Have a language expert improve your writing
Run a free plagiarism check in 10 minutes, generate accurate citations for free.
- Knowledge Base
Methodology
- Cross-Sectional Study | Definition, Uses & Examples
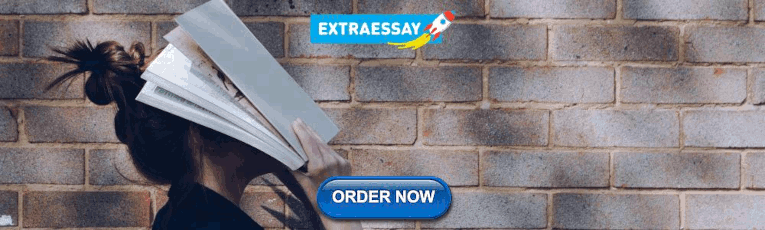
Cross-Sectional Study | Definition, Uses & Examples
Published on May 8, 2020 by Lauren Thomas . Revised on June 22, 2023.
A cross-sectional study is a type of research design in which you collect data from many different individuals at a single point in time. In cross-sectional research, you observe variables without influencing them.
Researchers in economics, psychology, medicine, epidemiology, and the other social sciences all make use of cross-sectional studies in their work. For example, epidemiologists who are interested in the current prevalence of a disease in a certain subset of the population might use a cross-sectional design to gather and analyze the relevant data.
Table of contents
Cross-sectional vs longitudinal studies, when to use a cross-sectional design, how to perform a cross-sectional study, advantages and disadvantages of cross-sectional studies, other interesting articles, frequently asked questions about cross-sectional studies.
The opposite of a cross-sectional study is a longitudinal study . While cross-sectional studies collect data from many subjects at a single point in time, longitudinal studies collect data repeatedly from the same subjects over time, often focusing on a smaller group of individuals that are connected by a common trait.
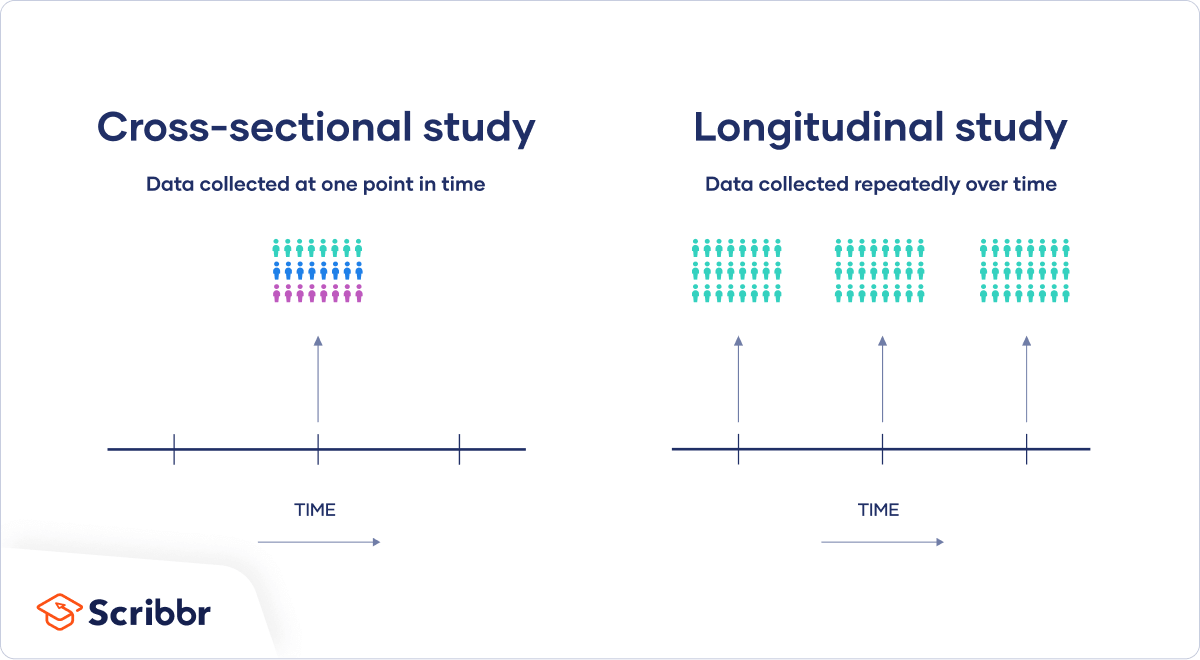
Both types are useful for answering different kinds of research questions . A cross-sectional study is a cheap and easy way to gather initial data and identify correlations that can then be investigated further in a longitudinal study.
Receive feedback on language, structure, and formatting
Professional editors proofread and edit your paper by focusing on:
- Academic style
- Vague sentences
- Style consistency
See an example
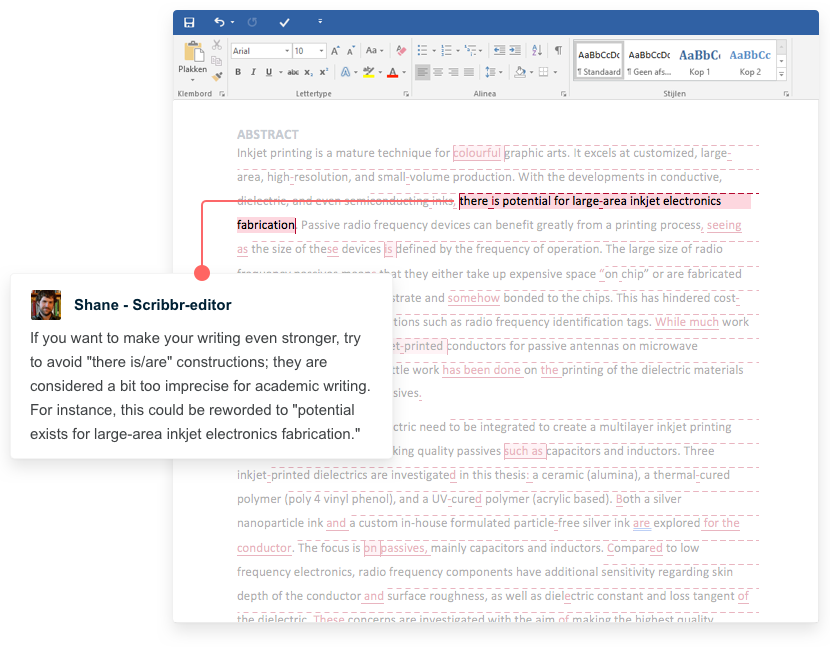
When you want to examine the prevalence of some outcome at a certain moment in time, a cross-sectional study is the best choice.
Sometimes a cross-sectional study is the best choice for practical reasons – for instance, if you only have the time or money to collect cross-sectional data, or if the only data you can find to answer your research question was gathered at a single point in time.
As cross-sectional studies are cheaper and less time-consuming than many other types of study, they allow you to easily collect data that can be used as a basis for further research.
Descriptive vs analytical studies
Cross-sectional studies can be used for both analytical and descriptive purposes:
- An analytical study tries to answer how or why a certain outcome might occur.
- A descriptive study only summarizes said outcome using descriptive statistics.
To implement a cross-sectional study, you can rely on data assembled by another source or collect your own. Governments often make cross-sectional datasets freely available online.
Prominent examples include the censuses of several countries like the US or France , which survey a cross-sectional snapshot of the country’s residents on important measures. International organizations like the World Health Organization or the World Bank also provide access to cross-sectional datasets on their websites.
However, these datasets are often aggregated to a regional level, which may prevent the investigation of certain research questions. You will also be restricted to whichever variables the original researchers decided to study.
If you want to choose the variables in your study and analyze your data on an individual level, you can collect your own data using research methods such as surveys . It’s important to carefully design your questions and choose your sample .
Like any research design , cross-sectional studies have various benefits and drawbacks.
- Because you only collect data at a single point in time, cross-sectional studies are relatively cheap and less time-consuming than other types of research.
- Cross-sectional studies allow you to collect data from a large pool of subjects and compare differences between groups.
- Cross-sectional studies capture a specific moment in time. National censuses, for instance, provide a snapshot of conditions in that country at that time.
Disadvantages
- It is difficult to establish cause-and-effect relationships using cross-sectional studies, since they only represent a one-time measurement of both the alleged cause and effect.
- Since cross-sectional studies only study a single moment in time, they cannot be used to analyze behavior over a period of time or establish long-term trends.
- The timing of the cross-sectional snapshot may be unrepresentative of behavior of the group as a whole. For instance, imagine you are looking at the impact of psychotherapy on an illness like depression. If the depressed individuals in your sample began therapy shortly before the data collection, then it might appear that therapy causes depression even if it is effective in the long term.
Prevent plagiarism. Run a free check.
If you want to know more about statistics , methodology , or research bias , make sure to check out some of our other articles with explanations and examples.
- Normal distribution
- Degrees of freedom
- Null hypothesis
- Discourse analysis
- Control groups
- Mixed methods research
- Non-probability sampling
- Quantitative research
- Ecological validity
Research bias
- Rosenthal effect
- Implicit bias
- Cognitive bias
- Selection bias
- Negativity bias
- Status quo bias
Longitudinal studies and cross-sectional studies are two different types of research design . In a cross-sectional study you collect data from a population at a specific point in time; in a longitudinal study you repeatedly collect data from the same sample over an extended period of time.
Longitudinal study | Cross-sectional study |
---|---|
observations | Observations at a in time |
Observes the multiple times | Observes (a “cross-section”) in the population |
Follows in participants over time | Provides of society at a given point |
Cross-sectional studies are less expensive and time-consuming than many other types of study. They can provide useful insights into a population’s characteristics and identify correlations for further research.
Sometimes only cross-sectional data is available for analysis; other times your research question may only require a cross-sectional study to answer it.
Cross-sectional studies cannot establish a cause-and-effect relationship or analyze behavior over a period of time. To investigate cause and effect, you need to do a longitudinal study or an experimental study .
Cite this Scribbr article
If you want to cite this source, you can copy and paste the citation or click the “Cite this Scribbr article” button to automatically add the citation to our free Citation Generator.
Thomas, L. (2023, June 22). Cross-Sectional Study | Definition, Uses & Examples. Scribbr. Retrieved September 3, 2024, from https://www.scribbr.com/methodology/cross-sectional-study/
Is this article helpful?
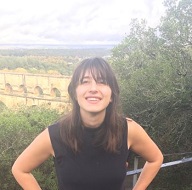
Lauren Thomas
Other students also liked, longitudinal study | definition, approaches & examples, correlational research | when & how to use, survey research | definition, examples & methods, "i thought ai proofreading was useless but..".
I've been using Scribbr for years now and I know it's a service that won't disappoint. It does a good job spotting mistakes”
Cross-Sectional Study: Definition, Designs & Examples
Julia Simkus
Editor at Simply Psychology
BA (Hons) Psychology, Princeton University
Julia Simkus is a graduate of Princeton University with a Bachelor of Arts in Psychology. She is currently studying for a Master's Degree in Counseling for Mental Health and Wellness in September 2023. Julia's research has been published in peer reviewed journals.
Learn about our Editorial Process
Saul McLeod, PhD
Editor-in-Chief for Simply Psychology
BSc (Hons) Psychology, MRes, PhD, University of Manchester
Saul McLeod, PhD., is a qualified psychology teacher with over 18 years of experience in further and higher education. He has been published in peer-reviewed journals, including the Journal of Clinical Psychology.
Olivia Guy-Evans, MSc
Associate Editor for Simply Psychology
BSc (Hons) Psychology, MSc Psychology of Education
Olivia Guy-Evans is a writer and associate editor for Simply Psychology. She has previously worked in healthcare and educational sectors.
On This Page:
A cross-sectional study design is a type of observational study, or descriptive research, that involves analyzing information about a population at a specific point in time.
This design measures the prevalence of an outcome of interest in a defined population. It provides a snapshot of the characteristics of the population at a single point in time.
It can be used to assess the prevalence of outcomes and exposures, determine relationships among variables, and generate hypotheses about causal connections between factors to be explored in experimental designs.
Typically, these studies are used to measure the prevalence of health outcomes and describe the characteristics of a population.
In this study, researchers examine a group of participants and depict what already exists in the population without manipulating any variables or interfering with the environment.
Cross-sectional studies aim to describe a variable , not measure it. They can be beneficial for describing a population or “taking a snapshot” of a group of individuals at a single moment in time.
In epidemiology and public health research, cross-sectional studies are used to assess exposure (cause) and disease (effect) and compare the rates of diseases and symptoms of an exposed group with an unexposed group.
Cross-sectional studies are also unique because researchers are able to look at numerous characteristics at once.
For example, a cross-sectional study could be used to investigate whether exposure to certain factors, such as overeating, might correlate to particular outcomes, such as obesity.
While this study cannot prove that overeating causes obesity, it can draw attention to a relationship that might be worth investigating.
Cross-sectional studies can be categorized based on the nature of the data collection and the type of data being sought.
Cross-Sectional Study | Purpose | Example |
---|---|---|
To describe the characteristics of a population. | Examining the dietary habits of high school students. | |
To investigate associations between variables. | Studying the correlation between smoking and lung disease in adults. | |
To gather information on a population or a subset. | Conducting a survey on the use of public transportation in a city. | |
To determine the proportion of a population with a specific characteristic, condition, or disease. | Assessing the prevalence of obesity in a country. | |
To examine the effects of certain occupational or environmental exposures. | Studying the impact of air pollution on respiratory health in industrial workers. | |
To generate hypotheses for future research. | Investigating relationships between various lifestyle factors and mental health conditions. |
Analytical Studies
In analytical cross-sectional studies, researchers investigate an association between two parameters. They collect data for exposures and outcomes at one specific time to measure an association between an exposure and a condition within a defined population.
The purpose of this type of study is to compare health outcome differences between exposed and unexposed individuals.
Descriptive Studies
- Descriptive cross-sectional studies are purely used to characterize and assess the prevalence and distribution of one or many health outcomes in a defined population.
- They can assess how frequently, widely, or severely a specific variable occurs throughout a specific demographic.
- This is the most common type of cross-sectional study.
- Evaluating the COVID-19 positivity rates among vaccinated and unvaccinated adolescents
- Investigating the prevalence of dysfunctional breathing in patients treated for asthma in primary care (Wang & Cheng, 2020)
- Analyzing whether individuals in a community have any history of mental illness and whether they have used therapy to help with their mental health
- Comparing grades of elementary school students whose parents come from different income levels
- Determining the association between gender and HIV status (Setia, 2016)
- Investigating suicide rates among individuals who have at least one parent with chronic depression
- Assessing the prevalence of HIV and risk behaviors in male sex workers (Shinde et al., 2009)
- Examining sleep quality and its demographic and psychological correlates among university students in Ethiopia (Lemma et al., 2012)
- Calculating what proportion of people served by a health clinic in a particular year have high cholesterol
- Analyzing college students’ distress levels with regard to their year level (Leahy et al., 2010)
Simple and Inexpensive
These studies are quick, cheap, and easy to conduct as they do not require any follow-up with subjects and can be done through self-report surveys.
Minimal room for error
Because all of the variables are analyzed at once, and data does not need to be collected multiple times, there will likely be fewer mistakes as a higher level of control is obtained.
Multiple variables and outcomes can be researched and compared at once
Researchers are able to look at numerous characteristics (ie, age, gender, ethnicity, and education level) in one study.
The data can be a starting point for future research
The information obtained from cross-sectional studies enables researchers to conduct further data analyses to explore any causal relationships in more depth.
Limitations
Does not help determine cause and effect.
Cross-sectional studies can be influenced by an antecedent consequent bias which occurs when it cannot be determined whether exposure preceded disease. (Alexander et al.)
Report bias is probable
Cross-sectional studies rely on surveys and questionnaires, which might not result in accurate reporting as there is no way to verify the information presented.
The timing of the snapshot is not always representative
Cross-sectional studies do not provide information from before or after the report was recorded and only offer a single snapshot of a point in time.
It cannot be used to analyze behavior over a period of time
Cross-sectional studies are designed to look at a variable at a particular moment, while longitudinal studies are more beneficial for analyzing relationships over extended periods.
Cross-Sectional vs. Longitudinal
Both cross-sectional and longitudinal studies are observational and do not require any interference or manipulation of the study environment.
However, cross-sectional studies differ from longitudinal studies in that cross-sectional studies look at a characteristic of a population at a specific point in time, while longitudinal studies involve studying a population over an extended period.
Longitudinal studies require more time and resources and can be less valid as participants might quit the study before the data has been fully collected.
Unlike cross-sectional studies, researchers can use longitudinal data to detect changes in a population and, over time, establish patterns among subjects.
Cross-sectional studies can be done much quicker than longitudinal studies and are a good starting point to establish any associations between variables, while longitudinal studies are more timely but are necessary for studying cause and effect.
Alexander, L. K., Lopez, B., Ricchetti-Masterson, K., & Yeatts, K. B. (n.d.). Cross-sectional Studies. Eric Notebook. Retrieved from https://sph.unc.edu/wp-content/uploads/sites/112/2015/07/nciph_ERIC8.pdf
Cherry, K. (2019, October 10). How Does the Cross-Sectional Research Method Work? Verywell Mind. Retrieved from https://www.verywellmind.com/what-is-a-cross-sectional-study-2794978
Cross-sectional vs. longitudinal studies. Institute for Work & Health. (2015, August). Retrieved from https://www.iwh.on.ca/what-researchers-mean-by/cross-sectional-vs-longitudinal-studies
Leahy, C. M., Peterson, R. F., Wilson, I. G., Newbury, J. W., Tonkin, A. L., & Turnbull, D. (2010). Distress levels and self-reported treatment rates for medicine, law, psychology and mechanical engineering tertiary students: cross-sectional study. The Australian and New Zealand journal of psychiatry, 44(7), 608–615.
Lemma, S., Gelaye, B., Berhane, Y. et al. Sleep quality and its psychological correlates among university students in Ethiopia: a cross-sectional study. BMC Psychiatry 12, 237 (2012).
Wang, X., & Cheng, Z. (2020). Cross-Sectional Studies: Strengths, Weaknesses, and Recommendations. Chest, 158(1S), S65–S71.
Setia M. S. (2016). Methodology Series Module 3: Cross-sectional Studies. Indian journal of dermatology, 61 (3), 261–264.
Shinde S, Setia MS, Row-Kavi A, Anand V, Jerajani H. Male sex workers: Are we ignoring a risk group in Mumbai, India? Indian J Dermatol Venereol Leprol. 2009;75:41–6.
Further Information
- Setia, M. S. (2016). Methodology series module 3: Cross-sectional studies. Indian journal of dermatology, 61(3), 261.
- Sedgwick, P. (2014). Cross sectional studies: advantages and disadvantages. Bmj, 348.
1. Are cross-sectional studies qualitative or quantitative?
Cross-sectional studies can be either qualitative or quantitative , depending on the type of data they collect and how they analyze it. Often, the two approaches are combined in mixed-methods research to get a more comprehensive understanding of the research problem.
2. What’s the difference between cross-sectional and cohort studies?
A cohort study is a type of longitudinal study that samples a group of people with a common characteristic. One key difference is that cross-sectional studies measure a specific moment in time, whereas cohort studies follow individuals over extended periods.
Another difference between these two types of studies is the subject pool. In cross-sectional studies, researchers select a sample population and gather data to determine the prevalence of a problem.
Cohort studies, on the other hand, begin by selecting a population of individuals who are already at risk for a specific disease.
3. What’s the difference between cross-sectional and case-control studies?
Case-control studies differ from cross-sectional studies in that case-control studies compare groups retrospectively and cannot be used to calculate relative risk.
In these studies, researchers study one group of people who have developed a particular condition and compare them to a sample without the disease.
Case-control studies are used to determine what factors might be associated with the condition and help researchers form hypotheses about a population.
4. Does a cross-sectional study have a control group?
A cross-sectional study does not need to have a control group , as the population studied is not selected based on exposure.
In a cross-sectional study, data are collected from a sample of the target population at a specific point in time, and everyone in the sample is assessed in the same way. There isn’t a manipulation of variables or a control group as there would be in an experimental study design.
5. Is a cross-sectional study prospective or retrospective?
A cross-sectional study is generally considered neither prospective nor retrospective because it provides a “snapshot” of a population at a single point in time.
Cross-sectional studies are not designed to follow individuals forward in time ( prospective ) or look back at historical data ( retrospective ), as they analyze data from a specific point in time.


Cross-Sectional Study in Research

Introduction
What is a cross-sectional study in research, what is the difference between cross-sectional and longitudinal research, cross-sectional study examples, types of cross-sectional studies, benefits of cross-sectional studies, challenges of cross-sectional studies.
Cross-sectional studies are a fundamental research method used across various fields to analyze data at a specific point in time. By comparing different subjects without considering the time variable, these studies can provide valuable insights into the prevalence and characteristics of phenomena within a population.
This article explores the concept of cross-sectional research, outlining its key features, applications, and how it differs from longitudinal studies. We will also examine examples of cross-sectional data, discuss the various types of cross-sectional studies, and highlight both the advantages and challenges associated with this research method. Understanding when and how to employ research methods for a cross-sectional study design is crucial for researchers aiming to draw accurate and meaningful conclusions from their data .
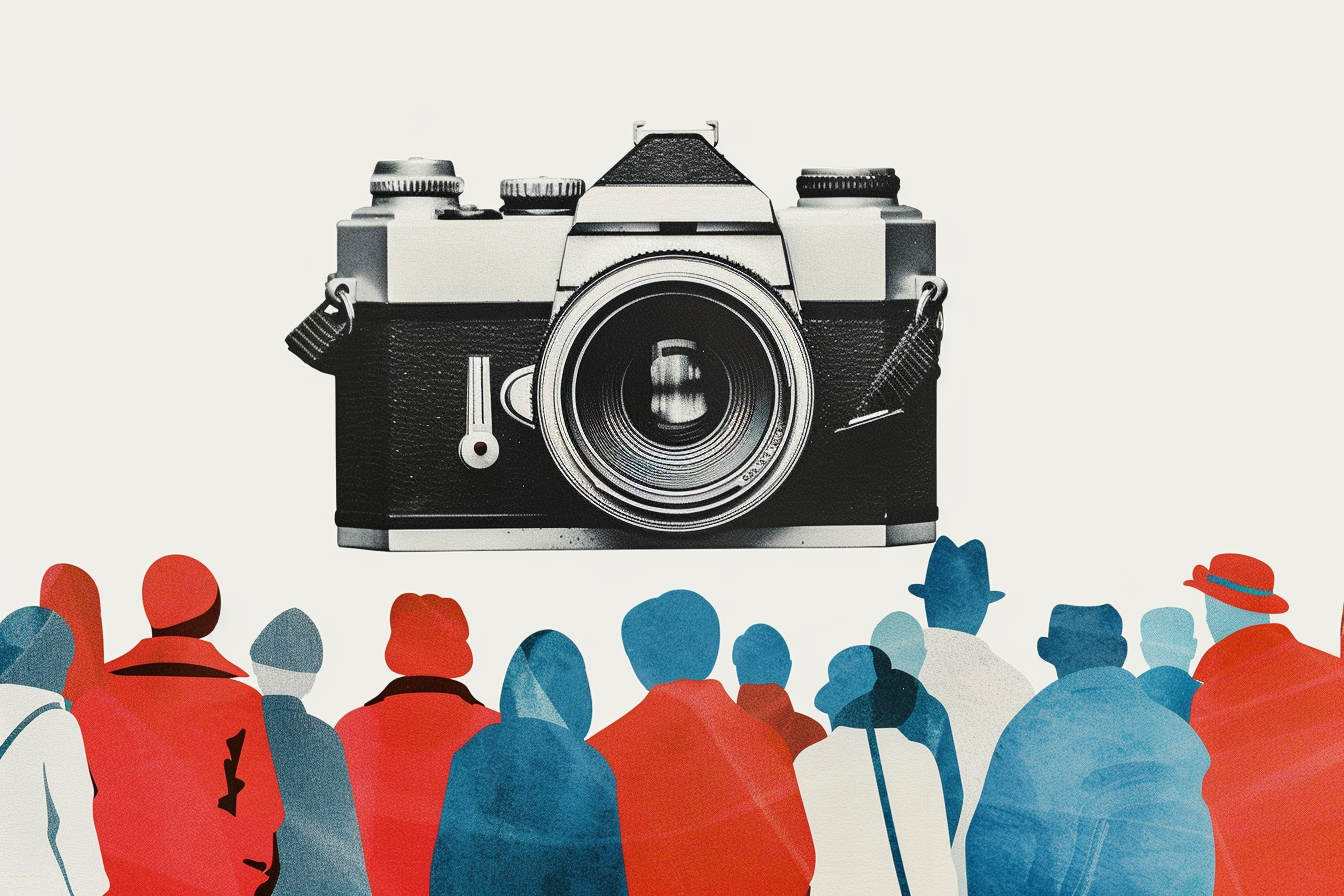
A cross-sectional study is a type of observational research design that analyzes data from a population, or a representative subset, at one specific point in time. Unlike longitudinal studies that observe the same subjects over a period of time to detect changes, cross-sectional studies focus on finding relationships and prevalences within a predefined snapshot. This method is particularly useful for understanding the current status of a phenomenon or to identify associations between variables without inferring causal relationships.
In practice, cross-sectional studies collect data across a wide range of subjects at a single moment, aiming to capture a comprehensive picture of a particular research question. Researchers might analyze various factors, including demographic information, behaviors, conditions, or outcomes, to discern patterns or correlations within the population studied.
Though these studies cannot determine cause and effect, they are invaluable for generating hypotheses or propositions, informing policy decisions, and guiding future research. Their descriptive nature and relative ease of execution make cross-sectional studies a common starting point in many research endeavors, providing a foundational understanding of the context and variables of interest.
The primary distinction between cross-sectional and longitudinal research lies in how and when the data is collected. Cross-sectional studies differ in that they capture data at a single point in time, offering a snapshot that helps to identify the prevalence and relationships between variables within a specific moment that further research might be able to explore. In contrast, a longitudinal study involves collecting data from the same subjects repeatedly over an extended period of time, enabling the observation of changes and developments in the variables of interest.
While cross-sectional studies are efficient for gathering data at one point in time and are less costly and time-consuming than longitudinal studies, they fall short in tracking changes over time or establishing cause-and-effect relationships. On the other hand, longitudinal studies excel in observing how variables evolve, providing insights into dynamics and causal pathways. However, longitudinal data collection requires more resources, time, and a rigorous design to manage participant attrition and ensure consistent data collection over the study period.
Another key difference is in the potential for cohort effects. A cross-sectional analysis might conflate age-related changes with generational effects because different age groups are compared at one particular point in time. Longitudinal research, by observing the same individuals over time, can differentiate between aging effects and cohort effects, offering a clearer view of how specific and multiple variables change throughout an individual's life or over time.
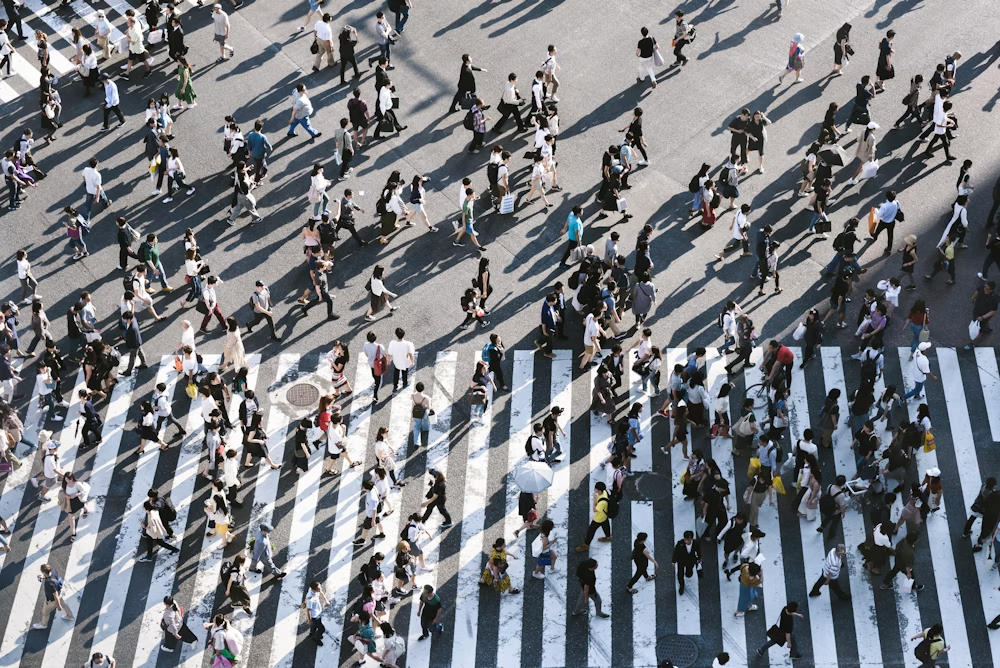
Cross-sectional studies are employed across various disciplines to investigate multiple phenomena at a specific point in time. These studies offer insights into the prevalence, distribution, and potential associations between variables within a defined population.
Below are three examples from different fields illustrating how cross-sectional research is applied to glean valuable findings.
Healthcare: Prevalence of a medical condition
In medical research, cross-sectional studies are frequently used to determine the prevalence of diseases or health outcomes in a population. For instance, a study might collect cross-sectional data from a diverse sample of individuals to assess the current prevalence of diabetes. By analyzing factors such as age, lifestyle, and comorbidities, researchers can identify patterns and risk factors associated with the disease, aiding in public health planning and intervention strategies.
Education: Analyzing student performance
Educational researchers often use a cross-sectional design to evaluate student performance across different grades or age groups at a single point in time. Such a study could compare test scores to analyze trends and disparities in educational achievement. By examining variables like socio-economic status, teaching methods, and school resources, educators and policymakers can identify areas needing improvement or intervention.
Economics: Employment trends analysis
In economics, a cross-sectional survey can provide snapshots of employment trends within a specific region or sector. An example might involve analyzing the employment rates, job types, and economic sectors in a country at a given time. This data can reveal insights into the economic health, workforce distribution, and potential areas for economic development or policy focus, informing stakeholders and guiding decision-making processes.

Turn data into insights with ATLAS.ti's analytical tools
Download a free trial to see how you can make the most of your data.
Cross-sectional studies can be categorized into different types based on their objectives and methodologies . These variations allow researchers to adapt the cross-sectional approach to suit specific research questions and contexts.
By understanding the different types of cross-sectional studies, researchers can select the most appropriate design to obtain reliable and relevant data. Below are four common types of cross-sectional studies, each with its unique focus and application.
Descriptive cross-sectional studies
Descriptive cross-sectional studies aim to provide a detailed snapshot of a population or phenomenon at a particular point in time. These studies focus on 'what exists' or 'what is prevalent' without delving into relationships between variables or concepts.
For example, a descriptive research study might catalog various health behaviors within a specific demographic group to inform public health initiatives. The primary goal is to describe characteristics, frequencies, or distributions as they exist in the study population.
Analytical cross-sectional studies
Unlike descriptive studies that focus on prevalence and distribution, analytical cross-sectional studies aim to uncover potential associations between variables. These studies often compare different groups within the population to identify factors that may correlate with certain outcomes.
For instance, an analytical cross-sectional study might investigate the relationship between lifestyle choices and blood pressure levels across various age groups. While these studies can suggest associations, they do not establish cause and effect.
Exploratory cross-sectional studies
Exploratory cross-sectional studies are conducted to explore potential relationships or hypotheses when little is known about a subject. These studies are particularly useful in emerging fields or for new phenomena. By examining available data, they can generate hypotheses for further research without committing extensive resources to long-term studies.
An example might be exploring the usage patterns of a new technology within a population to identify trends and areas for in-depth study.
Explanatory cross-sectional studies
Explanatory cross-sectional studies go beyond identifying associations; they aim to explain why certain patterns or relationships are observed. These studies often incorporate theoretical frameworks or models to analyze the data within a broader context, providing deeper insights into the underlying mechanisms or factors.
For example, an explanatory cross-sectional study could investigate why certain educational strategies are associated with better student outcomes, integrating theories of learning and cognition.
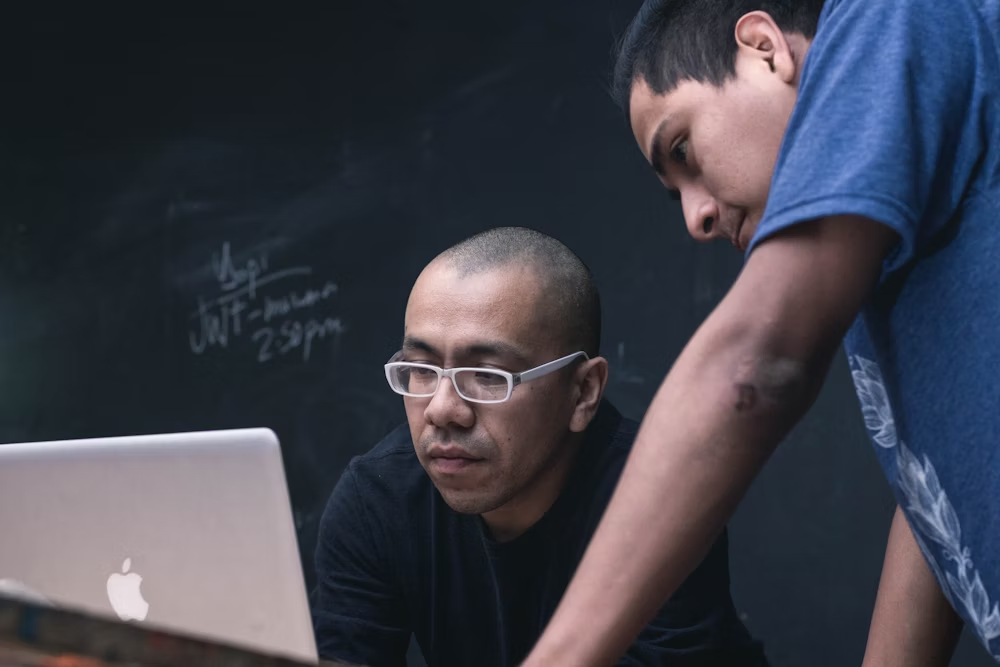
Cross-sectional studies are a crucial tool in the repertoire of research methodologies , offering unique advantages that make them particularly suitable for various research contexts. These studies are instrumental in providing a snapshot of a specific point in time, which can be invaluable for understanding the status quo and informing future research directions. Below, we explore three significant benefits of employing cross-sectional studies in research endeavors.
Cost-effectiveness
One of the primary benefits of cross-sectional studies is their cost-effectiveness compared to longitudinal studies . Since they are conducted at a single point in time and do not require follow-ups, the financial resources, time, and logistical efforts needed are considerably lower. This efficiency makes cross-sectional studies an appealing option for researchers with limited budgets or those seeking preliminary data before committing to more extensive research.
Cross-sectional studies are inherently timely, providing quick snapshots that are especially valuable in fast-paced research areas where timely data is crucial. They allow researchers to collect and analyze data relatively quickly, offering insights that are current and relevant. This timeliness is particularly beneficial for informing immediate policy decisions or for studies in fields where trends may change rapidly, such as technology or public health.
Versatility
The versatility of cross-sectional studies is evident in their wide applicability across various fields and purposes. They can be designed to explore numerous variables and their interrelations within different populations and settings. This flexibility enables researchers to tailor studies to specific research questions, making cross-sectional studies a versatile tool for exploratory research, hypothesis generation , or situational analysis across disciplines.
Despite their utility in various fields of research, cross-sectional studies face distinct challenges that can affect the validity and applicability of their findings. Understanding these limitations is crucial for researchers to design robust studies and for readers to interpret results appropriately. Here are three key challenges commonly associated with cross-sectional studies.
Causality determination
One of the inherent limitations of cross-sectional studies is their inability to establish causality. Since data is collected at a single point in time, it is challenging to ascertain whether a relationship between two variables is causal or merely correlational. This limitation necessitates cautious interpretation of results, as establishing temporal precedence is essential for causal inference, which cross-sectional designs cannot provide.
Selection bias
Selection bias can occur in cross-sectional studies if the sample is not representative of the population from which it was drawn. This can happen due to non-random sampling methods or non-response, leading to skewed results that do not accurately reflect the broader population. Such bias can compromise the generalizability of the study's findings, making it critical to employ rigorous sampling methods and consider potential biases during analysis.
Cross-sectional confounding
Cross-sectional studies can also be susceptible to confounding, where an external variable influences both the independent and dependent variables , creating a spurious association. Without longitudinal data , it is difficult to control for or identify these confounding factors, which can lead to erroneous conclusions. Researchers must carefully consider potential confounders and employ statistical methods to adjust for these variables where possible.

Analyze any qualitative data with ATLAS.ti
Powerful analysis tools are at your fingertips, starting with a free trial.
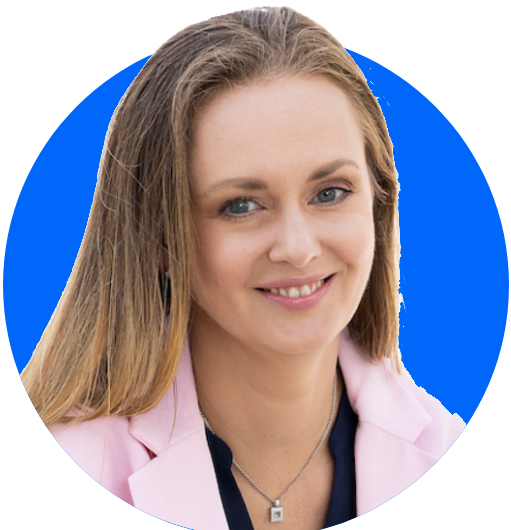
Thank you for visiting nature.com. You are using a browser version with limited support for CSS. To obtain the best experience, we recommend you use a more up to date browser (or turn off compatibility mode in Internet Explorer). In the meantime, to ensure continued support, we are displaying the site without styles and JavaScript.
- View all journals
- Explore content
- About the journal
- Publish with us
- Sign up for alerts
- Published: 24 March 2006
Study design III: Cross-sectional studies
- Kate Ann Levin 1
Evidence-Based Dentistry volume 7 , pages 24–25 ( 2006 ) Cite this article
337k Accesses
370 Citations
55 Altmetric
Metrics details
In this series, I previously gave an overview of the main types of study design and the techniques used to minimise biased results. Here, I describe cross-sectional studies, their uses, advantages and limitations.
You have full access to this article via your institution.
Cross-sectional studies are carried out at one time point or over a short period ( Figure 1 ). They are usually conducted to estimate the prevalence of the outcome of interest for a given population, commonly for the purposes of public health planning. Data can also be collected on individual characteristics, including exposure to risk factors, alongside information about the outcome. In this way cross-sectional studies provide a ‘snapshot’ of the outcome and the characteristics associated with it, at a specific point in time.
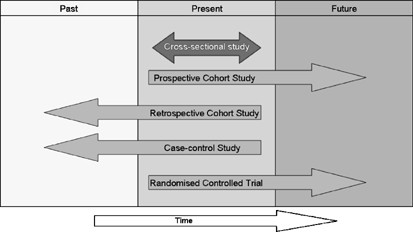
Cross-sectional studies.
Why carry out a cross-sectional study?
A cross-sectional study design is used when
The purpose of the study is descriptive, often in the form of a survey. Usually there is no hypothesis as such, but the aim is to describe a population or a subgroup within the population with respect to an outcome and a set of risk factors.
The purpose of the study is to find the prevalence of the outcome of interest, for the population or subgroups within the population at a given timepoint.
Cross-sectional studies are sometimes carried out to investigate associations between risk factors and the outcome of interest. They are limited, however, by the fact that they are carried out at one time point and give no indication of the sequence of events — whether exposure occurred before, after or during the onset of the disease outcome. This being so, it is impossible to infer causality.
The next four publications of Evidence-based Dentistry describe other study designs that may be more appropriate for the purposes of understanding associations between exposure to risk factors and the outcome of interest. Nevertheless, cross-sectional studies indicate associations that may exist and are therefore useful in generating hypotheses for future research.
Repeated cross-sectional studies may be carried out to give a pseudolongitudinal study, where the individuals included in the study are either chosen from the same sampling frame or from a different one. An example might be the British Association for the Study of Community Dentistry Survey in which 5-year-old children are examined annually and prevalence of caries is recorded. The prevalence of caries for this age group is monitored over time and this information is used in public health policy planning and in the development of targeting strategies.
Sample selection and response rates
The sample frame used to select a sample and the response rate determine how well results can be generalised to the population as a whole. The sample used in a large cross-sectional study is often taken from the whole population. This is the optimum situation: if the sample is selected using a random technique it is likely that it will be highly representative. In order for the results to be representative of the population, however, not only must the selected sample be representative but so must the responders. Nonresponse is a common problem in wide-scale surveys; techniques to minimise nonresponse include telephone and mail prompting, second and third mailing of surveys, letters outlining the importance of replying and a range of incentives.
The level of nonresponse is one concern, but a greater one still is that of biased response, where a person is more likely to respond when they have a particular characteristic or set of characteristics. Bias will occur when the characteristic in question is in some way related to the probability of having the outcome. The response rate of a survey conducted by door-to-door interview looking at a particular disease, for example, may be highest in the elderly and unemployed because these groups are more likely to be in their home during the day. These two groups are also more likely to experience higher levels of disease, therefore biasing the results.
Measures of outcome and exposure
A lot of information can be collected about potential risk factors in a cross-sectional study. Loss to follow-up is a common concern in longitudinal studies and one of the strategies used to overcome this is to minimise the amount of information collected. This is not a problem in cross-sectional study design.
It is advisable to think carefully about what might be relevant because this is a good opportunity to gain a broad base of knowledge about subjects who have/do not have the outcome of interest, but it is also important to maintain optimum response levels. Associations between outcomes and exposures of long duration are particularly difficult to establish using cross-sectional studies.
Advantages of cross-sectional studies
Relatively inexpensive and takes up little time to conduct;
Can estimate prevalence of outcome of interest because sample is usually taken from the whole population;
Many outcomes and risk factors can be assessed;
Useful for public health planning, understanding disease aetiology and for the generation of hypotheses;
There is no loss to follow-up.
Disadvantages of cross-sectional studies
Difficult to make causal inference;
Only a snapshot: the situation may provide differing results if another time-frame had been chosen;
Prevalence-incidence bias (also called Neyman bias). Especially in the case of longer-lasting diseases, any risk factor that results in death will be under-represented among those with the disease.
Bland M . An Introduction to Medical Statistics . 3rd Edn. Oxford: Oxford University Press; 2001.
Google Scholar
Pine CM, Pitts NB, Nugent ZJ . British Association for the Study of Community Dentistry (BASCD) guidance on sampling for surveys of child dental health. A BASCD coordinated dental epidemiology programme quality standard. Commun Dent Health 1997; 14 (Suppl 1):S10–S17.
Download references
Author information
Authors and affiliations.
Dental Health Services Research Unit, University of Dundee, Dundee, Scotland, UK
Kate Ann Levin
You can also search for this author in PubMed Google Scholar
Rights and permissions
Reprints and permissions
About this article
Cite this article.
Levin, K. Study design III: Cross-sectional studies. Evid Based Dent 7 , 24–25 (2006). https://doi.org/10.1038/sj.ebd.6400375
Download citation
Published : 24 March 2006
Issue Date : 01 March 2006
DOI : https://doi.org/10.1038/sj.ebd.6400375
Share this article
Anyone you share the following link with will be able to read this content:
Sorry, a shareable link is not currently available for this article.
Provided by the Springer Nature SharedIt content-sharing initiative
This article is cited by
Trait-level non-clinical adhd symptoms in a community sample and their association with technology addictions.
- Benjamin A. Parris
- Jacqui Taylor
Current Psychology (2024)
Local politics and the revenue collection effort in a developing country context: experiences from Ghana
- Patrick Yin Mahama
- Abdul-Gafaru Abdulai
- Kwame Asamoah
SN Social Sciences (2024)
Longitudinal Job Crafting Research: A Meta-Analysis
- Likitha Silapurem
- Gavin R. Slemp
- Aaron Jarden
International Journal of Applied Positive Psychology (2024)
Dark Triad and COVID-19 vaccine hesitancy: the role of conspiracy beliefs and risk perception
- Marco Giancola
- Massimiliano Palmiero
- Simonetta D’Amico
Topological features of functional brain networks and subclinical impulsivity: an investigation in younger and older adults
- Silvia Fornaro
- Arianna Menardi
- Antonino Vallesi
Brain Structure and Function (2024)
Quick links
- Explore articles by subject
- Guide to authors
- Editorial policies


I want to publish
To find out how to publish or submit your book proposal:
To find a journal or submit your article to a journal:
- Cross Sectional Study
- Medicine and Healthcare
- Epidemiology
Surveys, longitudinal, cross-sectional and trend studies
Louis Cohen, Lawrence Manion, Keith Morrison in Research Methods in Education , 2017
A cross-sectional study is one that produces a ‘snapshot’ of a population at one particular point in time. The epitome of the cross-sectional study is a national survey in which a representative sample of the population consisting of individuals of different ages, different occupations, different educational and income levels, and residing in different parts of the country, is interviewed on the same day. In education, cross-sectional studies can involve indirect measures of the nature and rate of changes in the physical and intellectual development of samples of children drawn from representative age levels. The single ‘snapshot’ of the cross-sectional study provides researchers with data for either a retrospective or a prospective enquiry. A cross-sectional study can also bear several hallmarks of a longitudinal study of parallel groups (e.g. age groups) which are drawn simultaneously from the population. For example, drawing students aged five, seven, nine and eleven at a single point in time would bear some characteristics of a longitudinal study in that developments over age groups could be seen, though, of course, it would not have the same weight as a longitudinal study conducted on the same age group over time. This is the case for international studies of educational achievement, requiring samples to be drawn from the same population (Lietz and Keeves, 1997, p. 122) and for factors that might influence changes in the dependent variables to remain constant across the age groups. Cross-sectional studies, catching a frozen moment in time, may be ineffective for studying change or causality. If changes are to be addressed through cross-sectional surveys, then this suggests the need for repeated applications of the survey, or the use of trend analysis. The main types of longitudinal study are illustrated in Figure 17.2.
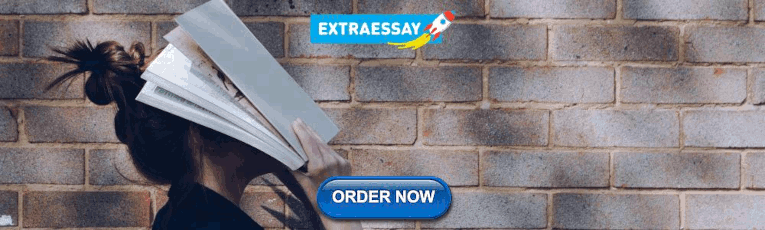
Critical appraisal of cross-sectional surveys
O. Ajetunmobi in Making Sense of Critical Appraisal , 2021
Cross-sectional studies are observational types of studies in which the prevalence of a trait or disease condition is measured by snapshot assessments across a given population. Compare this to randomized clinical trials (RCTs), which test hypotheses on the treatment of disease, or prospective and retrospective studies, which test hypotheses on the aetiology of disease. Prevalence and incidence rates are described in more detail in the ‘glossary of terms’ at the end of the book. Because subjects are not followed up in time, cross-sectional surveys are poor at measuring the rates of occurrence of new cases of a disease in the population, that is, ‘incidence rates’ but they can be used to measure prevalence rates of disease.
Observational Studies
Abhaya Indrayan in Research Methods for Medical Graduates , 2019
The cross-sectional format is rarely used for an analytical study because it fails to provide a good assessment of cause–effect types of relationships. However, it is suitable when it is not clear what the antecedent and what the outcome is. In the case of peptic ulcer and milk consumption, either could be a cause of the other. A cross-sectional study is a good tool to generate a hypothesis, which can be subsequently tested by a case–control or a prospective study. A cross-sectional study is certainly good as a descriptive study. It is quick and easy to complete. It starts with a reference population, so generalization is immediate when based on a genuine random sample. Repeated cross-sectional studies are good for detecting changes in known risk factors besides, of course, the time trend of the disease. As explained later in Chapter 7, it is often helpful to obtain sensitivity–specificity or predictivities of a test procedure as indicators of its validity. The former requires a case–control study and the latter a prospective study. Sometimes a cross-sectional study is used to calculate both types of indices. When the subjects are representative of the target population, the percentage of subjects with disease and without disease would be nearly the same as in the target population. The test positives and test negatives would also be in the representative proportion, but they will be prevalent cases and not incident cases, nor “incidence” of antecedents. As explained in a later chapter, prevalence may be in proportion to incidence under stable conditions.
Validity and Reliability of the Persian Version of Internet Sex Screening Test in Iranian Medical Students
Published in Sexual Addiction & Compulsivity , 2019
Mohammadreza Shalbafan , Mohammadreza Najarzadegan , Shiva Soraya , Vahid Rashedi , Ruohollah Najafian , Hamidreza Ahmadkhaniha , Ruohollah Seddigh , Morteza Hassanzadeh , Vahid Saeedi , Leila Kamalzadeh
This study investigated the cultural adaptation, validity, and reliability of the Persian version of Internet Sex Screening Test (P-ISST). In this cross-sectional study, 97 medical students were included. Content and face validity of the questionnaire were established by experts. The item-level content validity index ranged from 0.83 to 1.0. Internal consistency for the P-ISST total score (α = 0.923) was acceptable. Except for the “Online sexual spending” subscale, the Cronbach’s alpha values for subscales fell between 0.7 and 0.9. This instrument can be used as an easily administered screening tool for assessment of online sexual activities in university students.
Substance use among students in Thailand
Published in Journal of Ethnicity in Substance Abuse , 2019
Pimpisa Chomsri , Apinun Aramrattana , Penprapa Siviroj , Surinporn Likhitsathian
This study explored substance use prevalence, level of risk, and associated factors, especially related to binge drinking. A cross-sectional study was conducted among 306 students using questionnaires and the Alcohol, Smoking, and Substance Involvement Screening Test—Youth screening tool. The associations between binge drinking and risk factors were analyzed by generalized linear models. Our results showed current prevalence rates of alcohol, tobacco, and drugs use were 56.9%, 22.9%, and 2.3%, respectively. Multivariate analyses showed that being a binge drinker was significantly associated with low self-efficacy, high sensation seeking, moderate to high smoking risk, and low grade point average.
Shift work and serum 25-OH vitamin D status among factory workers in Northern Italy: Cross-sectional study
Published in Chronobiology International , 2015
Alessandro Romano , Luisella Vigna , Valentina Belluigi , Diana Misaela Conti , Claudia Eleonora Barberi , Laura Tomaino , Dario Consonni , Luciano Riboldi , Amedea Silvia Tirelli , Lars Louis Andersen
Low levels of vitamin D are related to muscle weakness, poor balance, and higher risk of falls, and can therefore have a major impact on performance and safety at work. Little knowledge exists on the association between work environment and vitamin D status. This study evaluates vitamin D status in shift workers. In this cross-sectional study, led during early springtime, 96 male shift workers at an engineering factory in Northern Italy, and 100 male daily workers operating nearby, participated. 25-OH vitamin D concentration, anthropometric indexes, fasting glycemia and triglycerides were detected. 51 shift workers underwent anamnesis collection on lifestyle and habits and determination of heel bone mineral density. Vitamin D levels were lower in shift workers than daily ones (13.4 ± 5.3 ng/mL versus 21.9 ± 10.7 ng/mL, p
Related Knowledge Centers
- Cohort Studies
- Epidemiologic Studies
- Longitudinal Studies
- Control Studies
- Sectional Studies
Current Research
- Clinical Trials (United States)
- Clinical Trials (Europe)
- Clinical Trials (Australia/New Zealand)
- Clinical Trials (India)
Knowledge is an evolving asset. Help us improve this page for a future release.
- Search Menu
Sign in through your institution
- Browse content in Arts and Humanities
- Browse content in Archaeology
- Anglo-Saxon and Medieval Archaeology
- Archaeological Methodology and Techniques
- Archaeology by Region
- Archaeology of Religion
- Archaeology of Trade and Exchange
- Biblical Archaeology
- Contemporary and Public Archaeology
- Environmental Archaeology
- Historical Archaeology
- History and Theory of Archaeology
- Industrial Archaeology
- Landscape Archaeology
- Mortuary Archaeology
- Prehistoric Archaeology
- Underwater Archaeology
- Zooarchaeology
- Browse content in Architecture
- Architectural Structure and Design
- History of Architecture
- Residential and Domestic Buildings
- Theory of Architecture
- Browse content in Art
- Art Subjects and Themes
- History of Art
- Industrial and Commercial Art
- Theory of Art
- Biographical Studies
- Byzantine Studies
- Browse content in Classical Studies
- Classical History
- Classical Philosophy
- Classical Mythology
- Classical Numismatics
- Classical Literature
- Classical Reception
- Classical Art and Architecture
- Classical Oratory and Rhetoric
- Greek and Roman Epigraphy
- Greek and Roman Law
- Greek and Roman Papyrology
- Greek and Roman Archaeology
- Late Antiquity
- Religion in the Ancient World
- Social History
- Digital Humanities
- Browse content in History
- Colonialism and Imperialism
- Diplomatic History
- Environmental History
- Genealogy, Heraldry, Names, and Honours
- Genocide and Ethnic Cleansing
- Historical Geography
- History by Period
- History of Emotions
- History of Agriculture
- History of Education
- History of Gender and Sexuality
- Industrial History
- Intellectual History
- International History
- Labour History
- Legal and Constitutional History
- Local and Family History
- Maritime History
- Military History
- National Liberation and Post-Colonialism
- Oral History
- Political History
- Public History
- Regional and National History
- Revolutions and Rebellions
- Slavery and Abolition of Slavery
- Social and Cultural History
- Theory, Methods, and Historiography
- Urban History
- World History
- Browse content in Language Teaching and Learning
- Language Learning (Specific Skills)
- Language Teaching Theory and Methods
- Browse content in Linguistics
- Applied Linguistics
- Cognitive Linguistics
- Computational Linguistics
- Forensic Linguistics
- Grammar, Syntax and Morphology
- Historical and Diachronic Linguistics
- History of English
- Language Acquisition
- Language Evolution
- Language Reference
- Language Variation
- Language Families
- Lexicography
- Linguistic Anthropology
- Linguistic Theories
- Linguistic Typology
- Phonetics and Phonology
- Psycholinguistics
- Sociolinguistics
- Translation and Interpretation
- Writing Systems
- Browse content in Literature
- Bibliography
- Children's Literature Studies
- Literary Studies (Asian)
- Literary Studies (European)
- Literary Studies (Eco-criticism)
- Literary Studies (Romanticism)
- Literary Studies (American)
- Literary Studies (Modernism)
- Literary Studies - World
- Literary Studies (1500 to 1800)
- Literary Studies (19th Century)
- Literary Studies (20th Century onwards)
- Literary Studies (African American Literature)
- Literary Studies (British and Irish)
- Literary Studies (Early and Medieval)
- Literary Studies (Fiction, Novelists, and Prose Writers)
- Literary Studies (Gender Studies)
- Literary Studies (Graphic Novels)
- Literary Studies (History of the Book)
- Literary Studies (Plays and Playwrights)
- Literary Studies (Poetry and Poets)
- Literary Studies (Postcolonial Literature)
- Literary Studies (Queer Studies)
- Literary Studies (Science Fiction)
- Literary Studies (Travel Literature)
- Literary Studies (War Literature)
- Literary Studies (Women's Writing)
- Literary Theory and Cultural Studies
- Mythology and Folklore
- Shakespeare Studies and Criticism
- Browse content in Media Studies
- Browse content in Music
- Applied Music
- Dance and Music
- Ethics in Music
- Ethnomusicology
- Gender and Sexuality in Music
- Medicine and Music
- Music Cultures
- Music and Religion
- Music and Media
- Music and Culture
- Music Education and Pedagogy
- Music Theory and Analysis
- Musical Scores, Lyrics, and Libretti
- Musical Structures, Styles, and Techniques
- Musicology and Music History
- Performance Practice and Studies
- Race and Ethnicity in Music
- Sound Studies
- Browse content in Performing Arts
- Browse content in Philosophy
- Aesthetics and Philosophy of Art
- Epistemology
- Feminist Philosophy
- History of Western Philosophy
- Metaphysics
- Moral Philosophy
- Non-Western Philosophy
- Philosophy of Science
- Philosophy of Mind
- Philosophy of Perception
- Philosophy of Language
- Philosophy of Action
- Philosophy of Law
- Philosophy of Religion
- Philosophy of Mathematics and Logic
- Practical Ethics
- Social and Political Philosophy
- Browse content in Religion
- Biblical Studies
- Christianity
- East Asian Religions
- History of Religion
- Judaism and Jewish Studies
- Qumran Studies
- Religion and Education
- Religion and Health
- Religion and Politics
- Religion and Science
- Religion and Law
- Religion and Art, Literature, and Music
- Religious Studies
- Browse content in Society and Culture
- Cookery, Food, and Drink
- Cultural Studies
- Customs and Traditions
- Ethical Issues and Debates
- Hobbies, Games, Arts and Crafts
- Natural world, Country Life, and Pets
- Popular Beliefs and Controversial Knowledge
- Sports and Outdoor Recreation
- Technology and Society
- Travel and Holiday
- Visual Culture
- Browse content in Law
- Arbitration
- Browse content in Company and Commercial Law
- Commercial Law
- Company Law
- Browse content in Comparative Law
- Systems of Law
- Competition Law
- Browse content in Constitutional and Administrative Law
- Government Powers
- Judicial Review
- Local Government Law
- Military and Defence Law
- Parliamentary and Legislative Practice
- Construction Law
- Contract Law
- Browse content in Criminal Law
- Criminal Procedure
- Criminal Evidence Law
- Sentencing and Punishment
- Employment and Labour Law
- Environment and Energy Law
- Browse content in Financial Law
- Banking Law
- Insolvency Law
- History of Law
- Human Rights and Immigration
- Intellectual Property Law
- Browse content in International Law
- Private International Law and Conflict of Laws
- Public International Law
- IT and Communications Law
- Jurisprudence and Philosophy of Law
- Law and Politics
- Law and Society
- Browse content in Legal System and Practice
- Courts and Procedure
- Legal Skills and Practice
- Legal System - Costs and Funding
- Primary Sources of Law
- Regulation of Legal Profession
- Medical and Healthcare Law
- Browse content in Policing
- Criminal Investigation and Detection
- Police and Security Services
- Police Procedure and Law
- Police Regional Planning
- Browse content in Property Law
- Personal Property Law
- Restitution
- Study and Revision
- Terrorism and National Security Law
- Browse content in Trusts Law
- Wills and Probate or Succession
- Browse content in Medicine and Health
- Browse content in Allied Health Professions
- Arts Therapies
- Clinical Science
- Dietetics and Nutrition
- Occupational Therapy
- Operating Department Practice
- Physiotherapy
- Radiography
- Speech and Language Therapy
- Browse content in Anaesthetics
- General Anaesthesia
- Browse content in Clinical Medicine
- Acute Medicine
- Cardiovascular Medicine
- Clinical Genetics
- Clinical Pharmacology and Therapeutics
- Dermatology
- Endocrinology and Diabetes
- Gastroenterology
- Genito-urinary Medicine
- Geriatric Medicine
- Infectious Diseases
- Medical Toxicology
- Medical Oncology
- Pain Medicine
- Palliative Medicine
- Rehabilitation Medicine
- Respiratory Medicine and Pulmonology
- Rheumatology
- Sleep Medicine
- Sports and Exercise Medicine
- Clinical Neuroscience
- Community Medical Services
- Critical Care
- Emergency Medicine
- Forensic Medicine
- Haematology
- History of Medicine
- Browse content in Medical Dentistry
- Oral and Maxillofacial Surgery
- Paediatric Dentistry
- Restorative Dentistry and Orthodontics
- Surgical Dentistry
- Browse content in Medical Skills
- Clinical Skills
- Communication Skills
- Nursing Skills
- Surgical Skills
- Medical Ethics
- Medical Statistics and Methodology
- Browse content in Neurology
- Clinical Neurophysiology
- Neuropathology
- Nursing Studies
- Browse content in Obstetrics and Gynaecology
- Gynaecology
- Occupational Medicine
- Ophthalmology
- Otolaryngology (ENT)
- Browse content in Paediatrics
- Neonatology
- Browse content in Pathology
- Chemical Pathology
- Clinical Cytogenetics and Molecular Genetics
- Histopathology
- Medical Microbiology and Virology
- Patient Education and Information
- Browse content in Pharmacology
- Psychopharmacology
- Browse content in Popular Health
- Caring for Others
- Complementary and Alternative Medicine
- Self-help and Personal Development
- Browse content in Preclinical Medicine
- Cell Biology
- Molecular Biology and Genetics
- Reproduction, Growth and Development
- Primary Care
- Professional Development in Medicine
- Browse content in Psychiatry
- Addiction Medicine
- Child and Adolescent Psychiatry
- Forensic Psychiatry
- Learning Disabilities
- Old Age Psychiatry
- Psychotherapy
- Browse content in Public Health and Epidemiology
- Epidemiology
- Public Health
- Browse content in Radiology
- Clinical Radiology
- Interventional Radiology
- Nuclear Medicine
- Radiation Oncology
- Reproductive Medicine
- Browse content in Surgery
- Cardiothoracic Surgery
- Gastro-intestinal and Colorectal Surgery
- General Surgery
- Neurosurgery
- Paediatric Surgery
- Peri-operative Care
- Plastic and Reconstructive Surgery
- Surgical Oncology
- Transplant Surgery
- Trauma and Orthopaedic Surgery
- Vascular Surgery
- Browse content in Science and Mathematics
- Browse content in Biological Sciences
- Aquatic Biology
- Biochemistry
- Bioinformatics and Computational Biology
- Developmental Biology
- Ecology and Conservation
- Evolutionary Biology
- Genetics and Genomics
- Microbiology
- Molecular and Cell Biology
- Natural History
- Plant Sciences and Forestry
- Research Methods in Life Sciences
- Structural Biology
- Systems Biology
- Zoology and Animal Sciences
- Browse content in Chemistry
- Analytical Chemistry
- Computational Chemistry
- Crystallography
- Environmental Chemistry
- Industrial Chemistry
- Inorganic Chemistry
- Materials Chemistry
- Medicinal Chemistry
- Mineralogy and Gems
- Organic Chemistry
- Physical Chemistry
- Polymer Chemistry
- Study and Communication Skills in Chemistry
- Theoretical Chemistry
- Browse content in Computer Science
- Artificial Intelligence
- Computer Architecture and Logic Design
- Game Studies
- Human-Computer Interaction
- Mathematical Theory of Computation
- Programming Languages
- Software Engineering
- Systems Analysis and Design
- Virtual Reality
- Browse content in Computing
- Business Applications
- Computer Security
- Computer Games
- Computer Networking and Communications
- Digital Lifestyle
- Graphical and Digital Media Applications
- Operating Systems
- Browse content in Earth Sciences and Geography
- Atmospheric Sciences
- Environmental Geography
- Geology and the Lithosphere
- Maps and Map-making
- Meteorology and Climatology
- Oceanography and Hydrology
- Palaeontology
- Physical Geography and Topography
- Regional Geography
- Soil Science
- Urban Geography
- Browse content in Engineering and Technology
- Agriculture and Farming
- Biological Engineering
- Civil Engineering, Surveying, and Building
- Electronics and Communications Engineering
- Energy Technology
- Engineering (General)
- Environmental Science, Engineering, and Technology
- History of Engineering and Technology
- Mechanical Engineering and Materials
- Technology of Industrial Chemistry
- Transport Technology and Trades
- Browse content in Environmental Science
- Applied Ecology (Environmental Science)
- Conservation of the Environment (Environmental Science)
- Environmental Sustainability
- Environmentalist Thought and Ideology (Environmental Science)
- Management of Land and Natural Resources (Environmental Science)
- Natural Disasters (Environmental Science)
- Nuclear Issues (Environmental Science)
- Pollution and Threats to the Environment (Environmental Science)
- Social Impact of Environmental Issues (Environmental Science)
- History of Science and Technology
- Browse content in Materials Science
- Ceramics and Glasses
- Composite Materials
- Metals, Alloying, and Corrosion
- Nanotechnology
- Browse content in Mathematics
- Applied Mathematics
- Biomathematics and Statistics
- History of Mathematics
- Mathematical Education
- Mathematical Finance
- Mathematical Analysis
- Numerical and Computational Mathematics
- Probability and Statistics
- Pure Mathematics
- Browse content in Neuroscience
- Cognition and Behavioural Neuroscience
- Development of the Nervous System
- Disorders of the Nervous System
- History of Neuroscience
- Invertebrate Neurobiology
- Molecular and Cellular Systems
- Neuroendocrinology and Autonomic Nervous System
- Neuroscientific Techniques
- Sensory and Motor Systems
- Browse content in Physics
- Astronomy and Astrophysics
- Atomic, Molecular, and Optical Physics
- Biological and Medical Physics
- Classical Mechanics
- Computational Physics
- Condensed Matter Physics
- Electromagnetism, Optics, and Acoustics
- History of Physics
- Mathematical and Statistical Physics
- Measurement Science
- Nuclear Physics
- Particles and Fields
- Plasma Physics
- Quantum Physics
- Relativity and Gravitation
- Semiconductor and Mesoscopic Physics
- Browse content in Psychology
- Affective Sciences
- Clinical Psychology
- Cognitive Psychology
- Cognitive Neuroscience
- Criminal and Forensic Psychology
- Developmental Psychology
- Educational Psychology
- Evolutionary Psychology
- Health Psychology
- History and Systems in Psychology
- Music Psychology
- Neuropsychology
- Organizational Psychology
- Psychological Assessment and Testing
- Psychology of Human-Technology Interaction
- Psychology Professional Development and Training
- Research Methods in Psychology
- Social Psychology
- Browse content in Social Sciences
- Browse content in Anthropology
- Anthropology of Religion
- Human Evolution
- Medical Anthropology
- Physical Anthropology
- Regional Anthropology
- Social and Cultural Anthropology
- Theory and Practice of Anthropology
- Browse content in Business and Management
- Business Strategy
- Business Ethics
- Business History
- Business and Government
- Business and Technology
- Business and the Environment
- Comparative Management
- Corporate Governance
- Corporate Social Responsibility
- Entrepreneurship
- Health Management
- Human Resource Management
- Industrial and Employment Relations
- Industry Studies
- Information and Communication Technologies
- International Business
- Knowledge Management
- Management and Management Techniques
- Operations Management
- Organizational Theory and Behaviour
- Pensions and Pension Management
- Public and Nonprofit Management
- Social Issues in Business and Management
- Strategic Management
- Supply Chain Management
- Browse content in Criminology and Criminal Justice
- Criminal Justice
- Criminology
- Forms of Crime
- International and Comparative Criminology
- Youth Violence and Juvenile Justice
- Development Studies
- Browse content in Economics
- Agricultural, Environmental, and Natural Resource Economics
- Asian Economics
- Behavioural Finance
- Behavioural Economics and Neuroeconomics
- Econometrics and Mathematical Economics
- Economic Systems
- Economic History
- Economic Methodology
- Economic Development and Growth
- Financial Markets
- Financial Institutions and Services
- General Economics and Teaching
- Health, Education, and Welfare
- History of Economic Thought
- International Economics
- Labour and Demographic Economics
- Law and Economics
- Macroeconomics and Monetary Economics
- Microeconomics
- Public Economics
- Urban, Rural, and Regional Economics
- Welfare Economics
- Browse content in Education
- Adult Education and Continuous Learning
- Care and Counselling of Students
- Early Childhood and Elementary Education
- Educational Equipment and Technology
- Educational Strategies and Policy
- Higher and Further Education
- Organization and Management of Education
- Philosophy and Theory of Education
- Schools Studies
- Secondary Education
- Teaching of a Specific Subject
- Teaching of Specific Groups and Special Educational Needs
- Teaching Skills and Techniques
- Browse content in Environment
- Applied Ecology (Social Science)
- Climate Change
- Conservation of the Environment (Social Science)
- Environmentalist Thought and Ideology (Social Science)
- Management of Land and Natural Resources (Social Science)
- Natural Disasters (Environment)
- Pollution and Threats to the Environment (Social Science)
- Social Impact of Environmental Issues (Social Science)
- Sustainability
- Browse content in Human Geography
- Cultural Geography
- Economic Geography
- Political Geography
- Browse content in Interdisciplinary Studies
- Communication Studies
- Museums, Libraries, and Information Sciences
- Browse content in Politics
- African Politics
- Asian Politics
- Chinese Politics
- Comparative Politics
- Conflict Politics
- Elections and Electoral Studies
- Environmental Politics
- Ethnic Politics
- European Union
- Foreign Policy
- Gender and Politics
- Human Rights and Politics
- Indian Politics
- International Relations
- International Organization (Politics)
- Irish Politics
- Latin American Politics
- Middle Eastern Politics
- Political Sociology
- Political Methodology
- Political Communication
- Political Philosophy
- Political Behaviour
- Political Economy
- Political Institutions
- Political Theory
- Politics and Law
- Politics of Development
- Public Administration
- Public Policy
- Qualitative Political Methodology
- Quantitative Political Methodology
- Regional Political Studies
- Russian Politics
- Security Studies
- State and Local Government
- UK Politics
- US Politics
- Browse content in Regional and Area Studies
- African Studies
- Asian Studies
- East Asian Studies
- Japanese Studies
- Latin American Studies
- Middle Eastern Studies
- Native American Studies
- Scottish Studies
- Browse content in Research and Information
- Research Methods
- Browse content in Social Work
- Addictions and Substance Misuse
- Adoption and Fostering
- Care of the Elderly
- Child and Adolescent Social Work
- Couple and Family Social Work
- Direct Practice and Clinical Social Work
- Emergency Services
- Human Behaviour and the Social Environment
- International and Global Issues in Social Work
- Mental and Behavioural Health
- Social Justice and Human Rights
- Social Policy and Advocacy
- Social Work and Crime and Justice
- Social Work Macro Practice
- Social Work Practice Settings
- Social Work Research and Evidence-based Practice
- Welfare and Benefit Systems
- Browse content in Sociology
- Childhood Studies
- Community Development
- Comparative and Historical Sociology
- Disability Studies
- Economic Sociology
- Gender and Sexuality
- Gerontology and Ageing
- Health, Illness, and Medicine
- Marriage and the Family
- Migration Studies
- Occupations, Professions, and Work
- Organizations
- Population and Demography
- Race and Ethnicity
- Social Theory
- Social Movements and Social Change
- Social Research and Statistics
- Social Stratification, Inequality, and Mobility
- Sociology of Religion
- Sociology of Education
- Sport and Leisure
- Urban and Rural Studies
- Browse content in Warfare and Defence
- Defence Strategy, Planning, and Research
- Land Forces and Warfare
- Military Administration
- Military Life and Institutions
- Naval Forces and Warfare
- Other Warfare and Defence Issues
- Peace Studies and Conflict Resolution
- Weapons and Equipment

- < Previous chapter
- Next chapter >
13. Cross-sectional studies
- Published: April 1997
- Cite Icon Cite
- Permissions Icon Permissions
Cross-sectional studies involve identifying a defined population at a given point in time and measuring a range of variables on an individual basis which can include current and past dietary exposures and well as health outcomes and disease status. They are also known as prevalence surveys. They include a type of migrant study. This chapter considers the use and limitations of cross-sectional studies and their value in generating hypotheses. It provides details of their design (including a flow chart and a consideration of the choice of variables) and discusses the approaches to analysis.
Personal account
- Sign in with email/username & password
- Get email alerts
- Save searches
- Purchase content
- Activate your purchase/trial code
- Add your ORCID iD
Institutional access
Sign in with a library card.
- Sign in with username/password
- Recommend to your librarian
- Institutional account management
- Get help with access
Access to content on Oxford Academic is often provided through institutional subscriptions and purchases. If you are a member of an institution with an active account, you may be able to access content in one of the following ways:
IP based access
Typically, access is provided across an institutional network to a range of IP addresses. This authentication occurs automatically, and it is not possible to sign out of an IP authenticated account.
Choose this option to get remote access when outside your institution. Shibboleth/Open Athens technology is used to provide single sign-on between your institution’s website and Oxford Academic.
- Click Sign in through your institution.
- Select your institution from the list provided, which will take you to your institution's website to sign in.
- When on the institution site, please use the credentials provided by your institution. Do not use an Oxford Academic personal account.
- Following successful sign in, you will be returned to Oxford Academic.
If your institution is not listed or you cannot sign in to your institution’s website, please contact your librarian or administrator.
Enter your library card number to sign in. If you cannot sign in, please contact your librarian.
Society Members
Society member access to a journal is achieved in one of the following ways:
Sign in through society site
Many societies offer single sign-on between the society website and Oxford Academic. If you see ‘Sign in through society site’ in the sign in pane within a journal:
- Click Sign in through society site.
- When on the society site, please use the credentials provided by that society. Do not use an Oxford Academic personal account.
If you do not have a society account or have forgotten your username or password, please contact your society.
Sign in using a personal account
Some societies use Oxford Academic personal accounts to provide access to their members. See below.
A personal account can be used to get email alerts, save searches, purchase content, and activate subscriptions.
Some societies use Oxford Academic personal accounts to provide access to their members.
Viewing your signed in accounts
Click the account icon in the top right to:
- View your signed in personal account and access account management features.
- View the institutional accounts that are providing access.
Signed in but can't access content
Oxford Academic is home to a wide variety of products. The institutional subscription may not cover the content that you are trying to access. If you believe you should have access to that content, please contact your librarian.
For librarians and administrators, your personal account also provides access to institutional account management. Here you will find options to view and activate subscriptions, manage institutional settings and access options, access usage statistics, and more.
Our books are available by subscription or purchase to libraries and institutions.
Month: | Total Views: |
---|---|
October 2022 | 6 |
November 2022 | 12 |
December 2022 | 21 |
January 2023 | 4 |
February 2023 | 3 |
March 2023 | 5 |
April 2023 | 6 |
June 2023 | 8 |
July 2023 | 4 |
August 2023 | 7 |
October 2023 | 1 |
November 2023 | 5 |
December 2023 | 4 |
January 2024 | 5 |
February 2024 | 4 |
March 2024 | 2 |
April 2024 | 2 |
May 2024 | 6 |
June 2024 | 2 |
July 2024 | 3 |
August 2024 | 1 |
- About Oxford Academic
- Publish journals with us
- University press partners
- What we publish
- New features
- Open access
- Rights and permissions
- Accessibility
- Advertising
- Media enquiries
- Oxford University Press
- Oxford Languages
- University of Oxford
Oxford University Press is a department of the University of Oxford. It furthers the University's objective of excellence in research, scholarship, and education by publishing worldwide
- Copyright © 2024 Oxford University Press
- Cookie settings
- Cookie policy
- Privacy policy
- Legal notice
This Feature Is Available To Subscribers Only
Sign In or Create an Account
This PDF is available to Subscribers Only
For full access to this pdf, sign in to an existing account, or purchase an annual subscription.
Log in using your username and password
- Search More Search for this keyword Advanced search
- Latest content
- Current issue
- BMJ Journals
You are here
- Volume 20, Issue 1
- Observational research methods. Research design II: cohort, cross sectional, and case-control studies
- Article Text
- Article info
- Citation Tools
- Rapid Responses
- Article metrics

- Department of Accident and Emergency Medicine, Taunton and Somerset Hospital, Taunton, Somerset, UK
- Correspondence to: Dr C J Mann; tonygood{at}doctors.org.uk
Cohort, cross sectional, and case-control studies are collectively referred to as observational studies. Often these studies are the only practicable method of studying various problems, for example, studies of aetiology, instances where a randomised controlled trial might be unethical, or if the condition to be studied is rare. Cohort studies are used to study incidence, causes, and prognosis. Because they measure events in chronological order they can be used to distinguish between cause and effect. Cross sectional studies are used to determine prevalence. They are relatively quick and easy but do not permit distinction between cause and effect. Case controlled studies compare groups retrospectively. They seek to identify possible predictors of outcome and are useful for studying rare diseases or outcomes. They are often used to generate hypotheses that can then be studied via prospective cohort or other studies.
- research methods
- cohort study
- case-control study
- cross sectional study
https://doi.org/10.1136/emj.20.1.54
Statistics from Altmetric.com
Request permissions.
If you wish to reuse any or all of this article please use the link below which will take you to the Copyright Clearance Center’s RightsLink service. You will be able to get a quick price and instant permission to reuse the content in many different ways.
Read the full text or download the PDF:
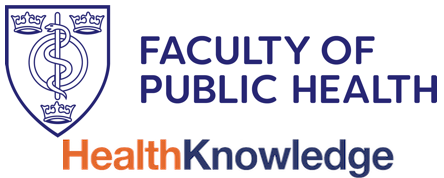
Design, applications, strengths and weaknesses of cross-sectional, analytical studies (including cohort, case-control and nested case-control studies), and intervention studies (including randomised controlled trials)
PLEASE NOTE:
We are currently in the process of updating this chapter and we appreciate your patience whilst this is being completed.
The prodominant study designs can be categorised into observational and interventional studies. Observational studies, such as cross-sectional, case control and cohort studies, do not actively allocate participants to receive a particular exposure, whilt interventional studies do. Each of the above study designs are described here in turn.
Cross-sectional Studies
In a cross-sectional study, data are collected on the whole study population at a single point in time to examine the relationship between disease (or other health-related outcomes) and other variables of interest (exposures).
Cross-sectional studies therefore provide a snapshot of the frequency of a disease or other health-related characteristics in a population at a given point in time. This methodology can be used to assess the burden of disease or health needs of a population, for example, and is therefore particularly useful in informing the planning and allocation of health resources.
Types of cross-sectional study
- Descriptive A cross-sectional study may be purely descriptive and used to assess the frequency and distribution of a particular disease in a defined population. For example, a random sample of schools across London may be used to assess the burden or prevalence of asthma among 12- to 14-year-olds.
- Analytical Analytical cross-sectional studies may also be used to investigate the association between a putative risk factor and a health outcome. However, this type of study is limited in its ability to draw valid conclusions about any association or possible causality because the presence of risk factors and outcomes are measured simultaneously. It is therefore not possible to confidently infer whether the disease or the exposure came first, so causation should always be confirmed by more rigorous studies. The collection of information about risk factors is also retrospective, running the risk of recall bias.
In practice cross-sectional studies often include an element of both types of design.
Issues in the design of cross-sectional surveys
- Choosing a representative sample A cross-sectional study should be representative of the whole of the population, if the findings are to have external validity. For example, a study of the prevalence of diabetes among women aged 40-60 years in Town A should comprise a random sample of all women aged 40-60 years in that town. If the study is to be representative, attempts should be made to include hard-to-reach groups, such as people in institutions or the homeless, to avoid selection bias.
- Sample size The sample size should be sufficiently large to estimate the prevalence of the conditions of interest with adequate precision. Sample size calculations can be carried out using sample size tables or statistical packages such as Epi Info . The larger the study, the less likely the results will be due to chance alone, but this will also have implications for cost.
- Data collection As data on exposures and outcomes are collected simultaneously, specific inclusion and exclusion criteria should be established at the design stage, to ensure that those with the outcome are correctly identified. The data collection methods will depend on the exposure, outcome and study setting, but include questionnaires and interviews, as well as medical examinations. Routine data sources may also be used.
Potential bias in cross-sectional studies
Non-response is a particular problem affecting cross-sectional studies and can result in bias of the measures of outcome. This is a particular problem when the characteristics of non-responders differ from responders. Recall bias can occur if the study asks participants about past exposures.
Analysis of cross-sectional studies
In a cross-sectional study all factors (exposure, outcome, and confounders) are measured simultaneously. The main outcome measure obtained from a cross-sectional study is prevalence:

For continuous variables such as blood pressure or weight, values will fall along a continuum within a given range. Prevalence may therefore only be calculated when the variable is divided into those values that fall below or above a particular pre-determined level. Alternatively, mean or median levels may be calculated.
In analytical cross-sectional studies the odds ratio can be used to assess the strength of an association between a risk factor and health outcome of interest, provided that the current exposure accurately reflects the past exposure.
Strengths and weaknesses of cross-sectional studies
- Relatively quick, cheap and easy to conduct (no long periods of follow-up).
- Data on all variables is only collected once.
- Able to measure prevalence for all factors under investigation.
- Multiple outcomes and exposures can be studied.
- The prevalence of disease or other health-related characteristics are important in public health for assessing the burden of disease in a specified population and in planning and allocating health resources.
- Good for descriptive analyses and for generating hypotheses.
- Difficult to determine whether the exposure or outcome came first (there may be reverse causality – see chapter 12 “ Association and Causation ” )
- Not suitable for studying rare diseases or diseases with a short duration.
- As cross-sectional studies measure prevalent rather than incident cases, the data will always reflect determinants of survival as well as aetiology. 1
- Unable to measure incidence.
- Associations identified may be difficult to interpret.
- Susceptible to biases such as responder bias, recall bias, interviewer bias and social acceptability bias.
Case Control Studies
In a case-control study the study group is defined by the outcome (e.g. presence of a disease), not by exposure to a risk factor. The study starts with the identification of a group of cases (individuals with a particular health outcome) in a given population and a group of controls (individuals without the health outcome) from the same population.
The prevalence of exposure to a potential risk factor is then compared between cases and controls. If the prevalence of exposure is more common among cases than controls, the exposure may be a risk factor for the outcome under investigation.
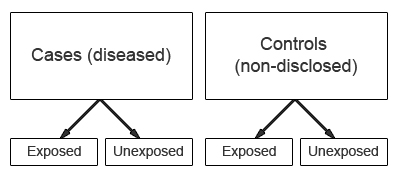
One of the advantages of case-control studies is that they can be used to study outcomes or diseases that are rare. However, a major characteristic is that data on potential risk factors are collected retrospectively and as a result may give rise to bias. This is a particular problem associated with case-control studies and therefore needs to be carefully considered during the design and conduct of the study.
Issues in the design of case-control studies
- Formulation of a clearly defined hypothesis As with all epidemiological investigations the beginning of a case-control study should begin with the formulation of a clearly defined hypothesis.
- Case definition It is essential that the case definition is clearly defined at the outset of the investigation to ensure that all cases included in the study are based on the same diagnostic criteria.
- Source of cases The source of cases needs to be clearly defined. It is important to ensure that cases selected for a study are representative of all cases of the disease in the population. Cases may be recruited from a number of sources; for example, they may be recruited from a hospital, clinic, GP registers or population registries. Population-based case-control studies are generally more expensive and difficult to conduct, but are also more representative of the spectrum of disease.
Incident cases comprise cases newly diagnosed during a defined time period. The use of incident cases is considered to be preferable because the recall of past exposure(s) may be more accurate amongst those who have been recently diagnosed with a condition. In addition, the temporal sequence of exposure and disease is easier to assess among incident cases.
Prevalent cases comprise individuals who have had the outcome under investigation for some time. Inclusion of prevalent cases may mean the results of the study are more generalisable to the wider population. However, it may also give rise to recall bias as prevalent cases may be less likely to accurately remember past exposures. As a result, the interpretation of results based on prevalent cases may prove more problematic as it may be more difficult to ensure that reported events (exposure) relate to a time before the development of disease rather than being a consequence of the disease process itself. For example, individuals may modify their exposure following the onset of disease. Another disadvantage of sampling prevalent cases is the risk of preferentially including the milder cases which have a better survival; these may not be representative of all disease cases, and may have different levels of the exposure(s) of interest.
The goal is to select individuals in whom the distribution of exposure status would be the same as that of the cases in the absence of an exposure-disease association. That is, if there is no true association between exposure and disease, the cases and controls should have the same distribution of exposure. To put it another way, controls should meet all the criteria for cases, apart from having the disease itself, so if the cases are women aged 50-70 years with breast cancer, the controls should be selected from a similar group who do not have breast cancer.
The source of controls is dependent on the source of cases. In order to minimise bias, controls should be selected to be a representative sample of the population which produced the cases. For example, if cases are selected from a defined population such as a GP register then controls should comprise a sample from the same GP register.
In case-control studies where cases are hospital based, it is common to recruit controls from the hospital population. However, the choice of controls from a hospital setting should not include individuals with an outcome related to the exposure being studied. For example in a case-control study of the association between smoking and lung cancer, the inclusion of controls being treated for a condition related to smoking (e.g. chronic bronchitis) may result in an underestimate of the strength of the association between the exposure (smoking) and outcome (lung cancer).
Recruiting more than one control per case may improve the statistical power of the study, especially where the number of cases is limited. However, there is little additional statistical power to be gained by recruiting more than 4 controls per case.
Note that in case-control studies the measurement of exposure is established after the development of disease and as a result is prone to both recall and observer bias.
Various methods can be used to ascertain exposure status. These include: ◦ Standardised questionnaires ◦ Biological samples ◦ Interviews with the subject ◦ Interviews with spouse or other family members ◦ Medical records ◦ Employment records ◦ Pharmacy records
The procedures used for the collection of exposure data should be the same for cases and controls.
Common sources of bias and confounding in case-control studies
Due to the retrospective nature of case-control studies they are particularly susceptible to the effects of bias which may be introduced as a result of a poor study design or during the collection of exposure and outcome data.
Because the disease and exposure have already occurred at the outset of a case-control study there may be differential reporting of exposure information between cases and controls based on their disease status. Cases and controls may recall past exposure differently, because knowledge of being a case may affect whether the individual remembers a certain exposure, for example (recall bias). Similarly, the recording of exposure information may vary depending on the investigator’s knowledge of an individual's disease status (interviewer/observer bias).
Selection bias can occur in case-control studies when the selected control group are not representative of the population from which the cases arose, thus comparisons of exposure distributions between cases and controls may give misleading results.
Temporal bias (also known as reverse causality) may also occur in case-control studies. When trying to establish a link between exposure and outcome, it must be clear that the exposure occurred well before the diagnosis of the disease of interest.
Therefore, the design and conduct of the study must be carefully considered as there are limited options for the control of bias during the analysis.
A confounder is a factor associated independently with both the exposure and outcome, and can be a problem where cases and controls differ with respect to a potential confounder. It can be dealt with at two stages:
- Design stage – when selecting controls, e.g. matching or restriction
- Analysis stage – statistical techniques including adjustment and multivariate techniques
More detail on adjusting for confounding can be found in the chapter “ Bias and confounding ” .
Analysis of case-control studies
The odds ratio (OR) is used in case-control studies to estimate the strength of the association between exposure and outcome. It is not possible to estimate the incidence or risk of disease from a case-control study, unless the study is population-based and all cases in a defined population are obtained.
The results of a case-control study can be presented in a 2x2 table as follows:
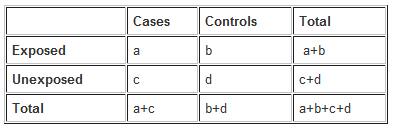
The odds ratio is a measure of the odds of exposure in the cases (= a/c), compared to the odds of exposure in the control group (= b/d). It is calculated as follows:
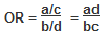
Example : Calculate the odds ratio from a hypothetical case-control study of smoking and pancreatic cancer among 100 cases and 400 controls, the results of which are shown below.
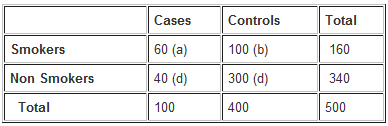
The OR calculated from the hypothetical data suggests that individuals with cancer of the pancreas (cases) are more likely to have smoked than those without the disease. Specifically, participants with pancreatic cancer have 4.5 times the odds of being smokers compared to those without pancreatic cancer.
NB: The odds ratio above has been calculated without adjusting for potential confounders. Further analysis of the data would involve stratifying by levels of potential confounders such as age. The 2x2 table can then be extended to allow stratum-specific rates of the confounding variables to be calculated and, where appropriate, an overall summary measure adjusted for the effects of confounding and a statistical test of significance. In addition, confidence intervals for the odds ratio would also be presented.
Strengths and weaknesses of case-control studies
- Cost-effective relative to other analytical studies such as cohort studies.
- Case-control studies are retrospective, cases are identified at the beginning of the study therefore there is no long follow-up period (compared to cohort studies).
- Efficient for the study of diseases with long latency periods.
- Efficient for the study of rare diseases.
- Good for examining multiple exposures simultaneously.
- Particularly prone to bias; especially selection, recall and observer bias.
- Case-control studies are limited to examining one outcome.
- Unable to estimate incidence rates of disease (unless study is population based).
- Poor choice for the study of rare exposures.
- The temporal sequence between exposure and disease may be difficult to determine.
Nested Case-control Studies
A nested case-control study is one where the cases and controls are selected from individuals within an established cohort study. The case-control study is thus said to be ‘nested’ within the cohort study.
Cases of a disease that arise within the defined cohort during the follow up period are identified, then a specified number of matched controls who have not developed the disease are selected from the same cohort. The main advantage of nested case-control studies is that certain exposure data will already have been collected for both cases and controls which limits the potential for recall bias.
Analysis is carried out in the same way as for normal case-control studies, with the calculation of odds ratios.
Strengths of nested case-control studies
- Relatively cheap and easy to conduct.
- Data related to exposure and confounding have often already been collected.
- Can utilise the baseline data on exposure and confounding collected before the onset of disease, which reduces the potential for recall bias and uncertainty regarding the temporal sequence between exposure and disease onset.
Weaknesses of nested case-control studies
- Causal inference is still limited.
- Not all relevant risk factor / exposure data may have been recorded.
- Data may not have been recorded in the same way, or with the same accuracy and consistency, over time.
- Recall bias may persist if some data are collected retrospectively.
- Non-diseased persons from whom the controls are selected may not be fully representative of the original cohort due to loss to follow-up or death.
Examples of case-control studies 2
Case-control studies have been used in a variety of situations to evaluate possible causes of rare conditions. Classic examples include the investigation of cases of childhood leukaemia near the nuclear procession plant at Sellafield in Cumbria (UK), as well as cases of vaginal adenocarcinoma, which is normally rare, but were seen in higher numbers than usual in the USA in the 1970s. The difference between the young women with vaginal adenocarcinoma and their comparison group was that the mothers of cases had taken stilboestrol during the pregnancy (to prevent miscarriage), but the mothers of the controls had not.
- Gardner M J et al. Methods and basic data of case-control study of leukaemia and lymphoma among young people near Sellafield nuclear plant in West Cumbria. BMJ 1990; 300(6722):429-34
- Gardner M J et al. Results of case-control study of leukaemia and lymphoma among young people near Sellafield nuclear plant in West Cumbria. BMJ 1990; 300(6722): 423-9
- Herbst A, Ulfelder H, Poskanzer D. Adenocarcinoma of the vagina. Association of maternal stilbestrol therapy with tumor appearance in young women. NEJM 1971; 284(15): 878-81
Cohort Studies
Cohort studies evaluate a possible association between exposure and outcome by following a group of exposed individuals over a period of time (often years) to see whether they develop the disease or outcome of interest. A cohort is a group of individuals who share a common characteristic, and may be chosen based on a population definition, or based on a particular exposure (see “ Selection of study groups” , below). The incidence of disease in the exposed individuals of the cohort is then compared to the incidence of disease in unexposed, or lowest risk, individuals, and a relative risk (incidence risk or incidence rate) is calculated to assess whether the exposure and disease are associated.
Cohort studies may be prospective or retrospective, but both types define the cohort on the basis of exposure, not outcome.
Prospective cohort studies – participants are identified and followed up over time until the outcome of interest has occurred, or the time limit for the study has been reached. A temporal relationship between exposure and outcome can thus be established.
Retrospective cohort studies – exposure and outcome have already occurred at the start of the study. Pre-existing data, such as medical notes, can be used to assess any causal links, so lengthy follow-up is not required. This type of cohort study is therefore less time consuming and costly, but it is also more susceptible to the effects of bias. For example, the exposure may have occurred some years previously and adequate, reliable data on exposures may be differentially recorded in eventual cases compared to controls. In addition, information on confounding variables may be unavailable, inadequate or difficult to collect.
Issues in the design of cohort studies
If the exposure is common, a defined study population can be selected for longitudinal assessment before classifying individuals as exposed or unexposed (population-based cohort study). This could involve, for example, a selection of the general population (e.g. defined by a geographical boundary) or all individuals who share a common workplace (occupational cohort).
If the exposure is rare, individuals may be chosen on the basis of exposure, to ensure sufficient exposed persons are enrolled. For example, this may be workers at a particular factory who regularly handle a chemical of interest. The comparison group might be workers at the same factory whose roles do not bring them into contact with the chemical. If a control group is chosen from the general population, there is a risk of bias due to the ‘healthy worker effect’: the general population is usually less healthy than the workforce because it includes those unable to work due to illness. 3
A particular problem within cohort studies is determining whether individuals in the control group are truly unexposed. For example, study participants may start smoking after enrolment, or they may fail to correctly recall past exposure. Similarly, those in the exposed group may change their behaviour in relation to the exposure such as diet, smoking or alcohol consumption. The ability to repeatedly measure exposures over time, and to account for these, helps mitigate against such changes in behavior.
Exposure data may be obtained from a number of sources, including medical or employment records, standardised questionnaires, interviews and by physical examination.
- Measuring outcome Outcome measures may be obtained from various sources including routine surveillance of cancer registry data, death certificates, medical records or directly from the participant. Note that the method used to ascertain outcome must be identical for both exposed and unexposed groups to avoid the risk of measurement bias. Information on outcomes should be collected by members of the study team who are blind to participants’ exposure status, to reduce the risk of observer bias.
- Methods of follow-up The follow-up of participants in a cohort study is a major challenge, and it may take many years for a sufficient proportion of participants to have developed the outcome. A great deal of cost and time is required to ensure adequate follow-up of cohort members and to update measures of exposures and confounders, as well as monitoring participants’ health outcomes. Failure to collect outcome data for all members of the cohort will affect the validity of the study results.
Potential sources of bias in cohort studies
A major source of potential bias in cohort studies is losses to follow-up. Cohort members may die, refuse to continue participation in the study or fail to maintain contact. Such events may be related to the exposure, outcome or both, resulting in loss to follow-up bias. For example, individuals who develop a precursor to the outcome, such as symptoms of angina where the outcome of interest is a heart attack, may be less likely to continue to participate in the study. The degree to which losses to follow-up are correlated with either exposure or outcome can lead to significant bias in the measurement of the relationship between the exposure and outcome. 1
Another source of potential bias in cohort studies arises from the degree of accuracy with which subjects have been classified with respect to their exposure or disease status. Differential misclassification – when one group of participants is more likely to have been misclassified than the other – can lead to an over- or underestimation of the relationship between the exposure and outcome. 1
Analysis of cohort studies
The analysis of a cohort study uses the ratio of either the risk or rate of disease in the exposed cohort, compared with the risk or rate in the unexposed cohort.
If follow-up times differ markedly between participants, a rate may be more appropriate. The risk ratio uses as a denominator the entire group recruited at the start of the study while the rate ratio uses as a denominator the person-years which accounts for different lengths of time spent in the study. Different lengths of time spent in a study are due to varying start points, and incorporate multiple possible end points (i.e. one is no longer in the study on developing the outcome, or following death or moving away).
Calculation of the rate ratio from a hypothetical cohort study of smoking and pancreatic cancer followed-up for 1 year.
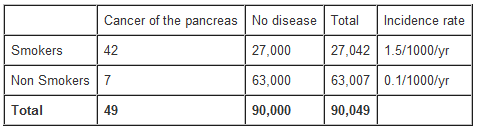
From the data in the table above, the rate ratio and attributable risk can be calculated as follows:
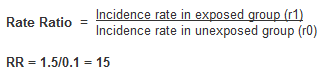
The rate ratio of 15 indicates that the risk of developing pancreatic cancer over 1 year is 15 times higher among smokers than non-smokers.
Attributable risk (AR)
AR = incidence rate among exposed (r1) – incidence rate among unexposed (r0)
AR = 1.5 – 0.1 = 1.4 /1000/yr
The attributable risk of pancreatic cancer due to smoking is 1.4 cases per 1000 per year
Attributable risk percentage (ARP), which gives the proportion of cases attributable (and thus avoidable) to an exposure in relation to all cases, is calculated as:

This can be interpreted as follows: smoking accounts for 93% of all cases of pancreatic cancer among smokers.
Standardised mortality and morbidity ratios (SMR) are another commonly used method of presenting results in a cohort study. See chapter “ Numerators, denominators and populations at risk ” for further information on SMR.
Strengths and weaknesses of cohort studies
- Multiple outcomes can be measured for any one exposure.
- Can look at multiple exposures.
- Exposure is measured before the onset of disease (in prospective cohort studies) i.e. the temporal relationship is certain.
- Demonstrates direction of causality.
- Minimises selection bias (in prospective cohort studies)
- Good for measuring rare exposures, for example among different occupations.
- Good for outcomes which occur long after exposure (in retrospective cohort studies).
- Can measure incidence and prevalence.
- Costly and time consuming.
- Prone to bias due to loss to follow-up.
- Knowledge of exposure status may bias classification of the outcome.
- Being in the study may alter participant behaviour.
- Inefficient for the study of a rare disease outcome.
- Classification of individuals (exposure or outcome status) can be affected by changes in diagnostic procedures.
- Cannot go back in time to collect information about exposure or confounders that were overlooked at the start of the study.
Examples of cohort studies 2
One of the most famous examples of a cohort study is Sir Richard Doll’s study of the hazards of cigarette smoking in a cohort of nearly 35,000 British doctors. Baseline information about their smoking habits was obtained in 1951, and periodically thereafter. Cause-specific mortality was then monitored for 50 years and the results showed an excess mortality associated with smoking, chiefly due to vascular, neoplastic, and respiratory diseases.
Other cohorts include the Framingham cardiovascular studies, following people living in the town of Framingham, Massachusetts. Much of our current knowledge about heart disease, such as the effects of diet, exercise, and common medications such as aspirin, is based on this longitudinal cohort study. In the UK, the Whitehall studies have followed cohorts of British civil servants, demonstrating how groups in the cohort with differing levels of a characteristic, such as cholesterol, subsequently have different rates of ischaemic heart disease.
Cohort studies are also useful to study the longer-term effects of an unusual event. The most famous example of this is the cohort of people who survived the atomic bomb explosions at Hiroshima and Nagasaki.
- Doll R et al. Mortality in relation to smoking: 50 years' observations on male British doctors. BMJ 2004; 328 (7455): 1519
- E G Rael et al. Sickness absence in the Whitehall II study, London: the role of social support and material problems. Journal of Epidemiology and Community Health 1995:49 (5): 474-81
Intervention Studies (including Randomised Controlled Trials) Intervention studies are designed to evaluate the effect of a specific treatment or practice and are considered to provide the most reliable evidence in epidemiological research.
Intervention studies can generally be considered as either preventative or therapeutic, and types of experimental intervention include: 1
- Therapeutic agents
- Prophylactic agents
- Surgical procedures
- Health service strategies
Therapeutic trials are designed to evaluate the effect of therapies, such as new drugs or surgical procedures. They may also be known as clinical trials, and are conducted among individuals with a particular disease to assess the effectiveness of an agent or procedure in achieving a specific outcome, such as reduced mortality. 1
Preventative trials are designed to evaluate whether an agent or procedure reduces the risk of developing a particular disease. They are carried out on individuals free from the disease at the beginning of the trial, but deemed to be at risk. 1 Preventative trials may be conducted among individuals or entire communities, and examples include evaluations of new vaccines or bed nets to prevent infection with malaria.
Characteristics of an intervention study
- The intervention being tested is allocated to a group of two or more study subjects (individuals, households, communities).
- Subjects are followed prospectively to compare the intervention vs . the control (standard treatment, no treatment or placebo).
The main intervention study design is the randomised controlled trial (RCT).
Randomised controlled trials
The randomised controlled trial is considered the most rigorous method of determining whether a cause-effect relationship exists between an intervention and outcome. 4 The strength of the RCT lies in the process of randomisation which is unique to this type of study design.
In an RCT, study participants are generally randomly assigned to one of two groups, the experimental group who will receive the intervention being tested, and a comparison group (controls) who receive a conventional treatment or placebo. 4 These groups are then followed prospectively to assess the effectiveness of the intervention compared with the standard or placebo treatment.
Subjects are randomly allocated to the two study arms so that the intervention and control groups are as similar as possible in all respects, apart from the treatment. Potential confounding factors should be equally distributed between the two groups. The choice of comparison treatments may include an existing standard treatment, no treatment, an alternative treatment or a placebo. A placebo is a substance that resembles the intervention treatment in all respects except that it contains no active ingredients.
Figure 1. General outline of a two-arm randomised controlled trial.
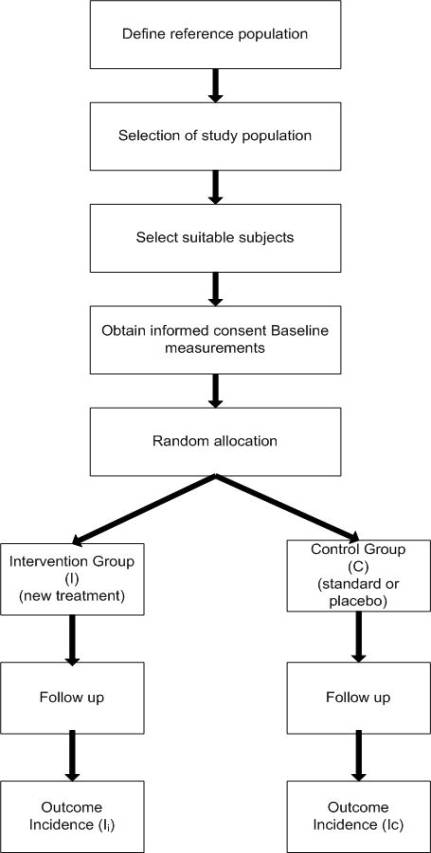
Basic outline of the design of a randomised controlled trial
Prior to the conduct of an RCT, a comprehensive study protocol should be developed and registered with a trials database, such as ClinicalTrials.gov . The study protocol will include information on:
- Aim and rationale of the trial
- Definition of the hypothesis
- Background/review of published literature
- Treatment schedules, dosage, toxicity data, etc.
- Ethical considerations
- Quality assurance and safety
- Formulation of hypothesis
- Objectives of the trial
- Sample size calculations
- Definition of the reference population
- Choice of a comparison treatment - placebo or current available best treatment
- Selection of intervention and control groups including: source, inclusion and exclusion criteria and methods of recruitment
- Informed consent procedures
- Choice of baseline measurements - include all variables considered or known to affect the outcome(s) of interest
- Method of random allocation of study participants to defined treatment groups (intervention vs. control)
- Follow-up of all treatment groups and assessment of outcomes
- Monitoring compliance and losses to follow-up
- Interim and final analysis plans
- Publication plans
The gold standard of intervention studies is the randomised, double-blind placebo-controlled trial. This attempts to reduce bias in the following ways: 3
- Selection bias – reduced by random allocation to treatment-arms, so each participant has an equal chance of receiving the intervention.
- Blinding – reduces measurement bias because both the investigators and the participants (hence ‘double-blind’) are unaware of the treatment allocations, so this cannot affect how they are assessed.
- Randomisation also ensures that the control group is as similar to the intervention group as possible, i.e. that confounders are equally distributed between the groups.
Randomisation
The aim of randomisation is to ensure that any observed differences between the study groups are due to differences in the treatment alone and not due to the effects of confounding or bias. Randomisation ensures that trial groups are similar at the outset in all respects with the exception of the intervention under investigation (i.e. they are balanced ). Note that there still may be differences in how the groups are handled as the trial progresses (introducing performance bias).
Methods of random allocation are used to ensure that all study participants have the same chance of allocation to the treatment or control group, and that the likelihood of receiving an intervention is equal regardless of when the participant entered the study. Therefore, the probability of any participant receiving the intervention should be independent of any other participant being assigned that treatment.
The assignment of study subjects to each intervention is determined by formal chance processes and cannot be predicted or influenced by the investigator or participant. In a well-designed RCT, the random allocation sequence is pre-determined and cannot be influenced. It is important that those responsible for recruiting participants into a study are unaware which study arm the individual will be allocated to. Allocation concealment avoids both conscious and unconscious selection of patients into the study, and attempts to ensure that the investigators cannot manipulate the trial by influencing which arm participants are enrolled into. One method of achieving this is by concealing allocation details in sealed, opaque envelopes. An alternative (and better) method is central randomisation by telephone, where the clinician calls a randomisation service to get the treatment allocation. The latter allocation mechanism is beyond the control of both the investigator and the participant, limiting potential bias in allocating the treatment. The process should be carried out once the participant has been determined to be eligible for inclusion and after they have given informed consent to participate.
Methods of treatment allocation
- Systematic allocation Participants can be allocated to study groups alternately, or on alternate days (“alternation”). Another method would be allocation on the basis of date of birth (“quasi-alternation”). These methods are not considered to be truly random and can result in selection bias. It may be possible for the investigator to manipulate the process, if they favour one study arm over another.
- Simple randomisation For example, using computer generated random number sequences (i.e. odd and even numbers can be used to allocate to one of two trial arms) or tossing a coin. Simple randomisation can suffer from chance bias – where the resulting groups are not balanced in important potential confounders by chance.
- Block randomisation Block randomisation is a method used to ensure that the numbers of participants assigned to each group is equally distributed and is commonly used in smaller trials. Participants are frequently allocated in blocks of 4, for example, so it is guaranteed that 2 in each block will be randomised to each study group. Other block sizes can be used, and block sizes can be varied within a trial. With blocks of 4, there are only six possible sequences to allocate two to group A and two to group B (AABB, ABAB, BABA, etc …) and blocks can be chosen at random to generate the allocation sequence. Large block sizes should be avoided, as this increases the chances of ending up with unequal group sizes. For example, a block size of 16 could have the sequence AAABAABAAABBBBBB. If the study was terminated part-way through this block, there would be a clear imbalance in the group sizes in favour of group A. Investigators should not know the block size, to prevent them from predicting allocation for the last few participants in each block.
- Stratified randomisation Stratified randomisation is used to ensure that potential confounding factors thought to be associated with the outcome are evenly distributed between groups. 2 Prior to randomisation, participants are separated into different subgroups or strata based on key risk factors that may influence outcome, for example sex or age. Individuals are then randomly allocated to each treatment arm from within the strata. This method of treatment allocation should be based on block randomisation within each stratum, to ensure there are similar numbers of participants in each arm. However, there are a limited number of baseline variables that can be balanced by stratification because of the potential for small numbers of subjects within each stratum.
- Minimisation Although technically not a random method, minimisation may be used in small trials where other methods of randomisation will not result in balanced groups. The treatment allocated to the next participant being enrolled in the trial depends on the characteristics and allocation of the participants already enrolled. The aim is that important potential confounding factors are distributed across the groups as equally as possible.
Once allocation is complete, the success of the randomisation process should be confirmed by comparing baseline factors between the two groups, to ensure that they are similar.
Advantages of randomisation
- Eliminates confounding - tends to create groups that are comparable for all factors that may influence outcome (known, unknown or difficult to measure). Therefore, the only difference between the groups should be the intervention.
- Eliminates treatment selection bias.
- Gives validity in statistical tests based on probability theory.
- Any baseline differences that exist between study groups are attributable to chance rather than bias - though this should still be considered as a potential concern.
Disadvantages of randomisation
- Does not guarantee comparable groups as differences in confounding variables may arise by chance.
Blinding in randomised controlled trials
Blinding is used in RCTs to ensure that there are no differences in the way the study arms are assessed or managed, thus minimising bias. Bias may be introduced, for example, if the investigator is aware which treatment a subject is receiving, as this may influence (intentionally or unintentionally) the way in which they measure or interpret the outcome data. Similarly, a subject's knowledge of treatment assignment may influence their response to a specific treatment.
Blinding also involves ensuring that the intervention and standard/placebo treatment appears the same. However, blinding is not always possible, for example when the treatment involved physiotherapy exercises, a weight loss programme or counselling.
In a double-blind trial, neither the investigator nor the study participant are aware of treatment assignments. However, this design is not always feasible and a single-blind RCT is where the investigator, but not the study participant, knows which treatment has been allocated.
Variations of the randomised control trial
- Crossover trials In a crossover trial each subject acts as their own control, and receives all the treatments under investigation in sequence. Random allocation determines the sequence in which each participant receives the treatments. This type of trial may be used when the intervention does not have long-term effects. A particular advantage of a crossover trial is that the groups are as similar as possible. In crossover trials it is common to have a 'washout period' where no treatment is given between each intervention to avoid the possibility of a residual effect from the previous treatment.
- Factorial trials A factorial trial is where two or more interventions are evaluated singly and simultaneously compared with a control group in the same trial. For example, where two interventions are being tested (A and B) along with a control, this would result in four groups: control, A only, B only, and both A and B. This type of RCT is commonly used to evaluate interactions between interventions.
- Cluster-randomised controlled trials Cluster randomised trials involve groups of individuals or communities, as opposed to individuals. Groups or communities are randomised to receive the intervention or standard/no treatment. This type of study design may be used to evaluate preventative health services such as smoking cessation programmes. For example, all patients attending a single general practice may be allocated to receive the new programme, whilst those at another practice will receive standard care. In this sort of trial, intra-cluster correlation needs to be taken into account in assessing effect size and sample size (see chapter “ Clustered data ”).
Ethical issues
The use of RCTs raises important ethical issues. For example, there must be sufficient doubt about the particular agent being tested to allow withholding of it from half the subjects, and at the same time there must be sufficient belief in the agent's potential to justify exposing the remaining half of all willing and eligible participants. 1 This is known as clinical equipoise .
In addition, there must be sufficient belief that the intervention under investigation is safe.
Informed consent is essential in RCTs (as it is in other study designs). Study subjects must understand that they are participating in an experiment and that in a placebo-controlled trial they may receive an inactive product. In addition, participants must be informed of the aims, methods and potential benefits or hazards of participating in the trial.
In a controlled trial careful consideration should also be given to what intervention is given to the control group. For example, if an effective treatment already exists, participants in the control group should not receive a placebo, depriving them of treatment. Any comparison should therefore be between the current standard treatment and the new treatment.
It is essential that study participants do not suffer as a consequence of a RCT. Most RCTs incorporate a data monitoring committee who are independent of the investigators, whose function is to review safety and efficacy data, and to ensure quality and compliance.
Analysis of RCTs
The analysis of RCT data is focused on estimating the size of the difference in predefined outcomes between the intervention and control groups. The main measure of effect obtained is the rate or risk ratio.
For trials of preventative interventions, the protective efficacy (or effectiveness) is calculated as:

(where R is the risk or rate)
Intention-to-treat analysis
When RCTs are analysed using an intention-to-treat analysis (ITT), participants’ results are analysed in the group to which they were originally assigned. 5 This should happen regardless of whether they were lost to follow-up, or whether they switched treatment groups during the trial. During the RCT subjects may refuse to continue to participate and stop taking their allocated treatment.
If the investigators exclude participants from the analysis where they have not adhered to their allocated treatment strategy, the estimate of the effect of the treatment is likely to be flawed. The aim of the intention-to-treat analysis is to provide a pragmatic (real-world) estimate of the benefit of the treatment under investigation, rather than of its potential benefit in patients who receive treatment exactly as planned. Subjects who failed to take their allocated treatment may have done so because of adverse side effects, or because they felt it was not working, and - in the real world - patients do not always take their medication as prescribed.
Additionally, there is evidence to suggest that those participants who are fully compliant with their prescribed medication do better than those who do not adhere, even after adjustment for all known prognostic factors and irrespective of assignment to active treatment or placebo.
Excluding non-compliant participants from the analysis leaves those who may be destined to have a better outcome, and destroys the unbiased comparison afforded by randomisation. 6 Where a comparison is performed using only those participants who completed the treatment originally allocated, this is known as a per protocol analysis.
Where full outcome data is not known for all subjects, then researchers can either impute an outcome for each individual lost based on shared characteristics with other individuals in the study, or use the last recorded measure.
In summary, during the statistical analysis of an RCT, all study subjects should ideally be retained in the group to which they were originally allocated regardless of whether or not that was the treatment received.
Strengths of randomised controlled trials
A well designed RCT provides the strongest epidemiological evidence of any study design about the effectiveness and safety of a given intervention.
- An RCT is considered to be the best type of epidemiological study from which to draw conclusions about causality.
- Randomisation is a powerful tool for controlling for confounding, even for factors that may be unknown or difficult to measure. If a study is designed and conducted rigorously, the possibility that an observed association is due to confounding should be minimal.
- Provides a strong basis for statistical inference.
- Enables blinding and therefore minimises bias.
- Can measure disease incidence and multiple outcomes.
Weaknesses of randomised controlled trials
- Ethical constraints.
- Expensive and time consuming.
- Requires complex design and analysis if unit of allocation is not the individual.
- Inefficient for rare diseases or diseases with a delayed outcome.
- There may be a placebo effect in the non-intervention group, which may result in an underestimation of the true treatment effect.
- Short follow up periods; not good at assessing long-term outcomes or harms.
- Generalisability: subjects in an RCT may be more willing to comply with the treatment regimen and therefore may not be representative of all individuals in a population eligible for the treatment.
Examples of RCTs
One of the first randomised trials to be carried out was the evaluation of streptomycin in the treatment of tuberculosis, published in 1948. Since then, there have been numerous other examples which have had a significant impact on clinical practice.
A non-randomised study published in 1980 evaluated the possible benefit of vitamin supplementation at the time of conception in women at high risk of having a baby with a neural tube defect. The investigators found that the vitamin group subsequently had fewer babies with neural tube defects than the placebo control group. The control group included women ineligible for the trial as well as women who refused to participate. As a consequence, the findings were not widely accepted, and the Medical Research Council later funded a large RCT to answer the question in a way that would be widely accepted. 7
- Streptomycin treatment of pulmonary tuberculosis: a Medical Research Council investigation . BMJ 1948;2:769-782.
- MRC Vitamin Study Research Group. Prevention of neural tube defects: results of the Medical Research Council vitamin study . Lancet 1991; 338: 131-137
- Hennekens CH, Buring JE. Epidemiology in Medicine, Lippincott Williams & Wilkins, 1987.
- http://www.edmundjessop.org.uk/fulltext.doc - Accessed 20/12/16
- Carneiro I, Howard N. Introduction to Epidemiology. Open University Press, 2011.
- Kendall JM. Designing a research project: Randomised Controlled trials and their principles,Emerg Med J. 2003, March;20(2)164-168.
- Hollis S, Campbell F, What is meant by intention to treat analysis? Survey of publishedrandomised controlled trials. BMJ 1999; 319;670-74.
- Montori V, Guyatt G. Intention-to-treat principle. CMAJ 2001; 165 (10)
- Altman D, Bland M. Treatment allocation in controlled trials: why randomise? BMJ 1999;318:1209-1209
Further Resources
- Pocock SJ. Clinical Trials: A practical approach, Chichester, Wiley, 1984.
- Sibbald B, Roland M, Understanding controlled trials: Why are randomised controlled trials important?, BMJ 1998, 316:201.
- Altman DG, Randomisation. BMJ 1991;302;1481-2.
© Helen Barratt, Maria Kirwan 2009, Saran Shantikumar 2018
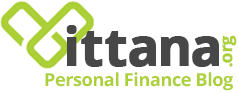
19 Advantages and Disadvantages of Cross Sectional Studies
A cross-sectional study involves the review of information from a population demographic at a specific point in time. The participants who get involved with this research are selected based on particular variables that researchers want to study. It is often used in developmental psychology, but this method is also useful in several other areas. Social sciences and educational processes benefit from this work.
Researchers who are following cross-sectional study techniques would study select groups of people in different age demographics. Their work would look at one investigatory point at a time. By taking this approach, any differences that exist between the demographics would be attributed to characteristics instead of something that happens.
These studies are observational in nature. They are sometimes described as descriptive research, but not causal or relational. That means researchers are unable to determine the cause of something, such as an illness, when using this method.
Several advantages and disadvantages are worth considering when looking at cross-sectional studies.
List of the Advantages of Cross-Sectional Studies
1. This study takes place during a specific moment in time. A cross-sectional study has defined characteristics that limit the size and scope of the work. Researchers look at specific relationships that happened during a particular moment in time. That means there are fewer risks to manage if tangents begin to develop in the data. The goal is to look for a meaningful result within an expected boundary.
2. No variable manipulation occurs with a cross-sectional study. Researchers directly observe the variables under study when using the cross-sectional technique. There is no reason to manipulate the environment because this is not an experimental technique. The data gathering process goes quickly because everything occurs within the scope of the research method. This advantage reduces the risk of having bias creep into the information being gathered.
3. It is an affordable way to conduct research. A cross-sectional study is much more affordable to complete when compared to the other options that are available to researchers today. No follow up work is necessary when taking this approach because once the information gets collected from the entire participant group, it can be analyzed immediately. This advantage as possible because only a single time reference is under consideration.
This approach allows for usable data to become available without the risk of a significant initial investment. Most of the data points collected using this method come from self-report surveys. Researchers can then collect a significant amount of information from a large pool of participants without a major time investment.
4. This study method provides excellent controls over the measurement process. Cross-sectional studies are only as good as the measurement processes that researchers used to collect data. Because there aren’t any long-term considerations involved with this specific approach, researchers have more control over the information acquisition process. Everything obtained during this work is quickly and easily measured and applied to the targeted demographics because the controls involved are straightforward to implement.
5. Researchers can look at several useful characteristics at once. Many researchers prefer the cross-sectional studies method because it allows them to look at numerous characteristics simultaneously. Instead of focusing on income, gender, age, or other separating factors, this method looks at each participant as an entire individual. That makes it possible for the work to include several useful characteristics that can each benefit from changing variables instead of using only one to determine an outcome.
This advantage is the reason why researchers often use cross-sectional studies to look at the prevailing characteristics in a given population. It is a process that lets different variables become the foundation of new correlations.
6. It provides relevant information in real-time updates. Cross-sectional studies provide us with a snapshot of a specific group of people at a particular point in time. Unlike other methods of research that look at demographics over an extended period, we use this information to look at what is happening in the present. That means the data researchers collect from this process is immediately relevant, giving us an opportunity to create real-time updates within specific population groups.
This process is how we can determine if there are specific risk factors that correlate to particular outcomes wit in that group. A cross-sectional study might look at a person’s past smoking and chewing habits to determine if there is a correlation with a recent lung cancer diagnosis. Although it won’t provide a cause-and-effect explanation, it does offer a fast look at potential correlations.
7. Cross-sectional studies miss fewer data points. The processes involved with cross-sectional studies reduce the risk of missing critical data points. Researchers have the ability to maximize their examination of the available information at any time because there are no time variables included in this work. That means a lower error rate typically occurs when using this method compared to the other approaches that are available to the scientific community.
8. It allows anyone to look at the data to determine a possible conclusion. The information that cross-sectional studies obtain is always suitable for secondary analysis. This advantage means that researchers can collect information for one set of purposes, and then use it to explore different variables that might exist in that specific demographic at the same time. That means an investment in this work can provide ongoing usefulness because it always applies to the people involved during that specific time. It is one of the easiest ways to maximize your research investment value.
9. Cross-sectional studies offer information that’s well-suited for descriptive analysis. If researchers want to develop a general hypothesis, then cross-sectional studies are the best way to generate specific situations that face a particular demographic. Each description of the critical data points creates the possibility of forwarding movement toward a future solution that may not have been considered previously.
Although this benefit doesn’t apply to causal relationships with this research method, the information collected from cross-sectional studies is a useful forward push toward additional research.
10. The focus of a cross-sectional study is to prove or disprove an assumption. A cross-sectional study is a research tool that is useful across various industries. The reason why it is such a generalized process that anyone can initiate is that the purpose of the work is to prove or disapprove and assumption or theory. Although health-related work tends to be the most popular industry that takes advantage of this approach, retail, education, social science, religion, and government industries can also benefit from this process.
The research that occurs allows each industry to learn more about the various demographics for the purpose of analyzing a target market. It creates data that’s useful when trying to determine what products or services to sell, or when it is necessary to look for specific patient outcomes.
List of the Disadvantages of Cross-Sectional Studies
1. It requires the entire population to be studied to create useful data. A correctly structured cross-sectional study must be representative of an entire demographic for it to provide useful information. If this representation is not possible, then the data collected from the participating individuals will have a built-in error rate that must come under consideration.
That’s why a complete generalization is not possible when using this approach. Environmental conditions, a person’s education, and several other factors can all change an individual’s perspective.
2. A researcher’s personal bias can influence the data from cross-sectional studies. Everyone has particular biases that influence their personality and general perspective on life. Many of these circumstances come from the conditioning that happens over the course of time. Even people who work hard to avoid showing bias in any situation can come under the influence of this disadvantage of cross-sectional studies.
Some demographics might include prison populations, the homeless, or people who are unable to leave their homes. If a researcher feels uncomfortable contacting individuals in these groups, then the final data points will not have as much relevance as they could.
3. The questions asked during cross-sectional studies may lead to specific results. If researchers want to achieve a specific result when performing a cross-sectional study, then they can ask questions in such a way that it leads participants to the desired answer. When there are surveys or questionnaires about specific aspects of a person’s life, then the answers received may not always result in an accurate report. Shared experiences can result in different perspectives.
We have seen this disadvantage play out numerous times throughout several generations. The people who were alive during the Vietnam war, the attack on Pearl Harbor, or the terrorist event in New York City on 9/11 have shared experiences that make them different from other age groups. The people who survived these events are another subgroup that can impact the quality of information gathered.
4. Large sample sizes are often necessary to generate usable information. A significant sample size is often necessary for a cross-sectional study to provide useful information. This disadvantage occurs because the entire population demographic must go through the research at once to prevent errors in the data. When a smaller sample size is the focus of the work, then the risk of errors entering into the information increases dramatically. There are more opportunities for coincidence or a chance to influence the results with a smaller research sample.
Although cross-sectional studies are often very affordable, the inclusion of an entire demographic pushes the cost of this work higher than it would be for other approaches.
5. Cross-sectional studies don’t offer any control over purpose or choice. When the data from a cross-sectional study is found useful for secondary data analysis, the bias of the researchers can influence the information without any future realization. The secondary approach has no control over how this work gets completed initially. That’s why an overview of the methods used and the purpose for collecting information in the first place are often included as part of the results of this work.
If these additional facts are not part of the final experience, and the usefulness of the information for future needs becomes questionable.
6. No information about causal relationships is possible with this approach. This research method does not provide information about causal relationships. The goal of this approach is to offer correlated data that is useful when drawing conclusions about a specific demographic. It can only let researchers see that a causal relationship exists without letting them know the reason behind its existence.
That’s why individualization is a disadvantage to this type of study. Researchers are wanting to see a generalized overview of a specific population sample instead of understanding why some people make particular choices.
7. Demographic definitions must be available to create a. successful result. The information collected during a cross-sectional study is not reliable unless there are specific definitions in place for a population sample that is large enough for generalization. If researchers want to look at a rare outcome or a unique event, then inappropriate conclusions could get gleaned from the collected data. Trying to force a specific question or result could encourage responses that are unnecessary within the study population.
The only way to avoid this disadvantage of a cross-sectional study is to create definitions that work specifically with the intended results.
8. Cross-sectional studies have no way to measure incidence. The goal of a cross-sectional study is to review the data that researchers collect as they study-specific variables. It does not take a look at the reason why the specific information points occur within the population demographics. This disadvantage limits the availability of an outcome for researchers in many situations because there is no determination available as to why the variables are present initially.
It only measures the existence and relationships that are present in that environment, not what triggers the variables.
9. It can be challenging to duplicate the results. Even though a large population sample is necessary to create an accurate dataset from a cross-sectional study, it is challenging to duplicate results from multiple efforts. This disadvantage occurs because work happens in real-time situations. What happens right now can create a very different result then what could happen in the future.
That’s why many institutions face some challenges when they attempt to put together a sampling pool. The variables that should be studied are available in complex ways that may be difficult to manage. This issue is so detrimental that the timing of a specific snapshot is never a 100% guarantee that it’s representative of the entire population group.
A cross-sectional study is a useful research tool in most areas of health and wellness. When we can learn more about what is happening within a specific population demographic, then researchers can better understand the relationships that could exist between particular variables. The information that comes out of this process allows us to develop further studies that can explore the results in greater depth.
Several other research study options are available when there is a need to collect information from a specific demographic. It is essential to compare the critical points from each approach to determine what the best possible solution will be for each situation.
These cross-sectional advantages and disadvantages show us that a massive undertaking of simultaneous data collection can provide unique results that can benefit an entire population group. Although there are some challenges to manage when taking this approach, it is one that most researchers find to be beneficial.

Critical Appraisal Resources for Evidence-Based Nursing Practice
- Levels of Evidence
- Systematic Reviews
- Randomized Controlled Trials
- Quasi-Experimental Studies
- Case-Control Studies
- Cohort Studies
- Analytical Cross-Sectional Studies
What is an Analytical Cross-Sectional Study?
Pro tips: analytical cross-sectional study checklist, articles on cross-sectional study design and methodology.
- Qualitative Research
E-Books for Terminology and Definitions
An analytical cross-sectional study is a type of quantitative, non-experimental research design. These studies seek to "gather data from a group of subjects at only one point in time" (Schmidt & Brown, 2019, p. 206). The purpose is to measure the association between an exposure and a disease, condition or outcome within a defined population. Cross-sectional studies often utilize surveys or questionnaires to gather data from participants (Schmidt & Brown, 2019, pp. 206-207).
Schmidt N. A. & Brown J. M. (2019). Evidence-based practice for nurses: Appraisal and application of research (4th ed.). Jones & Bartlett Learning.
Each JBI Checklist provides tips and guidance on what to look for to answer each question. These tips begin on page 4.
Below are some additional Frequently Asked Questions about the Analytical Cross-Sectional Studies Checklist that have been asked students in previous semesters.
Frequently Asked Question | Response |
A confounder or confounding factor/confounding variable is often referred to as a third variable that could potentially impact the study's results. Read a definition and description . Confounding factors/variables or confounders may be listed in the study's limitations section or within the study's main results section. | |
Check for or regression analysis in the study's data analysis/statistical analysis section. Read a definition and description . |
For more help: Each JBI Checklist provides detailed guidance on what to look for to answer each question on the checklist. These explanatory notes begin on page four of each Checklist. Please review these carefully as you conduct critical appraisal using JBI tools.
Kesmodel U. S. (2018). Cross-sectional studies - what are they good for? Acta Obstetricia et Gynecologica Scandinavica , 97 (4), 388–393. https://doi.org/10.1111/aogs.13331
Pandis N. (2014). Cross-sectional studies . American Journal of Orthodontics and Dentofacial Orthopedics , 146 (1), 127–129. https://doi.org/10.1016/j.ajodo.2014.05.005
Savitz, D. A., & Wellenius, G. A. (2023). Can cross-sectional studies contribute to causal inference? It depends . American Journal of Epidemiology , 192 (4), 514–516. https://doi.org/10.1093/aje/kwac037
Wang, X., & Cheng, Z. (2020). Cross-sectional studies: Strengths, weaknesses, and recommendations . Chest , 158 (1S), S65–S71. https://doi.org/10.1016/j.chest.2020.03.012
- << Previous: Cohort Studies
- Next: Qualitative Research >>
- Last Updated: Feb 22, 2024 11:26 AM
- URL: https://libguides.utoledo.edu/nursingappraisal
Information
- Author Services
Initiatives
You are accessing a machine-readable page. In order to be human-readable, please install an RSS reader.
All articles published by MDPI are made immediately available worldwide under an open access license. No special permission is required to reuse all or part of the article published by MDPI, including figures and tables. For articles published under an open access Creative Common CC BY license, any part of the article may be reused without permission provided that the original article is clearly cited. For more information, please refer to https://www.mdpi.com/openaccess .
Feature papers represent the most advanced research with significant potential for high impact in the field. A Feature Paper should be a substantial original Article that involves several techniques or approaches, provides an outlook for future research directions and describes possible research applications.
Feature papers are submitted upon individual invitation or recommendation by the scientific editors and must receive positive feedback from the reviewers.
Editor’s Choice articles are based on recommendations by the scientific editors of MDPI journals from around the world. Editors select a small number of articles recently published in the journal that they believe will be particularly interesting to readers, or important in the respective research area. The aim is to provide a snapshot of some of the most exciting work published in the various research areas of the journal.
Original Submission Date Received: .
- Active Journals
- Find a Journal
- Proceedings Series
- For Authors
- For Reviewers
- For Editors
- For Librarians
- For Publishers
- For Societies
- For Conference Organizers
- Open Access Policy
- Institutional Open Access Program
- Special Issues Guidelines
- Editorial Process
- Research and Publication Ethics
- Article Processing Charges
- Testimonials
- Preprints.org
- SciProfiles
- Encyclopedia
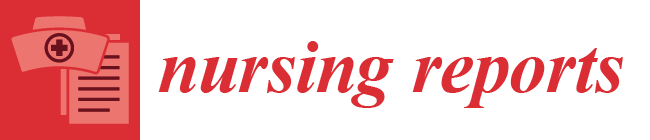
Article Menu

- Subscribe SciFeed
- Recommended Articles
- Google Scholar
- on Google Scholar
- Table of Contents
Find support for a specific problem in the support section of our website.
Please let us know what you think of our products and services.
Visit our dedicated information section to learn more about MDPI.
JSmol Viewer
Evaluation of the psychometric properties of the 18-items dysfunctional attitudes scale (form b) in a portuguese sample of people aged 60 and over.
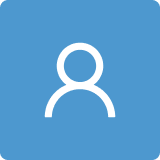
1. Introduction
2. materials and methods, 2.1. setting and data collection, 2.2. patient and public involvement, 2.3. sample, 2.4. measure, 2.4.1. sociodemographic questionnaire, 2.4.2. dysfunctional attitudes scale-18b (das-18b)—portuguese version, 2.4.3. beck’s depression inventory (bdi-ii), 2.4.4. analysis, 3.1. study sample and descriptive statistics, 3.2. construct validity, 3.3. reliability, 3.4. convergent validity, 3.5. intra-rater reliability, 4. discussion, limitations, 5. conclusions, author contributions, institutional review board statement, informed consent statement, data availability statement, public involvement statement, guidelines and standards statement, use of artificial intelligence, acknowledgments, conflicts of interest.
- World Health Organization Depression [Internet]. 2021. Available online: https://www.who.int/news-room/fact-sheets/detail/depression (accessed on 7 May 2023).
- James, S.L.; Abate, D.; Abate, K.H.; Abay, S.M.; Abbafati, C.; Abbasi, N.; Abbastabar, H.; Abd-Allah, F.; Abdela, J.; Abdelalim, A.; et al. Global, regional, and national incidence, prevalence, and years lived with disability for 354 diseases and injuries for 195 countries and territories, 1990–2017: A systematic analysis for the Global Burden of Disease Study 2017. Lancet 2018 , 392 , 1789–1858. [ Google Scholar ] [ CrossRef ] [ PubMed ]
- Santomauro, D.F.; Mantilla Herrera, A.M.; Shadid, J.; Zheng, P.; Ashbaugh, C.; Pigott, D.M.; Abbafati, C.; Adolph, C.; Amlag, J.O.; Aravkin, A.Y.; et al. Global prevalence and burden of depressive and anxiety disorders in 204 countries and territories in 2020 due to the COVID-19 pandemic. Lancet 2021 , 398 , 1700–1712. [ Google Scholar ] [ CrossRef ] [ PubMed ]
- Carvalho, J.; Borges-Machado, F.; Pizarro, A.N.; Bohn, L.; Barros, D. Home Confinement in Previously Active Older Adults: A Cross-Sectional Analysis of Physical Fitness and Physical Activity Behavior and Their Relationship With Depressive Symptoms. Front. Psychol. 2021 , 12 , 643832. [ Google Scholar ] [ CrossRef ]
- Silva, C.; Fonseca, C.; Ferreira, R.; Weidner, A.; Morgado, B.; Lopes, M.J.; Moritz, S.; Jelinek, L.; Schneider, B.C.; Pinho, L.G. Depression in older adults during the COVID-19 pandemic: A systematic review. J. Am. Geriatr. Soc. 2023 , 71 , 2308–2325. [ Google Scholar ] [ CrossRef ]
- Torres, Z.; Oliver, A.; Fernández, I. Older adults’ mental health during the COVID-19 pandemic: The association with social networks. Soc. Netw. 2024 , 78 , 164–172. [ Google Scholar ] [ CrossRef ]
- Ferri, P.M.; Mendes, V.S.; Peixoto, H.R.; Gilbert, N.D.S.; Pontes, A.C.B.; Mello, P.A.; Serafim, R.B. Impact of social isolation due to the COVID-19 pandemic on the health of older adults. In Challenges and Research in Health Sciences: A Multidisciplinary Approach ; Seven Editora: São José dos Pinhais, Brazil, 2024; Available online: https://sevenpublicacoes.com.br/index.php/editora/article/view/4868 (accessed on 23 August 2024).
- Beck, A.T. Thinking and Depression. Arch. Gen. Psychiatry 1963 , 9 , 324. [ Google Scholar ] [ CrossRef ]
- Beck, A.T. Thinking and Depression. Arch. Gen. Psychiatry 1964 , 10 , 561. [ Google Scholar ] [ CrossRef ] [ PubMed ]
- Beck, A.T. Cognition, Affect, and Psychopathology. Arch. Gen. Psychiatry 1971 , 24 , 495. [ Google Scholar ] [ CrossRef ]
- Beck, A.T. An Inventory for Measuring Depression. Arch. Gen. Psychiatry 1961 , 4 , 561. [ Google Scholar ] [ CrossRef ]
- Ward, C.H. Typical Dreams. Arch. Gen. Psychiatry 1961 , 5 , 606. [ Google Scholar ] [ CrossRef ]
- Beck, A.T. Cognitive Therapy and the Emotional Disorders ; Plume: Palo Alto, CA, USA, 1979. [ Google Scholar ]
- Almeida, F.A.D. Benefícios da terapia comportamental cognitiva em pacientes com depressão. In Terapia Comportamental Cognitiva: Base Teórica e Intervenções ; Editora Científica Digital: Sao Paulo, Brazil, 2021; pp. 12–26. [ Google Scholar ]
- Bahls, S.-C. Depressão: Uma breve revisão dos fundamentos biológicos e cognitivos. Interação Psicol. 1999 , 3 , 49–60. [ Google Scholar ] [ CrossRef ]
- Lampert, C.D.T.; Scortegagna, S.A. Avaliação das condições de saúde e distorções cognitivas de idosos com depressão. Rev. Avaliação Psicológica 2017 , 16 , 48–58. [ Google Scholar ] [ CrossRef ]
- Powell, V.B.; Abreu, N.; Oliveira, I.R.d.; Sudak, D. Terapia cognitivo-comportamental da depressão. Rev. Bras. Psiquiatr. 2008 , 30 (Suppl. 2), s73–s80. [ Google Scholar ] [ CrossRef ]
- Scher, C.; Segal, Z.; Ingram, R. Beck’s theory of depression. In Contemporary Cognitive Therapy: Theory, Research, and Practice ; The Guilford Press: New York, NY, USA, 2004; pp. 27–44. [ Google Scholar ]
- Beck, A.T.; Rush, A.J.; Shaw, B.F.; Emery, G. Cognitive Therapy of Depression ; Guilford Press: New York, NY, USA, 1979. [ Google Scholar ]
- Luty, S.E.; Joyce, P.R.; Mulder, R.T.; Sullivan, P.F.; McKenzie, J.M. The relationship of dysfunctional attitudes to personality in depressed patients. J. Affect. Disord. 1999 , 54 , 75–80. [ Google Scholar ] [ CrossRef ]
- Perez, J.; Rohan, K.J. Cognitive Predictors of Depressive Symptoms: Cognitive Reactivity, Mood Reactivity, and Dysfunctional Attitudes. Cognit. Ther. Res. 2021 , 45 , 123–135. [ Google Scholar ] [ CrossRef ]
- Jugessur, R.; Zhang, Y.; Qin, X.; Wang, M.; Lu, X.; Sun, J.; Dong, Q.; Zhang, L.; Liu, J.; Ju, Y.; et al. Childhood Maltreatment Predicts Specific Types of Dysfunctional Attitudes in Participants with and without Depression. Front. Psychiatry 2021 , 12 , 728280. [ Google Scholar ] [ CrossRef ]
- Xavier, S.; Branquinho, M.; Pires, R.; Moreira, H.; Coelho, M.; Araújo-Pedrosa, A. Dysfunctional Attitudes toward Motherhood and Depressive Symptoms in Portuguese Pregnant Women During COVID-19 Pandemic: The Mediating Roles of Self-Compassion and Mindful Self-Care. Mindfulness 2023 , 14 , 418–428. [ Google Scholar ] [ CrossRef ] [ PubMed ]
- Qin, X.; Sun, J.; Wang, M.; Lu, X.; Dong, Q.; Zhang, L.; Liu, J.; Ju, Y.; Wan, P.; Guo, H.; et al. Gender Differences in Dysfunctional Attitudes in Major Depressive Disorder. Front. Psychiatry 2020 , 11 , 86. [ Google Scholar ] [ CrossRef ]
- Kërqeli, A.; Kelpi, M.; Tsigilis, N. Dysfunctional Attitudes and Their Effect on Depression. Procedia Soc. Behav. Sci. 2013 , 84 , 196–204. [ Google Scholar ] [ CrossRef ]
- Yan, Y.; Du, X.; Lai, L.; Ren, Z.; Li, H. Prevalence of depressive and anxiety symptoms among Chinese older adults during the COVID-19 pandemic: A systematic review and meta-analysis. J. Geriatr. Psychiatry Neurol. 2022 , 35 , 182–195. [ Google Scholar ] [ CrossRef ]
- Bockting, C.L.; Hollon, S.D.; Jarrett, R.B.; Kuyken, W.; Dobson, K. A lifetime approach to major depressive disorder: The contributions of psychological interventions in preventing relapse and recurrence. Clin. Psychol. Rev. 2015 , 41 , 16–26. [ Google Scholar ] [ CrossRef ]
- Breedvelt, J.J.F.; Warren, F.C.; Segal, Z.; Kuyken, W.; Bockting, C.L. Continuation of Antidepressants vs Sequential Psychological Interventions to Prevent Relapse in Depression. JAMA Psychiatry 2021 , 78 , 868–875. [ Google Scholar ] [ CrossRef ] [ PubMed ]
- Moriarty, A.S.; Coventry, P.A.; Hudson, J.L.; Cook, N.; Fenton, O.J.; Bower, P.; Lovell, K.; Archer, J.; Clarke, R.; Richards, D.A.; et al. The role of relapse prevention for depression in collaborative care: A systematic review. J. Affect. Disord. 2020 , 265 , 618–644. [ Google Scholar ] [ CrossRef ] [ PubMed ]
- Weissman, A.N.; Beck, A. Development and Validation of the Dysfunctional Attitude Scale: A Preliminary Investigation. 1978. Available online: https://files.eric.ed.gov/fulltext/ED167619.pdf (accessed on 6 November 2022).
- Garnefski, N.; Grol, M.; Kraaij, V.; Hamming, J.F. Cognitive coping and goal adjustment in people with Peripheral Arterial Disease: Relationships with depressive symptoms. Patient Educ. Couns. 2009 , 76 , 132–137. [ Google Scholar ] [ CrossRef ] [ PubMed ]
- Kraaij, V.; Pruymboom, E.; Garnefski, N. Cognitive coping and depressive symptoms in the elderly: A longitudinal study. Aging Ment. Health 2002 , 6 , 275–281. [ Google Scholar ] [ CrossRef ]
- Broomfield, N.M.; Davies, R.; MacMahon, K.; Ali, F.; Cross, S.M.B. Further evidence of attention bias for negative information in late life depression. Int. J. Geriatr. Psychiatry 2007 , 22 , 175–180. [ Google Scholar ] [ CrossRef ]
- Köhler, S.; Unger, T.; Hoffmann, S.; Mackert, A.; Ross, B.; Fydrich, T. Dysfunctional cognitions of depressive inpatients and their relationship with treatment outcome. Compr. Psychiatry 2015 , 58 , 50–56. [ Google Scholar ] [ CrossRef ]
- Floyd, M.; Scogin, F.; Chaplin, W.F. The Dysfunctional Attitudes Scale: Factor structure, reliability, and validity with older adults. Aging Ment. Health 2004 , 8 , 153–160. [ Google Scholar ] [ CrossRef ]
- Koenig, H.G. Differences in Psychosocial and Health Correlates of Major and Minor Depression in Medically Ill Older Adults. J. Am. Geriatr. Soc. 1997 , 45 , 1487–1495. [ Google Scholar ] [ CrossRef ]
- Sarica Acaröz, Ö.; Işiktaş, S. Yetişkinlerde Fonksiyonel Olmayan Tutumların ve Olumsuz Otomatik Düşüncelerin Depresyona Etkisinin İncelenmesi. Uluslararası Beşeri Bilim. Eğitim Derg. 2023 , 9 , 311–331. [ Google Scholar ] [ CrossRef ]
- Xue, J.-M.; Su, J. The Effect of Aging Attitudes on the Depression of Older Adults in China. Nurs. Health Care 2023 , 5 , 1–7. [ Google Scholar ] [ CrossRef ]
- Brown, G.P.; Delgadillo, J.; Golino, H. Distinguishing the Dimensions of the Original Dysfunctional Attitude Scale in an Archival Clinical Sample. Cognit. Ther. Res. 2023 , 47 , 69–83. [ Google Scholar ] [ CrossRef ]
- Woods, C.; Richardson, T.; Palmer-Cooper, E. Are dysfunctional attitudes elevated and linked to mood in bipolar disorder? A systematic review and meta-analysis. Br. J. Clin. Psychol. 2024 , 63 , 16–53. [ Google Scholar ] [ CrossRef ]
- Gouveia, J.A.V.P. Factores Cognitivos de Vulnerabilidade Para a Depressão: Estudo da sua Interacção com os Acontecimentos de Vida ; University of Coimbra: Coimbra, Portugal, 1990. [ Google Scholar ]
- Weissman, A.N. The Dysfunctional Attitude Scale: A Validation Study ; University of Pennsylvania: Philadelphia, PA, USA, 1979. [ Google Scholar ]
- Rojas, R.; Geissner, E.; Hautzinger, M. DAS-18 Form A und Form B. Diagnostica 2015 , 61 , 173–183. [ Google Scholar ] [ CrossRef ]
- Rojas, R.; Geissner, E.; Hautzinger, M. DAS-18. Dysfunctional Attitude Scale 18—Deutsche Kurzfassung. In Open Test Arch ; Leibniz-Institut für Psychol (Hrsg): Trier, Germany, 2022. [ Google Scholar ]
- Beevers, C.G.; Keitner, G.I.; Ryan, C.E.; Miller, I.W. Cognitive predictors of symptom return following depression treatment. J. Abnorm. Psychol. 2003 , 112 , 488–496. [ Google Scholar ] [ CrossRef ] [ PubMed ]
- Forand, N.R.; DeRubeis, R.J. Extreme response style and symptom return after depression treatment: The role of positive extreme responding. J. Consult. Clin. Psychol. 2014 , 82 , 500–509. [ Google Scholar ] [ CrossRef ] [ PubMed ]
- Forand, N.R.; Strunk, D.R.; DeRubeis, R.J. Positive extreme responding after cognitive therapy for depression: Correlates and potential mechanisms. Behav. Res. Ther. 2016 , 83 , 11–18. [ Google Scholar ] [ CrossRef ]
- Brouwer, M.E.; Williams, A.D.; Forand, N.R.; DeRubeis, R.J.; Bockting, C.L.H. Dysfunctional attitudes or extreme response style as predictors of depressive relapse and recurrence after mobile cognitive therapy for recurrent depression. J. Affect. Disord. 2019 , 243 , 48–54. [ Google Scholar ] [ CrossRef ]
- Ezawa, I.D.; Forand, N.R.; Strunk, D.R. An examination of dysfunctional attitudes and extreme response styles as predictors of relapse in guided internet-based cognitive behavioral therapy for depression. J. Clin. Psychol. 2020 , 76 , 1047–1059. [ Google Scholar ] [ CrossRef ]
- Orsini, M.R.d.C.A.; Tavares, M.; Tróccoli, B.T. Adaptação Brasileira da Escala de Atitudes Disfuncionais (DAS). Psico-USF 2006 , 11 , 25–33. [ Google Scholar ] [ CrossRef ]
- Yesilyaprak, N.; Batmaz, S.; Yildiz, M.; Songur, E.; Akpinar Aslan, E. Automatic thoughts, cognitive distortions, dysfunctional attitudes, core beliefs, and ruminative response styles in unipolar major depressive disorder and bipolar disorder: A comparative study. Psychiatry Clin. Psychopharmacol. 2019 , 29 , 854–863. [ Google Scholar ] [ CrossRef ]
- Andreas, S.; Schulz, H.; Volkert, J.; Dehoust, M.; Sehner, S.; Suling, A.; Ausín, B.; Canuto, A.; Crawford, M.; Da Ronch, C.; et al. Prevalence of mental disorders in elderly people: The European MentDis_ICF65+ study. Br. J. Psychiatry 2017 , 210 , 125–131. [ Google Scholar ] [ CrossRef ]
- Kessler, R.C.; Birnbaum, H.; Bromet, E.; Hwang, I.; Sampson, N.; Shahly, V. Age differences in major depression: Results from the National Comorbidity Survey Replication (NCS-R). Psychol. Med. 2010 , 40 , 225–237. [ Google Scholar ] [ CrossRef ] [ PubMed ]
- Paiva, T.C.; Soares, L.; Faria, A.L. Depression in Elderly People. Encyclopedia 2023 , 3 , 677–686. [ Google Scholar ] [ CrossRef ]
- Agapitos, M.; Muniz-Terrera, G.; Robitaille, A. Older caregivers’ depressive symptomatology over time: Evidence from the Survey of Health, Ageing and Retirement in Europe. Eur. J. Ageing 2024 , 21 , 21. [ Google Scholar ] [ CrossRef ] [ PubMed ]
- Eimontas, J.; Gegieckaitė, G.; Zamalijeva, O.; Pakalniškienė, V. Unmet Healthcare Needs Predict Depression Symptoms among Older Adults. Int. J. Environ. Res. Public Health 2022 , 19 , 8892. [ Google Scholar ] [ CrossRef ]
- Cui, R.; Fiske, A. Relation Between Depression Symptoms and Suicide Risk in Adults and Older Adults: A Brief Report. J. Appl. Gerontol. 2022 , 41 , 176–180. [ Google Scholar ] [ CrossRef ]
- Baker, R.; Wilson, S.; Daunt, L. Depression in older adults. InnovAiT Educ. Inspir. Gen. Pract. 2022 , 15 , 25–32. [ Google Scholar ] [ CrossRef ]
- Fidelma Azzahra Pingkan Zondia; Fatichati Budiningsih; I Gusti Bagus Indro Nugroho; Dhani Redhono Harioputro The effect of depression on the quality of life of the elderly. GSC Biol. Pharm. Sci. 2024 , 27 , 195–203.
- Amridi, R.; Vimala, M. Depression, quality of life, and cognitive abilities among older adults. World J. Adv. Res. Rev. 2024 , 22 , 1504–1511. [ Google Scholar ] [ CrossRef ]
- Wiehn, J.; Kurth, T.; Ravens-Sieberer, U.; Prugger, C.; Piccininni, M.; Reiss, F. Effect of elevated depressive symptoms during adolescence on health-related quality of life in young adulthood—A six-year cohort study with repeated exposure measurements. Front. Pediatr. 2024 , 12 , 1252964. [ Google Scholar ] [ CrossRef ] [ PubMed ]
- Schieszler-Ockrassa, C.; Fitzgerald, M.; Janecek, J.; Swanson, S. 29 Quality of Life in Younger and Older Adults with Epilepsy. J. Int. Neuropsychol. Soc. 2023 , 29 , 30. [ Google Scholar ] [ CrossRef ]
- Shilpi, T. Depression in Elderly Life: Psychological and Psychosocial Approaches. Int. J. Depress. Anxiety 2020 , 3 , 1–3. [ Google Scholar ] [ CrossRef ] [ PubMed ]
- Schneider, B.C.; Bücker, L.; Riker, S.; Karamatskos, E.; Jelinek, L. A Pilot Study of Metacognitive Training (D-MCT) for Older Adults with Depression. Z. Neuropsychol. 2018 , 29 , 7–19. [ Google Scholar ] [ CrossRef ]
- Corrigan, P.W.; Nieweglowski, K.; Sayer, J. Self-stigma and the mediating impact of the “why try” effect on depression. J. Community Psychol. 2019 , 47 , 698–705. [ Google Scholar ] [ CrossRef ] [ PubMed ]
- Dubreucq, J.; Plasse, J.; Franck, N. Self-stigma in Serious Mental Illness: A Systematic Review of Frequency, Correlates, and Consequences. Schizophr. Bull. 2021 , 47 , 1261–1287. [ Google Scholar ] [ CrossRef ] [ PubMed ]
- von Elm, E.; Altman, D.G.; Egger, M.; Pocock, S.J.; Gøtzsche, P.C.; Vandenbroucke, J.P. The Strengthening the Reporting of Observational Studies in Epidemiology (STROBE) statement: Guidelines for reporting observational studies. Lancet 2007 , 370 , 1453–1457. [ Google Scholar ] [ CrossRef ]
- Pestano, M.H.; Gageiro, J.N. Análise de Dados Para Ciências Sociais: A Complementaridade do SPSS ; Edições Sílabo: Lisboa, Portugal, 2005. [ Google Scholar ]
- Kahn, J.H. Factor Analysis in Counseling Psychology Research, Training, and Practice. Couns. Psychol. 2006 , 34 , 684–718. [ Google Scholar ] [ CrossRef ]
- Worthington, R.L.; Whittaker, T.A. Scale Development Research. Couns. Psychol. 2006 , 34 , 806–838. [ Google Scholar ] [ CrossRef ]
- Arafat, S.; Chowdhury, H.; Qusar, M.; Hafez, M. Cross Cultural Adaptation and Psychometric Validation of Research Instruments: A Methodological Review. J. Behav. Health 2016 , 5 , 129. [ Google Scholar ] [ CrossRef ]
- Martins, A.; Coelho, R.; Ramos, E.; Barros, H. Administração Do BDI-II a Adolescentes Portugueses: Resultados Preliminares. Rev. Port. Psicossomática 2000 , 2 , 123–132. [ Google Scholar ]
- Araújo, M. A Doença Oncológica Na Criança: Adaptação e Bem-Estar Psicológico Das Mães Dos Seus Filhos e a Relação Entre Ambos ; Coisas de Ler: Lisboa, Portugal, 2011. [ Google Scholar ]
- Beck, A.T.; Steer, R.A.; Brown, G. Beck Depression Inventory-II Manual ; The Psychological Corporation: New York, NY, USA, 1966. [ Google Scholar ]
- Matos, D.; Rodrigues, E. Análise Fatorial ; Escola Nacional de Administração Pública—Enap: Brasília, Brazil, 2019. Available online: http://repositorio.enap.gov.br/handle/1/4790 (accessed on 15 April 2024).
- Olinger, L. Dysfunctional Attitudes, Performance Deficits, and Vulnerability to Depression ; Western University: London, ON, Canada, 1984; Available online: https://ir.lib.uwo.ca/digitizedtheses/1331/ (accessed on 19 November 2023).
- Rocha, A.S. Perfeccionismo e a relação com psicopatologias: Estudo integrativo. Res. Soc. Dev. 2021 , 10 , e59410213033. [ Google Scholar ] [ CrossRef ]
- Brown, G.P.; Hammen, C.L.; Craske, M.G.; Wickens, T.D. Dimensions of dysfunctional attitudes as vulnerabilities to depressive symptoms. J. Abnorm. Psychol. 1995 , 104 , 431–435. [ Google Scholar ] [ CrossRef ]
- Freitas, C. Perfeccionismo, Auto-Conceito e Sintomatologia Psicopatológica em Estudantes do Ensino Superior ; Universidade da Beira Interior: Covilhã, Portugal, 2011; Available online: https://ubibliorum.ubi.pt/bitstream/10400.6/2732/1/Tese_Clemence_Freitas.pdf (accessed on 19 November 2023).
- Silva, M. Perfeccionismo e Sintomatologia Psicopatológica e Rendimento Académico em Estudantes do Ensino Superior ; Universidade da Beira Interior: Covilhã, Portugal, 2014; Available online: https://ubibliorum.ubi.pt/bitstream/10400.6/6156/1/3933_7650.pdf (accessed on 19 November 2023).
- Chrousos, G.P.; Mentis, A.-F.A.; Dardiotis, E. Focusing on the Neuro-Psycho-Biological and Evolutionary Underpinnings of the Imposter Syndrome. Front. Psychol. 2020 , 11 , 1553. [ Google Scholar ] [ CrossRef ] [ PubMed ]
- Lima, D.F. A síndrome do impostor: Um olhar para a saúde mental de professores. Perspect. Diálogo Rev. Educ. Soc. 2023 , 10 , 144–158. [ Google Scholar ] [ CrossRef ]
- Smith, M.M.; Sherry, S.B.; Ray, C.; Hewitt, P.L.; Flett, G.L. Is perfectionism a vulnerability factor for depressive symptoms, a complication of depressive symptoms, or both? A meta-analytic test of 67 longitudinal studies. Clin. Psychol. Rev. 2021 , 84 , 101982. [ Google Scholar ] [ CrossRef ] [ PubMed ]
- Wright, A.; Fisher, P.L.; Baker, N.; O’Rourke, L.; Cherry, M.G. Perfectionism, depression and anxiety in chronic fatigue syndrome: A systematic review. J. Psychosom. Res. 2021 , 140 , 110322. [ Google Scholar ] [ CrossRef ]
- Gara, M.A.; Woolfolk, R.L.; Cohen, B.D.; Goldston, R.B.; Allen, L.A.; Novalany, J. Perception of self and other in major depression. J. Abnorm. Psychol. 1993 , 102 , 93–100. [ Google Scholar ] [ CrossRef ]
- Joiner, T.E.; Metalsky, G.I.; Katz, J.; Beach, S.R.H. Depression and Excessive Reassurance-Seeking. Psychol. Inq. 1999 , 10 , 269–278. [ Google Scholar ] [ CrossRef ]
- Raes, F.; Hermans, D.; Williams, J.M.G. Negative Bias in the Perception of Others’ Facial Emotional Expressions in Major Depression. J. Nerv. Ment. Dis. 2006 , 194 , 796–799. [ Google Scholar ] [ CrossRef ]
- Carvalho, S.; Dinis, A.; Pinto-Gouveia, J.; Estanqueiro, C. Memories of Shame Experiences with Others and Depression Symptoms: The Mediating Role of Experiential Avoidance. Clin. Psychol. Psychother. 2015 , 22 , 32–44. [ Google Scholar ] [ CrossRef ] [ PubMed ]
- Ribeiro, L.C.P.J.M.; Balestra, A.G.; Nicoletti, Ê.A.; Donadon, M.F. A espiritualidade na flexibilização de pensamentos e crenças de uma paciente ansiosa. Rev. Bras. Ter. Cogn. 2019 , 15 , 126–131. [ Google Scholar ] [ CrossRef ]
- Joeng, J.R.; Turner, S.L. Mediators between self-criticism and depression: Fear of compassion, self-compassion, and importance to others. J. Couns. Psychol. 2015 , 62 , 453–463. [ Google Scholar ] [ CrossRef ] [ PubMed ]
- Matos, M.; Pinto-Gouveia, J. Shamed by a Parent or by Others: The Role of Attachment in Shame Memories Relation to Depression. Int. J. Psychol. Psychol. Ther. 2014 , 14 , 217–244. [ Google Scholar ]
- Matos, M.; Pinto-Gouveia, J.; Costa, V. Understanding the Importance of Attachment in Shame Traumatic Memory Relation to Depression: The Impact of Emotion Regulation Processes. Clin. Psychol. Psychother. 2013 , 20 , 149–165. [ Google Scholar ] [ CrossRef ]
- Joiner, T.E.; Timmons, K.A. Depression in its interpersonal context. Handb. Depress. 2002 , 2 , 322–339. [ Google Scholar ]
- Bekker, M.H.J.; Croon, M.A. The roles of autonomy-connectedness and attachment styles in depression and anxiety. J. Soc. Pers. Relat. 2010 , 27 , 908–923. [ Google Scholar ] [ CrossRef ]
- Sacco, W.P.; Vaughan, C.A. Depression and the response of others: A social-cognitive interpersonal process model. In The Interpersonal, Cognitive, and Social Nature of Depression ; Routledge: Oxfordshire, UK, 2014; pp. 101–132. [ Google Scholar ]
- Thwaites, R.; Dagnan, D. Moderating variables in the relationship between social comparison and depression: An evolutionary perspective. Psychol. Psychother. Theory Res. Pract. 2004 , 77 , 309–323. [ Google Scholar ] [ CrossRef ]
- Zinbarg, R.E.; Revelle, W.; Yovel, I.; Li, W. Cronbach’s α, Revelle’s β, and Mcdonald’s ωH: Their relations with each other and two alternative conceptualizations of reliability. Psychometrika 2005 , 70 , 123–133. [ Google Scholar ] [ CrossRef ]
- Marôco, J. Análise Estatística com Utilização do SPSS Statistics ; Report Number: Lisboa, Portugal, 2021. [ Google Scholar ]
- Hill, M.; Hill, A. Investigação por Questionário ; Edições Sílabo: Lisboa, Portugal, 2009. [ Google Scholar ]
- Tajima, M.; Akiyama, T.; Numa, H.; Kawamura, Y.; Okada, Y.; Sakai, Y.; Miyake, Y.; Ono, Y.; Power, M.J. Reliability and validity of the Japanese version of the 24-item Dysfunctional Attitude Scale. Acta Neuropsychiatr. 2007 , 19 , 362–367. [ Google Scholar ] [ CrossRef ]
- Beck, A.T.; Bredemeier, K. A Unified Model of Depression. Clin. Psychol. Sci. 2016 , 4 , 596–619. [ Google Scholar ] [ CrossRef ]
- Barney, L.J.; Griffiths, K.M.; Christensen, H.; Jorm, A.F. The Self-Stigma of Depression Scale (SSDS): Development and psychometric evaluation of a new instrument. Int. J. Methods Psychiatr. Res. 2010 , 19 , 243–254. [ Google Scholar ] [ CrossRef ]
- Pellet, J.; Golay, P.; Nguyen, A.; Suter, C.; Ismailaj, A.; Bonsack, C.; Favrod, J. The relationship between self-stigma and depression among people with schizophrenia-spectrum disorders: A longitudinal study. Psychiatry Res. 2019 , 275 , 115–119. [ Google Scholar ] [ CrossRef ]
- Bratu, M.; Rizeanu, R. Stress, Depression and Dysfunctional Attitudes Among Elders. A Non-Experimental Approach. Rom. J. Psychol. Stud.—Hyperion Univ. Bucharest 2017 , 5 . Available online: https://papers.ssrn.com/sol3/papers.cfm?abstract_id=3098097 (accessed on 10 June 2024).
- Bellón, J.Á.; Moreno-Peral, P.; Motrico, E.; Rodríguez-Morejón, A.; Fernández, A.; Serrano-Blanco, A.; Zabaleta-del-Olmo, E.; Conejo-Cerón, S. Effectiveness of psychological and/or educational interventions to prevent the onset of episodes of depression: A systematic review of systematic reviews and meta-analyses. Prev. Med. 2015 , 76 , S22–S32. [ Google Scholar ] [ CrossRef ] [ PubMed ]
- Ormel, J.; Kessler, R.C.; Schoevers, R. Depression. Curr. Opin. Psychiatry 2019 , 32 , 348–354. [ Google Scholar ] [ CrossRef ] [ PubMed ]
Characteristics | N | % |
---|---|---|
Total sample | 207 | 100 |
Gender | ||
Female | 121 | 58.5 |
Male | 86 | 41.5 |
Age (years) | ||
60–70 | 141 | 68.1 |
71–80 | 55 | 26.6 |
>80 | 11 | 5.3 |
Employment situation | ||
Employee | 63 | 30.4 |
Unemployed | 13 | 6.3 |
Retired | 126 | 60.9 |
Retired on disability | 4 | 1.9 |
Medical Leave | 1 | 0.5 |
Marital status | ||
Single | 10 | 4.8 |
Married/cohabiting | 159 | 76.8 |
Widower | 26 | 12.6 |
Divorced | 12 | 5.8 |
School level | ||
Can read and write, but did not attended school | 14 | 6.8 |
Attended school but not Higher education | 167 | 80.6 |
Higher education | 26 | 12.6 |
Who you live with | ||
Alone | 28 | 13.5 |
Spouse/Partner | 160 | 77.4 |
Son/Daughter | 15 | 7.2 |
Brother/Sister | 1 | 0.5 |
Other family member | 3 | 1.4 |
Kaiser–Meyer–Olkin measure of sampling adequacy | 0.723 | |
Bartlett’s test of sphericity | Approx. chi-square | 641.074 |
χ | 55 | |
p | 0.000 |
Own Value | % of Variance | % Cumulative Variance | Own Value | % of Variance | % Cumulative Variance | |
---|---|---|---|---|---|---|
1 | 3.440 | 31.271 | 31.271 | 2.732 | 24.840 | 24.840 |
2 | 1.723 | 15.665 | 46.936 | 1.916 | 17.422 | 42.262 |
3 | 1.319 | 11.987 | 58.923 | 1.833 | 16.661 | 58.923 |
4 | 0.941 | 8.556 | 67.479 | |||
5 | 0.841 | 7.644 | 75.123 | |||
6 | 0.740 | 6.728 | 81.850 | |||
7 | 0.533 | 4.841 | 86.691 | |||
8 | 0.466 | 4.232 | 90.924 | |||
9 | 0.354 | 3.218 | 94.141 | |||
10 | 0.343 | 3.121 | 97.262 | |||
11 | 0.301 | 2.738 | 100.000 |
Items | Factors | |||
---|---|---|---|---|
Factor Assignment According to [ ] | 1 | 2 | 3 | |
DAS-18B-Q4 (It is only worth doing what we are sure of doing well) | Achievement | 0.839 | ||
DAS-18B-Q5 (If I fail partially that is as bad as if I fail totally) | Achievement | 0.737 | ||
DAS-18B-Q11 (People with good ideas are worth more than others) | Achievement | 0.703 | ||
DAS-18B-Q3 (Asking for help is a sign of weakness) | Achievement | 0.695 | ||
DAS-18B-Q1 (It is foolish to take even a small risk, because if things go wrong the consequences can be disastrous) | Achievement | 0.488 | ||
DAS-18B-Q16 (My happiness depends more on others than on me) | Recognition | 0.819 | ||
DAS-18B-Q18 (It is very important what others think about me) | Recognition | 0.769 | ||
DAS-18B-Q15 (If others do not like us, we cannot feel happy) | Recognition | 0.729 | ||
DAS-18B-Q6 (If others know how you really are, they will have a worse impression of you) | Achievement | 0.752 | ||
DAS-18B-Q9 (If I am not demanding of myself, I will probably end up as a mediocre person) | Achievement | 0.731 | ||
DAS-18B-Q10 (To be a valid person, I have to be “really good” at something) | Achievement | 0.436 | 0.682 |
Need for Recognition by Others | Performance Evaluation Based on Demand for Oneself | ||
---|---|---|---|
Test | Performance evaluation based on results | 0.203 ** | 0.449 ** |
Need for recognition by others | 0.166 * |
Total Item Correlation Corrected | Total Item Correlation Corrected Retest | Cronbach’s Alpha If the Item Is Deleted | Cronbach’s Alpha If the Item Is Deleted Retest | |
---|---|---|---|---|
DAS-18B-Q4 | 0.446 | 0.598 | 0.750 | 0.656 |
DAS-18B-Q5 | 0.608 | 0.529 | 0.729 | 0.672 |
DAS-18B-Q11 | 0.605 | 0.579 | 0.726 | 0.659 |
DAS-18B-Q3 | 0.453 | 0.282 | 0.749 | 0.708 |
DAS-18B-Q1 | 0.357 | 0.266 | 0.760 | 0.711 |
DAS-18B-Q16 | 0.362 | 0.345 | 0.759 | 0.700 |
DAS-18B-Q18 | 0.307 | 0.278 | 0.766 | 0.710 |
DAS-18B-Q15 | 0.207 | 0.366 | 0.775 | 0.697 |
DAS-18B-Q6 | 0.303 | 0.277 | 0.765 | 0.709 |
DAS-18B-Q9 | 0.384 | 0.024 | 0.758 | 0.745 |
DAS-18B-Q10 | 0.558 | 0.440 | 0.735 | 0.685 |
Cognitive Dimension | Affective Dimension | Somatic Dimension | |
---|---|---|---|
Performance evaluation based on results | −0.071 | −0.120 | −0.191 ** |
Need for recognition by others | −0.155 * | −0.283 ** | −0.260 ** |
Performance evaluation based on demand for oneself | −0.338 ** | −0.338 ** | −0.424 ** |
Intraclass Correlation | Confidence Interval 95% | F-Test with True Value 0 | |||||
---|---|---|---|---|---|---|---|
Lower Limit | Upper Limit | Value | df1 | df2 | Sig | ||
Unique measurements | 0.987 | 0.980 | 0.992 | 157.416 | 69 | 69 | 0.000 |
Average measurements | 0.994 | 0.990 | 0.996 | 157.416 | 69 | 69 | 0.000 |
The statements, opinions and data contained in all publications are solely those of the individual author(s) and contributor(s) and not of MDPI and/or the editor(s). MDPI and/or the editor(s) disclaim responsibility for any injury to people or property resulting from any ideas, methods, instructions or products referred to in the content. |
Share and Cite
Silva, C.; Ferreira, R.; Morgado, B.; Schneider, B.C.; João, A.; Sampaio, F.; Pinho, L.G.; Fonseca, C. Evaluation of the Psychometric Properties of the 18-Items Dysfunctional Attitudes Scale (Form B) in a Portuguese Sample of People Aged 60 and Over. Nurs. Rep. 2024 , 14 , 2209-2225. https://doi.org/10.3390/nursrep14030165
Silva C, Ferreira R, Morgado B, Schneider BC, João A, Sampaio F, Pinho LG, Fonseca C. Evaluation of the Psychometric Properties of the 18-Items Dysfunctional Attitudes Scale (Form B) in a Portuguese Sample of People Aged 60 and Over. Nursing Reports . 2024; 14(3):2209-2225. https://doi.org/10.3390/nursrep14030165
Silva, Celso, Rogério Ferreira, Bruno Morgado, Brooke C. Schneider, Ana João, Francisco Sampaio, Lara G. Pinho, and César Fonseca. 2024. "Evaluation of the Psychometric Properties of the 18-Items Dysfunctional Attitudes Scale (Form B) in a Portuguese Sample of People Aged 60 and Over" Nursing Reports 14, no. 3: 2209-2225. https://doi.org/10.3390/nursrep14030165
Article Metrics
Article access statistics, further information, mdpi initiatives, follow mdpi.
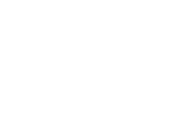
Subscribe to receive issue release notifications and newsletters from MDPI journals

An official website of the United States government
The .gov means it’s official. Federal government websites often end in .gov or .mil. Before sharing sensitive information, make sure you’re on a federal government site.
The site is secure. The https:// ensures that you are connecting to the official website and that any information you provide is encrypted and transmitted securely.
- Publications
- Account settings
Preview improvements coming to the PMC website in October 2024. Learn More or Try it out now .
- Advanced Search
- Journal List
- v.12; 2024 Jun
- PMC10874705
Risk of bias in cross-sectional studies: Protocol for a scoping review of concepts and tools
Shannon e. kelly.
a School of Epidemiology and Public Health, University of Ottawa, Ottawa, Ontario, Canada
b Cardiovascular Research Methods Centre, University of Ottawa Heart Institute, Ottawa, Ontario, Canada
Karima Benkhedda
c Bureau of Nutritional Sciences, Health Canada, Ottawa, Ontario, Canada
Stephen P.J. Brooks
d Department of Biology, Carleton University, Ottawa, Ontario, Canada
Amanda J. MacFarlane
e Agrilife Evidence Center at Texas A&M University, College Station, Fort Worth, Texas, USA
Linda S. Greene-Finestone
f Applied Research Division, Public Health Agency of Canada, Ottawa, Ontario, Canada
Becky Skidmore
g Independent Information Scientist, Ottawa, Ontario, Canada
Tammy J. Clifford
George a. wells, associated data.
- No data was used for the research described in the article.
Cross-sectional studies are commonly used to study human health and disease, but are especially susceptible to bias. This scoping review aims to identify and describe available tools to assess the risk of bias (RoB) in cross-sectional studies and to compile the key bias concepts relevant to cross-sectional studies into an item bank. Using the JBI scoping review methodology, the strategy to locate relevant RoB concepts and tools is a combination of database searches, prospective review of PROSPERO registry records; and consultation with knowledge users and content experts. English language records will be included if they describe tools, checklists, or instruments which describe or permit assessment of RoB for cross-sectional studies. Systematic reviews will be included if they consider eligible RoB tools or use RoB tools for RoB of cross-sectional studies. All records will be independently screened, selected, and extracted by one researcher and checked by a second. An analytic framework will be used to structure the extraction of data. Results for the scoping review are pending. Results from this scoping review will be used to inform future selection of RoB tools and to consider whether development of a new RoB tool for cross-sectional studies is needed.
Graphical abstract
Complete. Submitted as a separate file.

Specifications table
Subject area: | Research Methods |
More specific subject area: | Epidemiology, knowledge synthesis methods, study designs, risk of bias |
Name of your protocol: | Risk of bias in cross-sectional studies: protocol for a scoping review of concepts and tools |
Reagents/tools: | Not applicable |
Experimental design: | Not applicable. This protocol describes a scoping review. |
Trial registration: | Not applicable. This is not a trial. This scoping review is registered with the Open Science Framework ( ) |
Ethics: | This work did not involve human subjects, animal experiments or data collected from social media platforms. |
Value of the Protocol: | Scientific contribution: |
Description of protocol
A cross-sectional study is a type of observational research study that is used to examine a group of individuals at a specific point in time in order to understand their characteristics and behaviors. The objective of this scoping review is to identify tools used to evaluate the risk of bias (RoB) in cross-sectional studies of humans; and to identify, describe, and to compile the key items, domains or concepts relevant to RoB in cross-sectional studies into an item bank.
The proposed scoping review will be conducted in accordance with the JBI methodology for scoping reviews and reported in line with the Preferred Reporting Items for Systematic Reviews and Meta-Analyses extension for Scoping Reviews (PRISMA-ScR) [ 1 , 2 ].
Review questions
- 1. What tools are used to assess the risk of bias in cross-sectional studies?
- 2. What risk of bias items, domains or concepts are included in tools intended for cross-sectional study designs?
- 3. How do risk of bias concepts vary when tools are intended to be applied to analytic studies of association or prevalence?
Inclusion criteria
The Cochrane definition will be used to consider RoB as a measure of how features of the design, conduct or analysis of a study may cause systematic error in the results of a study; That is, whether the prevalence or associated effects for an outcome in a cross-sectional study may be over- or under-estimated. Since tools may be described inconsistently using language on methodological quality, quality tools will be eligible if they are judged by reviewers to contain one or more items relating to internal validity of the study.
This review will consider records for inclusion if they meet the following criteria:
- • Permit the assessment of RoB in human observational studies defined as cross-sectional studies, including analytic or prevalent/survey approaches;
- • Describe or permit global assessment of RoB for multiple study designs and originally developed for application to cross-sectional study designs; or,
- • Describe the application of a tool not originally designed for application to cross-sectional study designs which has been adapted or modified for application to cross-sectional study designs for the assessment of RoB; and,
- • Published or available in English.
“Tools” may be described as checklists, scored instruments, ‘question/item-based‘ or 'domain-based' according to groupings or categories of bias. Systematic reviews of RoB tools will also be included if they endeavoured to locate and/or include relevant tools/items/concepts for cross-sectional studies.
Records identified will be excluded if they 1) report tools intended for other study designs and are not intended for evaluation of cross-sectional study designs; or, 2) report single-study or multi-study tools modified for application to cross-sectional studies in a systematic review and the modified tool is not reported or cited.
Types of sources
This scoping review will consider records of any study design for inclusion. As there is a limited number of RoB tools published in peer-reviewed literature, four approaches will be used to search for and locate eligible RoB tools relevant to cross-sectional studies:
- 1. Bibliographic database search: This approach will be used to identify published RoB tools and relevant systematic reviews of RoB tools. For the search of bibliographic databases, an experienced medical information specialist developed and tested search strategies through an iterative process in consultation with the review team.
- 2. Prospective scan of PROSPERO registrations: This approach will be used to identify RoB tools identified in 1000 prospectively registered systematic review protocols in the PROSPERO database. In order to identify currently used tools, all records will be searched for proposals including the term “cross-sectional” and systematic reviews indicating the proposed inclusion of cross-sectional studies in the analysis will be retained. Applicable RoB tools will be identified from the details in the “Risk of bias (quality) assessment” section of the registration.
- 3. Outreach to key knowledge users: This approach will be used to gather information regarding RoB tools being applied in practice for cross-sectional studies. A group of users in the field of nutrition will be selected as they commonly use or assess cross-sectional research. The email-based outreach will briefly ask participants to reply if they are willing to share a citation or the name of a relevant RoB tool they use or are aware of.
- 4. Experiential knowledge of content experts: This approach will supplement the other approaches by collecting the experiential knowledge of key content experts to identify additional tools not represented they are aware of.
Bibliographic database search strategy
An experienced medical information specialist developed and tested the search strategies through an iterative process in consultation with the review team. A full search strategy for OvidMEDLINE® ALL, including Epub Ahead of Print, In-Process & Other Non-Indexed Citations, and Embase Classic+Embase will be searched on the Ovid platform, followed by a search of Web of Science (see Appendix I). The strategies utilize a combination of controlled vocabulary (e.g.,“Cross-Sectional Studies”, “Bias”,“Checklists”) and keywords (e.g.,“cross-sectional design”, “quality rating”,“tool”). The search strategy will be adapted for each included information source. The search strategy will aim to locate both published and unpublished tools. Results will be limited to the publication years 2011 to the present. Results will be downloaded and deduplicated using EndNote version 20.4.1 (Clarivate Analytics, PA, USA) and uploaded to Covidence systematic review software for screening (Veritas Health Innovation, Melbourne, Australia. Available at www.covidence.org ). The database search will be updated prior to completion of the scoping review.
Evidence selection
Following the search, all identified database records will be collated and uploaded into Covidence systematic review software (Veritas Health Innovation, Melbourne, Australia. Available at www.covidence.org ) and EndNote and duplicates removed. Following a pilot test, titles and abstracts will then be screened by two independent reviewers for assessment against the inclusion criteria for the review. Potentially relevant records will be retrieved in full and tracked through Covidence. The full text of selected citations will be assessed in detail against the inclusion criteria by one reviewer and included records will be confirmed by a second independent reviewer.
The included study lists of all eligible systematic reviews will be screened to identify potentially relevant RoB tools. All records identified through systematic reviews, the environmental scan, expert working group and PROSPERO registry search will be retrieved in full, assessed in detail against the inclusion criteria by one reviewer and confirmed by a second independent reviewer. All non-database records will be tracked using an MS Excel spreadsheet (Microsoft Corp. (2018).
Following a detailed assessment, a super-set of unique RoB tools relevant to cross-sectional studies will be created once de-duplication of records and tools across the approaches is complete.
Reasons for exclusion of full-text records that do not meet the inclusion criteria will be recorded and reported in the scoping review. Any disagreements that arise between the reviewers at each stage of the selection process will be resolved through discussion or with a third reviewer. The results of the search will be reported in full in the final scoping review and presented in a PRISMA flow diagram [ 3 ].
Data extraction
Data will be extracted from tools included in the scoping review by one reviewer and checked by a second reviewer. The data extracted will focus on descriptive information, characteristics of the tool, items and domains used to evaluate risk of bias and any psychometric properties reported, including:
- • citation, tool name;
- • reported study design(s) intended for use/application (single or multiple study designs);
- • tool development and testing: reported methods used to develop the tool, reported reliability, validity or usability testing, including whether the tool was developed or tested in a particular clinical area;
- • characteristics of the tool including but not limited to: use of domains/items/rating probes, format for completing the item or overall rating, whether rating summaries of quality or bias are used (e.g. poor/good/moderate) or if scoring is calculated;
- • discrimination between concepts of quality, risk of bias, and reporting quality;
- • how and why an existing tool was modified for application to cross-sectional studies; and,
- • declared organization supporting the tool development, any sponsors or reported funding.
Data extraction will be standardized using a framework reported for RoB tools by the National Toxicology Program of the United States Department of Health and Human Services [ 4 ]. The extraction framework focuses on key bias domains ( selection, exposure, outcome, confounding, loss-to-follow-up, analysis, selective reporting, conflict of interest and other ), the topic(s) within each domain, the questions and available response options. The extraction framework will be adapted if necessary for considerations specific to cross-sectional studies during a pilot to assess the feasibility of the process for extracting data from each included record. Any modifications will be detailed in the full scoping review. Any disagreements that arise between the reviewers will be resolved through discussion or with a third reviewer. All extracted information will be compiled into detailed evidence tables in Microsoft Excel.
Data analysis and presentation
Results will be presented in tables and figures (if applicable) and described in detail. A narrative summary will accompany the charted results and will describe how the results relate to the scoping review objective and questions. The final evidence product will be an item bank of risk of bias concepts relevant to cross-sectional studies.
This research did not receive any specific grant from funding agencies in the public, commercial, or not-for-profit sectors.
CRediT authorship contribution statement
Shannon E. Kelly: Conceptualization, Methodology, Validation, Writing – original draft, Writing – review & editing, Visualization. Karima Benkhedda: Conceptualization, Methodology, Writing – review & editing. Stephen P.J. Brooks: Conceptualization, Methodology, Writing – review & editing. Amanda J. MacFarlane: Conceptualization, Methodology, Writing – review & editing. Linda S. Greene-Finestone: Conceptualization, Methodology, Writing – review & editing. Becky Skidmore: Methodology, Validation, Writing – original draft, Writing – review & editing. Tammy J. Clifford: Supervision, Methodology, Writing – review & editing. George A. Wells: Conceptualization, Methodology, Supervision, Writing – review & editing.
Declaration of competing interest
The authors declare that they have no known competing financial interests or personal relationships that could have appeared to influence the work reported in this paper.
Supplementary material and/or additional information
Supplementary material, search strategy.
Database: Ovid MEDLINE(R) ALL 〈1946 to November 17, 2021〉
——————————————————————————–
1 Cross-Sectional Studies/ (398,684)
2 ((cross-section* or crosssection*) adj2 (study or studies or design?)).ti,kw,kf. (48,153)
3 1 or 2 [CROSS-SECTIONAL STUDIES] (412,208)
4 Cross-Sectional Studies/st [standards] (41)
5 Research Design/st [standards] (12,411)
6 Evidence-Based Medicine/mt, st [methods,standards] (9222)
7 or/4–6 [RESEARCH DESIGN STANDARDS] (21,297)
8 3 and 7 [CROSS-SECTIONAL STUDIES - RESEARCH DESIGN STANDARDS] (320)
9 exp bias/ (72,351)
10 bias$2.ti,kw,kf. (38,345)
11 ((assess* or apprais* or estimat* or grade? or grading) adj3 (bias* or quality)).tw,kw,kf. (118,427)
12 ((susceptib* or risk?) adj3 bias*).tw,kw,kf. (33,354)
13 ((rate or rated or rates or rating) adj2 quality).tw,kw,kf. (7834)
14 Research Design/ (116,838)
15 (research adj3 (appropriate* or design? or method* or protocol? or strateg* or valid*)).tw,kw,kf. (132,941)
16 (methodological* adj3 (appropriate* or consider* or decision? or design? or issue? or quality or valid*)).tw,kw,kf. (33,428)
17 ((design? or report* or study or studies) adj3 (appropriate* or quality or valid*)).tw,kw,kf. (160,543)
18 "Reproducibility of Results"/ (431,267)
19 reproducib*.ti,kw,kf. (18,259)
20 (reliabilit* or reliabl*).ti,kw,kf. (60,000)
21 (replicable or replicat*).ti,kw,kf. (58,248)
22 Valid*.ti,kw,kf. (142,161)
23 Confound*.ti,kw,kf. (5461)
24 Data Accuracy/ (3315)
25 ((calculat* or data or measur* or statistic*) adj3 (accurat* or accurac* or appropriate* or objective)).tw,kw,kf. (141,906)
26 ((calculat* or data or measur* or statistic*) adj3 (correct* or exact* or precis*)).tw,kw,kf. (50,759)
27 or/9–26 [QUALITY] (1,285,786)
28 3 and 27 [CSS - QUALITY] (38,988)
29 Checklists/ (7539)
30 (checklist* or check list*).ti,kw,kf. (8020)
31 (tool or tools).ti,kw,kf. (100,645)
32 (instrument or instruments).ti,kw,kf. (37,759)
33 (framework? or frame work?).ti,kw,kf. (58,639)
34 ((assess* or apprais* or grade? or grading or quality) adj3 (criteri* or guide or guideline? or guidance? or standard?)).ti,kw,kf. (7910)
35 or/29–34 [TOOLS/FRAMEWORKS] (215,219)
36 28 and 35 [CSS - QUALITY ASPECTS - TOOLS] (1844)
37 8 or 36 [CSS - QUALITY ASPECTS - TOOLS/STANDARDS] (2136)
38 limit 37 to yr="2010-current" (1713)
Additional information
In a cross-sectional study, a sample of individuals is selected from a larger population and data is collected from each individual in the sample. This data can then be analyzed to draw conclusions about the population as a whole ( 5 ). Researchers may use cross-sectional studies for population-based surveys, to estimate the prevalence of outcomes and/or intervention/exposures or to measure association between the intervention/exposure and outcome by calculating an odds ratio [ 5 , 6 ].
The strengths of this design are that studies can be conducted relatively more resource efficiently when compared to other study designs, they can provide information that can be used to plan subsequent research studies (hypothesis generating), as well as provide data to support planning, monitoring and evaluation of health services or public health programs [ 6 ]. They often employ survey questionnaires which can be quick and simple approaches to collect prevalence data [ 7 ]. At the population level, the resulting prevalence estimates can be informative and generalizable. However, there are several limitations associated with cross-sectional studies. Causal relationships cannot be derived from a one-time measurement of intervention/exposure and outcome and there are no temporal elements within the design to strengthen estimates or sufficiently determine trends [ 6 , 7 ]. It can be difficult to plan and conduct a high-quality cross-sectional study as this design is prone to real or potential bias related to difficulties obtaining representative samples, inaccuracies in collected information, unclear temporal relationships and the possibility of reverse causality. Like other observational study designs, a major limitation is the inability to account for unobservable confounding when associations of interest are identified [ 6 , [8] , [9] , [10] ].
When trying to answer research questions, knowledge users may use or synthesize evidence from cross-sectional studies. Including a risk-of-bias (RoB) assessment is crucial to understanding relationships among interventions, exposures and the parameters of interest, and interpreting study findings. Although several tools exist for assessing the RoB in cross-sectional studies, there is no consensus on a ‘gold standard’ tool.
Recent reviews of RoB tools have shown that existing tools may apply to one or more study design, but vary in their approach to scoring or rating, the included RoB concepts, and their response options, and variably include additional items related to generalizability, precision or reporting [ 11 , 12 ]. Previous research concluded that many RoB tools are developed explicitly for experimental research and do not adequately address key biases common in observational research [ 13 , 14 ]. Many tools developed broadly for multiple observational study designs are not specifically applicable to cross-sectional studies or fail to sufficiently assess important aspects of bias common in cross-sectional studies [ [14] , [15] , [16] ]. While multi-study RoB tools cover a breadth of methodological bias concepts, using design-specific RoB tools can help ensure that all relevant sources of bias are considered and evaluated appropriately. These design-specific tools may be more applicable, user-friendly and easier to interpret where there is no control group present [ 15 ].
Acknowledgments
The review team would like to acknowledge Beth Yetley for her early involvement and for help with conceptualization of the work.
Data availability
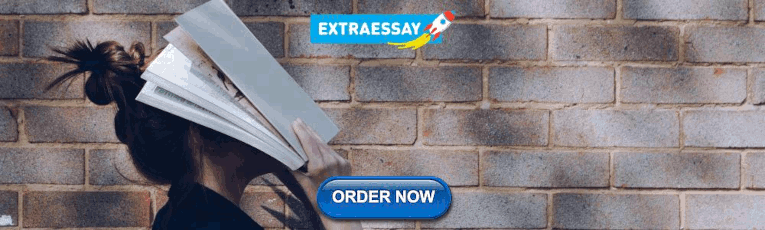
COMMENTS
Formulating Hypotheses for Different Study Designs. Generating a testable working hypothesis is the first step towards conducting original research. Such research may prove or disprove the proposed hypothesis. Case reports, case series, online surveys and other observational studies, clinical trials, and narrative reviews help to generate ...
A cross-sectional study is a type of research design in which you collect data from many different individuals at a single point in time. In cross-sectional research, you observe variables without influencing them. Researchers in economics, psychology, medicine, epidemiology, and the other social sciences all make use of cross-sectional studies ...
Cross-sectional studies. Studies with a cross-sectional study design involve the collection of information on the presence or level of one or more variables of interest (health-related characteristic), whether exposure (e.g., a risk factor) or outcome (e.g., a disease) as they exist in a defined population at one particular time.
Observational studies monitor study participants without providing study interventions. This paper describes the cross-sectional design, examines the strengths and weaknesses, and discusses some methods to report the results. Future articles will focus on other observational methods, the cohort, and case-control designs.
Hypothesis generating ... In a cross-sectional study that was published in 2019, Michelle Gosselin et al evaluated the mental health of orthopedic surgery residents. A survey was distributed electronically to program directors of orthopedic surgery residency programs in the United States and Canada. Consent was obtained electronically per ...
A cross-sectional study design is a type of observational study, or descriptive research, that involves analyzing information about a population at a specific point in time. This design measures the prevalence of an outcome of interest in a defined population. It provides a snapshot of the characteristics of the population at a single point in ...
A cross-sectional study is a type of observational research design that analyzes data from a population, or a representative subset, at one specific point in time. Unlike longitudinal studies that observe the same subjects over a period of time to detect changes, cross-sectional studies focus on finding relationships and prevalences within a ...
In medical research, epidemiology, social science, and biology, a cross-sectional study (also known as a cross-sectional analysis, transverse study, prevalence study) is a type of observational study that analyzes data from a population, or a representative subset, at a specific point in time—that is, cross-sectional data. [definition needed]In economics, cross-sectional studies typically ...
study. In medical research, a cross-sectional study is a type of observational study design that involves looking at data from a population at one specific point in time. In a cross-sectional study, investigators measure outcomes and exposures of the study subjects at the same time. It is described as taking a "snapshot.
Cross-sectional studies are observational studies that analyze data from a population at a single point in time. They are often used to measure the prevalence of health outcomes, understand determinants of health, and describe features of a population. Unlike other types of observational studies, cross-sectional studies do not follow individuals up over time. They are usually inexpensive and ...
A cross-sectional study design is used when. The purpose of the study is descriptive, often in the form of a survey. Usually there is no hypothesis as such, but the aim is to describe a population ...
3.1. Strengths: when to use cross-sectional data. The strengths of cross-sectional data help to explain their overuse in IS research. First, such studies can be conducted efficiently and inexpensively by distributing a survey to a convenient sample (e.g., the researcher's social network or students) (Compeau et al., 2012) or by using a crowdsourcing website (Lowry et al., 2016, Steelman et ...
A cross-sectional study is a good tool to generate a hypothesis, which can be subsequently tested by a case-control or a prospective study. A cross-sectional study is certainly good as a descriptive study. It is quick and easy to complete. It starts with a reference population, so generalization is immediate when based on a genuine random sample.
Abstract. Cross-sectional studies involve identifying a defined population at a given point in time and measuring a range of variables on an individual basis which can include current and past dietary exposures and well as health outcomes and disease status. They are also known as prevalence surveys.
Introduction. Cross-sectional study design is a type of observational study design. As discussed in the earlier articles, we have highlighted that in an observational study, the investigator does not alter the exposure status. The investigator measures the outcome and the exposure (s) in the population, and may study their association.
Cohort, cross sectional, and case-control studies are collectively referred to as observational studies. Often these studies are the only practicable method of studying various problems, for example, studies of aetiology, instances where a randomised controlled trial might be unethical, or if the condition to be studied is rare. Cohort studies are used to study incidence, causes, and prognosis.
Types of cross-sectional study. Descriptive A cross-sectional study may be purely descriptive and used to assess the frequency and distribution of a particular disease in a defined population. For example, a random sample of schools across London may be used to assess the burden or prevalence of asthma among 12- to 14-year-olds. Analytical
Cross-sectional studies offer information that's well-suited for descriptive analysis. If researchers want to develop a general hypothesis, then cross-sectional studies are the best way to generate specific situations that face a particular demographic. Each description of the critical data points creates the possibility of forwarding ...
Cross sectional studies are the best way to determine prevalence and are useful in identifying associations that can then be more rigorously studied using a cohort study or randomised controlled study. The most important problem with cross-sectional studies is differentiating cause and effect from simple association.
An analytical cross-sectional study is a type of quantitative, non-experimental research design. These studies seek to "gather data from a group of subjects at only one point in time" (Schmidt & Brown, 2019, p. 206). The purpose is to measure the association between an exposure and a disease, condition or outcome within a defined population.
The weaknesses of cross-sectional studies include the inability to assess incidence, to study rare diseases, and to make a causal inference. Unlike studies starting from a series of patients, cross-sectional studies often need to select a sample of subjects from a large and heterogeneous study population. Thus, they are susceptible to sampling ...
A majority of the case control studies reported in dermatology are actually analytical cross-sectional studies. Sometimes good studies have been misclassified as far as the study type is concerned. For example, in studies designated as case control studies, the cases and controls were not traced back in time. Such a study design is an example ...
The data for this cross-sectional study was collected from native speakers of Portuguese in Portugal during a face-to-face assessment in a community setting between April and June 2023. We used the STROBE Checklist for cross-sectional studies . This study was approved by the Ethics Committee of the University of Évora (Reference no. 22073) and ...
Abstract. Cross-sectional studies are commonly used to study human health and disease, but are especially susceptible to bias. This scoping review aims to identify and describe available tools to assess the risk of bias (RoB) in cross-sectional studies and to compile the key bias concepts relevant to cross-sectional studies into an item bank.