- Business Essentials
- Leadership & Management
- Credential of Leadership, Impact, and Management in Business (CLIMB)
- Entrepreneurship & Innovation
- Digital Transformation
- Finance & Accounting
- Business in Society
- For Organizations
- Support Portal
- Media Coverage
- Founding Donors
- Leadership Team
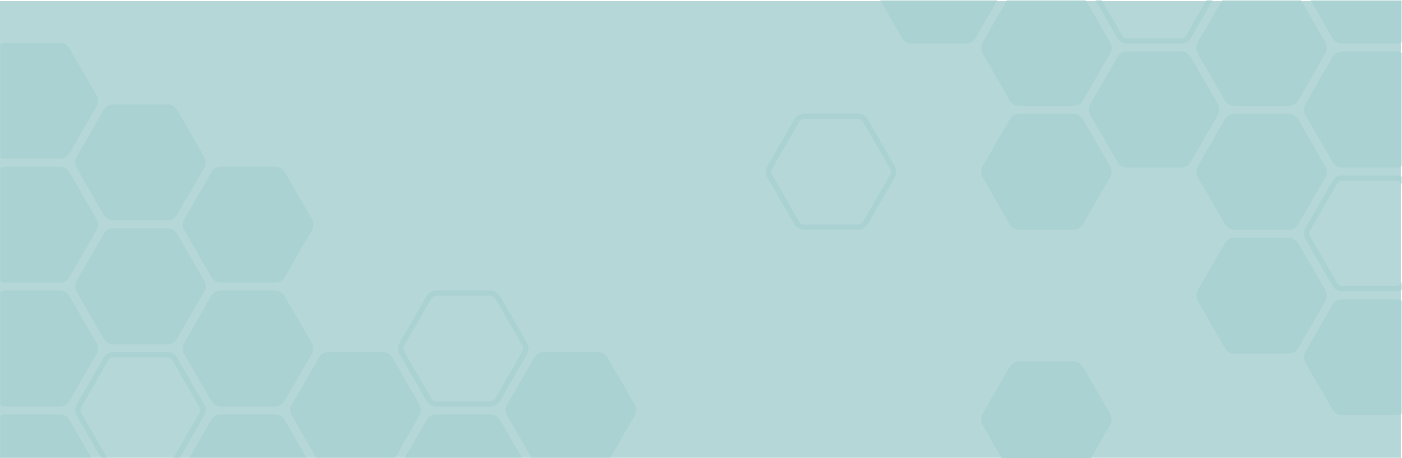
- Harvard Business School →
- HBS Online →
- Business Insights →
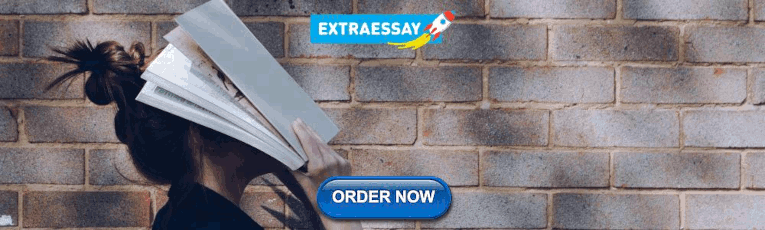
Business Insights
Harvard Business School Online's Business Insights Blog provides the career insights you need to achieve your goals and gain confidence in your business skills.
- Career Development
- Communication
- Decision-Making
- Earning Your MBA
- Negotiation
- News & Events
- Productivity
- Staff Spotlight
- Student Profiles
- Work-Life Balance
- AI Essentials for Business
- Alternative Investments
- Business Analytics
- Business Strategy
- Business and Climate Change
- Creating Brand Value
- Design Thinking and Innovation
- Digital Marketing Strategy
- Disruptive Strategy
- Economics for Managers
- Entrepreneurship Essentials
- Financial Accounting
- Global Business
- Launching Tech Ventures
- Leadership Principles
- Leadership, Ethics, and Corporate Accountability
- Leading Change and Organizational Renewal
- Leading with Finance
- Management Essentials
- Negotiation Mastery
- Organizational Leadership
- Power and Influence for Positive Impact
- Strategy Execution
- Sustainable Business Strategy
- Sustainable Investing
- Winning with Digital Platforms
7 Financial Forecasting Methods to Predict Business Performance
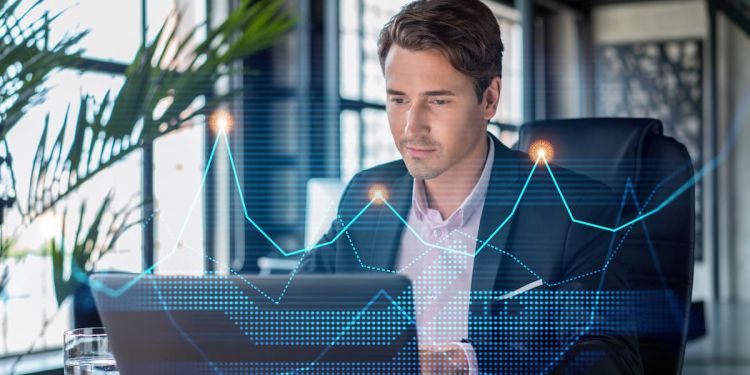
- 21 Jun 2022
Much of accounting involves evaluating past performance. Financial results demonstrate business success to both shareholders and the public. Planning and preparing for the future, however, is just as important.
Shareholders must be reassured that a business has been, and will continue to be, successful. This requires financial forecasting.
Here's an overview of how to use pro forma statements to conduct financial forecasting, along with seven methods you can leverage to predict a business's future performance.
Access your free e-book today.
What Is Financial Forecasting?
Financial forecasting is predicting a company’s financial future by examining historical performance data, such as revenue, cash flow, expenses, or sales. This involves guesswork and assumptions, as many unforeseen factors can influence business performance.
Financial forecasting is important because it informs business decision-making regarding hiring, budgeting, predicting revenue, and strategic planning . It also helps you maintain a forward-focused mindset.
Each financial forecast plays a major role in determining how much attention is given to individual expense items. For example, if you forecast high-level trends for general planning purposes, you can rely more on broad assumptions than specific details. However, if your forecast is concerned with a business’s future, such as a pending merger or acquisition, it's important to be thorough and detailed.
Forecasting with Pro Forma Statements
A common type of forecasting in financial accounting involves using pro forma statements . Pro forma statements focus on a business's future reports, which are highly dependent on assumptions made during preparation, such as expected market conditions.
Because the term "pro forma" refers to projections or forecasts, pro forma statements apply to any financial document, including:
- Income statements
- Balance sheets
- Cash flow statements
These statements serve both internal and external purposes. Internally, you can use them for strategic planning. Identifying future revenues and expenses can greatly impact business decisions related to hiring and budgeting. Pro forma statements can also inform endeavors by creating multiple statements and interchanging variables to conduct side-by-side comparisons of potential outcomes.
Externally, pro forma statements can demonstrate the risk of investing in a business. While this is an effective form of forecasting, investors should know that pro forma statements don't typically comply with generally accepted accounting principles (GAAP) . This is because pro forma statements don't include one-time expenses—such as equipment purchases or company relocations—which allows for greater accuracy because those expenses don't reflect a company’s ongoing operations.
7 Financial Forecasting Methods
Pro forma statements are incredibly valuable when forecasting revenue, expenses, and sales. These findings are often further supported by one of seven financial forecasting methods that determine future income and growth rates.
There are two primary categories of forecasting: quantitative and qualitative.
Quantitative Methods
When producing accurate forecasts, business leaders typically turn to quantitative forecasts , or assumptions about the future based on historical data.
1. Percent of Sales
Internal pro forma statements are often created using percent of sales forecasting . This method calculates future metrics of financial line items as a percentage of sales. For example, the cost of goods sold is likely to increase proportionally with sales; therefore, it’s logical to apply the same growth rate estimate to each.
To forecast the percent of sales, examine the percentage of each account’s historical profits related to sales. To calculate this, divide each account by its sales, assuming the numbers will remain steady. For example, if the cost of goods sold has historically been 30 percent of sales, assume that trend will continue.
2. Straight Line
The straight-line method assumes a company's historical growth rate will remain constant. Forecasting future revenue involves multiplying a company’s previous year's revenue by its growth rate. For example, if the previous year's growth rate was 12 percent, straight-line forecasting assumes it'll continue to grow by 12 percent next year.
Although straight-line forecasting is an excellent starting point, it doesn't account for market fluctuations or supply chain issues.
3. Moving Average
Moving average involves taking the average—or weighted average—of previous periods to forecast the future. This method involves more closely examining a business’s high or low demands, so it’s often beneficial for short-term forecasting. For example, you can use it to forecast next month’s sales by averaging the previous quarter.
Moving average forecasting can help estimate several metrics. While it’s most commonly applied to future stock prices, it’s also used to estimate future revenue.
To calculate a moving average, use the following formula:
A1 + A2 + A3 … / N
Formula breakdown:
A = Average for a period
N = Total number of periods
Using weighted averages to emphasize recent periods can increase the accuracy of moving average forecasts.
4. Simple Linear Regression
Simple linear regression forecasts metrics based on a relationship between two variables: dependent and independent. The dependent variable represents the forecasted amount, while the independent variable is the factor that influences the dependent variable.
The equation for simple linear regression is:
Y = Dependent variable (the forecasted number)
B = Regression line's slope
X = Independent variable
A = Y-intercept
5. Multiple Linear Regression
If two or more variables directly impact a company's performance, business leaders might turn to multiple linear regression . This allows for a more accurate forecast, as it accounts for several variables that ultimately influence performance.
To forecast using multiple linear regression, a linear relationship must exist between the dependent and independent variables. Additionally, the independent variables can’t be so closely correlated that it’s impossible to tell which impacts the dependent variable.

Qualitative Methods
When it comes to forecasting, numbers don't always tell the whole story. There are additional factors that influence performance and can't be quantified. Qualitative forecasting relies on experts’ knowledge and experience to predict performance rather than historical numerical data.
These forecasting methods are often called into question, as they're more subjective than quantitative methods. Yet, they can provide valuable insight into forecasts and account for factors that can’t be predicted using historical data.
6. Delphi Method
The Delphi method of forecasting involves consulting experts who analyze market conditions to predict a company's performance.
A facilitator reaches out to those experts with questionnaires, requesting forecasts of business performance based on their experience and knowledge. The facilitator then compiles their analyses and sends them to other experts for comments. The goal is to continue circulating them until a consensus is reached.
7. Market Research
Market research is essential for organizational planning. It helps business leaders obtain a holistic market view based on competition, fluctuating conditions, and consumer patterns. It’s also critical for startups when historical data isn’t available. New businesses can benefit from financial forecasting because it’s essential for recruiting investors and budgeting during the first few months of operation.
When conducting market research, begin with a hypothesis and determine what methods are needed. Sending out consumer surveys is an excellent way to better understand consumer behavior when you don’t have numerical data to inform decisions.

Improve Your Forecasting Skills
Financial forecasting is never a guarantee, but it’s critical for decision-making. Regardless of your business’s industry or stage, it’s important to maintain a forward-thinking mindset—learning from past patterns is an excellent way to plan for the future.
If you’re interested in further exploring financial forecasting and its role in business, consider taking an online course, such as Financial Accounting , to discover how to use it alongside other financial tools to shape your business.
Do you want to take your financial accounting skills to the next level? Consider enrolling in Financial Accounting —one of three courses comprising our Credential of Readiness (CORe) program —to learn how to use financial principles to inform business decisions. Not sure which course is right for you? Download our free flowchart .

About the Author
- Search Search Please fill out this field.
How Forecasting Works
Forecasting techniques, choosing the right forecasting method.
- Budgeting and Forecasting: What's The Difference?
12 Principles of Effective Forecasting
The bottom line.
- Technical Analysis
- Technical Analysis Basic Education
Forecasting: What It Is, How It’s Used in Business and Investing
:max_bytes(150000):strip_icc():format(webp)/IMG_7291_Crop-SomerAnderson-fdd793749683441487bbf0bd73328c59.jpg)
UCG/Getty Images
Forecasting is a technique that uses historical data to make informed decisions about future events or conditions. It isn't simply guessing. A tool for businesses and investors alike, forecasting takes expert analysis and applies complex models to allocate portfolios and budgets.
But just how reliable are these crystal ball-like predictions? After all, economists, investors, and financial planners frequently display a striking talent for mordant humor about the art of economic prediction. "The only function of economic forecasting," the famed 20th-century economist John Kenneth Galbraith said, "is to make astrology look respectable." Or, as an equally influential economist, Paul A. Samuelson, put it, Wall Street models "predicted nine of the last five recessions."
Key Takeaways
- Forecasting involves making predictions.
- In finance, companies use forecasting to estimate earnings or other data for later periods.
- Traders and analysts use forecasts in valuation models, to time trades, and to identify trends.
- Standard forecasting techniques include qualitative methods like expert opinion and quantitative methods like statistical models and trend analysis.
- The limits of forecasting include the uncertainty of future events and the potential for errors in data or assumptions used in the forecasting process.
Yet, forecasting is central to modern investing and business practices. Businesses hire and expand based on predicting sales figures, market demand, or economic indicators. Investors trade stocks, invest in funds, or rashly exit the market based on predictions about stock prices, interest rates, or broader market moves. However, the work of forecasting applies far beyond boardrooms and trading floors. Consumer spending patterns, job market trends, and even geopolitical events all fall under the purview of forecasters.
Keep in mind economist John Maynard Keynes's dictum that “the inevitable never happens. It is the unexpected always." We detail below the different forecasting methods and how they share a common goal: To reduce uncertainty and provide a basis for the planning we can do today. We also provide 12 principles you can apply when forecasting to get better results.
Theresa Chiechi / Investopedia
Today, forecasting blends data analysis, machine learning, statistical modeling, and expert judgment. Forecasting provides benchmarks for firms, which need a long-term perspective of operations. For example, much of the derivatives market in options and futures trading is an outgrowth of business and investor forecasting, all to hedge or insure businesses against adverse market changes that could hurt their firms.
Forecasting in Investing
Equity analysts use forecasting to predict how trends, such as gross domestic product (GDP) or unemployment, will change in the coming quarter or year. Statisticians employ forecasting to analyze the potential impact of a change in business operations. Analysts then derive earnings estimates that are often aggregated into a consensus number. If actual earnings announcements miss the estimates, it can have a large impact on a company’s stock price.
Forecasting in Business
In business management, forecasting serves as a cornerstone of strategic decisions, influencing almost every aspect of an organization's operations. By attempting to predict trends and conditions through qualitative and quantitative measures discussed below, companies aim to position themselves advantageously in the marketplace.
These predictions guide critical choices ranging from market entry strategies and product development to supply chain management and workforce planning, and so the task is often to move from forecasts to planning.
Putting Forecasts Into Action
The consequences of getting a forecast wrong can be far-reaching. Correct predictions allow businesses to improve how they divide their resources, whether they can capitalize on emerging prospects, and mitigate risks. Conversely, inaccurate forecasts can lead to misaligned strategies, inefficient use of resources, missed opportunities, and risks that weren't managed or insured for. Here are the ripple effects of forecasting on various business functions:
- Market strategy : Accurate projections of consumer demand and market trends inform which segments to target and how to pitch products and services.
- Production planning : Forecasts drive decisions on production volumes, helping to balance inventory costs with the ability to meet customer demand.
- Supply chain management : Predicting resource availability, supplier dependability, and the constraints on both is crucial for maintaining smooth operations and controlling costs.
- Human resources : Workforce planning relies heavily on forecasts for future business needs and labor conditions.
- Financial planning : Projections of revenue, costs, and market conditions underpin budgeting and investment decisions.
The consequences of poor forecasting are often severe. Companies may find themselves overextended in declining markets, struggling with excess inventory, or unable to meet unexpected surges in demand.
This is a good point to pause and review, with this table below, the differences between the two:
Forecasting vs. Planning in Business and Investing | ||
---|---|---|
To predict future outcomes based on historical data and trends | To outline specific actions and strategies to achieve specific goals | |
Primarily based on estimating what might happen | Primarily based on determining what should happen and how to make it happen | |
Can be short-term, medium-term, or long-term, depending on what's being forecast | Typically focused on the near future, but may include longer-term objectives, as in financial planning for retirement goals | |
Statistical analysis, trend extrapolation, expert judgment, simulations, etc. | Goal setting, resource allocation, budgeting, scheduling, contingency planning, etc. | |
Quantitative estimates (e.g., sales figures, stock prices, market share) and qualitative methods | Actionable plans, budgets, timelines, and performance measures | |
Predict stock prices, market trends, and economic indicators, informing investment decisions | Create investment portfolios, set financial goals, and develop strategies to attain those goals | |
Used to anticipate demand, estimate financial performance, assess market potential, and identify risks and prospects | Used to develop business strategies, allocate resources, manage operations, and review performance |
We can now explore the main methods used in forecasting, each with specific strengths and times when they're best applied:
Quantitative methods in forecasting
Quantitative forecasting techniques rely on numerical data and statistical models to predict future outcomes. These methods are particularly useful for short- to medium-term forecasts where historical data is available and patterns can be discerned.
The further out the forecast, the greater the likelihood that the forecast will be wrong.
Time Series Analysis
This method analyzes historical data points, such as sales figures or stock prices, to identify patterns or trends over time. These statistical relationships are then extrapolated into the future to generate forecasts with confidence intervals to understand the likelihood of specific outcomes. As with all forecasting methods, success is not guaranteed.
Techniques like moving averages and exponential smoothing help level out fluctuations to highlight underlying trends, making it easier to predict future values. This is at the heart of technical analysis in investing. In addition, time series forecasts often involve trend and cyclical fluctuation analysis.
Regression Analysis
Regression models assess the relationship between a dependent variable and one or more independent variables. For example, a company might use regression analysis to understand how their spending on marketing or economic conditions affects their sales. By establishing these relationships, businesses can know how to plan when marketing budgets are needed or as economic conditions change.
Econometric Models
Econometrics is a specialized field that bridges economics, mathematics, and statistics. It focuses on using statistical methods to analyze economic data and test economic theories. Econometricians develop models that quantify relationships between economic variables, such as how changes in interest rates affect investment or how government spending impacts economic growth.
Analysts use these models to predict GDP growth, inflation rates, and unemployment levels. Econometric models are particularly valuable for long-term planning and policymaking.
Quantitative models tend to share these aspects:
- Model building: Quantitative analysts create mathematical models based on economic and financial theory, incorporating variables that are believed to influence what's being studied, whether GDP or the price of a stock over time.
- Data analysis: Real-world data is collected and examined to estimate the relationships between variables.
- Hypothesis testing: Statistical tests are used to assess the validity of the model and its assumptions, determining whether the relationships identified are statistically significant. Simulations are often used at this point, whether in investing, business, or macroeconomic studies.
- Forecasting: Quantitative models can then be used to generate predictions about future economic outcomes, so decision-makers can begin planning.
Strengths and Weaknesses of Quantitative Methods in Forecasting | |
---|---|
Objective: Based on numerical data and statistical models, cutting the potential impact of personal bias | Rigid: May struggle to adapt to sudden changes or events not captured in historical data |
Consistent: Provides standardized and repeatable results, facilitating comparisons across time periods | Can miss non-quantitative information: Doesn't readily incorporate qualitative factors or expert opinions |
Quantifies relationships: Can uncover correlations and patterns within large datasets | Requires sufficient data: Relies on having enough historical data to build reliable models |
Scalable: Can be applied to large datasets and complex forecasting problems | Can be overly complex: Sophisticated models may be difficult to interpret or explain to stakeholders |
Qualitative Techniques in Forecasting
Qualitative forecasting methods rely on expert opinions and market insights rather than purely numerical data. Researchers also call this area "judgmental forecasting." Examples of qualitative forecasting models include interviews, on-site visits, market research, polls, and surveys that apply the Delphi method (which relies on aggregated expert opinions).
Gathering data for qualitative analysis can sometimes be difficult or time-consuming. The CEOs of large companies aren't going to take a phone call from a retail investor or show them around a facility. However, you can still sift through news reports and the text included in companies’ filings to get a sense of managers’ records, strategies, and philosophies. These techniques are especially valuable in situations where historical data is limited or in a period where previous data is unreliable since the market is changing.
Delphi Method
This structured technique involves a panel of experts who provide their forecasts and assumptions anonymously. Their responses are aggregated and shared with the group, followed by rounds of discussion and revision until a consensus is reached. The Delphi method is often used in all kinds of studies where expertise is needed but it's especially worthwhile for long-term strategic planning and for forecasting in uncertain or rapidly changing environments.
Market Research
Surveys, focus groups, and interviews are common tools used to gather qualitative data from customers, industry experts, and other stakeholders. This information can reveal emerging trends, shifts in consumer preferences, and other factors that might not yet be seen in numerical data.
Scenario Analysis
This technique involves developing multiple, plausible scenarios based on different assumptions about future conditions. Businesses can then assess how each scenario might impact their operations and plan accordingly. This is a crucial tool in risk management .
Strengths and Weaknesses of Qualitative Methods in Forecasting | |
---|---|
Responsive: Can adapt to changing conditions or new information | Subjective: Relies heavily on human judgment, which is subject to bias and inconsistency |
Incorporates insider information: Can take up experience and knowledge that might not be captured in numerical data | Limited backward view: Human forecasters may overlook or underemphasize certain factors because of cognitive limits or |
Can handle one-off or unusual events: Quantitative methods are highly reliant on previous data, which may leave them unprepared for unusual situations in a way that isn't the case with qualitative approaches | Short-termism: Forecasters may rely too heavily on recent events and neglect longer-term patterns or those they didn't experience first hand |
Encourages ownership: The process itself can foster buy-in from stakeholders involved | Could encourage too much ownership: While quantitative approaches can also foster a bias to defend one's conclusions, those can be checked by data as it comes in, which might not occur with qualitative forecasting |
Hybrid or Combined Approaches to Forecasting
Researchers have long been interested in which kind of methods work best and in which circumstances. Of course, different parts of finance and other disciplines tend to side toward quantitative or qualitative methods (e.g., quants are unlikely to yield studies on the gains of market research or other judgmental methods).
But researchers have often found that determining which method to use depends on a variety of factors. For instance, a company might use time series analysis to identify historical trends and supplement this with insights from market research to account for recent changes in consumer behavior.
Given this, it's no surprise that researchers have demonstrated empirically that combined or hybrid approaches often do best by pulling in the best of both worlds for more informed predictions. Neither human judgment nor quantitative methods are universally superior. Instead, their strengths are often complementary.
Flawed forecasts are commonly a central part of the story when businesses fail.
Quantitative methods do best in processing large data sets and identifying patterns in them, especially when augmented with AI and machine learning , while human judgment is best in interpreting ambiguous situations and incorporating contextual knowledge that may not be captured by data alone. Reviewing recent work in this area, we culled these key points about combining qualitative and quantitative approaches:
- Combine at the end, not the beginning : Each forecasting approach should be conducted on its own. This independence ensures that each method provides its own perspective on future outcomes, free from the influence of other forecasting techniques.
- Don't use qualitative judgments to correct for data : The last point goes hand in hand with the errors often found by using qualitative expertise to correct for quantitative data. This is the most popular way to combine approaches, but it's often the least accurate.
- Use diverse sources of information : An effective hybrid approach relies on methods that draw from distinct pools of data. For instance, statistical forecasts typically use historical data and quantitative metrics, while judgmental forecasts often incorporate qualitative insights, market sentiment, and expert intuition. This diversity in information sources enhances how robust the conclusions can be.
- Expertise matters : When incorporating judgmental forecasts, it's crucial that they derive from individuals with relevant domain expertise. This criterion acknowledges that effective judgmental forecasting requires more than general intuitions about a field. It requires a deep understanding of the specific field or industry for which the forecast is being done. In short, while it might seem fine to include experts with more general knowledge, the more granular the expertise, the better.
The correct forecasting method depends on the type and scope of the forecast. Qualitative methods are more time-consuming and costly but can make very accurate forecasts given a limited scope. For instance, they might be used to predict how well a company’s new product launch might be received by the public.
For quicker analyses that can encompass a larger scope, quantitative methods are often more useful. Looking at big data sets, statistical software packages today can crunch the numbers in a matter of minutes or seconds. However, the larger the data set and the more complex the analysis, the pricier it can be.
Thus, forecasters often perform a quick cost-benefit analysis—a mini-forecast—to determine which method will increase their chances of accurate predictions for the least cost in time and money.
Budgeting and Forecasting: What's The Difference?
While often mentioned in the same breath, budgeting and forecasting serve separate yet complementary roles in financial planning, investing, and business management. Budgeting is primarily a planning tool, so it has some of the elements seen above when we covered planning as opposed to forecasting.
Budgeting is a detailed estimate of future income and expenses so you have a road-map for allocating resources and setting performance targets. Budgets are often more static documents, representing management's commitments and expectations for the period. Here are the main characteristics of budgeting:
- Goal-oriented : Budgets reflect specific financial targets.
- Expense monitoring : They provide a basis for monitoring and controlling expenses.
- Fixed time frame : Usually covers a specific fiscal period, often one year.
- Internal focus : Primarily used for internal management and accounting.
Meanwhile, forecasting is about predicting financial outcomes based on present and historical data. So, when you forecast, you aren't setting targets. Instead, you anticipate what will happen in the future and why you do it, helping organizations and investors adjust strategies and respond to changing conditions. Here are the main characteristics of forecasting:
- Predictive : Aims to project likely outcomes rather than set targets.
- Adaptable : Regularly updated to incorporate new information.
- Various time horizons : Can cover short- or long-term periods.
- Considers external factors : Takes into account market conditions and trends, not just the internal conditions of a portfolio, business, or economic sector.
Budgeting vs. Forecasting
Purpose and focus : Budgeting sets financial targets and allocates resources
Time horizon : Typically covers a fixed period (usually one year)
Specificity : Highly detailed with specific line items
Application : Used for setting goals, controlling costs, and measuring performance
Frequency of review and adjustment : Reviewed and adjusted annually—relatively static
Purpose and focus : Forecasting predicts future financial outcomes and trends
Time horizon : Can be short-term (monthly/quarterly) or long-term
Specificity : Less detailed, focusing on broader financial trends
Application : Used for strategic planning and decisions
Frequency of review and adjustment : Reviewed and adjusted regularly (monthly/quarterly)
Effective forecasting is a critical skill in business and finance, providing a basis for the decisions that can make or break a business or portfolio. While no forecast is perfect, companies and investors with dependable forecasts are better equipped to navigate uncertainties, seize prospects, and maintain a competitive edge. The following principles, drawn from expert insights and those with practical experience, form the core of effective forecasting:
- Be methodical : The best outcomes derive from forecasting that adheres to a systematic, well-defined process. Using a systematic, repeatable approach ensures consistency, allows for continuous improvement, and improves the reliability of your predictions.
- Look back to look forward : A rule some researchers cite is looking back at least twice as far into the past as you are forecasting into the future. This simply means looking back at history. Forecasters can identify patterns that can help predict the future if they examine trends over a long period. The recent past is unreliable, so forecasters should look back at least twice as far into the past as they forecast into the future. The unexpected happens, and history doesn't always repeat itself. Be mindful of history, not a prisoner of it.
- Embrace uncertainty : Perfect forecasts are for the gods, not for you. All predictions carry a degree of uncertainty, reflecting the complex and dynamic nature of business environments.
- Quantify your uncertainty where possible : Forecasts that can provide a "distribution" of possibilities—an airline predicting the price of jet fuel between minimum and maximum likely prices, for example—help the most in planning since that doesn't make the prediction an all-or-nothing affair.
- Be aware of wild cards: These are low-probability events with high potential impact at the edges of the possible range. Acknowledging outliers and unexpected events is crucial for comprehensive forecasting and, especially, for risk management.
- Greater accuracy is found in the aggregate : Forecasts are more precise when applied to broader categories or groups rather than individual items. This principle, known as the law of large numbers, is at the heart of statistics and means that forecasts yield more reliable predictions for aggregate data.
- Take heed of the "S curve" : In data science, the S-shaped curve occurs when some process starts slowly, speeds up quickly, and then levels off. According to Silicon Valley-based forecaster Paul Saffo, recognizing this pattern early can help you anticipate different stages of development in various fields and plan accordingly. Forecasters should identify the S-curve pattern as it begins to emerge, and they can then look for precursors to an inflection point rather than the inflection point itself (when changes made might be too late).
- The longer the time, the more that can go wrong : The accuracy of forecasts typically diminishes as the time horizon extends. Near-term predictions generally offer greater precision than long-range projections. Unforeseen variables can simply compound their effects over time.
- Look for the oddballs : Embrace things that don't fit. New ideas often emerge as weak signals that can be hard to recognize because they seem strange or don't fit into existing categories. These "oddball curiosities" sometimes are indicators of future trends. For example, the sale of virtual goods in online games in the late 1990s foreshadowed the rise of virtual world commerce like Second Life. Forecasters should be tuned to these indicators, which often appear as mere curiosities or failures but can actually be forerunners of significant change.
- Hold strong views weakly : Don't fall in love with the oddballs, though. The golden rule of forecasting, as one researcher puts it, is to "be conservative" and rely only on knowledge and methods consistent with the problem at hand. This means also that forecasters should be open to new information that contradicts their initial assumptions. Sometimes, substantial evidence can be misleading, while seemingly weak evidence can signify a future trend. Forecasters should always be willing to revise or discard forecasts when contradictory evidence surfaces. If forecasters adopt a process of strong opinions, weakly held, their range of uncertainty will be refined over time toward more accurate predictions. This rule encourages continuous refining rather than relying on a single, unyielding forecast.
- Combine methods independently : When using multiple forecasting methods, ensure they are generated separately, based on different information sources, and incorporate domain expertise where relevant. Researchers have found that this makes for more accurate predictions.
- Know when not to forecast : Sometimes, the future is too uncertain to make any predictions. During periods of dramatic, rapid transformation, a good forecaster will refrain from making definitive predictions and instead look for emerging indicators that can provide clues about the future. This is just a reflection of the reality that even in times of significant change, there are often more elements that remain constant than new elements that arise. Forecasters should be aware of the limits of their knowledge and avoid making pronouncements when there's too much uncertainty.
In addition to these rules, you'll want to practice the number one principle for what to do after you put a forecast into action through planning and budgets: Regularly reassess the forecast accuracy using appropriate measures and adjust your models as needed to improve performance over time.
What Are Some Limits of Forecasting?
A major constraint on forecasting is that it involves the future, which is fundamentally unknowable. As a result, forecasts can only be educated conjectures. While there are several methods of improving the reliability of forecasts, the assumptions or data that go into the models have to be correct. Otherwise, the result will be "garbage in, garbage out." Even if the data is good, forecasting often relies on historical data, which is not guaranteed to be valid in the future, as things can and do change over time. It's also impossible to correctly factor in unusual or one-off events, like a crisis or disaster.
Can Forecasting Be Used To Predict The Stock Market?
Perfectly predicting the market's ups and downs is impossible. However, investors can use forecasts to analyze company valuations, identify growth sectors, and manage risk within their portfolios. That said, unforeseeable events always impact the market, so forecasts should be just one piece of the investment puzzle.
What's a Major Economic Prediction That Went Wrong?
The 2007-08 financial crisis stands out as a major event that seemed, to most, to occur out of nowhere. More recently, one of the most notable economic predictions that went wrong was the underestimated impact of the COVID-19 pandemic on the global economy. In early 2020, many experts and financial institutions, including the International Monetary Fund (IMF) and various central banks, initially predicted a relatively swift economic recovery following short-term disruptions. However, the prolonged nature of the pandemic, with multiple waves of infection and varying responses by different countries, led to far more severe and long-lasting economic consequences than anticipated. Then the IMF failed to predict the surge in inflation that arrived afterward.
Forecasts help managers, analysts, and investors make informed decisions about the future. Without good forecasts, many of us would be in the dark and would resort to guesses or speculation. By using qualitative and quantitative data analysis, forecasters can better understand what lies ahead.
Businesses use forecasts and projections to inform managerial decisions and capital allocations. Analysts use forecasts to estimate corporate earnings for subsequent periods. Economists may make more macro-level forecasts as well, such as predicting GDP growth or changes to employment. However, since we cannot definitively know the future, and since forecasts often rely on historical data, their accuracy will always come with some room for error—and, in some cases, may end up being way off.
Susan Ratcliffe. " Oxford Essential Quotations ." (Online Version)
Samuelson, Paul J. "Science and Stocks: Sense and Nonsense." Newsweek , p. 92.
Devon Powers. " On Trend : The Business of Forecasting the Future ," Pages 3-10. University of Illinois Press, 2019.
Nada Sanders. " Forecasting Fundamentals ," Chapter 1. Business Expert Press, 2015.
Michael Gilliland et al. " Business Forecasting: The Emerging Role of Artificial Intelligence and Machine Learning ," Pages 3-8. John Wiley & Sons, 2022.
Baker, H. Kent, et al. “ Equity Markets, Valuation, and Analysis ,” Pages 83–85. John Wiley & Sons, 2020.
Michael Gilliland et al. " Business Forecasting: The Emerging Role of Artificial Intelligence and Machine Learning ," Pages 161-185. John Wiley & Sons, 2022.
Mark Andrew Lim. " The Handbook of Technical Analysis ," Pages 3-11. John Wiley & Sons, 2015.
Mark Andrew Lim. " The Handbook of Technical Analysis ," Pages 484-485, 691. John Wiley & Sons, 2015.
International Monetary Fund. " What Is Econometrics? "
Zellner, Maximilian, et al. " A Survey of Human Judgment and Quantitative Forecasting Methods ." Royal Society: Open Science , vol. 8, no. 2 (online).
Arvan, M. et al. " Integrating Human Judgment Into Quantitative Forecasting Methods: A Review ." Omega , vol. 86 (2019), pp. 237-252.
Nada Sanders. " Forecasting Fundamentals ," Pages 51-63. Business Expert Press, 2015.
Ulrich Frische, et al. " Futures Past. Economic Forecasting in the 20th and 21st Century ," Pages 11-30. Peter Lang, 2020.
Ulrich Frische, et al. " Futures Past. Economic Forecasting in the 20th and 21st Century ," Pages 139-162. Peter Lang, 2020.
Paul Saffo. "Six Rules For Effective Forecasting ." Harvard Business Review (2007).
Fridson, Martin S., and Fernando Alvarez. " Financial Statement Analysis: A Practitioner's Guide ," Pages 225-241. John Wiley & Sons, 2022.
Armstrong, J. S. " Golden Rule of Forecasting: Be Conservative. " Journal of Business Research , vol. 68, no. 8, pp. 1717-1731.
International Monetary Fund. " How We Missed the Recent Inflation Surge ."
International Monetary Fund. " Forecasting for COVID-19 Has Failed ."
:max_bytes(150000):strip_icc():format(webp)/GettyImages-1490447956-c8e00e91cc2846ee840300f88cc5bc5c.jpg)
- Terms of Service
- Editorial Policy
- Privacy Policy

- SUGGESTED TOPICS
- The Magazine
- Newsletters
- Managing Yourself
- Managing Teams
- Work-life Balance
- The Big Idea
- Data & Visuals
- Reading Lists
- Case Selections
- HBR Learning
- Topic Feeds
- Account Settings
- Email Preferences
Six Rules for Effective Forecasting
The goal of forecasting is not to predict the future but to tell you what you need to know to take meaningful action in the present.
Reprint: R0707K
The primary goal of forecasting is to identify the full range of possibilities facing a company, society, or the world at large. In this article, Saffo demythologizes the forecasting process to help executives become sophisticated and participative consumers of forecasts, rather than passive absorbers. He illustrates how to use forecasts to at once broaden understanding of possibilities and narrow the decision space within which one must exercise intuition.
The events of 9/11, for example, were a much bigger surprise than they should have been. After all, airliners flown into monuments were the stuff of Tom Clancy novels in the 1990s, and everyone knew that terrorists had a very personal antipathy toward the World Trade Center. So why was 9/11 such a surprise? What can executives do to avoid being blindsided by other such wild cards, be they radical shifts in markets or the seemingly sudden emergence of disruptive technologies?
In describing what forecasters are trying to achieve, Saffo outlines six simple, commonsense rules that smart managers should observe as they embark on a voyage of discovery with professional forecasters. Map a cone of uncertainty, he advises, look for the S curve, embrace the things that don’t fit, hold strong opinions weakly, look back twice as far as you look forward, and know when not to make a forecast.
People at cocktail parties are always asking me for stock tips, and then they want to know how my predictions have turned out. Their requests reveal the common but fundamentally erroneous perception that forecasters make predictions. We don’t, of course: Prediction is possible only in a world in which events are preordained and no amount of action in the present can influence future outcomes. That world is the stuff of myth and superstition. The one we inhabit is quite different—little is certain, nothing is preordained, and what we do in the present affects how events unfold, often in significant, unexpected ways.
- PS Paul Saffo ( [email protected] ) is a forecaster based in Silicon Valley, in California.
Partner Center
Financial Forecasting: How to Do It with Different Methods, Models, & Software
.jpeg?width=48&height=48&name=1666292200070%20(2).jpeg)
Published: June 07, 2023
Planning for your company's future is significantly easier and more effective when you have a picture of what that future might look like. That's why any business interested in sound financial planning needs to have a grip on financial forecasting — the process of making accurate projections that can frame thoughtful, productive financial decisions in real time.
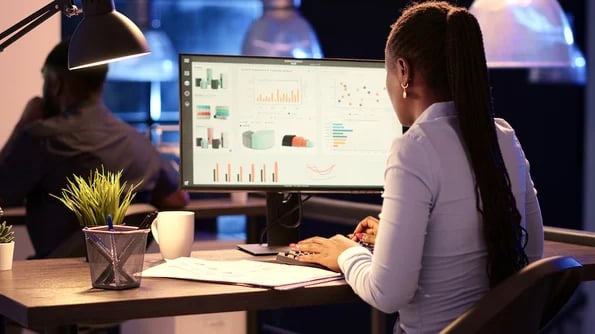
Here, we'll explore the concept of financial forecasting in depth, review some popular financial forecasting models, go over some prominent financial forecasting methods, and see some of the best financial forecasting software solutions on the market.

1. What is financial forecasting?
Forecasting vs. Budgeting
2. Financial Forecasting Models
- Top-Down Financial Forecasting
- Delphi Financial Forecasting
- Statistical Forecasting
- Bottom-Up Financial Forecasting
3. Financial Forecasting Methods
- Straight Line
- Simple Linear Regression
- Multiple Linear Regression
- Moving Average
4. How to do Financial Forecasting
5. Financial Forecasting Software
What is financial forecasting?
Financial forecasting is a process where a business leverages its current and past financial information to project its future financial performance. Forecasts are typically applied to assist with budgeting, financial modeling, and other key financial planning activities.
Financial forecasting is often conflated with the other key financial planning processes it generally informs — namely, budgeting. Though the two activities are often closely linked, it's important to differentiate between them.
The difference between a financial forecast and a budget boils down to the distinction between expectations and goals. A forecast details what a business can realistically expect to achieve over a given period.
When done correctly, it represents a reasonable estimate of how a company will likely perform — based on current and historical financial data, broader economic trends, foreseeable factors that might impact performance, and other variables that can be viably accounted for.
A budget, on the other hand, is the byproduct of a financial analysis rooted in what a business would like to achieve. It's typically updated once per year and is ultimately compared to the actual results a business sees to gauge the company's overall performance.
Now that we have a picture of what financial forecasting is, let's take a look at some of its most popular models.
Financial Forecasting Models
1. top-down financial forecasting.
Top-down forecasting is a financial forecasting model where a company starts by analyzing broader market data and ultimately whittles down company-specific revenue projections from there.
It's one of the more simple, straightforward forecasting models — essentially amounting to a company looking at its total market size and calculating potential revenue based on its assumed market share with the help of fp&a software to collect all the data you need.
Top-Down Financial Forecasting Example
Let's say a company occupies space in a market that generates an estimated $1,000,000,000 in revenue annually. If the business assumes it will have a market share of 2.5%, a top-down forecast would suggest that it will see $25,000,000 in revenue in the coming year.
Benefits of Top-Down Forecasting
- It provides a more streamlined approach for larger, established businesses with diverse revenue sources than a concentrated, product-level forecast.
- It's often the only viable forecasting avenue for early-stage companies without extensive financial data.
Drawbacks of Top-Down Forecasting
- It's often seen as hastier and more superficial than more granular forecasting methods.
- A top-down forecast is generally seen more as a starting point than a concrete projection.
2. Delphi Forecasting
The term "Delphi" here is a reference to the ancient Greek city where the Greeks consulted the mythical oracle Pythia . Fittingly, the Delphi forecasting method involves financial forecasters consulting experts for their takes on projections.
With this method, a business sends multiple rounds of questionnaires to a panel of experts, covering the company's financial data. With each new round, the experts see an aggregated summary of the previous round and adjust their perspectives accordingly. Ultimately, the hope is that a few rounds can produce a consensus among the experts that can be applied to the company's financial projections.
Delphi Financial Forecasting Example
If a company were to leverage the Delphi model, it would gather a diverse array of experts and send them questionnaires without any of them ever meeting face-to-face. After one round, the experts would each receive a summary, detailing what the other experts thought with respect to the business's potential financial performance.
The experts would be at least partially moved by the group response and submit a new questionnaire accordingly. The panel would continue to receive questionnaires until it arrived at a consensus, and the forecast would be based on that insight.
Benefits of Delphi Forecasting
- It tends to be more objective than conventional, in-house forecasting.
- Contributions are anonymous, so respondents can answer candidly.
Drawbacks of Delphi Forecasting
- The method doesn't allow for a productive, open dialogue like a face-to-face meeting would.
- Response times can be long or unpredictable, prolonging forecast delivery.
3. Statistical Forecasting
Statistical forecasting is a broad term that accounts for a variety of forecasting methods. At its core, the model is exactly what it sounds like — forecasting based on statistics. More specifically, the term is essentially a catch-all that covers forecasting rooted in the use of statistics derived from historical, quantitative data.
Statistical Financial Forecasting Example
One method that generally falls under the statistical financial forecasting umbrella is the moving average method listed below. A company might look at the revenue it generated over the past 100 days and apply that statistic to its potential performance over the next similar period.
Benefits of Statistical Forecasting
- It rests on a more solid basis than other methods.
- It can be more straightforward than other methods — provided you have the right data.
Drawbacks of Statistical Forecasting
- Certain methods that fall under this umbrella can provide relatively hasty estimates, relative to other models.
- Companies without extensive historical data might not be able to produce reliable statistical forecasts.
4. Bottom-Up Financial Forecasting
As you can probably assume, bottom-up financial forecasting is essentially the opposite of top-down forecasting — it's a model where a company starts by referencing its detailed, ground-level customer or product information and works its way up to a broader revenue projection.
Bottom-Up Financial Forecasting Example
A bottom-up financial forecast could start with a business taking a look at its sales volume — or the total number of units of its product it moved in a given period — from the previous year. Then, it would estimate the price it expects to charge for that product in the coming year. From there, it would calculate its projected revenue by multiplying the two figures.
Obviously, that example is unrealistically straightforward. In most cases, the business in question here would consider other lower-level variables as well — potentially including customer-related information like total customers or retention rate.
Benefits of Bottom-Up Forecasting
- The model allows for more detailed analysis than most others.
- It offers more room for input from various departments.
Drawbacks of Bottom-Up Forecasting
- Any errors made at the micro-level can be amplified to the macro-level with this model.
- A thorough bottom-up forecast can be time-consuming and particularly labor-intensive.
Financial Forecasting Methods
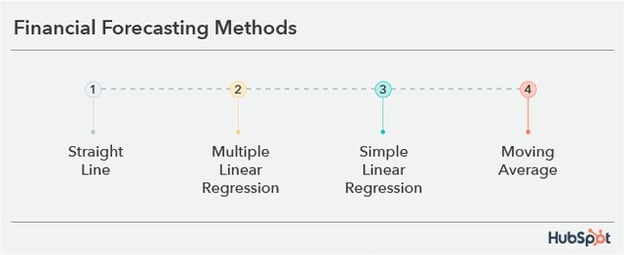
1. Straight Line
True to its name, straight line forecasting is probably the most straightforward financial forecasting method businesses can leverage. It's rooted in basic math and tends to provide rougher projections than the other, more sophisticated methods listed here.
With straight line forecasting, a business gathers rough growth estimates — typically pulled from past figures — and applies them to coming months, quarters, or years. It's generally employed when a company assumes it will see steady growth over a given period.
For instance, if your business has seen revenue reliably grow 5% year over year for the past four years, you might use that figure to guide your straight line forecasting and assume that level of growth will continue for the next few years.
2. Simple Linear Regression
The simple linear regression is a common financial forecasting method where a business explores the relationship between two variables — one independent and one dependent. For instance, a company could use this method to forecast revenue by gauging how it might be impacted by shifts in GDP.
3. Multiple Linear Regression
Simple linear regression analysis often isn't enough to make accurate financial projections, as financial performance is rarely a function of a single factor. The nature of the multiple linear regression is covered by its name — instead of trying to predict how financial performance will play out in response to a single variable, the model considers two or more independent factors.
4. Moving Average
Moving average forecasting is a method most commonly used to identify the trend-direction of a stock, but businesses can still leverage it to project their financial performance. It involves taking the arithmetic mean of a dataset from a past period and applying that average to future projections. The method is typically used to evaluate potential performance over shorter periods — like weeks, months, or quarters.
How to do Financial Forecasting
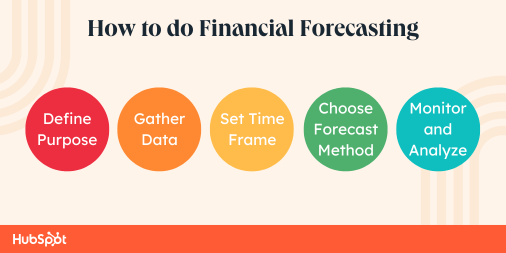
1. Define your purpose for using a financial forecast.
To get the most out of a financial forecast, you have to know why you're using it in the first place. Ask yourself questions such as:
- What are you hoping to learn and take away from its results?
- Are you trying to get a better gauge of the company budget?
- Are you trying to reach a certain goal or threshold for product sales?
When you have clear intent behind your financial forecast, you'll have a more concise and clear result to search for once you begin.
2. Gather historical data.
To track the progress of your financial forecast, you have to have a good idea of your current and past finances. Take the time to analyze your historical financial data and records, including:
- Revenue and losses
- Equity and liabilities
- Fixed costs
- Investments
- Earnings per share
Your forecast will only be as accurate as the information you collect, so get as much relevant data as possible for better results and understanding.
3. Set a time frame for your forecast.
Decide how far into the future you're committed to recording and documenting your business' financial performance. This can look like weeks, months, quarters, or even years of data collection.
It's most common for a business to conduct a forecast over the course of a fiscal year, but it's unique for every business. And if you need to adjust your forecast as time goes by, or if your goals change, you're ultimately in control and can make adjustments if need be.
4. Choose a forecasting method.
We've already give you four financial forecasting methods, so when choosing the one for your business, make sure it aligns with your previously declared purpose and goals.
5. Monitor and analyze your forecast results.
As your financial forecast delivers new data, you should monitor and analyze it differently. When you get enough data, try to think about how you can use it:
- Identify potential issues: Monitoring and analyzing financial results can help a business identify potential issues before they become more significant problems. For example, if expenses are higher than anticipated, a business can identify the cause and take corrective action to prevent it from negatively impacting financial performance.
- Measure progress towards goals: A financial forecast provides a business with financial goals and expectations. Weighing financial results against these goals enables a business to measure its progress toward achieving them. This can help the business identify where it is falling short and adjust to get back on track.
- Manage cash flow: Monitoring and analyzing financial results can give a business insights into its cash flow situation. By understanding how much cash is coming in and going out, a business can make smarter decisions about budgeting and spending.
And it doesn't have to be a tedious task to analyze your financial data, thankfully there's plenty of forecasting, decision-making and financial-planning tools available for this purpose. Let's go through some of our favorites.
Financial Forecasting Software
1. sage intacct, pricing: contact for pricing.
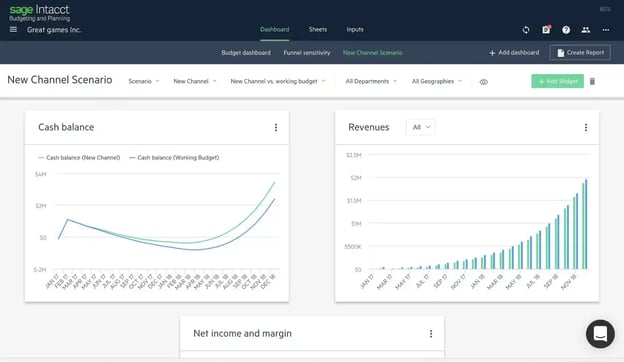
Sage Intacct is a multifaceted accounting and financial planning software with an accessible interface and a suite of features that can streamline your financial forecasting time by over 50%. The platform's automated forecasting resources effectively eliminate the stress, legwork, and room for error that often come with financial planning via spreadsheets.
Best for Collaboration
Sage Intacct separates itself from similar applications through its accessibility and room for collaboration. The software is particularly user-friendly and offers a singular, centralized solution for virtually any stakeholder within an organization to easily contribute to and make sense of financial projections.
2. PlanGuru
Pricing: plans starting at $99 per month.
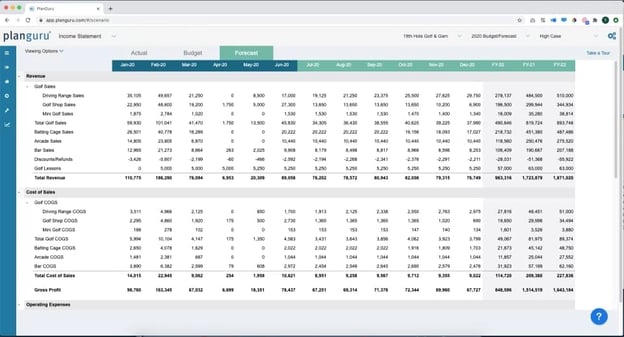
PlanGuru is a dedicated financial forecasting software — supporting 20 separate forecasting methods that can cover projections of up to 10 years. The program also allows you to incorporate non-financial data into your forecasts and has scenario analysis features to help you interpret the ramifications of potentially impactful events. PlanGuru also offers a range of plans to suit most SMBs' budgets.
Best for Pure Financial Forecasting
Some of the other resources listed here are multifaceted accounting solutions that happen to cover financial forecasting — not PlanGuru. This application is primarily dedicated to creating financial projections.
As I mentioned, it offers 20 unique financial forecasting methods to support more effective strategic planning — along with a host of other features tailored to help you gauge your future financial performance. If you're interested in a cost-effective, forecasting-specific platform, look into PlanGuru.
3. Workday Adaptive Planning
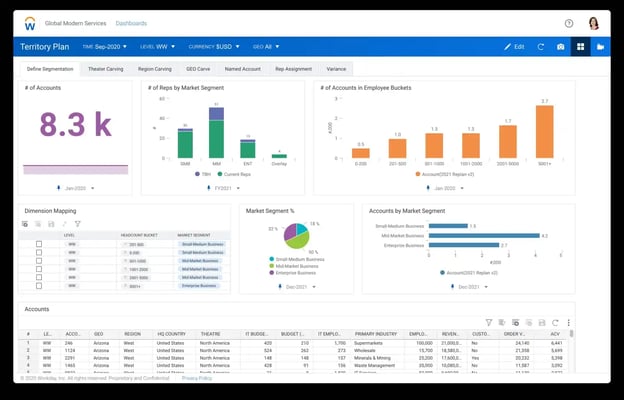
Workday Adaptive Planning provides financial forecasting resources that reconcile accessibility with powerful functionality. The software lets you leverage both real-time financial and operational data to create and compare multiple accurate, effective what-if scenario models. It also allows you to forecast across any time horizon — whether it be daily, monthly, quarterly, or long-term.
Best for a Dynamic Range of Forecasting Options
Workday Adaptive Planning's ability to support detailed bottom-up and top-down forecasts makes it a particularly attractive option for businesses of virtually any size. It allows you to create compelling forecasts based on targets from executive guidance or ground-level operational plans.
That dynamic range of forecasting options helps set the program apart from similar options. If you're interested in software that lets you forecast from various perspectives without sacrificing accuracy or effectiveness, look into Workday Adaptive Planning.
4. Limelight
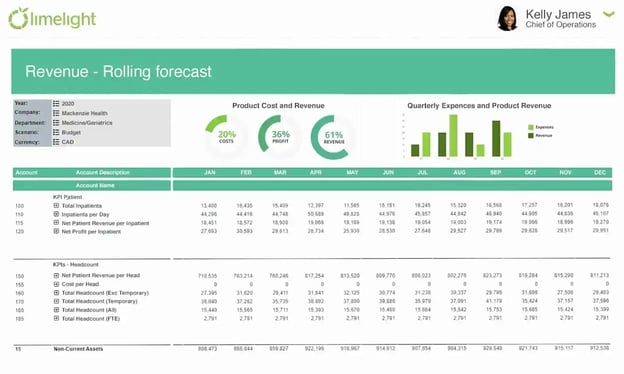
Limelight is an integrated, web-based financial planning that provides businesses with a centralized solution for almost all of their forecasting needs. Designed primarily to suit finance and accounting teams, the software offers powerful general automation and automated data integration to streamline and simplify forecasting without losing out on quality.
Best for a Familiar, Excel-Esque UX
Limelight's user experience is designed to reflect Excel — making it a familiar, particularly easy option for CFOs, controllers, budget managers, and other users to adapt to. If you're interested in a powerful forecasting resource with that kind of accessibility, Limelight might be your best option.
It's never too late to run a financial forecast.
Forecasting is a central component of sound, productive financial planning. If you have no idea what to expect financially, you'll have a hard time preparing for obstacles, setting attainable goals, and identifying aspects of your business that should be of particular interest. No matter the scale or nature of your organization, having a pulse on your financial future is always in your best interest.
Editor's note: This article was originally published in June 2022 and has been updated for comprehensiveness.
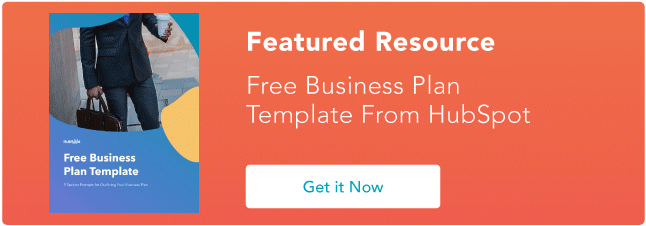
Don't forget to share this post!
Related articles.
![business planning forecasting techniques How to Build A Winning Hair Brand in 2024 [+ Hair Care Trends]](https://www.hubspot.com/hubfs/Copy%20of%20Featured%20Image%20Template%20Backgrounds%20%2840%29.png)
How to Build A Winning Hair Brand in 2024 [+ Hair Care Trends]
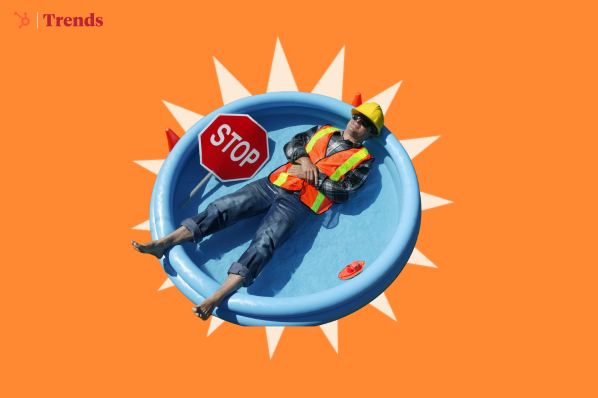
Cruel Summer: 4 Business Opportunities Inspired by The Extreme Heat
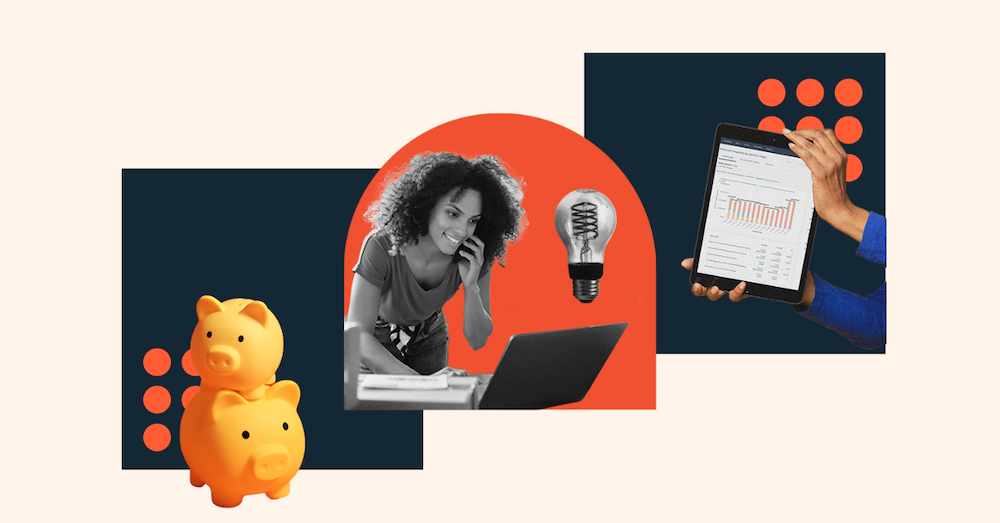
78 Stats to Know About Entrepreneurship in 2024
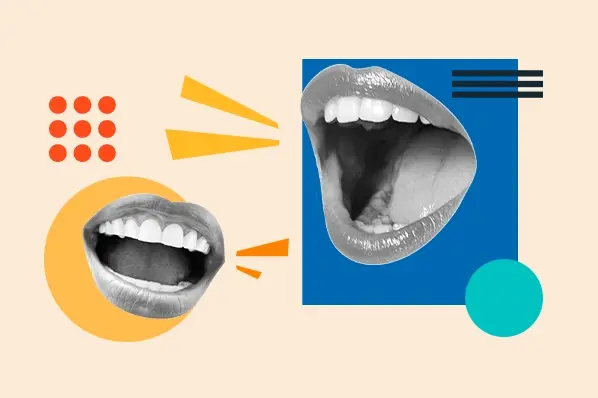
Navigating the Early Challenges of Entrepreneurship & How to Overcome Them
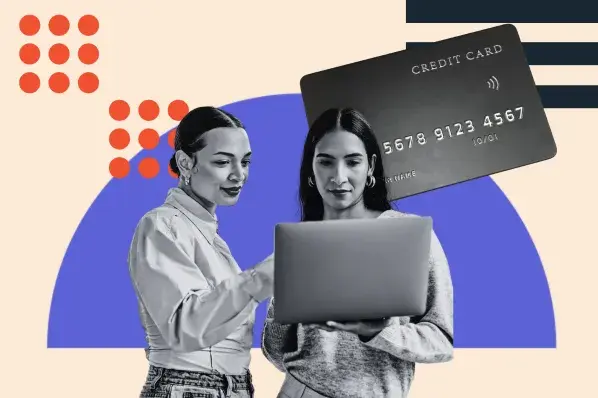
I Found 10 Best Etsy Alternatives to Sell Your Crafts
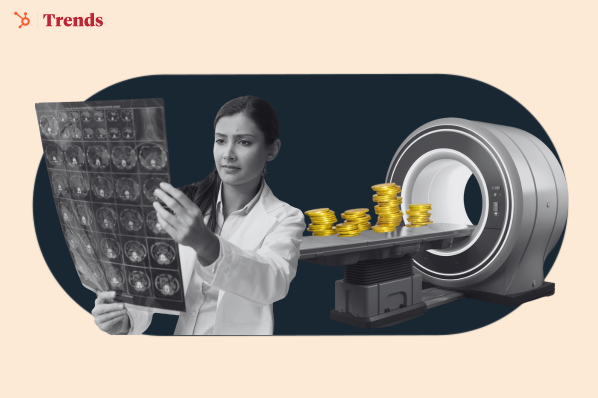
3 Business Opportunities in Imaging Boutiques & Health Concierges
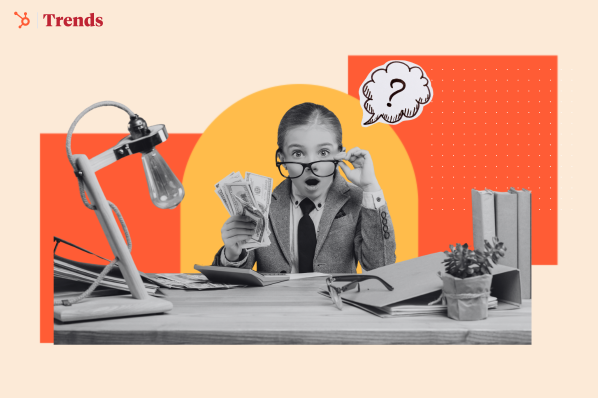
The Business of Helping Gen Alpha Manage Their Millions
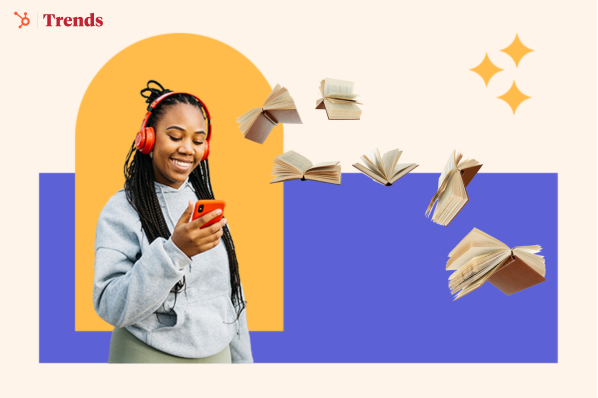
Sound Investment: Tap Into The $2 Billion Audiobook Market
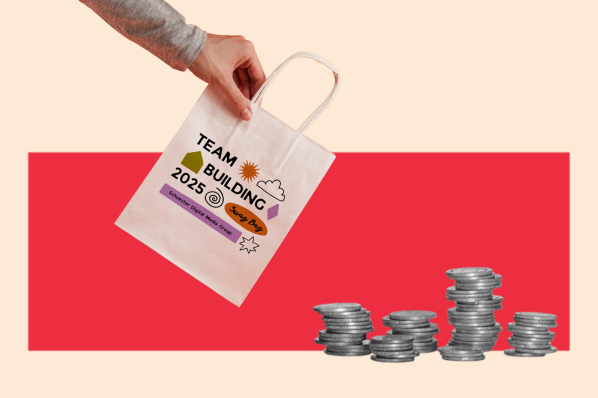
How This Swag Startup Made $3.3 Million in Year One
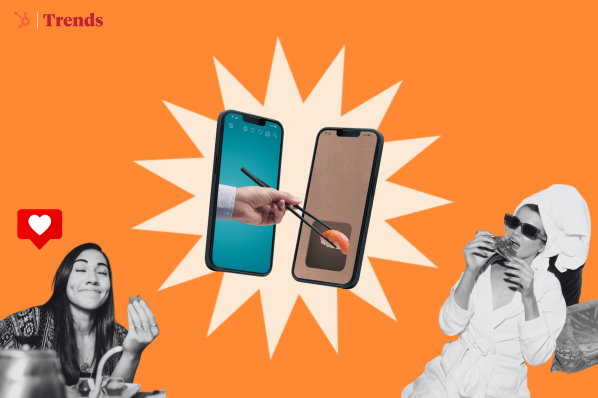
Fast and Fabulous: Tap into The Billion-Dollar Gourmet Convenience Market
2 Essential Templates For Starting Your Business
Powerful and easy-to-use sales software that drives productivity, enables customer connection, and supports growing sales orgs
Bringing a real-world edge to forecasting
CFOs know what a “good” forecasting process should look like: it should be accurate and comprehensive but flexible enough to inform a range of critical business decisions—capital reallocation, hiring, strategy, sales, production, and more.
But CFOs also recognize that there is no “typical” forecasting process: it will look different in different organizations based on sector-specific factors, feedback cycles, and, most critically, how the forecast is being used. A maker of packaged foods that is releasing new products every quarter will rely on the forecast to keep a close watch on inventory, while a mining company that is considering new plant construction over the next three years will use the forecast to predict capacity and pricing.
How do your forecasts roll?
The best predictor of satisfaction among the CFOs we surveyed was whether or not they used a “rolling” forecast—one that provides frequent updates and adjusts inputs in a predictable way as conditions change. These types of forecasts are common in a retail or software setting, where customers provide near-real-time feedback through usage, traffic, and purchasing patterns. Rolling forecasts can be used to great effect in other situations as well. An organization that maintained industrial equipment built a simple model to update its forecasts as equipment came into the shop, rather than waiting until end-of-year estimates to adjust financial figures. This helped the company avoid sudden swings in recognized revenue in a percent-complete contract model. It also put the management team on the offensive as certain contracts over- or underperformed during the year.
Data availability is typically a major inhibitor of rolling forecasts. But it doesn’t have to be. Many companies have reams of data at their fingertips but don’t know where to get started. In our survey, we found that less than half of companies use any given form of nonfinancial internal data in creating their forecasts. Only 35 percent use external market data, and only 18 percent use additional types of data like weather, traffic, and other external factors as leading indicators of the business (exhibit). With such scant inputs, it’s no surprise that forecast outputs are often underwhelming.
Of course, there is still an important place for one-time forecasting to make major decisions. When it comes to drug development, the initiation of capital projects, or the decision to enter new markets, for instance, senior leaders’ overoptimism about projects, concerns about sunk costs, and other biases can get in the way of their review of the full range of potential outcomes. In these cases, conducting an assessment against a carefully selected reference class of similar business scenarios can produce valuable insights.
What’s more, companies’ access to ever-larger data sets continues to complicate the forecasting process as much as it enlightens it, leading to even more variety in how forecasts are built. Many of the 130 CFOs we surveyed in a recent study 1 We polled 130 CFOs—90 from small and medium-size companies (less than $200 million in revenue) and 40 from large companies (greater than $1 billion in revenue). Half of them were members of the CFO Leadership Council, a North American professional association of finance executives. The research base included companies from a range of sectors, including technology, healthcare, and industrials. say they now run more than one type of forecasting process in their organizations—rolling forecasts to manage the business, and ad hoc processes to make specific decisions (see sidebar, “How do your forecasts roll?”).
But while many of the CFOs we surveyed expressed general satisfaction with the results of their forecasting efforts (exhibit), some 40 percent also told us that their forecasts are not particularly accurate and that the process takes far too much time.
That’s likely because they use financial measures rather than operational outcomes as indicators of forecasting effectiveness—if they review the success of their forecasting efforts at all. A focus only on financial inputs can mask big issues with companies’ forecasting processes. By contrast, incorporating real-world operations insights into the financial-forecasting process can help CFOs and finance teams predict bottom-line issues early, based on a careful assessment of quality, operations, and customer-retention measurements. Senior leaders can then address performance issues before they become big problems, and the incentives of even the smallest subunit of the business would be targeted toward long-term value creation.
Integrating operations data within forecasts won’t be easy, of course. Finance and business leaders will need to let go of traditional budgeting mindsets and explore new ways of working together. The good news? Automation and other digital technologies now make that easier. And four criteria show promise for injecting more accuracy and reliability into forecasting models, regardless of industry: build a momentum case separate from the business plan, use a variety of operational and external inputs, automate the forecast, and measure effectiveness with a fine-grained level of detail.
Building a better forecast
The typical forecasting process follows a pattern that contributes to inaccurate projections and a defeating, self-reinforcing cycle.
At one large industrial manufacturing and services company, for instance, managers in the business units and subunits are held to earnings targets that are rolled up into the overarching forecast. Over the years, these managers have become adept at finding the one or two things that will help them make their number, often at the expense of longer-term investment in quality, customer retention, and operational efficiency. Under the typical finance-focused forecasting exercise, no one checks operational metrics so long as the bottom line comes in strong, which it had for a while. Now the company’s performance is stuck in low gear: the most successful business units keep committing to higher numbers that eventually lead to a deterioration in quality, while the least successful businesses get scrutinized, adjusted, and fixed. The winners and losers flip, and the cycle repeats itself.
Some companies have worked at breaking this disappointing pattern. They’ve begun rethinking how they measure the success of their forecasting processes—focusing on the following four questions:
Have we built a momentum case? Many financial-planning and analysis (FP&A) teams spend most of their time looking at historical data to explain current outcomes. When they do get to look forward, they are likely focused on the budget, or on rolling up commitments from different business units into the overall business plan. Sometimes the business plan itself passes for the forecast. This, of course, just creates an echo chamber. No one is explicitly discussing how external factors and impending market shifts could affect forecasts. A better approach is to create a market-momentum case that relies on internal and external data as well as end-market trends to build the forecast. Once this unbiased momentum case is in place, senior managers can layer new and additional market information on top. Then any initiatives, investments, and strategic moves can be assessed relative to the base case.
In one industrial company that makes construction products, for instance, initiatives proposed across different lines of business were being valued in a vacuum. The teams charged with managing grouts and concrete, for instance, had no line of sight into what was going on with the frame-protection or frame-reinforcement business units. It was hard for senior leaders, then, to understand how to react to market shifts throughout the year and what actions the company should take. The FP&A team built a momentum case that set targets based on market dynamics in individual lines of business rather than allocating a single rate of improvement to all products. These targets much more closely reflected the full potential of the individual business lines, and, when compared with base-case and other scenarios, allowed the company to allocate resources and take on initiatives to address market changes with much more agility than before.
The CFO’s role in this process is to work with business-unit leaders to set realistic but aggressive targets in light of the market environment in which they operate. That means providing clear direction to all the business-unit finance leaders about which basic assumptions to use in the forecasts—for instance, market-growth rates—even if the process of forecasting sales and profit for each business remains distributed.
Are we using a variety of operational indicators and external inputs? Operational inputs are important leading indicators of performance; often, line leaders know how the company is faring months before the financial reports appear. Many times, however, operating data sit in disparate systems that don’t work well with financial enterprise resource planning (ERP) systems. And rather than adhere to a standard set of key performance indicators (KPIs) for use throughout the organization, managers at different levels use different indicators. Some track the business, some manage individual performance, and some review indicators of financial performance.
One aerospace company had collected thousands of data points on every aircraft in its fleet. However, when the time came to create the annual forecast, it used only a small fraction of the data because much of the information was inaccessible. Operational and financial data were siloed, spread across many different IT systems. Recognizing a lost opportunity, the company created a thin analytics layer—a simple rules-based SQL program in a data lake—on top of its existing IT infrastructure. This program automatically gathered data from the multiple systems, allowing business-unit leaders to see information about the entire fleet on a single screen. They finally had operational KPIs integrated into the financial picture. If one site went overbudget on a repair, that information would get immediately recorded in the master model. Leaders at the company are now more confident about setting targets and stretch goals based on a distinct set of operational issues.
Surprisingly, the technical changes required were not difficult; in most cases, it takes no more than a few weeks or months (depending on product complexity) to rebuild forecasting models and link them to the company’s financial-management systems.
Would you like to learn more about our Strategy and Corporate Finance Practice ?
Have we explored automation? Once business leaders have identified the most critical inputs to include in the forecast model, they should consider ways to automate the process and make it easier for business and operations teams to work together on forecasts.
In some cases, the company will need to explore new technologies and modeling techniques. Leadership at a multinational pharmaceutical company, for instance, used machine learning and advanced analytics to understand the variables affecting the performance of its clinical trials. The operations team worked with the business side to aggregate five years of data from more than 300 separate clinical trials (involving more than 100,000 patients) and to evaluate factors such as the clinical trials’ time and costs across multiple geographies. They saw correlations between the rate of enrollment in certain sites and the success of the trials, and they used the data to introduce improvements.
In other instances, the whole forecasting model may be run automatically using macro commands in an Excel spreadsheet, with only a handful of manual inputs from the operations team, the CFO, and the finance team.
Whichever method is chosen, companies can use data that already exist in the company’s ERP or other functional databases and, with simple transformations, spit out a real-time dashboard.
Once the CFO or another senior finance leader decides that automation is a high priority for FP&A, he or she should convene a small team (no more than three to five people from IT and finance) to “connect the pipes.” The team should tackle this challenge incrementally—automating some elements of the forecasting process initially and adding others once the value of the effort is proved.
Are we measuring effectiveness at a fine-grained level? Once the forecast incorporates a range of internal and external inputs, CFOs can test the accuracy of each input, as well as the accuracy of aggregate estimates. By monitoring detailed measures, such as labor productivity, on-time delivery, and other metrics associated with costs and revenues, business leaders will be able to spot the “softer” KPIs that are being overlooked in light of temporarily strong bottom-line performance. They can then react accordingly.
When the CFO and operations leader at one consumer-goods company reviewed underlying performance metrics for each of the business lines, they saw that a major business unit was being propped up by one rapidly growing product. Based on this insight, senior leadership decided to sell the underperforming parts of that business and double-down where they saw profitable growth. The team had until that point not looked past the simple financial performance of the business unit to the product-level sales and profitability.
If finance and operations leaders can maintain the forecast as a living model, with a clear feed-back loop, they can ensure that any forecasting failures (and there will be failures) lead to real improvements.

The right role for multiples in valuation
Getting started.
Making these changes to the forecasting processes can seem like a monumental shift—“rebuilding the plane while it is flying” is a common complaint among finance teams. With that in mind, the most effective move is to start small. For instance, the CFO should task the FP&A team in a single business unit or region to come up with a model and pressure test it both in the finance function and with nonfinancial leaders. Once there is agreement that the model is unearthing valuable insights, it can be automated, and a similar process can be scaled to the rest of the business.
It’s important that the forecast be pulled out of the politics of budgeting, and that inputs are streamlined, automated, and pressure tested. Even if the business units each manage their own forecast, there is a role for central FP&A to debias the process. The FP&A team at one fashion company, for instance, built a simple regression analysis to understand which business units were forecasting statistically significant changes in their performance or growth trajectory. The outliers were required to provide a detailed buildup of initiatives to prove the forecast was achievable.
Once the hard work of process reengineering is done, finance teams will see a dramatic change in the value of forecasts to the business. They can use time previously spent justifying assumptions to focus on delivering new ideas for improving the performance of the business—and serving as proactive business partners.
Ankur Agrawal is a partner in McKinsey’s New York office, and Jonathan Slonim is a consultant in the New Jersey office. Mark Khavkin is the chief financial officer of Pantheon Platform in San Francisco.
The authors wish to thank the CFO Leadership Council for its contributions to this article.
Explore a career with us
Related articles.

Is your budget process stuck on last year’s numbers?
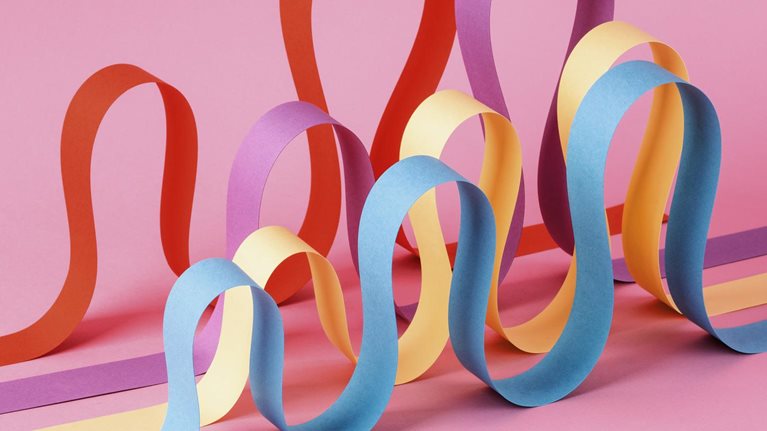
Bias Busters: Being objective about budgets

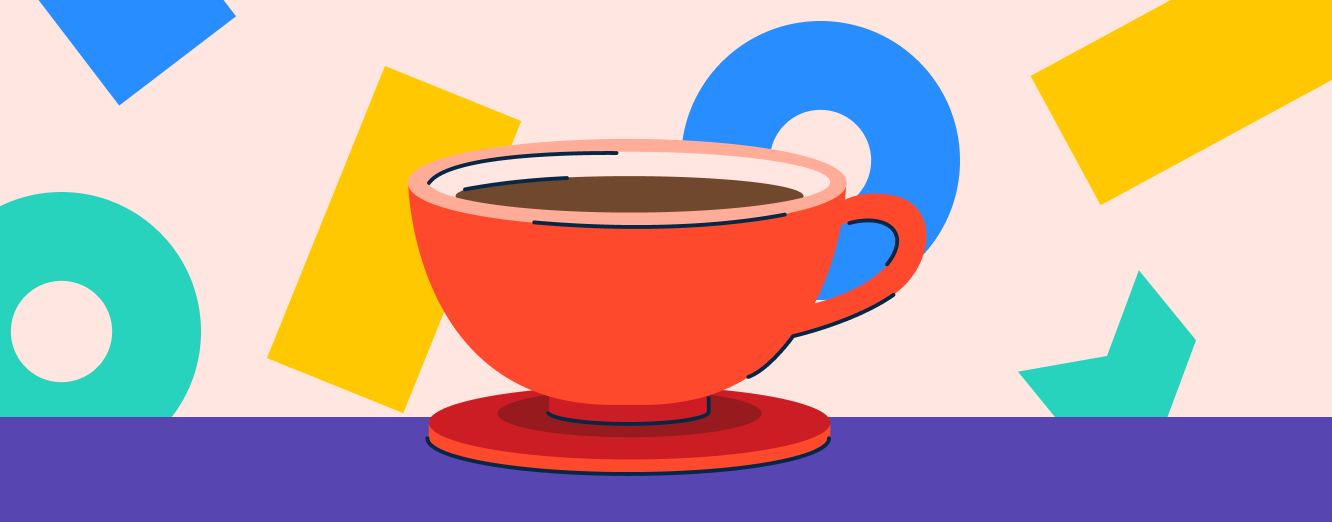
Nice to meet you.
Enter your email to receive our weekly G2 Tea newsletter with the hottest marketing news, trends, and expert opinions.
What Is Business Forecasting? Predictions to Drive Success
November 29, 2021
by Alexandra Vazquez
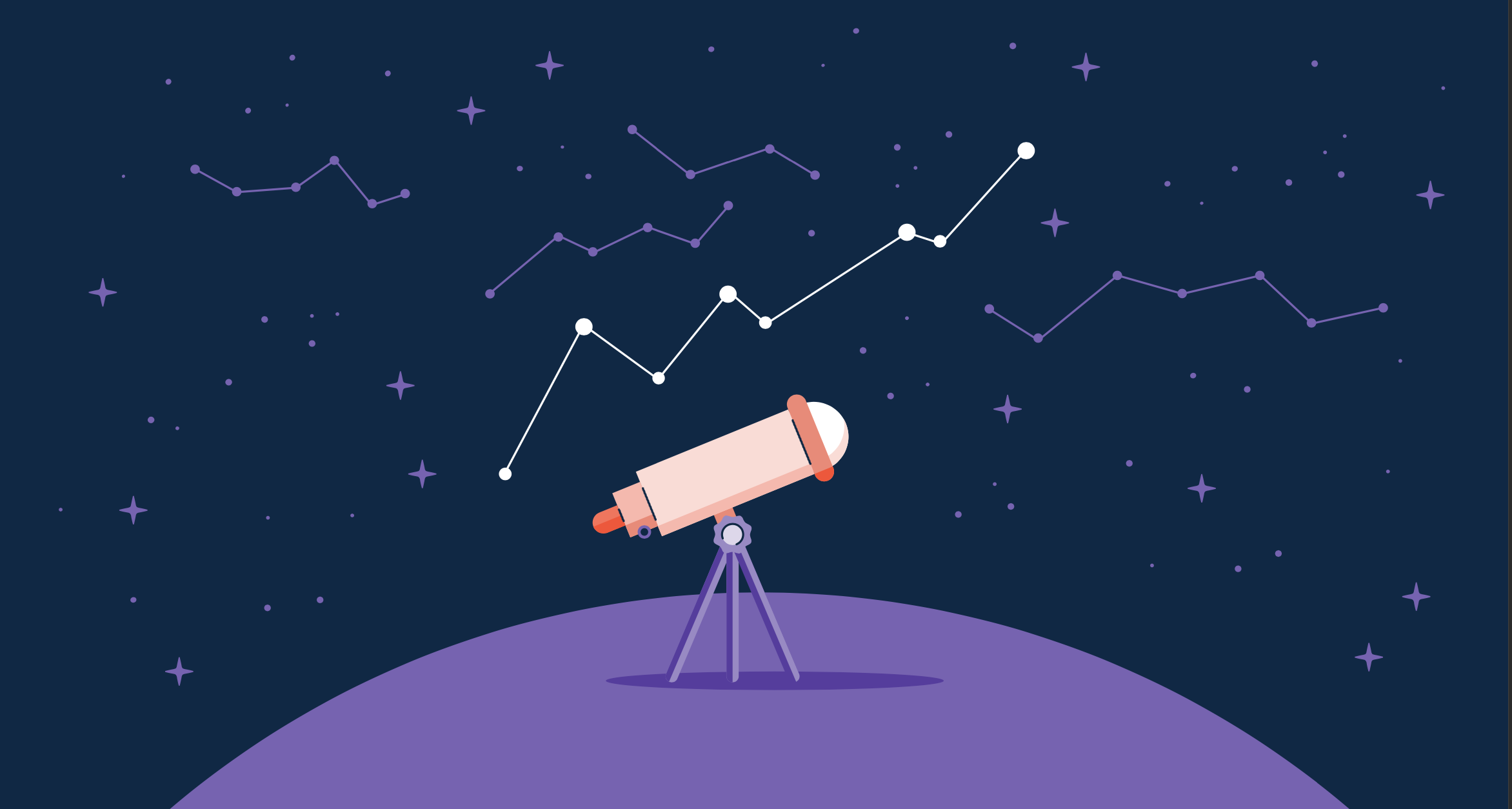
Types of business forecasts
Business forecasting methods, benefits of business forecasting, business forecasting challenges, business forecasting vs. scenario planning, business forecasting process, business forecasting examples.
It’s time to look inside your crystal ball and start forecasting. Forecasting gives you the tools you need to make reliable predictions about foreseeable events.
What is business forecasting?
Business forecasting is the process of analyzing data to predict future company needs and make insight-driven development decisions.
There’s really no downside to being prepared! Building a strong forecast prepares businesses for potential issues and identifies areas for profitable growth. Even if your predictions end up being inaccurate, you’ll have all the necessary data and information to get closer to the final forecast.
Some companies utilize predictive analytics software to collect and analyze the data necessary to make an accurate business forecast. Predictive analytics solutions give you the tools to store data, organize information into comprehensive datasets, develop predictive models to forecast business opportunities, adapt datasets to data changes, and allow import/export from other data channels.
Businesses can create various types of forecasts with business forecasting strategies. Because historical data and market trends affect so many aspects of business, comprehensive predictions can help prepare almost every element of your company.
- General business forecasting predicts overall market trends and external factors that affect your business’ success.
- Accounting forecasting creates projections of future business costs .
- Budget forecasting makes predictions for allocating the budget needed for future projects or addressing potential issues. Budgeting and forecasting software is an indispensable tool if you’re looking to forecast for budgeting your business activities.
- Financial forecasting projects a company’s monetary value as a whole. You can use the current assets and liabilities from your balance sheet to help you make a prediction.
- Demand forecasting predicts the future needs of your target customer base.
- Supply forecasting works with demand forecasting to allocate the necessary resources for fulfilling upcoming customer demands.
- Sales forecasting predicts the expected success of the company offerings and how it’ll affect future sales and cash flow.
- Capital forecasting makes predictions about a company’s future assets and liabilities.
There are two main types of business forecasting methods: quantitative and qualitative. While both have unique approaches, they’re similar in their goals and the information used to make predictions – company data and market knowledge.
Quantitative forecasting
The quantitative forecasting method relies on historical data to predict future needs and trends. The data can be from your own company, market activity, or both. It focuses on cold, hard numbers that can show clear courses of change and action. This method is beneficial for companies that have an extensive amount of data at their disposal.
There are four quantitative forecasting methods:
- Trend series method: Also referred to as time series analysis, this is the most common forecasting method. Trend series collects as much historical data as possible to identify common shifts over time. This method is useful if your company has a lot of past data that already shows reliable trends.
- The average approach: This method is also based on repetitive trends. The average approach assumes that the average of past metrics will predict future events. Companies most commonly use the average approach for inventory forecasting.
- Indicator approach: This approach follows different sets of indicator data that help predict potential influences on the general economic conditions, specific target markets, and supply chain. Some examples of indicators include changes in Gross Domestic Product (GDP), unemployment rate, and Consumer Price Index (CPI). By monitoring the applicable indicators, companies can easily predict how these changes may affect their own business needs and profitability by observing how they interact with each other. This approach would be the most effective for companies whose sales are heavily affected by specific economic factors.
- Econometric modeling: This method takes a mathematical approach using regression analysis to measure the consistency in company data over time. Regression analysis uses statistical equations to predict how variables of interest interact and affect a company. The data used in this analysis can be internal datasets or external factors that can affect a business, such as market trends, weather, GDP growth, political changes, and more. Econometric modeling observes the consistency in those datasets and factors to identify the potential for repeat scenarios in the future.
Qualitative forecasting
The qualitative forecasting method relies on the input of those who influence your company’s success. This includes your target customer base and even your leadership team. This method is beneficial for companies that don’t have enough complex data to conduct a quantitative forecast.
There are two approaches to qualitative forecasting:
- Market research: The process of collecting data points through direct correspondence with the market community. This includes conducting surveys, polls, and focus groups to gather real-time feedback and opinions from the target market. Market research looks at competitors to see how they adjust to market fluctuations and adapt to changing supply and demand . Companies commonly utilize market research to forecast expected sales for new product launches.
- Delphi method: This method collects forecasting data from company professionals. The company’s foreseeable needs are presented to a panel of experts, who then work together to forecast the expectations and business decisions that can be made with the derived insights. This method is used to create long-term business predictions and can also be applied to sales forecasts.
There are several benefits to making effective forecasts for your business. You gain valuable insights into its different aspects and the future of its success.
- Foresee upcoming changes with a heads up on potential market changes that can affect your business. With the right prediction, you can strategize the decisions to succeed in the face of the challenges ahead before they become costly surprises.
- Decrease the cost of unexpected demand by preparing ahead of time. Business forecasting is a great starting point for demand planning . If you plan to incorporate demand forecasting into your business processes, you’ll be prepared for upcoming market demands and avoid the extra costs associated with an influx of demand that you weren’t ready for.
- Increase customer satisfaction by giving them what they want, when they want it. Demand planning doesn’t just benefit you. With the right business forecast, your company can offer products or services to the target industry and meet their expectations. A company ready to serve its market is always met with customer satisfaction and loyalty.
- Set long- and short-term goals by tracking your progress. Business forecasting tools help you outline your future company objectives. Continuous predictions allow you to track the progress of your proposed goals as those future expectations become the present reality.
- Learn from the past by analyzing it. Forecasting enables you to collect and study extensive historical company data. Keeping a close eye on this data can help you identify where things may have gone wrong in the past. With this new information, your company can make the necessary adjustments to avoid similar mistakes in the future.
While the benefits of business forecasting highlight all of the amazing advantages it has to offer, it’s not a surefire way to prepare for the future. Companies who plan to forecast should also keep the challenges in mind and make sure that forecasting has more pros than cons for their business. Below are some of the notable challenges of business forecasting.
- You can’t always expect the unexpected. While old data can help you gain insights into company processes and learn from mistakes, history doesn’t always repeat itself. Business forecasting isn’t a perfect process, and although helpful, it may not precisely predict future trends or business matters using old company data alone. It operates on the assumption that what happened will most likely happen again. Unfortunately, this is not always the case, and the hard work put into preparing for a forecasted event may never come to fruition.
- It takes time to create an accurate forecast. Forecasting can be a lengthy process when started from scratch. Some companies find it challenging to gather the resources needed to begin predicting and allocate the time to do it correctly.
- Historical data will always be outdated. There’s no way to know what’ll happen next. Although historical information is very valuable, it’s forever considered “old”. Forecasts are never based on the present and, therefore, are only as accurate as the data you already collected.
Business forecasting is often confused with scenario planning because of their shared goal of preparing for the future. Both rely on learning from past mistakes and reflecting on what decisions must be made to drive success. However, business forecasting and scenario planning differ in the preparation process.
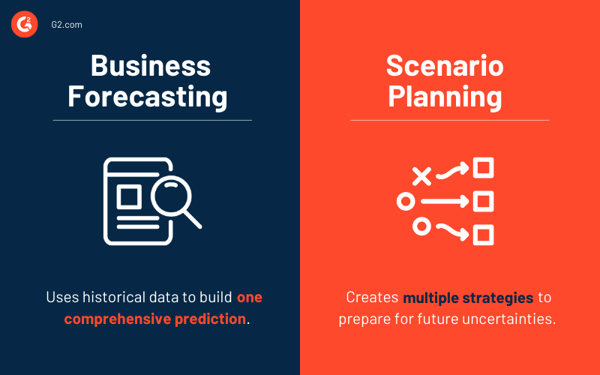
Business forecasting focuses on a problem at hand and uses historical data to predict what might happen next. It emphasizes predictive analytics and the need to eliminate existing uncertainties. The problem can be as broad as the actual performance of the entire company, or as specific as how a single product might sell in the future based on past market trends.
While built on tangible data, forecasting is essentially a guess of the future and you need to make assumptions ahead of time to prepare for any predicted issues. Forecasting is an all-hands-on-deck approach that involves many departments, including analysts, economists, managers, and more.
Scenario planning creates multiple scenarios to help prepare for the future. With these scenarios in mind, a company can begin planning a course of action to achieve the desired outcome. This includes creating step-by-step strategies and timelines for achieving objectives.
While business forecasting focuses on past information, scenario planning takes the past, present, and future into consideration with learnings from the past, understanding the capabilities of the present, and aspiring for future success. Although a team’s input is important in scenario planning, company’s primary decision-makers carry out the bulk of the process.
The way a company forecasts is always unique to its needs and resources, but the primary forecasting process can be summed up in five steps. These steps outline how business forecasting starts with a problem and ends with not only a solution but valuable learnings.
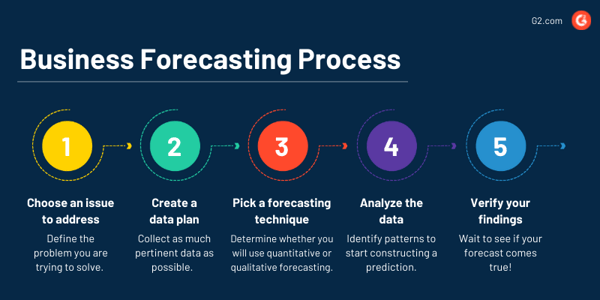
1. Choose an issue to address
The first step in predicting the future is choosing the problem you’re trying to solve or the question you’re trying to answer. This can be as simple as determining whether your audience will be interested in a new product your company is developing. Because this step doesn’t yet involve any data, it relies on internal considerations and decisions to define the problem at hand.
2. Create a data plan
The next step in forecasting is to collect as much data as possible and decide how to use it. This may require digging up some extensive historical company data and examining the past and present market trends. Suppose your company is trying to launch a new product. In this case, the gathered data can be a culmination of the performance of your previous product and the current performance of similar competing products in the target market.
3. Pick a forecasting technique
After collecting the necessary data, it’s time to choose a business forecasting technique that works with the available resources and the type of prediction. All the forecasting models are effective and get you on the right track, but one may be more favorable than others in creating a unique, comprehensive forecast.
For example, if you have extensive data on hand, quantitative forecasting is ideal for interpretation. Qualitative forecasting is best if you have less hard data available and are willing to invest in extensive market research.
4. Analyze the data
Once the ball starts rolling, you can begin identifying patterns in the past and predict the probability of their repetition. This information will help your company’s decision-makers determine what to do beforehand to prepare for the predicted scenarios.
5. Verify your findings
The end of business forecasting is simple. You wait to see if what you predicted actually happens. This step is especially important in determining not only the success of your forecast but also the effectiveness of the entire process. Having done some forecasting, you can compare the present experience with these forecasts to identify potential areas for growth.
When in doubt, never throw away “old” data. The final information of one forecasting process can also be used as the past data for another forecast. It’s like a life cycle of business development predictions.
With the different types of business forecasting come different potential use cases. A company may choose to utilize several elements of business forecasting to prepare for various situations. Here are some real-life examples where business forecasting would be valuable.
The seasoned veteran
Suppose you represent a company that has been in the market for a long time but has never tried business forecasting. Because of the long history of company data, you choose to try out quantitative business forecasting. Your aim is to make predictions using the most cost-effective and least time-consuming method. With those considerations, you may opt for the trend series method to manually identify common trends in old data, determine the likelihood of repeat instances, and forecast accordingly.
The new kid on the block
Imagine you are a new company that has entered the market to start selling your own brand of smartphones. You may think that business forecasting is impossible because you don’t have any historical company data to work off of. However, you can utilize qualitative business forecasting! Because the smartphone industry is a highly competitive one, you can use market research to take advantage of publicly available market data.
The one who wants the best of both worlds
Imagine you work for a recruiting company that has noticed that the country’s unemployment rate heavily affects company performance and has the data to prove it. As you have a clear indicator that directly impacts the potential for success, using the indicator approach to create long-term predictions would be the right call.
However, your company stresses the importance of integrating expert knowledge into the forecasting process. This extra note means that some qualitative forecasting can be used as well. You may choose to use the Delphi method to collect expert opinions and weigh that into the final forecasts as well.
What do the stars have in store for you?
Creating comprehensive predictions isn’t rocket science. With business forecasting, seeing the future is as easy as learning from the past. What you do with your findings is what will set you apart.
Want to start forecasting for your business? Learn more about business analytics and how it helps collect the necessary data and insights.
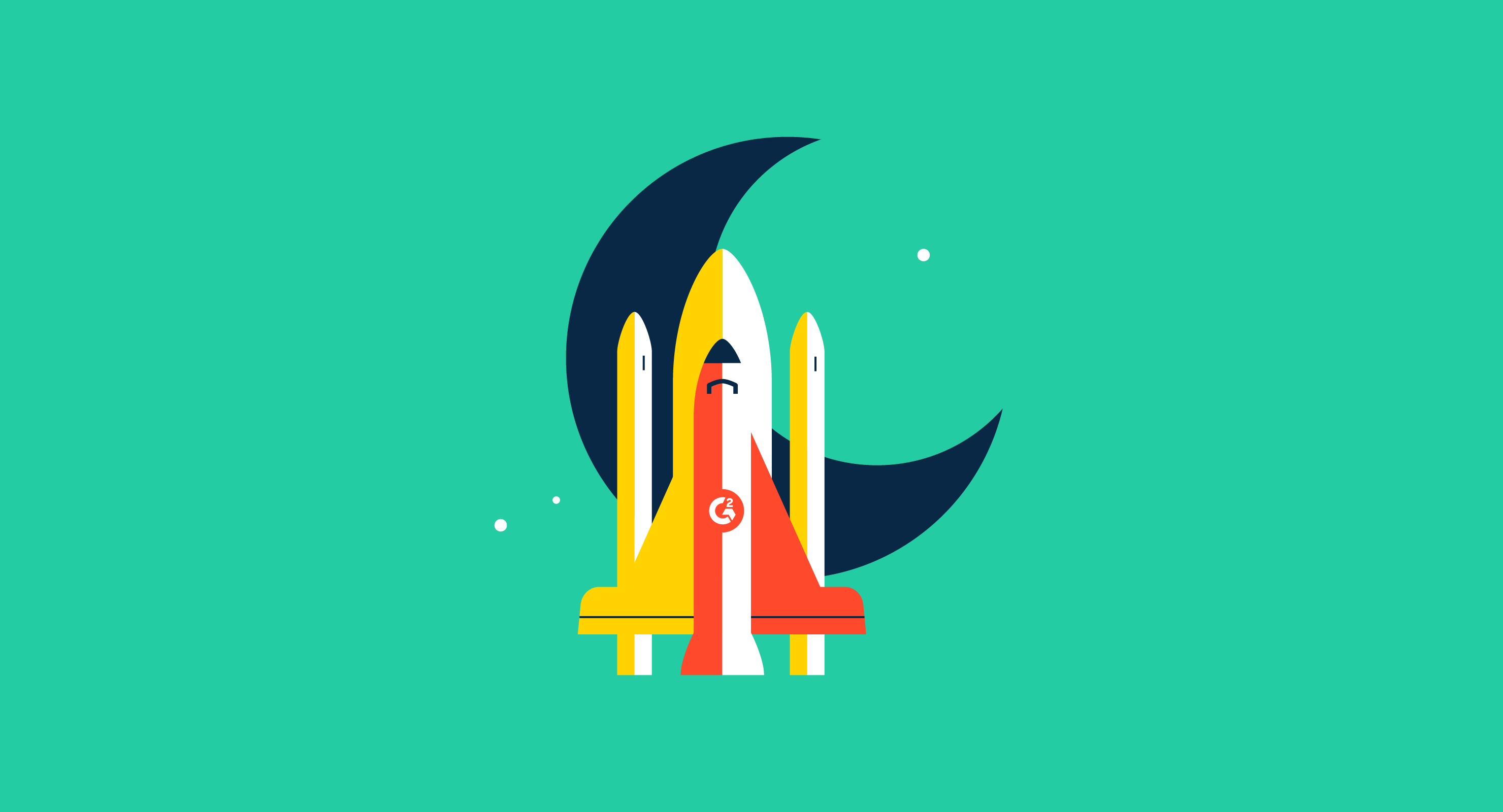
Automate business forecasting data analysis with predictive analytics software.
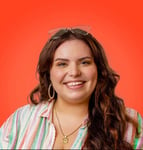
Alexandra Vazquez is a Senior Content Marketing Specialist at G2. She received her Business Administration degree from Florida International University and is a published playwright. Alexandra's expertise lies in writing for the Supply Chain and Commerce personas, with articles focusing on topics such as demand planning, inventory management, consumer behavior, and business forecasting. In her spare time, she enjoys collecting board games, playing karaoke, and watching trashy reality TV.
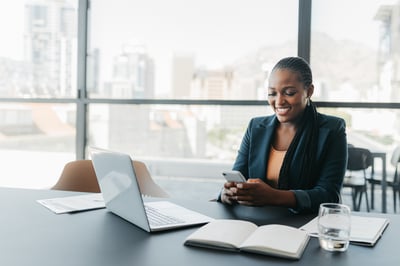
Contributor Network
AI's transformative impact has grown across all aspects of our lives. From anticipating retail...
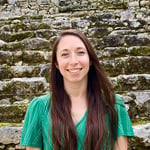
When was the last time you attended a business event and returned empty-handed? It's hard to...
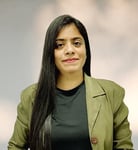
Sustainability is no longer a choice; it's a necessity.
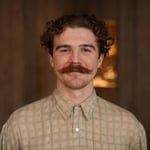
Never miss a post.
Subscribe to keep your fingers on the tech pulse.
By submitting this form, you are agreeing to receive marketing communications from G2.
- Company management
Navigating the future with business forecasting

In today's dynamic and ever-changing business environment, organisations must make accurate future predictions to stay competitive and thrive. Business forecasting is one tool that enables companies to make informed decisions about their future.
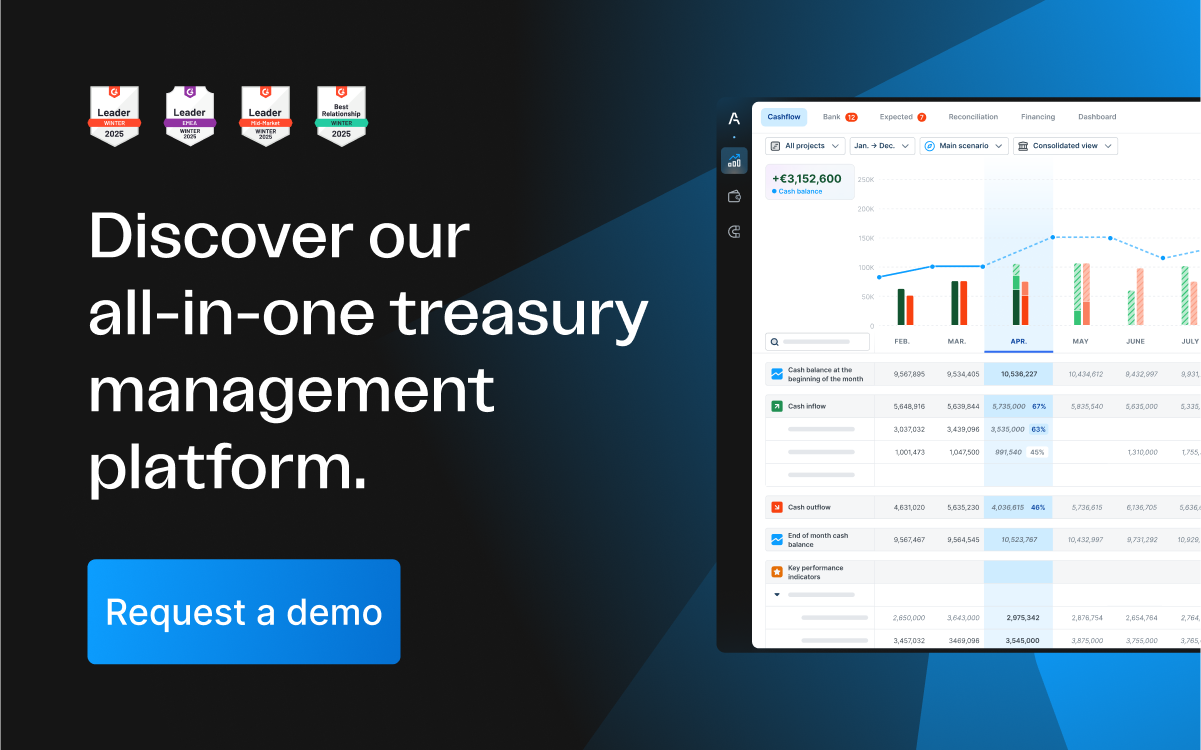
What is business forecasting?
Business forecasting is predicting future outcomes based on past and present data. This involves analysing historical trends, market conditions, customer behaviour, and other relevant factors to determine prospects and threats.
The main goal of business forecasting is to develop an informed estimate of future events and circumstances . This enables businesses to make strategic decisions and prepare for future expansion.
Why is business forecasting important?
Forecasting is important for businesses as :
- It helps organisations to make strategic plans for the future.
- Helps organisations to allocate resources effectively and efficiently.
- Plays a crucial role in financial planning by helping businesses estimate future revenues, expenses, and profits.
- Helps organisations to identify problems, potential risks and uncertainties and develop risk management strategies to mitigate them.
- Provides decision-makers with valuable insights and data, which can help them make better-informed decisions and develop short & long term success strategies .
Related article: The best cash flow forecasting software in 2023
Business forecasting process
Effective business forecasting requires careful planning and execution. The following steps provide a comprehensive guide on how to develop a successful forecasting plan:
Define the objective : The first step in the business forecasting process is to define the objective of the forecast by identifying the key performance indicators (KPIs) such as sales, revenue, or market share.
Gather data : Next, gather relevant data, including historical data on the KPIs, market trends, and other variables that may impact the forecast.
Analyse the data : Once the data has been gathered, it must be analysed to identify patterns, trends, and other factors that may impact the forecast. This can be done using statistical models, machine learning algorithms, or other analytical tools.
Develop the forecast : Based on the analysis of the data, a forecast can be developed using the insights gained from the data analysis to generate a prediction of future performance on the KPIs.
Validate the forecast : Now, it should be validated to ensure its accuracy. This can be done by comparing the forecast to actual performance data from past periods.
Implement the forecast : Finally, the forecast can be used to make informed decisions about business operations. This involves adjusting resource allocation, pricing strategies, or other aspects of the business based on the predicted future performance.
As business forecasting is an iterative process, it's important to monitor actual performance against the forecast and adjust the forecast as required to ensure its accuracy over time.
👉 Watch our video on cash flow forecasting
What are 2 basic methods of forecasting in business?
Two main methods of forecasting are:
Qualitative forecasting : This approach uses expert opinions, market research, surveys, and other subjective data to predict future trends. Qualitative forecasting is useful when historical data is limited, and the future is uncertain.
Quantitative forecasting : This method relies on historical data and statistical analysis to predict future trends. Quantitative forecasting is suitable for industries with a lot of historical data and stable market conditions.
4 Basic forecasting techniques
The four basic forecasting techniques are:
Trend analysis : This method identifies patterns and trends in historical data to predict future values. Trend analysis helps forecast long-term trends.
Regression analysis : Regression analysis is helpful for forecasting in complex environments where multiple variables are involved. This approach identifies the relationship between two or more variables to predict future values.
Moving average : This method calculates the average of past data points to identify trends and predict future values. Moving averages are helpful for forecasting in stable and predictable environments.
Exponential smoothing : Exponential smoothing is helpful for forecasting in rapidly changing environments. This technique assigns more weight to recent data points than older ones to predict future values.
A few other business forecasting techniques are:
- Scenario analysis
- Judgmental forecasting
- Causal forecasting
- Econometric forecasting
- Delphi method
- Simulation modelling
See also: Improving liquidity in your business in 5 easy ways
5 Forecast models:
Forecast models in business forecasting are mathematical or statistical tools used to predict future trends and outcomes based on historical data and various influencing factors. These models are designed to analyse patterns, relationships, and dependencies within the data to generate reliable forecasts.
The forecast models serve as valuable tools for businesses to anticipate demand, sales, market trends, financial performance, and other crucial factors, enabling them to make informed decisions and develop effective strategies. By leveraging these forecast models, businesses can make data-driven decisions, improve resource allocation, optimise inventory levels, and enhance operational efficiency.
There are several types of forecast models commonly used in business forecasting, such as:
1. Time Series Models: These models analyse historical data to identify patterns and make predictions based on the assumption that future trends will continue in a similar pattern. Time series models, such as moving averages and exponential smoothing, are commonly used in businesses to forecast demand, sales, and financial metrics. These models can predict future trends by analysing historical patterns and seasonality and help businesses optimise inventory management, production planning, and resource allocation.
2. Regression Models: Regression analysis uses historical data to establish relationships between variables, allowing for the prediction of one variable based on the values of other related variables. It is widely applied in business forecasting to understand the relationships between variables. For example, businesses may use regression models to forecast sales based on factors like marketing expenditure, pricing, and macroeconomic indicators. These models provide insights into the impact of different variables on business performance and inform strategic decision-making.
3. Exponential Smoothing Models: Exponential smoothing models place greater emphasis on recent data points, giving them more weight in the forecast calculation while gradually decreasing the impact of older data. Exponential smoothing models are helpful for short-term forecasting and are commonly employed in inventory management and sales forecasting. By assigning different weights to recent and older data points, these models give more significance to recent trends, allowing businesses to adapt quickly to changes in demand.
4. Econometric Models: These models incorporate economic theory and statistical techniques to forecast business outcomes by considering factors such as GDP, inflation, interest rates, and other macroeconomic indicators. These models are applied in areas such as financial forecasting, market analysis, and pricing strategies. By considering macroeconomic factors and their impact on specific industries, businesses can predict market conditions and adjust their strategy accordingly.
5. Machine Learning Models: Machine learning algorithms can analyse large volumes of data, identify complex patterns, and make forecasts based on the identified patterns. Machine learning algorithms, including neural networks, decision trees, and random forests, can be utilised to forecast various business metrics. These models can analyse large datasets, identify complex patterns, and make accurate predictions. Businesses apply machine learning models for demand forecasting, customer behaviour analysis, fraud detection, and personalised marketing campaigns.

What are examples of business forecasts?
Let's take the example of business forecasting for a company that manufactures and sells organic skincare products. Based on the assumptions, that the company has been in business for a few years and has historical sales data, it could use that data to forecast its sales using trend analysis for the next year.
They might consider such cases and scenarios, as mentioned under:
- Past sales trends : If sales have steadily increased by 10% each year, they might assume they will see similar growth in the coming year.
- Market trends : They would also look at broader trends - Whether more people are becoming interested in organic products. Is there a new ingredient that is gaining popularity? These factors could influence the company's sales.
- Marketing initiatives: If the company plans to launch a new product line or run a major advertising campaign, it might expect a boost in sales.
Using this information, the company could create a sales forecast for the following year. They might forecast a 10% increase in sales based on historical trends, plus an additional 5% increase based on market trends and marketing initiatives.
Business Forecasting Software
Business forecasting software uses cutting-edge algorithms and statistical approaches to analyse historical data and current market conditions to generate accurate forecasts about future outcomes. It helps organisations to predict future trends, patterns, and behaviours related to their business operations.
Business forecasting software is used by companies in various industries. Its key features include data visualisation tools, predictive analytics, scenario planning, and automated reporting.
Using a business forecasting software:
- Businesses can make more informed decisions about resource allocation, budgeting, and strategic planning.
- Businesses can identify potential risks and opportunities and adjust their operations to stay competitive in a rapidly changing market.
Wrapping Up
Business forecasting emerges as a vital tool for organisations aiming to make well-informed decisions regarding the future.
By following a comprehensive process that includes defining the objective, gathering data, selecting the methodology, developing the forecast, and monitoring and reviewing, companies can develop accurate and reliable predictions that inform strategic decisions and drive growth.
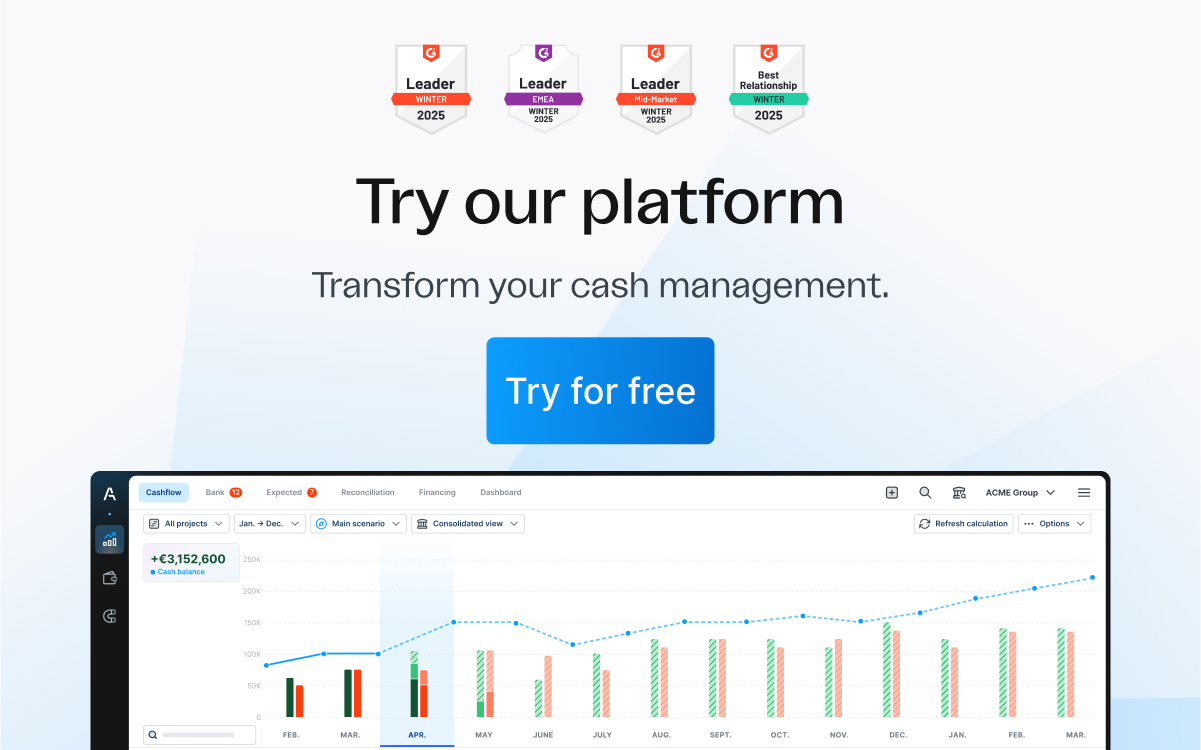
Subscribe to our newsletter
You may also like.
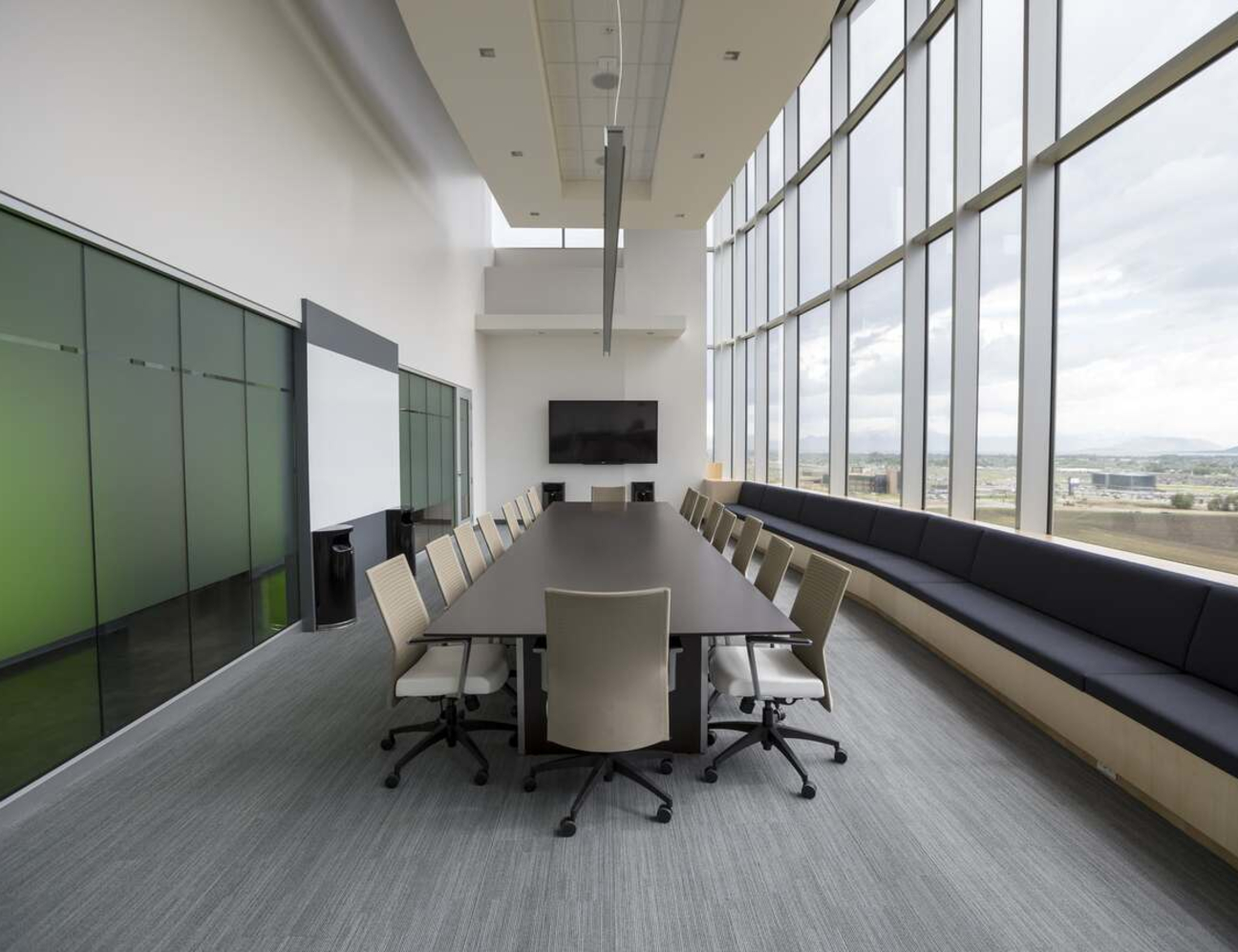
201 Borough High Street London SE1 1JA
- Cash management
- Liquidity planning
- Banking & ERP
- Supplier management
- Cash collection
- Cash flow monitoring
- Cash flow forecast
- Consolidation
- Debt management
- Late payment reminders
- Supplier Invoice Management
- Custom dashboards
- Manufacturing
- Restaurants
- Construction
- Real estate
Company size
- Mid-sized Companies
- £10M - £50M revenue
- £1M - £10M revenue
- Resource center
- Excel models
- Practical guides
- Cashflow management
- Costs and revenue management
- Financial management
- Company creation
- Terms of Use
- General Terms of Service
- Privacy Policy
- Legal Notice
- Integrations
- We're hiring
Financial Forecasting: Key Strategies and Tactics for Business Growth
- Banking & Finance
- Bookkeeping
- Business Operations
- Starting a Business
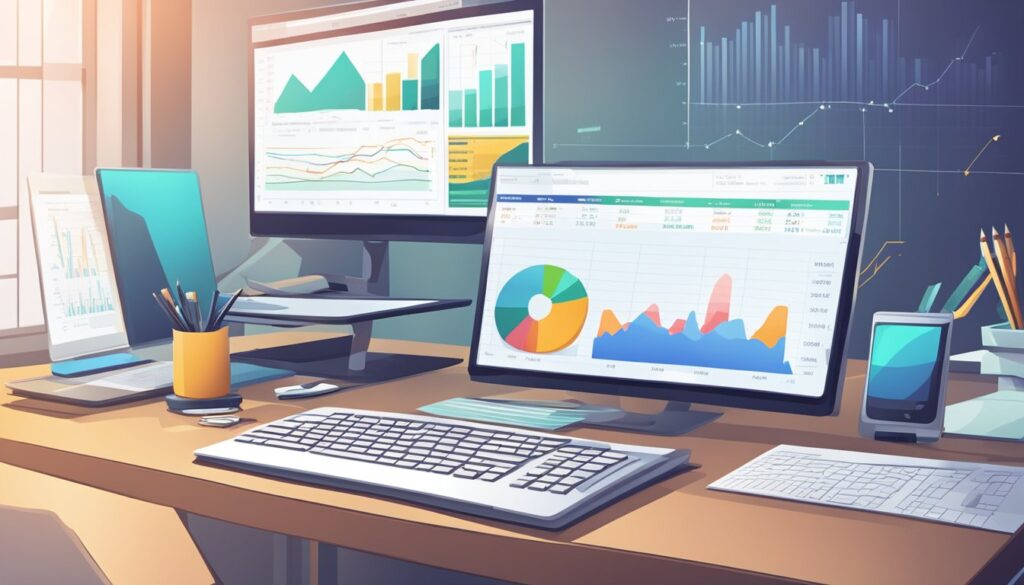
Financial forecasting is a crucial aspect of finance and business management, involving the process of estimating or predicting how a business will perform in the future. It helps organizations to strategize, plan, and budget effectively by utilizing historical data, expert insights, and various forecasting methods. Financial forecasting not only assists businesses with managing their financial resources but also serves as a key element in making informed decisions for future growth and expansion.

There are several techniques and models used in financial forecasting, ranging from top-down approaches to statistical methods and software-guided predictions. These methods analyze historical data, taking into account both internal and external factors, and produce results that guide crucial financial decisions. Depending on the business needs and specific objectives, organizations can adopt various forecasting methods to enhance accuracy and improve decision-making.
Furthermore, it is essential to differentiate between short-term and long-term forecasting, with varying time horizons and purposes, and consider how budgeting is intertwined with forecasting processes. Leveraging software tools and integrating forecasting into strategic planning are essential aspects of successful business management. Effective communication with stakeholders and addressing frequently asked questions related to financial forecasting are also integral to maintaining its transparency and accuracy.
Key Takeaways
- Financial forecasting is essential for strategic planning, budgeting, and informed decision-making in business.
- Various techniques and models are utilized in forecasting, depending on the business context and objectives.
- It is crucial to differentiate between short-term and long-term forecasting, understand their relationship with budgeting, and utilize software tools for increased accuracy.
Fundamentals of Financial Forecasting
Understanding forecasting.
Financial forecasting is the process of estimating or predicting how a business will perform in the future. It involves analyzing historical data, current trends, and other relevant factors to make informed projections about a company’s future financial performance. Forecasting methods include qualitative approaches, which rely on expert opinions and educated assumptions, and quantitative techniques, which use mathematical models and statistical analysis.
There are various forecasting methods available, such as the Delphi method, simple linear regression, and time series analysis. Choosing the right method depends on the specific circumstances, the available data, and the goals of the business.
Importance in Business Planning
Financial forecasting is an essential aspect of business planning, as it allows companies to set realistic expectations, identify potential risks, and make informed decisions. Here are some of the key benefits of financial forecasting:
- Budgeting: Accurate financial forecasts help businesses determine their budget and allocate resources efficiently.
- Strategic planning: By predicting future performance, companies can develop a clear roadmap and set achievable goals.
- Risk management: Forecasting enables companies to identify potential challenges and prepare accordingly, mitigating risks and preventing financial losses.
- Performance evaluation: Regularly comparing actual performance to forecasts helps identify areas where a business is underperforming or overperforming, and adjust strategies accordingly.
In conclusion, financial forecasting is a vital component of business planning, helping companies set goals, allocate resources, and manage risks effectively. By leveraging historical data and various forecasting methods, businesses can make informed decisions, optimize their performance, and drive growth.
Financial Statements and Forecasting
Financial forecasting is an essential process for businesses to estimate their future performance and make informed decisions. One crucial aspect of financial forecasting involves the projection of key financial statements such as the balance sheet, income statement, and cash flow statement. These financial statements offer a comprehensive view of a company’s financial health and help in predicting the business’s future performance based on historical performance and current trends.
Balance Sheet Projections
The balance sheet provides a snapshot of a company’s assets, liabilities, and equity at a specific point in time. In financial forecasting, balance sheet projections are vital in determining how these items will evolve over time.
To forecast the balance sheet, it is necessary to:
- Project current assets, such as cash , accounts receivable , and inventory . This may involve analyzing historical patterns and incorporating relevant industry trends.
- Estimate non-current assets like property, plant and equipment (PPE) , taking into account depreciation and new investments.
- Forecast short-term and long-term liabilities, including accounts payable , debt , and other obligations .
- Calculate equity by forecasting changes in retained earnings , common stock , and additional paid-in capital .
Income Statement Projections
The income statement displays a company’s revenues, expenses, and net income over a specific period. Income statement projections are crucial in forecasting business performance and driving the predictions for the balance sheet and cash flow statement.
When projecting the income statement, consider the following steps:
- Forecast revenue by analyzing historical sales trends and considering factors such as business seasonality, market conditions, and potential growth opportunities.
- Estimate cost of goods sold (COGS) based on the relationship between sales and COGS, inflation, and industry trends.
- Project operating expenses such as selling, general and administrative (SG&A) expenses, by analyzing historical data and factoring in any anticipated changes in spending.
- Calculate the effect of non-operating items, including interest expenses and other income or expenses not associated with the main operations.
Cash Flow Statement Projections
The cash flow statement provides insight into a company’s cash inflows and outflows during a specific period, showing how the business generates and spends cash. Cash flow projections are essential in determining the liquidity and overall financial health of the company.
In forecasting the cash flow statement, consider three principal categories:
- Operating cash flows: Derived from income statement items, they include changes in working capital like accounts receivable, inventory, and accounts payable.
- Investing cash flows: They represent cash spent on investments, such as the acquisition of new PPE or the sale of existing investments.
- Financing cash flows: These include cash transactions related to raising and repaying debt, issuing new stock, or paying dividends.
By combining accurate projections for balance sheet, income statement, and cash flow statement items, proficient financial forecasting can be achieved, supporting businesses in making well-informed decisions and estimating future performance.
Forecasting Techniques and Models
Financial forecasting is an essential process for businesses to predict future financial performance. There are two primary categories of financial forecasting methods: quantitative and qualitative. Both techniques aid in decoding different aspects of financial forecasting, and we will explore each in detail below.
Quantitative Methods
Quantitative forecasting involves using historical data and mathematical models to predict future financial outcomes. These methods are most suitable when there is sufficient, reliable data, and relatively stable market conditions. Some popular quantitative forecasting techniques include:
- Time Series Analysis: This method observes patterns in historical data and extrapolates those patterns to predict future performance. Examples of time series analysis include moving averages and exponential smoothing.
- Regression Analysis: Regression is a statistical technique used to model the relationship between a dependent variable and one or more independent variables. There are various types of regression analysis, such as simple linear regression, multiple linear regression, and exponential regression.
- Financial Forecasting Models: Businesses can use top-down or bottom-up approaches in financial forecasting models. Top-down forecasting is when higher-level executives make predictions based on industry and market trends. In contrast, bottom-up forecasting aggregates data from lower levels of the organization, such as sales data from various departments.
Qualitative Methods
Qualitative forecasting relies on expert opinions, experience, and subjective evaluations to analyze and predict future financial performance. These methods are most valuable when historical data is scarce or unreliable, or when dealing with unprecedented market situations. Some qualitative forecasting techniques include:
- Delphi method: The Delphi method involves gathering opinions from a panel of experts, with each member providing their individual forecasts. This process is iterative, and panel members may revise their opinions based on the feedback from other experts. The final forecast is derived from the consensus of the expert panel.
- Market research: In this method, businesses can gather valuable information on consumer behavior, preferences, and upcoming trends by conducting surveys, focus groups, and interviews.
- Scenario analysis: Scenario analysis involves building multiple possible future situations and analyzing their potential financial implications, thus helping businesses to be better prepared for different outcomes.
By combining and carefully selecting appropriate quantitative and qualitative financial forecasting techniques, businesses can create a comprehensive financial model that guides informed decision-making and ensures sound financial management.
Budgeting and Its Relationship with Forecasting
Developing a budget.
Budgeting is a crucial financial practice that helps businesses and individuals plan for their financial future. It involves calculating expected revenues, expenses, capital expenditures, and the cost of goods sold, to align financial resources with strategic objectives. Budgeting enables businesses to maintain financial discipline, allocate resources effectively, and make well-informed decisions.
At the beginning of the budgeting process, it is essential to identify the financial goals and objectives for a given period, usually a year. This could include increasing revenue, reducing expenses, or achieving a specific level of profitability. Next, businesses need to estimate the anticipated revenues from various sources such as sales, investments, and other income streams. The following table illustrates a simple breakdown of revenues and expenses in a budget:
Revenue | |
– Sales | |
– Investments | |
– Other income | |
Expenses | |
– Cost of goods sold | |
– Operating expenses | |
– Capital expenditures |
Budget Forecasting
Budget forecasting is the process of closely monitoring the actual financial performance of a business and updating the original budget based on current data and market changes. Forecasting allows companies to compare their actual performance against the budgeted figures, helping them understand if they are on track to achieve their financial goals.
Integrating financial forecasting with the budget process is essential to maintaining fiscal discipline and delivering essential services. The forecast should extend several years into the future and cover major revenues and expenditures. It should be updated frequently to reflect actual performance and inform strategic decision-making.
Financial forecasting relies on both historical data and market trends, making it a dynamic and ongoing activity throughout the financial year. A budget forecast can help organizations:
- Adjust their strategies in response to market changes
- Identify and address financial challenges early
- Optimize their resource allocation
- Make better-informed decisions based on real-time financial data
- Evaluate the success of their strategic initiatives
In conclusion, budgeting and financial forecasting are closely related financial management activities that complement each other. By integrating budget forecasting with the budget preparation process, businesses can effectively track their progress, make necessary adjustments, and optimize their financial performance.
Short-Term vs. Long-Term Forecasting
Cash flow forecasting.
Short-term cash flow forecasting focuses on planning and budgeting cash for periods less than a year, usually spanning one to six months. The objective is to minimize short-term debt, idle cash, and maintain cash buffers. This type of forecasting is crucial for making immediate business decisions, such as optimizing short-term lending and borrowing.
On the other hand, long-term cash flow forecasting looks at a business’s financial future beyond the immediate 12-month window. It can include projections for cash inflows and outflows spanning multiple years. Long-term forecasts help uncover potential problems and trends that may arise in the future, enabling businesses to make strategic decisions.
Sales Forecasting
Short-term sales forecasting involves predicting a company’s sales performance within a 12-month period or less. This type of forecasting typically relies on historical sales data, market research, and trends to anticipate upcoming sales performance. It’s essential for businesses to adjust their sales strategies, such as inventory management and pricing, in response to short-term sales forecasts.
In contrast, long-term sales forecasting focuses on a broader time frame, often several years into the future. This approach may consider factors such as market conditions, industry trends, and technological advancements to project a company’s sales growth over an extended period. Long-term forecasts help businesses evaluate their overall strategy, assess growth opportunities, and allocate resources for the future.
Here’s a concise summary of the differences between short-term and long-term forecasting:
Type | Time Frame | Objective | Examples |
---|---|---|---|
Short-term | Up to 12 months | Make immediate business decisions, optimize short-term operations | Sales targets, cash flow management |
Long-term | Over 12 months | Identify trends, evaluate strategy, allocate resources for growth | Market expansion, product development |
Both short-term and long-term forecasting methods contribute to effective financial planning and decision-making for a company. By generating accurate cash flow and sales forecasts, businesses can adapt their strategies and better prepare for the future.
Using Software and Tools in Forecasting
Financial forecasting has evolved over time, with technology playing an increasingly important role in improving accuracy and efficiency. In this section, we will explore some software and tools that are commonly used in financial forecasting.
Excel for Forecasting
Microsoft Excel is a widely-used spreadsheet program that many businesses rely on for financial forecasting. With its advanced functionalities and comprehensive suite of formulas, Excel provides an excellent foundation for creating and customizing financial forecasting models.
Some of the key features in Excel for financial forecasting include:
- Formulas : Excel has a wide range of built-in formulas which enable users to perform complex calculations, such as linear regression, moving averages, or exponential growth.
- Pivot Tables : These powerful tools allow users to summarize, analyze, and derive insights from large datasets, making it easier to forecast financial performance.
- Charts and Graphs : Visualizing data is crucial in financial forecasting, and Excel offers various options for generating charts and graphical representations of data to enhance the forecasting process.
Advanced Forecasting Software
While Excel is a versatile and robust tool, some businesses may require more sophisticated solutions for financial forecasting. Advanced financial forecasting software can offer additional functionality and, in some cases, use predictive analytics to improve the accuracy of forecasts.
A few notable financial forecasting software options are:
- Adaptive Insights : This cloud-based platform offers tools for budgeting, forecasting, and financial reporting, as well as advanced analytics features to help identify trends and predict future performance.
- Anaplan : As a comprehensive financial management software, Anaplan includes capabilities for financial planning, budgeting, and forecasting, combined with predictive analytics to drive deeper insights.
- Prophix : Designed for financial professionals, Prophix provides budgeting, planning, reporting, and forecasting capabilities. The software also enables users to create a centralized financial database for improved collaboration and decision-making.
- Sage Intacct : A cloud-based financial management software, Sage Intacct offers robust financial forecasting tools with features such as automated reporting and real-time analytics.
When selecting financial forecasting software, it is essential to consider factors such as the size of your organization, your specific forecasting needs, and the level of customization required. The most suitable software should be intuitive, reliable, and have a proven track record of supporting financial forecasting processes effectively.
Financial Forecasting for Strategic Planning
Financial forecasting is a critical component of the strategic planning process. By predicting future financial performance, businesses can set realistic goals, identify problem areas, and make informed decisions to ensure the success of their organization.
Setting Realistic Business Goals
One of the primary aims of financial forecasting is to establish achievable business objectives. By leveraging past and current financial data, organizations can plot the expected trajectory of their profits and revenues. This empowers them to set targets that align with their financial capabilities. For instance:
- Short-term goals : these could include increasing monthly sales by a specific percentage or reducing operational costs within a stipulated timeframe.
- Long-term goals : such as expanding into new markets or launching a new product line in the coming years.
When setting goals, it’s essential to strike a balance between ambition and feasibility, ensuring that objectives are challenging yet attainable.
Identifying Problem Areas
Financial forecasting can also play a crucial role in detecting potential issues in a company’s operations. By analyzing trends and patterns in financial data, organizations can identify areas that may require attention or improvement. Some examples of problem areas may include:
- Inefficient processes: Operational inefficiencies often lead to increased costs and reduced profits. Financial forecasts can help companies spot patterns indicative of such issues, allowing them to enact process improvements and mitigate potential risks.
- Underperforming products or services: By comparing projected revenues with actual results, businesses can ascertain the performance of their offerings.
- Cash flow challenges: Financial forecasting can reveal potential cash flow problems that may hinder the growth and stability of the organization in the future.
In conclusion, financial forecasting is a valuable tool when it comes to strategic planning. It allows businesses to establish realistic goals based on their financial capabilities and identify potential problem areas before they become major obstacles. By incorporating financial forecasting into their strategic planning, organizations can make informed decisions and ensure a clear direction for growth and success.
Communicating Forecasts to Stakeholders
Building trust with investors.
Effective communication of financial forecasts is vital in building and maintaining trust with investors. Investors require clear, concise, and accurate information to make informed decisions based on a company’s financial projections. To establish this trust, ensure that your financial plan is:
- Transparent: Openly share the assumptions, data sources, and methodology used to arrive at the forecasts.
- Consistent: Apply a standardized approach to both the preparation and presentation of financial forecasts, comparable across time.
- Realistic: Avoid overly optimistic or pessimistic projections, as they can lead to broken trust and skepticism in future communications.
Displaying a strong understanding of finance coupled with effective communications skills conveys confidence in your forecasts, creating a positive impact on investors’ decision-making process.
Forecast Reports and Presentations
When presenting financial forecasts to stakeholders, clarity is key. Tailor your reports and presentations to the audience’s needs, emphasizing the most relevant and essential information. Consider the following strategies to enhance the effectiveness of your communication:
- Know Your Audience: Understand stakeholders’ backgrounds and needs, presenting insights in a way that resonates with their expertise and concerns.
- Use Visual Aids: Leverage graphs, charts, and tables to showcase patterns, trends, and key data points that support your financial projections.
- Highlight Key Metrics: Clearly highlight the most important performance indicators, providing context and insights for stakeholders to interpret the numbers.
By implementing these recommendations, reports and presentations on financial forecasts will better serve the stakeholders, emphasizing transparency, clarity, and confidence in the company’s financial plan.
Frequently Asked Questions
What are the different methods used in financial forecasting.
There are several methods used in financial forecasting, including the Straight-Line method, Moving Average method, Linear Regression, and Exponential Smoothing. These methods vary in complexity and are selected based on the nature of the data, business requirements, and forecasting goals.
Can you provide examples where financial forecasting is effectively applied in business?
Financial forecasting is effectively applied in various scenarios such as budget planning, performance evaluation, risk management, and investment decisions. For example, businesses use forecasting to estimate revenue, expenses, and cash flow for upcoming fiscal periods. Retailers may forecast sales trends to plan for inventory management or promotional campaigns, while financial institutions might use forecasting to estimate loan defaults, market fluctuations, or economic growth.
How does financial forecasting integrate with overall financial management?
Financial forecasting plays a crucial role in the overall financial management of a business. By providing insights into future operational and financial performance, it helps organizations make informed strategic decisions, plan for growth, allocate resources effectively, and manage potential risks. Furthermore, accurate financial forecasts enable businesses to set realistic expectations and improve budgeting, ultimately allowing for better financial control and management.
Which tools are commonly utilized for accurate financial forecasting?
Organizations use various tools and software solutions for accurate financial forecasting. These may include spreadsheet programs like Microsoft Excel, dedicated forecasting tools such as GMDH Streamline, or specialized enterprise resource planning (ERP) systems. Additionally, businesses may utilize custom-built models and applications, depending on their specific forecasting requirements and industry needs.
Why is it essential for organizations to conduct financial forecasts?
Financial forecasting is essential for organizations to make well-informed decisions, secure their future growth, and maintain a competitive edge within their industry. It helps businesses identify potential issues, evaluate the effectiveness of strategic initiatives, allocate resources more efficiently, and set realistic financial goals. Moreover, accurate forecasts enable decision-makers to anticipate market changes, quickly adapt to unforeseen events, and minimize risks associated with financial management.
How can financial forecasting influence the decision-making process in business planning?
Financial forecasting directly influences the decision-making process in business planning by providing key insights into future performance, opportunities, and threats. These insights help decision-makers evaluate different strategic alternatives, prioritize actions, and allocate resources effectively. Additionally, financial forecasts serve as a basis for setting short-term and long-term financial goals, tracking business progress, and adjusting plans as needed, ultimately promoting better business planning and decision-making.
- 1-800-711-3307
- Expense management
- Corporate card
- Tax returns & preparation
- Payment processing
- Tax compliance
- Vision & clarity
- Accounting mobile app
- Reduce your accounting expenses
- What does a bookkeeper do
- Why outsource
- Cash vs. Accrual Accounting
- Guides & ebooks
- How Finally works
- Privacy policy
- Terms of service
*Finally is not a CPA firm © 2024 Finally, Backoffice.co , Inc. All rights reserved.
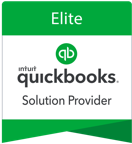
- Contact sales
Start free trial
Business Forecasting: Why You Need It & How to Do It

Table of Contents
What is business forecasting, the importance of business forecasting, business forecasting process, business forecasting methods, elements of business forecasting, sources of data for forecasting, business forecasting only goes so far, how projectmanager helps business forecasting.
Well-run organizations don’t fly by the seat of their pants; they’re constantly working on business forecasting and business planning. Every decision and every process is based on data obtained from business forecasting, business intelligence tools, market research and scenario planning. Companies focus their energies on ways to predict market trends to help them set successful long-term strategies.
Some business forecasts are based on highly sophisticated statistical methods while others are based on experience and past data. Others simply follow a gut feeling. One thing remains constant: all industries rely on business forecasting.
Business forecasting refers to the process of predicting future market conditions by using business intelligence tools and forecasting methods to analyze historical data.
Business forecasting can be either qualitative or quantitative. Quantitative business forecasting relies on subject matter experts and market research while quantitative business forecasting focuses only on data analysis.
You can access historical data with project management tools such as ProjectManager , project management software that delivers real-time data for more insightful business forecasting. Our live dashboard requires no setup and automatically captures six project metrics which are displayed in easy-to-read graphs and charts. Get a high-level view of your project for better business planning. Get started with ProjectManager for free today.
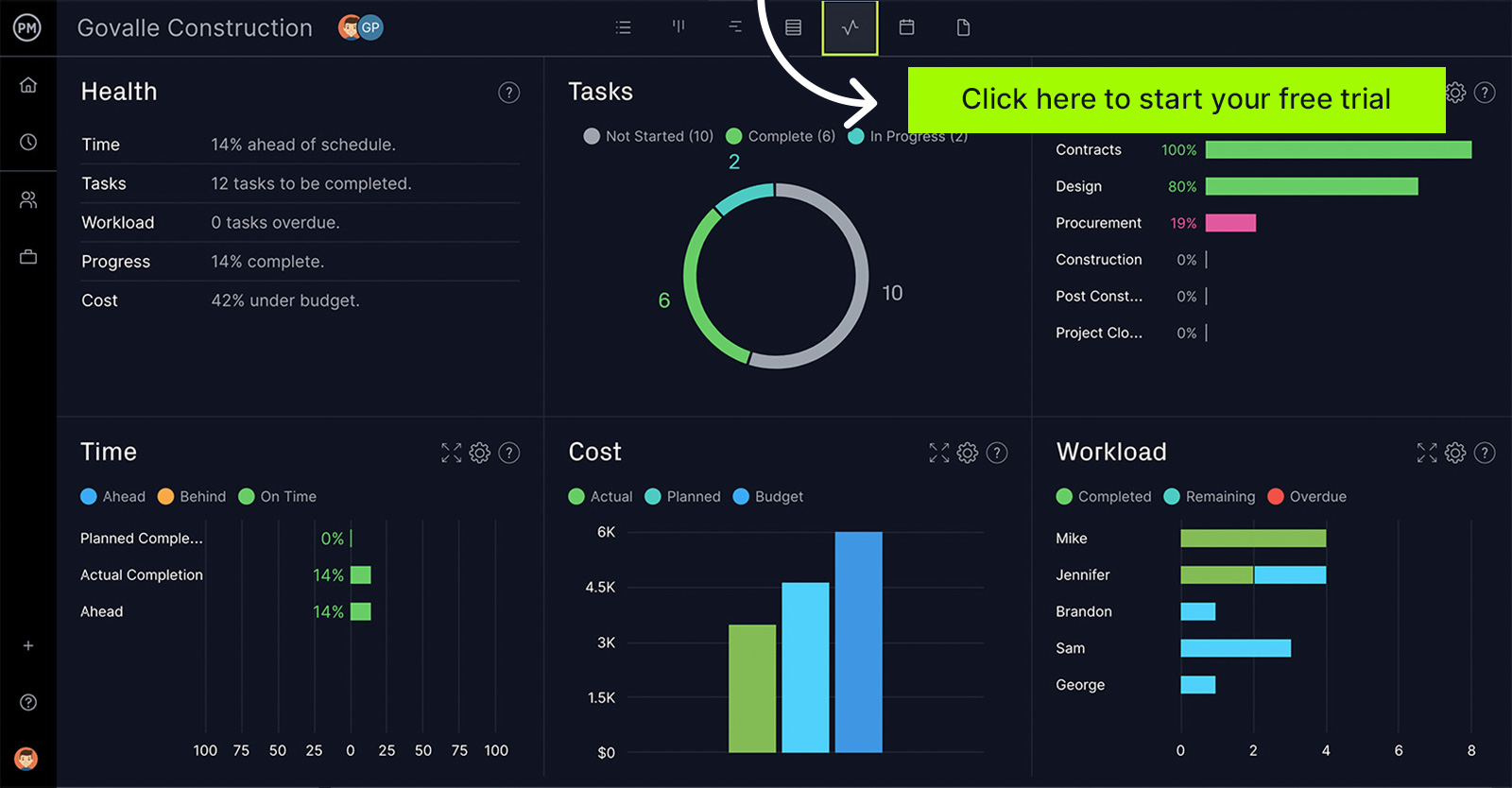
Quantitative Forecasting
Quantitative forecasting is applicable when there is accurate past data available to predict the probability of future events. This method pulls patterns from the data that allow for more probable outcomes. The data used in quantitative forecasting can include in-house data such as sales numbers and professionally gathered data such as census statistics. Generally, quantitative forecasting seeks to connect different variables in order to establish cause and effect relationships that can be exploited to benefit the business.
Qualitative Forecasting
Qualitative forecasting is based on the opinion and judgment of consumers and experts. This business forecasting method is useful if you have insufficient historical data to make any statistically relevant conclusions. In such cases, an expert can help piece together the known bits of data you do have to try to make a qualitative prediction from that known information.
Qualitative business forecasting is also useful when little is known about the future in your industry. Relying on historical data is useless if that data is not relevant to the uncharted future you are approaching. This can be the case in innovative industries, or if there’s a new constraint entering the market that has never occurred before such as new tax law.
Business forecasting is critical for businesses whenever the future is uncertain or whenever an important strategic business decision is being made. The more the business can focus on the probable outcome, the more success the organization has as it moves forward.
Here are the steps that a business forecaster should typically follow:
- Define the question or problem you need to solve with your business forecasting efforts. For example, you might be interested in estimating whether your organization will be able to meet product demand for the next quarter.
- Identify the datasets and variables that need to be taken into consideration. In this case, datasets such as the sales records from the previous year and variables related to capacity, production and demand planning .
- Choose a business forecasting method that adjusts to your dataset and forecasting goals. That depends on whether your problem or question can be solved using a qualitative, quantitative or mixed approach.
- Based on the analysis of historical data, you can proceed to estimate future business performance. Keep in mind that the accuracy of your business forecasting depends on the quality of your data.
- Determine the discrepancy between your business forecast and actual business performance. Document your findings and improve your business forecasting process.
As stated above, there are two main types of business forecasting methods, qualitative and quantitative. We’ve compiled some of the more common forecasting models from both sides below.
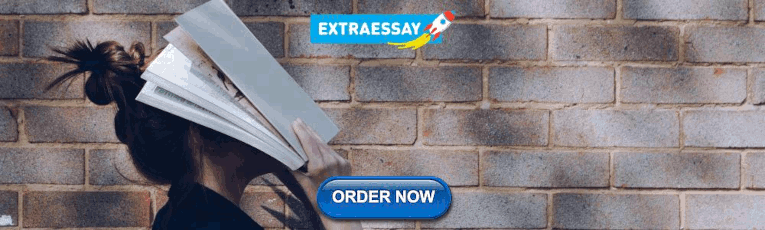
Delphi Method
This qualitative business forecasting method consists in gathering a panel of subject matter experts and getting their opinions on the same topic in a manner in which they can’t know each other’s thoughts. This is done to prevent bias , which makes it possible for a manager to objectively compare their opinions and see if there are patterns, consensus or division.
Market Research
There are many market research techniques that evaluate the behavior of customers and their response to a certain product or service. Some of those market research methods collect and analyze quantitative data, such as digital marketing metrics and others qualitative data, such as product testing, or customer interviews.
Time Series Analysis
Also referred to as “trend analysis method,” this business forecasting technique simply requires the forecaster to analyze historical data to identify trends. This data analysis process requires statistical analysis as outliers need to be removed. More recent data should be given more weight to better reflect the current state of the business.
The Average Approach
The average approach says that the predictions of all future values are equal to the mean of the past data. Past data is required to use this method, so it can be considered a type of quantitative forecasting. This approach is often used when you need to predict unknown values as it allows you to make calculations based on past averages, where one assumes that the future will closely resemble the past.
The Naïve Approach
The naïve approach is the most cost-effective and is often used as a benchmark to compare against more sophisticated methods. It’s only used for time series data where forecasts are made equal to the last observed value. This approach is useful in industries and sectors where past patterns are unlikely to be reproduced in the future. In such cases, the most recent observed value may prove to be the most informative.
- Develop the Basis: Before you can start forecasting, you must develop a system to investigate the current economic situation around you. That includes your industry and its present position as well as its popular products to better estimate sales and general business operations.
- Estimating Future Business Operations: Now comes the estimation of future conditions, such as the course that future events are likely to take in your industry. Again, this is based on collected data to help with quantitative estimates for the scale of operations in the future.
- Regulating Forecasts: Whatever your forecast is, it must be compared to actual results. This is the only way to find deviations from the norm. Then the reasons for those deviations must be figured out, so action can be taken to correct those deviations in the future.
- Reviewing Forecasting Process: By reviewing the deviations between forecasts and actual performance data, improvements are made in the process, allowing you to refine and review the information for accuracy.
Your forecast will only be as good as the data you put into it. Before collecting data, ask yourself these questions:
- Why collect data?
- What kind of data?
- When to collect it?
- Where to collect it?
- Who will collect it?
- How will it be collected?
These are the questions that will shape your plan for the collection of data, a crucial facet of business forecasting. Once you have your plan, you can collect data from a variety of sources.
Primary Sources
Primary sources contain first-hand data, often collected with reporting tools . These are the ones that you or the person assigned this task to collect personally. If primary data is not available, you must go out and source it through interviews, questionnaires or observations.
Secondary Sources
Secondary sources contain published data or data that has been collected by others. This includes official reports from governments, publications, financial statements from banks or other financial institutions, annual reports of companies, journals, newspapers, magazines and other periodicals.
If business forecasting were a crystal ball, then everyone would be reaping the rewards of their foresight. While business forecasting is a tool to get a better view of what the future might have in store, there’s the argument that it’s wasting valuable time and resources on little return.
It’s true; you can follow the steps, use a variety of methodologies and still get it wrong. It is, after all, the future. There’s no way to ever manage all the variables that can impact future events. There are errors in calculations and the innate prejudices of the people managing the process, all of which add to the unpredictability of the results.
While you’re not going to have a clear, unobscured vision of the future by using business forecasting, it can provide you with insight into probable future trends to give your organization an advantage. Even a small step can be a great leap forward in the highly competitive world of business. By combining statistical and econometric models with experience, skill and objectivity, business forecasting is a formidable tool for any organization looking for a competitive advantage.
Clearly, business forecasting is a project unto itself. To manage a project and collect the data in a way that’s useful in the future, you need a project management tool that can help you plan your process and select the data that helps you decide on a way forward.
ProjectManager is award-winning software that organizes projects with features that address every phase. The first thing in forecasting is choosing how you’ll take action and make a plan. For example, if you’re going to interview customers to see where the market is likely headed, you’ll need to schedule those interviews. Our online Gantt chart places those interviews as tasks on a timeline so you can get everyone interviewed before your deadline.
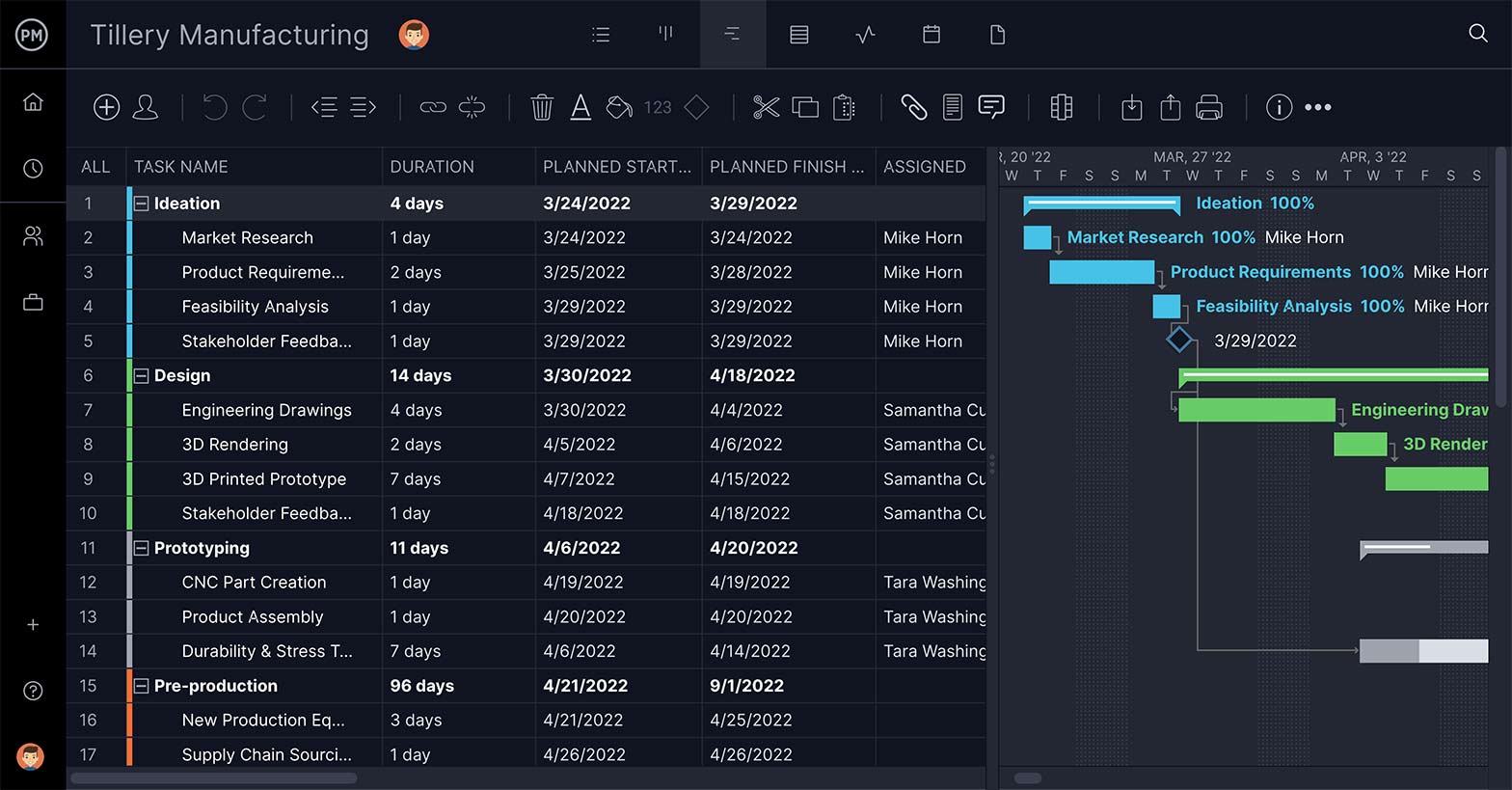
Store All of Your Data in One Place
Those interviews will produce a lot of paperwork, and your data needs to be collected and stored somewhere easily accessible. You can attach notes to each task so the paperwork for each interviewee is saved with the notes that you took. You can also tag those tasks to make it easier to filter the project and locate the interview subjects for which you’re looking. If you’re worried that there’ll be too many documents and images attached to one task, don’t worry as we have unlimited file storage.
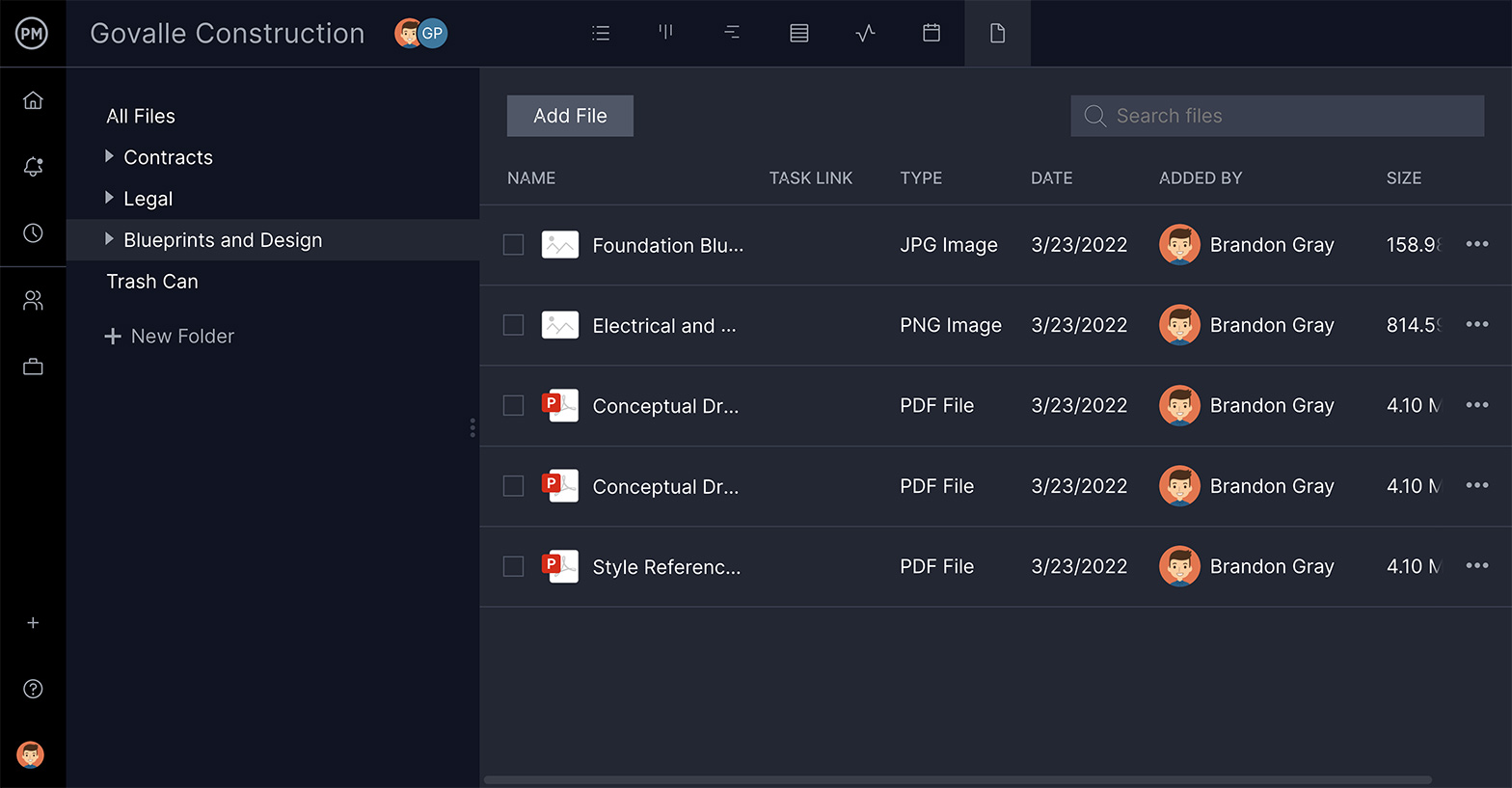
ProjectManager can’t predict the future, but it does provide you with the tools you need to take advantage of business forecasting. Our project management software collects data in real-time, and stores past data, allowing you to filter information and pull up the metrics you need to make the right decision. Try it today with this free 30-day trial.

Deliver your projects on time and on budget
Start planning your projects.
- Creative & Design
- See all teams
For industries
- Manufacturing
- Professional Services
- Consumer Goods
- Financial Services
- See all industries
- Resource Management
- Project Management
- Workflow Management
- Task Management
- See all use cases
Explore Wrike
- Book a Demo
- Take a Product Tour
- ROI Calculator
- Customer Stories
- Start with Templates
- Gantt Charts
- Custom Item Types
- Project Resource Planning
- Project Views
- Kanban Boards
- Dynamic Request Forms
- Cross-Tagging
- See all features
- Integrations
- Mobile & Desktop Apps
- Resource Hub
- Educational Guides
Upskill and Connect
- Training & Certifications
- Help Center
- Wrike's Community
- Premium Support Packages
- Wrike Professional Services
What Is Business Forecasting? Why It Matters
April 25, 2021 - 10 min read

Companies conduct business forecasts to determine their goals, targets, and project plans for each new period, whether quarterly, annually, or even 2–5 year planning.
Forecasting helps managers guide strategy and make informed decisions about critical business operations such as sales, expenses, revenue, and resource allocation . When done right, forecasting adds a competitive advantage and can be the difference between successful and unsuccessful companies.
In this guide to business forecasting, we'll cover:
- What is business forecasting?
- What are the best forecasting techniques?
- Why forecasting in management is important
- How to conduct business forecasts
- A few forecasting examples for businesses
An introduction to business forecasting
What is business forecasting? Business forecasting is a projection of future developments of a business or industry based on trends and patterns of past and present data.
This business practice helps determine how to allocate resources and plan strategically for upcoming projects, activities, and costs. Forecasting enables organizations to manage resources , align their goals with present trends, and increase their chances of surviving and staying competitive.
The purpose of forecasts is to develop better strategies and project plans using available, relevant data from the past and present to secure your business's future . Good business forecasting allows organizations to gain unique, proprietary insights into likely future events, leverage their resources, set product team OKR , and become market leaders.
Managers conduct careful and detailed business forecasts to guarantee sound decision-making based on data and logic, not emotions or gut feelings.
What are important business forecasting methods?
There are several business forecasting methods. They fall into two main approaches:
- Quantitative forecasting
Qualitative forecasting
Quantitative and qualitative forecasting techniques use and provide different sets of data and are needed at different stages of a product's life cycle.
Note that significant changes in a company, such as new product focus, new competitors or competitive strategies, or changing compliance requirements diminish the connection between past and future trends. This makes choosing the right forecasting method even more important.
Quantitative business forecasting
Use quantitative forecasting when there is accurate past data available to analyze patterns and predict the probability of future events in your business or industry.
Quantitative forecasting extracts trends from existing data to determine the more probable results. It connects and analyzes different variables to establish cause and effect between events, elements, and outcomes. An example of data used in quantitative forecasting is past sales numbers.
Quantitative models work with data, numbers, and formulas. There is little human interference in quantitative analysis. Examples of quantitative models in business forecasting include:
- The indicator approach : This approach depends on the relationship between specific indicators being stable over time, e.g., GDP and the unemployment rate. By following the relationship between these two factors, forecasters can estimate a business's performance.
- The average approach : This approach infers that the predictions of future values are equal to the average of the past data. It is best to use this approach only when assuming that the future will resemble the past.
- Econometric modeling : Econometric modeling is a mathematically rigorous approach to forecasting. Forecasters assume the relationships between indicators stay the same and test the consistency and strength of the relationship between datasets.
- Time-series methods : Time-series methods use historical data to predict future outcomes. By tracking what happened in the past, forecasters expect to get a near-accurate view of the future.
Qualitative business forecasting is predictions and projections based on experts' and customers' opinions. This method is best when there is insufficient past data to analyze to reach a quantitative forecast. In these cases, industry experts and forecasters piece together available data to make qualitative predictions.
Qualitative models are most successful with short-term projections. They are expert-driven, bringing up contrasting opinions and reliance on judgment over calculable data. Examples of qualitative models in business forecasting include:
- Market research : This involves polling people – experts, customers, employees – to get their preferences, opinions, and feedback on a product or service.
- Delphi method : The Delphi method relies on asking a panel of experts for their opinions and recommendations and compiling them into a forecast.
How do you choose the right business forecasting technique?
- Choosing the right business forecasting technique depends on many factors. Some of these are:
- Context of the forecast
- Availability and relevance of past data
- Degree of accuracy required
- Allocated time to conduct the forecast
- Period to be forecast
- Costs and benefits of the forecast
- Stage of the product or business needing the forecast
Managers and forecasters must consider the stage of the product or business as this influences the availability of data and how you establish relationships between variables. A new startup with no previous revenue data would be unable to use quantitative methods in its forecast.
The more you understand the use, capabilities, and impact of different forecasting techniques, the more likely you will succeed in business forecasting.
Why is business forecasting important?
Any insight into the future puts your organization at an advantage. Forecasting helps you predict potential issues, make better decisions, and measure the impact of those decisions.
By combining quantitative and qualitative techniques, statistical and econometric models , and objectivity, forecasting becomes a formidable tool for your company.
Business forecasting helps managers develop the best strategies for current and future trends and events. Today, artificial intelligence, forecasting software, and big data make business forecasting easier, more accurate, and personalized to each organization.
Forecasting does not promise an accurate picture of the future or how your business will evolve, but it points in a direction informed by data, logic, and experiential reasoning.
What are the integral elements of business forecasting?
While there are different forecasting techniques and methods, all forecasts follow the same process on a conceptual level. Standard elements of business forecasting include:
- Prepare the stage : Before you begin, develop a system to investigate the current state of business.
- Choose a data point : An example for any business could be "What is our sales projection for next quarter?"
- Choose indicators and data sets : Identify the relevant indicators and data sets you need and decide how to collect the data.
- Make initial assumptions : To kickstart the forecasting process, forecasters may make some assumptions to measure against variables and indicators.
- Select forecasting technique : Pick the technique that fits your forecast best.
- Analyze data : Analyze available data using your selected forecasting technique.
- Estimate forecasts : Estimate future conditions based on data you've gathered to reach data-backed estimates.
- Verify forecasts : Compare your forecast to the eventual results. This helps you identify any problems, tweak errant variables, correct deviations, and continue to improve your forecasting technique.
- Review forecasting process : Review any deviations between your forecasts and actual performance data.
How do you do business forecasting?
Successful business forecasting begins with a collaboration between the manager and forecaster. They work together to answer the following questions:
- What is the purpose of the forecast? How will it be used?
- What are the components and dynamics of the system the forecast is focused on?
- How relevant is past data in estimating the future?
Once these answers are clear, choose the best forecasting methods based on the stage of the product or business life cycle, availability of past data, and skills of the forecasters and managers leading the project.
With the right forecasting method, you can develop your process using the integral elements of business forecasting mentioned above.
How do you get data for business forecasting?
A forecast is only as good as the data supplied. Before collecting data, ask:
- Why do you need it?
- What kind of data do you need?
- When will you collect it?
- Where will you gather it?
- Who is in charge of collecting it?
- How will you collect it?
- How will you analyze it?
When you have these answers, you can start collecting data from two main sources:
- Primary sources : These sources are gathered first-hand using reporting tools — you or members of your team source data through interviews, surveys, research, or observations.
- Secondary sources : Secondary sources are second-hand information or data that others have collected. Examples include government reports, publications, financial statements, competitors' annual reports, journals, and other periodicals.
Business forecasting examples
Some forecasting examples for business include:
- Calculating cash flow forecasts, i.e., predicting your financial needs within a timeframe
- Estimating the threat of new entrants into your market
- Measuring the opportunity of developing a new product or service
- Estimating the costs of recurring bills
- Predicting future sales growth based on past sales performance
- Analyzing relationships between variables, e.g., Facebook ads and potential revenue
- Budgeting contingencies and efficient allocation of resources
- Comparing customer acquisition costs and customer lifetime value over time
What are the limits of business forecasting?
You can follow the rules, use the right methods, and still get your business forecast wrong. It is, after all, an attempt to predict the future. Some limits to business forecasting include:
- Biases and errors by the forecasters or managers
- Incorrect information from employees, experts, or customers
- Inaccurate past numbers
- Sudden change in market conditions
- New industry regulations
How Wrike helps with business forecasting
The more accurate your business forecasting, the more effective your strategies and plans can be. While many things in business are out of your control, having an informed forecast of what lies ahead makes you prepared and confident about the future.
Wrike helps gather data in one central platform, extract insights, and communicate findings with forecasters and managers. Other benefits of Wrike include real-time data, integrations with other forecasting software, streamlined collaboration, and visibility into every business forecasting project.
Are you ready to make projections for your business, allocate your resources for the best results, and improve your business forecasting process? Get started with a two-week free trial of Wrike today.
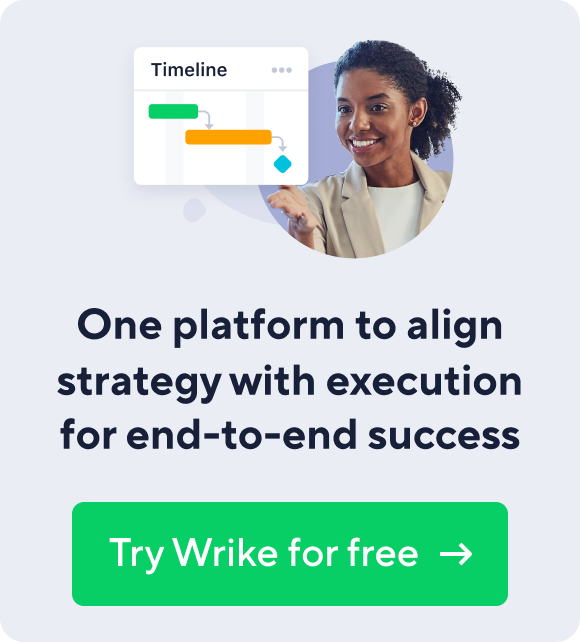
Kelechi Udoagwu
Kelechi is a freelance writer and founder of Week of Saturdays, a platform for digital freelancers and remote workers living in Africa.
Related articles
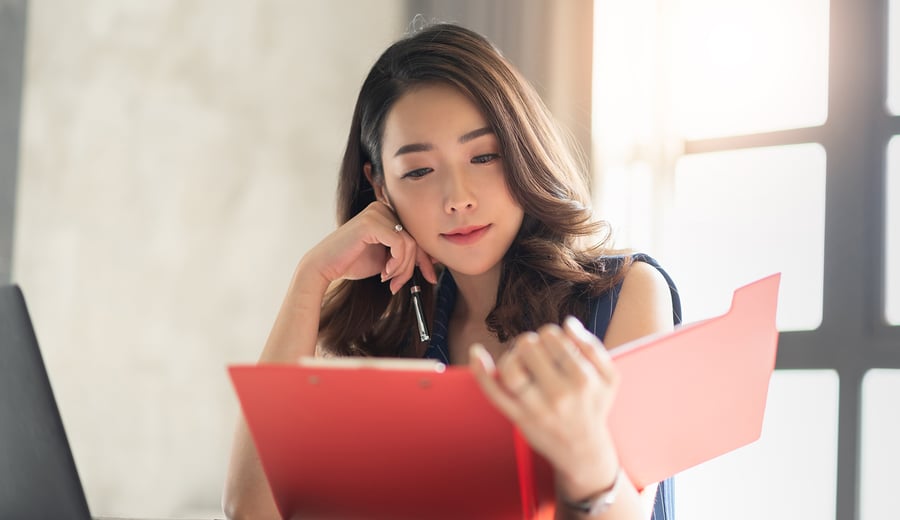
Strengthen and Optimize Agency Resource Management
Improve your agency’s resource management processes and learn how Wrike boosts performance across the board with our robust project management software.
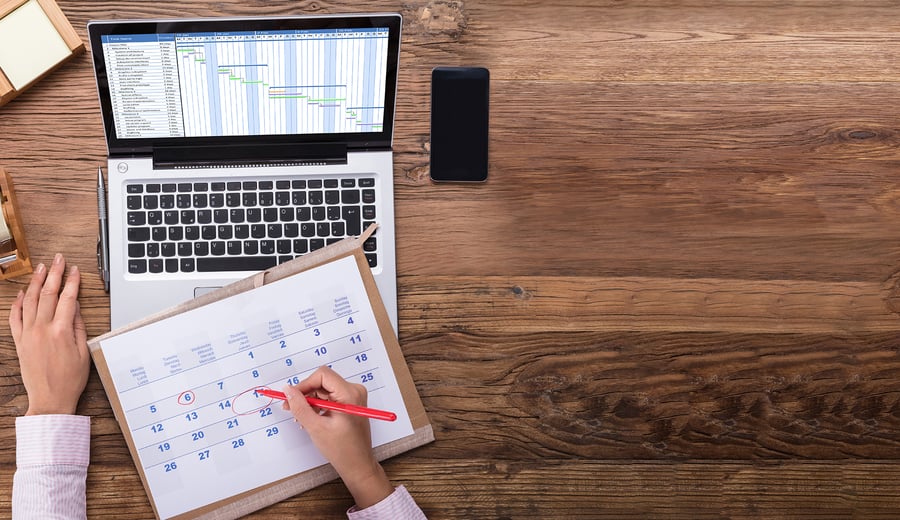
Release Management: Definition, Phases, and Benefits
What is release management and how can it improve software development strategy? In this guide, we talk about release management processes and their benefits.
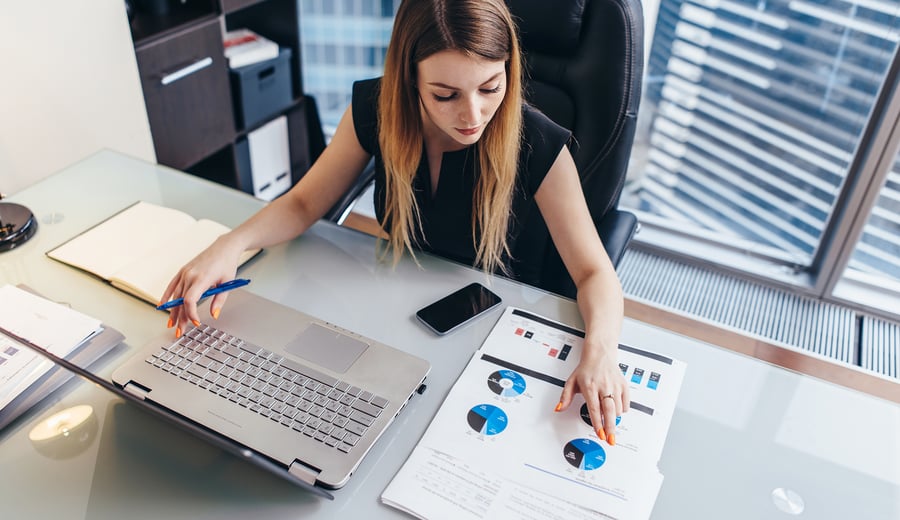
The Definitive Guide to Data-Driven Marketing
Wondering what data-driven marketing is and how to reap its benefits for your business? Find out how to create your own data-driven strategy with our guide.
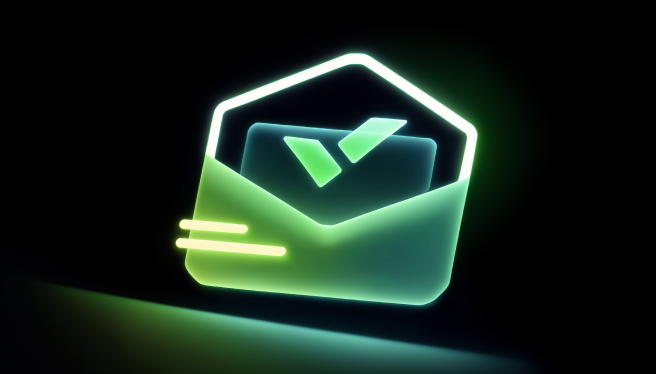
Get weekly updates in your inbox!
You are now subscribed to wrike news and updates.
Let us know what marketing emails you are interested in by updating your email preferences here .
Sorry, this content is unavailable due to your privacy settings. To view this content, click the “Cookie Preferences” button and accept Advertising Cookies there.
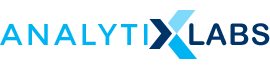
What is Business Forecasting And Its Methods?
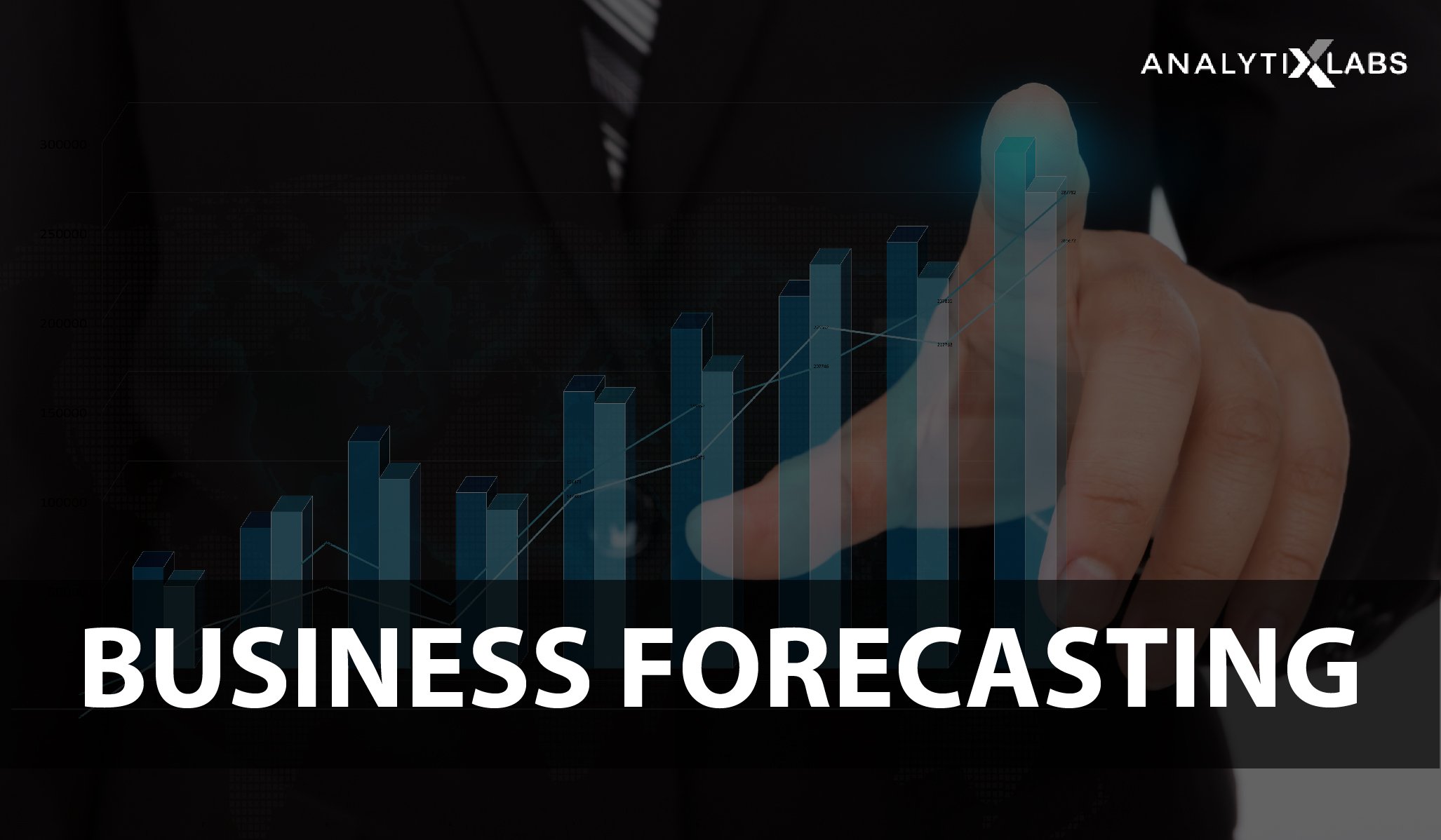
Forecasting plays an important role in developing business strategies as it helps make the leadership understand the upcoming future scenarios and how it might affect their business. Given the importance of business forecasting in today’s world, this article explores what is forecasting in business, the business forecasting methods, elements, and the role of it in business analytics.
Table of Contents
Business Forecasting Meaning
Business Forecasting is a broad term that refers to business forecasting techniques through the development of sophisticated models. These forecasting models help predict the numerous business developments that can happen in the near future which helps the business leaders make better decisions and avoid potential pitfalls.
About AnalytixLabs
AnalytixLabs is the premier Data Analytics Institute that specializes in training individuals as well as corporates to gain industry-relevant knowledge of Data Science and its related aspects. It is led by a faculty of McKinsey, IIT, IIM, and FMS alumni who have a great level of practical expertise. Being in the education sector for a long enough time and having a wide client base, AnalytixLabs helps young aspirants greatly to have a career in Data Science .
What is Business Forecasting ?
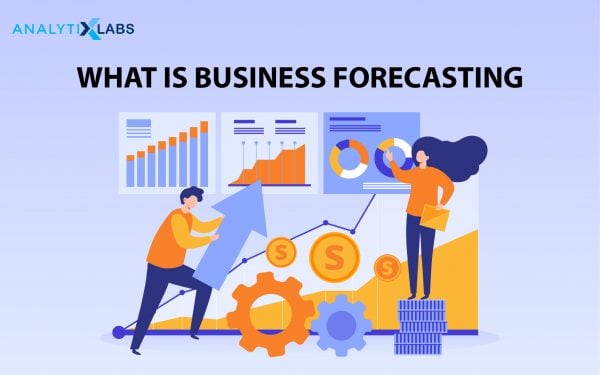
We all at one point or another have imagined how our life would have been if we knew what we know today. Imagine the catastrophe that could have been avoided if, for example, banking firms knew of the 2008 Global Recession or the profit that could have been made if investors knew of the potential of cryptocurrency. All of this leads to the need to develop strategies to forecast the future.
One must know that in Data Science, the terms “prediction” and “forecasting” differ significantly. Prediction refers to the prediction of a value by analyzing the relationship between a set of input variables and a target variable. On the other hand, forecasting is a whole different phenomenon that refers to foreseeing how some value or event would turn out to be over a period of time. Here, the past data is analyzed at the backdrop of time as the role of time is also taken into account to come up with the forecasted value.
While all businesses do some form of forecasting or other, the level of their business forecasting techniques can differ substantially. While a simple analysis can be done of the past data to understand the pattern and forecast the future of the business or economic events, other sophisticated statistical models can be put to use to know of the future. With the advancement of Machine Learning and Deep Learning, algorithms working under such an advanced setup can also be used.
Models of Business Forecasting
As mentioned earlier, there are various techniques through which business forecasting can be performed. These different business forecasting techniques give birth to the model of business forecasting where different techniques can be grouped as a model. There are mainly two types of models- Qualitative Models and Quantitative Models.
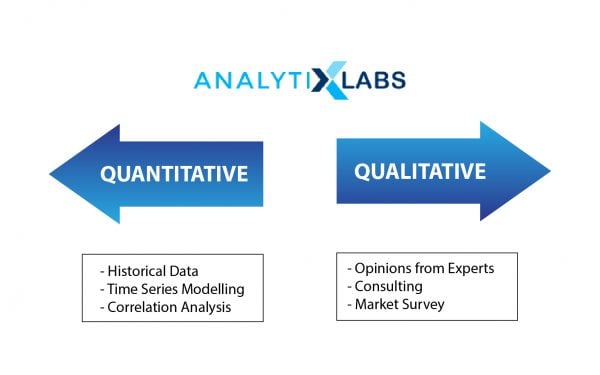
Qualitative Models
This forecasting model is used when trying to predict business important prediction over a short period of time. Here the business experts are consulted and their opinion forms one of the crucial inputs in coming up with the forecasted values. These business forecasting methods also are commonly used when there is a lack of historical data or the future seems too unstable to forecast using strictly a mathematical or statistical tool. While there are various methods that come under this model, the two most common business forecasting methods include Market Research and Delphi Model.
Quantitative Model
Unlike the Qualitative method with a human component involved in the forecasting that can potentially add to human bias or error in the process, the quantitative method solely relies on the historical data and understands the underlying pattern to know the future. This model is used when there is no dearth of historical data and there is a need to forecasting no only for the short term but medium or even long term. Among the Quantitative Model, there are a number of commonly used business forecasting techniques such as-.
Time Series Analysis
Time Series data focuses on the patterns found in the historical data and uses statistical methods to understand how time affects the target variable. Here, concepts such as analysis of the seasonality, trend, cyclicity, and irregularity found in historical data are used to understand the future better. This method emphasizes the use of statistics and methods such as ARIMA and SARIMA fall under it.
Econometric Method
Unlike Time Series analysis which uses the discipline of statistics, Econometric method uses a mathematically exhaustive technique to perform business forecasting. While Time Series analysis is used in a more general business environment, the econometric method is used in the world of academia to analyze the outcome of economic policies. The data used in such a form of analysis is highly complex and these techniques take into account factors such as the fluctuating significance of past data points and the fact that the impact of predictors on the target variable can change over time.
Indicator Approach
As the name suggests, this method is taken into account certain indicators to estimate the future. These indicators can be Key Performance Index (KPI) measuring the business performance, or government-provided data such as employment rate, inflation rate, GDP, etc. Here the relationship between these indicators is analyzed and uses the leading indicators to estimate the lagging ones.
While these are the main models of business forecasting, the readers must know that this is not the exhaustive list and other models such as projection models and casual models also exist that are used in specific scenarios.
What is Forecasting in Business?
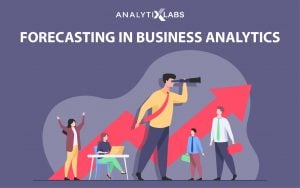
Business Analytics refers to that peculiar stream of analytics that deals with business and business-related problems. Often exploratory analysis is enough to understand the business scenario and bring to light the unknown facts by exposing hidden patterns inside the data. However, there are times when the business requires knowledge of the future and knowledge of the possible events that can happen in the future such as strikes, changes in demand, anomalies in the economy, or even the things that the competitors might do to change the business landscape drastically. While these examples are particular, these can be as simple as knowing the future sales, demand, etc to plan out logistics, inventory management or make the day to day processes more efficient. All these factors combined make the business leadership wish for tools that can predict the future so that they have enough time to prepare for and avoid catastrophic events. As this forecasting directly affects the business, it plays a crucial role in Business Analytics.
Today, Business Analysts need to come up with at least an annual forecast, make sure that the data is collected so that forecasted techniques can be implemented, and are tasked with making these projections available to the leadership in a simple, easy to understand manner. A good Business Analyst has to be good with the statistical and mathematical techniques and has to have good instincts, experience, business acumen, and economic policy knowledge to come up with accurate forecasts.
Elements of Business Forecasting
While there are various elements to any business forecasting model, some elements of forecasting form the core of any model and must be followed by anyone involved in the development of such models.
- Basic Development
The most basic elements of forecasting is setting up an in-depth process to know its current situations. This includes knowing the present stage the business is at and the development status of its various products. An analysis is also to be done regarding the present economic conditions, industrial policies, and the overall business scenario. This helps in knowing the starting point from where the forecasting is to be performed.
- Business Development
Once the basics of forecasting are developed, the next important element is to estimate the development of the business. This includes knowing the steps that the business is taking in the upcoming time to achieve some goals that the business leaders have in mind and the course of action the business is going to take. In addition to this, the upcoming conditions and plausible events that can happen in the concerned industry are estimated. This estimation is done based on experienced experts’ insight (qualitative model) or the analysis of the historical data (quantitative model) or both.
- Tuning of Model
This element focuses on the evaluation of the forecasting model by comparing the forecast done for a historical timeframe and comparing it with the actual values. Here the error needs to be quantified and then minimized by understanding the reasons for the error. These errors or deviations form the basis to fine-tune the model, take anomalies/outliers into account, and use different approaches until that method is figured out, which gives the best result.
- Implementing of Model
Once the model is finalized, the model is to be implemented to perform forecasting. On the basis of the forecasted values, business strategies are to be formulated and policies are to be driven. All of this must be done to keep in mind the error that can be there between the forecasted and actual values. The crucial element here is the perpetual improvement that is to be made in the data collection, model implementation, and other processes to maximize the accuracy of the forecasted values.
Importance of Business Forecasting
The importance of Business Forecasting can be understood once we understand the challenges different industries face and how forecasting can dramatically reduce those challenges. All these problems can be solved by forecasting different ranges viz. short, medium, and long term forecasting.
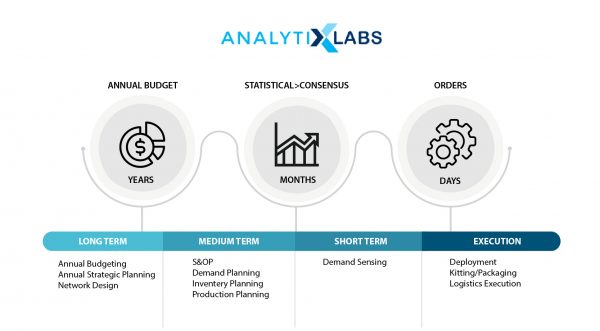
- Short Term Forecasting
This forecasting level is generally doney for up to 1 year (and is often less than 3 months). A business that faces challenges such as inventory management and needs to know the number of products to purchase this forecasting type. This idea can be extended to forecasting workforce requirements, job assignments, etc.
- Medium-Term Forecasting
The importance of business forecasting can be best understood here as often businesses face challenges in knowing the scenario that happens at a medium time range. This range is generally considered between 1 year (or 3 months) to 3 years. Business leaders often need to forecast sales, demand, profit, or loss to form strategies, budgets, and other things, which is where forecasting techniques come in handy. Also, businesses need to delve into research and development of products before time. The competitors don’t have the edge over them, and for this, demand for certain products is to be forecasted.
- Long Term Forecast
Forecasting is done over 3 years time span is considered as Long Term Forecasting. Her,n economic forecasting is often done,e such as understanding Macro Economic, which includes knowing of inflation, job requirements, money supply, etc. Knowing all this can help governments and other big entities to plan their policies accordingly.
Why is Forecasting So Important
Business Forecasting is useful in making a better decision and reducing the chances of a business venture to fail, however, forecasting in itself is also of high importance. Forecasting apart from the typical business environment can be used for other causes. For example, the most common forecast, weather forecast allows saving lives by alerting the authorities of natural calamities such as floods, hurricanes, etc.T his forecasting is now helping the government, NGOs, and other agencies prepare for contingencies such as water scarcity that can hit various countries in the near future, or the rising water levels that can engulf various coastal cities throughout the world.
You may also like to read about other important Data Mining Techniques & Methods
Conclusion: Authors Opinion
Everyone wants to know about the future, this has been the case for hundreds of years (e.g. since the Maya Civilization), thus no one can underestimate the importance of forecasting. Today, business forecasting is considered one of the most important and crucial aspects of any business working. Thus, any professional in the field of Data Science, Analysis, Strategist, or Policymaking need to know of the various methodologies and usage of forecasting and must use it to its full potential.
To get started with Business Forecasting, you can start learning from our premium business analytics learning materials . Have more doubts, you can also book a demo with us!
- What do you mean by business forecasting?
Business forecasting refers to the field of forecasting that deals with forecasting business scenarios or events or predicting values over time that will be generated by a certain business. It has emerged as the most important aspect of planning and operating any form of business.
- What are the types of business forecasting?
There are multiple types of business forecasting. These include forecasting using experts with in-depth business knowledge,e which forms a kind of business forecasting model known as qualitative models. Another type is of a quantitative model that removes human involvement and purely uses data to perform forecasting. Business forecasting methods such as time series forecasting and econometric methods are considered part of it.
- How is forecasting used in business?
The importance of business forecasting can be understood by knowing the multiple ways in which various business entities use forecasting. Companies require forecasting to know their product’s demands or sales to manage their inventory to create budgets. Forecasting also helps to know the future profit or losses that a company might incur r, which can help create appropriate policies. Also, forecasting helps in knowing the future competition and the products that might be there in the market which can help the business to design better products. Apart from inventory and workforce management, forecasting can help the business leaders know of the future economic situation that can help them take important investment-related decisions.
- Engagement: Ask readers to post their queries & opinions in the comments below. So that we can help them out.
This article aimed at providing the reader with an understanding of Business Forecasting (and forecasting in general too), its role and importance in the world of Business, and the various techniques and models related to it. If you have any opinions or queries related to this article, please feel free to post and help us get more insights.
You may also like to read: 1. Top 40 Data Analyst Interview Questions & Answers 2. What is Linear Regression In ML? With Example Codes 3. Logistic Regression in R (With Examples)
Related Posts

Top 12 AI Tools for Data Analysis To Include In Your Tech Stack

How To Become a Business Analyst – Steps and Learning Roadmap
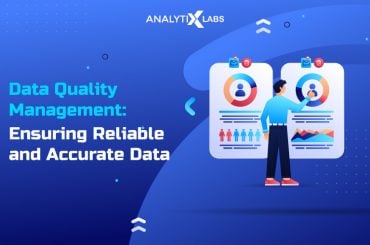
What Is Data Quality Management (DQM): Importance, Framework, Challenges and more
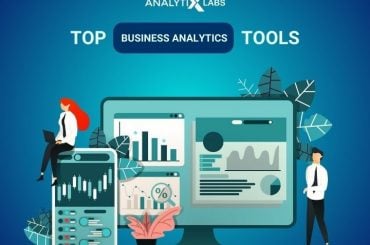
Top 15+ Essential Business Analytics and Associated Tools
Your blog post on business forecasting models is a concise yet informative guide to the world of predictive analytics. I appreciated how you provided a comprehensive overview of various forecasting techniques and their applications in strategic decision-making.
Write A Comment Cancel Reply
Save my name, email, and website in this browser for the next time I comment.
Type above and press Enter to search. Press Esc to cancel.
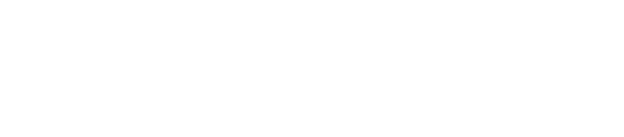
Thank You For Your Interest
We have received your query and will get back to you in 24 hours.
Need help? Call us at +91-95552-19007
Want to browser our courses? Visit our Homepage
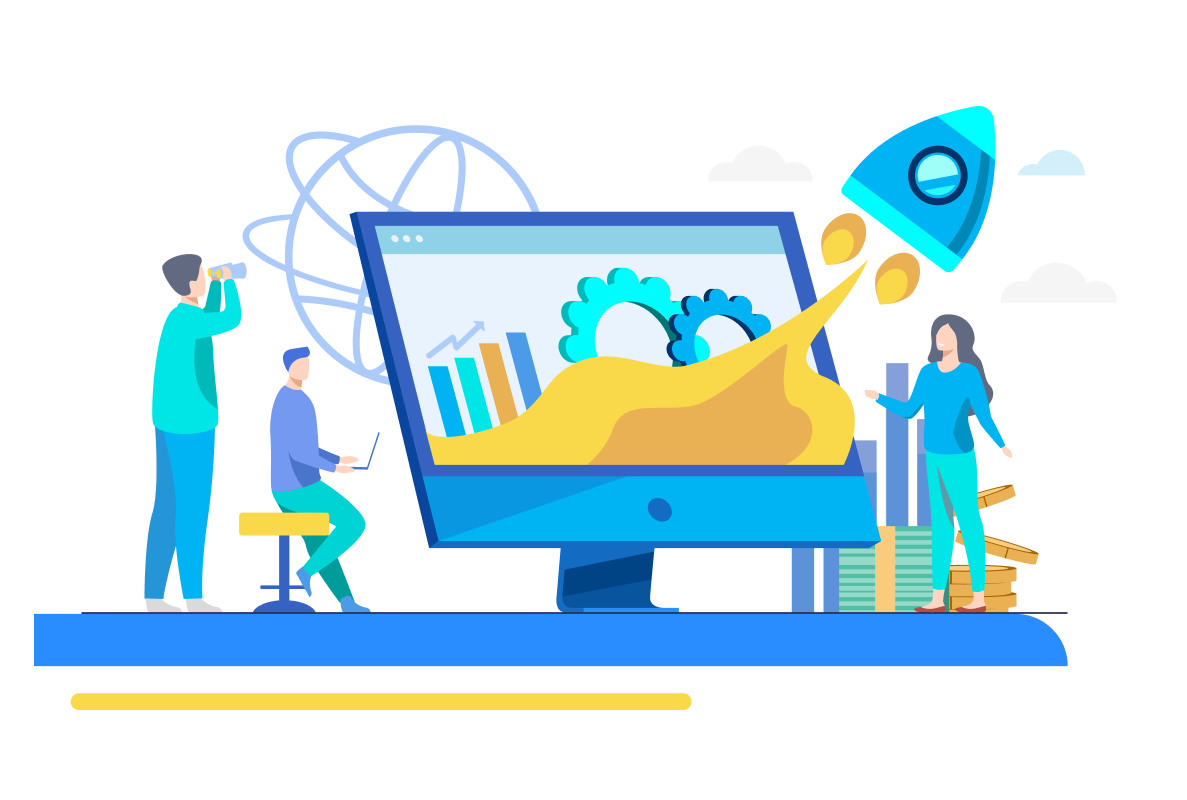
Business Forecasting: Types, Techniques, Need, Advantages, Limitations
Types of business forecasting, general business forecasting, sales forecasting, capital forecasting, techniques of business forecasting, quantitative techniques, qualitative techniques, steps involved in business forecasting, understanding the problem, developing the groundwork, selecting and analyzing data, estimating future events, need for business forecasting, production planning, financial planning, economic planning, workforce scheduling and handling, decision making, advantages of business forecasting, focuses on future, focuses on customer satisfaction, considers risk, allows learning from past mistakes.
Business forecasting enables an organization to look into the past and learn from its mistakes. It lets a business know what mistakes have been made in the past and should not be repeated in the future. It prevents you from taking such decisions, which have caused a huge loss or problems in the past.
Disadvantages Of Business Forecasting
Forecasting is never accurate, time consuming.
Forecasting is a very time consuming process as it requires gathering of several past information, checking the accuracy of information, analyzing it, grouping of similar data, finding relationship or pattern among them and afterwards judging and making interpretations. This takes a lot of time. In fact, gathering all relevant data from several departments and processes and analyzing and grouping them takes a lot of time, which the rest of the process cannot take.
Concluding Remarks
Business forecasting is a very important process as it helps an organization predict the uncertain future and develop itself to face any crisis or risk. Although forecasting is never accurate, it still helps business enterprises a lot to make assumptions related to the opportunities and threats in the future. However, it depends on the size of the organization whether it wants to include business forecasting in its operations or not.
Related posts:
Add commercemates to your homescreen.

- Group Training
- Individual Training

- Individual Certification
- Corporate Certification
- Companies Certified
- Testimonial
- Companies w/ Certification
- Levels of Certification
- Certification Details
- Sample Exams
- Recertification
- Preparation Materials
- Exam Schedule
- CPF Prep/Review

- Research Reports
- Virtual Conference Recordings

- Cancellation Policy
- Refund Policy for Events
- Ambassador Program
- Member Companies
- Partnership
- Apply to Speak
- Subscription
- S&OP Self-Assessment
- Sponsored Content
- Become a Sponsor
- Exhibit at IBF Events

--> --> |
by clicking on "ACCEPT" |
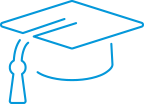
IBF started in 1981 with the Journal of Business Forecasting aiming to advance the growth of business forecasting and planning.
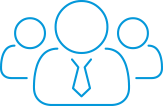
Active IBF members benefit from access to research, our Journal of Business Forecasting (JBF), discounted conferences, certification, training, and a host of online resources.
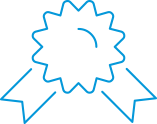
There’s a CPF or ACPF professional in every Fortune 500 company and other major global organizations, providing valuable insight and driving growth.
The Institute of Business Forecasting and Planning is the membership organization for S&OP, forecasting, demand planning, business analytics, and supply chain professionals. Through our industry leading conferences, training and industry-recognized certifications, we advance the forecasting and planning disciplines, and the careers of those in the field.
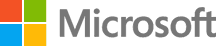
What is Forecasting?
Budgeting vs. forecasting.
- Forecasting Methods
Features of Forecasting
The process of forecasting, sources of data for forecasting, additional resources, forecasting.
Predicting what will happen in the future by taking into consideration the events from the past and present
Forecasting refers to the practice of predicting what will happen in the future by taking into consideration events in the past and present. Basically, it is a decision-making tool that helps businesses cope with the impact of the future’s uncertainty by examining historical data and trends. It is a planning tool that enables businesses to chart their next moves and create budgets that will hopefully cover whatever uncertainties may occur.
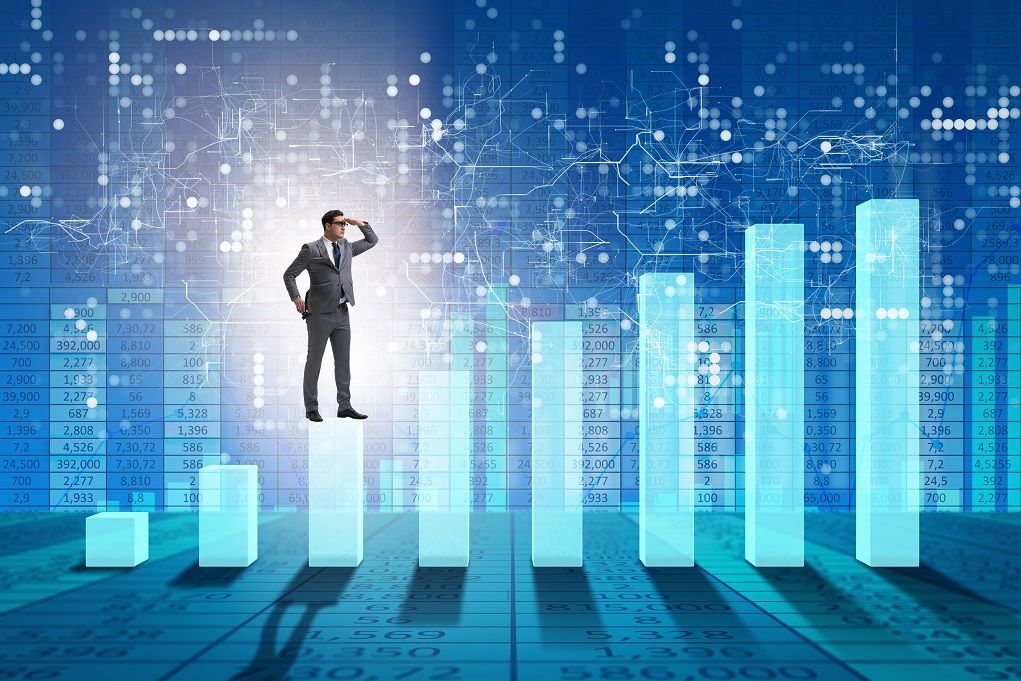
Budgeting and forecasting are both tools that help businesses plan for their future. However, the two are distinctly different in many ways:
- Budgeting involves creating financial statements for a specific period, such as projected revenue , expenses, cash flow, and investments. It is usually conducted with input from many different departments, because it requires input from multiple departments in order to come up with a holistic and detailed report. Therefore, the budgeting process takes time to complete. The company uses the budget to guide it in its financial activities. In other words, a budget is a plan for a company’s future.
- While budgets are usually made for an entire year, forecasts are usually updated monthly or quarterly. Through forecasting, a company can project where it’s going, and it may adjust its budget and allocate more or less funds to an activity, depending on the forecast. In summary, budgets depend on the forecast.
Key Highlights
- Forecasting refers to the practice of predicting what will happen in the future by taking into consideration events in the past and present.
- While related, budgets and forecasts are separate concepts: a budget is a plan for a company’s future, whereas a forecast is a sign of where the company is going. Based on the forecast, a budget may be altered to better reflect reality.
- Both qualitative and quantitative methods are used when developing a forecast.
Businesses choose between two basic methods when they want to predict what can possibly happen in the future: qualitative and quantitative methods.
1. Qualitative method
Otherwise known as the judgmental method, qualitative forecasting offers subjective results, as it is comprised of personal judgments by experts or forecasters. Forecasts are often biased because they are based on the expert’s knowledge, intuition and experience, making the process non-mathematical.
One example is when a person forecasts the outcome of a finals game in the NBA based more on personal motivation and interest. The weakness of such a method is that it can be inaccurate and biased.
2. Quantitative method
The quantitative method of forecasting is a mathematical process, making it consistent and objective. It steers away from basing the results on opinion and intuition, instead utilizing large amounts of data and figures that are interpreted.
Here are some of the features of making a forecast:
1. Involves future events
Forecasts are created to predict the future, making them important for planning.
2. Based on past and present events
Forecasts are based on opinions, intuition, guesses, as well as on facts, figures, and other relevant data. All of the factors that go into creating a forecast reflect some extent what happened with the business in the past and what is considered likely to occur in the future.
3. Uses forecasting techniques
Most businesses use the quantitative method, particularly in planning and budgeting .
Forecasters need to follow a careful process in order to yield accurate results. Here are some steps in the process:
1. Develop the basis of forecasting
The first step in the process is investigating the company’s condition and identifying where the business is currently positioned in the market.
2. Estimate the future operations of the business
Based on the investigation conducted during the first step, the second part of forecasting involves estimating the future conditions of the industry where the business operates and projecting and analyzing how the company will fare in the future.
3. Regulate the forecast
This involves looking at different forecasts in the past and comparing them with what actually happened with the business. The differences in previous results and current forecasts are analyzed, and the reasons for the deviations are considered.
4. Review the process
Every step is checked, and refinements and modifications are made.
1. Primary sources
Information from primary sources takes time to gather because it is first-hand information, also considered the most reliable and trustworthy sort of information. The forecaster does the collection, and may do so through things such as interviews , questionnaires, and focus groups.
2. Secondary sources
Secondary sources supply information that has been collected and published by other entities. An example of this type of information might be industry reports. As this information has already been compiled and analyzed, it makes the process quicker.
Thank you for reading CFI’s guide to Forecasting. To keep learning and advancing your career, the following CFI resources will be helpful:
- Prediction Market
- Regression Analysis
- See all valuation resources
- See all accounting resources
- Share this article
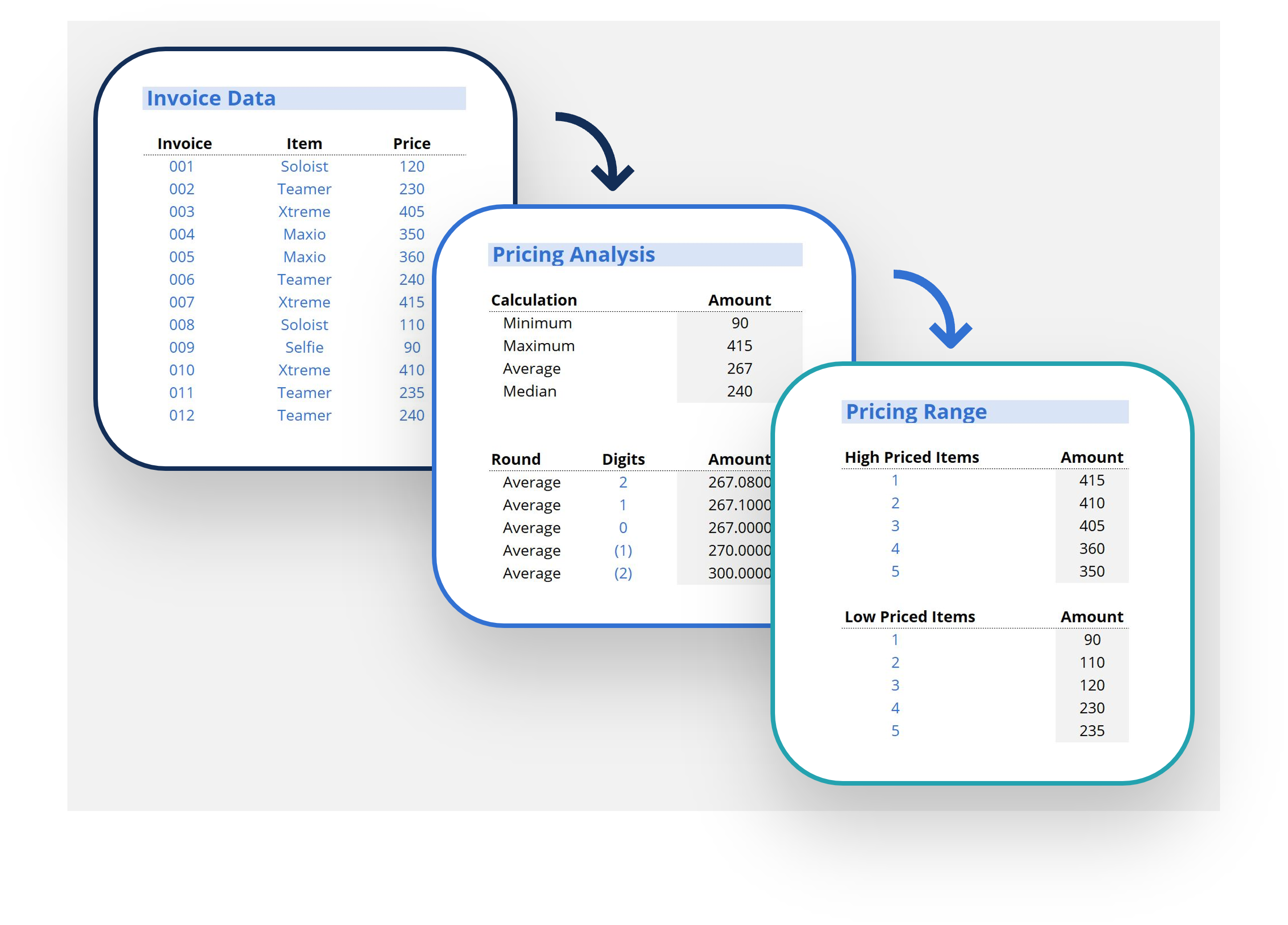
Create a free account to unlock this Template
Access and download collection of free Templates to help power your productivity and performance.
Already have an account? Log in
Supercharge your skills with Premium Templates
Take your learning and productivity to the next level with our Premium Templates.
Upgrading to a paid membership gives you access to our extensive collection of plug-and-play Templates designed to power your performance—as well as CFI's full course catalog and accredited Certification Programs.
Already have a Self-Study or Full-Immersion membership? Log in
Access Exclusive Templates
Gain unlimited access to more than 250 productivity Templates, CFI's full course catalog and accredited Certification Programs, hundreds of resources, expert reviews and support, the chance to work with real-world finance and research tools, and more.
Already have a Full-Immersion membership? Log in
- Build Compensation Plans
- Automate Commissions
- Pay Sales Commissions
- Drive Sales Revenue
- Integrations
- Resource Center
- Reports and Guides
- Learning Center
- Compensation Plan Templates
- Calculators
- Partner Program
- Talk to Sales
- Try for Free
How To Get An Accurate Sales Forecast With Demand Forecasting
By QuotaPath Team | August, 2024 | 9 mins
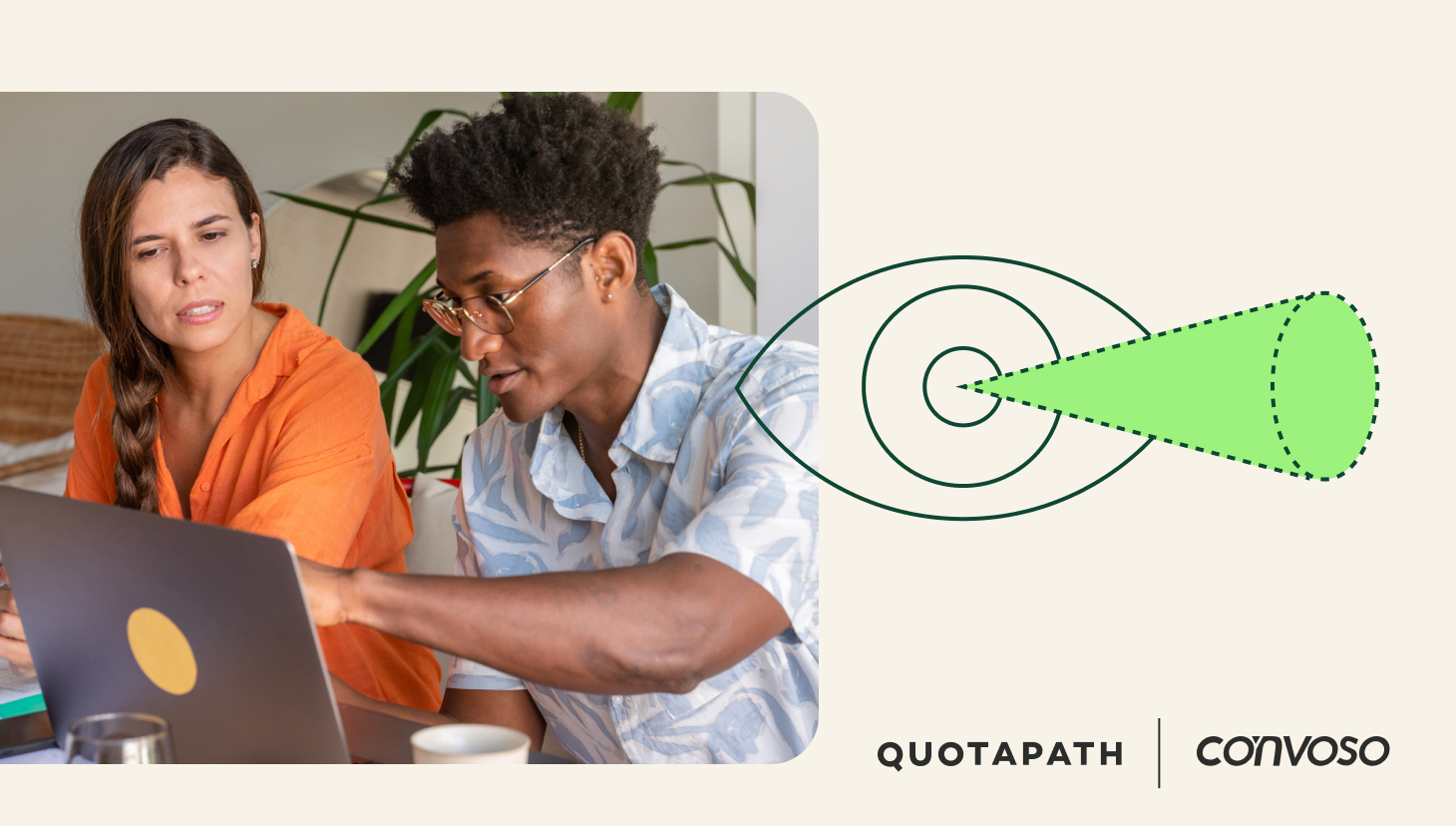
Sales forecasting is one of the most essential yet challenging aspects of business. However, having an accurate estimate of revenue through a sales forecast can shape your success.
Everything from developing budgets to planning production capacity relies on this forecast. That’s why accurate insights are crucial.
The solution? Demand forecasting. Here, we’ll explore why this process is so beneficial and strategies to implement.
What is demand forecasting and why is it important?
Demand forecasting is the process of estimating future sales of your products or services by using relevant data to predict demand. The aim is to remove the guesswork by gaining a more accurate view of predicted revenue streams to help better manage budgets and sales cycles .
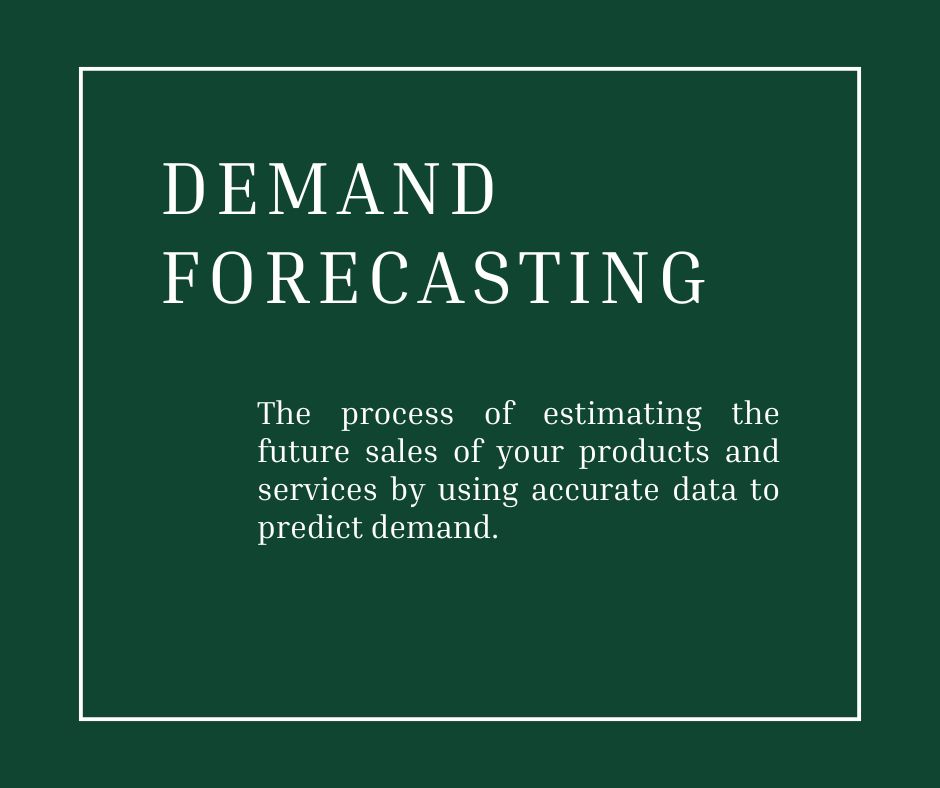
Why is this important? One aspect is streamlining. Accurate forecasts allow you to anticipate fluctuations in demand to better allocate resources. Determining stock levels helps avoid understocking inventory or product obsolescence. It’s also beneficial for avoiding lost sales opportunities.
All of this has a knock-on effect on operational efficiency and, consequently, brand awareness . It ensures you’re always able to meet customer demand. This, in turn, can increase your company’s profitability, protect your brand reputation, and ensure steady business growth.
Key benefits of accurate demand forecasting
As we mentioned, demand forecasting offers numerous benefits, from resource allocation to sales opportunities. Let’s dive into these in more detail.
Inventory management
Demand forecasting in supply chain and inventory management is essential for maintaining optimal stock levels throughout the year.
Rather than guessing what you need and when, you can use forecasts to reduce the potential of stockouts, improve ordering efficiency, optimize revenue management, and save on the cost of storage. By ensuring a healthy fill rate , you can meet customer demand consistently while minimizing the risk of understocking or overstocking inventory. You could even save on transport costs by spotting ways to consolidate orders.
Resource allocation
Anticipating demand equips you with the knowledge to better plan and allocate resources. Whether it’s production capacity, manpower, or marketing budgets, you can approach allocation strategically to be more effective during times of high demand and scale back during quieter periods.
Budgeting and planning
Demand forecasting gives you some of the best insights to plan budgets. This can help you decide how much to spend on areas like marketing, hiring, or product development, depending on what resources you will need and how much revenue you anticipate coming in.
Utilizing budgeting software can streamline this process further by automating data analysis and providing real-time insights into budget allocation. With budgeting software, you can efficiently track expenses, identify areas for cost-saving, and make informed decisions to maximize your resources and optimize financial performance.
You may even be able to spot investment opportunities. Automation is becoming increasingly prevalent in businesses today, so you may free up the budget for click to call dialer software to support your sales team’s outreach efforts or customer service chatbots to improve conversion rates.
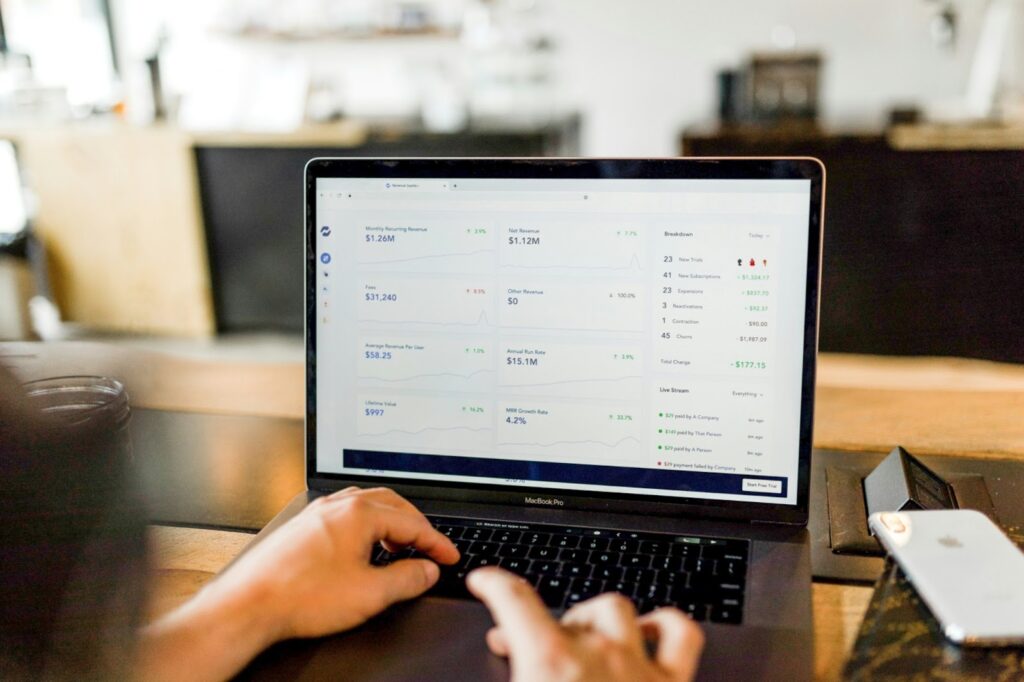
Risk management
Demand forecasting helps identify potential risks and uncertainties related to sales performance. This way, you can be better prepared to manage challenges.
For example, if your demand forecast indicates a potential downturn in sales during a specific season, you can adjust inventory levels or implement targeted email marketing campaigns to give sales a boost.
Improved decision-making
Any forecasting model replaces guesswork with reliable information specific to your business and customers. With that information, you can make more informed decisions about future sales trends.
For example, if your forecast indicates a surge in demand for a particular product, you can adjust your pricing strategies. This could mean offering promotions or increasing sales incentives during peak demand periods to capitalize on increased customer interest.
Understanding cross-channel demand patterns, particularly in the context of omnichannel ecommerce , becomes crucial for effectively allocating resources and maximizing sales across multiple sales channels.
5 steps for effective demand forecasting
While demand forecasting can benefit your company, it’s important to do it correctly if your strategy is to be successful. We’ve broken it down into five easy steps.
1. Collect data relevant to your goals
Begin by collecting and analyzing relevant data available that aligns with your sales and business growth goals. This information could include any or all of the following:
- Historical data: Past sales data can tell you a lot about what might happen in the future. Pull data from sources like your CRM, inventory management software, call center reporting tools , customer forms, and even other departments. Review previous demand, looking out for patterns, trends, and seasonal fluctuations that are likely to repeat.
- Market research: Gather information about your target customers, including their preferences, buying habits, and what influences their purchasing decisions. This could be done through surveys, interviews, or analyzing existing market studies.
- External factors: Industry trends and competitor activities can impact your forecast. But it’s also worth gathering data on economic conditions and socio-political factors like regulation changes or consumer sentiment. For example, a new competitor entering the market might affect demand for your products, or a change in government policy might influence consumer buying behavior.
2. Implement your chosen forecasting methods
Once you have the data, you must analyze it to draw conclusions. You can do this manually or with the help of automation. Let’s explore a few options.
Statistical models
Statistical techniques like time series analysis and regression analysis are useful for creating forecasting models.
Time series analysis looks at patterns in historical data over time, such as increases or decreases in sales during certain months.
Regression analysis identifies relationships between variables. It considers how factors like marketing spend, promotions, and economic conditions have impacted sales.
Conducting an in-depth analysis of large data sets manually can be challenging. However, machine learning algorithms make this process more efficient and can help identify complex patterns, resulting in more accurate predictions.
Forecasting software
As with many business processes, software can make forecasting much smoother. If you choose the right model, it can help to automate data collection and analysis and generate reports for you.
But it’s crucial to choose the right solution for your business needs. You should also understand how that solution creates the forecasts so you can make better decisions.
Remember that your existing software such as your ERP system may have built-in forecasting capabilities. Or you may need a customized program for your specific industry.
Collaborative forecasting
Technology isn’t always the answer. Bring together different departments to share insights and perspectives to help shape your forecast.
For example, a software company launching call scri pt software might get input from their sales team on customer demand and predicted sales. The marketing team would provide campaign insights, while the finance department could offer financial projections.
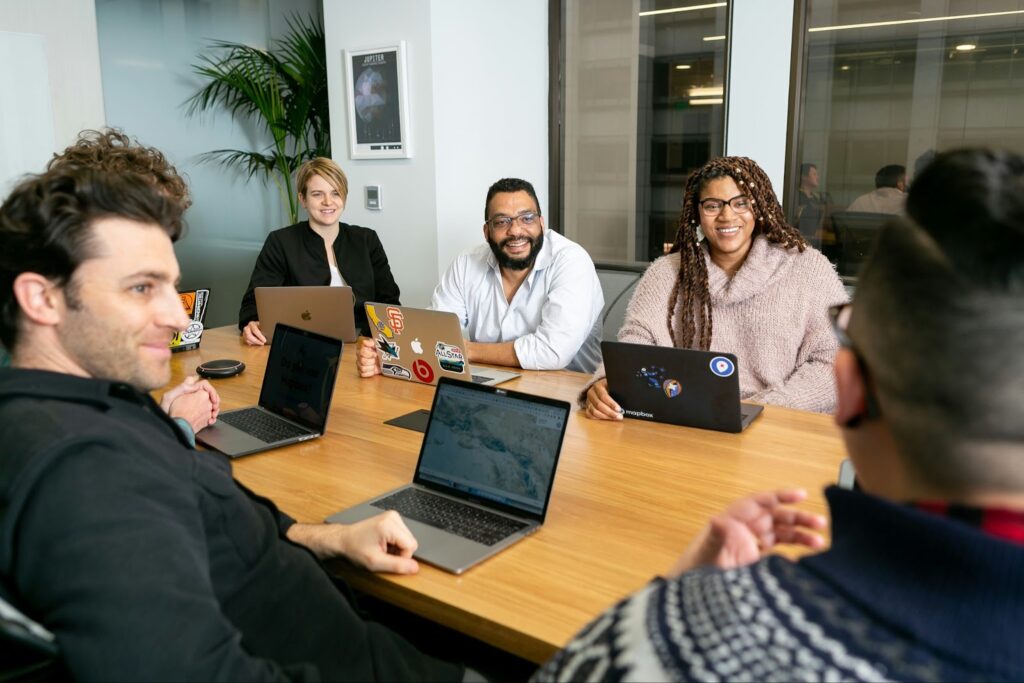
3. Segment your market
Market segmentation can refine your sales forecast by accounting for demand drivers and behavior variations across different segments.
After segmenting your audience or products, you can develop separate forecasts for each category. This allows you to account for demand drivers and behavior variations across different segments.
Here are two ways you might segment your market.
Customer segmentation
Narrow your wider target market into smaller groups based on different characteristics that influence purchasing patterns. This could be:
- Demographics: Age, gender, income
- Psychographics Lifestyle, values, interests
- Geographic location
- Buying behavior
Product segmentation
Categorize your products based on their attributes, such as price, functionality, and lifecycle stage. This helps you tailor your forecasting approach to the different characteristics of each product category.
You might forecast higher-priced products differently from budget items or adjust forecasts based on a product’s lifecycle (e.g., introduction, growth, maturity, decline).
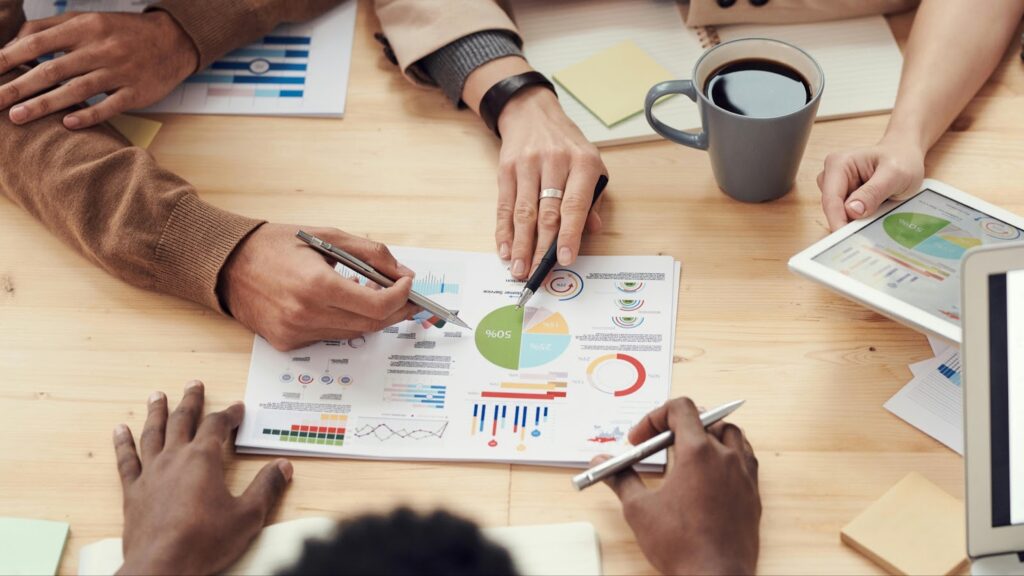
4. Monitor sales performance and make adjustments
Tracking sales performance is crucial to improving the accuracy of your forecast. This allows you to make changes when needed and stay responsive to changing market dynamics.
Keep an eye on key performance indicators (KPIs), sales metrics, and market trends. How do they compare with your forecasted values? If your actual sales drop below what you forecasted, it could be a sign that you need to adjust your strategy or allocate resources differently to adapt to changing market conditions.
Remember that while it’s great to have a demand forecast in place, there are some things you can’t predict. So, it’s essential to remain agile in response to new information or unexpected events that may impact demand.
It’s likely that you will need to revise forecasts, reallocate resources, or adjust marketing strategies along the way. For example, a competitor launching a similar product could unexpectedly reduce your demand, meaning you need to consider scaling back production.
As part of this step, establish a feedback mechanism. Gather regular insights from frontline employees, customers, and partners about their experiences and perceptions. Their feedback can provide valuable information about changing customer preferences, market trends, or other factors that may influence demand.
5. Review and repeat
After making forecasts, it’s essential to compare them with actual sales data to identify discrepancies and understand their root causes. You may need to change your data collection tactics or forecasting methods in the future.
Forecasting isn’t a job you can do at the start of the financial year and forget about. It’s an ongoing process that needs continuous refinement. Regularly review and refine your forecasting models based on stakeholder feedback, performance metrics, and learnings from past forecasting experiences.
Consider building a scenario analysis into your forecasting process, too. This involves preparing for the potential impact of various scenarios or events on your sales forecast. For example, you might consider what would happen if there’s a recession, if you launch a new product, or if a competitor starts a price war.
Imagining these scenarios and their potential effects on sales helps you better prepare and make plans to respond effectively.
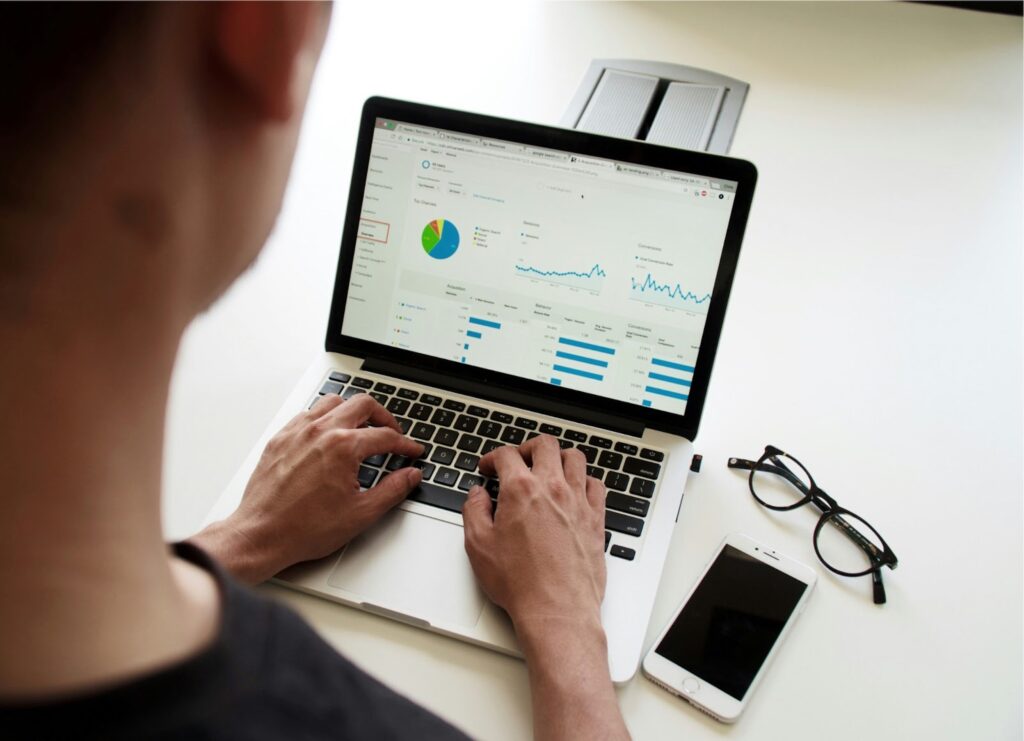
Demand forecasting: Unlock powerful insights to drive sales
While we can’t see into the future, demand forecasting could be as close to a crystal ball as it gets.
Accurate sales forecasts help businesses make more informed decisions and plan strategically. The ability to anticipate future demand means you can make the right adjustments to everything from stock levels and manpower to meet customer needs.
This not only contributes to a more efficient business model but also reduces inefficiencies and reduces costs, leading to a more profitable business and satisfied customers.
Related Blogs
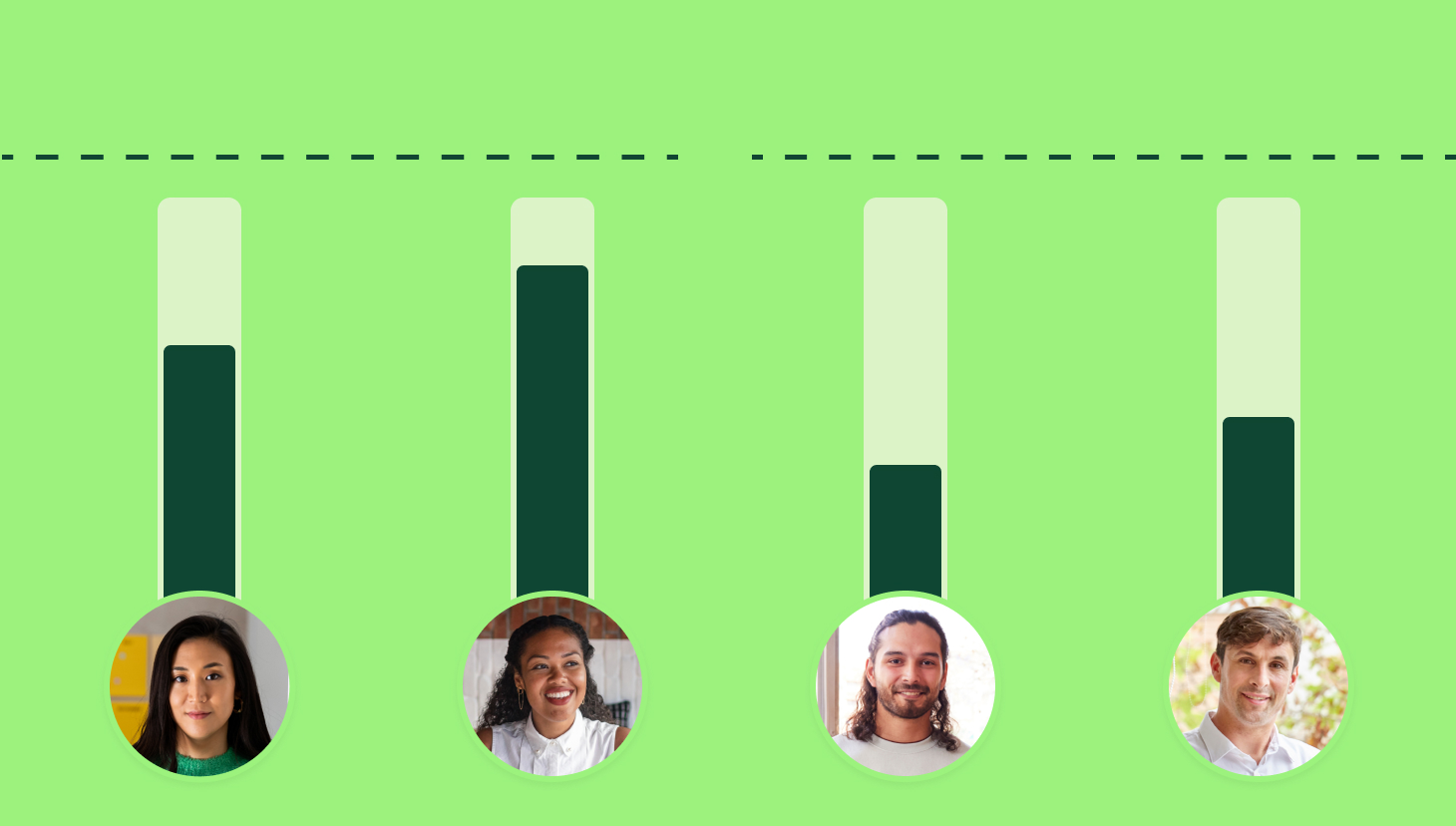
How to Set a Sales Quota
According to our compensation data, 91% of teams missed sales quota last year. Market conditions were the leading reason, along with misaligned sales activity and unrealistic quotas or sales goals....
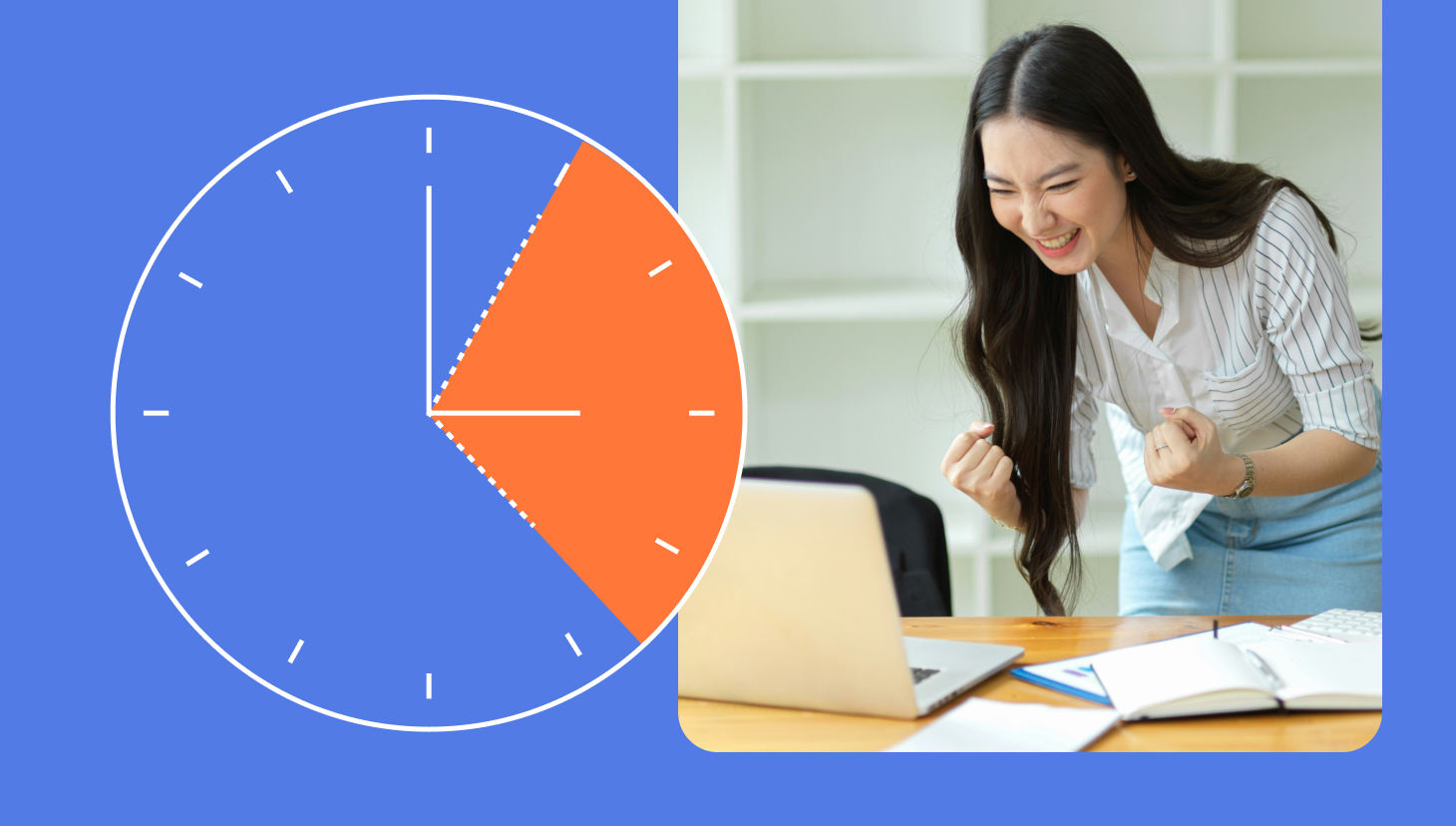
Guide to Spiff Program Management
Spiff program management refers to the design, strategy, and execution of SPIFFs (short for sales performance incentive fund formula) to maximize sales revenue during a set period. It’s a common...
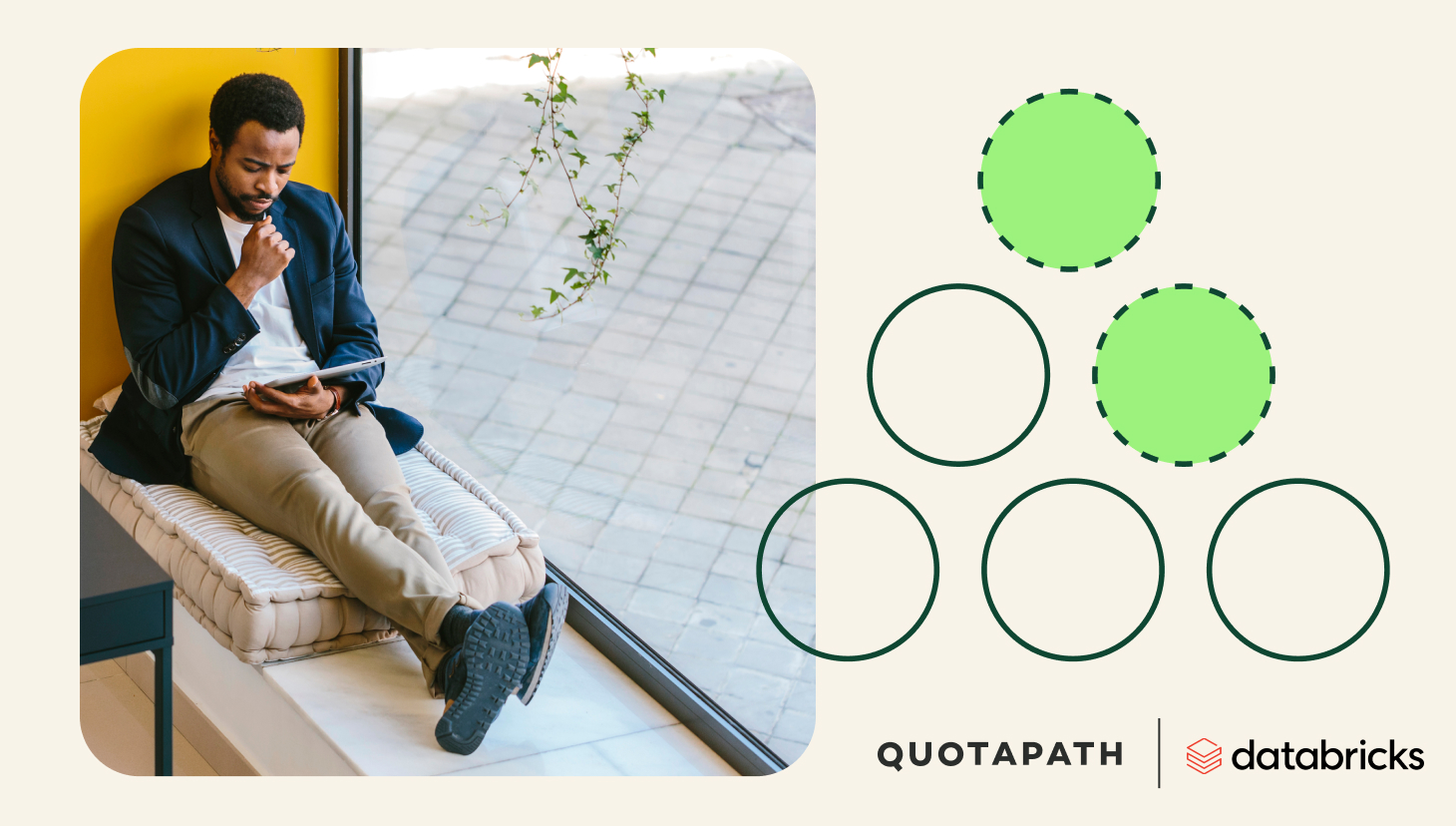
How AI Lead Scoring Optimizes Your Sales Forecasting Strategy
This is a guest blog from the data and AI company, Databricks. Sales forecasting is supposed to make everyone’s lives easier. Sales and marketing have their targets. Customer services and...
Keep up with our content
Subscribe to our newsletter and get fresh insights monthly

Back to blog
Using predictive analytics to forecast business trends.
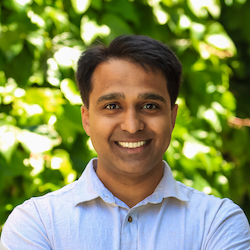
July 30, 2024 | Jimit Mehta
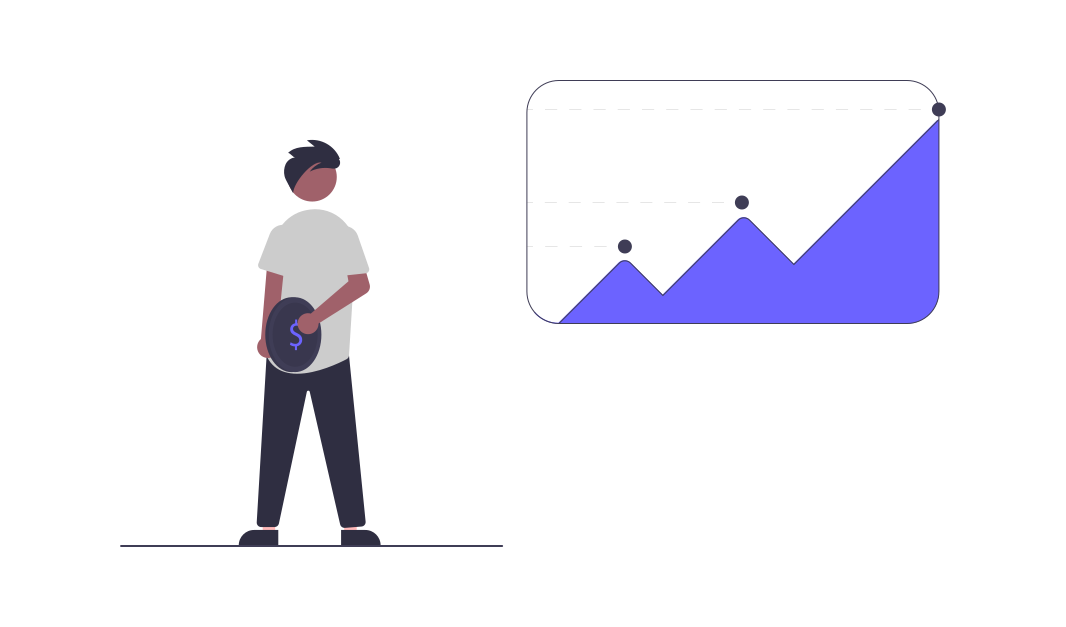
In today's fast-paced and data-driven world, businesses are constantly seeking ways to stay ahead of the competition. Predictive analytics has emerged as a powerful tool that enables companies to forecast business trends with remarkable accuracy. By leveraging advanced algorithms and machine learning techniques, predictive analytics provides insights that can shape strategic decisions, optimize operations, and drive growth.
What is Predictive Analytics?
Predictive analytics involves analyzing current and historical data to make informed predictions about future events. It employs statistical techniques, machine learning algorithms, and data mining to identify patterns and trends. These predictions can encompass a wide range of business areas, including customer behavior, market trends, inventory management, and financial performance.
The Role of Data in Predictive Analytics
The foundation of predictive analytics is data. High-quality, relevant data is essential for generating accurate predictions. Businesses collect data from various sources such as customer transactions, social media interactions, website visits, and more. This data is then cleaned, processed, and analyzed to uncover hidden patterns and correlations.
Key Components of Predictive Analytics
Data Collection and Preparation :
- Gathering data from diverse sources.
- Cleaning and preprocessing the data to ensure accuracy and completeness.
- Transforming data into a suitable format for analysis.
Model Building :
- Selecting appropriate predictive models based on the data and the business problem.
- Training models using historical data to recognize patterns and relationships.
- Validating models to ensure they accurately predict future outcomes.
Model Deployment :
- Integrating predictive models into business processes.
- Continuously monitoring and updating models to maintain accuracy over time.
- Using predictions to inform decision-making and strategy.
Applications of Predictive Analytics
Predictive analytics has a wide array of applications across different industries. Here are a few key areas where it makes a significant impact:
Customer Insights and Personalization : Predictive analytics can help businesses understand customer preferences and behaviors, enabling them to personalize marketing efforts and enhance customer satisfaction.
Demand Forecasting : Businesses can use predictive analytics to forecast product demand, optimize inventory levels, and reduce the risk of overstocking or stockouts.
Financial Planning and Risk Management : Predictive analytics aids in financial forecasting, identifying potential risks, and developing strategies to mitigate them.
Operations Optimization : By predicting equipment failures and maintenance needs, businesses can optimize operations, reduce downtime, and improve overall efficiency.
Benefits of Predictive Analytics
Implementing predictive analytics offers numerous benefits to businesses:
Informed Decision-Making : Predictive analytics provides actionable insights that empower businesses to make data-driven decisions with confidence.
Competitive Advantage : Businesses that leverage predictive analytics gain a competitive edge by anticipating market trends and staying ahead of competitors.
Cost Savings : By optimizing operations and reducing inefficiencies, predictive analytics can lead to significant cost savings.
Improved Customer Experience : Predictive analytics enables businesses to tailor their offerings to meet customer needs, resulting in higher customer satisfaction and loyalty.
Risk Mitigation : Predictive analytics helps identify potential risks and develop proactive strategies to address them, minimizing adverse impacts on the business.
Implementing Predictive Analytics in Your Business
To successfully implement predictive analytics, businesses should follow these steps:
Define Clear Objectives : Identify the specific business problems or opportunities you want to address with predictive analytics.
Gather and Prepare Data : Collect relevant data from various sources and ensure it is clean and ready for analysis.
Choose the Right Tools and Techniques : Select appropriate predictive models and tools that align with your business objectives and data characteristics.
Build and Validate Models : Develop predictive models using historical data, and validate their accuracy and reliability.
Deploy and Monitor Models : Integrate predictive models into your business processes, and continuously monitor their performance to ensure they remain effective over time.
Challenges and Considerations
While predictive analytics offers numerous benefits, it also presents certain challenges:
Data Quality : Ensuring high-quality data is critical for accurate predictions. Poor data quality can lead to unreliable results.
Model Complexity : Building and maintaining predictive models can be complex and require specialized skills and expertise.
Integration : Integrating predictive analytics into existing business processes and systems can be challenging and may require significant effort.
Privacy and Security : Handling sensitive data requires strict adherence to privacy and security regulations to protect customer information.
Future of Predictive Analytics
As technology continues to evolve, the capabilities of predictive analytics are expected to expand further. Advancements in artificial intelligence and machine learning will enhance the accuracy and speed of predictions, making predictive analytics an even more indispensable tool for businesses.
In conclusion, predictive analytics is transforming the way businesses forecast trends and make strategic decisions. By harnessing the power of data and advanced algorithms, companies can anticipate future events, optimize operations, and achieve sustainable growth. Embracing predictive analytics is not just a competitive advantage but a necessity in today's dynamic business environment.
Related posts
Leveraging predictive analytics in industrial marketing for proactive business strategies.
In the competitive world of industrial marketing, the ability to anticipate market trends and customer needs can significantly impact a company’s success. Predictive analytics offers businesses the power to transform raw data into actionable insights, enabling proactive decision-making. This blog...
Leveraging Predictive Analytics to Enhance Omnichannel Marketing Strategies
In the fast-evolving world of digital marketing, staying ahead of customer needs is crucial. Predictive analytics offers a powerful way to anticipate behaviors, refine strategies, and enhance engagement across all channels. This blog explores the fundamentals of predictive analytics and how it can...

Published: 23 July 2024 Contributor: Tim Mucci
Data-driven decision-making (DDDM) is an approach that emphasizes using data and analysis instead of intuition to inform business decisions. It involves leveraging data sources such as customer feedback, market trends and financial data to guide the decision-making process. By collecting, analyzing and interpreting data, organizations can make better decisions that more closely align with business goals and objectives.
Humanity generates over 402.74 million terabytes of data daily 1 (link resides outside ibm.com). This abundance of data, if collected and processed, enables businesses to make effective decisions that serve business goals and provide good customer experiences. Data-driven decision-making allows businesses to generate real-time insights and predictions, optimize performance and test new strategies. Such informed decisions lead to sustainable growth and profitability, whereas relying on gut feelings can result in the opposite. Data provides a solid foundation for making decisions, reducing uncertainty and increasing confidence.
Discover how to harness the power of analytics and business intelligence to enhance business planning and forecasting.
Organizations that adopt a data-driven culture see benefits such as improved customer satisfaction, better strategic planning and more.
A global online retailer uses customer data extensively to build targeted marketing campaigns and enhance its recommendation engine. Analyzing this data allows the organization to create personalized shopping experiences and highly targeted marketing campaigns.
In addition to personalizing product recommendations, the organization uses customer data for dynamic pricing strategies. By monitoring competitor prices, market trends and customer demand in real time, the organization adjusts its prices to remain competitive and optimize revenue.
A popular online streaming service uses data to personalize recommendations and reduce customer churn. The platform uses vast amounts of customer data, including viewing history, ratings and even the amount of time spent watching specific content, to tailor its recommendations. This personalization is driven by sophisticated algorithms that analyze user behavior to suggest content that aligns with individual preferences.
The organization employs several strategies to further retain customers and reduce churn. One of the most effective methods is its recommendation algorithm, which continually presents users with content that matches their tastes. This algorithm both recommends what to watch next and also customizes the visual presentation of titles to appeal specifically to different viewers. By keeping users engaged with content they are likely to enjoy, they minimize the risk of subscribers canceling their service.
Predictive analytics allow businesses to anticipate trends or challenges and take preemptive actions. Financial institutions use advanced machine learning (ML) algorithms to detect and prevent fraud. A proactive approach to fighting fraud protects customers from financial loss and enhances trust in the organization.
Utility companies employ machine learning and data analytics to predict energy consumption patterns accurately. Analyzing large volumes of real-time data involves developing predictive analytics that consider factors such as the time of day, the day of the week and historical energy loads. Organizations can use the same process in manufacturing and supply chain for real-time, on-demand forecasting.
Data insight helps formulate realistic strategic plans. A global coffee brand optimizes its site selection strategy by using geographic information system (GIS) technology. This technology allows it to analyze local demographics, traffic patterns and other relevant data—this precision site selection strategy results in improved performance and higher sales for new stores.
E-commerce retailers who analyze and understand market dynamics and customer preferences can identify untapped customer segments and develop innovative products and services to identify new markets, customer segments and product opportunities.
The iterative data-driven decision-making approach enables businesses to refine their strategies and remain competitive in a rapidly changing environment. A major streaming video service uses data analytics to inform aspects of its content creation and market expansion process.
A multinational retailer uses data to manage its inventory, particularly in preparation for natural disasters. By analyzing historical sales data, the organization discovered that certain products had a significant sales spike before hurricanes. This insight, derived from mining trillions of bytes of sales data, allowed leaders to stock these items heavily in anticipation of storms, to meet higher customer demand.
Additionally, the retailer employs predictive analytics to forecast demand for various products based on historical data, weather patterns and other external factors. So, the company can dynamically adjust its inventory levels to make sure essential items are available when customers need them. Their use of big data and analytics extends to optimizing supply chain operations, where real-time data helps the business manage inventory levels across its stores and distribution centers efficiently.
Data-driven decisions minimize personal bias and safeguard objectivity. A US-based energy company implemented various debiasing techniques as part of its decision-making process. The organization established programs to raise awareness of cognitive biases among its leadership and employees. These strategies protect different perspectives and certify that decisions are not influenced by hierarchical or confirmation biases.
Every organization can benefit from taking six steps to integrate data-based decisions throughout the company. By adopting these best practices, strategies derived from data analysis can be implemented and their impact measured.
- Define objectives: This step involves clearly articulating the organization's goals. After objectives are defined, the company can make a focused and purposeful effort to meet them.
- Identify, prepare and collect data: In this step, the organization sets clear objectives, determines data needs, evaluates and prepares data sources and then systematically gathers and validates the data.
- Organize and explore: Here, the data is structured to uncover new patterns, trends and valuable insights. Cleaning data protects its accuracy and reliability. Visualizing the data helps identify patterns, outliers and trends that are not immediately obvious from raw data.
- Perform data analysis: This step transforms raw data into actionable insights by using various techniques and methodologies to uncover patterns, correlations and trends that inform business strategy. By performing data analysis, organizations can drive strategic decisions and improve overall performance.
- Draw conclusions: Here, key data analysis findings are reviewed and placed in the correct business context to form actionable insights and recommendations. Practical conclusions lead to meaningful actions that drive business success.
- Implement and evaluate: This final step is crucial for validating insights and measuring outcomes. Action plans are developed based on data-driven recommendations, resources are allocated and the initiative's progress is continuously monitored. Outcomes are measured against predefined key performance indicators (KPIs), results are analyzed, feedback is gathered and based on that information, adjustments are made in a continuous cycle of monitoring and iterative improvement. This step provides a basis for making informed adjustments to strategies and plans based on empirical evidence and feedback.
When implementing data-driven decision-making, several challenges arise that organizations need to navigate effectively. One significant issue is neglecting data quality. Poor-quality data can lead to inaccurate analyses and misguided decisions, undermining the value of data-driven strategies.
In many organizations, data resides in disparate systems and formats across departments, making it difficult to consolidate and analyze it comprehensively. Implementing data integration solutions is essential to overcoming this hurdle and enabling holistic decision-making.
Data illiteracy is often another critical challenge. Employees may lack the necessary skills to interpret and use data effectively, leading to misinterpretations and sub optimal decisions. Providing ongoing training and fostering a data-literate culture is vital for maximizing the benefits of data-driven approaches.
Overreliance on historical data can also be problematic. While past data is valuable, it might not accurately predict future trends, especially in rapidly changing environments. Balancing historical data with real-time analytics and forward-looking indicators is essential for relevant and timely decision-making.
Confirmation bias can skew data analysis. Decision-makers might selectively interpret data to support preconceived notions, leading to biased outcomes. Encouraging objective analysis and critical thinking can help mitigate this bias. Also, poor communication of insights is a similar challenge; even the most accurate data can be ineffective if not communicated clearly and compellingly to stakeholders.
Neglecting data security poses risks. Protecting data from breaches and certifying compliance with data privacy regulations is paramount to maintain trust and avoid legal repercussions.
Businesses can benefit from understanding the types of business analytics that can inform their decision-making processes.
Descriptive analysis aims to describe and summarize historical data through data aggregation and mining, providing insights into past performance. This type of analysis is used to create monthly sales reports, customer satisfaction surveys and website traffic analyses.
Diagnostic analysis focuses on determining why certain events occurred. It involves data discovery, mining and identifying correlations to uncover the root causes of trends or incidents, such as a drop in sales or a surge in customer complaints.
Predictive analysis forecasts future trends or outcomes based on historical data. Businesses can more effectively predict sales and customer behavior and manage risks by using statistical models, machine learning and forecasting techniques.
Prescriptive analysis goes a step further by recommending actions based on data. This type combines predictive analytics with optimization algorithms to suggest the best course of action, aiding in supply chain optimization, marketing campaign strategies and resource allocation decisions.
Exploratory analysis is used to discover patterns, relationships or anomalies in data without specific hypotheses. Data visualization , clustering and dimensionality reduction techniques help identify new market segments, explore customer demographics and uncover unexpected correlations.
Inferential analysis uses a data sample to make inferences about a population. It employs statistical tests such as regression analysis, hypothesis testing and confidence intervals and is useful in market research studies, product testing and consumer preference surveys.
Qualitative analysis focuses on non-numeric data to understand concepts, opinions or experiences. Methods such as content analysis, thematic analysis and text mining are employed to analyze customer feedback and social media sentiment and conduct market research interviews.
Quantitative analysis analyzes numeric data to quantify variables and uncover patterns using statistical analysis, mathematical modeling and computational techniques. This type of analysis is essential for financial modeling, operational metrics analysis and performance measurement.
Real-time analysis involves analyzing data as it is generated, providing immediate insights. Using streaming analytics, real-time dashboards and event processing, businesses can detect fraud, manage inventory in real-time and monitor live customer support.
For organizations looking to invest in data-driven decision-making tools, several advanced technologies and platforms are available to help collect, analyze and use data effectively.
Business intelligence tools
BI tools provide data visualization capabilities that allow users to create interactive and shareable dashboards. They connect to multiple data sources and are widely used for data analysis.
Data warehousing solutions
Cloud-based data warehouses offer scalability and flexibility and support various data integration and analytics tools.
Machine learning and AI platforms
These platforms provide a comprehensive suite of machine learning tools and services, such as AutoML, which allows users to build customized ML models without extensive coding experience. These platforms often offer cloud-based training environments and integrate with various analytics services.
Data integration and ETL (extract, transform, load) tools
These tools help organizations manage and transform data from various sources into a unified format. They often support data governance and cloud data management for complex integration needs.
Data analytics and visualization
These tools offer data visualizations and support associative data indexing so users can uncover hidden insights. They can also connect directly to databases to allow for real-time data analysis and reporting.
Big data processing frameworks
There are many open-source big data processing frameworks. Organizations that need to use large amounts of data need to consider a platform that supports batch and stream processing for speed and the ability to handle large-scale analytics.
Data governance tools
Governance platforms help organizations manage data quality, lineage and compliance. They provide tools for data stewardship and metadata management.
In a data-driven organization, several key data science roles are essential for using data effectively and creating a data-driven culture. In addition to data analysts, data managers and data scientists, there are other important roles that guide an organization toward success in its data-driven decision-making initiatives.
Data engineers design, build and maintain the infrastructure and systems required for data collection, storage and processing. Data engineers safeguard data pipelines so they are efficient, scalable and reliable, enabling smooth data flow from various sources to analytical platforms.
Data architects are responsible for designing and implementing an organization's overall data architecture. They create blueprints for data management systems, so data is organized, integrated and accessible.
Business intelligence (BI) developers create and manage BI solutions, such as dashboards and reporting systems. They transform raw data into meaningful insights through visualization tools, to help stakeholders make informed decisions.
Machine learning engineers are specialists who build, deploy and maintain machine learning models. They work closely with data scientists to implement algorithms that can predict outcomes and automate decision-making processes.
Chief data officer (CDO) is an executive role that oversees an organization's data strategy and governance. They ensure that data initiatives align with business objectives, compliance standards and best practices.
Chief artificial intelligence officer ( CAIO ) is an executive role that guides the organization through the complexities of AI adoption. They provide strategic leadership and oversee the development, strategy and implementation of AI technologies.
Data analysts are professionals who analyze and interpret complex datasets to provide actionable insights. They use statistical methods and tools to identify trends, patterns and correlations.
Database administrators (DBAs) manage and maintain database systems. They protect data and confirm it is stored securely, backed up regularly and retrievable efficiently. DBAs also optimize database performance and resolve any data-related issues.
Data privacy officers are responsible for ensuring that data handling practices comply with privacy laws and regulations. They develop policies and practices to protect sensitive information and maintain customer trust.
AI/ML operations ( MLOps ) engineers manage the deployment, monitoring and maintenance of machine learning models in production environments. MLOps engineers guarantee that models operate efficiently and are updated as needed.
Learn how IBM® Cognos® Analytics uses AI automation to enhance data visualization, prediction and reporting. The platform helps users uncover hidden trends, create interactive dashboards and integrate advanced analytics into daily operations.
See how IBM Planning Analytics uses AI forecasting and real-time data analysis to enhance business planning and decision-making. Planning Analytics offers tools for scenario planning, data integration and collaboration across departments, making it suitable for finance, supply chain, HR and IT management.
Explore how IBM watsonx™ BI Assistant provides AI-powered business analysis and advice, offering clear explanations for confident decision-making. Watsonx BI Assistant enables users to uncover trends, predict outcomes and take action based on personalized metrics and intelligent alerts.
Integrating AI-powered tools helps improve financial targets, operational capacity and sales goals, while eliminating data silos and empowering users to make informed decisions.
Learn how IBM Consulting® works with ESPN to enhance the fantasy football experience using AI-insights and data analysis.
Planning, budgeting and forecasting solutions integrate AI and data analytics to enhance financial planning processes.
IBM Cognos Analytics is a self-service business intelligence and data visualization tool. The platform integrates cognitive computing technology, including artificial intelligence and machine learning, so organizations can visualize data, share new insights, and encourage data-driven decision-making.
1 https://explodingtopics.com/blog/data-generated-per-day#how-much (link resides outside ibm.com)
- Planning under uncertainty: Statistical vs. probabilistic approaches and what each offers to your business
- Kinaxis Blog
Stefan de kok, CEO of Wahupa and Masoud Chitsaz, Technical Thought Leader at Kinaxis
Uncertainty exists! What matters the most is how we make decisions.
Many businesses use advanced probability theory in action and these days it has become an indispensable part of their operations. Examples can be found in the applications of forecasting, risk management, and decision analysis. No surprise, there is no simple solution to these business challenges.
However, we can improve the quality of our business decisions by embracing uncertainty and applying the science of probability in the decision-making process. The intent of applying probability concepts in business operations is to get better knowledge about whether and to what extent events, actions, and outcomes are correlated. These concepts play a key role to determine the risk level of business actions in the face of uncertainties which helps decision-making to be data-driven.
The frameworks for making decisions under uncertainty can be of statistical or probabilistic type. Each of these frameworks can return deterministic or stochastic solutions that include confidence ranges for the decisions. Each type of framework and resulting solution has its use cases and benefits. Depending on the type of business decision, its criticality, and the availability of data, we can choose the proper type of framework and resulting solution category to employ.
A deeper understanding of each category and possible combinations will help to choose the right one for the business.
Deterministic vs. stochastic results
Stochastic results, on the other hand, depend on the probability distributions instead of exact numbers for any value representing anything uncertain in the future (Figure 1).
This could include quantities, lead times, production rates, yields, and so forth. Stochastic output is not guaranteed to be identical if the same calculation is executed multiple times, but only up to a given precision.
Statistical vs. probabilistic calculations
Obtaining the knowledge about the pattern of change and variation in various involved parameters is the first step when uncertainty is involved in controlling a system or making decisions for business operations. To do so, we can either use statistical calculations and use standard or well-known distributions or we can employ probabilistic methods and make little to no assumptions about the pattern of the distribution.
In the first case, we need to make strict a priori assumptions about the pattern of the underlying data. The two most common assumptions are that the data is exact or that it is normally (Gaussian) distributed. An example of exactness is a demand forecast expressed in simple numbers: next month we will sell 100 units of product A. An example of assuming a normal distribution can be found in all commonly used safety stock formulas, where it is implicit in both the service factor and the use of standard deviations on both demand and lead time. Both these cases, allow simple calculations, which explains their popularity.
The second case, probabilistic methods, comes in two main variants: parametric and non-parametric. Both have upsides and downsides. Parametric probabilistic methods are highly scalable and need little more data than statistical methods. They differ from the latter, mainly in that the parametric distribution first needs to be determined. And depending on which distribution is determined, parameters may be much harder to estimate accurately. An extreme example is to apply a Tweedie distribution, which can mimic almost any actual distribution, but is near impossible to fit accurately.
Estimating a distribution nonparametrically requires a large amount of data, especially continuous distributions. The question is how many data points do we need to properly describe an empirical distribution? Well, it really depends on the importance of the business problem and the level of precision needed in modeling the system. To get a better understanding, let’s look at a numerical example within the following chart.
If we generate random samples for a fictitious demand with a mean of 100 and standard deviation of 25, how many samples does it take before the empirical distribution (orange bars) starts to match the true distribution (blue line)? The three charts on the left have 10, 50 and 100 samples from the actual demand quantities. The lack of approximation even with 100 samples is evident. The three charts on the right are obtained by rounding the actuals to multiples of 10 (quantity bins). We can observe some convergence with 100 samples. As a rule of thumb, we need at least about 5 times the number of samples as quantity bins before empirical distributions get adequately close to true distributions to be representative.
Fitting a parametric distribution to the data, and using it in a closed form method, will not face this issue because such method can be equivalent to calculating the distribution parameters by minimizing a loss function. The cost to pay, when using fitting a parametric distribution is that the accuracy is limited by the choice of distribution.
The process of obtaining both deterministic and stochastic results can be either statistical or probabilistic.
The process of preparing the input data as well as the inner calculations in a model can follow statistical or probabilistic approaches. Interestingly, the outcomes of such analysis can be either deterministic or stochastic depending on the business decision requirements. Let’s explore several examples for the possible combinations.
Statistical calculations for a deterministic result
MRP (material requirements planning) is a method to plan production and procurement orders considering demand, bill of materials and production lead times. This is all straightforward to calculate if the independent demand (for final product) and the lead times in all levels of supply are assumed to be deterministic. But production systems must deal with uncertainty, and statistical calculations can then help by capturing the uncertainty through calculated buffers for demand and lead times in the system.
Statistical forecasting is the process of fitting a curve to a set of historical data. The curve is usually chosen based on the statistical assumption that forecast residuals are independent and identically, normally distributed around zero to be unbiased. The output is a time-series of exact numbers.
Probabilistic calculations for a deterministic result
- In Probabilistic Multi-Echelon Inventory Optimization, we consider the probability distributions of the lead times and demands to optimize the overall level of inventory at different levels of supply and distribution system. The output, reorder levels or safety stock targets, are exact numbers.
- Fair Share Allocation of available inventory to customer orders when the availability is less than required. The objective is to provide each customer the same probability of stock out instead of targeting the same fill rate for different customers. The output are exact allocation quantities.
Statistical calculations for a stochastic result
- Monte Carlo Risk Assessment and Monte Carlo S&OP Scenarios return the possible behaviors based on the changes in the input parameters. The Monte Carlo simulation will repeatedly generate random but exact numbers for different scenarios following an assumed probability distribution of those problem parameters. The calculations are traditional deterministic, but the results can again be presented as empirical probability distributions where each iteration of the simulation provides one observation.
Probabilistic calculations for a stochastic result
- A quantile is the value below which a fraction of observations in a group fall. For example, a 0.75 quantile forecast should over-predict 75% of the times. Quantile forecasts are often provided for multiple quantiles simultaneously, such as 10 quantiles in the M5 forecasting competition. In such cases the output is stochastic.
- Probabilistic forecast fits distributions instead of curves. To envision this, imagine a stationary demand pattern, one where every time period has the same distribution of possible quantities. One could create a histogram for the frequency (y axis) of distinct demand quantities (x axis) similar to Figure 2 above. This histogram then displays the density of the empirical demand distribution. A proper probabilistic forecast will fit distributions even under the much more complicated situation where demand patters are not stationary. More details on probabilistic forecasting can be found in this article by Stefan De Kok .
Example in inventory control
Suppose a retail store wants to determine the optimal reorder point for a specific product to ensure adequate stock levels. The store has historical sales data for this product, but they do not know how it is distributed around the long-term patterns.
In a statistical approach, the store would assume a Gaussian (normal) distribution for the demand based on industry norms or general assumptions. Let's say the store assumes a normal distribution with a mean of 100 units and a standard deviation of 20 units for the product's demand over lead time. Using this assumption, the store calculates the reorder point based on a desired service level, such as a 95% probability of not experiencing a stockout. With the normal distribution assumption, the store might determine a reorder point of 125 units, corresponding to the mean demand plus a certain number of standard deviations to ensure sufficient inventory.
On the other hand, a probabilistic approach considers the actual data and can extract either an empirical or a parametric distribution directly from it, rather than assume one. Since the store is only learning about probabilistic methods, they choose the easier empirical approach. The store analyzes the historical sales data and constructs an empirical distribution based on the observed demand levels. It could be a histogram or a kernel density estimation, capturing the unique characteristics of the demand pattern without assuming a specific distribution shape. Using the empirical distribution, the store calculates the optimal reorder point for the desired service level. Let's say the analysis suggests a reorder point of 130 units based on the historical demand patterns.
This example highlights the distinction between statistical and probabilistic approaches in inventory control and ordering lies in the assumptions made about the distribution of demand data. While statistical approaches rely on predefined assumptions such as the normal distribution, probabilistic approaches extract the distribution directly from the data, accommodating the unique demand patterns without imposing specific assumptions. By utilizing an accurate distribution derived from the data, inventory control decisions can be enhanced in terms of accuracy and effectiveness, resulting in optimized stock levels and cost reduction.
Employing the science of probability drives better business decisions
In business decision-making, incorporating uncertainty is crucial. Different methods have been developed to handle decision-making under uncertainty, aiming to extract valuable information from data and utilize it for improved decision-making. These methods can be categorized into statistical and probabilistic frameworks.
One important distinction between these frameworks is that statistical methods rely on assumptions about the presence of specific distributions and patterns in the data. On the other hand, probabilistic methods impose minimal assumptions regarding the pattern of distribution in the data. Consequently, purpose-built probabilistic models tend to offer greater accuracy and enable better-informed business decisions.
The amount of available and high-quality data is the major criteria to choose between empirical and closed-form methods. But probabilistic methods, empirical or closed form, require no more data than their statistical equivalents, only maybe more granular. Statistical engines may allow modeling supply chains, stock markets, and airline flight control, but will be outclassed by probabilistic models in their area of focus. One last key takeaway is that the process of obtaining deterministic or stochastic results can be either statistical or probabilistic as depicted in various examples.
One of the most important applications of probabilistic models in supply chain planning is on maximizing service levels and lowering the inventory costs through probabilistic multi-echelon inventory optimization (MEIO). You can check out the Kinaxis MEIO that is integrated in RapidResponse. It optimizes the inventory across product mix, inventory tiers, and production stages, while considering physical and monetary constraints.
“First, the only certainty is that there is no certainty. Second, every decision as a consequence is a matter of weighing probabilities. Third, despite uncertainty we must decide and we must act. And lastly, we need to judge decisions not only on the results, but how those decisions were made.” - Robert Rubin
Leave a Reply
Get blog updates.
Stay up to date with blog posts by email:
© Kinaxis Inc. 2024. All Rights Reserved.
More From Forbes
Going from founder-led sales to a predictable demand generation engine.
- Share to Facebook
- Share to Twitter
- Share to Linkedin
Duane Tursi, CEO, Ascension Group International .
Transitioning a business from the startup phase to a more mature, scalable operation is filled with challenges. For entrepreneurs aiming to advance their business, mastering the art of sales is crucial.
In my time, I've found that this process can be broken down into two key components: Building a repeatable and predictable demand generation engine and transitioning from founder-led sales to leadership-led sales.
The first step involves thoroughly understanding your market and identifying your ideal customer profile. Sure, that may sound like a no-brainer, but you'd be surprised how many people speed through this step, neglecting customer interviews and thorough market research.
Before really digging in, it's important to roll up your sleeves and analyze your existing customer base, study market trends and leverage data to pinpoint the characteristics of your most profitable and satisfied customers. Once that's done, heed these key steps to building success in sales.
Elon Musk’s Experimental School In Texas Is Now Looking For Students
Nyt ‘strands’ hints, spangram and answers for thursday, august 1st, the top 10 richest people in the world (august 2024), 1. develop a fully integrated sales and marketing strategy..
While your sales team plays a pivotal role in customer acquisition and customer relationship management, don’t overlook your marketing efforts. Your marketing strategy should be tactical and speak to your target audiences. After all, 96% of prospects do their own research before speaking with a human sales rep, per Hubspot’s " 2024 State of Sales Report " (download required).
This means they’re reading user reviews, visiting your website, reading your blog and scoping out your social media channels. Make sure you’re delivering content where your audience is most likely to look for it.
2. Start with the right content.
Depending on your business's size and budget, a marketing strategy may involve various channels. Understanding where to focus is key to meeting your audience where they are. Focus on high-quality content to build awareness and provide tools for your sales team. In fact, 74% of companies say that content marketing increases lead generation.
Here are some critical places to start:
• Content marketing: Your content program should include several different types such as blogs, ebooks, videos and webinars (the medium will vary depending on your market, goals and budget). These can all serve as tools for your sales team.
• SEO: Invest in search engine optimization (SEO) to ensure that people can easily find your content. SEO evolves on a regular basis, so it's important to follow the latest best practices. Get tactical and ensure you look at all your content channels, not just your company’s website.
• Social media: According to a Forbes writeup , "Social media and community-building efforts are where businesses spend most of their content marketing budget." Use these platforms to show your company's human side; interact with your audience, share content, build trust and foster a vibrant community around your brand.
• Email marketing. Implement targeted email campaigns and leverage this channel to cross-promote content that you've already created for your owned channels.
3. Hire proven sales leaders.
Focus on hiring experienced sales leaders who share your vision and understand your market. These individuals should not just be any sales leaders; they should be the trailblazers in your company who will lead your business into sales leadership.
They should be capable of performing sales development representative (SDR) functions and using platforms like LinkedIn and email to generate new leads and nurture customers throughout the sales process. Be careful not to add too many tools that could create cognitive overload, though; in its recent "State of AI Report," Hubspot found that 45% of sales professionals are overwhelmed by the number of tools in their tech stack.
Your sales leader should also create a repeatable SDR playbook and close deals. Developing these skills takes time, so I recommend seeking candidates with a track record of at least two to three years of successful demand generation and account management.
4. Define a clear sales process and set measurable goals.
Once you have the right sales leaders in place, work together to define a clear and repeatable sales process, which should be documented in your SDR playbook. Outline each step of the sales journey, from lead generation to closing deals. These should include:
• Lead qualification. Establish criteria for qualifying leads to ensure your sales team focuses on high-potential opportunities.
• Sales training. Provide comprehensive training to your sales team on your products, market, and sales techniques.
• CRM implementation. Invest in a customer relationship management (CRM) system to manage customer interactions, track activities, and analyze performance. Use these analytics to measure against your goals.
5. Create accountability while empowering your sales team.
The success of your sales team hinges on their empowerment. Equip them with the tools, resources and autonomy to thrive. Provide access to sales enablement materials, conduct regular training sessions and foster an environment that champions collaboration and innovation.
Establish transparent and fair performance metrics and attractive incentive structures to instill accountability and help drive motivation. Metrics such as sales targets, deal closure rates and customer satisfaction scores can effectively monitor progress and pinpoint areas for enhancement.
Additionally, crafting a compensation plan that acknowledges high performers and aligns with your business objectives ensures a sense of fairness and equity in the system, making the sales team feel connected and committed to your company's goals.
6. Overcome growth pains.
As your business scales, it is important to maintain the consistent quality of your product or service. You can achieve this by implementing quality control systems and providing regular employee training.
While meeting immediate sales targets is key, focusing on long-term strategic goals is equally important. To help with balance, I think sales leaders should be incentivized based on quarterly results and their contribution to the company's strategic objectives.
Final Takeaways
The journey from startup to fully-fledged business is full of challenges. However, with the right sales strategies and leadership in place, I've found that these difficulties can be transformed into opportunities for success.
As startups broaden and become more established, the focus must shift from simply generating demand to building a sustainable and efficient sales system led by experienced professionals.
Forbes Business Council is the foremost growth and networking organization for business owners and leaders. Do I qualify?
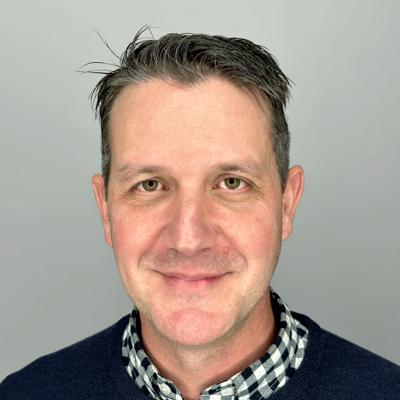
- Editorial Standards
- Reprints & Permissions
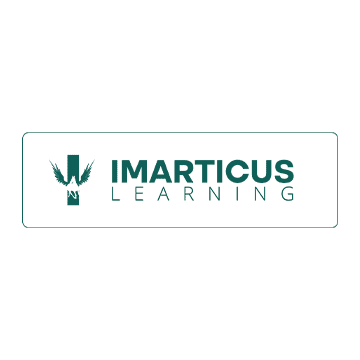
Budgeting and Forecasting: Learn the Key Differences!
- July 29, 2024
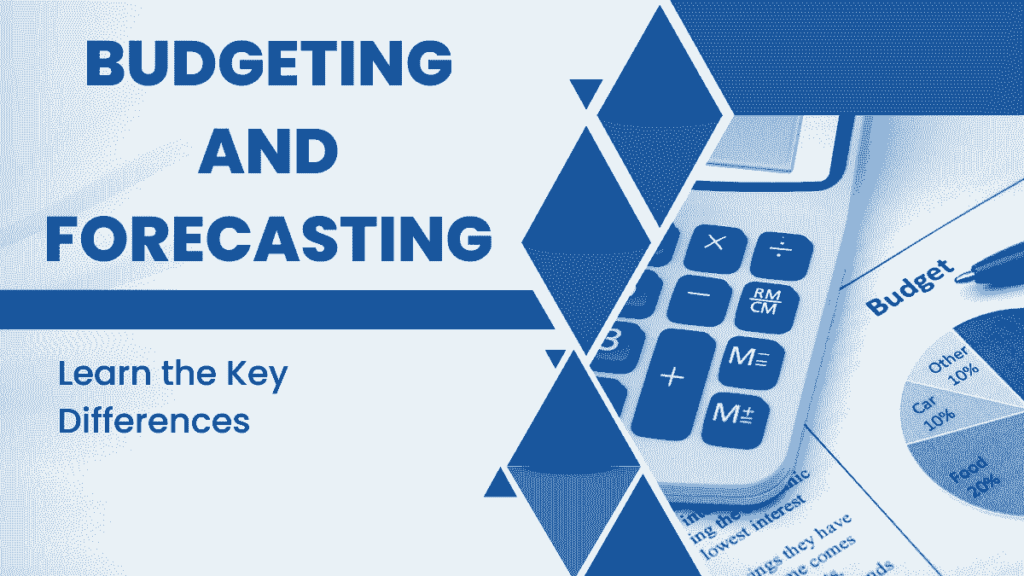
For any business, budgeting and forecasting are crucial for financial planning and analysis. These two activities not only help make decisions and allocate resources, but also ensure an organisation's long-term financial stability. Read on to know more about these concepts, including the difference between budget and forecast .
Understanding Budgeting
Budgeting involves planning and allocating resources for a specific period of time. Also, it includes tasks like setting revenue and expense targets, managing cash flow and determining resource needs.
Forming the basis of any business operations, a budget represents how a company will do business in the year ahead. Further, it dictates decisions associated with investments, cost cutting and where to focus specific efforts.
Understanding Forecasting
Offering an estimate of what the business will achieve if it continues to perform the same way, a forecast helps to quantify and manage the gap between the original budget and the reality. In short, it shows where the company is genuinely headed.
Difference Between Budgeting and Forecasting
After setting up the budget, businesses must reflect on what actually happened versus the budget, every quarter. As everything doesn’t go according to the plan, financial forecasting becomes important to manage the lag. Let’s delve deeper to learn the differences between budgeting and forecasting.
Accuracy | Though based on well-researched assumptions and estimates, there may be significant deviations from actual results | Affected by unpredictable events and market changes |
Basis of Comparison | Helps compare actual performance against planned results | Helps evaluate potential risks and predict future results |
Complexity of Financial Models | May involve less complex financial models | Often utilises models and algorithms to predict future outcomes |
Compliance | Sometimes, it is associated with regulatory requirements and financial reporting standards | Not necessarily involve the same level of compliance |
Continuous Process | Typically done annually or semi-annually | A continuous process that is updated when needed as per changes in market conditions |
Decision Making | A key decision-making tool that helps in allocating resources and prioritising initiatives | Inform and support by offering a view of potential future outcomes |
Dynamic vs Static | A process that is set for a certain period | A process that changes over time |
Emphasis | Controlling costs and managing resources | Anticipating future financial outcomes |
Flexibility | Static and sometimes difficult to change | Can be updated as new information becomes available |
Inputs | Begin with setting financial goals and allocating resources to achieve them | Utilises past financial data and market trends to make predictions |
Input from Other Departments | May require inputs from other departments like marketing and sales | Involves fewer inputs and may be primarily done by finance and data analytics teams |
Iterative Process | May involve an iterative process with many rounds of revisions and updates | Features a more dynamic and ongoing process |
Level of Detail | Usually more detailed | Less granular |
Purpose | Creating a financial plan for a defined period | Predicting future financial outcomes |
Precision | Often detailed and precise | Less precise owing to the uncertainty of future events |
Role of Management | Often requires input and approval from management | May be done by finance teams |
Relevance | Plan and manage money | Know and prepare for future market conditions |
Real-time vs Historical Data | Involves historical data and assumptions | Typically uses real-time data and market trends |
Time Horizon | A long-term planning tool covering a fiscal year or more | Can be done for any period of time, from the next month, quarter, 1 year, etc. |
Use | Guides financial decision making and measures performance against goals | Anticipates future events to adjust plans accordingly |
Notably, budgeting and forecasting are connected but have different purposes. While budgets play a vital role in decision making and resource planning, forecasts are important to manage gaps between the budget and the reality. If you want to make a career in finance, then enrol in a reputable course like ISB Chief Financial Officer Programme . Connect with Imarticus Learning to know more!
Share This Post
Subscribe to our newsletter, get updates and learn from the best, more to explore.
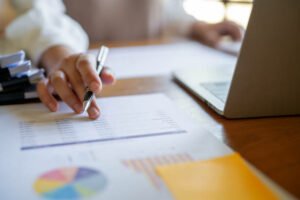
Your Ultimate Guide to Becoming a Chartered Financial Analyst
Your ultimate guide to acca exam dates: stay ahead of the curve, our programs.
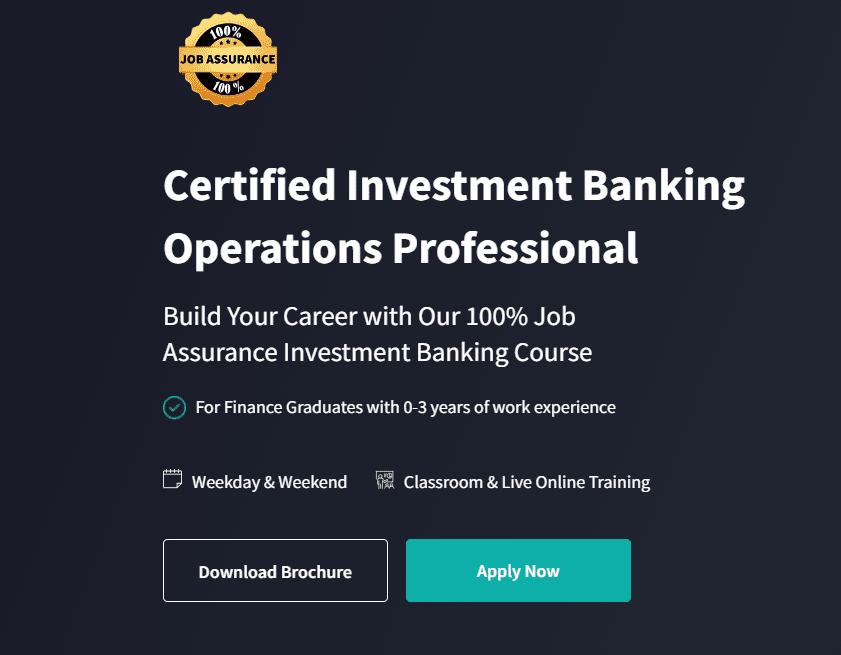
Certified Investment Banking Operations Professional
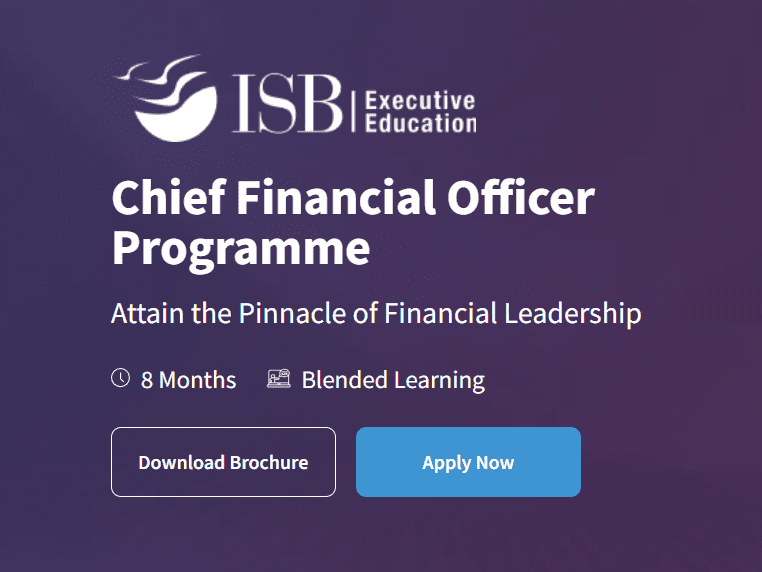
Chief Financial Officer Programme
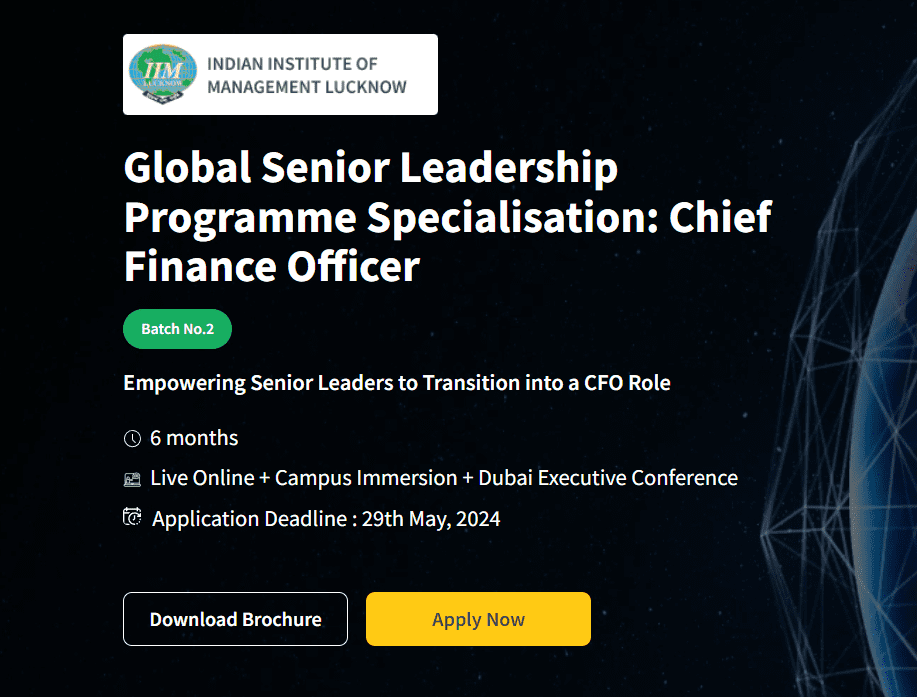
Global Senior Leadership Programme Specialisation: Chief Finance Officer
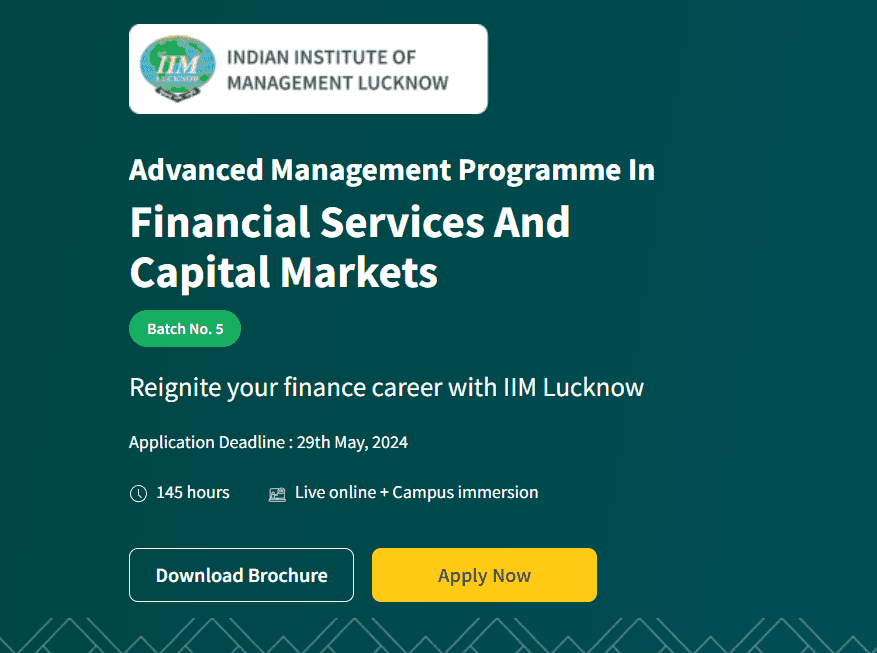
Advanced Management Programme In Financial Services And Capital Markets
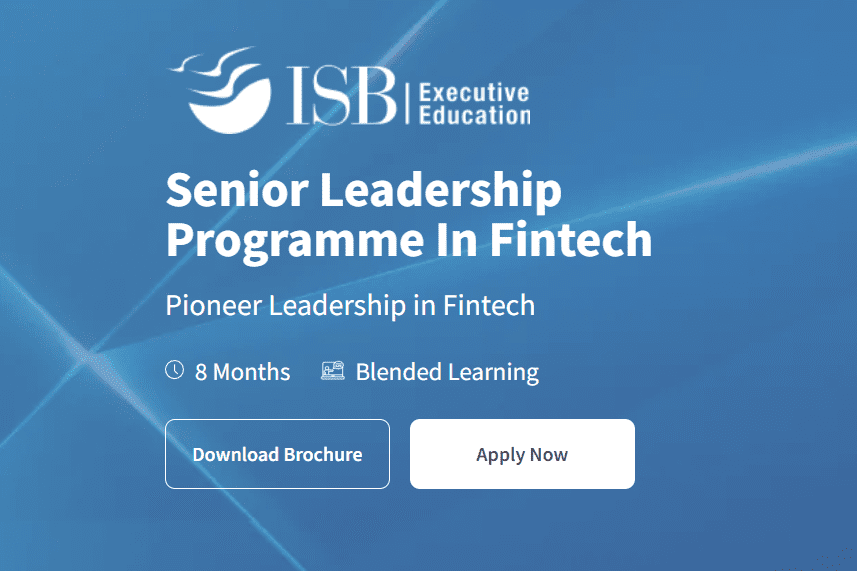
Senior Leadership Programme In Fintech
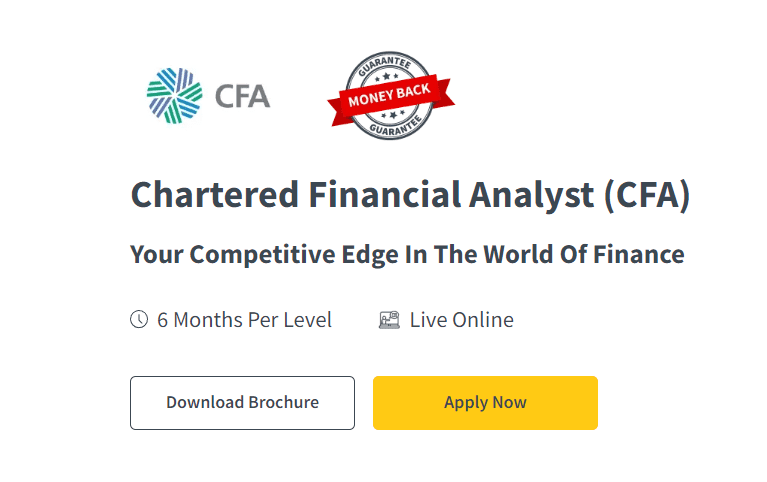
Chartered Financial Analyst (CFA)
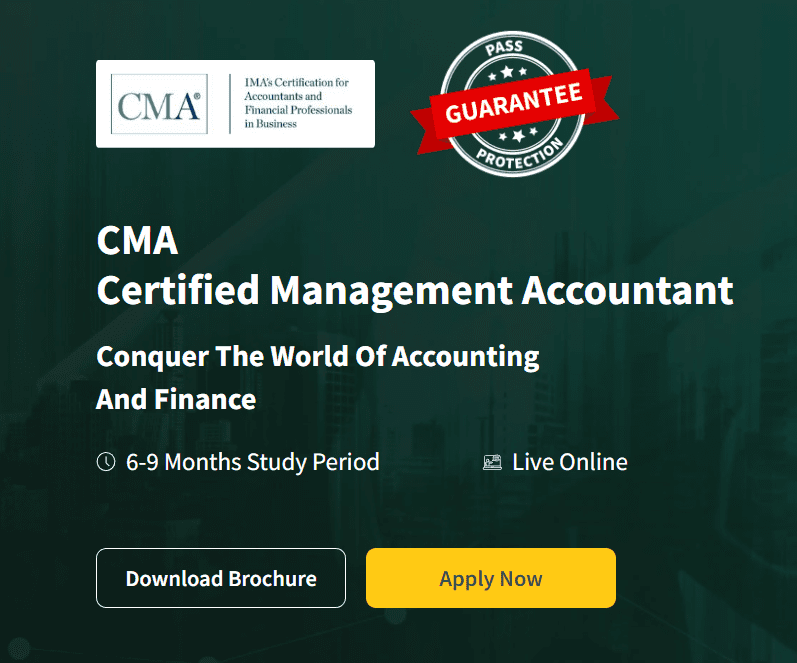
Certified Management Accountant
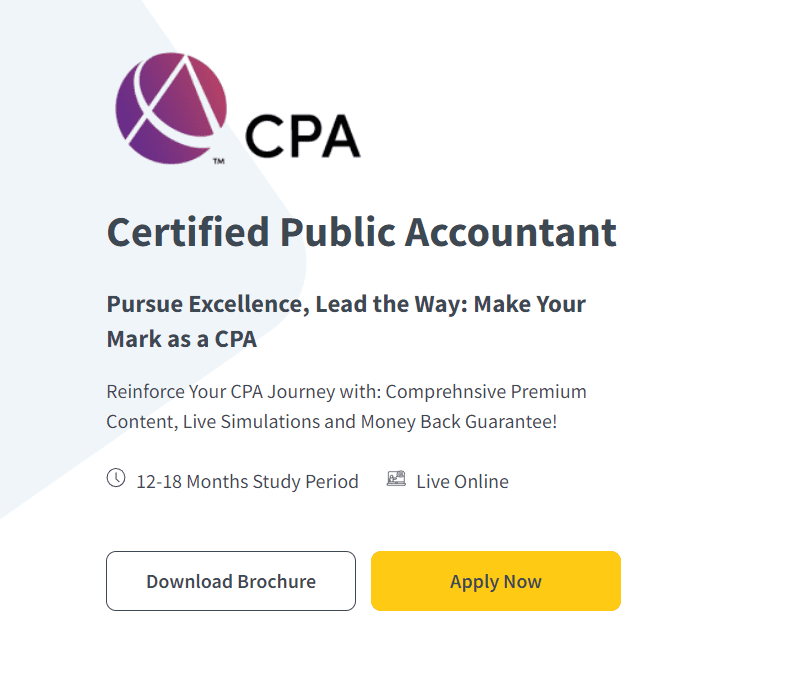
Certified Public Accountant
Do you want to boost your career, drop us a message and keep in touch.
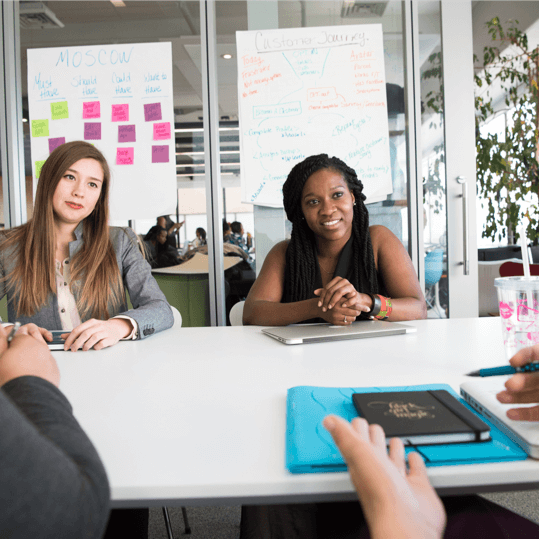
Keep In Touch
- Small Language Model
- Computer Vision
- Federated Learning
- Reinforcement Learning
- Natural Language Processing
- New Releases
- AI Webinars
- 🔥 Promotion/Partnership
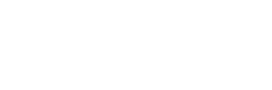
Darts is Python library that aims to be the scikit-learn for time series analysis. By providing a unified and consistent API, Darts simplifies the end-to-end process of working with time series data. It integrates various functionalities—data manipulation, model fitting, forecasting, and backtesting—into a single framework, making it easier for users to switch between models and approaches without dealing with compatibility issues.
At the core of Darts is the TimeSeries data type, designed to represent multivariate and potentially probabilistic time series. This format ensures that time series are well-formed with a proper time index and can handle multiple samples for probabilistic models. Users can easily convert Pandas DataFrames into TimeSeries objects, facilitating seamless integration with existing data workflows.
Darts mimics the scikit-learn model interface, where the fit() method is used for training models and the predict() method for making forecasts. This consistent interface allows users to experiment with different models, from traditional methods like Exponential Smoothing and Auto-ARIMA to advanced neural network-based models like RNNs and Transformers. The library supports both univariate and multivariate time series, and can generate deterministic or probabilistic forecasts.
For example, training an Exponential Smoothing model on a time series of air passenger data involves just a few lines of code. The trained model can then generate forecasts, which can be visualized along with the actual data. Darts also supports backtesting, enabling users to evaluate model performance by simulating real-time forecasting scenarios and comparing historical forecasts with actual outcomes.
Darts offers a wide range of built-in models, including Exponential Smoothing, (V)ARIMA, Facebook Prophet, and various deep learning models like RNNs, TCNs, and Transformers. These models can be easily interchanged and compared, thanks to the unified fit() and predict() interface. Additionally, Darts provides robust support for deep learning, allowing models to be trained on multiple time series and covariates, with the capability to leverage GPUs for large datasets.
The library includes tools for backtesting and model evaluation, such as the historical_forecasts() function, which generates forecasts for specified horizons and timestamps, and calculates error metrics like the Mean Absolute Percentage Error (MAPE). This functionality enables users to fine-tune models and assess their accuracy and reliability over time.
Darts also supports more advanced features like probabilistic filtering, grid search for hyperparameter tuning, and automatic model selection. Its design ensures that TimeSeries objects are immutable, promoting a functional programming style and reducing the risk of unintended side effects.
Darts addresses the inherent complexities of time series analysis by offering a comprehensive, unified framework that simplifies model training, forecasting, and evaluation. By integrating various functionalities into a single, consistent API, Darts enhances the user experience and boosts productivity, making it an invaluable tool for data scientists and analysts working with time series data. The ongoing development and open-source nature of Darts ensure that it will continue to evolve, incorporating new features and improvements driven by community contributions.

Shreya Maji
Shreya Maji is a consulting intern at MarktechPost. She is pursued her B.Tech at the Indian Institute of Technology (IIT), Bhubaneswar. An AI enthusiast, she enjoys staying updated on the latest advancements. Shreya is particularly interested in the real-life applications of cutting-edge technology, especially in the field of data science.
- Optimizing Memory for Large-Scale NLP Models: A Look at MINI-SEQUENCE TRANSFORMER
- Is the Future of Agentic AI Personal? Meet PersonaRAG: A New AI Method that Extends Traditional RAG Frameworks by Incorporating User-Centric Agents into the Retrieval Process
- Theory of Mind Meets LLMs: Hypothetical Minds for Advanced Multi-Agent Tasks
- Transforming Database Access: The LLM-based Text-to-SQL Approach
RELATED ARTICLES MORE FROM AUTHOR
Gemma 2-2b released: a 2.6 billion parameter model offering advanced text generation, on-device deployment, and enhanced safety features, meet torchchat: a flexible framework for accelerating llama 3, 3.1, and other large language models across laptop, desktop, and mobile, how important is the reference model in direct preference optimization dpo an empirical study on optimal kl-divergence constraints and necessity, introducing jcds and jwds: novel approaches for dense subgraph detection in temporal graphs, this ai paper from apple introduces the foundation language models that power apple intelligence features: afm-on-device and afm-server, meta ai introduces meta segment anything model 2 (sam 2): the first unified model for segmenting objects across images and videos, gemma 2-2b released: a 2.6 billion parameter model offering advanced text generation, on-device deployment,..., meet torchchat: a flexible framework for accelerating llama 3, 3.1, and other large language..., how important is the reference model in direct preference optimization dpo an empirical study..., this ai paper from apple introduces the foundation language models that power apple intelligence....
- AI Magazine
- Privacy & TC
- Cookie Policy
- 🐝 Partnership and Promotion
🐝 FREE AI WEBINAR: Learn how to fine-tune Meta's SAM 2 with Your Own Data (8th August 10am PST)
Thank You 🙌
What Is Sales Planning?
Joseph Tsidulko | Content Strategist | July 15, 2024

In This Article
Sales Planning vs Sales Plan
Sales planning explained, why is sales planning important, role of sales planning in the business, elements of successful sales planning, 5 sales planning best practices and tips, sales planning process: how to create a sales plan, improve efficiency and increase revenue with oracle sales planning, sales planning faqs.
Markets remain erratic, supply chain snags linger, inflation remains stubbornly high, and sales activity continues its shift away from conventional channels. With this kind of uncertainty, drawing up a sales plan on a spreadsheet won’t cut it anymore. These cascading challenges have pushed sophisticated sales planning practices, and the technology solutions that support them, to the forefront. Businesses want to formulate and revise their sales plans quickly. They want to set attainable revenue targets and push those targets to a chief revenue officer to assign territories and set quotas for sales reps. They want to track performance at a granular level, as well as adopt compensation models that motivate everyone to execute and succeed.
But achieving those goals takes a lot more than breaking away from the spreadsheets of old. Businesses need to holistically implement time-tested strategies and best practices, make decisions based on data and not just instinct, and deploy cloud-based applications designed to overcome the many stumbling blocks in the sales cycle. Modern sales planning strategies and methodologies help sellers win the trust of customers by nurturing interpersonal relationships. And in a world where more and more deals originate and close online, they must also provide visibility into accounts entirely lacking a human touch.
Sales planning entails developing and documenting a strategy for how the sales organization allocates its limited resources to best achieve revenue targets and broader company objectives. The process yields a sales plan that outlines the steps toward optimizing sales team performance. The steps involve include establishing revenue goals, identifying target markets, assigning sales territories , setting quotas and compensation models for sales reps, evaluating consumer buying trends and market dynamics, and consistently collecting data to evolve the plan over the lifetime of a sales campaign.
Sales planning is a process used to create yields a sales plan that clearly documents sales targets, goals, and strategies over a fixed period. But a sales plan is never carved in stone. Sales planning is an ongoing process where sales leaders continually monitor real-world results, assess evolving market conditions, and create new forecasts based on shifting data and models. And when these results and forecasts stray from initial expectations, planners will amend the existing sales plan accordingly.
Key Takeaways
- Sales planning is the process by which sales professionals develop and document the strategy they think is most likely to achieve targets.
- These prescriptive steps are documented in a sales plan that sets out territory assignments, quotas for regional teams, commissions for sales reps, and the tools the reps should use to achieve the outcomes expected of them.
- The global pandemic—with its travel bans, lockdowns, and social distancing mandates—has drastically disrupted typical sales motions. B2B and B2C sales activity has surged online, and sales teams need to adjust to that enduring reality.
- Sales planners have traditionally used cumbersome spreadsheets to document their sales execution plans. But cutting-edge tools, including those with built-in AI, can provide sales leaders with greater visibility into sales performance so they can model scenarios and stay on track to achieve their targets. Such applications also make it easier to adjust projections and quotas based on frequently shifting goals and risks; they also deliver more reliable forecasts.
Sales planning is a multifaceted process that yields a detailed, prescriptive plan to drive sales for a company’s products across geographic regions and target markets.
The process usually starts with financial leaders, ideally in consultation with sales leaders, setting revenue targets that are both ambitious and realistic. Finance pushes those targets to the sales organization, often through the office of the chief revenue officer.
The CRO and his or her management team must then formulate a plan to achieve the desired results. That starts with identifying markets that are likely to prove fruitful with sales resources dedicated to them. The CRO also sets quotas for broad regions, localized teams, and individual sales reps, as well as commissions that incentivize everyone to execute and succeed.
Sales plans need to be precise, yet flexible enough to adjust to changing market conditions. Sales leaders must track multiple performance metrics on a granular level—including conversion rates, customer acquisition costs, and customer feedback—to know when to change up their plans.
Sales planning is important because it has an outsized impact on a product’s success in the market. An effective plan can increase revenue by positioning sales reps to close more, and larger, deals, by applying data-driven insights to assign territories, targets, and quotas to optimize sales results. This has the added benefit of motivating existing sales reps and boosting their productivity, while also attracting talented sellers with earnings potential, thus further fueling into the sales motion. A well-conceived sales plan also helps with customer relations in that it ensures that knowledgeable sellers are accessible to the largest number of potential and existing customers and that regional teams are staffed and equipped to service important accounts.
Sales planning can depend on, and affect, many separate company divisions. For that reason, the sales org should never be siloed. Because sales leaders have unique visibility and insights into sales volumes, they should work closely with financial leaders to establish revenue targets. Targets set too high can engender dissatisfaction and turnover on the sales team; targets set too low don’t take advantage of a team’s full potential.
The sales org also needs to coordinate with HR to structure commissions. Sales leaders might have a good sense of what profit margins on which products and in which regions will best motivate their teams, but they don’t directly control the payroll.
And it’s vital for the sales org to be aligned with marketing when it comes to generating customer interest. Marketing campaigns lay the groundwork for successful sales motions. Meanwhile, sales reps who interact directly with customers often develop unique insights as to where demand lies, where marketing resources can best foster product awareness, and which campaigns are proving effective. Sales and marketing are highly interdependent—success depends on these teams articulating common messaging.
Ultimately, sales planning should draw from the instincts of seasoned sales leaders, supported by rigorous data analysis, to advance the sales force’s mission of driving revenue. However, the following time-tested tactics and tools are common to most successful sales strategies.
- Set realistic targets and quotas : Sales planners should work hand in hand with financial leaders to make sure revenue targets are ambitious, but attainable. Unrealistic revenue targets get converted into unrealistic sales quotas that can demoralize talented sellers. The quotas that sales reps are expected to meet should be based on the specific products they’re selling and the markets they’re selling into. Everyone, from regional managers to team leaders to individual reps, should understand what’s expected of them and feel empowered to share input.
- Identify target markets and key accounts : In their plans, sales planners should strive to methodically identify and clearly document the geographic and demographic markets most likely to be receptive to specific products, as well as their key accounts within those markets. That requires a rigorous analysis of consumer dynamics, market trends, seasonal shifts, and regional and global events that could impact consumption, among other factors.
- Evaluate the competition : Understanding the approaches that successful competitors are taking to sell comparable products is extremely valuable when developing a sales plan, especially for companies launching new products. Planners should study competitors’ sales data and try to glean insights from the various strategies they employ.
- Carefully consider compensation : Compensation incentivizes success. The commissions kept by sales reps, and the margins that determine them, can motivate reps to work harder and pitch products more effectively. Healthy commissions also make it more likely that a company will recruit and retain top sales talent. But setting required margins too high can hurt sales, particularly in a highly competitive market, and ultimately eat away at profits. The margins set in compensation models should be thought through carefully and fine-tuned in line with metrics for specific products and regions.
- Coordinate across departments : The sales org should never operate independently of other business departments. Sales teams that don’t closely coordinate their planning with finance, HR, and marketing are bound to fall short of their potential.
- Embrace AI : Artificial intelligence gives planners a powerful new tool for forecasting sales and analyzing deals. Applications that leverage AI algorithms trained on contextual data can help sales organizations better allocate resources for the next quarter or year, reevaluate customer opportunities, align with broader company objectives, and highlight the most effective sales methodologies.
Even as sales activity is increasingly moving to online platforms, the following tried-and-true approaches to sales planning remain highly effective.
1. Align with finance
Sales execution plans should align with financial plans to best achieve the strategic goals of the business. That means sales leaders need real-time visibility into the CFO’s targets for margins and revenue—when they’re first set and as they’re updated—so they can rapidly adjust forecast models and reallocate resources accordingly.
2. Coordinate compensation with HR
The CRO has to motivate sellers with compensation plans. However, structuring sales margins that vary among products and regions, and that ultimately determine each sales agent’s commissions and total salary, are decisions that must be closely coordinated with the HR department.
3. Coordinate with marketing
Businesses are best served by a synergistic process in which marketers generate customer interest that redounds to sales reps closing deals. Conversely, sales reps interacting with customers in the field can share unique insights with marketers regarding where demand lies, where to invest marketing resources to drive product awareness, and which marketing campaigns are having the greatest impact.
4. Forecast proficiently
Trying to look into the future can seem like a fool’s errand, but sales leaders need to predict sales performance to effectively set quotas and allocate their resources. Naturally, the intuition of experienced sales professionals plays a key role here. However, contextual data, market trends, and customer behavior—analyzed with cutting-edge AI and big data tools—can significantly improve accuracy. With these resources, educated guesses can become intelligent forecasts, and gut feelings and intuition can be corrected, or corroborated, by advanced computational methods.
5. Equip with technology
Effective sales plans rely on visibility into key performance metrics, the careful analysis of large amounts of public and internal data, and close collaboration within sales teams and across business divisions. Modern cloud applications help sales planners accomplish all of the above. Sales planners need to have a clear line of communication with the IT department to make sure that the people who oversee software acquisition, deployment, and spending will provision them with the right tools to get the job done.
The process of creating a sales plan typically originates outside of the sales org.
The finance department sets revenue targets for a fiscal quarter or year, while executive management articulates broader company objectives such as the market penetration of a new product or expansion into new markets for an existing one.
Sales planners need to convert these financial targets and company objectives into clear sales goals. These goals and quotas don’t always prioritize maximum total sales. In fact, there are times when companies want to intentionally limit availability of their products at a given price, either to invoke the prestige of owning something scarce or to avoid overwhelming existing production capacity.
The next step is to define an actionable strategy to realize sales goals. That involves identifying specific markets, both by region and demographic, that will be fertile ground for the product being sold—if you’re selling surfboards, for example, you probably shouldn’t dedicate resources to landlocked states. Sales leaders should lean on the experience and insights of their teams when targeting markets, while also incorporating large amounts of market research data and analytics.
Sales planners must then take stock of the resources they have available to get the job done. They will be working within an allotted budget that limits how much they can spend on hiring sales managers and reps, generating leads, visiting customers, and provisioning sales tools. They’ll need to intelligently deploy those resources across geographic areas, product lines, and accounts based on potential returns. Sales orgs might also shuffle territory assignments, or hire new reps to fill gaps, as they shift focus to new products or implement new plans.
The sales plan should set quotas for regions, teams, and individual reps. It should also prescribe selling tactics, including cold-call scripts, tips for working with resellers, guidance on how to shepherd deals that originate on online platforms, and the rules for entertaining prospects. The sales plan should stipulate how reps receive training in these tactics and product functionality, how they’re allocated leads, and how they’ll access selling systems to help them collaborate and review their metrics.
To motivate success, sales leaders set margins for each product that will determine the commissions that reps receive when they close a deal. Insufficient margins might frustrate reps and fail to attract selling talent to the org, but overly high margins could turn off customers and eat into revenue and profits.
Finally, it’s important to remember that a sales plan isn’t static. Plans should always be adjusted to the reality in the field—or be abandoned altogether if they fail to yield results. To that end, sales leaders must ensure they’re collecting quality performance metrics. That means tracking leads, deals, revenue, and satisfied clients at the level of reps, teams, and regions.
The latest and greatest sales planning tools, if siloed, will fall short of delivering the benefits they promise. Point solutions and fragmented systems can’t adequately meet the complex needs of sales orgs in increasingly complex markets.
Oracle Sales Planning and Oracle Sales Performance Management , part of the Oracle Customer Experience suite of applications, connect seamlessly with finance and workforce planning modules included in the Oracle Enterprise Performance Management (EPM) suite. These sales planning tools provide real-time visibility into the performance of sales teams. With these tools, sales leaders can set, analyze, and adjust quotas for large teams and individual reps. They can also use the applications to sharpen forecasts, optimize territory assignments, generate leads, clear bottlenecks, identify effective tactics, and align with other business divisions. Oracle’s cloud-based sales planning solutions harness the power of data and AI to deliver a degree of insight that sales leaders could once only dream of.
How did sales activity change during the COVID-19 pandemic? With the pandemic’s lockdowns, social distancing measures, and travel restrictions, the shift to online sales accelerated. While some lament the declining interpersonal dimension, the torrent of data streaming in from an ever-increasing share of deals done online can be a boon to sales planners.
How does sales planning extend outside the sales org? Sales planners need to work closely with finance leaders to make sure the revenue goals set by the CFO translate into achievable sales quotas. They need to coordinate with HR to provide compensation packages that incentivize sales reps. And they need to work with the marketing department to ensure common, unified messaging that generates customer interest.
How are sales quotas set? Sales planners set quotas for regions, teams, and individual reps based on the revenue targets passed down from the finance department and executive management.
Which technologies are revolutionizing sales planning? The increasing complexity of the sales motion is spurring adoption of cloud-based planning solutions integrated with the larger estate of enterprise finance, HR, marketing, performance management, and other applications. Once the sales org has populated these integrated applications with quality data culled from sales automation platforms, they can then unleash AI to hone forecasts, improve execution plans, and deliver insights valuable to the field reps who are still schmoozing with clients over dinner.

See real-world examples of companies’ planning strategies around finance, marketing, workforce, and more.
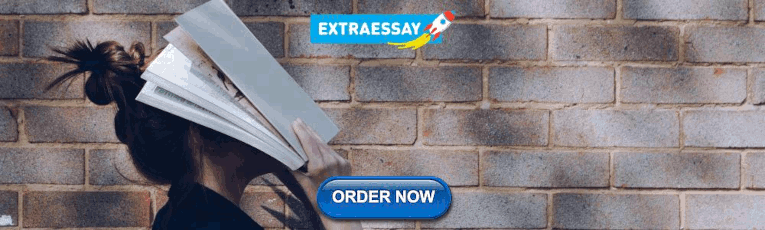
IMAGES
VIDEO
COMMENTS
6. Delphi Method. The Delphi method of forecasting involves consulting experts who analyze market conditions to predict a company's performance. A facilitator reaches out to those experts with questionnaires, requesting forecasts of business performance based on their experience and knowledge.
Forecasting should (1) start with identifying the problem. It should then (2) gather all the information necessary to solve the problem. After gathering data, (3) a business should run a ...
Forecasting is the use of historic data to determine the direction of future trends. Businesses utilize forecasting to determine how to allocate their budgets or plan for anticipated expenses for ...
Top Forecasting Methods. There are four main types of forecasting methods that financial analysts use to predict future revenues, expenses, and capital costs for a business. While there are a wide range of frequently used quantitative budget forecasting tools, in this article we focus on four main methods: (1) straight-line, (2) moving average ...
Reprint: R0707K The primary goal of forecasting is to identify the full range of possibilities facing a company, society, or the world at large. In this article, Saffo demythologizes the ...
2. Simple Linear Regression. The simple linear regression is a common financial forecasting method where a business explores the relationship between two variables — one independent and one dependent. For instance, a company could use this method to forecast revenue by gauging how it might be impacted by shifts in GDP.
Planning, budgeting and forecasting is typically a three-step process for determining and mapping out an organization's short- and long-term financial goals. Planning provides a framework for a business' financial objectives — typically for the next three to five years. Budgeting details how the plan will be carried out month to month and ...
The typical forecasting process follows a pattern that contributes to inaccurate projections and a defeating, self-reinforcing cycle. At one large industrial manufacturing and services company, for instance, managers in the business units and subunits are held to earnings targets that are rolled up into the overarching forecast.
General business forecasting predicts overall market trends and external factors that affect your business' success. Accounting forecasting creates projections of future business costs . Budget forecasting makes predictions for allocating the budget needed for future projects or addressing potential issues. Budgeting and forecasting software ...
Business forecasting aims to estimate customer demand for products or services, project sales or estimate growth and expansion. It can facilitate the allocation of budgets, capital, human resources and more. In short, business forecasting helps inform the decision-making process. Forecasting is often associated with big data analytics and ...
Business Forecasting Drives Better Decision Making. Business Forecasting is the process of using analytics, data, insights, and experience to make predictions and respond to various business needs. The insight gained by Business Forecasting enables companies to automate and optimize their business processes. A Forecaster's goal is to go ...
Financial forecasting is the process of estimating or predicting how a business will perform in the future. ... Financial Planning & Wealth Management (FPWM™) FinTech Industry Professional (FTIP™) ... finance, Excel, business valuation, budgeting/forecasting, PowerPoint presentations, accounting and business strategy. A well rounded ...
Effective business forecasting requires careful planning and execution. The following steps provide a comprehensive guide on how to develop a successful forecasting plan: Define the objective : The first step in the business forecasting process is to define the objective of the forecast by identifying the key performance indicators (KPIs) such ...
Financial forecasting is a crucial aspect of finance and business management, involving the process of estimating or predicting how a business will perform in the future. It helps organizations to strategize, plan, and budget effectively by utilizing historical data, expert insights, and various forecasting methods. Financial forecasting not only assists businesses with managing their ...
Clearly, business forecasting is a project unto itself. To manage a project and collect the data in a way that's useful in the future, you need a project management tool that can help you plan your process and select the data that helps you decide on a way forward. ProjectManager is award-winning software that organizes projects with features ...
Now that you understand the basics of business forecasting, it's time to see how it works in practice. Read the following examples to better understand the different approaches to business forecasting. 1. A company forecasting its sales through the end of the year. Let's suppose a small greeting card company wants to forecast its sales ...
Why It Matters. Companies conduct business forecasts to determine their goals, targets, and project plans for each new period, whether quarterly, annually, or even 2-5 year planning. Forecasting helps managers guide strategy and make informed decisions about critical business operations such as sales, expenses, revenue, and resource allocation.
The term Forecasting Methods in finance refers to techniques for analyzing business performance over time. Generally, Quantitative Financial Forecasting Methods are used over Qualitative methods. Forecasting is a tool used by businesses to plan for future spending or to decide how best to allocate their budgets.
These different business forecasting techniques give birth to the model of business forecasting where different techniques can be grouped as a model. There are mainly two types of models- Qualitative Models and Quantitative Models. ... It has emerged as the most important aspect of planning and operating any form of business. What are the types ...
This method is known as business forecasting. Business forecasting refers to the techniques, methods, ways, or tools that are used to predict development in a business. These developments may be related to sales, expenditure, profits, innovations, and upcoming trends. The main motive behind business forecasting is to develop better strategies ...
Models & Methods . Top Misconceptions in Forecasting. 13 Dec, 2017, 18:07 . Models & Methods ... The Institute of Business Forecasting and Planning is the membership organization for S&OP, forecasting, demand planning, business analytics, and supply chain professionals. Through our industry leading conferences, training and industry-recognized ...
All of the factors that go into creating a forecast reflect some extent what happened with the business in the past and what is considered likely to occur in the future. 3. Uses forecasting techniques. Most businesses use the quantitative method, particularly in planning and budgeting. The Process of Forecasting
Forecasting isn't a job you can do at the start of the financial year and forget about. It's an ongoing process that needs continuous refinement. Regularly review and refine your forecasting models based on stakeholder feedback, performance metrics, and learnings from past forecasting experiences. Consider building a scenario analysis into ...
Demand Forecasting: Businesses can use predictive analytics to forecast product demand, optimize inventory levels, and reduce the risk of overstocking or stockouts. Financial Planning and Risk Management : Predictive analytics aids in financial forecasting, identifying potential risks, and developing strategies to mitigate them.
Discover how to harness the power of analytics and business intelligence to enhance business planning and forecasting. Benefits of data-driven decision-making ... machine learning and forecasting techniques. Prescriptive analysis goes a step further by recommending actions based on data. This type combines predictive analytics with optimization ...
Many businesses use advanced probability theory in action and these days it has become an indispensable part of their operations. Examples can be found in the applications of forecasting, risk management, and decision analysis. However, we can improve the quality of our business decisions by embracing uncertainty and applying the science of probability in the decision-making process.
In my time, I've found that this process can be broken down into two key components: Building a repeatable and predictable demand generation engine and transitioning from founder-led sales to ...
Read on to know more about the difference between budget and forecast. Delve into the process of both Budgeting and Forecasting. ... July 29, 2024; For any business, budgeting and forecasting are crucial for financial planning and analysis. These two activities not only help make decisions and allocate resources, but also ensure an organisation ...
Meet Darts, a Python library that aims to be the scikit-learn for time series analysis.By providing a unified and consistent API, Darts simplifies the end-to-end process of working with time series data. It integrates various functionalities—data manipulation, model fitting, forecasting, and backtesting—into a single framework, making it easier for users to switch between models and ...
Sales planning is a process used to create yields a sales plan that clearly documents sales targets, goals, and strategies over a fixed period. But a sales plan is never carved in stone. Sales planning is an ongoing process where sales leaders continually monitor real-world results, assess evolving market conditions, and create new forecasts ...