel, 2016 ). Government policies that favor the development of higher education and science and technology tend to foster greater levels of research productivity, but also a greater intensity of knowledge and technology transfer behaviors by academics (e.g., Kowalczewska & Behagel, 2019 ). The introduction of the new Government dimension allows the removal of several redundant items to accommodate the new questions without increasing the survey’s length. Specifically, one item is removed from each of the Divergence, Collaboration, Discovery, and Society Driven scales. Table 1 summarizes the dimensions of the MDRAI-R/ MDRAI-R-S.
SRAs and expected preferences for basic and applied research and experimental development
The relationships between some SRA dimensions and preferences for basic research, applied research, or experimental development are unclear in the literature. For example, Ranga et al. ( 2003 ) show that academics’ publication profiles are often a mix of basic and applied research, which suggests that the influences of Scientific Ambition, Discovery, and Collaboration on research preferences are difficult to assess. These dimensions are highlighted in relation to academics’ publication profiles, as a recent study showed that they are associated with academics’ research productivity throughout their careers (Santos et al., 2022 ). Academics’ willingness to do research even with low or no funding (i.e., TTLF) is also difficult to assess when related to preferences for basic and applied research because funding may be a consideration or may be allocated by funders for some basic and applied research fields or contexts but not for others (e.g., Overland & Sovacool, 2020 ). However, a negative association between experimental development and a high TTLF score in that dimension may be expected because of the high costs that experimental development projects usually entail (see Hirzel et al., 2018 ).
The evidence of the role of Mentor Influence in an academic’s specific research focus is also expectedly mixed. Full professors, who are the most common mentors for Ph.D. students and the most influential, tend to be more engaged in applied research and experimental development than associate and assistant professors (e.g., Gulbrandsen & Smeby, 2005 ). However, a mentor’s influence is strongest in the early stages of an academic’s career. As academics need to publish, at the beginning of their career, they may be required to focus on basic research with some applied research, rather than full-on applied research and experimental development (Santos & Horta, 2018 ). For the divergence dimension, there is an expectation that academics focused on interdisciplinary and translational research may prefer applied research (Valentin et al., 2016 ). Considering the emphasis that many scientific communities, governments, and universities are placing on the production of knowledge that can be used by non-academic stakeholders, academics scoring high on the Academia Driven and Government dimensions (Jongbloed et al., 2008 ) are expected to favor applied research. Similarly, academics who are more socially oriented are likely to engage in applied research and experimental development, as their research will focus on problem-solving, targeted research, and development of products, services, or solutions to a problem (Raynor, 2019 ).
Data collection
The first step in data collection was identifying all of the academics working in some of the most research-oriented universities in Mexico (UNAM, ITESM, UAM, UANL, and BUAP). A total of 15,093 individuals were identified on university websites. They were contacted via e-mail in three waves between April and July 2021 with an invitation to complete a survey. A total of 1160 valid responses were collected, representing a response rate of 7.68%. The survey began with an informed consent form that the participants were required to sign before proceeding to the translated and updated version of the MDRAI-R (henceforth, MDRAI-R-S) and other questions relevant to the analysis. Table 2 contains details on the sampling, notably the population size per institution, the sample size per institution, and the relative difference in percentage. Overall, across the nine institutions, there is an average distribution difference of 2.98%. A paired samples t-test used to compare the population percentage with the sample percentage for each institution showed no significant differences ( t ( 8 ) = 1.413, p = 0.195), confirming the similarity of the population’s and the sample’s distribution in terms of institutions.
We conducted several analyses. The first was the validation of the MDRAI-R-S, which used structural equation modeling, specifically confirmatory factor analysis (CFA; Kline, 2016 ; Marôco, 2010 ). As this is the most technical section of the paper, the implementation is described in some depth. In the second analysis, we conducted a cluster analysis with three input variables: share of time dedicated to basic research; share of time dedicated to applied research; and share of time dedicated to experimental development. The cluster analysis was an exploratory procedure used to identify patterns in the sample (Hair et al., 2014 ; Marôco, 2003 ) and has been used in other studies to categorize individuals based on science indicators (Almeida et al., 2009 ; Santos & Horta, 2015 ). The goal of this analysis was to identify research agenda profiles based on the allocation of time to different types of research. For this purpose, a two-step clustering algorithm in SPSS 26 was used, which is generally considered a superior alternative to classical hierarchical clustering (Norusis, 2012 ; Zhang et al., 1996 ). Following this clustering procedure, a multinominal regression analysis was performed with the clustering variables as dependent variables, and the MDRAI-R-S dimensions—as well as controls—as predictors. The aim was to identify whether there were differences across research profiles.
This section defines the variables used in the multinominal regression analysis. The primary independent variables were the SRA dimensions, described above. These were complemented with control variables drawn from previous studies characterizing academics’ preference for basic research, applied research, or experimental development (i.e., Bentley et al., 2015 ; Gulbrandsen & Kyvik, 2010 ; Gulbrandsen & Smeby, 2005 ; Ranga et al., 2003 ; Werker & Hopp, 2020 ): gender (reference category: female); field of science Footnote 3 ( FOS ; reference category: agricultural sciences); non-academic experience , which indicates whether the academic has work experience outside academia; full professor , which is a dummy variable indicating whether the participant is a full professor; and external funding , which is a dummy variable indicating whether the participant has received external funding in the past 3 years. These control variables allowed us to assess whether our findings matched those of other studies of research preferences, which have generally been undertaken in advanced scientific systems, whereas ours focused on a developing scientific system.
We also used a number of control variables not included in previous empirical research on this topic. For example, academic career duration is a self-explanatory variable that assesses the possibility of academics shifting their focus from research and publications to administration, knowledge exchange, and other activities more related to financial rewards as their careers progress, leading them to focus more on experimental development over time (Mittermeir & Knorr, 1979 ). Academic mobility may also have a role. In a study of academic inbreeding in Mexico, Horta et al. ( 2010 ) find that non-mobile academics are more likely to be engaged in knowledge transfer activities than their peers, suggesting that they are more oriented toward applied research and experimental development than their more mobile peers. Accordingly, we used the non-mobile academics category (i.e., academics hired by the university where they obtained their Ph.D. who remain there for their professional career) as the baseline for our measure of academic mobility. The other categories of mobility were as follows: silver-corded (those currently working in the university where they earned a Ph.D., but who have worked in other universities), adherents (those who were hired by a different university than the one where they completed their Ph.D. and stayed at that university), mobile national (those who have held academic jobs at several Mexican universities), and mobile international (those who have held several academic jobs including some at non-Mexican universities). The final control variables categorized academics by the percentage of time they dedicate to each of the following activities: teaching, research, knowledge exchange, administrative tasks, and supervision of students. It is known that these activities sometimes complement each other, and at other times constrain each other, but how they relate to academics’ focus on basic research, applied research, and experimental development is unknown. Table 3 summarizes the descriptive statistics for the variables employed in this study.
Analysis 1—MDRAI-R-S validation
To specify the model, the missing data were imputed using a linear regression method (Zhang, 2016 ). This was required, as the built-in function in AMOS for handling missing data does not permit the computation of modification indices (MI). Following the model specification stage, and once MI estimation was complete, a full-information maximum likelihood (FIML) estimation was applied, as this is considered a superior method for managing missing data (Enders & Bandalos, 2001 ). This analysis therefore incorporates data for the full working sample ( N = 1160).
Model specification
As this instrument has been validated using a global sample, and the factorial structure of the items—with the exception of the new scale—is well documented (Horta & Santos, 2020 ), the specification strategy was merely to replicate the structure identified in previous studies. The new items were specified as new, independent factors. As expected, since the previous validation exercise already solved all detected issues with the scale, the initial solution was immediately admissible with no required re-specification steps.
The second step in the model specification was locating items with poor loadings, as these are a threat to factorial validity. As expected, no items exhibited factorial loadings under the 0.50 threshold (Kline, 2016 ; Marôco, 2010 ), so there were no candidates for removal.
The third step in the model specification was evaluating the MIs. Although the initial model already exhibited good fit (as described below), it was decided that MI evaluation should still be done for the sake of completeness. The MIs were scanned at the 11 threshold, which corresponds to a Type I error probability of 0.001 (Marôco, 2010 ). Although some proposed covariances met the required threshold, none of them were eligible, as they represented inter-factor covariances or non-valid latent factor covariances (Hair et al., 2014 ; Kline, 2016 ). Accordingly, the initial model was also the final one.
Fit evaluation
Following best practices, a range of fit indices were used to assess model fit (Barrett, 2007 ; Kline, 2016 ): the X 2 /df index (Arbuckle, 2007 ), the comparative-fit index (CFI; Bentler, 1990 ), its parsimony-adjusted variant, the PCFI (Marôco, 2010 ), and the root-mean-square error of approximation (RMSEA; Steiger et al., 1985 ).
After model specification, the model was estimated and the fit was qualitatively assessed as good ( X 2 / df = 2.512; CFI = 0.956; PCFI = 0.799; RMSEA = 0.036). Table 4 compares the fit of the MDRIA-R-S with that of the original instrument; they were very similar, confirming the robustness of the instrument even when applied to a completely independent sample.
The next step was a CFA of the specified model. Figure 1 illustrates the model, and Table 5 presents the factorial loadings of the various items in our analysis and in the original scale. The loadings were very similar, another indication of the scale’s robustness.
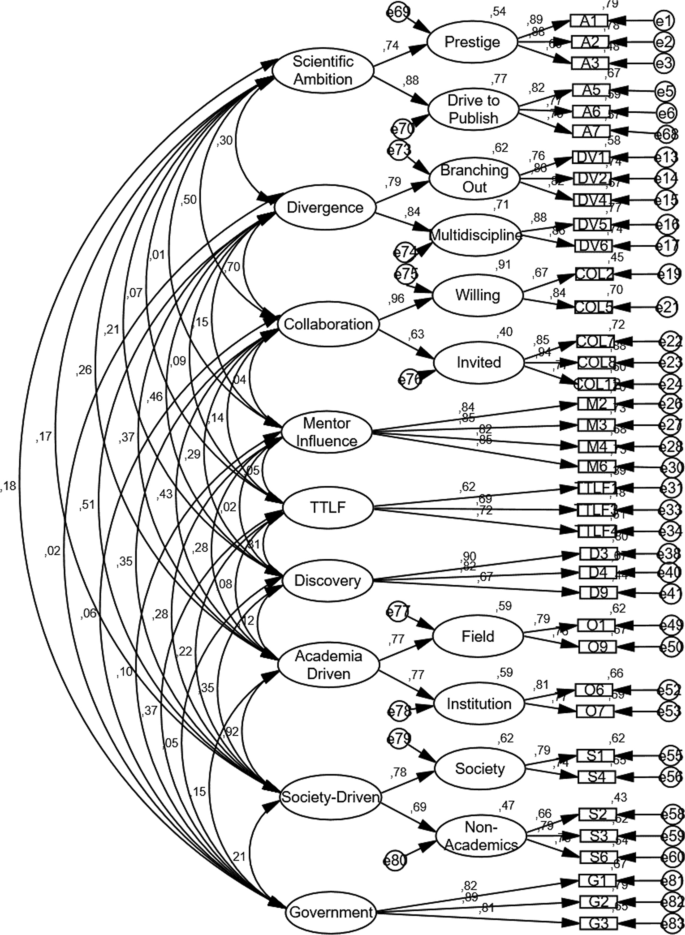
Measurement model for the MDRAI-R-S, with standardized regression weights (loadings). Note Ellipses indicate latent variables, and squares indicate manifest variables. Disturbance terms are indicated by the latent variables labeled “e.”
Validity, reliability, and sensitivity
We evaluated MDRAI-R-S’ psychometric properties. All of the calculations were conducted using the Validity Master macro in James Gaskin’s Stats Tool Package ( 2016 ). The calculations, referred to throughout this discussion, are shown in Table 6 .
We first evaluated the factorial, convergent, and discriminant validity of the dimensions (Anastasi & Urbina, 1997 ). Factorial validity requires all of the items to have loadings of at least 0.50 (Marôco, 2010 ). This was verified by the CFA, discussed above, which confirmed the factorial validity.
Second, we evaluated the convergent validity , which occurs when the manifest variables exhibit very high loadings into the respective latent variables. A strict measure of this can be attained through the average variance extracted indicator (AVE; Fornell & Larcker, 1981 ), which is given by
where j indicates a given factor, i a given item, λ a factorial loading, and ε an error term. As per the Fornell–Larcker criterion, an AVE of more than 0.50 indicates convergent validity. This threshold was fully met for all of the sub-scales, with the exception of TTLF, which had an AVE slightly below the cutoff point (0.461). This may have been caused by the exclusion of one of the items belonging to this sub-scale. This suggests that future revisions should reintroduce the item. Nevertheless, as the AVE for TTLF was only a few decimal points under the threshold, it is likely that this will have little practical impact. Interestingly, there was a similar result for the Discovery sub-scale in the original instrument (Horta & Santos, 2020 ), which seems to have been resolved in this version. Again, this might be related to the removal of one of the items in the Discovery scale. Accordingly, the permanent removal of that item might be warranted.
The third aspect of validity is discriminant validity , which requires that the various sub-scales do not conceptually overlap—in other words, constructs should have a low degree of inter-factor correlations or cross-loadings. We tested this using the maximum shared variance (MSV), which is the square of the highest of the inter-factorial correlations, and the average shared variance (ASV), which is the average of the sum of squared inter-factorial correlations. To demonstrate discriminant validity, the square root of the AVE must exceed the value of all of the inter-factorial correlations; cumulatively, the AVE for a factor must be greater than that factor’s MSV and ASV. These criteria were met for all factors, with the exception of Academia Driven and Society Driven; these two factors exhibited a correlation of 0.915, substantially higher than that observed in the original scale (0.760). There are two possible explanations for this. First, Academia Driven and Society Driven goals might be strongly aligned in Mexico, causing the scores of these sub-scales to naturally converge. Alternately, this alignment of academic and social goals might not be specific to Mexico, but part of a worldwide trend that has developed since the scale was first validated. Although this is speculative—and we currently have no data to test this—the COVID-19 pandemic, which began after the original validation exercise, might have pushed academic and societal goals closer together, with the result that these two sub-scales are no longer fully differentiated. If such a global trend is confirmed, then these two sub-scales might merge at some point in the future. For this study, the implication was that the scores across these two sub-scales were expected to be very highly correlated.
The next psychometric property to be evaluated was reliability . For this purpose, we computed the composite reliability (CR; Fornell & Larcker, 1981 ). CR is given by the following formula:
with the same notations as the calculation for AVE. The generally accepted threshold for CR is 0.70 (Hair et al., 2014 ). All of the dimensions exceeded this threshold, demonstrating the reliability of the MDRAI-R-S.
Finally, we calculated the scale’s sensitivity , which is its ability to differentiate between individuals. This property is demonstrated when each item is sufficiently close to a normal distribution (Marôco, 2010 ), which is commonly achieved when an item’s skewness and kurtosis are under the absolute value of 3 (Kline, 2016 ). As can be seen in Table 7 , this was the case for all of the items.
Analysis 2—Cluster analysis
Three variables were used as predictors for clustering—the share of time dedicated to basic research, to applied research, and to experimental development. One hundred and twenty seven participants skipped this section of the survey and as such were not eligible for data imputation. The working sample for this analysis was therefore lower ( N = 1033). This analysis yielded a three− cluster solution with a good fit: 0.5 on the silhouette measure of cohesion and separation (Kaufman & Rousseeuw, 2009 ; Rousseeuw, 1987 ). Table 8 describes the characteristics of these clusters based on the predictor variables.
The first cluster, “Applied Researchers,” consisted of academics who allocated most of their time to applied research. They also allocated a reasonable amount of time to basic research, but very little time to experimental development. The second cluster, “Basic Researchers,” showed the opposite pattern, with a large share of time dedicated to basic research, a fraction dedicated to applied research, and a very small amount to experimental development. It is noteworthy that the proportion of basic research in the Basic Researchers’ cluster was substantially higher than the proportion of time allocated to applied research in the Applied Researchers’ cluster, suggesting that the applied researchers were more open to research focus complementarity and less specialized than the basic researchers. Finally, the last cluster, “Balanced Researchers,” distributed their time somewhat equitably across all three research focuses; these academics match the definition of ambidextrous scholars in Werker and Hopp’s ( 2020 ) paper. Having classified the academics into these three clusters, the second step of the cluster analysis was to determine whether the SRAs varied between clusters. For this purpose, we computed the average scores of the items for each dimension in each cluster (DiStefano et al., 2009 ). Additionally, an analysis of variance (ANOVA) was conducted to identify which SRA dimensions differed significantly across clusters (Table 9 ), with the goal of understanding how SRA are associated with their preferences for the different types of research. Tukey’s HSD post− hoc tests (Tukey, 1953 ) were used to triangulate specific pairs with differences.
Significant differences were shown across clusters for Divergence ( F (2, 1,030) = 12.920, p < 0.001), with the Basic cluster having the lowest scores, followed by Applied and Balanced. Collaboration exhibited significant differences ( F (2, 1,030) = 3.053, p < 0.05) in the omnibus ANOVA test, but the post− hoc test failed to identify specific pairs with significantly different scores. As such, the evidence for differences in Collaboration was inconclusive. Mentor Influence exhibited significant differences across clusters ( F (2, 1,030) = 6.569, p < 0.01), with the Basic cluster having lower scores than the Applied and Balanced clusters, which did not differ from each other. Discovery also exhibited significant differences across clusters ( F (2, 1,030) = 7.740, p < 0.001). Again, the Basic cluster had the lowest scores for Discovery, followed by Applied and then Balanced. Academia Driven ( F (2, 1,030) = 15.960, p < 0.001) and Society Driven ( F (2, 1,030) = 55.030, p < 0.001) also differed significantly across clusters, following the same pattern: Basic had the lowest scores, Applied had mid− level scores, and Balanced had the highest scores. Figure 2 shows the significant differences for specific pairs.
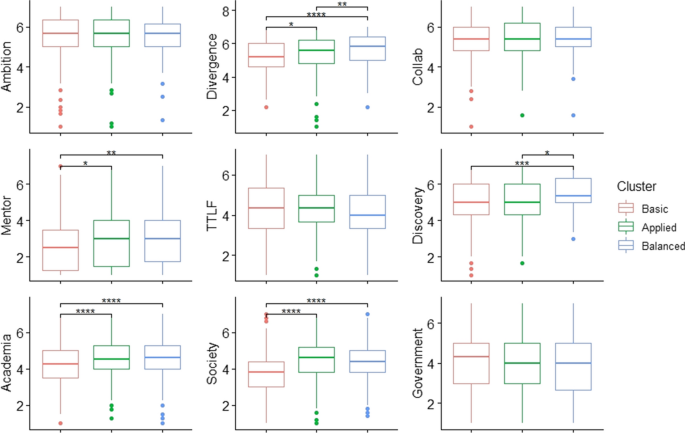
Cluster comparison, with Tukey’s HSD post− hoc comparisons (Tukey, 1953 ). **** p < 0.0001; *** p < 0.001; ** p < 0.01; * p < 0.05
Analysis 3—Multinominal regression
In our final analysis, we conducted a multinominal regression on the clustering membership variable, using the full suite of SRA dimension scores and several control variables. Three hundred and sixty one of the participants skipped the survey questions on career data, which were required to produce the control variables; they were therefore excluded, which reduced the working sample for this analysis to 799 participants. In this regression, the “Balanced” cluster was used as the baseline. The results are shown in Table 10 .
Among the SRA variables, a high Discovery score reduced the odds of placement Footnote 4 in both the Basic (B = − 0.273, p < 0.05, OR 0.761) and Applied (B = − 395, p < 0.01, OR 0.673) clusters. Divergence only reduced the odds of placement in the Applied cluster ( B = − 0.318, p < 0.05, OR 0.728), whereas higher Mentor Influence scores reduced the odds of being in the Basic cluster ( B = − 0.131, p < 0.1, OR 0.877). Finally, high Society Driven scores led to a reduced propensity for Basic research ( B = − 0.307, p < 0.01, OR 0.735) and a greater likelihood of belonging to the Applied cluster ( B = 0.404, p < 0.01, OR 1.497). The main findings regarding SRA can be better visualized through a forest plot, shown as Fig. 3 .
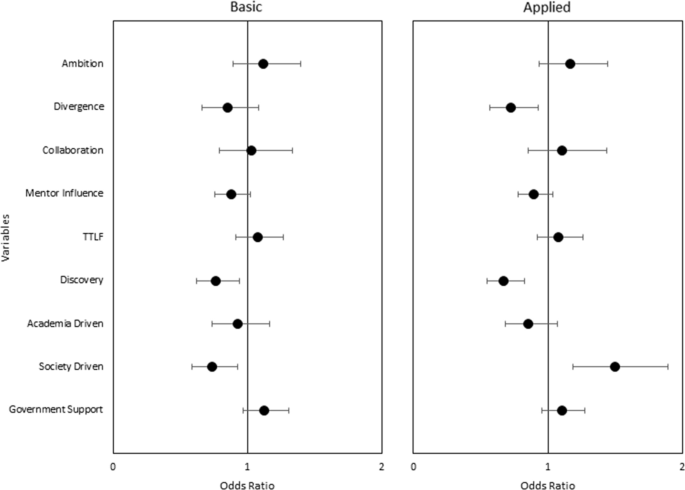
Forest plot of odds ratio for the various SRA variables
In terms of the control variables, most were not statistically significant. However, having non-academic experience increased the likelihood of belonging to the Applied cluster, relative to the Balanced cluster ( B = 0.887, p < 0.01, OR 2.427). Being silver-corded rather than non-mobile increased the odds of membership in the Basic cluster relative to the Balanced cluster ( B = 0.918, p < 0.05, OR 2.504). This was also the case for being a Mobile International ( B = 0.679, p < 0.05, OR 1.972), but none of the other mobility types had a significant impact on placement in the Applied cluster. Finally, an increased percentage of time dedicated to Knowledge Transfer reduced the odds of placement in the Basic cluster ( B = − 0.068, p < 0.01, OR 0.934). Contrary to the literature, we found that full professors were more engaged in basic research than associate and assistant professors ( B = − 0.498, p < 0.1, OR 0.608), and there were no statistical differences between genders, recipients of external funding, or between fields of science, suggesting that the research dynamics of academics in developing scientific systems may be quite distinct from those in developed scientific systems.
This paper’s results regarding research preference clustering are very similar to those of Werker and Hopp ( 2020 ). Only a relatively small number of academics can synergistically pursue basic research, applied research, and experimental development. This group of academics was the smallest of the three research preference clusters in our sample. The other two clusters, although showing marginal levels of complementarity, were dominated by a single research preference, either basic or applied research. This is somewhat at odds with the findings of Gulbrandsen and Kyvik ( 2010 ) and Bentley et al. ( 2015 ), as our findings suggest relatively strong research specialization, as evidenced by low levels of complementarity and research focuses that are moderately dominated by a single preference (e.g., basic research). Nonetheless, similarly to Gulbrandsen and Kyvik ( 2010 ) and Bentley et al. ( 2015 ), we found that the number of academics who prefer applied research exceeds those preferring basic research and that external funding and national and institutional strategies had little or no effect on academics’ research focuses. Our findings may differ from the papers mentioned above for two reasons. First, those papers are not recent, and academia has recently endured substantial pressure that has transformed the way academics conceptualize research and how they act when doing research. Second, it is possible that in countries with developing scientific and academic systems, stronger specializations in basic and applied research may still exist either because academic knowledge production is still dominated by traditional, disciplinary, and hierarchical modes of knowledge production or because there are fewer opportunities for triple, quadruple, or quintuple helixes in the academic sector (Jaramillo et al., 2016 ).
Responding to the second research question driving this study, we demonstrated that four of academics’ SRAs were moderately associated with their individual preferences for basic research, applied research, or experimental development. Notably, high Discovery scores were associated with a lower preference for basic and applied research; higher Divergence scores were associated with a lower preference for applied research; higher Mentor Influence was associated with a lower preference for basic research; and higher Society Driven scores were associated with a lower preference for basic research but a higher preference for applied research.
Regarding the research questions driving this study, we made two other major findings.
The first important finding is related to the relationships between the individual SRA dimensions and research focus preferences. In particular, we find that academics of the balanced cluster have high scores on the Discovery dimension. This suggests that academics interested in research that has the potential to result in breakthroughs generally combine the three types of research. This may be because combining focuses results in a complex articulation of ideas, research approaches, and uses for the knowledge they acquire, leading to the creation of new knowledge, products, and services with the potential for added value. However, it may also relate to the high stakes, high risks, and high costs of the development of products and services that is typical of experimental development. Since the Divergence scores (i.e., multidisciplinarity) for academics in the balanced cluster are not statistically different from those in the basic research cluster, Footnote 5 the latter explanation may be the most likely. However, there are no statistical differences between fields of science, suggesting that the higher Discovery score of academics who adopt a balanced research focus does not seem to have more to do with the riskiness of experimental development. Experimental development can be found in all fields of science, although it is riskier and costlier in some than others (see Olmos-Peñuela et al., 2014 ; Sandoz, 2021 ). This is a finding which explanation is hard to pinpoint and requires further research. The fact that there are no statistical differences in the research preferences of academics in different fields of science is also important per se; although some fields of science might be expected to be more applied than others (see Gulbrandsen & Kyvik, 2010 ), this does not seem to influence the research focus preferences of the academics in our sample. The same is true of the findings concerning gender: we do not find different research focus preferences between male and female academics, which is inconsistent with other studies indicating that male academics lean toward the basic sciences and female academics lean toward the applied sciences (Zhang et al., 2021 ). Furthermore, full professors in Mexican research universities do not seem to lean toward applied research, as other studies have found (e.g., Gulbrandsen & Smeby, 2005 ); instead, they prefer to focus on basic research. The explanation for the inconsistency of these findings when compared to the literature seems to be related to differences between academics working in developed and developing countries and are relevant for policy purposes, underlining the relevance of understanding national and developmental characteristics and dynamics. Our finding that academics who have worked outside academia tend to have a more applied research profile is consistent with the literature.
Our second main finding is related to the additional control variables, which have not been tested previously. Most of them have little effect on the research preferences of academics. Career mobility has limited effect on the research preferences of academics: academics who are currently working in the university where they obtained their Ph.D. after having worked somewhere else and academics with work experiences abroad are more inclined to prefer basic research to a more balanced approach than academics in the career immobile group. Work allocation also has a small impact on the research preferences of academics: academics dedicating more time to knowledge transfer activities are less likely to engage in basic research, which is consistent with the literature (e.g., Gulbrandsen & Thune, 2017 ). The number of years in academia has no influence on research preferences.
In addition to these findings, we test and validate the MDRAI-R in a new setting. We demonstrate strong psychometric properties, consistent with previous validation exercises. We also introduce a new dimension (i.e., Government), transforming the MDRAI-R into the MDRAI-R-S, which is a more optimized instrument, now available in both English (Appendix 1) and Spanish (Appendix 2). This will allow researchers to use the instrument in Spanish-speaking countries, particularly in Latin America, where it can be of important practical use for policymakers, research managers, academics, and researchers in or outside of academia.
This study has certain limitations. Two issues typically arise from non-probabilistic sampling: undercoverage, which occurs when members of the population have a zero chance of being selected, and the inability to accurately calculate the probability of a given member of the population being selected for the sample (Hirschauer et al., 2020 ). Undercoverage was not an issue because the entire population of interest was contacted. The second problem was not initially an issue because each member of the population had an ex-ante equal probability of being part of the sample: 100%. However, any response rate that falls short of 100% leads to the possibility of self-selection bias. Although we compared the sample to the population distribution of institutions, and it was nearly identical, potential confounding factors that could lead to self-exclusion from the survey, such as gender, age, or other socio-demographical characteristics, were not addressed. The literature has acknowledged the impossibility of accounting for all the potential confounding factors that can lead to self-exclusion (Hirschauer et al., 2020 ), and as such, while there is evidence in favor of the sample’s representativeness at least as far as the population’s institutions are concerned, the reader should be aware of the non-probabilistic nature of the sample when evaluating our findings. Additionally, this study focused specifically on Mexican institutions, and the findings may not be generalizable to other populations.
In some countries, such as China, the opposite trend is observed, with governments attempting to shift the focus from applied to basic research, although an overwhelming amount of government funding is still directed to the natural sciences and engineering (Huang et al., 2014 ).
SRAs are personal choices that result from a combination of factors related to individual and social goals and interests, influenced by scholarly communities and others, as well as by other considerations, including career perspectives and institutional pressures that are bound to influence topical research choices and engagement (Santos et al., 2020 ).
For Field of Science classification, the participants were manually classified by the authors using the OECD’s Frascati Manual classification scheme (OECD, 2015 ), under one of its six categories: Natural Sciences, Engineering & Technology, Medical & Health Sciences, Agricultural Sciences, Social Sciences, and Humanities.
The Odds Ratios are reported throughout this section as “OR”.
Some of the SRA variables that were shown to vary between clusters in the ANOVA analysis (Analyses 3), such as Divergence, Mentor Influence, and Academia Driven, lost statistical significance after the introduction of control variables in the multinominal regression (Table 4 ). Although Divergence and Mentor Influence retained statistical significance for some pairs, Academia Driven became completely insignificant.
Abreu, M., & Grinevich, V. (2016). Gender patterns in academic entrepreneurship. The Journal of Technology Transfer, 42 , 763–794.
Article Google Scholar
Almeida, J. A. S., Pais, A. A. C. C., & Formosinho, S. J. (2009). Science indicators and science patterns in Europe. Journal of Informetrics, 3 (2), 134–142.
Anastasi, A., & Urbina, S. (1997). Psychological testing . Prentice Hall/Pearson Education.
Google Scholar
Arbuckle, J. (2007). Amos 16.0 user’s guide. SPSS, Chicago, IL.
Barrett, P. (2007). Structural equation modelling: Adjudging model fit. Personality and Individual Differences, 42 (5), 815–824.
Article MathSciNet Google Scholar
Bentler, P. M. (1990). Comparative fit indexes in structural models. Psychological Bulletin, 107 (2), 238–246.
Bentley, P. J., Gulbrandsen, M., & Kyvik, S. (2015). The relationship between basic and applied research in universities. Higher Education, 70 (4), 689–709.
DiStefano, C., Zhu, M., & Mindrila, D. (2009). Understanding and using factor scores: Considerations for the applied researcher. Practical Assessment, Research & Evaluation, 14 (20), 1–11.
Enders, C. K., & Bandalos, D. L. (2001). The relative performance of full information maximum likelihood estimation for missing data in structural equation models. Structural Equation Modeling, 8 (3), 430–457.
Fan, X., Yang, X., & Yu, Z. (2021). Effect of basic research and applied research on the universities’ innovation capabilities: The moderating role of private research funding. Scientometrics, 126 , 5387–5411.
Fornell, C., & Larcker, D. F. (1981). Evaluating structural equation models with unobservable variables and measurement error. Journal of Marketing Research, 18 , 39–50.
Gaskin, J. (2016). Stats Tool Package. http://statwiki.kolobkreations.com
Gläser, J., & Laudel, G. (2016). Governing science: How science policy shapes research content. European Journal of Sociology, 57 (1), 117–168. https://doi.org/10.1017/S0003975616000047
Godin, B. (2002). Outline for a history of science measurement. Science, Technology, and Human Values, 27 (1), 3–27.
Godin, B. (2006). Research and development: How the ‘D’ got into R&D. Science and Public Policy, 33 (1), 59–76.
Gulbrandsen, M., & Kyvik, S. (2010). Are the concepts of basic research, applied research and experimental development still useful? An empirical investigation among Norwegian academics. Science and Public Policy, 37 (5), 343–353.
Gulbrandsen, M., & Smeby, J.-C. (2005). Industry funding and university professors’ research performance. Research Policy, 34 (6), 932–950.
Gulbrandsen, M., & Thune, T. (2017). The effects of non-academic work experience on external interaction and research performance. The Journal of Technology Transfer, 42 , 795–813.
Hair, J. F., Black, W. C., Babin, B. J., & Anderson, R. E. (2014). Multivariate data analysis . Pearson Education Limited.
Hirschauer, N., Grüner, S., Mußhoff, O., Becker, C., & Jantsch, A. (2020). Can p-values be meaningfully interpreted without random sampling? Statistics Surveys, 14 , 71–91. https://doi.org/10.1214/20-SS129
Article MathSciNet MATH Google Scholar
Hirzel, S., Hettesheimer, T., Viebahn, P., & Fischedick, M. (2018). A decision support system for public funding of experimental development in energy research. Energies, 11 (6), 1357. https://doi.org/10.3390/en11061357
Horta, H., & Santos, J. M. (2020). The multidimensional research agendas inventory-revised (MDRAI-R): Factors shaping researchers’ research agendas in all fields of knowledge. Quantitative Science Studies, 1 (1), 60–93.
Horta, H., Feng, S., & Santos, J. M. (2021). Homophily in higher education research: A perspective based on co-authorships. Scientometrics . https://doi.org/10.1007/s11192-021-04227-z
Horta, H., Veloso, F. M., & Grediaga, R. (2010). Navel gazing: Academic inbreeding and scientific productivity. Management Science, 56 (3), 414–429.
Huang, C., Su, J., Xie, X., & Li, J. (2014). Basic research is overshadowed by applied research in China: A policy perspective. Scientometrics, 99 , 689–694.
Jaramillo, P., Estefania, I., & Güemes Castorena, D. (2016). Identification of key factors of academia in the process of linking in the triple helix of innovation model in Mexico: A state of the art matrix. Nova Scientia, 8 (16), 246–277.
Jongbloed, B., Enders, J., & Salerno, C. (2008). Higher education and its communities: Interconnections, interdependencies and a research agenda. Higher Education, 56 , 303–324.
Kaufman, L., & Rousseeuw, P. J. (2009). Finding groups in data: An introduction to cluster analysis (Vol. 344). Wiley.
MATH Google Scholar
Kline, R. B. (2016). Principles and practice of structural equation modeling . Guilford Press.
Kowalczewska, K., & Behagel, J. (2019). How policymakers’ demands for usable knowledge shape science-policy relations in environmental policy in Poland. Science and Public Policy, 46 (3), 381–390.
Larivière, V., Macaluso, B., Mongeon, P., Siler, K., & Sugimoto, C. R. (2018). Vanishing industries and the rising monopoly of universities in published research. PLoS ONE, 13 (8), e0202120. https://doi.org/10.1371/journal.pone.0202120
Mallon, M., Duberley, J., & Cohen, L. (2005). Careers in public sector science: Orientations and implications. R&D Management, 35 , 395–407.
Marôco, J. (2003). Análise estatística: Com utilização do SPSS . Edições Sílabo.
Marôco, J. (2010). Análise de equações estruturais: Fundamentos teóricos, software & aplicações. ReportNumber, Lda.
Marques, M., Powell, J. J. W., Zapp, M., & Biesta, G. (2017). How does research evaluation impact educational research? Exploring intended and unintended consequences of research assessment in the United Kingdom, 1986–2014. European Educational Research Journal, 6 (6), 820–842.
Mittermeir, R., & Knorr, K. D. (1979). Scientific productivity and accumulative advantage: A thesis reassessed in the light of international data. R&D Management, 9 , 235–239.
Norusis, M. J. (2012). IBM SPSS statistics 19 statistical procedures companion . Prentice Hall.
OECD. (2015). Frascati Manual 2015: Guidelines for Collecting and Reporting Data on Research and Experimental Development . OECD Publishing.
Book Google Scholar
Olmos-Peñuela, J., Benneworth, P., & Castro-Martinez, E. (2014). Are sciences essential and humanities elective? Disentangling competing claims for humanities’ research public value. Arts and Humanities in Higher Education, 14 (1), 61–78.
Overland, I., & Sovacool, B. K. (2020). The misallocation of climate research funding. Energy Research & Social Science, 62 , 101349. https://doi.org/10.1016/j.erss.2019.101349
Ranga, L., Debackere, K., & Tunzelmann, N. (2003). Entrepreneurial universities and the dynamics of academic knowledge production: A case study of basic vs. applied research in Belgium. Scientometrics, 58 , 301–320.
Raynor, K. (2019). Participatory action research and early career researchers: The structural barriers to engagement and why we should do it anyway. Planning Theory & Practice, 20 (1), 130–136.
Roll-Hansen, N. (2017). A historical perspective on the distinction between basic and applied science. Journal of General Philosophy of Science, 48 , 535–551.
Rousseeuw, P. J. (1987). Silhouettes: A graphical aid to the interpretation and validation of cluster analysis. Journal of Computational and Applied Mathematics, 20 , 53–65. https://doi.org/10.1016/0377-0427(87)90125-7
Article MATH Google Scholar
Salter, A. J., & Martin, B. R. (2001). The economic benefits of publicly funded basic research: A critical review. Research Policy, 30 (3), 509–532.
Sandoz, R. (2021). Thematic reclassifications and emerging sciences. Journal for General Philosophy of Science, 52 , 63–85.
Santos, J. M., & Horta, H. (2015). The generational gap of science: A dynamic cluster analysis of doctorates in an evolving scientific system. Scientometrics, 104 (1), 381–406.
Santos, J. M., & Horta, H. (2018). The research agenda setting of higher education researchers. Higher Education, 76 , 649–668.
Santos, J. M., & Horta, H. (2020). The association between researchers’ conceptions of research and their strategic research agendas. Journal of Data and Information Science, 5 (4), 56–74.
Santos, J. M., Horta, H., & Amâncio, L. (2021). Research agendas of female and male academics: A new perspective on gender disparities in academia. Gender and Education, 33 (5), 625–643.
Santos, J. M., Horta, H., & Li, H. (2022). Are the strategic research agendas of researchers in the social sciences determinants of research productivity? Scientometrics . https://doi.org/10.1007/s11192-022-04324-7
Santos, J. M., Horta, H., & Zhang, L.-F. (2020). The association of thinking styles with research agendas among academics in the social sciences. Higher Education Quarterly, 74 (2), 193–210.
Sapir, A. (2017). Protecting the purity of pure research: Organizational boundary work at an institute of basic research. Minerva, 55 , 65–91.
Schauz, D. (2014). What is basic research? Insights from historical semantics. Minerva, 52 , 273–328.
Steiger, J. H., Shapiro, A., & Browne, M. W. (1985). On the multivariate asymptotic distribution of sequential chi-square statistics. Psychometrika, 50 (3), 253–263.
Tukey, J. (1953). Multiple comparisons. Journal of the American Statistical Association, 48 (263), 624–625.
Valentin, F., Norn, M. T., & Alkaersig, L. (2016). Orientations and outcome of interdisciplinary research: The case of research behavior in translational medical science. Scientometrics, 106 , 67–90.
Werker, C., & Hopp, C. (2020). Balancing act between research and application: How research orientation and networks affect scholars’ academic and commercial output. Journal of Business Economics, 90 , 1171–1197.
Zapp, M., & Powell, J. J. (2017). Moving towards Mode 2? Evidence-based policy-making and the changing conditions for educational research in Germany. Science and Public Policy, 44 (5), 645–655.
Zhang, L., Sivertsen, G., Duj, H., Huang, Y., & Glanzel, W. (2021). Gender differences in the aims and impacts of research. Scientometrics, 126 , 8861–8886.
Zhang, Z. (2016). Missing data imputation: Focusing on single imputation. Annals of Translational Medicine, 4 (1), 9. https://doi.org/10.3978/j.issn.2305-5839.2015.12.38
Zhang, T., Ramakrishnon, R., & Livny, M. (1996). BIRCH: An efficient data clustering method for very large data bases (pp. 103–114). Montreal, Canada
Download references
Funding was provided by CONACYT (Grant No. 2018-000070-02EXTF-00077).
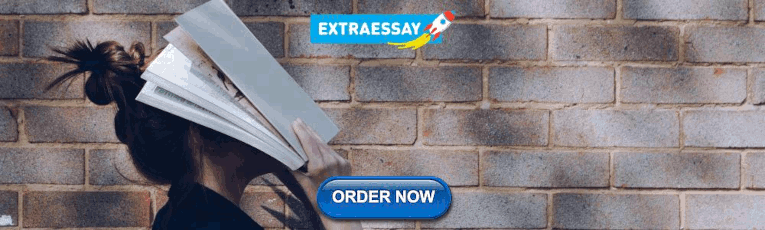
Author information
Authors and affiliations.
Iscte – Instituto Universitário de Lisboa, Centro de Investigação e Estudos de Sociologia (Cies_Iscte), Lisbon, Portugal
J. M. Santos
Social Contexts and Policies of Education, Faculty of Education, The University of Hong Kong, Hong Kong SAR, China
H. Horta & H. Luna
You can also search for this author in PubMed Google Scholar
Corresponding author
Correspondence to H. Horta .
Multi-Dimensional Research Agendas Inventory – Revised-S (MDRAI-R-S)
You will be asked a series of questions regarding your motivations and goals as an academic. Please read and determine your level of agreement with each statement. Then, check one of the seven boxes next to the corresponding item. If you do not know or a particular sentence does not apply to you, check the N/A box.
Some questions will ask about your field, and others will ask about your research topics. Please consider “field” to be the main theme of your research (for example, "higher education"), and “research topic” as a specific subject within the main theme (e.g., "doctoral education" and “access to higher education” would be research topics in the "higher education" theme). “Field community” is also a term that you will encounter while you complete the survey. “Field community” is defined as the research/scholarly community(ies) with which you identify. Keep these definitions in mind when you respond to the questions.
There are no right or wrong answers. Please read each statement and check the box that best applies to you. How much do you agree with the following statements?
A continuación se le harán una serie de preguntas acerca de sus motivaciones y metas como académico.
Por favor haga click en solo una de las siete opciones a la derecha de cada planteamiento dependiendo de su nivel de acuerdo o desacuerdo. siendo por ejemplo el número 1 el nivel de mayor desacuerdo, así como el número 7 el número de mayor acuerdo para cada enunciado.
Algunas preguntas se harán de acuerdo a su campo de trabajo y otra respecto a su campo de investigación.
Favor de considerar “campo de trabajo” como el tema principal de su investigación (por ejemplo, “educación superior”) y su “campo de investigación” como un área específica de su tema principal (e.g. “educación doctoral” o “acceso a la educación superior”, serán considerados como temas de investigación entre los temas a abordar dentro del área de “educación superior”), “comunidad de investigación” es un término que se encontrará durante el transcurso de esta encuesta. “Comunidad de investigación” es definida como la comunidad escolar o académica con la cual usted se identifica. Es importante tener estas definiciones en mente al responder a las preguntas que enseguida se muestran.
Rights and permissions
Reprints and permissions
About this article
Santos, J.M., Horta, H. & Luna, H. The relationship between academics’ strategic research agendas and their preferences for basic research, applied research, or experimental development. Scientometrics 127 , 4191–4225 (2022). https://doi.org/10.1007/s11192-022-04431-5
Download citation
Received : 31 January 2022
Accepted : 31 May 2022
Published : 15 July 2022
Issue Date : July 2022
DOI : https://doi.org/10.1007/s11192-022-04431-5
Share this article
Anyone you share the following link with will be able to read this content:
Sorry, a shareable link is not currently available for this article.
Provided by the Springer Nature SharedIt content-sharing initiative
- Strategic research agendas
- Basic research
- Applied research
- Experimental development
- Academic preferences
- Find a journal
- Publish with us
- Track your research
Strategic Research Agenda
The 2020 Strategic Research Agenda (SRA) is an update from the 2016 SRA and takes into account FACCE-JPI’s past work and achievements and input from the members of FACCE-JPI as represented in the Governing Board. Member States’ national priorities that need to be highlighted were specified and integrated in the text, along with the JPI’s past and ongoing work with other European and international initiatives. It also takes into account the context of Horizon Europe with its new mission areas and partnerships.
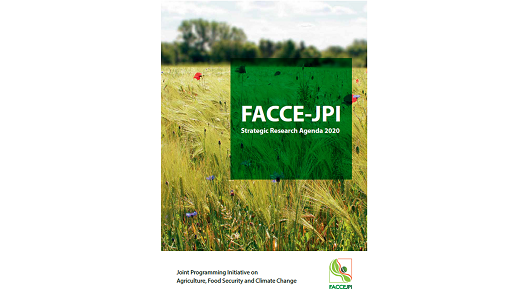
The new FACCE-JPI Strategic Research Agenda 2020 was presented at the FACCE-JPI 10 Year Event “A world in transition: Journey towards a sustainable future”.
The European Commission has set out to make Europe the frontrunner for climate and green ambitions, requiring significant investment in research and innovation, which should provide the foundation of future policy decisions. European Member States and Associated Countries need to cooperate more than ever to cope with the growing challenges and the European Research Area needs to be reinforced. FACCE-JPI is a leading Member State-driven initiative that over the last ten years has worked at the intersection of challenges linked to agriculture, food security and climate change. In the current context of continued urgent global challenges and changing political priorities, FACCE-JPI is rising to the occasion with its new and ambitious Strategic Research Agenda. Accordingly, the FACCE-JPI Scientific Advisory Board and Stakeholder Advisory Board have worked with the Governing Board to propose a new Vision and Mission and 4 new Core Themes, which are presented in an accessible document. As before, this SRA will be implemented through successive Implementation Plans.
Through the co-creation approach used to prepare this document, FACCE-JPI hopes to create a positive environment in which we can work together to tackle the enormous challenges we face in an effective and synergistic manner to achieve a positive impact on Agriculture, Food Security and Climate Change. The JPI aims to reinforce infrastructures and platforms, training and capacity building in Europe and knowledge exchange. It also foresees evaluation and monitoring of its activities.
In order to demonstrate impact and increase visibility, it is necessary to exploit and disseminate the results coming from different FACCE-JPI funded activities, as well as to propose the best ways to optimise their value and communicate them by using the most adapted tools for different target audiences. The FACCE-JPI Communication and Valorisation Strategy “Science for Policy and Impact” , currently being updated, aims to provide a strategic framework for all joint actions and activities funded by FACCE-JPI, from its start to 2020 and beyond.

Research Application Michigan Education Data Center
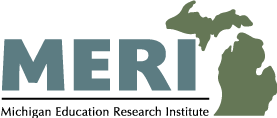
Michigan Department of Education | Strategic Research Agenda
We invite researchers to propose projects to help further the goals outlined in Michigan’s Top 10 Strategic Education Plan:
- Expand early childhood learning opportunities
- Improve early literacy achievement
- Improve the health, safety, and wellness of all learners
- Expand secondary learning opportunities for all students
- Increase the percentage of all students who graduate from high school
- Increase the percentage of adults with a postsecondary credential
- Increase the numbers of certified teachers in areas of shortage
- Provide adequate and equitable school funding
Programmatic Priority Areas for MDE
We have particular interest in research partnerships that help us with our three priority areas:
- Literacy and numeracy
- Supporting the whole child
- Supporting our youngest learners (prenatal to age 8)
Note: Within those areas, we need to consider policies; student/instructional level programs and interventions or strategies; educator workforce capacity; resources; and barriers to implementation. Those considerations could be impacted by very different types of studies.
The MDE supports research that helps us implement Michigan's Top 10 Strategic Education Plan. We seek partners who are willing to co-develop and implement research studies that help us examine the priorities listed below.
Strategic Education Principle 1: All students have access to high-quality instruction regardless of their gender, sexual orientation, ethnicity, race, economic status, native language, or physical, emotional, and cognitive abilities to close the student achievement and opportunity gaps that currently exist.
Priority Research Topics:
- What instructional approaches best support student learning, with particular attention paid to questions of implementation, scale up, and conditions necessary for success?
- Research into strategies, practices, and interventions that best support Michigan’s diverse learner population, including English learners, students with disabilities, economically disadvantaged students, Michigan’s indigenous students, etc.
- How can the MDE leverage social media, social networks, and/or technology to disseminate and share information related to high quality instructional practices in new ways?
- How is our Partnership District model working to turn around low-performing schools and districts?
- Studies that provide the MDE with evidence on promising practices related to supporting the needs of the whole child.
- Financial equity studies related to school finance.
- How can personalized learning approaches and competency-based education help with student outcomes—academic as well as other outcomes of interest?
Strategic Education Principle 2: All educators are encouraged to be creative and innovative. All educators are adequately compensated and respected for their professionalism, and have the resources, support, and training needed to educate students.
- Implementing and evaluating strategies to increase the diversity of the educator workforce, as well as identifying research-based strategies for this work.
- Understanding how the educator pipeline is functioning at each stage (recruitment, preparation, certification, placement, mentoring/induction, evaluation and professional learning) and what helps ensure the highest-quality teachers possible in Michigan?
- Impact of potential changes to the pipeline
- What are some evidence-based recruitment strategies, and which strategies are at the SEA level, the LEA level and elsewhere?
Strategic Education Principle 3: All students are encouraged to express their creativity, have voice in their own learning, feel connected to their schools, and have authentic, meaningful relationships with educators.
- Engaging student voice to identify programs and supports needed in Michigan schools and districts, and to evaluate the efficacy of programs already in place.
- Research into the impact of diverse curricular materials on student engagement, aspirations and outcomes.
- Understanding the landscape of P-20 programming and opportunities in Michigan LEAs, and how those various pathways relate to student outcomes—academic, whole child, and postsecondary transitions?
- How are we using career pathways as a state and how do those impact student outcomes?
- Deeper research into any component of the P-20 system including the early childhood, K-12 and postsecondary programmatic offerings and connection to outcomes.
Strategic Education Principle 4: All students are provided every opportunity to achieve the broadest range of life dreams.
- Research outlined under Principle 3 above related to the P-20 pipeline and various student pathways.
- Research and partnerships that provide evidence about the success of various types of partnerships with job providers, community colleges and higher education.
Strategic Education Principle 5: Families and communities are essential partners of teachers, support staff, and administrators in the education of students.
- Partnerships and studies that test various strategies to increase parental engagement and provide the MDE with information on “what works” in terms of increased parent/guardian engagement and satisfaction.
- Partnerships and studies that investigate the relationship between parent/guardian engagement and student outcomes (academic and non-academic/whole child).
Strategic Education Principle 6: In support of students and their achievement, the Michigan Department of Education is coordinated, aligned, and properly resourced, and collaborates with school districts and a wide range of partners and stakeholders.
- What types of preparation and training help create SEA leaders and employees who can enact a vision of an innovative and cohesive state education agency?
Detailed List of Possible Questions
The MDE has a more detailed list of possible questions of interest, prioritized internally. However, this is not a comprehensive list; research partners can and should propose other topics that broadly fit the goals outlined above. The prioritization can also change based on available partners and/or funding—something that is described as “long term” may move to “near future” with the right combination of interest, partners and funding.
© 2024 All Rights Reserved - Michigan Education Research Institute

An official website of the United States government
The .gov means it’s official. Federal government websites often end in .gov or .mil. Before sharing sensitive information, make sure you’re on a federal government site.
The site is secure. The https:// ensures that you are connecting to the official website and that any information you provide is encrypted and transmitted securely.
- Publications
- Account settings
Preview improvements coming to the PMC website in October 2024. Learn More or Try it out now .
- Advanced Search
- Journal List
- Insights Imaging
- v.10; 2019 Dec

Strategic research agenda for biomedical imaging
The european institute for biomedical imaging research (eibir).
The European Institute for Biomedical Imaging Research, Vienna, Austria
This Strategic Research Agenda identifies current challenges and needs in healthcare, illustrates how biomedical imaging and derived data can help to address these, and aims to stimulate dedicated research funding efforts.
Medicine is currently moving towards a more tailored, patient-centric approach by providing personalised solutions for the individual patient. Innovation in biomedical imaging plays a key role in this process as it addresses the current needs for individualised prevention, treatment, therapy response monitoring, and image-guided surgery.
The use of non-invasive biomarkers facilitates better therapy prediction and monitoring, leading to improved patient outcomes. Innovative diagnostic imaging technologies provide information about disease characteristics which, coupled with biological, genetic and -omics data, will contribute to an individualised diagnosis and therapy approach.
In the emerging field of theranostics, imaging tools together with therapeutic agents enable the selection of best treatments and allow tailored therapeutic interventions.
For prenatal monitoring, the use of innovative imaging technologies can ensure an early detection of malfunctions or disease.
The application of biomedical imaging for diagnosis and management of lifestyle-induced diseases will help to avoid disease development through lifestyle changes.
Artificial intelligence and machine learning in imaging will facilitate the improvement of image interpretation and lead to better disease prediction and therapy planning.
As biomedical imaging technologies and analysis of existing imaging data provide solutions to current challenges and needs in healthcare, appropriate funding for dedicated research is needed to implement the innovative approaches for the wellbeing of citizens and patients.
- Innovative biomedical imaging plays a crucial role in personalised medicine, disease prevention and therapy monitoring
- Innovative biomedical imaging technologies and analysis of existing imaging data provide solutions to current challenges and needs in healthcare
- Appropriate funding for biomedical imaging research is necessary to implement innovative approaches addressing citizens’ and patients’ needs
Introduction
Medicine and healthcare in general are experiencing a major change, moving from reactive to proactive approaches by providing predictive, preventive and personalised medical solutions for the individual patient. Particularly, the concept of personalised medicine is promoted by the European Commission as it addresses the challenges of ineffective treatment and rising healthcare costs with patient-centred prevention and treatment plans. Personalised and precision medicine has a huge growth potential, which provides Europe’s healthcare industry with the opportunity to further expand its leading position, contributing to economic growth and job creation.
Furthermore, Europe needs research, innovation and applications for the benefit of all. In this context, areas of research have to be prioritised and research programmes should focus on high-impact outcomes [ 1 ]. In its recently launched work programmes [ 2 ], the European Commission has identified a number of healthcare challenges, e.g. the rising costs of health and care or the influence of external environmental factors including climate change on health, that need to be addressed by Europe’s research community to develop sustainable solutions that will help to overcome those challenges, improve the treatment of patients and lead to better health and wellbeing for European citizens. The development and adoption of good-quality and safe e-services can ensure that the health systems of Member States become more efficient and sustainable [ 3 ].
However, as stated in the European Council’s conclusions on Health in the Digital Society [ 4 ], new opportunities are arising from big data and improved data analytics capabilities, yet barriers to scaling up the potential in digital health and connected care still need to be overcome. These include the dominance of data silos, lack of interoperability and of common standards for measuring clinical and patient reported outcomes, and limited access and use of large databases for research and innovation purposes. Moreover, a lack of funding and financial incentives exists, which together with market fragmentation in the EU and across the spectrum of services further impedes innovation. Progress in implementing data-driven digital solutions in healthcare requires rigorous validation and testing of new solutions’ clinical effectiveness.
It is the aim of this Strategic Research Agenda for Biomedical Imaging to:
- Identify the current challenges and needs in medicine and healthcare
- Illustrate how biomedical imaging can help address these challenges
- Stimulate dedicated research funding efforts
Innovative imaging technologies are now allowing researchers to visualise, characterise and measure biological and molecular phenomena with a hitherto unattainable precision. For the first time in history, hallmark processes of human disease, such as tumourigenesis or molecular deposits, can be visualised in real time. Imaging technologies and computerised radiomics allow breakthrough discoveries and their application in healthcare and are thus the central tool driving fundamental research in biomedicine. Technological innovation in biomedical imaging has been growing in the last decades and significant advances in the research fields of photonics, physics, chemistry and computing are often quickly translated into instrumentation or procedures for biomedical imaging. This may accelerate medical advances, but also calls for a need to adapt existing and to implement new methods and care pathways for better patient outcomes.
Biomedical imaging is a key component in personalised medicine [ 5 ]:
- Personalised prevention will rely on non-invasive or minimally invasive image-based screening programmes [ 5 ]
- Structural, functional, physiological and molecular imaging biomarkers affect decisions on the type and intensity of treatment [ 5 ]
- Treatment response assessment with imaging biomarkers will improve personalised and targeted treatment [ 5 ]
- Imaging-supported non- or minimally invasive intervention integrates precision diagnosis and personalised treatment [ 5 ] and will contribute to value-based healthcare [ 6 ]
Moreover, biomedical imaging is a forerunner in the digital transformation of healthcare and a leading discipline in the adoption of new innovative solutions like deep learning and artificial intelligence. Digital health is essential for ensuring that breakthroughs or innovations in clinical research are translated into practice cost-effectively.
The European Institute for Biomedical Imaging Research (EIBIR) is a non-profit organisation with a network of more than 80 leading European research institutions and 11 European scientific societies related to biomedical imaging as shareholders. EIBIR is committed to coordinate and support the development of biomedical imaging technologies and the dissemination of knowledge with the ultimate goal of improving the diagnosis, treatment and prevention of disease. It supports research networking activities and plays a key role in spreading good practice and promoting common initiatives and interoperability in the field of biomedical imaging research ( www.eibir.org ).
EIBIR, together with its members and shareholder organisations, has identified five main challenges which biomedical imaging can help overcome:
- Challenge 1 : Meeting the healthcare demands of Europe’s population through personalised disease prevention and therapy monitoring facilitated by medical imaging
- Challenge 2 : Developing new disease-specific, targeted and image-guided therapies
- Challenge 3 : Contributing to a healthy start by delivering early and improved information on foetal health and prenatal growth, and preventing the development of anomalies using advanced imaging technologies
- Challenge 4 : Providing accurate assessments of the impact of lifestyle and environmental factors on health supported by medical imaging
- Challenge 5 : Making Europe the world leader in machine-learning and artificial intelligence in medical imaging by exploiting existing data and expertise to implement digital solutions after rigorous clinical validation.
Challenge 1: Meeting the healthcare demands of Europe’s population through personalised disease prevention and therapy monitoring facilitated by medical imaging
Precision medicine has emerged as a novel healthcare paradigm during the past decade.
The approach for risk prediction and disease prevention taking into account individual gene variability as well as environmental and lifestyle factors involves the integration of information from multiple sources to achieve patient population stratification and provide more specific diagnoses, focused treatment and better response assessment. Deep phenotyping in combination with genetic, biochemical and physiologic biomarkers allows prediction and early assessment of disease. The emerging field of radiomics links genotypic information to phenotypic disease manifestations using imaging. Radiomics can greatly contribute to patient-tailored prevention and care.
Special attention should be devoted to the development of safe and more specific contrast agents, and the optimisation of acquisition procedures to the characteristics of the treated patient/pathology enabling precision radiology.
Clinically oriented research on quality and safety of medical imaging is an essential aspect when advancing precision radiology. In 2017, five EIBIR shareholder organisations established the European Alliance for Medical Radiation Protection Research (EURAMED; www.euramed.eu ) and published a strategic research agenda for radiation protection in medicine [ 7 ], highlighting the research needs in this field and encouraging sustainable European efforts.
Imaging biomarkers are objectively measurable indicators of biological processes and can be used for the prediction of patient outcome (regardless of therapy) and response to specific therapies, and for response assessment (monitoring) [ 8 ]. The use of imaging biomarkers is non-invasive—a clear advantage over invasive biopsies [ 8 ]. Another advantage is the assessment and quantification of cellular targets for the entire disease burden, avoiding sampling errors that can occur with heterogeneous expressions, as well as the potential for serial studies of the in vivo effects of a drug or action on the target [ 8 ]. In addition, imaging biomarkers can unravel specific localised biological pathways and enable therapeutic targeting of such specific biomolecular mechanisms [ 8 ].
Joint efforts between research institutions and technology vendors should lead to the implementation of standards in image acquisition, production, archiving and distribution, and ethical use of sensitive data. Such approaches are a prerequisite for sharing large imaging data sets across Europe and for the discovery, adoption and wider use of predictive, prognostic and diagnostic imaging biomarkers. The European Imaging Biomarker Alliance (EIBALL) is a joint initiative operating in conjunction with EIBIR and in collaboration with the Quantitative Imaging Biomarkers Alliance (QIBA) and the European Organisation for Research and Treatment of Cancer (EORTC) for the development and validation of new imaging biomarkers.
Examples addressing Challenge 1
Example 1: brca1/2 carriers, enhanced imaging-based monitoring for breast and ovarian cancer (conventional mammography vs. contrast-enhanced mri).
Persons carrying mutations in the BRCA1/2 gene are more likely to develop breast cancer (men and women), ovarian cancer in women and prostate cancer in men. Genetic screening of high-risk families allows the possibility of early detection of disease or indeed prevention. There is now compelling evidence that MRI is the most appropriate screening test for breast cancer in BRCA1/2 carriers. However, screening programmes are predominantly based on mammography. Future screening programmes are needed to fully evaluate the position of MRI in screening women in high-risk categories. In ovarian cancer (Fig. 1 ), but also in other cancers such as prostate cancer, biomarkers of early disease in BRCA carriers, such as circulating DNA, are highly likely to form the basis of future screening in BRCA carriers in conjunction with imaging tests, including ultrasound (US) and MRI. Imaging has been highly successful in determining the likelihood of malignancy in ovarian and prostate masses. Research into translating this to screening practice is of utmost importance, particularly in relation to early detection of disease and treatment stratification by combining circulating and imaging biomarkers.

MRI scan of early-stage 1A high-grade serous ovarian cancer. The BRCA1-positive patient was identified through ultrasound screening, confirmed by MRI. The right ovary contains as cyst with an enhancing nodule (red arrows) demonstrated on the T1 fat-saturated image before ( a ) and after ( b ) contrast administration. The nodule enhances brightly, similar to the adjacent uterine myometrium (white arrow). Detection of ovarian cancer at this early stage is associated with a significantly improved 5-year survival rate compared with later stages of disease (courtesy: A. Rockall)
Example 2: Development of arterial spin labelling as a brain imaging biomarker
Arterial spin labelling (ASL) to measure perfusion has been at the forefront of physiological imaging without the use of contrast agents for more than three decades now. In spite of its obvious advantages in numerous diseases, such as brain tumours (Fig. 2 ), dementia or stroke, ASL has remained underused. In particular, it has been shown [ 9 ] that relative tumour blood flow values can be used as an independent biomarker of glioma grading, while the same biomarker can accurately distinguish pseudo-progression from true progression, thereby providing a very important tool for therapy monitoring [ 9 ]. In 2014, a landmark paper established a roadmap for all manufacturers and users of this technology. Based on this, an on-going effort is taking place to establish a jointly developed EIBALL-QIBA profile detailing guidelines on how to use ASL in clinical practice. As part of this initiative, a commercial ASL calibration phantom was recently launched, together with an online calibration tool. For ASL to finally become accepted, it will be critical for new studies to show the repeatability and reproducibility of the assessment of perfusion over time and its increased usefulness in many clinical neurological affections.

a – c Example of a 24-year-old female patient, with oligodendroglioma (grade II) in the left frontal lobe. a Cerebral blood flow, imaged by ASL. Red indicates high blood flow. b T1-Gd and c FLAIR. d – f Example of a 63-year-old female patient with GBM (grade IV) in the right temporal lobe. d Cerebral blood flow, imaged by ASL. Red indicates high blood flow. e T1 post Gd. f FLAIR. A clear difference in tumour blood flow can be seen between both patients (courtesy: A. Alsaedi, S. Bisdas)
Challenge 2: Developing new disease-specific, targeted and image-guided therapies
Biomedical imaging is an indispensable tool in personalised medicine providing information on localisation, extent, homogeneity and aggressiveness of disease.
Diagnostic imaging procedures are increasingly used to support individualised and targeted treatment and represent a mainstay of the progress of personalised medicine [ 10 ]. This may include:
- The use of an imaging technology to monitor a therapy [ 10 ]
- The administration of an imaging agent acting as a drug surrogate to predict the biodistribution of the drug [ 10 ]
- The co-administration of both a drug and an imaging agent to assess in real time the delivery/release of the therapeutic agent at the pathological site [ 10 ]
- The use of an imaging agent to guide surgeons or interventional radiologists in a (minimally) invasive procedure [ 10 ].
Recent advances in molecular biology proved that drug response is often a result of different genetic alterations, but can also arise from micro-environmental and microbiome exposure. Altered environmental and genetic factors can facilitate cancer progression and may also influence drug effectiveness, if gene mutations are involved in drug metabolism. A better understanding of the disease biology as well as molecular changes and altered signalling pathways will enable the identification of patients who may benefit from such treatments. It will catalyse the development of targeted therapies which counter the influence of specific molecular drivers contributing to the development and spread of disease, providing the foundation of precision medicine.
Theranostics is a novel emerging field of treatment in which diagnostic imaging tools coupled with therapeutic agents allow precise targeting of a disease at a molecular level. Theranostics enables tailored interventions, further personalising healthcare practices to individual patients for whom a standard therapy is not suitable. However, more work is needed to identify novel theranostic combinations. New diagnostic tracers can be tailored to the specific needs of stratified patient groups or to rare diseases for which other therapeutic approaches are not yet available.
For most currently available radionuclide therapies, a fixed amount of radioactivity is administered, regardless of patient weight or body surface area. Although these empirical dosing methods are well-established, safe and effective, the theranostic approach offers the possibility for an upfront evaluation of the biodistribution, quantification and calculation of the absorbed dose to the target volumes and critical organs. The development of a personalised dosimetry-based approach might further improve the outcome and cost benefit of radionuclide therapies. Although dosimetry is essential for the development of radiopharmaceuticals, its potential and clinical use to tailor the administered amount of radioactivity for each individual patient still needs to be established and evaluated by randomised controlled trials comparing dosimetry-based versus fixed-activity approaches.
Examples addressing Challenge 2
Example 3: theranostics for treatment of metastatic pancreatic cancer.
Figure 3 shows an example of theranostics in a patient with a metastatic pancreatic neuroendocrine tumour. In the first panel, staging with 68 Ga-Dotatate-PET shows a high expression of somatostatin receptors in the pancreatic tumour, locoregional lymph nodes and liver metastases. Panel b shows evaluation with SPECT after treatment with 177 Lu-Dotatate, confirming adequate targeting of the tumour sites. The end-of-treatment 68 Ga-Dotatate-PET scan (panel c) shows a partial remission, particularly of the metastases in the liver. A recent phase III study shows that treatment with 177 Lu-Dotatate results in a significantly higher response rate and longer progression-free survival than high-dose octreotide among patients with advanced midgut neuroendocrine tumours. A first interim analysis shows preliminary evidence of an overall survival benefit as well [ 11 ]. Similar theranostic approaches, such as 68 Ga-/ 177 Lu-PSMA in prostate cancer, are currently under evaluation in clinical trials with promising preliminary results.

Theranostics in a patient with a metastatic pancreatic neuroendocrine tumour. a 68 Ga-Dotatate-PET scan for staging. b SPECT evaluation after treatment. c End-of-treatment 68 Ga-Dotatate-PET scan (courtesy of J. Kunikowska, Warsaw)
Example 4: Cell recruitment to detect and target cancer for therapy
Recent studies have shown that it is possible to use fibroblast recruitment as a tool for detection of cancer and targeted therapy [ 12 ]. Cells like fibroblasts labelled with a fluorescent reporter can be used as beacons for guidance of biopsy or surgery, as well as for therapy (Fig. 4 ). This figure illustrates study results that show fibroblasts migrate towards cancer cells. Additionally, a therapeutic effect could be seen in this study. Delivery of the labelled cells stopped the production of ascitic fluid in the abdomen in an orthotropic model of ovarian cancer metastasis and prolonged survival in mice.

Fluorescence imaging of a mouse showing fibroblast (red) migration from injection site to location of tumour cells (green). ( a ) Fibroblast recruitment to tumour injection site next to intensine. ( b ) Fibroblast recruitment to tumour patches attached to the peritoneal wall. ( c ) Fibroblast scattered in the abdomen of a control mouse without tumour injection. ( d ) High resolution image of fibroblasts recruited to a tumour. ( e ) experimental design (green) [ 12 ]
Challenge 3: Contributing to a healthy start by delivering improved information on foetal health and prenatal growth and preventing development of anomalies using advanced imaging technologies
Biomedical imaging plays an important role in assessing foetal and prenatal health.
The placenta is one of the most important organs influencing not just the health of a woman and her foetus during pregnancy, but also the lifelong health of both. When it malfunctions, serious problems such as gestational diabetes, preterm labour and stillbirth can occur. Placental dysfunction may also lead to health problems later in life for both mother and child. The early diagnosis of foetal malformations and growth anomalies has the potential to improve foetal prognosis by granting a treatment plan and by making specialist units and treatments from birth accessible. The diagnostic accuracy of ultrasound (US), the currently most-used imaging modality, is however limited.
Novel imaging technologies should be used in clinical settings. The use of advanced US tools (e.g. elastography), optoacoustic imaging and quantitative MRI can be essential for the assessment of placenta function, transport of nutrients, oxygen, and therapeutics across the placenta while also detecting aberrations in foetal development from implantation to birth. Studies should focus on maternal and foetal risk models including genetic, infectious and environmental influences for disease prevention.
Enhanced prenatal monitoring has the potential for early detection and intervention to reduce maternal and foetal morbidity and mortality. Furthermore, among the diseases and anomalies encountered in high-risk patients during pregnancy, e.g. preeclampsia, intrauterine growth restriction, multi-foetal pregnancies, increased maternal age and infection (e.g. ZIKA virus) can be diagnosed and monitored by imaging. In general it can be expected that further advances in imaging hold a striking promise for the study of the placenta and the foetus and emerging technologies can be translated to other organs and open new research avenues for the benefit of human health.
Examples addressing Challenge 3
Example 5: foetal and perinatal imaging (us and mri).
Imaging techniques for prenatal imaging should be developed to identify in utero foetal growth disturbance, including foetal growth restriction and movement disorders. Normal foetal movement in the womb is critical to normal musculoskeletal development, and novel imaging strategies can play a critical part in diagnosis and monitoring of these diseases. For example, a spectrum of techniques including US elastography, micro CT, quantitative CT and MRI can be used to develop models of normal foetal movement (Fig. 5 ), against which diseases such as developmental hip dysplasia and foetal akinesia can be assessed. Early diagnosis and recognition will help reduce the huge societal and financial impact these diseases have on chronic musculoskeletal conditions in childhood, in combination with neonatal screening programmes for, e.g. hip dysplasia. The understanding and modelling of relationships between concurrent developments such as movement, skeletal system and the brain are key to the exploitation of perinatal imaging.

Computational pipeline for developing stress modelling of foetal movements. Foetal joint movements are tracked in utero ( a ), with finite element modelling of reaction forces ( b ) combined with musculoskeletal modelling to predict muscle forces ( c ) which are then applied to finite element models of foetal geometries ( d ). Adapted from [ 21 ]
Example 6: BOLD imaging of the placenta
While structural imaging of the foetus and the placenta are technically established, though not widely available, the assessment of placental function during pregnancy is currently a field of research. The gas and nutrient exchange between the mother’s and the foetus’ blood circulation via the placenta is essential for the development of the foetus. Early delivery is recommended for placental dysfunction but has to be weighed against the risks of preterm birth. Oxygen exchange is a most relevant function of the placenta and, due to the high blood volume methods adopted from functional brain imaging with MRI, has been proposed to evaluate placental function (Fig. 6 ). During an oxygen challenge, the change in blood oxygen level-dependent (BOLD) signal is measured. In particular, the time constant of the related oxygen change on the foetal blood circuit side of the placenta has been shown in early studies to be related to birth weight or foetal brain and liver volumes. Biomarkers derived from US measurements of the placenta and foetus have not correlated similarly well to postnatal pathologic assessment of the placenta as BOLD MRI variations. The quantitative determination of placental oxygenation remains a challenge since the BOLD signal depends on many parameters that are difficult to assess non-invasively.

BOLD imaging analysis of pregnant mice. Representative spatial distribution maps of the oxygen-haemoglobin dissociation inside the placenta and foetal liver on days 14.5 ( a ) and 17.5 ( b ) show distribution and variability [ 22 ]
Challenge 4: Providing accurate assessment of lifestyle and environmental impact factors on health supported by medical imaging
The impact of lifestyle and environmental factors can be assessed by medical imaging.
According to the WHO [ 13 ], 60% of the factors contributing to individual health and quality of life are correlated with lifestyle. Millions of people follow an unhealthy lifestyle and encounter illness, disability and even death because of it. Problems such as metabolic diseases, joint and skeletal pathologies, cardiovascular diseases, hypertension and violence can be caused by an unhealthy lifestyle. The relationship between lifestyle and health should be considered as established. Also the interaction between human health and the environment has been extensively studied and environmental risks have been proven to significantly impact human health, either directly by exposing people to harmful agents, or indirectly by disrupting life-sustaining ecosystems. Although the exact contribution of environmental factors to the development of disease and death cannot be precisely determined, the WHO has estimated that 13 million deaths annually are attributable to preventable environmental causes [ 14 ].
The timely use of diagnostic imaging tools as a preventive measure, followed by lifestyle changes, can prevent disease development [ 15 ]. Due to the increasing prevalence of obesity and its metabolic manifestations, it is important to obtain patient-specific quantitative body composition knowledge to predict and prevent disease [ 16 ]. The role of metabolically active tissues such as brown adipose tissue needs to be studied with new imaging methods to measure both mass and metabolic activity, enabling accurate assessments of energy turnover under normal and stimulated conditions. The identification of management strategies in cardiovascular diseases (CVDs) is of utmost importance, as the WHO projected that in 10 years from now more than 23 million people will die annually due to CVDs.
The use of biomedical imaging is essential due to its strategic advantages in diagnostic and therapeutic decision-making and as it delivers inputs for different stages of disease management, including prediction, screening, early diagnosis, staging, prognosis and follow-up. Deep phenotyping using imaging in combination with information acquired from wearable sensors has the significant potential to monitor subjects and patients during daily activities, providing information on external factors influencing biological processes at different levels.
Examples addressing Challenge 4
Example 7: body fat imaging with quantification.
The increase in obesity and metabolic-related diseases is one the challenges for global healthcare systems. Fat accumulation in organs and muscles is a strong biomarker of diabetes, the metabolic syndrome and obesity. A comprehensive understanding of the volume, distribution and quality of fat and muscles in the human body is important for diagnosis and treatment of illness. There is a need to develop automated segmentation and quantification of muscle and fat tissue volumes in the body (Fig. 7 ) which can be addressed by MRI. This technique has the potential to foster links between endocrine and cancer MRI disciplines and to develop biomarkers of morbidity in cancer survivors and links between disease entities such as body fat, diabetes, cancer and sarcopenia. In addition, the amount of subcutaneous and visceral adipose tissue in the abdomen plays an essential role in the determination of health risks.

Men matched for the same BMI and total body fat: Differing ‘adiposity phenotypes’ regarding visceral obesity and subcutaneous obesity and with different risk profiles (courtesy: A. Persson)
Example 8: MR phenotyping in diabetes and cardiovascular diseases
Imaging plays a highly promising role in individualised risk assessment, particularly regarding population-relevant diseases such as diabetes mellitus and hypertension. In subjects with established diabetes or hypertension, there is strong evidence that the level of detectable subclinical disease burden, such as an altered myocardial perfusion and/or delayed enhancement as assessed by MRI, is highly variable among patients and has strong prognostic relevance beyond left and right ventricular function for the occurrence of cardiovascular events. Thus, a more personalised risk stratification is clearly attainable. Less is known in subjects with prediabetes or pre-hypertensive stages of the disease, although there is early evidence that prediabetes is associated with increased subclinical disease burden, such as carotid plaque volume and arterial stiffness by ultrasound, changes of left ventricular function by echocardiography, or findings on native or contrast-enhanced cardiac MRI (Fig. 8 ). However, these observations lack a prospective design or reference group, comprise significant selection bias or are not generalisable to a preventive setting [ 17 ]. In order to confirm these early findings and establish their role in improving clinical care, further cross-sectional and longitudinal research in specific cohorts of patients is strongly warranted [ 17 ]. This may include prospective or nested cohort studies and randomised diagnostic trials [ 17 ]. As such, medical imaging may also add to the understanding of the relationship between changes in the phenotype and the individual predisposition for metabolic and cardiovascular diseases or the interplay with neurovascular diseases from a pathophysiology point of view. Accurate MRI evaluation of fat deposits within the liver and pancreas are also critical hallmarks of the metabolic syndrome.

Imaging findings in a 61-year-old male indicating extensive subclinical disease burden. a Two-point DIXON T1-weighted sequence for the assessment of visceral adipose tissue volume from the femoral head to the cardiac apex (arrow) indicating high levels of fat as well as hepatic proton density fat fraction (asterisk, measured on multi-echo VIBE T1-weighted sequences). b Fluid-attenuated inversion recovery sequences demonstrating mild white matter lesions (arrowhead). c Atherosclerotic carotid plaque was determined on black-blood T1-weighted fat-suppressed sequences in the common carotid artery (arrow), the carotid bulb and the proximal internal carotid artery. d Cine-SSFP sequences were evaluated for the calculation of volume and mass left ventricle (LV). e late gadolinium enhancement was detected on fast-low-single-shot inversion recovery sequence four-chamber view [ 23 ]
Challenge 5: Making Europe the world leader in machine learning and artificial intelligence in medical imaging by exploiting existing data and expertise to implement digital solutions after rigorous clinical validation
Machine learning and artificial intelligence can substantially improve disease diagnostics and prediction.
Medical image data has been accumulating exponentially since the introduction of digital radiology in the 1990s. For this reason, being already completely computerised, radiology is bound to be the first of all medical specialties embracing the use of machine-learning and artificial intelligence. By 2020, global medical data is expected to double every 73 days, and on average, each person generates one petabyte of health-related data across his or her lifetime [ 18 ]. Medical images are a substantial portion of all medical data, estimated to amount to 30% of all data storage [ 19 ]. The amount of data being collected exceeds our capacity to interpret them, especially when integrating multiple modalities from vastly different fields such as radiology, metabolomics and genetics.
Available medical imaging data can be a highly valuable resource for research on diagnostics, epidemiology and drug development. This has led to a higher interest in the development of data-driven models based on machine learning (ML) and artificial intelligence (AI) [ 18 ]. Deep learning, based on artificial neural networks, emerged in recent years as a powerful tool for ML and promises to reshape the future of AI. Increasing computational power allows ML, and AI in general, to more accurately identify and generate semantic interpretations from medical imaging data, supporting diagnoses.
Interpreting images can be highly subjective. ML and AI can be used to interpret images more consistently, as well as document metadata and perform data entry. ML techniques not only have significant potential to improve diagnoses from medical images, but may also improve disease prediction, decision-making, treatment planning and treatment response predictions [ 20 ].
Currently, ML and AI in medical imaging are not being used to their full extent. Beyond supporting diagnoses, ML and AI can reduce cost by cutting scan times, automating post-processing and reducing computation times for model-based image interpretation. Furthermore, ML and AI can accelerate drug discovery and leverage data from multiple sources (including sources other than medical images) for better care coordination.
Examples addressing Challenge 5
Example 9: identifying novel phenotypes in lung diseases.
A key ability of ML is the exploitation of complex features drawn from multiple modalities and across the entire patient. Current finding and diagnostic categories in lung diseases such as fibrosis are lacking in terms of repeatability and their ability to predict outcome and treatment response. Machine learning can contribute by identifying those patterns that can be extracted with high stability and reliability from clinical imaging data (Fig. 9 ). It can connect these measurements with patient information such as smoking history, or age. Finally, it can mine the resulting descriptions for reliable predictors linking current patient status with outcome and risk that is relevant for individual treatment decisions to identify predictive markers. Initial results point to the power of machine learning in this direction, and we expect dramatic changes in both the characteristics we use for prognosis as well as the way we use them to treat an individual through the emerging integration of machine learning in the development of diagnostic tools, and the day-to-day clinical decision support.

The landscape of lung disease patients based on their CT image data. The distribution illustrates clusters of patients with similar imaging characteristics confirmed in reported findings. It is a step towards the identification of phenotypes in large-scale medical imaging data. [ 24 ]
Example 10: Automation of detection and measurements
A wealth of known markers are tedious and time-consuming to extract from clinical imaging data. The widening gap between the rapidly increasing amounts of imaging data acquired during clinical routine, and the available number of radiologists who can read images, requires an increase of efficiency, and the delegation of tasks to machine learning-based algorithms. While the expert focuses on integrating individual measurements into a comprehensive assessment of the patient, the machine can reduce the time needed to screen hundreds of image slices. Examples comprising vast amounts of imaging data in need of analysis to improve disease diagnosis and prediction include the detection of nodules in lung imaging, polyps in CT-colonography, measurement of brain structures in neurological disease and evaluation of tumour characteristics for diagnosis and therapy outcome prediction (Fig. 10 ).

Radiomics pipeline, with the aim to link imaging features to clinically relevant parameters such as tumour subtype, patient prognosis and therapy outcome prediction (Courtesy Martijn Starmans and Stefan Klein, Rotterdam)
Overcoming challenges in healthcare through biomedical imaging research
The EIBIR Strategic Research Agenda for Biomedical Imaging identifies research areas critical to overcoming current challenges in healthcare:
- Innovative biomedical imaging plays a key role in field of personalised medicine as it addresses the current needs for individualised prevention, treatment, therapy response monitoring and image-guided surgery. In the area of disease prevention and therapy monitoring, the use of non-invasive biomarkers will lead to improved patient outcome, therapy prediction and monitoring.
- Innovative diagnostic imaging technologies and procedures provide information about disease characteristics and, coupled with biological, genetic and -omics data, will contribute to an individualised diagnosis and consecutive targeted therapy approach. In the emerging field of theranostics, imaging tools together with therapeutic agents allow for tailored therapeutic interventions and the selection of most effective treatments.
- In the area of prenatal monitoring, the use of advanced imaging technologies with higher diagnostic accuracy will ensure the early detection of malfunctions or disease and benefit maternal and foetal health providing a healthy start in life.
- The use of biomedical imaging for diagnosis and management of lifestyle-induced diseases followed by lifestyle changes will help to prevent disease development.
- The advanced application of Artificial Intelligence and Machine Learning in imaging data will help to improve image interpretation and lead to better disease prediction, decision making and treatment planning.
In summary, biomedical imaging has the proven potential to address the major challenges in healthcare and to contribute to the wellbeing of European citizens and patients.
To realise this potential, investments in biomedical imaging research are needed to enable the translation of innovative solutions into clinical practice. Moreover, the appropriate funding will ensure that the European research community stays in the forefront of medical science.
Decision makers are invited to consider this document in the definition of research topics strategically recommendable for financing in the next decades.
Supporting societies
This Strategic Research Agenda for Biomedical Imaging was developed by EIBIR with the support and input of its shareholder societies:
European Society of Radiology (ESR)
The ESR is a non-profit organisation representing the general interests of radiology in Europe ( www.myesr.org ). The aims of ESR are to serve the healthcare needs of the general public through the support of science, teaching and research and the quality of service in the field of radiology as well as the promotion and coordination of the scientific, philanthropic, intellectual and professional activities of radiology in all European countries. The ESR has over 80,800 individual members as well as 109 member societies of which 47 are European national radiology societies, 15 are European radiological subspecialty societies and European allied sciences and 47 non-European national radiology societies.
Cardiovascular and Interventional Radiological Society of Europe (CIRSE)
CIRSE is a non-profit, educational and scientific association aiming to improve patient care through the support of teaching, science, research and clinical practice in the field of cardiovascular and interventional radiology (IR) ( www.cirse.org ). CIRSE aims to support education and further research in IR, as well as ensuring excellent patient safety and timely access to IR therapies. CIRSE also actively collaborates with and supports other scientific, educational, governmental, professional, national and international organisations considered useful to the aims of the Society.
European Coordination Committee of the Radiological, Electromedical and Healthcare IT Industry (COCIR)
COCIR is the European Trade Association representing the medical imaging, radiotherapy, health ICT and electromedical industries ( www.cocir.org ). COCIR is a non-profit association and provides a wide range of services on regulatory, technical, market intelligence, environmental, standardisation, international and legal affairs. COCIR promotes harmonisation of regulatory frameworks, supported by state-of-the-art international standards. Its industry provides safe and high-quality products and services, which contribute to reducing health inequalities and enhance cost efficiency in healthcare systems.
European Association of Nuclear Medicine (EANM)
EANM is the umbrella organisation representing nuclear medicine in Europe and represents 40 National Member Societies, approximately 3200 individual members and around 30,000 professionals working in nuclear medicine in Europe ( www.eanm.org ). EANM aims to advance science and education in nuclear medicine for the benefit of public health, relating to the diagnosis, treatment, research and prevention of diseases through the use of unsealed radioactive substances and the properties of stable nuclides in medicine, throughout Europe.
European Federation of Organisations for Medical Physics (EFOMP)
The EFOMP serves as an umbrella organisation representing 34 national member and affiliated organisations of more than 8100 physicists and engineers working in the field of medical physics in Europe ( www.efomp.org ). EFOMP aims to harmonise and advance medical physics in both its professional clinical and scientific expression throughout Europe by bringing about and maintaining systematic exchange of professional and scientific information, through the formulation of common policies, and by promoting education and training programmes.
European Federation of Radiographer Societies (EFRS)
The EFRS is a non-profit umbrella organisation representing 39 professional societies and 60 educational institutions representing over 100,000 radiographers across Europe ( www.efrs.eu ). The aims of the EFRS are to represent, promote and develop the profession of radiography in Europe, across medical imaging, nuclear medicine and radiotherapy areas of radiography practice.
European Organisation for Research and Treatment of Cancer (EORTC)
EORTC is an independent, non-profit cancer research organisation, with the mission to coordinate and conduct international translational and clinical research to improve the standard of cancer treatment for patients ( www.eortc.org ). EORTC aims ultimately to increase people’s survival and quality of life by testing new therapeutic strategies based on existing drugs, surgery and radiotherapy and also helps develop new drugs and approaches in partnership with the pharmaceutical industry and in patients’ best interests.
European Society for Magnetic Resonance in Medicine and Biology (ESMRMB)
ESMRMB is a non-profit society, which aims to support educational activities and research in the widest sense in the field defined by the society’s name ( www.esmrmb.org ). The ESMRMB is open to physicians, engineers, scientists and other individuals who are interested in the developments or the introduction of magnetic resonance techniques in the fields of medicine and biology.
European Society of Paediatric Radiology (ESPR)
ESPR aims to organise and bring together physicians involved in the field of paediatric imaging, to contribute to the progress of paediatric imaging particularly within but also outside Europe, to encourage training and education with other branches of medical imaging and paediatrics in clinical, scientific, education and research fields ( www.espr.org ).
European Society for Radiotherapy and Oncology (ESTRO)
ESTRO is a non-profit scientific organisation representing radiation oncologists, medical physicists, radiobiologists and radiation therapists with over 5000 members both within and outside Europe ( www.estro.org ). ESTRO aims to foster the role of radiation oncology in order to improve patient care in the multimodality treatment of cancer by promoting innovation, research and dissemination of science through its congresses, special meetings, educational courses and publications.
European Society of Medical Imaging Informatics (EuSOMII)
EuSoMII is a professional healthcare organisation that provides its members and the radiological community with up-to-date information on the latest innovations and achievements in medical imaging informatics by supporting education, research and events related to the top-tier software in radiology ( www.eusomii.pro ).
Acknowledgements
The European Institute for Biomedical Imaging Research and Pamela Zolda would like to thank the following people for their in-depth review of the SRA and suggestions: Silvio Aime, Angel Alberich, Anja Almen, Owen Arthurs, Henryk Barthel, Olivier Clément, Michael Crean, Nandita de Souza, Florian Demuth, Marc Dewey, Vincent Dousset, Alejandro Frangi, Casper Garos, Xavier Golay, Peter Gordebeke, Matthias Günther, Horst Hahn, Monika Hierath, Christoph Hoeschen, Myriam Hunink, Hans-Ulrich Kauczor, Gabriel Krestin, Katharina Krischak, Georg Langs, Yan Liu, Luis Marti-Bonmati, Celso Matos, Ulrike Mayerhofer-Sebera, Jonathan McNulty, Kristoff Muylle, Michal Neeman, Wiro Niessen, Konstantin Nikolaou, Philippe Pereira, Anders Persson, Antonio Pifferi, Katrine Riklund, Andrea Rockall, Karen Rosendahl, Francesco Sardanelli, Steven Sourbron, Oliver Speck and Vincenzo Valentini.
Author’s contributions
The author read and approved the final manuscript.
Competing interests
The author declares no competing interests.
Publisher’s Note
Springer Nature remains neutral with regard to jurisdictional claims in published maps and institutional affiliations.
Contributor Information
Silvio aime, angel alberich, owen arthurs, henryk barthel, olivier clément, michael crean, nandita de souza, florian demuth, vincent dousset, alejandro frangi, casper garos, xavier golay, peter gordebeke, matthias günther, monika hierath, christoph hoeschen, myriam hunink, hans-ulrich kauczor, gabriel krestin, katharina krischak, georg langs, luis marti-bonmati, celso matos, ulrike mayerhofer-sebera, jonathan mcnulty, kristoff muylle, michal neeman, wiro niessen, konstantin nikolaou, philippe pereira, anders persson, antonio pifferi, katrine riklund, andrea rockall, karen rosendahl, francesco sardanelli, steven sourbron, oliver speck, vincenzo valentini, pamela zolda.
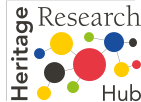
- Heritage Research Community
- Strategies and Policies
- EC Projects
- Reserved Area
- Strategic Research and Innovation Agenda
- Heritage Projects
- Training & Vacancies
- About the Hub
- Science4Heritage
- It-Fr Cooperation
Strategic Research and Innovation Agenda 2020 (SRIA)
Final version of the updated jpi ch sria published.
Home News Strategic Research and Innovation Agenda 2020 : Final version of the updated JPI CH SRIA published
The Strategic Research and Innovation Agenda 2020 builds on the SRA 2014 and reflects the political, economic, socio-cultural and technological changes that have occurred in recent years. It capitalises on the increasing policy developments both within Europe and beyond, which demonstrate the intrinsic and instrumental values of heritage.
Overarching research principles have been agreed which will underpin the future joint activities of the JPI CH. They reaffirm that the initiative promotes a holistic approach to cultural heritage and encourages collaborative, transdisciplinary, interdisciplinary, innovative, basic and applied research, to meet societal challenges and contribute to sustainable development. Public participation and engagement should be at the heart of the joint activities, and education and training opportunities should be developed. SRIA 2020 aims to ensure that the JPI CH supports digital innovation within the heritage sector and contributes to the UN Sustainable Development Goals.
- A reflective heritage for a resilient society – this Priority Area focuses on cultural heritage as an empowering force and a collective good for society.
- Sustainable management of cultural heritage – this Priority Area focuses on participatory governance, the added value of cultural heritage and emerging technologies.
- Cultural heritage in a changing context – this Priority Area focuses on urban and rural development, globalisation, demographic change and tourism. It will explore both the positive and negative implications for cultural heritage.
- Cultural heritage facing climate and environmental change – this Priority Area focuses on the impact of climate and environmental changes on cultural and natural heritage and how cultural and natural heritage could be a resource for Sustainable Development.
Illustration – Banner: The Pagaie des Mapipis, Martinique, France, © Patrick Joseph-Auguste
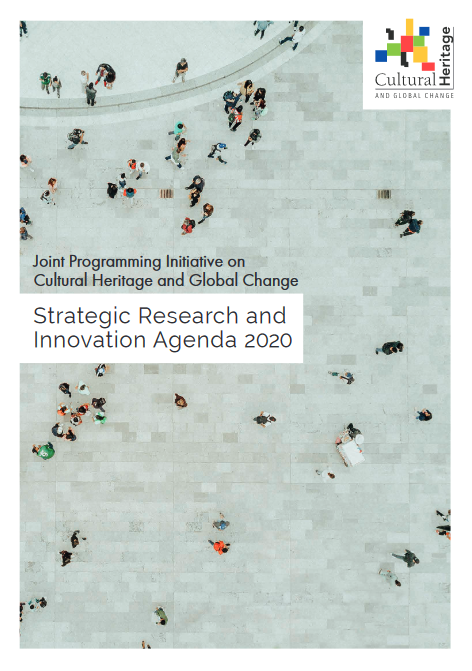
Newsletter subcription
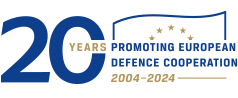
- Publications & Data /
- Brochures /
OSRA – Overarching Strategic Research Agenda and CapTech SRAs Harmonisation
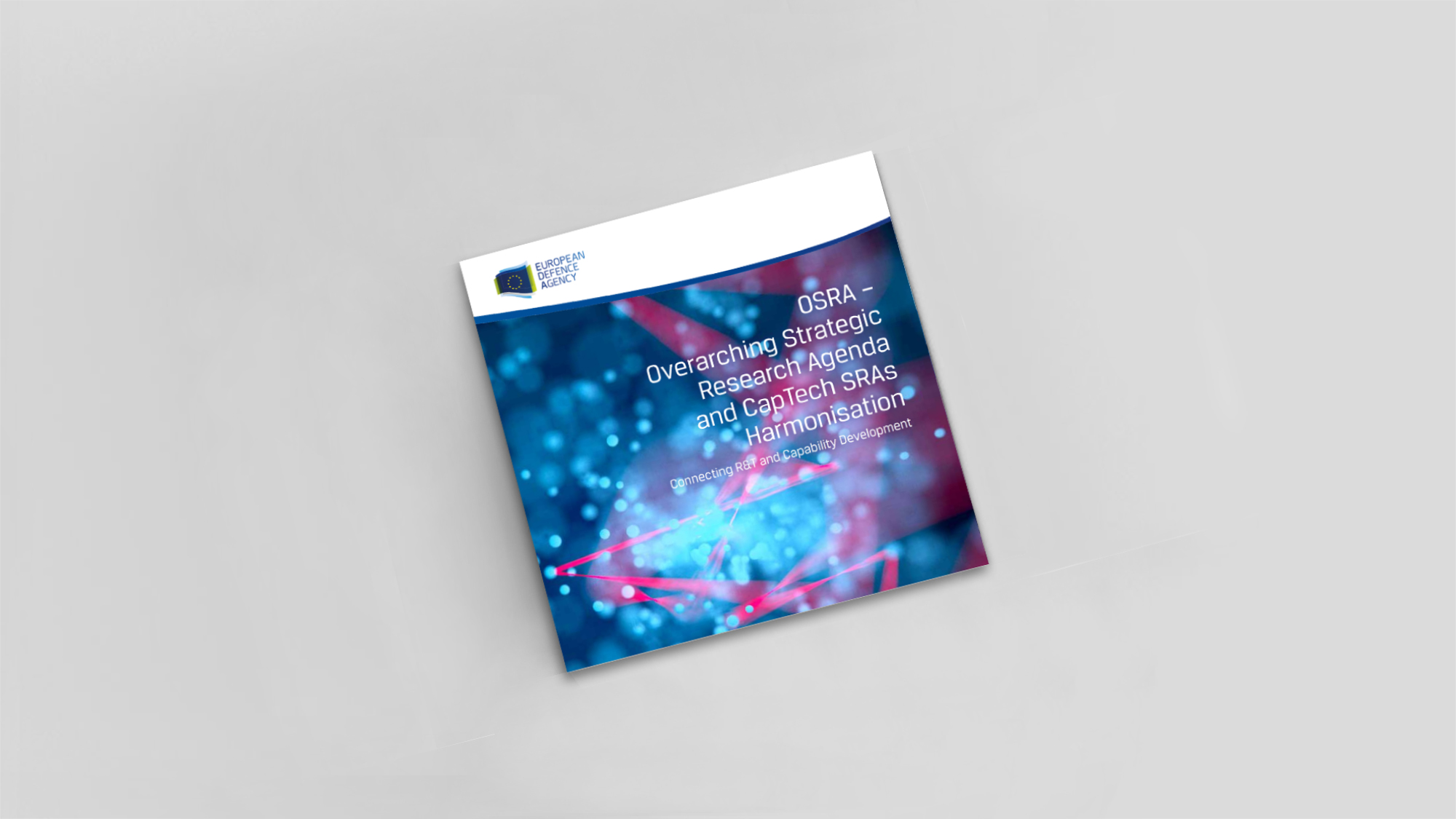
The OSRA is a new approach for aligning strategic research agendas with Member States’ operational needs and requirements. This will provide a systematic and clear mechanism for collaborative European Defence Research for the future, such as the upcoming EU framework programme and Preparatory Action. In addition to the bottomup process of identifying common areas of R&T interests, the so-called technology push process, a top-down capability-pull process is foreseen that links operational requirements directly to technologies in a systematic and traceable manner, and moreover leads towards the development of an European overarching strategic research agenda.
Research Strategy 2032
Queensland Health is driving innovation by setting the agenda for a dynamic research ecosystem that translates ground breaking research into effective and efficient healthcare delivery.
Propelled by the HEALTHQ32 vision, the Research Strategy 2032 will build upon Queensland Health’s research strengths to address complex challenges and empower the health workforce for the next decade.
Research and innovation are at the heart of a world-class health system and drive the generation of new insights, discoveries and evidence that underpins and delivers better health outcomes, improved health system sustainability and economic growth.
The goals of the Research Strategy 2032 will be achieved by supporting the full research pipeline from ‘ideas into research excellence into implementation’ and building a cohesive and dynamic ecosystem. You can learn more about how Queensland Health is leading the way in research in the one-page summary and action plan.
Download the Research Strategy 2032
- Research Strategy 2032 (PDF 7402 kB)
- Research Strategy 2032 one-page summary (PDF 1304 kB)
Planning the horizons for the Research Strategy 2032
Queensland Health has developed the first action plan covering Horizon 1 (2023-25) as the first stage in the implementation of the Research Strategy 2032. This is the first of three action plans to be developed over the life of the Research Strategy.
Horizon 1 (2023-25) - Immediate Impact
Focus on actions that can be delivered quickly and achieve the greatest impact within available resources, while also setting up the foundations of long-term change and new initiatives.
Read the one-page summary and Horizon 1 action plan (PDF 1304 kB) .
Horizon 2 (2025-28) - Strengthen and Grow
Review and leverage achievements and lessons from Horizon 1, scaling up successful initiatives, expanding ambitions (in line with available resources) and continuing commitments to long-term actions.
Horizon 3 (2028-32) - Sustaining Change
Focus on actions that secure and embed progress toward a transformed research and innovation organisational ecosystem, and complete delivery of the most complex commitments from Horizons 1 and 2.
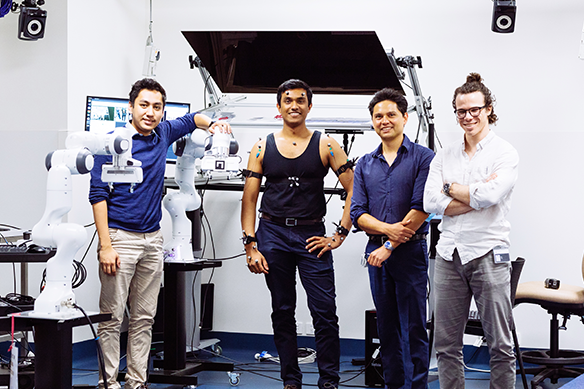
Focus areas and objectives
Focus area 1: foster innovative ideas.
- Research is promoted as a valuable part of the health system with research achievements acknowledged, celebrated and disseminated.
- Research and innovation are supported by mixed streams of funding from a range of external sources of investment.
- Pipelines of career opportunities are available to researchers in Queensland Health.
- Patients, consumers and the broader community influence research priorities and are empowered to participate in research.
Outcome: Queensland Health’s environment and culture promote innovative thinking and value research.
Focus Area 2: Turn ideas into research excellence
- Partnerships with academia, industry and others provide high quality research informed by health needs.
- Approval processes are quick and easy to navigate for researchers and patients and ensure studies are safe and ethical.
- Tailored education, training and advice upskill staff for designing and undertaking Research and Development in the health system.
- Health system infrastructure and technology absorb translational research seamlessly.
Outcome: Queensland Health’s processes and systems encourage and enable high quality research in the health system.
Focus Area 3: Translate research into better healthcare
- Research outcomes are routinely scaled-up and adopted across the health system to improve frontline healthcare delivery.
- Queensland is an international clinical trials hub giving patients access to cutting-edge treatment options close to home.
- The wealth of discoveries is captured to realise health and economic potential.
Outcome: Queensland Health collaborates with diverse stakeholders to drive research discoveries quickly and safely into practice.
For more information contact [email protected] .
Last updated: 9 April 2024

Site Search
- About ARPA-E
- Team Directory
- ARPA-E History
- Annual Reports
- Budget Requests
- Apply For Funding
- Authorization
- View Active Programs
- Search Our Programs
- Search Individual Projects
- Interactive Project Map
- Exploratory Topics
- The SCALEUP Program
- OPEN Programs
- Project Guidance
- ARPA-E Technology-to-Market
- Technology Commercialization
- External Engagement Model
- Investor Updates
- ARPA-E News & Media
- Press Releases
- ARPA-E Disruptors
- Publications
- ARPA-E Events
- Energy Innovation Summit
- Careers at ARPA-E
- Job Opportunities
- Life at ARPA-E
- ARPA-E FAQs
- General Questions
- Current Funding Opportunities
- Closed Funding Opportunities
Press Release
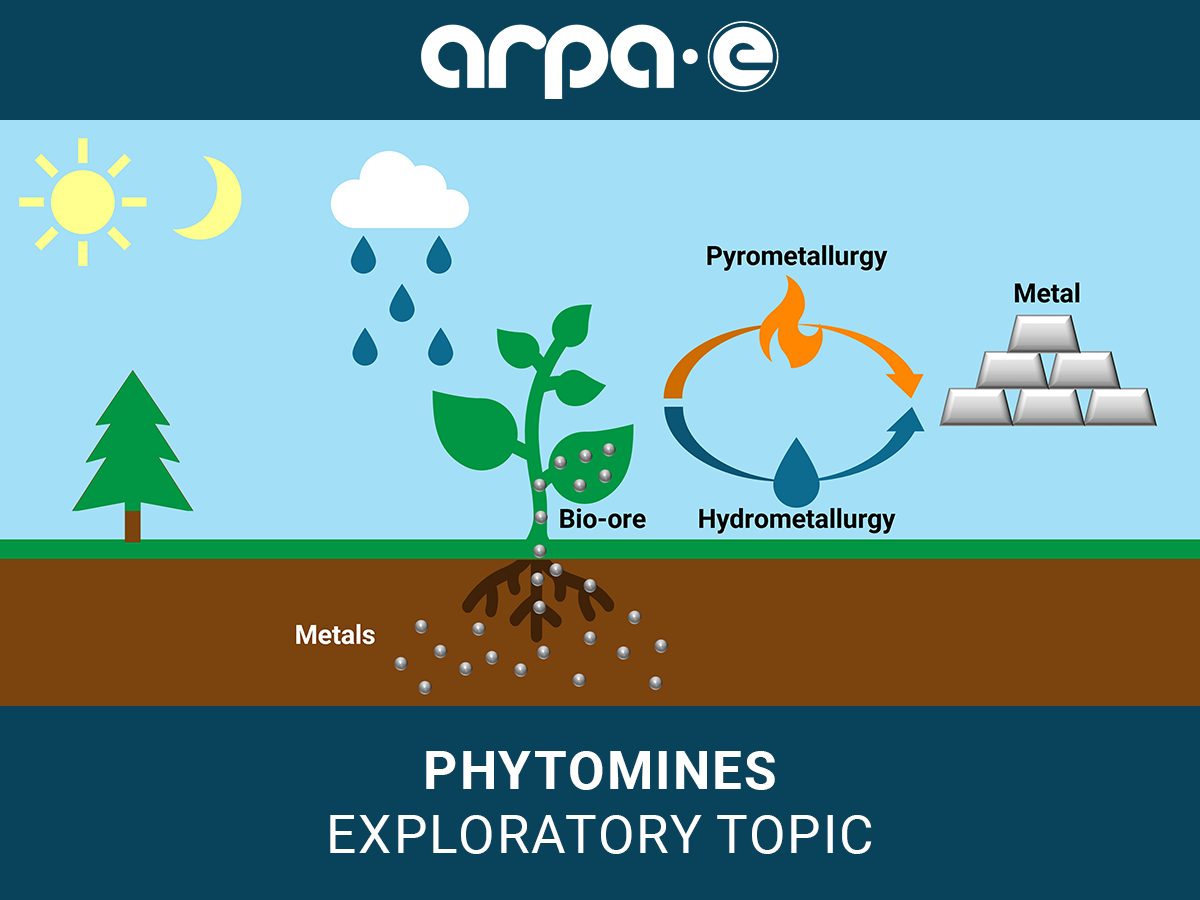
U.S. Department of Energy Announces $10 Million to Explore Using Plants to Extract Critical Materials from Soil to Support Domestic Supply Chain
WASHINGTON, D.C. — The U.S. Department of Energy (DOE) today announced up to $10 million in funding to explore nickel extraction from soil using plants—a process known as phytomining—to establish a competitive domestic supply chain, supplement conventional mining methods, and reduce nickel imports. Managed by the Advanced Research Projects Agency-Energy (ARPA-E), this effort supports President Biden’s Investing in America agenda to strengthen domestic critical materials supply chains, enhance our economic and national security, and meet the growing demand for critical materials needed to ensure America leads the world in the emerging clean energy economy.
“In order to accomplish the goals laid out by President Biden to meet our clean energy targets, and support our economy and national security, it’s going to take all-hands-on-deck approach and innovative solutions,” said ARPA-E Director Evelyn N. Wang. “By exploring phytomining to extract nickel as the first target critical material, ARPA-E aims to achieve a cost-competitive and low-carbon footprint extraction approach needed to support the energy transition.”
Among the critical materials named in the DOE Critical Materials Assessment (CMA), nickel serves as an ideal target to validate the viability of phytomining in the U.S. due to the large number of documented nickel hyperaccumulation (HA) plants. Nickel is used in the cathodes of lithium-ion batteries present in electric vehicles, consumer electronics, stationary storage, stainless steel, metallurgy, coatings, electroplating, and other alloys. Nickel is crucial to global clean energy technology supply chains and future demand is expected to grow.
The new ARPA-E Exploratory Topic announced today, Plant HYperaccumulators TO MIne Nickel-Enriched Soils (PHYTOMINES) , seeks to spur the technological development of phytomining in the United States that could complement current and future domestic sources of nickel and catalyze phytomining of critical minerals beyond nickel.
The targeted outcomes of PHYTOMINES are:
- Technologies could be interventions in the soil or plant microbiome or the development of plant traits that enable the accumulation of nickel at an enhanced rate. ARPA-E envisions these projects as early-stage proof-of-concepts likely to take place in closed or open-air laboratories, greenhouses, or confined fields where light, humidity, and temperature regimes can be fully programmed.
- Possible projects include mapping HA species of interest, mineral characteristics in soil, and land ownership data for natural habitats and adjacent areas viable for phytomining, scaling opportunities, and technoeconomic and lifecycle analyses of phytomining projects.
PHYTOMINES encourages partnerships between farmers, scientists, battery manufacturers, steel and mining industries, and more. You can access more information on ARPA-E Exchange .
ARPA-E advances high-potential, high-impact clean energy technologies across a wide range of technical areas that are strategic to America's energy security. Learn more about these efforts and ARPA-E's commitment to ensuring the United States continues to lead the world in developing and deploying advanced clean energy technologies.
Press and General Inquiries: 202-287-5440 [email protected]
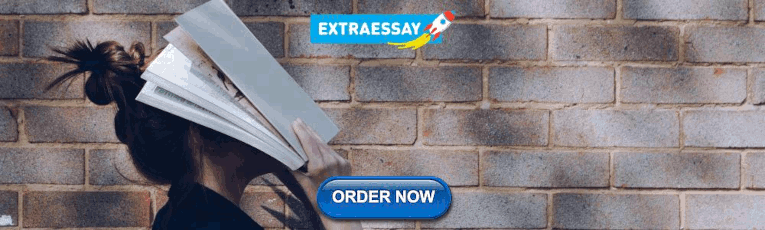
IMAGES
COMMENTS
This study analyzes the association between the strategic research agendas of researchers in the social sciences and their research performance. Based on a worldwide sample of 604 researchers, this study assesses whether researchers' strategic research agendas are predictors of both short-term (last 3 years) and long-term career publications and citations, after controlling for relevant ...
The roadmapping of the European HPC technology research is the main aim of our Association and the ETP4HPC Strategic Research Agenda (SRA) is our key deliverable. We have now issued five instances of our SRA, which have served as the basis for the HPC R&D Work Programmes to date. We make this SRA available to the EuroHPC JU and in particular ...
These findings show that strategic research agendas are associated with preferences for basic research, applied research, or experimental development, but only to some extent. We also extend the Multi-Dimensional Research Agendas Inventory - Revised, a widely used instrument for measuring strategic research agendas, by adding a new dimension ...
The Strategic Research Agenda, which was published in 2015, included major changes expected in technology and resources (so-called "Game Changers") and research objectives for the short- (1 to 5 years), medium- (5 to 10 years) and long-term (beyond 10 years). The short-term objectives have been largely achieved and we are now well advanced ...
This Strategic Research Agenda identifies current challenges and needs in healthcare, illustrates how biomedical imaging and derived data can help to address these, and aims to stimulate dedicated research funding efforts.Medicine is currently moving towards a more tailored, patient-centric approach by providing personalised solutions for the individual patient. Innovation in biomedical ...
he document presents the full updated Strategic Research and Industry Agenda (SRIA 2030) for Quantum Technologies (QT) in the European Union (EU). It follows a preliminary document published in November 2022, with the goal of aligning the Strategic Research Agenda (SRA, published in 2020), which articulates the European Research Community's
This review incorporates strategic planning research conducted over more than 30 years and ranges from the classical model of strategic planning to recent empirical work on intermediate outcomes, such as the reduction of managers' position bias and the coordination of subunit activity. Prior reviews have not had the benefit of more socialized ...
The strategic research agendas of the three clusters are distinguished from each other along four main dimensions: Divergence, Discovery, Mentor Influence, and Social Orientation. These findings show that strategic research agendas are associated with preferences for basic research, applied research, or experimental development, but only to ...
The Strategic Research Agenda (SRA) 2011 has been published, and the IGD-TP's Deployment Plan (DP) is in public consultation at the time of writing. The Vision Report provides information on the benefits of joining the IGD-TP and on how to participate in the work.
Strategic Research Agenda VISION 2050: "People and goods can reach their destinations in cities in a way that is healthy, safe, affordable, reliable and comfortable" • Walking, cycling, collective and shared mobility services forming the backbone of the urban mobility transport, complemented by private vehicles.
Its Strategic Research and Innovation Agenda will support international collaborations accelerating the clinical evaluation and implementation of interventions against poverty-related infectious diseases including the neglected ones affecting sub-Saharan Africa. By building research capacity, it will also enhance the ability of
The 2020 Strategic Research Agenda (SRA) is an update from the 2016 SRA and takes into account FACCE-JPI's past work and achievements and input from the members of FACCE-JPI as represented in the Governing Board. Member States' national priorities that need to be highlighted were specified and integrated in the text, along with the JPI's past and ongoing work with other European and ...
Strategic Education Principle 1: All students have access to high-quality instruction regardless of their gender, sexual orientation, ethnicity, race, economic status, native language, or physical, emotional, and cognitive abilities to close the student achievement and opportunity gaps that currently exist. Priority Research Topics:
Strategic Research and Innovation Agenda (SRIA). This report details how the . internal process of topic identification and prioritization progressed within .
Michael C. Withers is an associate professor of management, the Gina and Anthony Bahr ('91) Professor in Business, Presidential Impact Fellow, and Chancellor EDGES Fellow in Mays Business School, Texas A&M University. He received his Ph.D. in strategic management from the W. P. Carey School of Business at Arizona State University. His research interests include corporate governance, director ...
SRAs (Strategic Research Agenda) on different levels, e.g. with regard to a unified SRA development and maintenance protocol, a shared and agreed-upon main terminology, general structure-related and specific content-related aspects. In particular, main issues to be resolved include a) inclusiveness of SRAs (each CapTech SRA should work
This Strategic Research Agenda identifies current challenges and needs in healthcare, illustrates how biomedical imaging and derived data can help to address these, and aims to stimulate dedicated research funding efforts. Medicine is currently moving towards a more tailored, patient-centric approach by providing personalised solutions for the ...
The Strategic Research and Innovation Agenda 2020 builds on the SRA 2014 and reflects the political, economic, socio-cultural and technological changes that have occurred in recent years. It capitalises on the increasing policy developments both within Europe and beyond, which demonstrate the intrinsic and instrumental values of heritage. ...
Overview. This document describes a long-term global strategy for filovirus research and monitoring. This strategy is based on three principles described in this document, namely, anticipation, reinforcement and cure. The anticipation principle intends to foster research areas that may lead to improve our capacity to predict future outbreaks ...
1.1 Purpose of this strategic research and innovation agenda This document describes the Strategic Re-search and Innovation Agenda (SRIA) to support the delivery of the Digital European Sky. Togeth-er with the proposal for an "Integrated ATM" partnership [14], these documents present the scope and approach to further modernisation of
Download. The OSRA is a new approach for aligning strategic research agendas with Member States' operational needs and requirements. This will provide a systematic and clear mechanism for collaborative European Defence Research for the future, such as the upcoming EU framework programme and Preparatory Action. In addition to the bottomup ...
A major step in this prioritisation process was to develop a Strategic Research Agenda (SRA). An EC-funded Network of Excellence in Radioecology, called STAR (Strategy for Allied Radioecology 3 ), was formed to, among other tasks, develop the SRA. This manuscript is the first journal-published draft of the SRA.
Strategy. NIJ's approach to accomplishing its mission involves achieving four goals: Invest in Needed Research: NIJ supports basic and applied, intramural and extramural scientific research to develop needed knowledge and tools to advance policy and practice in eight broad research areas, primarily through a competitive grant program: Crime ...
The purpose of this study is to develop a proposal for a future research agenda on university rankings, based on a systematic review of the existing literature, ith emphasis on the following criteria: objectives, countries, types, variables, methodologies, and future lines of research. The analysis of university rankings revealed a need to review evaluation methodologies and reflect on their ...
Research Strategy 2032. Queensland Health is driving innovation by setting the agenda for a dynamic research ecosystem that translates ground breaking research into effective and efficient healthcare delivery. Propelled by the HEALTHQ32 vision, the Research Strategy 2032 will build upon Queensland Health's research strengths to address ...
WASHINGTON, D.C. — The U.S. Department of Energy (DOE) today announced up to $10 million in funding to explore nickel extraction from soil using plants—a process known as phytomining—to establish a competitive domestic supply chain, supplement conventional mining methods, and reduce nickel imports. Managed by the Advanced Research Projects Agency-Energy (ARPA-E), this effort supports ...