
Statistics Made Easy
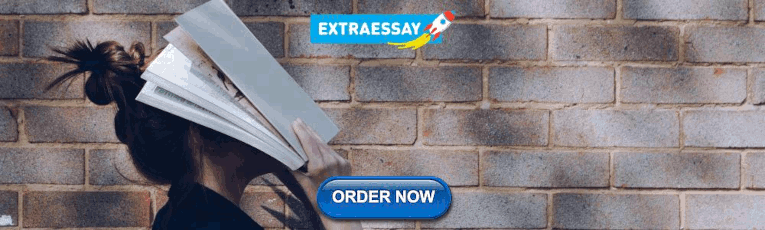
How to Write Hypothesis Test Conclusions (With Examples)
A hypothesis test is used to test whether or not some hypothesis about a population parameter is true.
To perform a hypothesis test in the real world, researchers obtain a random sample from the population and perform a hypothesis test on the sample data, using a null and alternative hypothesis:
- Null Hypothesis (H 0 ): The sample data occurs purely from chance.
- Alternative Hypothesis (H A ): The sample data is influenced by some non-random cause.
If the p-value of the hypothesis test is less than some significance level (e.g. α = .05), then we reject the null hypothesis .
Otherwise, if the p-value is not less than some significance level then we fail to reject the null hypothesis .
When writing the conclusion of a hypothesis test, we typically include:
- Whether we reject or fail to reject the null hypothesis.
- The significance level.
- A short explanation in the context of the hypothesis test.
For example, we would write:
We reject the null hypothesis at the 5% significance level. There is sufficient evidence to support the claim that…
Or, we would write:
We fail to reject the null hypothesis at the 5% significance level. There is not sufficient evidence to support the claim that…
The following examples show how to write a hypothesis test conclusion in both scenarios.
Example 1: Reject the Null Hypothesis Conclusion
Suppose a biologist believes that a certain fertilizer will cause plants to grow more during a one-month period than they normally do, which is currently 20 inches. To test this, she applies the fertilizer to each of the plants in her laboratory for one month.
She then performs a hypothesis test at a 5% significance level using the following hypotheses:
- H 0 : μ = 20 inches (the fertilizer will have no effect on the mean plant growth)
- H A : μ > 20 inches (the fertilizer will cause mean plant growth to increase)
Suppose the p-value of the test turns out to be 0.002.
Here is how she would report the results of the hypothesis test:
We reject the null hypothesis at the 5% significance level. There is sufficient evidence to support the claim that this particular fertilizer causes plants to grow more during a one-month period than they normally do.
Example 2: Fail to Reject the Null Hypothesis Conclusion
Suppose the manager of a manufacturing plant wants to test whether or not some new method changes the number of defective widgets produced per month, which is currently 250. To test this, he measures the mean number of defective widgets produced before and after using the new method for one month.
He performs a hypothesis test at a 10% significance level using the following hypotheses:
- H 0 : μ after = μ before (the mean number of defective widgets is the same before and after using the new method)
- H A : μ after ≠ μ before (the mean number of defective widgets produced is different before and after using the new method)
Suppose the p-value of the test turns out to be 0.27.
Here is how he would report the results of the hypothesis test:
We fail to reject the null hypothesis at the 10% significance level. There is not sufficient evidence to support the claim that the new method leads to a change in the number of defective widgets produced per month.
Additional Resources
The following tutorials provide additional information about hypothesis testing:
Introduction to Hypothesis Testing 4 Examples of Hypothesis Testing in Real Life How to Write a Null Hypothesis

Published by Zach
Leave a reply cancel reply.
Your email address will not be published. Required fields are marked *
How to State the Conclusion about a Hypothesis Test
After you have completed the statistical analysis and decided to reject or fail to reject the Null hypothesis, you need to state your conclusion about the claim. To get the correct wording, you need to recall which hypothesis was the claim.
If the claim was the null, then your conclusion is about whether there was sufficient evidence to reject the claim. Remember, we can never prove the null to be true, but failing to reject it is the next best thing. So, it is not correct to say, “Accept the Null.”
If the claim is the alternative hypothesis, your conclusion can be whether there was sufficient evidence to support (prove) the alternative is true.
Use the following table to help you make a good conclusion.

The best way to state the conclusion is to include the significance level of the test and a bit about the claim itself.
For example, if the claim was the alternative that the mean score on a test was greater than 85, and your decision was to Reject then Null , then you could conclude: “ At the 5% significance level, there is sufficient evidence to support the claim that the mean score on the test was greater than 85. ”
The reason you should include the significance level is that the decision, and thus the conclusion, could be different if the significance level was not 5%.
If you are curious why we say “Fail to Reject the Null” instead of “Accept the Null,” this short video might be of interest: Here
2 thoughts on “How to State the Conclusion about a Hypothesis Test”
It is concluded that the null hypothesis Ho is not rejected proportion p is greater than 0.5, at the 0.05 significance
People living in rural Idaho community live longer than 77 years
Leave a Reply Cancel reply
This site uses Akismet to reduce spam. Learn how your comment data is processed .
User Preferences
Content preview.
Arcu felis bibendum ut tristique et egestas quis:
- Ut enim ad minim veniam, quis nostrud exercitation ullamco laboris
- Duis aute irure dolor in reprehenderit in voluptate
- Excepteur sint occaecat cupidatat non proident
Keyboard Shortcuts
5.2 - writing hypotheses.
The first step in conducting a hypothesis test is to write the hypothesis statements that are going to be tested. For each test you will have a null hypothesis (\(H_0\)) and an alternative hypothesis (\(H_a\)).
When writing hypotheses there are three things that we need to know: (1) the parameter that we are testing (2) the direction of the test (non-directional, right-tailed or left-tailed), and (3) the value of the hypothesized parameter.
- At this point we can write hypotheses for a single mean (\(\mu\)), paired means(\(\mu_d\)), a single proportion (\(p\)), the difference between two independent means (\(\mu_1-\mu_2\)), the difference between two proportions (\(p_1-p_2\)), a simple linear regression slope (\(\beta\)), and a correlation (\(\rho\)).
- The research question will give us the information necessary to determine if the test is two-tailed (e.g., "different from," "not equal to"), right-tailed (e.g., "greater than," "more than"), or left-tailed (e.g., "less than," "fewer than").
- The research question will also give us the hypothesized parameter value. This is the number that goes in the hypothesis statements (i.e., \(\mu_0\) and \(p_0\)). For the difference between two groups, regression, and correlation, this value is typically 0.
Hypotheses are always written in terms of population parameters (e.g., \(p\) and \(\mu\)). The tables below display all of the possible hypotheses for the parameters that we have learned thus far. Note that the null hypothesis always includes the equality (i.e., =).
Have a language expert improve your writing
Run a free plagiarism check in 10 minutes, automatically generate references for free.
- Knowledge Base
- Methodology
- How to Write a Strong Hypothesis | Guide & Examples
How to Write a Strong Hypothesis | Guide & Examples
Published on 6 May 2022 by Shona McCombes .
A hypothesis is a statement that can be tested by scientific research. If you want to test a relationship between two or more variables, you need to write hypotheses before you start your experiment or data collection.
Table of contents
What is a hypothesis, developing a hypothesis (with example), hypothesis examples, frequently asked questions about writing hypotheses.
A hypothesis states your predictions about what your research will find. It is a tentative answer to your research question that has not yet been tested. For some research projects, you might have to write several hypotheses that address different aspects of your research question.
A hypothesis is not just a guess – it should be based on existing theories and knowledge. It also has to be testable, which means you can support or refute it through scientific research methods (such as experiments, observations, and statistical analysis of data).
Variables in hypotheses
Hypotheses propose a relationship between two or more variables . An independent variable is something the researcher changes or controls. A dependent variable is something the researcher observes and measures.
In this example, the independent variable is exposure to the sun – the assumed cause . The dependent variable is the level of happiness – the assumed effect .
Prevent plagiarism, run a free check.
Step 1: ask a question.
Writing a hypothesis begins with a research question that you want to answer. The question should be focused, specific, and researchable within the constraints of your project.
Step 2: Do some preliminary research
Your initial answer to the question should be based on what is already known about the topic. Look for theories and previous studies to help you form educated assumptions about what your research will find.
At this stage, you might construct a conceptual framework to identify which variables you will study and what you think the relationships are between them. Sometimes, you’ll have to operationalise more complex constructs.
Step 3: Formulate your hypothesis
Now you should have some idea of what you expect to find. Write your initial answer to the question in a clear, concise sentence.
Step 4: Refine your hypothesis
You need to make sure your hypothesis is specific and testable. There are various ways of phrasing a hypothesis, but all the terms you use should have clear definitions, and the hypothesis should contain:
- The relevant variables
- The specific group being studied
- The predicted outcome of the experiment or analysis
Step 5: Phrase your hypothesis in three ways
To identify the variables, you can write a simple prediction in if … then form. The first part of the sentence states the independent variable and the second part states the dependent variable.
In academic research, hypotheses are more commonly phrased in terms of correlations or effects, where you directly state the predicted relationship between variables.
If you are comparing two groups, the hypothesis can state what difference you expect to find between them.
Step 6. Write a null hypothesis
If your research involves statistical hypothesis testing , you will also have to write a null hypothesis. The null hypothesis is the default position that there is no association between the variables. The null hypothesis is written as H 0 , while the alternative hypothesis is H 1 or H a .
Hypothesis testing is a formal procedure for investigating our ideas about the world using statistics. It is used by scientists to test specific predictions, called hypotheses , by calculating how likely it is that a pattern or relationship between variables could have arisen by chance.
A hypothesis is not just a guess. It should be based on existing theories and knowledge. It also has to be testable, which means you can support or refute it through scientific research methods (such as experiments, observations, and statistical analysis of data).
A research hypothesis is your proposed answer to your research question. The research hypothesis usually includes an explanation (‘ x affects y because …’).
A statistical hypothesis, on the other hand, is a mathematical statement about a population parameter. Statistical hypotheses always come in pairs: the null and alternative hypotheses. In a well-designed study , the statistical hypotheses correspond logically to the research hypothesis.
Cite this Scribbr article
If you want to cite this source, you can copy and paste the citation or click the ‘Cite this Scribbr article’ button to automatically add the citation to our free Reference Generator.
McCombes, S. (2022, May 06). How to Write a Strong Hypothesis | Guide & Examples. Scribbr. Retrieved 2 April 2024, from https://www.scribbr.co.uk/research-methods/hypothesis-writing/
Is this article helpful?
Shona McCombes
Other students also liked, operationalisation | a guide with examples, pros & cons, what is a conceptual framework | tips & examples, a quick guide to experimental design | 5 steps & examples.
Statistical Thinking: A Simulation Approach to Modeling Uncertainty (UM STAT 216 edition)
2.14 drawing conclusions and “statistical significance”.
We have seen that statistical hypothesis testing is a process of comparing the real-world observed result to a null hypothesis where there is no effect . At the end of the process, we compare the observed result to the distribution of simulated results if the null hypothesis were true, and from this we determine whether the observed result is compatible with the null hypothesis.
The conclusions that we can draw form a hypothesis test are based on the comparison between the observed result and the null hypothesis. For example, in the Monday breakups study , we concluded:
The observed result is not compatible with the null hypothesis. This suggests that breakups may be more likely to be reported on Monday.
There are two important point to notice in how this conclusion is written:
- The conclusion is stated in terms of compatibility with the null hypothesis .
- The conclusion uses soft language like “suggests.” This is becuase we did not prove that breakups are more likely to be reported on Monday. Instead, we simply have strong evidence against the null hypothesis (that breakups are equally likely each day). This, in turn, suggests that breakups are more likely to be reported on Mondays.
Similarly, if the observed result had been within the range of likely results if the null hypothesis were true, we would still write the conclusion in terms of compatibility with the null hypothesis:
The observed result is compatible with the null hypothesis. We do not have sufficient evidence to suggest that breakups are more likely to be reported on Monday.
In both cases, notice that the conclusion is limited to whether there is an effect or not. There are many additional aspects that we might be interested in, but the hypothesis test does not tell us about. For example,
- We don’t know what caused the effect.
- We don’t know the size of the effect. Perhaps the true percentage of Monday breakups is 26%. Perhaps it is slightly more or slightly less. We only have evidence that the results are incompatible with the null hypothesis.
- We don’t know the scope of the effect. Perhaps the phenomenon is limited to this particular year, or to breakups that are reported on facebook, etc.
(We will learn about size, scope, and causation later in the course. The key point to understand now is that a hypothesis test, by itself, can not tell us about these things and so the conclusion should not address them.)
2.14.1 Statistical significance
In news reports and scientific literature, we often hear the term, “statistical significance.” What does it mean for a result to be “statistically significant?” In short, it means that the observed result is not compatible with the null hypothesis.
Different scientific communities have different standards for determining whether a result is statistically significant. In the social sciences, there are two common approaches for determining statistical significance.
- Use the range of likely results: The first approach is to determine whether the observed result is within the range of likely results if the null hypothesis were true. If the observed result is outside the range of likely values if the null hypothesis were true, then social scientists consider A second common practice is to use \(p\) -values. Commonly, social scientists consider that to be sufficient evidence that the observed result is not compatible with the null hypothesis, and thus that the observed result is statistically significant.
- Use p < 0.05: A second common approach is to use a \(p\) -value of 0.05 as a threshold. If \(p<0.05\) , social scientists consider that to be sufficient evidence that the observed result is not compatible with the null hypothesis, and thus that the observed result is statistically significant.
Other scientific communities may have different standards. Moreover, there is currently a lot of discussion about whether the current thresholds should be reconsidered, and even whether we should even have a threshold. Some scholars advocate that researchers should just report the \(p\) -value and make an argument as to whether it provides sufficient evidence against the null model.
For our class, you can use either the “range of likely values” approach, the “ \(p<0.05\) ” approach, or the “report the p-value and make an argument” approach to determining whether an observed result is statistically significant. As you become a member of a scientific community, you will learn which approaches that community uses.
2.14.2 Statistical significance vs. practical significance
Don’t confuse statistical significance with practical significance. Often, statistical significance is taken to be a indication of whether the result is meaningful in the real world (i.e., “practically significant”). But statistical significance has nothing to do with real-world importance. Remember, statistical significance just tells us whether the observed result is compatible with the null hypothesis. The question of whether the result is of real-world (or practical) significance cannot be determined statistically. Instead, this is something that people have to make an argument about.
2.14.3 Other things that statistical significance can’t tell us.
Again, statistical significance only tells us that an observed result is not compatible with the null hypothesis. It does not tell us about other important aspects, including:
- Statistical significance does not mean that we have proven something. It only tells us that the there is evidence against a null model, which in turn would suggest that the effect is real.
- Statistical significance says nothing about what caused the effect
- Statistical significance does not tell us the scope of the effect (that is, how broadly the result apply).
2.14.4 Examples
Here is how to write a conclusion to a hypothesis test.
If the result is statistically significant:
The observed result is not compatible with the null hypothesis. This suggests that there may be an effect.
If the result is not statistically significant:
The observed result is compatible with the null hypothesis. We do not have sufficient evidence to suggest that there is an effect.
2.14.5 Summary
The box below summarizes the key points about drawing conclusions and statistical significance. statistical hypothesis testing.
Key points about drawing conclusions and statistical significance
Conclusions from a hypothesis test are stated in terms of compatibility with the null hypothesis
We do not prove anything, so conclusions should use softer language like suggests
Statistical significance simply means that the observed result is not compatible with the null hypothesis
- Statistical significance does not tell us the size of the effect, or whether it is large enough to have real-world importance.

Statistics Resources
- Excel - Tutorials
- Basic Probability Rules
- Single Event Probability
- Complement Rule
- Levels of Measurement
- Independent and Dependent Variables
- Entering Data
- Central Tendency
- Data and Tests
- Displaying Data
- Discussing Statistics In-text
- SEM and Confidence Intervals
- Two-Way Frequency Tables
- Empirical Rule
- Finding Probability
- Accessing SPSS
- Chart and Graphs
- Frequency Table and Distribution
- Descriptive Statistics
- Converting Raw Scores to Z-Scores
- Converting Z-scores to t-scores
- Split File/Split Output
- Partial Eta Squared
- Downloading and Installing G*Power: Windows/PC
- Correlation
- Testing Parametric Assumptions
- One-Way ANOVA
- Two-Way ANOVA
- Repeated Measures ANOVA
- Goodness-of-Fit
- Test of Association
- Pearson's r
- Point Biserial
- Mediation and Moderation
- Simple Linear Regression
- Multiple Linear Regression
- Binomial Logistic Regression
- Multinomial Logistic Regression
- Independent Samples T-test
- Dependent Samples T-test
- Testing Assumptions
- T-tests using SPSS
- T-Test Practice
- Predictive Analytics This link opens in a new window
- Quantitative Research Questions
- Null & Alternative Hypotheses
- One-Tail vs. Two-Tail
- Alpha & Beta
- Associated Probability
- Decision Rule
- Statement of Conclusion
- Statistics Group Sessions
Statement of the Conclusion
When writing your results, you’re going to write the decision regarding the null, but you also want to state the results in layman’s terms. Tie the statistical results back to the original claim and interpret what those statistics mean, without all the quantitative jargon.
1) Claim : Females run faster than males. Results of the test : t o > t c Decision : Reject Null Hypothesis. Conclusion : There is sufficient evidence to suggest that females run faster than males.
2) Claim : There is a difference in the highest level of education obtained based on socioeconomic status. Results of the test : p > α Decision : Fail to Reject Null Hypothesis. Conclusion : There is not enough evidence to suggest that highest level of education differs based on socioeconomic status.
3) Claim : The number of calories consumed and the number of hours spent exercising each week are significant predictors of weight. Results of the test : p < α Decision : Reject Null Hypothesis. Conclusion : The results of the hypothesis test suggest that a person’s weight can be predicted given caloric intake and the number of hours spent exercising each week.
Was this resource helpful?
- << Previous: Decision Rule
- Next: Statistics Group Sessions >>
- Last Updated: Apr 2, 2024 6:35 PM
- URL: https://resources.nu.edu/statsresources

How to Write Hypothesis Test Conclusions (With Examples)
A hypothesis test is used to test whether or not some hypothesis about a population parameter is true.
To perform a hypothesis test in the real world, researchers obtain a random sample from the population and perform a hypothesis test on the sample data, using a null and alternative hypothesis:
- Null Hypothesis (H 0 ): The sample data occurs purely from chance.
- Alternative Hypothesis (H A ): The sample data is influenced by some non-random cause.
If the p-value of the hypothesis test is less than some significance level (e.g. α = .05), then we reject the null hypothesis .
Otherwise, if the p-value is not less than some significance level then we fail to reject the null hypothesis .
When writing the conclusion of a hypothesis test, we typically include:
- Whether we reject or fail to reject the null hypothesis.
- The significance level.
- A short explanation in the context of the hypothesis test.
For example, we would write:
We reject the null hypothesis at the 5% significance level. There is sufficient evidence to support the claim that…
Or, we would write:
We fail to reject the null hypothesis at the 5% significance level. There is not sufficient evidence to support the claim that…
The following examples show how to write a hypothesis test conclusion in both scenarios.
Example 1: Reject the Null Hypothesis Conclusion
Suppose a biologist believes that a certain fertilizer will cause plants to grow more during a one-month period than they normally do, which is currently 20 inches. To test this, she applies the fertilizer to each of the plants in her laboratory for one month.
She then performs a hypothesis test at a 5% significance level using the following hypotheses:
- H 0 : μ = 20 inches (the fertilizer will have no effect on the mean plant growth)
- H A : μ > 20 inches (the fertilizer will cause mean plant growth to increase)
Suppose the p-value of the test turns out to be 0.002.
Here is how she would report the results of the hypothesis test:
We reject the null hypothesis at the 5% significance level. There is sufficient evidence to support the claim that this particular fertilizer causes plants to grow more during a one-month period than they normally do.
Example 2: Fail to Reject the Null Hypothesis Conclusion
Suppose the manager of a manufacturing plant wants to test whether or not some new method changes the number of defective widgets produced per month, which is currently 250. To test this, he measures the mean number of defective widgets produced before and after using the new method for one month.
He performs a hypothesis test at a 10% significance level using the following hypotheses:
- H 0 : μ after = μ before (the mean number of defective widgets is the same before and after using the new method)
- H A : μ after ≠ μ before (the mean number of defective widgets produced is different before and after using the new method)
Suppose the p-value of the test turns out to be 0.27.
Here is how he would report the results of the hypothesis test:
We fail to reject the null hypothesis at the 10% significance level. There is not sufficient evidence to support the claim that the new method leads to a change in the number of defective widgets produced per month.
Additional Resources
The following tutorials provide additional information about hypothesis testing:
Introduction to Hypothesis Testing 4 Examples of Hypothesis Testing in Real Life How to Write a Null Hypothesis
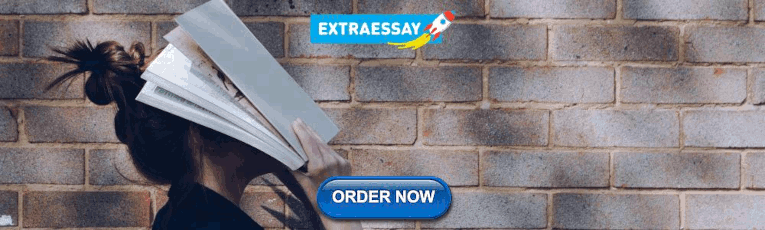
10 Examples of Using Probability in Real Life
Mongodb: how to find document by id, related posts, how to normalize data between -1 and 1, how to interpret f-values in a two-way anova, how to create a vector of ones in..., vba: how to check if string contains another..., how to determine if a probability distribution is..., what is a symmetric histogram (definition & examples), how to find the mode of a histogram..., how to find quartiles in even and odd..., how to calculate sxy in statistics (with example), how to calculate sxx in statistics (with example).
If you're seeing this message, it means we're having trouble loading external resources on our website.
If you're behind a web filter, please make sure that the domains *.kastatic.org and *.kasandbox.org are unblocked.
To log in and use all the features of Khan Academy, please enable JavaScript in your browser.
AP®︎/College Statistics
Course: ap®︎/college statistics > unit 10.
- Idea behind hypothesis testing
- Examples of null and alternative hypotheses
- Writing null and alternative hypotheses
- P-values and significance tests
- Comparing P-values to different significance levels
- Estimating a P-value from a simulation
- Estimating P-values from simulations
Using P-values to make conclusions
- (Choice A) Fail to reject H 0 A Fail to reject H 0
- (Choice B) Reject H 0 and accept H a B Reject H 0 and accept H a
- (Choice C) Accept H 0 C Accept H 0
- (Choice A) The evidence suggests that these subjects can do better than guessing when identifying the bottled water. A The evidence suggests that these subjects can do better than guessing when identifying the bottled water.
- (Choice B) We don't have enough evidence to say that these subjects can do better than guessing when identifying the bottled water. B We don't have enough evidence to say that these subjects can do better than guessing when identifying the bottled water.
- (Choice C) The evidence suggests that these subjects were simply guessing when identifying the bottled water. C The evidence suggests that these subjects were simply guessing when identifying the bottled water.
- (Choice A) She would have rejected H a . A She would have rejected H a .
- (Choice B) She would have accepted H 0 . B She would have accepted H 0 .
- (Choice C) She would have rejected H 0 and accepted H a . C She would have rejected H 0 and accepted H a .
- (Choice D) She would have reached the same conclusion using either α = 0.05 or α = 0.10 . D She would have reached the same conclusion using either α = 0.05 or α = 0.10 .
- (Choice A) The evidence suggests that these bags are being filled with a mean amount that is different than 7.4 kg . A The evidence suggests that these bags are being filled with a mean amount that is different than 7.4 kg .
- (Choice B) We don't have enough evidence to say that these bags are being filled with a mean amount that is different than 7.4 kg . B We don't have enough evidence to say that these bags are being filled with a mean amount that is different than 7.4 kg .
- (Choice C) The evidence suggests that these bags are being filled with a mean amount of 7.4 kg . C The evidence suggests that these bags are being filled with a mean amount of 7.4 kg .
- (Choice A) They would have rejected H a . A They would have rejected H a .
- (Choice B) They would have accepted H 0 . B They would have accepted H 0 .
- (Choice C) They would have failed to reject H 0 . C They would have failed to reject H 0 .
- (Choice D) They would have reached the same conclusion using either α = 0.05 or α = 0.01 . D They would have reached the same conclusion using either α = 0.05 or α = 0.01 .
Ethics and the significance level α
Want to join the conversation.
- Upvote Button navigates to signup page
- Downvote Button navigates to signup page
- Flag Button navigates to signup page

Have a language expert improve your writing
Run a free plagiarism check in 10 minutes, generate accurate citations for free.
- Knowledge Base
- Null and Alternative Hypotheses | Definitions & Examples
Null & Alternative Hypotheses | Definitions, Templates & Examples
Published on May 6, 2022 by Shaun Turney . Revised on June 22, 2023.
The null and alternative hypotheses are two competing claims that researchers weigh evidence for and against using a statistical test :
- Null hypothesis ( H 0 ): There’s no effect in the population .
- Alternative hypothesis ( H a or H 1 ) : There’s an effect in the population.
Table of contents
Answering your research question with hypotheses, what is a null hypothesis, what is an alternative hypothesis, similarities and differences between null and alternative hypotheses, how to write null and alternative hypotheses, other interesting articles, frequently asked questions.
The null and alternative hypotheses offer competing answers to your research question . When the research question asks “Does the independent variable affect the dependent variable?”:
- The null hypothesis ( H 0 ) answers “No, there’s no effect in the population.”
- The alternative hypothesis ( H a ) answers “Yes, there is an effect in the population.”
The null and alternative are always claims about the population. That’s because the goal of hypothesis testing is to make inferences about a population based on a sample . Often, we infer whether there’s an effect in the population by looking at differences between groups or relationships between variables in the sample. It’s critical for your research to write strong hypotheses .
You can use a statistical test to decide whether the evidence favors the null or alternative hypothesis. Each type of statistical test comes with a specific way of phrasing the null and alternative hypothesis. However, the hypotheses can also be phrased in a general way that applies to any test.
Receive feedback on language, structure, and formatting
Professional editors proofread and edit your paper by focusing on:
- Academic style
- Vague sentences
- Style consistency
See an example
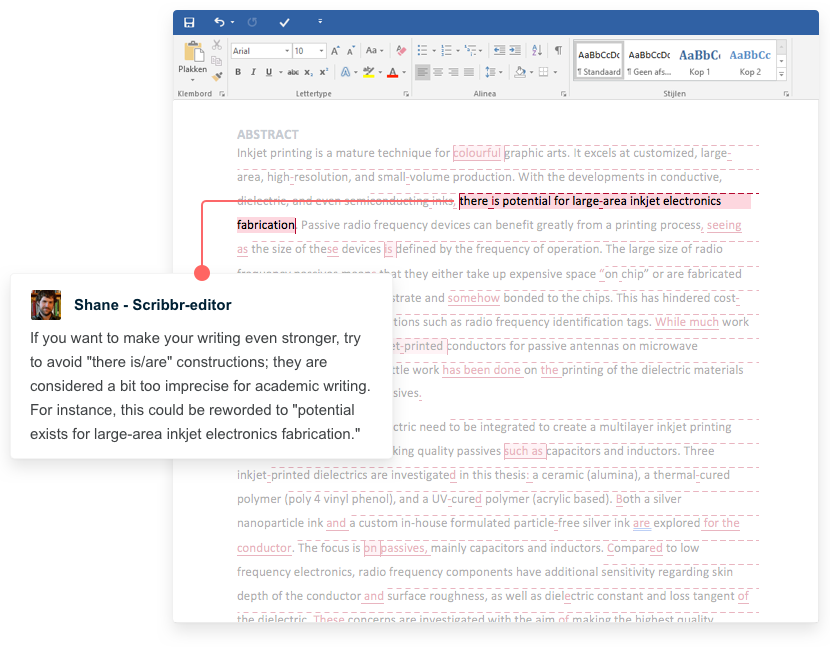
The null hypothesis is the claim that there’s no effect in the population.
If the sample provides enough evidence against the claim that there’s no effect in the population ( p ≤ α), then we can reject the null hypothesis . Otherwise, we fail to reject the null hypothesis.
Although “fail to reject” may sound awkward, it’s the only wording that statisticians accept . Be careful not to say you “prove” or “accept” the null hypothesis.
Null hypotheses often include phrases such as “no effect,” “no difference,” or “no relationship.” When written in mathematical terms, they always include an equality (usually =, but sometimes ≥ or ≤).
You can never know with complete certainty whether there is an effect in the population. Some percentage of the time, your inference about the population will be incorrect. When you incorrectly reject the null hypothesis, it’s called a type I error . When you incorrectly fail to reject it, it’s a type II error.
Examples of null hypotheses
The table below gives examples of research questions and null hypotheses. There’s always more than one way to answer a research question, but these null hypotheses can help you get started.
*Note that some researchers prefer to always write the null hypothesis in terms of “no effect” and “=”. It would be fine to say that daily meditation has no effect on the incidence of depression and p 1 = p 2 .
The alternative hypothesis ( H a ) is the other answer to your research question . It claims that there’s an effect in the population.
Often, your alternative hypothesis is the same as your research hypothesis. In other words, it’s the claim that you expect or hope will be true.
The alternative hypothesis is the complement to the null hypothesis. Null and alternative hypotheses are exhaustive, meaning that together they cover every possible outcome. They are also mutually exclusive, meaning that only one can be true at a time.
Alternative hypotheses often include phrases such as “an effect,” “a difference,” or “a relationship.” When alternative hypotheses are written in mathematical terms, they always include an inequality (usually ≠, but sometimes < or >). As with null hypotheses, there are many acceptable ways to phrase an alternative hypothesis.
Examples of alternative hypotheses
The table below gives examples of research questions and alternative hypotheses to help you get started with formulating your own.
Null and alternative hypotheses are similar in some ways:
- They’re both answers to the research question.
- They both make claims about the population.
- They’re both evaluated by statistical tests.
However, there are important differences between the two types of hypotheses, summarized in the following table.
Here's why students love Scribbr's proofreading services
Discover proofreading & editing
To help you write your hypotheses, you can use the template sentences below. If you know which statistical test you’re going to use, you can use the test-specific template sentences. Otherwise, you can use the general template sentences.
General template sentences
The only thing you need to know to use these general template sentences are your dependent and independent variables. To write your research question, null hypothesis, and alternative hypothesis, fill in the following sentences with your variables:
Does independent variable affect dependent variable ?
- Null hypothesis ( H 0 ): Independent variable does not affect dependent variable.
- Alternative hypothesis ( H a ): Independent variable affects dependent variable.
Test-specific template sentences
Once you know the statistical test you’ll be using, you can write your hypotheses in a more precise and mathematical way specific to the test you chose. The table below provides template sentences for common statistical tests.
Note: The template sentences above assume that you’re performing one-tailed tests . One-tailed tests are appropriate for most studies.
If you want to know more about statistics , methodology , or research bias , make sure to check out some of our other articles with explanations and examples.
- Normal distribution
- Descriptive statistics
- Measures of central tendency
- Correlation coefficient
Methodology
- Cluster sampling
- Stratified sampling
- Types of interviews
- Cohort study
- Thematic analysis
Research bias
- Implicit bias
- Cognitive bias
- Survivorship bias
- Availability heuristic
- Nonresponse bias
- Regression to the mean
Hypothesis testing is a formal procedure for investigating our ideas about the world using statistics. It is used by scientists to test specific predictions, called hypotheses , by calculating how likely it is that a pattern or relationship between variables could have arisen by chance.
Null and alternative hypotheses are used in statistical hypothesis testing . The null hypothesis of a test always predicts no effect or no relationship between variables, while the alternative hypothesis states your research prediction of an effect or relationship.
The null hypothesis is often abbreviated as H 0 . When the null hypothesis is written using mathematical symbols, it always includes an equality symbol (usually =, but sometimes ≥ or ≤).
The alternative hypothesis is often abbreviated as H a or H 1 . When the alternative hypothesis is written using mathematical symbols, it always includes an inequality symbol (usually ≠, but sometimes < or >).
A research hypothesis is your proposed answer to your research question. The research hypothesis usually includes an explanation (“ x affects y because …”).
A statistical hypothesis, on the other hand, is a mathematical statement about a population parameter. Statistical hypotheses always come in pairs: the null and alternative hypotheses . In a well-designed study , the statistical hypotheses correspond logically to the research hypothesis.
Cite this Scribbr article
If you want to cite this source, you can copy and paste the citation or click the “Cite this Scribbr article” button to automatically add the citation to our free Citation Generator.
Turney, S. (2023, June 22). Null & Alternative Hypotheses | Definitions, Templates & Examples. Scribbr. Retrieved April 2, 2024, from https://www.scribbr.com/statistics/null-and-alternative-hypotheses/
Is this article helpful?
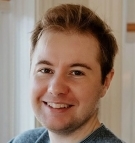
Shaun Turney
Other students also liked, inferential statistics | an easy introduction & examples, hypothesis testing | a step-by-step guide with easy examples, type i & type ii errors | differences, examples, visualizations, what is your plagiarism score.
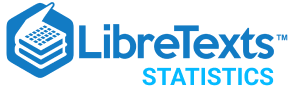
- school Campus Bookshelves
- menu_book Bookshelves
- perm_media Learning Objects
- login Login
- how_to_reg Request Instructor Account
- hub Instructor Commons
- Download Page (PDF)
- Download Full Book (PDF)
- Periodic Table
- Physics Constants
- Scientific Calculator
- Reference & Cite
- Tools expand_more
- Readability
selected template will load here
This action is not available.
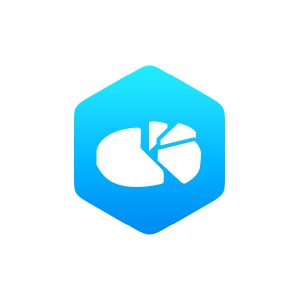
8.1: Steps in Hypothesis Testing
- Last updated
- Save as PDF
- Page ID 10970
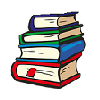
CHAPTER OBJECTIVES
By the end of this chapter, the student should be able to:
- Differentiate between Type I and Type II Errors
- Describe hypothesis testing in general and in practice
- Conduct and interpret hypothesis tests for a single population mean, population standard deviation known.
- Conduct and interpret hypothesis tests for a single population mean, population standard deviation unknown.
- Conduct and interpret hypothesis tests for a single population proportion
One job of a statistician is to make statistical inferences about populations based on samples taken from the population. Confidence intervals are one way to estimate a population parameter. Another way to make a statistical inference is to make a decision about a parameter. For instance, a car dealer advertises that its new small truck gets 35 miles per gallon, on average. A tutoring service claims that its method of tutoring helps 90% of its students get an A or a B. A company says that women managers in their company earn an average of $60,000 per year.
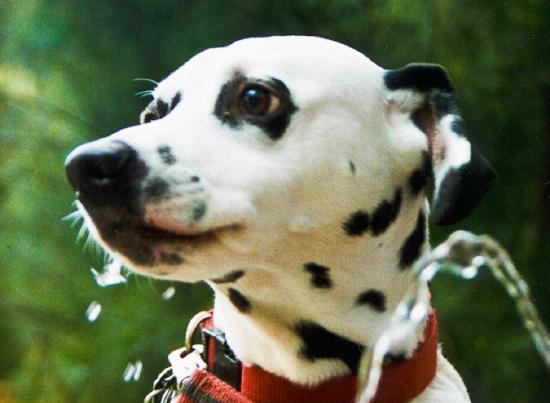
A statistician will make a decision about these claims. This process is called "hypothesis testing." A hypothesis test involves collecting data from a sample and evaluating the data. Then, the statistician makes a decision as to whether or not there is sufficient evidence, based upon analysis of the data, to reject the null hypothesis. In this chapter, you will conduct hypothesis tests on single means and single proportions. You will also learn about the errors associated with these tests.
Hypothesis testing consists of two contradictory hypotheses or statements, a decision based on the data, and a conclusion. To perform a hypothesis test, a statistician will:
- Set up two contradictory hypotheses.
- Collect sample data (in homework problems, the data or summary statistics will be given to you).
- Determine the correct distribution to perform the hypothesis test.
- Analyze sample data by performing the calculations that ultimately will allow you to reject or decline to reject the null hypothesis.
- Make a decision and write a meaningful conclusion.
To do the hypothesis test homework problems for this chapter and later chapters, make copies of the appropriate special solution sheets. See Appendix E .
- The desired confidence level.
- Information that is known about the distribution (for example, known standard deviation).
- The sample and its size.
Psychological Research
Drawing conclusions from statistics, learning objectives.
- Describe the role of random sampling and random assignment in drawing cause-and-effect conclusions
Generalizability
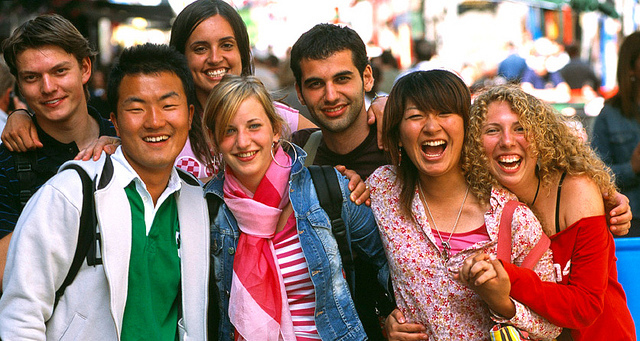
Figure 1 . Generalizability is an important research consideration: The results of studies with widely representative samples are more likely to generalize to the population. [Image: Barnacles Budget Accommodation]
One limitation to the study mentioned previously about the babies choosing the “helper” toy is that the conclusion only applies to the 16 infants in the study. We don’t know much about how those 16 infants were selected. Suppose we want to select a subset of individuals (a sample ) from a much larger group of individuals (the population ) in such a way that conclusions from the sample can be generalized to the larger population. This is the question faced by pollsters every day.
Example 1 : The General Social Survey (GSS) is a survey on societal trends conducted every other year in the United States. Based on a sample of about 2,000 adult Americans, researchers make claims about what percentage of the U.S. population consider themselves to be “liberal,” what percentage consider themselves “happy,” what percentage feel “rushed” in their daily lives, and many other issues. The key to making these claims about the larger population of all American adults lies in how the sample is selected. The goal is to select a sample that is representative of the population, and a common way to achieve this goal is to select a random sample that gives every member of the population an equal chance of being selected for the sample. In its simplest form, random sampling involves numbering every member of the population and then using a computer to randomly select the subset to be surveyed. Most polls don’t operate exactly like this, but they do use probability-based sampling methods to select individuals from nationally representative panels.
In 2004, the GSS reported that 817 of 977 respondents (or 83.6%) indicated that they always or sometimes feel rushed. This is a clear majority, but we again need to consider variation due to random sampling . Fortunately, we can use the same probability model we did in the previous example to investigate the probable size of this error. (Note, we can use the coin-tossing model when the actual population size is much, much larger than the sample size, as then we can still consider the probability to be the same for every individual in the sample.) This probability model predicts that the sample result will be within 3 percentage points of the population value (roughly 1 over the square root of the sample size, the margin of error ). A statistician would conclude, with 95% confidence, that between 80.6% and 86.6% of all adult Americans in 2004 would have responded that they sometimes or always feel rushed.
The key to the margin of error is that when we use a probability sampling method, we can make claims about how often (in the long run, with repeated random sampling) the sample result would fall within a certain distance from the unknown population value by chance (meaning by random sampling variation) alone. Conversely, non-random samples are often suspect to bias, meaning the sampling method systematically over-represents some segments of the population and under-represents others. We also still need to consider other sources of bias, such as individuals not responding honestly. These sources of error are not measured by the margin of error.
Cause and Effect
In many research studies, the primary question of interest concerns differences between groups. Then the question becomes how were the groups formed (e.g., selecting people who already drink coffee vs. those who don’t). In some studies, the researchers actively form the groups themselves. But then we have a similar question—could any differences we observe in the groups be an artifact of that group-formation process? Or maybe the difference we observe in the groups is so large that we can discount a “fluke” in the group-formation process as a reasonable explanation for what we find?
Example 2 : A psychology study investigated whether people tend to display more creativity when they are thinking about intrinsic (internal) or extrinsic (external) motivations (Ramsey & Schafer, 2002, based on a study by Amabile, 1985). The subjects were 47 people with extensive experience with creative writing. Subjects began by answering survey questions about either intrinsic motivations for writing (such as the pleasure of self-expression) or extrinsic motivations (such as public recognition). Then all subjects were instructed to write a haiku, and those poems were evaluated for creativity by a panel of judges. The researchers conjectured beforehand that subjects who were thinking about intrinsic motivations would display more creativity than subjects who were thinking about extrinsic motivations. The creativity scores from the 47 subjects in this study are displayed in Figure 2, where higher scores indicate more creativity.
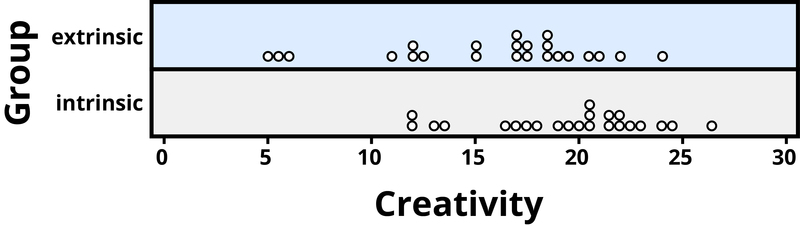
Figure 2 . Creativity scores separated by type of motivation.
In this example, the key question is whether the type of motivation affects creativity scores. In particular, do subjects who were asked about intrinsic motivations tend to have higher creativity scores than subjects who were asked about extrinsic motivations?
Figure 2 reveals that both motivation groups saw considerable variability in creativity scores, and these scores have considerable overlap between the groups. In other words, it’s certainly not always the case that those with extrinsic motivations have higher creativity than those with intrinsic motivations, but there may still be a statistical tendency in this direction. (Psychologist Keith Stanovich (2013) refers to people’s difficulties with thinking about such probabilistic tendencies as “the Achilles heel of human cognition.”)
The mean creativity score is 19.88 for the intrinsic group, compared to 15.74 for the extrinsic group, which supports the researchers’ conjecture. Yet comparing only the means of the two groups fails to consider the variability of creativity scores in the groups. We can measure variability with statistics using, for instance, the standard deviation: 5.25 for the extrinsic group and 4.40 for the intrinsic group. The standard deviations tell us that most of the creativity scores are within about 5 points of the mean score in each group. We see that the mean score for the intrinsic group lies within one standard deviation of the mean score for extrinsic group. So, although there is a tendency for the creativity scores to be higher in the intrinsic group, on average, the difference is not extremely large.
We again want to consider possible explanations for this difference. The study only involved individuals with extensive creative writing experience. Although this limits the population to which we can generalize, it does not explain why the mean creativity score was a bit larger for the intrinsic group than for the extrinsic group. Maybe women tend to receive higher creativity scores? Here is where we need to focus on how the individuals were assigned to the motivation groups. If only women were in the intrinsic motivation group and only men in the extrinsic group, then this would present a problem because we wouldn’t know if the intrinsic group did better because of the different type of motivation or because they were women. However, the researchers guarded against such a problem by randomly assigning the individuals to the motivation groups. Like flipping a coin, each individual was just as likely to be assigned to either type of motivation. Why is this helpful? Because this random assignment tends to balance out all the variables related to creativity we can think of, and even those we don’t think of in advance, between the two groups. So we should have a similar male/female split between the two groups; we should have a similar age distribution between the two groups; we should have a similar distribution of educational background between the two groups; and so on. Random assignment should produce groups that are as similar as possible except for the type of motivation, which presumably eliminates all those other variables as possible explanations for the observed tendency for higher scores in the intrinsic group.
But does this always work? No, so by “luck of the draw” the groups may be a little different prior to answering the motivation survey. So then the question is, is it possible that an unlucky random assignment is responsible for the observed difference in creativity scores between the groups? In other words, suppose each individual’s poem was going to get the same creativity score no matter which group they were assigned to, that the type of motivation in no way impacted their score. Then how often would the random-assignment process alone lead to a difference in mean creativity scores as large (or larger) than 19.88 – 15.74 = 4.14 points?
We again want to apply to a probability model to approximate a p-value , but this time the model will be a bit different. Think of writing everyone’s creativity scores on an index card, shuffling up the index cards, and then dealing out 23 to the extrinsic motivation group and 24 to the intrinsic motivation group, and finding the difference in the group means. We (better yet, the computer) can repeat this process over and over to see how often, when the scores don’t change, random assignment leads to a difference in means at least as large as 4.41. Figure 3 shows the results from 1,000 such hypothetical random assignments for these scores.
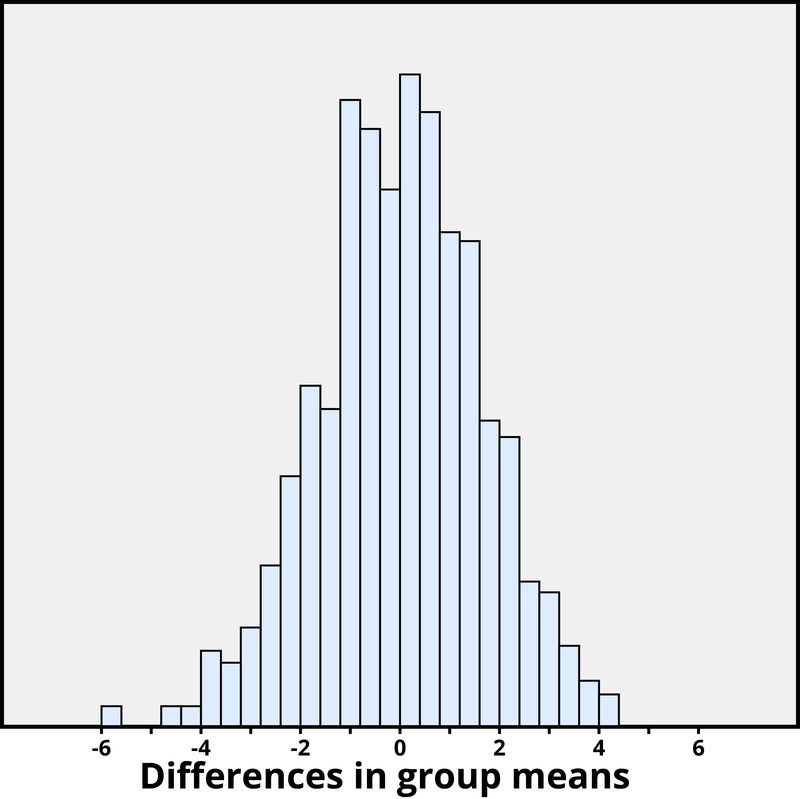
Figure 3 . Differences in group means under random assignment alone.
Only 2 of the 1,000 simulated random assignments produced a difference in group means of 4.41 or larger. In other words, the approximate p-value is 2/1000 = 0.002. This small p-value indicates that it would be very surprising for the random assignment process alone to produce such a large difference in group means. Therefore, as with Example 2, we have strong evidence that focusing on intrinsic motivations tends to increase creativity scores, as compared to thinking about extrinsic motivations.
Notice that the previous statement implies a cause-and-effect relationship between motivation and creativity score; is such a strong conclusion justified? Yes, because of the random assignment used in the study. That should have balanced out any other variables between the two groups, so now that the small p-value convinces us that the higher mean in the intrinsic group wasn’t just a coincidence, the only reasonable explanation left is the difference in the type of motivation. Can we generalize this conclusion to everyone? Not necessarily—we could cautiously generalize this conclusion to individuals with extensive experience in creative writing similar the individuals in this study, but we would still want to know more about how these individuals were selected to participate.
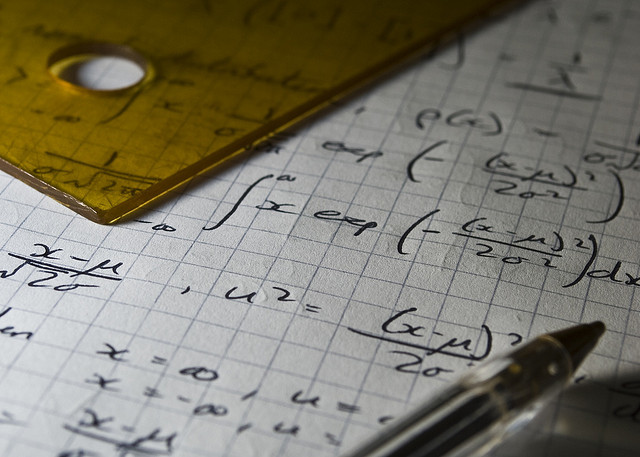
Figure 4 . Researchers employ the scientific method that involves a great deal of statistical thinking: generate a hypothesis –> design a study to test that hypothesis –> conduct the study –> analyze the data –> report the results. [Image: widdowquinn]
Statistical thinking involves the careful design of a study to collect meaningful data to answer a focused research question, detailed analysis of patterns in the data, and drawing conclusions that go beyond the observed data. Random sampling is paramount to generalizing results from our sample to a larger population, and random assignment is key to drawing cause-and-effect conclusions. With both kinds of randomness, probability models help us assess how much random variation we can expect in our results, in order to determine whether our results could happen by chance alone and to estimate a margin of error.
So where does this leave us with regard to the coffee study mentioned previously (the Freedman, Park, Abnet, Hollenbeck, & Sinha, 2012 found that men who drank at least six cups of coffee a day had a 10% lower chance of dying (women 15% lower) than those who drank none)? We can answer many of the questions:
- This was a 14-year study conducted by researchers at the National Cancer Institute.
- The results were published in the June issue of the New England Journal of Medicine , a respected, peer-reviewed journal.
- The study reviewed coffee habits of more than 402,000 people ages 50 to 71 from six states and two metropolitan areas. Those with cancer, heart disease, and stroke were excluded at the start of the study. Coffee consumption was assessed once at the start of the study.
- About 52,000 people died during the course of the study.
- People who drank between two and five cups of coffee daily showed a lower risk as well, but the amount of reduction increased for those drinking six or more cups.
- The sample sizes were fairly large and so the p-values are quite small, even though percent reduction in risk was not extremely large (dropping from a 12% chance to about 10%–11%).
- Whether coffee was caffeinated or decaffeinated did not appear to affect the results.
- This was an observational study, so no cause-and-effect conclusions can be drawn between coffee drinking and increased longevity, contrary to the impression conveyed by many news headlines about this study. In particular, it’s possible that those with chronic diseases don’t tend to drink coffee.
This study needs to be reviewed in the larger context of similar studies and consistency of results across studies, with the constant caution that this was not a randomized experiment. Whereas a statistical analysis can still “adjust” for other potential confounding variables, we are not yet convinced that researchers have identified them all or completely isolated why this decrease in death risk is evident. Researchers can now take the findings of this study and develop more focused studies that address new questions.
Explore these outside resources to learn more about applied statistics:
- Video about p-values: P-Value Extravaganza
- Interactive web applets for teaching and learning statistics
- Inter-university Consortium for Political and Social Research where you can find and analyze data.
- The Consortium for the Advancement of Undergraduate Statistics
Think It Over
- Find a recent research article in your field and answer the following: What was the primary research question? How were individuals selected to participate in the study? Were summary results provided? How strong is the evidence presented in favor or against the research question? Was random assignment used? Summarize the main conclusions from the study, addressing the issues of statistical significance, statistical confidence, generalizability, and cause and effect. Do you agree with the conclusions drawn from this study, based on the study design and the results presented?
- Is it reasonable to use a random sample of 1,000 individuals to draw conclusions about all U.S. adults? Explain why or why not.
cause-and-effect: related to whether we say one variable is causing changes in the other variable, versus other variables that may be related to these two variables. generalizability : related to whether the results from the sample can be generalized to a larger population. margin of error : the expected amount of random variation in a statistic; often defined for 95% confidence level. population : a larger collection of individuals that we would like to generalize our results to. p-value : the probability of observing a particular outcome in a sample, or more extreme, under a conjecture about the larger population or process. random assignment : using a probability-based method to divide a sample into treatment groups. random sampling : using a probability-based method to select a subset of individuals for the sample from the population. sample : the collection of individuals on which we collect data.
Contribute!
Improve this page Learn More
- Modification, adaptation, and original content. Authored by : Pat Carroll and Lumen Learning. Provided by : Lumen Learning. License : CC BY: Attribution
- Statistical Thinking. Authored by : Beth Chance and Allan Rossman, California Polytechnic State University, San Luis Obispo. Provided by : Noba. Located at : http://nobaproject.com/modules/statistical-thinking . License : CC BY-NC-SA: Attribution-NonCommercial-ShareAlike
- The Replication Crisis. Authored by : Colin Thomas William. Provided by : Ivy Tech Community College. License : CC BY: Attribution
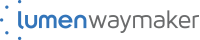
Science Fair Wizard
- Pick a topic
- Determine a problem
- Investigate your problem
- Formulate a hypothesis
Experimenting
- Design an experiment
- Test your hypothesis
- Presenting your data
- Using Statistics to Analyze Data
- Writing up your conclusions
- Re-testing your hypothesis
- Write your research paper
- Construct your exhibit
- Prepare your presentation
- Show Time! Pre-science fair checklist
- Submit your paperwork

Step 7C: Writing up your conclusions
Your conclusion should revisit the purpose of your experiment and hypothesis in light of your data analysis. Make sure you address your original question or problem when you interpret your data. Your conclusions should be valid (that is, logical) and limited to the results of the experiment.
Evaluate your data. Explain the effect of experimental error or any procedural changes on your results. Were there variables you couldn’t control for, such as age or other characteristics? Why is this information (your data analysis) important or significant? What is the relevance of your data to everyday life? Science is a process that doesn’t only try to answer a question but generates more questions. What new questions do you have as a result of your experiment?
Does your data support your hypothesis or not? If your hypothesis is incorrect, think about the reasons why this might have happened. This doesn’t mean you didn’t carry out your experiment correctly. Revisit your notes. Did you change anything about the procedure and materials that could explain what happened?
Tip: Use the worksheets you have been completing along the way to refresh your memory about your library research and procedure as you write your conclusion. These worksheets will be helpful when it is time to put together your Science Project Paper.
Scientists at Argonne National Laboratory can help you with your project. ( just ask )

The digital library project
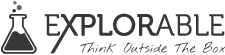
- Foundations
- Write Paper
Search form
- Experiments
- Anthropology
- Self-Esteem
- Social Anxiety

- Research Paper >
How to Write a Hypothesis
Often, one of the trickiest parts of designing and writing up any research paper is writing the hypothesis.
This article is a part of the guide:
- Outline Examples
- Example of a Paper
- Introduction
- Example of a Paper 2
Browse Full Outline
- 1 Write a Research Paper
- 2 Writing a Paper
- 3.1 Write an Outline
- 3.2 Outline Examples
- 4.1 Thesis Statement
- 4.2 Write a Hypothesis
- 5.2 Abstract
- 5.3 Introduction
- 5.4 Methods
- 5.5 Results
- 5.6 Discussion
- 5.7 Conclusion
- 5.8 Bibliography
- 6.1 Table of Contents
- 6.2 Acknowledgements
- 6.3 Appendix
- 7.1 In Text Citations
- 7.2 Footnotes
- 7.3.1 Floating Blocks
- 7.4 Example of a Paper
- 7.5 Example of a Paper 2
- 7.6.1 Citations
- 7.7.1 Writing Style
- 7.7.2 Citations
- 8.1.1 Sham Peer Review
- 8.1.2 Advantages
- 8.1.3 Disadvantages
- 8.2 Publication Bias
- 8.3.1 Journal Rejection
- 9.1 Article Writing
- 9.2 Ideas for Topics
The entire experiment revolves around the research hypothesis (H 1 ) and the null hypothesis (H 0 ), so making a mistake here could ruin the whole design .
Needless to say, it can all be a little intimidating, and many students find this to be the most difficult stage of the scientific method .
In fact, it is not as difficult as it looks, and if you have followed the steps of the scientific process and found an area of research and potential research problem , then you may already have a few ideas.
It is just about making sure that you are asking the right questions and wording your hypothesis statements correctly.
Once you have nailed down a promising hypothesis, the rest of the process will flow a lot more easily.
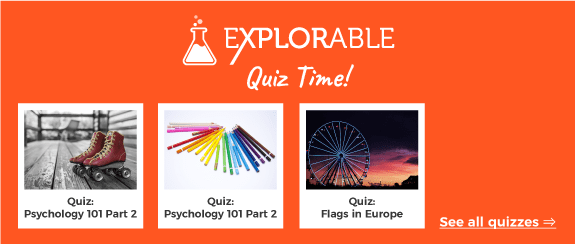
The Three-Step Process
It can quite difficult to isolate a testable hypothesis after all of the research and study. The best way is to adopt a three-step hypothesis; this will help you to narrow things down, and is the most foolproof guide to how to write a hypothesis.
Step one is to think of a general hypothesis, including everything that you have observed and reviewed during the information gathering stage of any research design . This stage is often called developing the research problem .
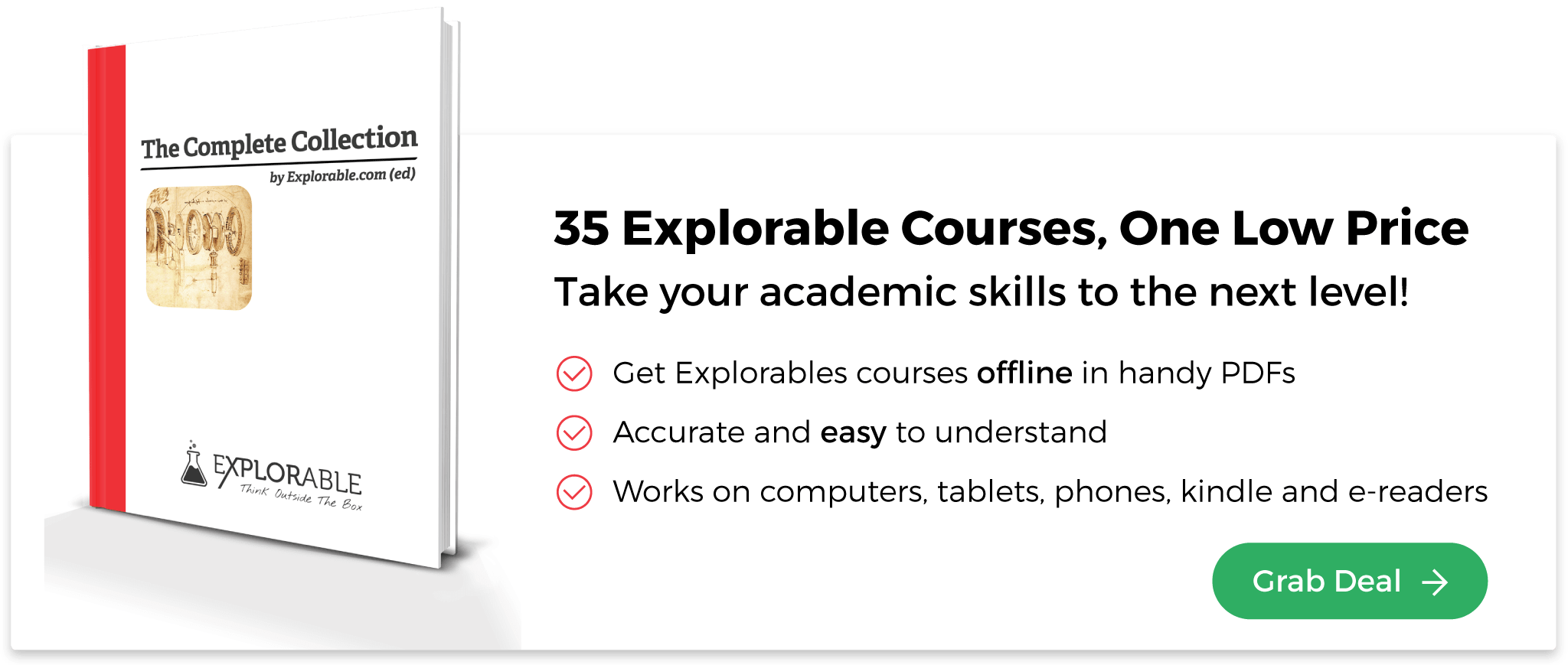
An Example of How to Write a Hypothesis
A worker on a fish-farm notices that his trout seem to have more fish lice in the summer, when the water levels are low, and wants to find out why. His research leads him to believe that the amount of oxygen is the reason - fish that are oxygen stressed tend to be more susceptible to disease and parasites.
He proposes a general hypothesis.
“Water levels affect the amount of lice suffered by rainbow trout.”
This is a good general hypothesis, but it gives no guide to how to design the research or experiment . The hypothesis must be refined to give a little direction.
“Rainbow trout suffer more lice when water levels are low.”
Now there is some directionality, but the hypothesis is not really testable , so the final stage is to design an experiment around which research can be designed, i.e. a testable hypothesis.
“Rainbow trout suffer more lice in low water conditions because there is less oxygen in the water.”
This is a testable hypothesis - he has established variables , and by measuring the amount of oxygen in the water, eliminating other controlled variables , such as temperature, he can see if there is a correlation against the number of lice on the fish.
This is an example of how a gradual focusing of research helps to define how to write a hypothesis .
The Next Stage - What to Do with the Hypothesis
Once you have your hypothesis , the next stage is to design the experiment , allowing a statistical analysis of data, and allowing you to test your hypothesis .
The statistical analysis will allow you to reject either the null or the alternative hypothesis. If the alternative is rejected, then you need to go back and refine the initial hypothesis or design a completely new research program.
This is part of the scientific process, striving for greater accuracy and developing ever more refined hypotheses.
- Psychology 101
- Flags and Countries
- Capitals and Countries
Martyn Shuttleworth (Aug 1, 2009). How to Write a Hypothesis. Retrieved Apr 03, 2024 from Explorable.com: https://explorable.com/how-to-write-a-hypothesis
You Are Allowed To Copy The Text
The text in this article is licensed under the Creative Commons-License Attribution 4.0 International (CC BY 4.0) .
This means you're free to copy, share and adapt any parts (or all) of the text in the article, as long as you give appropriate credit and provide a link/reference to this page.
That is it. You don't need our permission to copy the article; just include a link/reference back to this page. You can use it freely (with some kind of link), and we're also okay with people reprinting in publications like books, blogs, newsletters, course-material, papers, wikipedia and presentations (with clear attribution).
Want to stay up to date? Follow us!
Check out the official book.
Learn how to construct, style and format an Academic paper and take your skills to the next level.
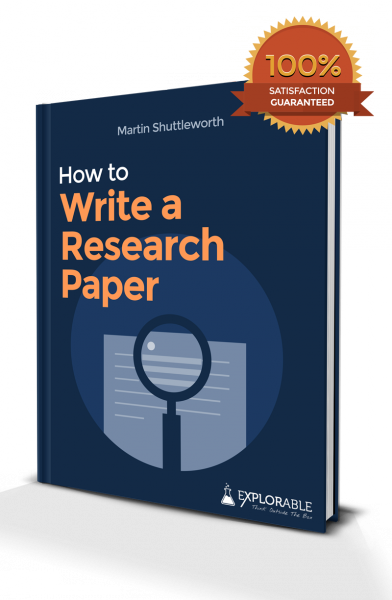
(also available as ebook )
Save this course for later
Don't have time for it all now? No problem, save it as a course and come back to it later.
Footer bottom
- Privacy Policy
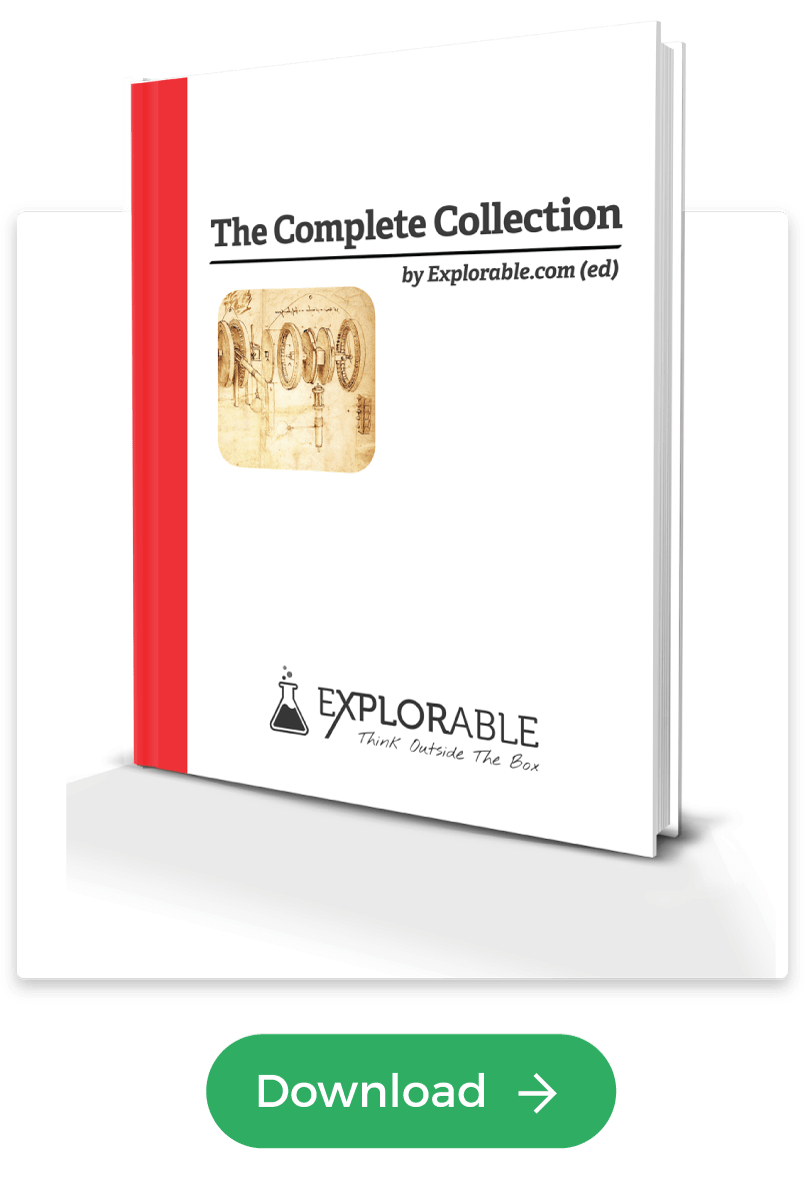
- Subscribe to our RSS Feed
- Like us on Facebook
- Follow us on Twitter
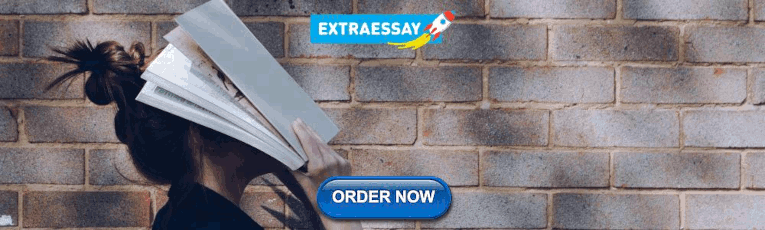
IMAGES
VIDEO
COMMENTS
When writing the conclusion of a hypothesis test, we typically include: Whether we reject or fail to reject the null hypothesis. The significance level. A short explanation in the context of the hypothesis test. For example, we would write: We reject the null hypothesis at the 5% significance level.
There are 5 main steps in hypothesis testing: State your research hypothesis as a null hypothesis and alternate hypothesis (H o) and (H a or H 1 ). Collect data in a way designed to test the hypothesis. Perform an appropriate statistical test. Decide whether to reject or fail to reject your null hypothesis. Present the findings in your results ...
5. Phrase your hypothesis in three ways. To identify the variables, you can write a simple prediction in if…then form. The first part of the sentence states the independent variable and the second part states the dependent variable. If a first-year student starts attending more lectures, then their exam scores will improve.
Use the following table to help you make a good conclusion. The best way to state the conclusion is to include the significance level of the test and a bit about the claim itself. " At the 5% significance level, there is sufficient evidence to support the claim that the mean score on the test was greater than 85.
The Four Steps in Hypothesis Testing. STEP 1: State the appropriate null and alternative hypotheses, Ho and Ha. STEP 2: Obtain a random sample, collect relevant data, and check whether the data meet the conditions under which the test can be used. If the conditions are met, summarize the data using a test statistic.
5.2 - Writing Hypotheses. The first step in conducting a hypothesis test is to write the hypothesis statements that are going to be tested. For each test you will have a null hypothesis ( H 0) and an alternative hypothesis ( H a ). When writing hypotheses there are three things that we need to know: (1) the parameter that we are testing (2) the ...
Hypothesis testing is a crucial procedure to perform when you want to make inferences about a population using a random sample. These inferences include estimating population properties such as the mean, differences between means, proportions, and the relationships between variables. This post provides an overview of statistical hypothesis testing.
To test a null hypothesis, find the p -value for the sample data and graph the results. When deciding whether or not to reject the null the hypothesis, keep these two parameters in mind: α > p − value, reject the null hypothesis. α ≤ p − value, do not reject the null hypothesis.
Step 5: Phrase your hypothesis in three ways. To identify the variables, you can write a simple prediction in if … then form. The first part of the sentence states the independent variable and the second part states the dependent variable. If a first-year student starts attending more lectures, then their exam scores will improve.
2.14 Drawing conclusions and "statistical significance". We have seen that statistical hypothesis testing is a process of comparing the real-world observed result to a null hypothesis where there is no effect.At the end of the process, we compare the observed result to the distribution of simulated results if the null hypothesis were true, and from this we determine whether the observed ...
Tie the statistical results back to the original claim and interpret what those statistics mean, without all the quantitative jargon. Examples: 1) Claim: Females run faster than males. Results of the test: t o > t c Decision: Reject Null Hypothesis. Conclusion: There is sufficient evidence to suggest that females run faster than males.
Review. In a hypothesis test, sample data is evaluated in order to arrive at a decision about some type of claim.If certain conditions about the sample are satisfied, then the claim can be evaluated for a population. In a hypothesis test, we: Evaluate the null hypothesis, typically denoted with \(H_{0}\).The null is not rejected unless the hypothesis test shows otherwise.
Unit test. Significance tests give us a formal process for using sample data to evaluate the likelihood of some claim about a population value. Learn how to conduct significance tests and calculate p-values to see how likely a sample result is to occur by random chance. You'll also see how we use p-values to make conclusions about hypotheses.
When writing the conclusion of a hypothesis test, we typically include: Whether we reject or fail to reject the null hypothesis. The significance level. A short explanation in the context of the hypothesis test. For example, we would write: We reject the null hypothesis at the 5% significance level.
Onward! We use p -values to make conclusions in significance testing. More specifically, we compare the p -value to a significance level α to make conclusions about our hypotheses. If the p -value is lower than the significance level we chose, then we reject the null hypothesis H 0 in favor of the alternative hypothesis H a .
Null hypothesis (H 0): Independent variable does not affect dependent variable. Alternative hypothesis (H a): Independent variable affects dependent variable. Test-specific template sentences. Once you know the statistical test you'll be using, you can write your hypotheses in a more precise and mathematical way specific to the test you chose ...
Step 2: State the Alternate Hypothesis. The claim is that the students have above average IQ scores, so: H 1: μ > 100. The fact that we are looking for scores "greater than" a certain point means that this is a one-tailed test. Step 3: Draw a picture to help you visualize the problem. Step 4: State the alpha level.
Collect sample data (in homework problems, the data or summary statistics will be given to you). Determine the correct distribution to perform the hypothesis test. Analyze sample data by performing the calculations that ultimately will allow you to reject or decline to reject the null hypothesis. Make a decision and write a meaningful conclusion.
Statistics: Hypothesis Testing . A hypothesis is a claim made about a population. A hypothesis test uses sample data to test the validity of the claim. This handout will define the basic elements of hypothesis testing and provide the steps to perform hypothesis tests using the P-value method and the critical value method.
Statistical thinking involves the careful design of a study to collect meaningful data to answer a focused research question, detailed analysis of patterns in the data, and drawing conclusions that go beyond the observed data. Random sampling is paramount to generalizing results from our sample to a larger population, and random assignment is ...
Presenting. Write your research paper. Construct your exhibit. Prepare your presentation. Show Time! Pre-science fair checklist. Submit your paperwork. Step 7C: Writing up your conclusions. Your conclusion should revisit the purpose of your experiment and hypothesis in light of your data analysis.
It can quite difficult to isolate a testable hypothesis after all of the research and study. The best way is to adopt a three-step hypothesis; this will help you to narrow things down, and is the most foolproof guide to how to write a hypothesis. Step one is to think of a general hypothesis, including everything that you have observed and ...